- 1Life Cycle Assessment, Institute for Sustainability Sciences, Agroscope, Zurich, Switzerland
- 2ETH Zurich, Institute of Agricultural Sciences, Zurich, Switzerland
- 3Agricultural Landscapes and Biodiversity, Institute for Sustainability Sciences, Agroscope, Zurich, Switzerland
- 4Sol Agro et Hydrosystème Spatialisation (SAS), INRA, Agrocampus Ouest, Rennes, France
Mountain farming areas are associated with high nature value and offer attractive landscapes, but farming in these areas is less viable than farming in more favorable regions. Consequently, there is a threat of land abandonment. Additionally, due to lower productivity of mountain farms, their products often bear a higher environmental burden than those from other areas. An optimal division of labor between mountain farms and farms in more favorable regions based on comparative advantages could help maintain attractive landscapes and reduce environmental impacts of agricultural production. An established Swiss contract rearing system, in which dairy farms from the agriculturally favorable lowlands collaborate with heifer rearing farms in the mountains, represents a promising approach for such a division of labor. In this system, the intensive phase of dairy production is performed in the lowlands, while the less intensive phase is performed in the mountains. Here, we analyzed a sample of 16 farms to compare the contract rearing system to a situation in which both, mountain and lowland farms produce milk and rear their own restocking animals. We performed a life cycle assessment to quantify environmental impacts of the dairy production systems, assessing environmental impacts both per kg of milk and per hectare of agricultural area. This assessment was supplemented with analysis of the workload of the farms, since increased work efficiency is one reason that farmers engage in contract rearing. Workload was calculated with a workload budgeting tool. We found that collaboration reduced environmental impacts as well as the workload per kg of milk. Collaboration had no effect on environmental impacts per hectare of agricultural area or the workload on lowland farms, while on mountain farms the environmental impacts and workload were reduced. In particular, reduction in environmental impacts of mountain farms is expected to foster the high nature value of this farmland and the provisioning of important ecosystem services. This case study of a contract rearing system thus illustrates how collaboration based on comparative advantages can benefit both environmental impacts of agricultural products and the high nature value of agriculturally less favorable farmland.
Introduction
Farming activities in mountainous regions face natural constraints that inhibit high productivity. Instead, such areas are often of high nature value and feature attractive landscapes. They provide important ecosystem services, such as maintenance of genetic resources, storage, and purification of water, as well as cultural and heritage services (Grêt-Regamey et al., 2012; Plieninger and Bieling, 2013). This high nature value farmland has been shaped through traditional low-intensity agricultural systems (Lomba et al., 2014). Today, such areas are threatened by two trends: intensification and land abandonment. Land abandonment mainly results from lower economic viability (Strