- 1UMI 209 UMMISCO, IRD/UPMC/Sorbonne Universités, Paris, France
- 2ICT Lab, University of Science and Technology of Hanoi, Hanoi, Vietnam
- 3PDI-MSC, IRD/UPMC/Sorbonne Universités, Paris, France
- 4CTU/IRD JEAI DREAM, CICT, Can Tho University, Can Tho, Vietnam
- 5CTU/IRD JEAI DREAM, CENRES, Can Tho University, Can Tho, Vietnam
The Vietnamese Mekong Delta has undergone in recent years a considerable transformation in agricultural land-use, fueled by a boom of the exportation, an increase of population, a focus on intensive crops, but also environmental factors like sea level rise or the progression of soil salinity. These transformations have been, however, largely misestimated by the 10-year agricultural plans designed at the provincial levels, on the predictions of which, though, most of the large-scale investments (irrigation infrastructures, protection against flooding or salinity intrusion, and so on) are normally planned. This situation raises the question of how to explain the divergence between the predictions used as a basis for these plans and the actual situation. Answering it could, as a matter of fact, offer some insights on the dynamics at play and hopefully allow designing them more accurately. The dynamics of land-use change at a scale of a region results from the interactions between heterogeneous actors and factors at different scales, among them institutional policies, individual farming choices, land-cover and environmental changes, economic conditions, social dynamics, just to name a few. Understanding its evolution, for example, in this case, to better support agricultural planning, therefore requires the use of models that can represent the individual contributions of each actor or factor, and of course their interactions. We address this question through the design of an integrated hybrid model of land-use change in a specific and carefully chosen case study, which relies on the central hypothesis that the main force driving land-use change is actually the individual choices made by farmers at their local level. Farmers are the actors who decide (or not) to switch from one culture to another and the shifts observed at more global levels (village, district, province, region) are considered, in this model, as a consequence of the aggregation of these individual decisions. The central component of our hybrid model is then an agent-based model of farmers, provided with a sophisticated mechanism of decision-making that is influenced, at different degrees, by their perception of the contexts in which they act or interact with other actors. The economic context, accessible by them through the market prices of crops, plays a role, as well as the changes observed or forecasted in their physical context (land-cover changes, salinity rise) or the decisions made by others in their social context (neighbors, family members, opinion leaders). The model of farmers is coupled, through this decision-making mechanism, with other independent sub-models, each of them carrying out a realistic description of one of these contexts. Since the dynamics depicted in these sub-models obey to different logics, operate at different scales and rely on different data, they are represented using appropriate modeling techniques: the spatial model is based on GIS information on parcels, soils, and rivers; a cellular automaton is used to account for the evolution of land-cover changes and the diffusion of salinity; an aggregated mathematical model represents the fluctuation of prices on the regional and national markets; and a graph-based social network model is used to represent familial networks of influence. Beyond the descriptions of these models, the paper is organized around a discussion about the two main outcomes of this research work. The first one is applicative: the way we have calibrated, coupled together, and experimented in different scenarios these five models is presented and we show that some findings obtained with the resulting hybrid model could not have been obtained with more traditional techniques. The second one is methodological: the underlying co-modeling architecture we used for declaring and running this assembly of heterogeneous models, implemented in the GAMA modeling and simulation platform, is presented and we show how it can be generalized to arbitrary hybridizations of models.
1. Introduction
1.1. Context: Land-Use Change in the Vietnamese Mekong Delta
The region of the Vietnamese Mekong Delta (VMD), composed of 13 provinces including a municipality and was home of approximately 18 million of inhabitants, was by far the most productive region of Vietnam in agriculture and aquaculture in 2014. In terms of rice production, for instance, 47% of the cultivated areas in Vietnam were situated in the VMD, and they outputted 54% of the total production; in terms of aquaculture, 2/3 of the Vietnamese production originated from the VMD. According to Young et al. (2002), these performances, which have roughly tripled in the last 30 years in all sectors, have fueled the boom of the Vietnamese exports of agricultural products (especially rice, shrimps, and fruits). This spectacular rise is due to a number of factors: a better economic environment (thanks to reforms more favorable to the private sector), the adoption of modern techniques (fertilizers, mechanical harvesting, progresses in aquaculture), yield improvements, improved irrigation and drainage, among others.
This growth has logically been accompanied by a deep transformation of the agricultural land-use. However, other factors, like the sea level rise, the general urbanization of the country or the progression of soil salinity (Smajgl et al., 2015), have also played a role in this transformation, and it is not trivial to sort out its different causes. In a country like Vietnam, this difficulty raises some concerns because agricultural land-use is traditionally strictly planned under the control, and following the national circulars, of the Ministry of Natural Resources and Environment (MONRE, 2009; VNA, 2013). Plans are produced every 10 years and readjusted at mid-term using a land-use inventory in order to rectify divergences with the reality. This actually results in two five-years long plans, detailed down to the level of provinces, that both recommend a given distribution in terms of land-use and cultivation types, but also schedule national and provincial investments (irrigation infrastructures, protection against flooding or salinity intrusion, transportation infrastructures, and so on) based on this distribution. In an ideal situation, where every province would follow the plan, there would not be any difference between the recommended distribution of land-use and its forecast. However, during the period covered by the latest plan (from 2000 to 2010), the planned—and then expected—distribution has been systematically offset, sometimes by an important margin, from the reality of land-use as measured by remote-sensing techniques. In Figure 1, for instance, it is easy to see that land-use has had a trend to shift from rice to shrimps. The surface dedicated to rice crops has strongly decreased, while the one dedicated to shrimp aquaculture has increased.
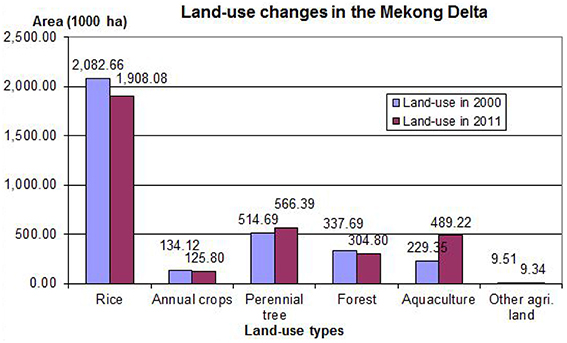
Figure 1. Land-use area in the Mekong Delta in 2000 and in 2011. Source: Vietnamese General Statistics Office (VGSO, 2000) and Ministry of Natural Resources and Environment (MONRE, 2012) of Vietnam.
At the levels of provinces, the same shift can be observed. For instance, in the province of Ben Tre (see Figure 2), a total cultivated area of 175,824 ha was planned for 2010, but it eventually reached 179,671 ha. This average value (which gathers all kinds of agricultural activities) might be seen as a not so significant change at the macro-level, but profound divergences can be unveiled when studying the numbers in more details and especially the spatial inscription of the changes. For example, the rice area expanded to 38,000 ha, compared to a planned 30,000 ha (+ 27%); the surface devoted to aquaculture, which was supposed to reach 39,200 ha, only reached 30,289 ha (−23%); and the forests, expected to cover 350 ha (PCBT, 2011), remained at a low 1.30 ha. At the scale of villages, the divergences are sometimes even more spectacular, as entire villages have shifted to one type of production to another without any incentives from the plans—and without, of course, following their recommendations. To understand the importance of these changes, we consider in this paper a specific case study, which will be the focus of the remaining sections. This case study comprises five villages situated in the middle of the Thanh Phu district (Ben Tre province). They have been carefully chosen as they exhibit a variety of land cover characteristics while remaining geographically close to each other, at least close enough to reasonably allow us to consider that the farmers living in these villages share common “cultural traits” and traditions. As shown on Figure 3), four of these villages are situated in areas invaded by brackish water, while the remaining one benefits from fresh water due to its inclusion in a dike-protected area; the three villages to the west are bordering the river, the easternmost is located near a mangrove and the remaining one has no direct access to the river.
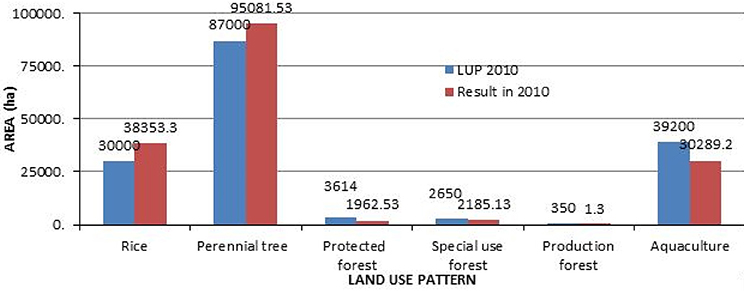
Figure 2. Land-use planning assessment of Ben Tre province in 2010. Source: Department of Environmental and Natural resources of Ben Tre province, Vietnam (PCBT, 2011).
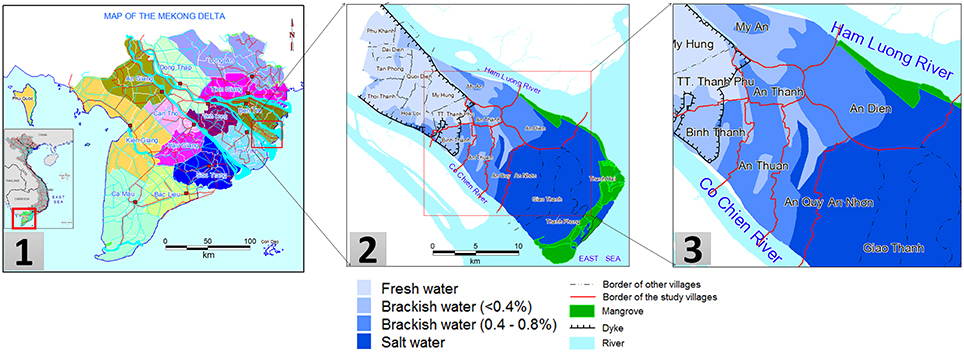
Figure 3. Maps of the five villages of Thanh Phu district in the Mekong Delta. Source: Combined from the source of Land resource department, Can Tho University, Vietnam (Vo and Le, 2006) and the Department of Environmental and Natural resources of Ben Tre province, Vietnam (PCBT, 2011).
On Figure 4, we show the results of a study we conducted on these five villages in order to assess the shift of land-use between, on one hand, the two projections for year 2010 of the plans produced in 2000 and 2005 and, on the other hand, the actual land-use map in 2010 (PCBT, 2011). Changes are measured using a Fuzzy Kappa indicator, a variant of Kappa (Cohen, 1960) that provides a measure close to how humans compare maps. The darkness of the areas on the two right-hand maps is proportional to the change in land-use. It is easy to see that, while the average changes for the whole province may not be spectacular, they translate into local changes that mark complete shifts from one type of production to another. With respect to this, the plan published in 2000 is completely wrong in its projections (almost all parcels have changed) and the rectified plan published in 2005, while correct for the most part, completely misses the shifts in two villages and along the canals.
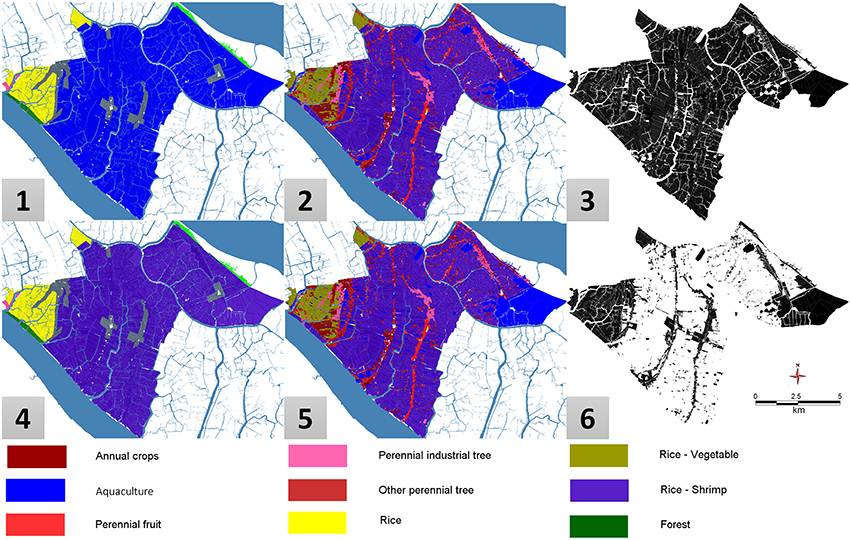
Figure 4. Comparison of land-use planning map and land-use map in 2010 of 5 villages of Thanh Phu district, Ben Tre province. (1) Land-use planning map 2010 (planned in 2001), (4) Land-use planning map 2010 (modified in 2005), (2), (5) Land-use map in 2010, (3) Fuzzy Kappa map between 1 and 2, (6) Fuzzy Kappa map between 4 and 5.
This finding would not be a concern if these projections were not also used to plan long-term investments. When a plan projects an extension of rice areas in a particular place, this usually is accompanied by a planning of irrigation infrastructures, dikes, or other facilities supposed to foster the cultivation of rice. But what happens, for instance, when the farmers living in this particular place take the decision to massively switch from rice cultivation to shrimp aquaculture? Would the investments be canceled, or, even worse, maintained and then play a counter-productive role? Land-use planning, as it goes in Vietnam, and despite its inherent interest, is not precisely the most appropriate way to react to such changes in a timely manner. This implies two possible views on how to better connect investments with the reality of land-use. The first option is to completely abandon the use of a global plan as it is designed now, and opt for a more decentralized and reactive land-use planning strategy, which would allow districts or even villages to become responsible for their own local land-use allocation strategy (or the best part of it). Considering and discussing the potential strengths (better and more timely adaptation) and drawbacks (possible lack of coordination between adjacent areas) of this option clearly goes beyond the scope of this article and would be, in any case, ultimately, a political decision. A second option would be, therefore, to investigate how the predictions/recommendations of the plans could be made more accurate, so as to support planners in designing more realistic plans. This is the option we take in this article.
One major difficulty in this option is that we do not have access to the methodology used by planners to produce their plans. Their predictions, moreover, possess an ambiguous status, as they serve both as forecasts (what they expect the land-use to become in 10 years) and recommendations (what they want the land-use to become in 10 years). As such, they are probably designed using a mixture of science and politics: models based on past performances certainly play a role, together with data collection and aggregation (from villages to provinces), but it is difficult to sort out what belongs to political or economic wishes and what is the fruit of a scientific approach to build accurate projections. In that respect, it is appropriate to start by the beginning and try to understand—or at least draw serious hypotheses about—the causes behind the current trends in land-use change in the VMD.
As pointed by Lambin and Geist (2007) and JunJie (2008), the dynamics of land-use change at a regional scale results from the interactions of various actors and factors at different scales, among them institutional policies, individual farming choices, land-cover and environmental changes, economic conditions, social dynamics, just to name a few. Understanding its evolution, for example, in our case, to better support future planning efforts, requires to use models that can represent the individual contributions of each of these actors, and of course a description of how these models interact, based on a more general theory of the interplay of the different causes.
1.2. Proposal: Design of an Integrated Hybrid Model
We address this question through the design of an integrated model of land-use change in the case study presented above (Figure 3), which relies on the central hypothesis that the main force driving land-use change is the individual choices made by farmers at their local level. Farmers are the actors who decide (or not), given their knowledge, preferences or interactions, to switch from one culture to another. In the model, the shifts observed at more global levels (village, district, province, and region) are then a consequence of the aggregation—and interactions–of individual models of farmers' decisions.
Given the multiplicity of factors that can be represented in such a model, we need to rely on a very flexible modeling architecture in order to easily combine models and test their different combinations. We need also to avoid reinventing the wheel: the reuse (and comparison) of legacy models of specific factors (economic models, for instance), when they are available, is clearly a necessity, which in turn implies that we use a framework capable of supporting and coupling models expressed using multiple modeling paradigms. These two reasons have led us, among different choices, to rely on the multi-agent based “co-modeling” architecture of Huynh et al. (2014) and to implement it in the open-source GAMA platform (GAMA, 2007), which possesses the advantage of providing, out of the box, a first-class support for spatial data and spatial analysis, as well as a componential architecture that supports the concurrent use of multiple formalisms within a model (Grignard et al., 2013). The methodology we followed is discussed in this paper and represents one of the two outcomes of this research, which we hope will be seen as generic enough to be reusable by modelers in other contexts.
The second outcome is the integrated model itself. As stated above, the central component of this model is a model of farmers, provided with a sophisticated mechanism of decision-making that can be influenced, at different degrees, by their perception of the contexts in which they act or interact with other actors. Three of these “contexts” are described in the paper: the economic context, represented by a model of market prices of the agricultural products; the environmental context, represented by several models of the changes observed or planned in the farmers' physical environment (e.g., natural or man-made land-cover changes, rise of salinity, and so on); and finally the social context in which the farmers interact with other human actors (e.g., neighbors, family members, opinion leaders, policy makers), represented by a model of the dynamics of their social network.
The sub-model of farmers uses an agent-based BDI formalism (Rao and Georgeff, 1991) to describe their decision-making process. This sub-model is then coupled with other sub-models, each of them built using a suitable formalism for carrying out a realistic description of the context they represent. As a matter of fact, the dynamics present in these sub-models obey to different logical processes, operate at different scales and rely on different datasets, so they need to be designed with specific modeling techniques. In its most stable form, the one discussed in this paper, the integrated model makes use of a GIS-based model of parcels, soils, and rivers; a cellular automaton is then used to account for the evolution of land-cover changes and the diffusion of salinity; an aggregated mathematical model represents the fluctuation of prices on the regional and national markets; and, finally, a graph-based social network is used to represent familial networks of influence.
The validation of the resulting model makes full use of the “co-modeling” architecture to produce meaningful outcomes. On the methodological side, we show how to conduct experiments that use different combinations of these sub-models, exchange them with alternative models, or explicitly modify their relationships and interactions, thereby providing modelers with an extraordinary flexibility for conducting controlled experiments and testing various hypotheses. On the applicative side, we show how certain combinations of causes (represented by corresponding combinations of sub-models) can provide new insights on the land-use processes at play in the VMD and discuss how these insights could be reused to improve land-use planning in the future.
2. Integrated Models of Land-Use Changes
2.1. State of the Art and Applications of Land-Use Change Models
Land-use and land-cover changes (LUCC) models have a long and rich history in spatial modeling (Parker et al., 2002). They can be broadly classified into two, not necessarily exclusive, categories: in the first one, we find models we might describe as “descriptive,” whose primary concern is not to represent realistic mechanisms but to faithfully reproduce high-level dynamics of land-use change (Lambin, 1997); these models are essentially used for monitoring or prediction. They habitually rely on a discretization of the space, identifying “parcels” or “patches,” and aggregating the influence of several drivers into high-level rules (equations in mathematical models Serneels and Lambin, 2001, transition rules in Cellular Automata models Zhao and Peng, 2012; Subedi et al., 2013), transition functions or matrices in Markov Chain models (Kemeny and Snell, 1983) to describe how these patches change state over time. The second category groups “explicative” models, seeking to generate realistic dynamics of land-use change based on a detailed and faithful representation of the possible drivers of the changes; these models are more explicitly targeting decision-support, providing for example a support to “what-if” experiments (Trickett and Trafton, 2007). In the last 10 years, thanks to the generalization of developments in agent-based modeling (ABM, Michel et al., 2009), the design of large-scale, data-driven, individual-based models has even made possible the modeling of individual land-use change decisions and the simulation of their collective effects at higher geographical scales (Parker et al., 2003, Valbuena et al., 2010, Mena et al., 2011, Bakker et al., 2015), albeit with simple behavioral models. It is only recently that more sophisticated representations of the stakeholders' behaviors have emerged, again based on ABM, for example with the use of the the cognitive formalism known as BDI (Belief Desire Intention; Taillandier and Therond, 2011, Truong et al., 2016), which allows to model human actors as agents with beliefs concerning the world (i.e., the context outside the agent but accessible by it through its perceptual mechanisms), desires for their long term activities (i.e., goals), and intentions (i.e., the short-term actions that need be applied to fulfill their desires).
These two categories of LUCC models have long remained somehow separated, firstly because they had different objectives and, secondly, because they relied on different modeling paradigms. But their objectives are, actually, quite convergent: explaining large-scale changes in land-use without taking into account human activities, and especially their variability over time, casts doubt on the ability of the first category of models to produce realistic predictive models; conversely, the “environment” of the agents cannot be considered solely as a product of their activity. Especially in countries (like Vietnam) that are threatened by climate change, land-cover changes as well as other stressors (economy, innovations) need to be taken into account—and the first category of models can become essential in that respect, in conjunction, of course, with models of the second category. These reasons, and others, have led to the emergence of a new type of models, known in the literature as “hybrid models” (Parrott, 2011), which basically combine different sub-models into one to produce richer insights, at the price, however, of an increased complexity: a complexity in the design of these combinations of models; and a complexity in their exploration. LUDAS (Le et al., 2008), built in NetLogo, or Aporia (Murray-Rust et al., 2014), built on top of the Repast Semaphore platform (North et al., 2006), are two good examples of this trend, and underline both the potentialities of this new approach to modeling, but also its drawbacks, which are summarized in the three following points:
• Lack of “necessary complexity”: despite their goal, most of the hybrid LUCC models (Zhao and Peng, 2012, Subedi et al., 2013) remain quite simple in that they do not treat the different dynamics equally. When the environmental factors are represented with great detail, the behavior of stakeholders remains simple (e.g., Lambin and Geist, 2007). And when this behavior is modeled using advanced mechanisms, like the BDI architecture (Taillandier and Therond, 2011, Truong et al., 2016), it is the environment that lacks a proper representation. Of course, everyone prefers to deal with simple models, more tractable from a simulation point of view, but it is their own complexity that, sometimes, provide LUCC models their heuristic power in terms of decision-support (Edmonds and Moss, 2005).
• Lack of genericity: until now, despite the similarity between the objects, processes or actors that can be found across different LUCC case studies, when a model is developed for one case study, it usually remains specific to it. In particular, no real effort is made to generalize and share methodological outcomes (architectures, sub-models, patterns), because they rely on assumptions that cannot be easily translated to other contexts: Aporia (Murray-Rust et al., 2014), for instance, is dedicated to European farmers and their environment, while LUDAS (Le et al., 2008) remains restricted to highlands and mountainous areas in Vietnam.
• Lack of flexibility: finally, with the notable exception of Aporia (which partially supports the change of sub-models), most of the existing hybrid LUCC models are designed as a static composition of carefully chosen (or written) sub-models. This does not allow to consider sub-models as possible parameters of experiments, something that can be necessary to explore different configurations or scenarios. In our case, given the variety of factors identified, explaining LUCC in the Mekong Delta with an integrated model requires that we explore several causes, some of them represented not only by parameters, but by entire sub-models or specific combinations of them. The underlying software architecture need then to provide a high degree of modularity and flexibility, in order to easily add, remove or change sub-models, but also to change their way of interacting, exchanging information and contributing to the overall outcome.
2.2. Models Coupling in Socio-Environmental Modeling
A handful of research works have already addressed the problem of coupling multidisciplinary models in the general domain of socio-environmental modeling. We can classify these proposals in two extreme groups: on one hand, the ones that propose a strong coupling between a given set of well identified sub-models, supporting complete interactions between them; on the other hand, a weak coupling which mainly relies on an interface supporting data exchanges between multiple models described by their input and output parameters. Both groups have their advantages and drawbacks: while the former can produce really integrated solutions, it lacks flexibility in that sub-models cannot easily be replaced; and the latter, more flexible in theory, imposes an interface which also limits the types of sub-models that can be used.
An interesting example of weak coupling is the work of Nicolai et al. (2011), who have coupled UrbanSim with MATSim in order to produce an integrated model of urban mobility. UrbanSim contains information on residential locations, workplaces and urban development, while MATSim provides access to large-scale models of land-use, transportation network and economic dynamics. The two models are synchronized through the exchange of specific data (mobility needs from UrbanSim to MATSim, accessibility indicators in the reverse way). The literature provides other examples, like Yez et al. (2008), with an integrated model of a marine environment composed of local ecosystems of pelagic species, which adds an explicit translation between models at different scales, or Steiner et al. (2009), which couples a community land model with the regional climate model of the West African monsoon, and of course others. Simple exchanges of data is in general not sufficient in weak coupling approaches because the sub-models, which can be legacy models that cannot be modified, can operate at different scales of space and time and can also have different objectives (Rajeevan and Nanjudiah, 2009). There is a need, then, to provide a form of translation (Moreira et al., 2009 calls it a “coupler”) that takes into account the peculiarities of each model, often linked to the formalism in which it is described (e.g., agent-based modeling, discrete event, continuous equations). Numerous works have therefore addressed the problem of combining or coupling models described using different paradigms, like for example Rochette et al. (2012) on the coupling of hydrodynamic continuous models and individual-based models, Quesnel (2005) on the coupling of physical and social models, Rousseaux et al. (2012) on the coupling of continuous and discrete formalisms in ecological modeling or Nguyen et al. (2008) on the coupling between agent-based models and equation-based models through the use of intermediate graph-based representations.
But even complex exchanges of data or parameters between models cannot solve the problems raised by the necessity, sometimes, to control how the different sub-models are executed or simulated within an integrated model. In that case, a stronger coupling, which involves some kind of functional control in addition to the exchange of data, must be used (Huang et al., 2008). Strong coupling requires relying on some kind of operational architecture or framework, which can provide a way to express the control over sub-models. This framework can either use an existing modeling paradigm (like Li et al., 2013, which proposes an “agent-centered” approach in which different modeling formalisms can be translated to individual or agent-based models) or make use of specialized software architectures dedicated to the functional coupling of models, like the High-Level Architecture (HLA; Dahmann and Morse, 1998; Dahmann et al., 1998; Hill, 2002; Kim, 2005; SISO, 2010), the Discrete Event Systems (DEVS Zeigler et al., 1997; Hild, 2000; Vangheluwe, 2000), of the Functional Mock-up Interface (Blochwitz et al., 2012; Bertsch et al., 2014). HLA is a standard and a software framework that has been originally designed as an infrastructure dedicated to the synchronization of simulators whenever they exchange data. The principle of HLA is to consider each simulator as a federate in a Federation, itself defined by three core elements: an object model which describes the simulators and the federation in terms of input and outputs, an interface specification within the RTI (Runtime Infrastructure), and a set of control rules (which more or less represent the behavior of the integrated model resulting from the coupling of the simulators). Despite its numerous advantages in the handling of completely heterogeneous simulators, the main problem of HLA remains its complexity for non-computer scientists, which makes it out of reach of most modelers. DEVS is initially a formalism proposed to model discrete event systems. Its interest for models coupling lies in its recursive definition: a model described in DEVS (with its set of inputs, outputs, states, etc.) can be considered as either “atomic” or “coupled,” in which case it is described with additional features like the models it couples (which can themselves be atomic or coupled), a translation function and the influences between these models. DEVS is particularly elegant in its concepts, and very well adapted for building composite models, but relies on an internal description of models that is not easy to provide for stochastic models or complex legacy models. Finally, FMI is an industrial standard for co-simulation, where each sub-model or simulator is wrapped and exposed to the others using a functional interface which specifies how it can be accessed or manipulated.
Despite being operational, HLA, DEVS, and FMI are almost completely absent from the literature on hybrid modeling (Parrott, 2011) for decision-making in environmental or ecological issues. The main reason is that these solutions address the software engineering side of the problem of coupling and require modelers to learn and master quite complex techniques. In the worst cases, sub-models even need to be completely rewritten or redesigned to adapt to the interface required by the coupling infrastructure. Furthermore, these techniques use formalisms and languages that are different from the ones commonly used to build socio-environmental models (like agent-based models, cellular automata, or mathematical models), which is actually a source of confusion for many researchers. That is the reason why most of the existing integrated models make use of ad-hoc techniques, designed for the purpose of providing an infrastructure for one specific set of sub-models and unfortunately almost impossible to reuse in other contexts. For example, in Nicolai et al. (2011), the connection between UrbanSim et MATSim is completely bound to this particular instance of integration and there is no way one can reuse it, adapt it or extend it, for instance with an environmental model.
We propose in this paper another way of seeing the problem of coupling, detailed in Section 4.1. It is conceptually close to the notion of “multi-model ecologies” promoted by Bollinger et al. (2015) and comes with a complete agent-based implementation in the GAMA platform. Beyond the particular integrated model we present in the next section, this proposal aims at being as generic as possible and constitutes both the basis and one of the outcomes of our research.
3. Integrated Land-Use Change Model
Our proposal is organized to fulfill the requirements expressed in the Introduction and address the limitations of the existing land-use change models exposed in Section 2.1. Following Lambin and Geist (2007) and JunJie (2008), for whom the farmers' land-use change decisions are influenced by a complex combination of social, economic and environmental factors, the integrated model we have designed relies on four main hypotheses (and some associated assumptions) that individually shape the sub-models used to represent these factors and their interactions.
3.1. Hypotheses Regarding the Dynamics of Land-Use Change
When analyzing the map on Figure 4, one can easily see that the changes in land-use are localized in specific sub-regions (exactly, specific villages) of the district. However, the environmental contexts of these villages are completely different: for instance, one of these villages is located in an area under the protection of dikes (to prevent salt intrusion), while the others are not. The same village borders the main branch of the river, while the others either border canals and roads, or do not seem to follow any specific pattern. In all these cases, it is clear that the farmers do not put the same exact rules into practice. What does it mean in terms of behavior? A first hypothesis would be that farmers from different villages have completely different decision-making behaviors. A second, more realistic, is that farmers, whilst equipped with the same decision-making behaviors, and influenced by the same core factors, including their income, for instance, dynamically adapt the priority of these behaviors with respect to their environmental—or social—contexts, thereby taking into account a rather large number of criteria. This observation is in line with those of Lambin and Geist (2007) and Beratan (2007). This brings us to formulate our first hypothesis:
- Hypothesis H1: Farmers use a complex decision-making process based on multiple criteria, which are adjusted based on their perceptions or individual beliefs. These processes, performed independently, drive the global land-use change process.
More precisely, Ahnstrm et al. (2009) points out that economic factors, such as the price of products, costs of production and benefits expected are the most important factors influencing the decision of farmers. Farmers usually tend to produce products that are supposed to provide them with the highest income in the future. Figure 5 shows the evolution of average prices of the four main products (rice, vegetables, shrimp, coconut) in Ben Tre and An Giang provinces from 2005 to 2010. These products correspond to different land-use types (Rice, Rice-Vegetables, Rice-Shrimp, Shrimp, Annual crops, Industrial perennial and Fruit perennial). This leads us to establish our second hypothesis:
- Hypothesis H2: Among the various factors driving land-use changes, the market price of products and the income they expect from them play a pivotal role in the decision of farmers.
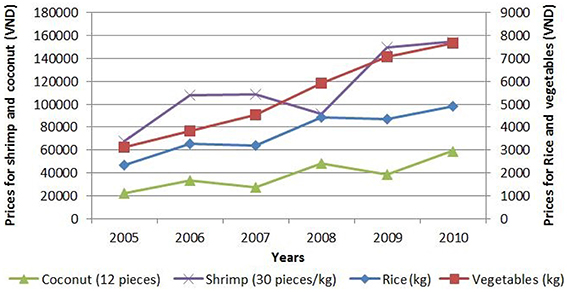
Figure 5. Market prices of the most popular products in the Mekong Delta from 2005 to 2010. Source: Combined from various sources (BTCA, 2015; DARD, 2015; VASEP, 2015; VFA, 2015) (1USD ≈ 21,840VND).
Farmers, when deciding which type of production they will choose, need of course to take the suitability of their parcel i.e., the type and quality of the soil and the quality of water available, into account. Smajgl et al. (2015) points out that environmental factors, like saltwater intrusion, implicit effects of infrastructure planning, etc., have a negative impact on this suitability, especially in coastal regions, and will then even indirectly lead to changes in land-use. This provides us with a third hypothesis:
- Hypothesis H3: Environmental characteristics, such as saltwater intrusion, soil type, etc., change the suitability of parcels with respect to some agricultural productions and directly or indirectly influence the strategy of farmers.
Finally, the decision of farmers are influenced by the decision of their neighbors (Case, 1992) or their family. In Vietnam, for example, when farmers succeed with a new land-use type, they usually gain a lot of attention from their neighbors or through their social relationships; moreover, a number of associations of farmers exist in every village, where they are encouraged to exchange their experiences and techniques of cultivation with different networks. This brings us to formulate a fourth hypothesis:
- Hypothesis H4: Social interactions, between neighbors, within families or more extended social networks, exert an influence on the decisions of farmers, either because of the exchanges of information or because of the display of successful experiments.
3.2. Sub-Models Organization
The four hypotheses presented above rely on quite heterogeneous data and studies. Actually, testing them all at once in one single model would certainly result in a complex construction difficult to design, maintain, adapt and experiment. But we can exploit their independence and consider each of them to be the subject of a much simpler (sub-)model, in order to test them individually, but also to test their respective influences in various patterns of composition. To this aim, we propose in Section 4 a novel approach to models coupling that provides modelers with more flexibility than existing approaches (it allows them to freely and dynamically add, remove, or substitute models).
Concretely, the four hypotheses are translated into five independent sub-models, each of them representing the factors or part of the factors present in each hypothesis, so that they could be tested in isolation or in interaction with each other. Figure 6 summarizes this decomposition. We provide more details on each sub-model in the following sections.
3.3. M_S: Sub-Model of Soil Salinity
3.3.1. Presentation
As discussed in Section 3.1, environmental factors influence the decisions of farmers and the salinity of the soil is one of the most important factors farmers take into account when they need to assess the suitability of parcels for different types of cultures. We then designed a first sub-model exclusively dedicated to the reproduction of the dynamics of soil salinity from 2005 to 2010. As shown in Figure 7, the input of this first sub-model are the salinity maps of 2005 Vo and Le (2006) and 2010, available thanks to an efficient regional network, the GIS data on dikes and dike-protected areas for the year 2010 (PCBT, 2011), the GIS data on parcels and their land-use and the GIS data on rivers and canals.
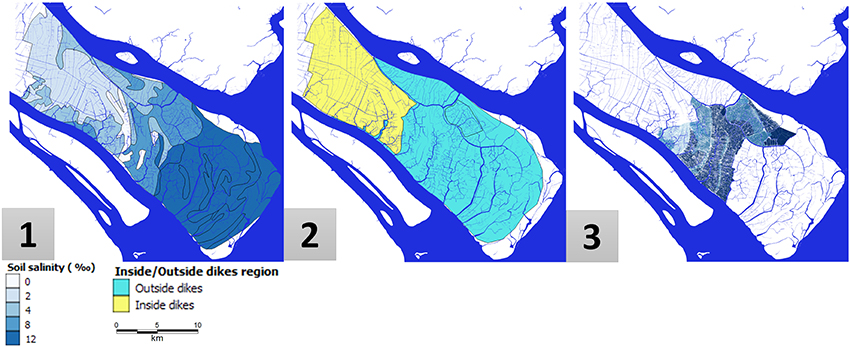
Figure 7. Data for the soil salinity model: (1) Soil salinity map in 2005, (2) Regions protected by dikes in 2010, (3) Soil salinity map in 2010.
3.3.2. Model Design
This sub-model relies on a discretization of the environment in 18,400 parcels, obtained from the land-use map, where each parcel is linked with its immediate neighbors in a radius of 100 m and is provided with a set of attributes, among them its salinity [classified into 4 levels (less than 0.4%; 0.4–0.8%; 0.8–1.2%; greater than 1.2%)] whether or not it is in dike-protected area, and whether or not it is bordering a river (obtained by overlapping the rivers and canals maps). Initial salinity levels in Thanh Phu district, in 2005, are computed after (Vo and Le, 2006).
The dynamics of the model is voluntarily kept simple and deterministic: at each iteration (1 year) it reevaluates, like in a cellular automaton, the level of salinity of each parcel. Parcels considered as protected by dikes do not change. Parcels bordering rivers see their salinity automatically rise up to 1.2%. And salinity is diffused in the remaining parcels using the following function:
One major limitation of this model is that we did not consider the various flooding episodes that occurred during these 5 years, principally to keep it as generic as possible, and also because of the lack of accurate data on these episodes. Taking them into account would probably require the use of a stochastic component, which could in any case be added later if necessary. In its deterministic form, the model has nevertheless been successfully validated by comparing the map obtained by simulation and the actual map of salinity for the year 2010 using the fuzzy-kappa indicator (see Section 5.1.1), with a score of 62.24%, and the absolute deviation percentage (24.28%). Both have been considered as satisfying with respect to its limitations.
3.4. M_P: Sub-Model of Parcels
3.4.1. Presentation
The environment in which land-use changes are simulated is represented by a set of parcel agents, initialized after a land-use map at the level of villages (Figure 8). By combining this map with a soil map and a flooding map, each parcel agent is provided with a given land-use and other attributes such as its soil type, its level of salinity, and the extent and depth of flooding episodes on it. The two main purposes of this sub-model are, on one hand, to provide other sub-models with a unified way of accessing and modifying these attributes and, on the other hand, to compute a synthetic indicator called “land suitability.” Land suitability represents the compatibility of a given parcel with the different land-use types. It can take 4 values (S1: Highly suitable; S2: Moderately suitable; S3: Marginally suitable; N: Not suitable) (Soil Resources, Management and Conservation Service, 1981). Based on the type of soil and the level of salinity, we defined (with the help of domain experts) a suitability matrix for each of the 8 land-use types considered in the model (e.g., Rice, Rice-Vegetables, Rice-Shrimp, Shrimp, Annual crops, Industrial perennial, Fruit perennial and Other perennial).
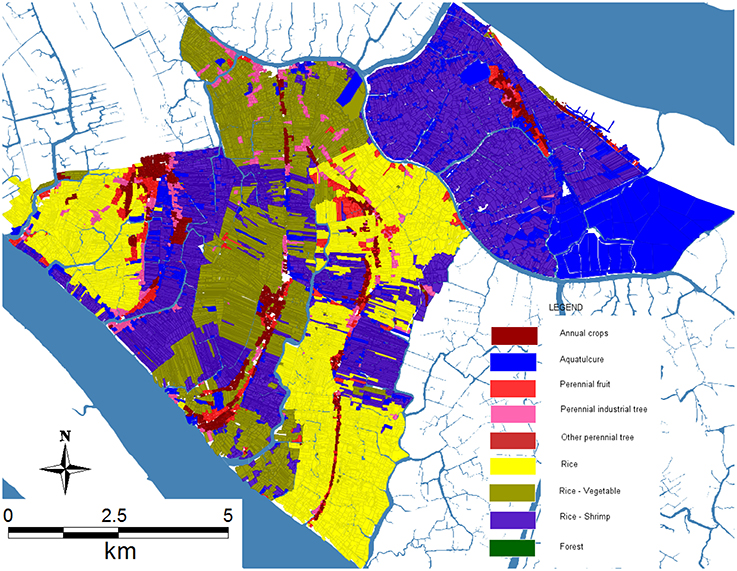
Figure 8. Land-use map of five villages (An Thanh, Binh Thanh, An Thuan, An Quy, An Nhon, An Dien) of Thanh Phu district in 2005.
3.4.2. Model Design
In the current instantiation of the integrated model, this sub-model is not provided with any internal dynamics. Instead, it is supposed that some attributes can be manipulated by external models (e.g., M_S for the level of salinity) and that the type of the soil remains unchanged. Each year, each of the parcels then simply computes and updates its land suitability matrix.
3.5. M_E: Sub-Model of Economic Factors
3.5.1. Presentation
In H2 (see Section 3.1), we make the hypothesis that economic factors like regional market prices and costs of production strongly influence the decision of farmers regarding the land-use to adopt. To verify this, we then designed a simple economic model to represent the evolution of these two factors. The data concerning market prices has been collected from 2005 to 2010 (averaged every year) from regional sources. However, the costs of production within the corresponding period could not be obtained so easily; we then used the costs in 2010 (evaluated in Nguyen et al., 2014) and extrapolated them from 2005 to 2010 using the regression equations depicted in Figure 9 (Judge et al., 1988). Two main assumptions are made in this sub-model: (1) the investment cost and selling price of each agricultural product are the same for all farmers; (2) external incentives (such as the opening of new markets) and perturbations (such as economic crises) are not taken into account.
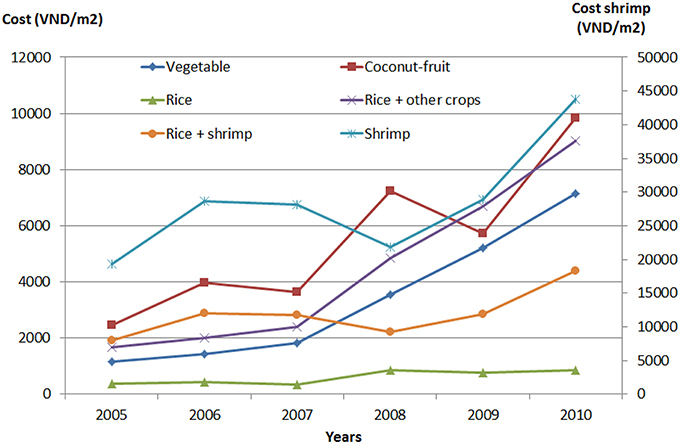
Figure 9. Production costs of the most popular products in the Mekong Delta from 2005 to 2010. Source: Computed from the prices from 2005 to 2010 and the production costs in 2010 (1USD ≈ 21,840VND).
3.5.2. Model Design
The main components of the model are the 5 regression equations below, where “x” represents the time in year from 1 to 5 (i.e., from 2005 to 2010) and the parameters have been computed after the values for 2010. The costs are expressed in the Vietnamese currency, Dongs, per square meter (VND/m2). Such a model allows to easily compute the expected benefit of a given production, by subtracting its cost from its selling price, multiplied by the surface of the parcel on which it is cultivated.
3.6. M_F: Sub-Model of Farmers Behavior
3.6.1. Presentation
In order to validate the hypothesis that farmers use complex decision-making processes based on a multitude of factors (discussed in Section 3.1), we designed an agent-based model that represents farmers (or households, as we do not distinguish individuals from their immediate familial environment) as agents provided with behaviors expressed in the BDI formalism (Caillou et al., 2015). In Truong et al. (2016), the authors have compared three formalisms (i.e., BDI, multi-criteria and probabilistic) for representing complex individual decision-making processes in agent-based models and arrived to the conclusion that BDI is the most relevant when dealing with heterogeneous factors such as the ones we consider in our integrated model. Basically, BDI is a cognitive architecture that relates beliefs (i.e., how the agents perceive their world), desires (i.e., what their goals are) and intentions (i.e., what plans can be undertaken to reach these goals).
The data used to define these behavioral components is based on a survey of 25 households who have changed at least two times their land-use from 1997 to 2014. All come from the village of Binh Thanh, Thanh Phu district, Ben Tre province. The pie chart on Figure 10 depicts the results of this survey, and we can see that there is a diversity of motivations and expectations that emerge from them. Looking for a higher profit is important, but following what the neighbors do or adapting to changes in land suitability are also frequently cited. This sub-models relies on two main assumptions: (1) these is a 1:1 relationship between farmers and parcels (one farmer only exploits one parcel); (2) the productivity of farmers remains constant and is not affected by technical progress or population growth. While these assumptions are mostly correct for the period of our study, they would need to be reevaluated for longer periods.
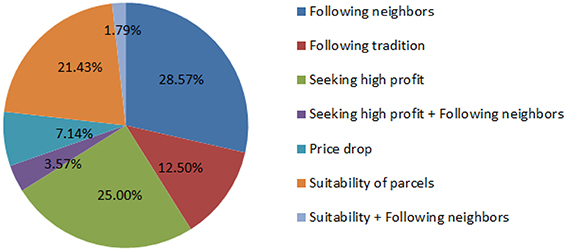
Figure 10. Survey results about the main motivations behind land-use change in Binh Thanh Village, Thanh Phu district, Ben Tre province, Vietnam.
3.6.2. Model Design
Farmer agents are defined as in Figure 11, with their attributes, potential actions and potential plans. Each agent is also provided with specific properties, called predicates, that can be either true or false. These predicates, which usually represent a combination of attributes and perceptions of the agent, are used to track which belief, desire or intention should be made active.
• Beliefs: Beliefs are a set of predicates, which represent the perception farmers have of their environment and themselves.
The beliefs base is updated by a function called update_beliefs() at initialization and after each step of the simulations. The beliefs of farmers are defined in Table 1, together with the conjunction of conditions to meet to make each belief become true. The three first beliefs are computed based on the available amount of money of farmers. The others are based on the perception of farmers agent regarding the prices of products, the costs of production and the land suitability of their parcel.
• Desires: Desires represent a set of objectives that farmers would like to achieve based on their beliefs. Each desire has a priority, which is used to choose the next intention of farmers. A desire can be achieved only when the predicate of the desire is added into the beliefs base or is destroyed by the agent (i.e., when the agent believes that the desire has been realized). Based on our survey data (5), each farmer can have different desires which are not necessarily exclusive. The desires of farmers are listed in Table 2. These desires are set or added by an action called update_desires(), which allows farmers to update their desires based on their beliefs base.
- Farmers try_not_to_change.
Every farmer can have this desire (it is their initial one), notably after they have changed to their preferred land-use. During simulations, this desire is added to the base when farmers believe that they do not have enough money to change, or that their income is greater than the average income, or that they use their land like their neighbors, or, finally, that the price of their product has not changed.
- Farmers want to minimize_risks (environmental and financial ones).
This desire is designed for farmers who believe that they belong to an average category, that the price of their products is increasing, and that they do not have enough money to select another land-use type. It is also influenced by the belief regarding the suitability of their parcel. If it appears to be (or to have become) not suitable for their current land-use, they will load the desire to change to another one, even if it does not provide the highest income.
- Farmers want to earn_the_highest_possible_income.
Normally, the land uses that require high investments will provide higher incomes. This desire is used by standard farmers who believe they have enough money to shift to a land-use type providing the highest possible profit.
- Farmers want to imitate_their_successful_neighbors.
This desire is setup for farmers who believe that they are poor. If they perceive that their neighbors have changed their land use, and that they have been successful in doing so, then they have the desire to change to the same land-use; this desire will also trigger the desire to loan money from banks if the shifting cost is higher than their money.
- Farmers want to loan_money_from_banks in order to shift to a new land-use type.
After farmers select a land-use type different from the one they currently undertake, and if they believe they do not have enough money to change, they add a temporary desire to loan money from a bank, which is provided with the highest priority until they can effectively change.
• Intentions: An intention (i.e., what the agent intends to do) is defined for every desire with the goal of fulfilling it as soon as possible. Theoretically, when an agent has two or more simultaneous desires, the corresponding intentions are executed simultaneously. However, because of technical limitations of the BDI architecture we used, intentions are organized sequentially. For example, if a farmer has three simultaneous desires, the three corresponding intentions are added into a first-in-last-out stack of intentions, and the last one is set as the current intention while the others are put on hold. The current intention will determine the selected plan. Plans are a set of actions, which can be executed over the course of several steps of simulation until its post-conditions become true or the related intention is removed from the base of intentions. The relationships amongst Beliefs, Desires and Intentions are showed in Table 2. For example, when farmers intend to change their land-use to earn_the_highest_possible_income but do not have_money_to_change, they desire to loan_money_from_banks, which triggers the corresponding plan (loan_from_banks) and postpones change_to_highest_income.
The set of plans defined for farmers is:
- “change_to_land_suitability()”: This plan is executed when “minimize_risk” is set as the current intention. In this plan, farmer agents select a new land-use type based on the criteria of highest suitability, so as to minimize potential risks, even if the expected income is not the highest.
- “change_to_highest_income()”: This plan is executed when farmers have the intention to “earn_the_highest_possible_income.” In this plan, they select a land-use type that is expected to provide them with the highest income, even if it is risky (if the land is not really suitable, for instance).
- “change_to_land_use_of_neighbors()”: This plan is executed when farmers have the intention to “imitate_their_successful_neighbors.” In this plan, agents undertake the necessary actions to change to the land-use type chosen by their immediate (geographical) neighbors who have earned a higher income than them the year before.
- “loan_from_banks()”: In this plan, farmers request a loan from banks when they have planned to change their land use but do not have enough money to invest in this shift. This request is not automatically fulfilled; as it is the case in reality, this intention is added sequentially when farmers execute the intentions “imitate_their_successful_neighbors” and “earn_the_highest_possible_income.” The result of the loan request is decided by the use of a probabilistic function, which takes into account the beliefs of farmers and the compatibility of the new land-use with that projected in the provincial plan (see Table 2).
- “keep_current_land_use()”: This plan is executed when farmers have the intention to “try_not_to_change.” It does not change anything.
In addition to the actions defined in these different plans, each farmer agent undertakes a number of mandatory actions every simulation step, such as paying back loans to the bank or computing their income.
3.7. M_N: Sub-Model of Farmers Relationships
3.7.1. Presentation
In Hamill and Gilbert (2009), the author supposes the existence of a network in which farmers can be influenced by and can influence their “neighbors.” This concept of “neighborhood” can take many forms, from topological or geographical relationships, which rely on the proximity between farmers, to familial or social-economic ones, in which, for instance, the level of income would be used as a filter. A first assumption is made here by considering that the familial network is superseded by the proximity network since in Vietnam, especially in rural areas, it is common that members of the same family live next to each other. A second assumption is that the exchanges of influence take place between farmers that belong to the same “social level” (or income group).
Statistical population data used at the provincial level (VGSOBT, 2010) distinguishes between 3 different profiles of farmers, essentially based on their level of income: (1) P1: rich and standard farmers, (2) P2: average farmers, (3) P3: poor and nearly poor farmers. We reuse this classification and couple it with the proximity network in order to produce an “influence network” for each farmer.
3.7.2. Model Design
This network is recomputed at every iteration of the simulation (as farmers may change their income) and its main purpose is to serve as a “social topology” for farmers, i.e., to modify the way they compute their set of neighbors. In the absence of this sub-model, the neighbors of a farmer are the farmers located in a radius of 100 m around it. When this sub-model is used, the neighbors become the farmers located in the same radius and belonging to the same profile.
4. Integration of the Sub-Models
4.1. Models as Agents
To couple these different models, and to address the limitations pointed out in Section 2.2, we introduce in this section the concept of “co-modeling.” This concept is relying on a multi-agent approach to knowledge and control decentralization, but also borrows from DEVS its recursive design of models.
Basically, a “co-model” is an agent-based model in which some agents represent other models (called, in that case, “micro-models”). As in any “regular” ABM, these agents can be added or removed dynamically from their “macro-model.” They have their own attributes, life cycle, operations, collaborations, conflict resolution mechanisms, etc. and the only difference with regular agents is that they wrap one or several instances of other models (which can, themselves, be co-models).
The interest for modelers (at least for modelers already using the ABM paradigm) is that they don't have to learn a new set of concepts for coupling sub-models. If they know how to write an agent-based model, they are normally able to write a co-model. One difference, though, is that a co-model is intended to capture and represent a particular collaboration between these “micro-models,” which can be based on a collaboration scheme of experts, for instance, or on any other way of organizing their contributions. Conversely, any regular ABM can be viewed as a very specific implementations of co-models, where agents only wrap models of individuals.
This proposal, implemented in the GAMA simulation platform (but which could be implemented in any other platform as long as its meta-model supports multi-level definitions and recursivity within agents), does not solve the numerous problems (of relating the inputs and outputs, harmonizing the scales, and so on) raised by coupling heterogeneous models. Its main purpose is to introduce a natural way of writing elegant solutions to these problems, where the relationships between models are viewed as interactions between artificial agents. The whole literature on multi-agent systems (Michel et al., 2009) can then be used to imagine and implement specific collaboration protocols between micro-models, which can consist in exchanges of data, of course, but also more sophisticated interactions (control of one model by another, self-organization of micro-models, hierarchical organization of micro-models, exchange of semantic information, etc.). Furthermore, considering models as agents allows to envision new ways of designing integrated models, for instance through an interactive participatory process with the modeler (Guyot et al., 2005).
This approach to models coupling is still experimental for the moment, and it needs to be validated especially in its advanced uses, for example dynamically coupling/uncoupling models during simulations. What is interesting, however, is that it supports modelers in designing flexible experiments, with the possibility to add, remove or change micro-models at runtime. In our case, this possibility will be extensively used for testing, individually and collectively, the different hypotheses.
As specified in Section 2.2, current land-use change integrated models are not flexible enough when it comes to switch between different micro-models. By considering a model as an agent, and an integrated model as a multi-agent system, our approach tries to bring this flexibility to modelers. In addition, GAMA offers several software design capabilities that support very powerful features: because models are agents, they can inherit from other agent definitions, even abstract ones that only define interfaces for getting and setting parameters or manipulating the model. This allows to define abstract integrated models (like frameworks use to be defined in an object-oriented approach) in which micro-models, which only need to respect the interfaces, are instantiated at runtime, whatever their actual contents or definition. Thanks to its multi-formalism approach, GAMA also allows these models to be written in whatever formalism is available as a plug-in to the platform. Finally, because of the possibility to call external programs or libraries during simulations, a model in GAMA can perfectly be a simple wrapper around a legacy model written in another language or on another platform, provided this model can be launched and monitored during execution by an external process.
In Figure 6 we present the conceptual diagram that describes the wrapping and the coupling of the models listed above: M_F, M_E, M_P, M_S, M_N respectively represent the models of farmers, parcels, environment, economy and social relationships. M_F is coupled with the other models by the way of data exchanges: it requires inputs like product prices (called LU price in M_E), current parcel suitability (LU suitability in M_P) or the decision of neighbors (LU type of M_N). Conversely, it makes the new land use type chosen by the farmers available for other models (it is for example used by M_E and M_N). The two models M_S and M_P are also coupled in that they exchange the salinity attribute.
4.2. Implementation Using GAMA/GAML
The integrated co-model is implemented in the GAML language (Grignard et al., 2013). Its workflow is presented on Figure 12. The micro-models are first imported as “wrapping” agents, which need to be instantiated and initialized like any other agent. Each step of the co-model simulation corresponds to 1 year, during which micro-models are executed depending on their temporal scale (from 1 month to 1 year), their inputs and outputs being taken in charge by the co-model in a completely transparent way.
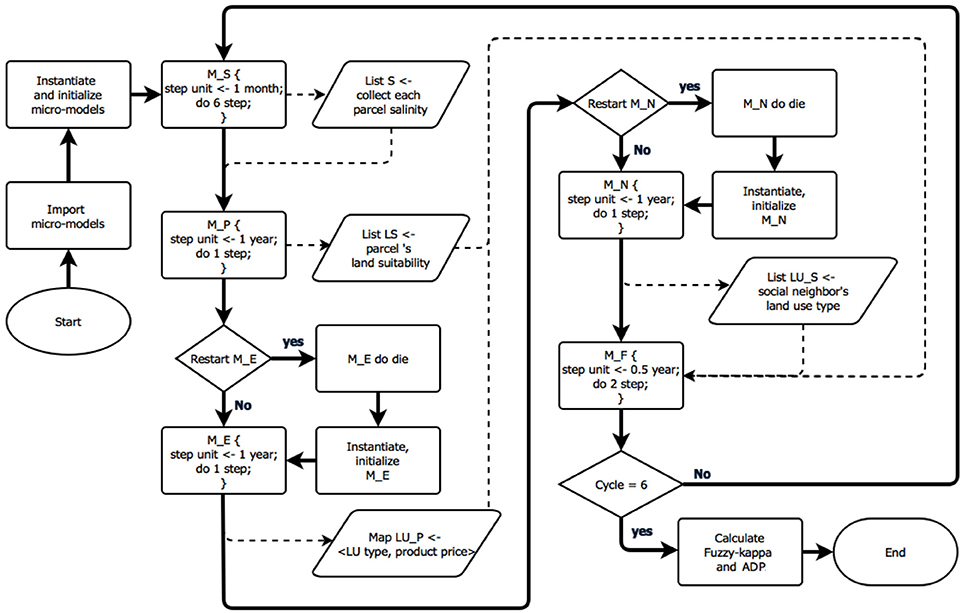
Figure 12. Co-model workflow implemented in GAMA. Bold arrows are the flow of processes. Dotted lines represent the flows of data.
The full GAML code used to implement the co-model can be found in the Annex. In the following, we highlight some of its parts, mainly to show that manipulating micro-models is not different than manipulating agents. The life cycle of the co-model begins with the importation of the micro-models, which are provided with an alias identifier:
import <model_file.gaml> as <micro−model
identifier>
Micro-models can then be instantiated using the regular “create” statement that specifies the name of the experiment (in GAML, a model is a specification of a simulation, and an experiment is a particular way of instantiating this simulation) and the number of instances of this experiment to create.
create <micro−model identifier >.
<experiment name> [number: number];
As for any regular agents, access to the attributes and actions of micro-models use the “ask” statement
ask <micro—model identifier>.<experiment
name {do action;}
The synchronization of the temporal scales of micro-models is realized by asking their instances to run one or several times, for example, we ask model M_S do 6 simulation cycles.
ask M_S.simEnv {loop times:6 {do _step_;}}
Finally, a micro-model can be dynamically destroyed (e.g., “ask micro_model do die;”) and re-created during the course of the co-model, which allows to run it in its virgin state every time it is needed.
5. Experiments
5.1. Input Data
In order to validate our integrated model, we chose a scenario with initial data for the agents in the sub-models are resumed in Table 3.
5.1.1. Indicators and Comparison
The main outcome of each simulation of the integrated model is a map of land-use in 2010. To assess its validity, two indicators are used for comparing it to the land-use map observed in 2010: Absolute Deviation Percentages (ADP), which measures the global absolute difference between the maps, and Fuzzy Kappa (FKappa, Visser and de Nijs, 2006), used to measure their similarity based on local correlations, as defined in Section 1.1. These indicators are already implemented in the GAMA platform.
with: the observed quantity of parcels with land-use i and Xi the simulated quantity of parcels with land-use i.
An experiment is defined as a specific combination of micro-models. Because of the possible stochasticity of some of these combinations, 100 simulations are launched for every experiment. Their ADP and Fuzzy Kappa are computed, and we use a one-way ANOVA with the assumption of equal variances and a 95% confidence interval (Bewick et al., 2004) to produce their average values.
These two average values are then compared to the ones obtained for, respectively, a hypothetical scenario characterized by an absence of changes (i.e., where the map obtained in 2010 is supposed to be the same as the one observed in 2005), a hypothetical random model (i.e., a model where farmers choose their land-use randomly every year) and, finally, the map used by the planners in 2005 to estimate the situation in 2010 (see Figure 4).
The random model experiments output an average FKappa of 8% and an ADP of 126%, displaying a very low correlation with reality, which is not really a surprise given the randomness of the farmers' behaviors. We can nevertheless note that these random simulations give on average a better result than the projected map established in 2000 by the planners (which FKappa and ADP respectively amount to −0.007% and 186%) !
In the “no changes” scenario, the comparison between the initial situation (2005) and the final one (2010) provides a FKappa with a value of 24.4% and an ADP of 94%, which acknowledges the fact that, actually, numerous changes occurred during these 5 years. The surprise is that this low correlation is still beating the one computed for the projected map revised in 2005, with an FKappa of 17,5% and an ADP of 59%. Although these numbers represent an improvement over both the map for year 200 and the random map, they represent an extremely low correlation with the observed land-use, moreover affected by a strong variance.
5.1.2. Validation of the Co-Model
In order to verify the relevance of the hypotheses discussed in Section 3.1, three experiments have been designed and will be presented here. They are summarized in Table 4.
- The first experiment supposes that the farmer decision is based on the sole criterion of the products markets prices. It is therefore a combination of M_P (basic model of parcels, without salinity, which means that the land suitability does not evolve), M_F (model of farmers) and M_E (economic model). Note that the BDI architecture is a bit underused in this case, as only one desire will be triggered in the absence of other predicates.
- The second experiment consists in the coupling of the M_S (salinity) micro-model with the previous ones. This aims at feeding the beliefs, desires and intentions of the agents with dynamic values of land suitability in addition to the market prices. The BDI architecture is then really put to test in this second experiment.
- Finally, the third experiment consists in adding M_N (neighbors) to the previous micro-models, which fuels beliefs and desires with new predicates about the neighbors attitudes.
5.2. Experimental Results
The results of the three experiments are displayed in Figure 13. For the first experiment, FKappa is measured at 39.4% and ADP at 43.22%. While definitely more accurate than the maps used by planners, the comparison between simulated and real land-use maps reveals a high variance that prevents them to be really exploitable. The assessment of these results can only conclude that, while the economic factors certainly play a role in explaining land-use changes dynamics, they cannot explain the totality of the changes observed in reality.
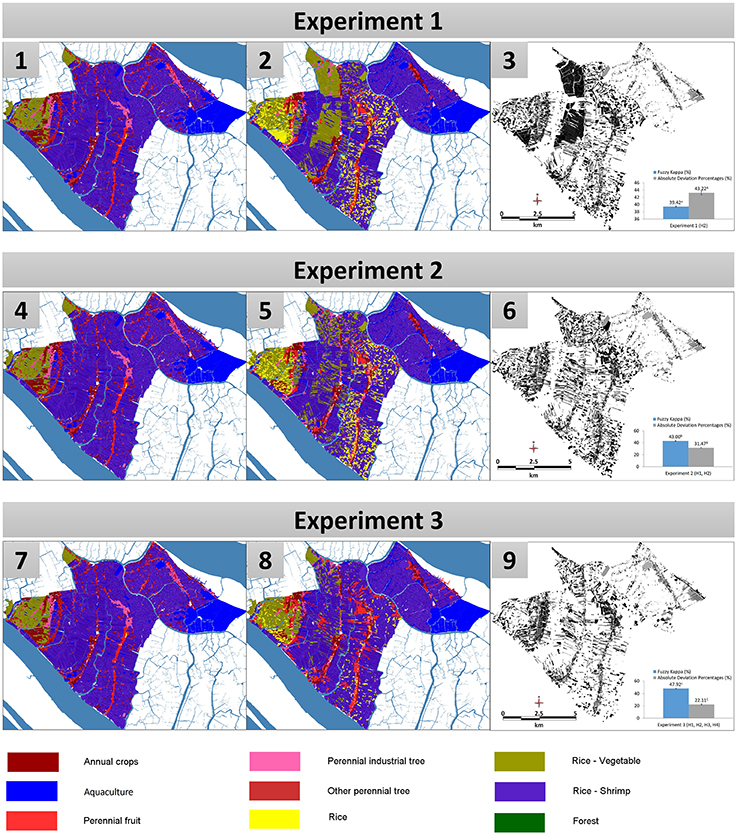
Figure 13. Experiment 1: Comparison between the simulated and observed land-use maps in 2010. Experiment 2: Comparison between the simulated and observed land-use maps in 2010. Experiment 3: Comparison between the simulated and observed land-use maps in 2010. (1), (4), (7) are the results of simulations. (2), (5), (8) are the observed land-use maps in 2010. (3), (6), (9) are the FKappa maps, which show the differences between the two other ones.
By coupling the salinity model to the other micro-models, and therefore adding the criterion of land suitability to the beliefs of farmers, the second experiment produces better results. FKappa increases from 39.42% to almost 43.00%, while the ADP sharply decreases from 43.22% to 31.47%. The dynamic combination of the three first hypotheses (use of the BDI architecture (H1), influence of market prices (H2) and land suitability (H3)) produces a land-use change dynamics that explains nearly half of the observed changes, which is a definite improvement over the maps produced by planners.
The results of the third experiment reveal an ADP of 22% (meaning a global accuracy of 78% in terms of surfaces devoted to each land-use) and a FKappa of 47.92%. The very simple social model M_N, coupled with the three previous ones, appears to improve again the accuracy of the integrated model over its previous incarnations. Although this model represents only one basic way to implement hypothesis H4, it underlines the importance of social factors in the decision-making of farmers, and paves the way for the design of more complex social models (fed with more accurate data on farmers' associations, familial networks, and farmers' local interactions).
5.3. Assessment of the Co-Modeling Architecture
These three experiments have allowed us to test the agent-based co-modeling architecture used to build the different integrated models. By considering each micro-model as an agent, co-modeling definitely eased the experimental process depicted above, allowing us to add or remove micro-models with a minimum of efforts (a few code changes, and only in the co-model, not in any of the micro-models). Moreover, we have been able to manipulate, without any particular constraints, models defined in different formalisms and make them communicate and exchange data and control in a completely transparent way.
This experimental flexibility will be precious to progressively refine and test either the integrated model or the existing micro-models. Adding, removing, changing and verifying hypotheses translates, in the co-modeling architecture, into adding, removing, changing and running micro-models. The only changes required concern the “glue” between micro-models (i.e., the code inside the co-model itself), which allows, if the initial code is sufficiently modular, to create hierarchies of co-models, from the simplest ones to more sophisticated ones.
5.4. Assessment and Discussion
The use of a model based on the decision-making of farmers for better predicting land-use changes in the Mekong Delta was the first aim of this research and the three experiments described above are an indication that this approach is clearly promising. When we look at Figure 14, it is easy to see that the projections used by planners, in 2000 and 2005, do not even show a remote correlation with the state of land-use in 2010. However, starting with the same input data (the land-use map of 2005) and progressively providing farmer agents with knowledge about their economic, environmental and social contexts (with appropriate decision-making processes and behaviors), we have been able to reach nearly 50% of similarity between the map produced by simulation and the map of 2010. Given the extreme simplicity of two of the micro-models employed (the economic and social micro-models), this already represents an interesting achievement, and our plan is to push this further and contact provincial planning authorities so that this model, or a particular instantiation of it, becomes the de facto standard for projecting future land-use in the Mekong Delta. However, it is still, today, in its preliminary stage and we need to improve it if we want it to become really usable by land-use planners.
The first improvement concerns enhancing the realism of the economic and social models, M_E and M_N. The former, which is presently based on the assumption that market prices will follow the dynamics observed in the years 2005-2010, should at least take into account: (1) the global evolution of agricultural trade in South-East Asia, because Vietnam is a net exporter of various products (rice, shrimps, etc.); (2) the local balance between demand and offer, since, besides the price of rice, which is guaranteed by the Vietnamese government, all other products are dependent on the market. Moreover, the market also influences the amount of the investment necessary for shifting from one land-use to another: the prices of fertilizers, seeds and facilities are fluctuating depending on the demand but also on the government's incentive policies. All of these factors need to be taken into account in a more realistic economic model. Regarding the social model, the network of influence goes actually well beyond the immediate neighbors, as is the case in the current model. Farmers' trade unions, extended family networks, political organizations, TV broadcasts all contribute to how innovation or experiments can spread to one village to another. In Vietnam's rural areas, moreover, people often bypass traditional lending institutions when it comes to invest and rely instead on groups of people, often families or a combination of family and trusted friends and neighbors, to lend them money through a mixed mechanism of money pool and lottery (called “hui,” translated by “tontine”). A more accurate social model would need to take into account these different networks and how they add up to facilitate the diffusion of new agricultural trends and practices. Crucial data is however missing in the latter case, as very few studies have been undertaken to understand how these networks are constituted and their real influence on farmers. This is where a model like the one presented in this paper is also important, as it points social scientists toward the necessity to undertake specific surveys or fieldwork.
Another area of improvement concerns the addition of a micro-model that would represent the role of the provincial authorities themselves. In our current model, institutions are not taken into account, but they nevertheless play a crucial role in reality, by promoting the plan (through social or political channels), regulating the access to loans or parcels, providing training on agricultural techniques or undertaking large infrastructure investments (like sluice gates and dikes building, irrigation facilities, but also transport infrastructure). Neglecting this direct or indirect role in land-use change, especially in a country like Vietnam where the state is so present, is of course not possible in a complete integrated model. An interesting side effect of incorporating institutions in the model would also be to engage planners in the design of the model by allowing them, maybe in participatory setups, to study the impacts of different policies or investment scenarios.
6. Conclusion
In this article, we have proposed a solution for improving land-use change planning, through the design of a hybrid integrated model that captures environmental, social and economic dynamics of this complex phenomenon. The two main outcomes of our work are, on one hand, the model itself, which have proved, despite the simplicity of some sub-models, to be already more accurate than the projections used by planners in the Vietnamese Mekong Delta, and, on the other hand, a flexible agent-based approach to the coupling of heterogeneous models, implemented in an existing simulation platform.
The necessity to use an integrated model has been established from a careful review of the context and existing research, which showed that land-use change should be understood as a consequence of the interplay of numerous factors and actors, including of course farmers. We then proposed a breakdown into several sub-models, each of them representing one of these factors or conjunction of factors, and aiming at verifying one hypothesis. The most central of them, the models of farmers, is implemented using a cognitive AI architecture, BDI, which allows to represent them as complex actors whose beliefs help them to arbitrate between several, sometimes contradictory, desires.
All these sub-models are composed using a new coupling infrastructure called “co-modeling,” where they are represented as agents belonging to a higher-level agent-based model (called macro-model); this infrastructure, implemented on the GAMA modeling and simulation platform, provides the flexibility missing in most of the existing proposals, either in the field of land-use change modeling (where the majority of models is specific to a given case study), or in the more general field of multi-modeling, where the price to pay for building integrated models is generally higher in terms of technological investment.
This flexibility has been tested when designing the experiments presented in this article: adding, removing or switching models, even dynamically, has allowed us to present a progressive assessment of the different hypotheses on which our approach to land-use change was based. Though we did not test all the possible combinations of micro-models and did not propose alternate versions of them, this possibility exists and will be exploited in the future versions of our integrated model. In its current state, the integrated model already offers an interesting combination of modeling techniques and formalisms: a BDI agent-based model of farmers, a cellular automaton based model of diffusion of salinity, a dynamic GIS data based model of parcels, an economic equation-based model and a graph-based social network model. All of them, for the sake of the article, have been programmed in the GAML language (which natively offers the support of multiple formalisms), but reusing legacy models just involves wrapping their invocation in the definition of an agent behavior.
There are three main perspectives of this research that we plan to explore in the near future. The first one will consist in refining the current integrated model, by progressively completing it with more micro-models targeting the other factors identified in land-use change (e.g., an institutional model, local climatic models, models of urban growth) or enhancing the realism of the existing micro-models (by conducting more focused surveys and fieldwork with farmers). The second one will consist in expanding the case study so as to address the problem of land-use change in non-coastal regions of the Mekong Delta, where the environmental conditions are quite different. This perspective will allow us to test one very important feature of the co-modeling architecture, namely the possibility to use different versions of the same micro-model depending on some conditions (for instance, using different environmental micro-models depending on the location of the parcels). Finally, the third perspective will consist in abstracting, as much as we can, the macro-model and the model of farmers decision-making, so as to propose a generic framework that could be instantiated in other geographical contexts. These three perspectives will be pursued throughout 2016 as part of a cooperation between the IRD and Can Tho University.
Author Contributions
The three authors have worked together, each in his own specialty, to design and describe the research presented in the paper. AD is the lead designer of the GAMA platform, on which the experiments have been performed, and one of the researchers behind the idea of the “co-modeling” architecture. He has mainly worked on the general outline, introductory and conclusive parts of the paper. NH is the main developer of the “co-modeling” architecture and responsible for its implementation in the GAMA platform. He has also designed some sub-models, among which the salinity model, and helped designing complex experiments. QT is the developer of the BDI decision-making architecture used for farmer agents and the main designer of the integrated model. He has also been responsible for collecting the social, spatial and environmental data used in the model and analysing and validating the outputs of simulations.
Conflict of Interest Statement
The authors declare that the research was conducted in the absence of any commercial or financial relationships that could be construed as a potential conflict of interest.
Acknowledgments
The authors would like to thank Prof. Patrick Taillandier (IDEES, France), Prof. Benoit Gaudou (IRIT, France), Prof. Jean-Daniel Zucker (IRD, France), Prof. Philippe Caillou (LRI, France), Prof. Huynh Xuan Hiep (CICT, Vietnam), Prof. Nguyen Hieu Trung (CENRES, Vietnam), and Prof. Vo Quang Minh (CENRES, Vietnam) for their contributions to the various stages of this research.
References
Ahnstrm, J., Hckert, J., Berge, H. L., Francis, C. A., Skelton, P., and Hallgren, L. (2009). Farmers and nature conservation: what is known about attitudes, context factors and actions affecting conservation? Renew. Agric. Food Syst. 24, 38. doi: 10.1017/S1742170508002391
Bakker, M. M., Alam, S. J., van Dijk, J., and Rounsevell, M. D. A. (2015). Land-use change arising from rural land exchange: an agent-based simulation model. Landsc. Ecol. 30, 273–286. doi: 10.1007/s10980-014-0116-x
Beratan, K. K. (2007). A cognition-based view of decision processes in complex social ecological systems. Ecol. Soc. 12:27.
Bertsch, C., Ahle, E., and Schulmeister, U. (2014). “The functional mockup interface - seen from an industrial perspective,” in Linköping Electronic Conference Proceedings, 2014 (Lund), 27–33. doi: 10.3384/ecp1409627
Bewick, V., Cheek, L., and Ball, J. (2004). Statistics review 9: one-way analysis of variance. Crit. Care 8, 130–136. doi: 10.1186/cc2836
Blochwitz, T., Otter, M., Akesson, J. R., Arnold, M., Clau, C., Elmqvist, H., et al. (2012). “The functional mockup interface for tool independent exchange of simulation models,” in Proceedings of the 9th International MODELICA Conference (Munich), 173–184.
Bollinger, L. A., Nikoli, I., Davis, C. B., and Dijkema, G. P. (2015). Multimodel ecologies: cultivating model ecosystems in industrial ecology. J. Indust. Ecol. 19, 252–263. doi: 10.1111/jiec.12253
BTCA (2015). Weekly Newsletters of Coconut Prices. Ben Tre: Ben Tre Coconut Association. Retrieved from: http://hiephoiduabentre.com.vn/index.php?Module=Content&Action=blogCategory&id=108&Itemid=207
Caillou, P., Gaudou, B., Grignard, A., Truong, Q. C., and Taillandier, P. (2015). “A simple-to-use BDI architecture for agent-based modeling and simulation,” in The Eleventh Conference of the European Social Simulation Association (Groningen).
Case, A. (1992). Neighborhood influence and technological change. Regional Sci. Urban Econ. 22, 491–508. doi: 10.1016/0166-0462(92)90041-X
Cohen, J. (1960). A coefficient of agreement for nominal scales. Educ. Psychol. Meas. 20, 37–46. doi: 10.1177/001316446002000104
Dahmann, J., Fujimoto, R., and Weatherly, R. (1998). “The DoD high level architecture: an update,” in Simulation Conference Proceedings, 1998, Winter, Vol. 1 (Washington, DC: IEEE), 797–804. doi: 10.1109/wsc.1998.745066
Dahmann, J. S., and Morse, K. L. (1998). “High level architecture for simulation: an update,” in Proceedings of the Second International Workshop on Distributed Interactive Simulation and Real-Time Applications, DIS-RT '98 (Washington, DC: IEEE Computer Society), 32. doi: 10.1109/disrta.1998.694563
DARD (2015). Weekly Newsletters of Agricultural Prices. An Giang: Department of Agriculture. Retrieved from: http://sonongnghiep.angiang.gov.vn/wps/portal/
Edmonds, B., and Moss, S. (2005). “From KISS to KIDS - an “anti-simplistic” modelling approach,” in Multi-Agent and Multi-Agent-Based Simulation, volume 3415 of Lecture Notes in Computer Science, eds P. Davidsson, B. Logan, and K. Takadama (Berlin; Heidelberg: Springer), 130–144.
GAMA (2007). GAMA Platform - GAMA is an Agent-Based, Spatially Explicit, Modeling and Simulation Platform. Available online at: http://gama-platform.org
Grignard, A., Taillandier, P., Gaudou, B., Vo, D. A., Huynh, N. Q., and Drogoul, A. (2013). “GAMA 1.6: advancing the art of complex Agent-Based Modeling and Simulation,” in PRIMA 2013: Principles and Practice of Multi-Agent Systems, Number 8291 in Lecture Notes in Computer Science, eds G. Boella, E. Elkind, B. T. R. Savarimuthu, F. Dignum, and M. K. Purvis (Berlin; Heidelberg: Springer), 117–131.
Guyot, P., Drogoul, A., and Lemaître, C. (2005). “Using emergence in participatory simulations to design multi-agent systems,” in Proceedings of the Fourth International Joint Conference on Autonomous Agents and Multiagent Systems, AAMAS '05 (New York, NY: ACM), 199–203. doi: 10.1145/1082473.1082503
Hamill, L., and Gilbert, N. (2009). Social circles: a simple structure for agent-based social network models. J. Artif. Soc. Soc. 12:3.
Hild, D. R. (2000). Discrete Event System Specification (Devs) Distributed Object Computing (Doc) Modeling and Simulation. PhD thesis, The University of Arizona.
Hill, D. R. C. (2002). Theory of modelling and simulation: integrating discrete event and continuous complex dynamic systems: second edition by B. P. Zeigler, H. Praehofer, T. G. Kim, Academic Press, San Diego, CA, 2000. Int. J. Robust Nonlin. Control 12, 91–92. doi: 10.1002/rnc.610
Huang, H., Wang, L., Zhang, X., Luo, Y., and Zhao, L. (2008). “Coupling multi-agent model and GIS to simulate pine wood nematode disease spread in ZheJiang province,” in Proceedings of Spie the International Society for Optical Engineering, International Conference, 16th Geoinformatics (Guangzhou). doi: 10.1117/12.812557
Huynh, N. Q., Huynh, H. X., Drogoul, A., and Cambier, C. (2014). “Co-modeling: an agent-based approach to support the coupling of heterogeneous models,” in Nature of Computation and Communication, Number 144 in Lecture Notes of the Institute for Computer Sciences, Social Informatics and Telecommunications Engineering, International Conference, ICTCC 2014, eds P. C. Vinh, E. Vassev, and M. Hinchey (Ho Chi Minh City: Springer), 156–170.
Judge, G. G., Hill, R. C., Griffiths, W., Lutkepohl, H., and Lee, T.-C. (1988). Introduction to the Theory and Practice of Econometrics. Singapore: Wiley.
JunJie, W. (2008). Land use changes: economic, social, and environmental impacts. Choices 23, 6–10. Available online at: http://purl.umn.edu/94681
Kemeny, J. G., and Snell, J. L. (1983). Finite Markov Chains: With a New Appendix “Generalization of a Fundamental Matrix”. New York, NY: Springer.
Kim, K. T. G. (2005). “Proposal of high level architecture extension,” in Artificial Intelligence and Simulation, number 3397 in Lecture Notes in Computer Science, ed T. G. Kim (Berlin; Heidelberg: Springer), 128–137.
Lambin, E., and Geist, H. J. (2007). Causes of Land-Use and Land-Cover Change. Retrieved from: http://www.eoearth.org/view/article/150964
Lambin, E. F. (1997). Modelling and monitoring land-cover change processes in tropical regions. Prog. Phys. Geogr. 21, 375–393. doi: 10.1177/030913339702100303
Le, Q. B., Park, S. J., Vlek, P. L. G., and Cremers, A. B. (2008). Land-use dynamic simulator (LUDAS): a multi-agent system model for simulating spatio-temporal dynamics of coupled humanlandscape system. I. Structure and theoretical specification. Ecol. Inform. 3, 135–153. doi: 10.1016/j.ecoinf.2008.04.003
Li, X., Wang, W., Lei, Y., and Li, Q. (2013). “An agent-centered multi-formalism modeling framework for SoS behavior,” in Proceedings of the 2nd International Conference on Computer Science and Electronics Engineering (ICCSEE 2013), Advances in Intelligent Systems Research Series (Paris: Atlantis Press), 624–629. doi: 10.2991/iccsee.2013.159
Mena, C. F., Walsh, S. J., Frizzelle, B. G., Xiaozheng, Y., and Malanson, G. P. (2011). Land use change on household farms in the Ecuadorian Amazon: design and implementation of an agent-based model. Appl. Geogr. 31, 210–222. doi: 10.1016/j.apgeog.2010.04.005
Michel, F., Ferber, J., and Drogoul, A. (2009). “Multi-agent systems and simulation: a survey from the agents community's perspective,” in Multi-Agent Systems: Simulation and Applications, Computational Analysis, Synthesis, and Design of Dynamic Systems, ed A. U. Danny Weyns (CRC Press; Taylor & Francis), 47.
MONRE (2009). Detailing the Establishment, Regulation and Evaluation Planning, Land-Use Planning. Circular of Ministry of Natural Resources and Environment, No 19/2009/TT-BTNMT, 2009, Hanoi.
MONRE (2012). Decision on Publishing Statistical Data of Land-Use Change in 2011. Decision of Minister, Ministry of Natural Resources and Environment of Vietnam, No 1482/QD-BTNMT, 2012, Hanoi.
Moreira, E., Costa, S., Aguiar, A. P., Cmara, G., and Carneiro, T. (2009). Dynamical coupling of multiscale land change models. Landsc. Ecol. 24, 1183–1194. doi: 10.1007/s10980-009-9397-x
Murray-Rust, D., Robinson, D. T., Guillem, E., Karali, E., and Rounsevell, M. (2014). An open framework for agent based modelling of agricultural land use change. Environ. Model. Softw. 61, 19–38. doi: 10.1016/j.envsoft.2014.06.027
Nguyen, M. T. H., Vo, M. Q., and Vo, G. T. (2014). Suitable land use zoning in line with climate change scenarios for three coastal districts in Ben Tre province. Vietnam Soil Sci. 44, 68–74.
Nguyen, N. D., Drogoul, A., and Auger, P. (2008). “Methodological steps and issues when deriving individual based-models from equation-based models: a case study in population dynamics,” in Proceedings of the Intelligent Agents and Multi-Agent Systems, 11th Pacific Rim International Conference on Multi-Agents, PRIMA 2008, Hanoi, December 15–16, 2008 (Hanoi), 295–306. doi: 10.1007/978-3-540-89674-6_33
Nicolai, T. W., Wang, L., Nagel, K., and Waddell, P. (2011). “Coupling an urban simulation model with a travel modela first sensitivity test,” in Proceedings of the Computers in Urban Planning and Urban Management (CUPUM '11) (Lake Louise, AB), 11–07.
North, M. J., Collier, N. T., and Vos, J. R. (2006). Experiences creating three implementations of the repast agent modeling toolkit. ACM Trans. Model. Comput. Simul. 16, 1–25. doi: 10.1145/1122012.1122013
Parker, D. C., Berger, T., and Manson, S. M. (2002). “Agent-based models of land-use and land-cover change,” in Report and Review of an International Workshop (Irvine, CA).
Parker, D. C., Manson, S. M., Janssen, M. A., and Hoffman, M. J. P. D. (2003). Multi-agent systems for the simulation of land-use and land- cover change: a review. Ann. Assoc. Am. Geograph. 93, 316–340. doi: 10.1111/1467-8306.9302004
Parrott, L. (2011). Hybrid modelling of complex ecological systems for decision support: recent successes and future perspectives. Ecol. Inform. 6, 44–49. doi: 10.1016/j.ecoinf.2010.07.001
PCBT (2011). Report of Land-Use Zoning in 2020 and the Land-Use Plan for 5 Early Years 2011–2015 of Ben Tre Province. Ben Tre: People's Committee of Ben Tre Province.
Quesnel, G. V. D. (2005). “Coupling of physical models and social models: multi-modeling and simulation with VLE,” in Joint Conference on Multi-Agent Modelling for Environmental Management (CABM-HEMA-SMAGET05) (Bourg Saint Maurice), 21–25.
Rajeevan, M., and Nanjudiah, R. (2009). Coupled model simulations of twentieth century climate of the indian summer monsoon. Curr. Trends Sci. 537–567.
Rao, A., and Georgeff, M. (1991). “Modeling rational agents within a BDI-architecture,” in Proceedings of the 2nd International Conference on Principles of Knowledge Representation and Reasoning (San Mateo, CA), 473–484.
Rochette, S., Huret, M., Rivot, E., and Le Pape, O. (2012). Coupling hydrodynamic and individual-based models to simulate long-term larval supply to coastal nursery areas. Fish. Oceanogr. 21, 229–242. doi: 10.1111/j.1365-2419.2012.00621.x
Rousseaux, F., Bocher, E., Gourlay, A., and Petit, G. (2012). “Toward a coupling between GIS and agent simulation: USM, an OrbisGIS extension to model urban evolution at a large scale,” in OGRS 2012 Proceedings (Switzerland), 206–214.
Serneels, S., and Lambin, E. F. (2001). Proximate causes of land-use change in Narok District, Kenya: a spatial statistical model. Agric. Ecosyst. Environ. 85, 65–81. doi: 10.1016/S0167-8809(01)00188-8
SISO, S. (2010). IEEE Standard for Modeling and Simulation (M&S) High Level Architecture (HLA) – Framework and Rules. IEEE Std 1516-2010 (Revision of IEEE Std 1516-2000) (New York, NY), 1–38.
Smajgl, A., Toan, T. Q., Nhan, D. K., Ward, J., Trung, N. H., Tri, L. Q., et al. (2015). Responding to rising sea levels in the Mekong Delta. Nat. Clim. Change 5, 167–174. doi: 10.1038/nclimate2469
Soil Resources Management Conservation Service (ed.). (1981). “A framework for land evalution,” in Number 32 FAO Soils Bulletin, Vol. 2, Print Edition (Rome: FAO).
Steiner, A. L., Pal, J. S., Rauscher, S. A., Bell, J. L., Diffenbaugh, N. S., Boone, A., et al. (2009). Land surface coupling in regional climate simulations of the west african monsoon. Clim. Dyn. 33, 869–892. doi: 10.1007/s00382-009-0543-6
Taillandier, P., and Therond, O. (2011). “Use of the belief theory to formalize agent decision making processes: application to cropping plan decision making,” in European Simulation and Modelling Conference (Guimarães), 138–142.
Trickett, S. B., and Trafton, J. G. (2007). What if: the use of conceptual simulations in scientific reasoning. Cogn. Sci. 31, 843–875. doi: 10.1080/03640210701530771
Truong, Q. C., Taillandier, P., Gaudou, B., Vo, M. Q., Nguyen, T. H., and Drogoul, A. (2016). “Exploring agent architectures for farmer behavior in land-use change. A case study in coastal area of the Vietnamese Mekong Delta,” in Multi-Agent Based Simulation XVI, Lecture Notes in Computer Science, eds B. Gaudou and J. S. Sichman (Istanbul: Springer International Publishing), 146–158. doi: 10.1007/978-3-319-31447-1_10
Valbuena, D., Verburg, P. H., Bregt, A. K., and Ligtenberg, A. (2010). An agent-based approach to model land-use change at a regional scale. Landsc. Ecol. 25, 185–199. doi: 10.1007/s10980-009-9380-6
Vangheluwe, H. (2000). “DEVS as a common denominator for multi-formalism hybrid systems modelling,” in IEEE International Symposium on Computer-Aided Control System Design, ed A. Varga (Anchorage, AL: IEEE Computer Society Press), 129–134.
VASEP (2015). Price of Aquaculture Products in Soc Trang Province from 2005 to 2010. Vietnam Association of Seafood Exporters and Producers. Retrieved from: http://vasep.com.vn/119/Thong-ke-thuy-san/Gia-trong-nuoc.htm
VFA (2015). Prices of Domestic Rice from 2007 to 2010. Ho Chi Minh City: Vietnam Food Association. Retrived from: http://www.vietfood.org.vn/vn/default.aspx?c=101
VGSO (2000). Report of Census for Land-Use Area in 2000. Vietnamese General Statistics Office. Retrived from: http://www.gso.gov.vn/Modules/Doc_Download.aspx?DocID=659
VGSOBT (2010). Statistical Yearbook of Ben Tre Province 2010. Ben Tre: Vietnamese General Statistics Office.
Visser, H., and de Nijs, T. (2006). The map comparison kit. Environ. Model. Softw. 21, 346–358. doi: 10.1016/j.envsoft.2004.11.013
Vo, Q. M., and Le, Q. T. (2006). “Soils of the Mekong delta, based on WRB (World Reference Based) - FAO system, at the scale 1/250.000,” in Collection of the research scientific of College of Agriculture and Applied Biology, Can Tho University, 147–156.
Yez, E., Hormazbal, S., Silva, C., Montecinos, A., Barbieri, M. A., Valdenegro, A., et al. (2008). Coupling between the environment and the pelagic resources exploited off northern chile: ecosystem indicators and a conceptual model. Lat. Am. J. Aquat. Res. 36, 159–181. doi: 10.3856/vol36-issue2-fulltext-3
Young, K. B., Wailes, E. J., Cramer, G. L., and Khiem, N. T. (2002). Vietnam's Rice Economy: Developments and Prospects. Research report, Arkansas Agricultural Experiment Station, Arkansas. Available online at: http://arkansasagnews.uark.edu/968.pdf
Zeigler, B., Moon, Y., Kim, D., and Ball, G. (1997). The DEVS environment for high-performance modeling and simulation. IEEE Comput. Sci. Eng. 4, 61–71. doi: 10.1109/99.615432
Keywords: integrated model, land-use change, hybrid modeling, agent-based modeling, models coupling, cognitive model, Mekong Delta
Citation: Drogoul A, Huynh NQ and Truong QC (2016) Coupling Environmental, Social and Economic Models to Understand Land-Use Change Dynamics in the Mekong Delta. Front. Environ. Sci. 4:19. doi: 10.3389/fenvs.2016.00019
Received: 29 June 2015; Accepted: 02 March 2016;
Published: 30 March 2016.
Edited by:
Christian E. Vincenot, Kyoto University, JapanReviewed by:
Costica Nitu, University Politehnica of Bucharest, RomaniaQing Tian, George Mason University, USA
Copyright © 2016 Drogoul, Huynh and Truong. This is an open-access article distributed under the terms of the Creative Commons Attribution License (CC BY). The use, distribution or reproduction in other forums is permitted, provided the original author(s) or licensor are credited and that the original publication in this journal is cited, in accordance with accepted academic practice. No use, distribution or reproduction is permitted which does not comply with these terms.
*Correspondence: Alexis Drogoul, YWxleGlzLmRyb2dvdWxAaXJkLmZy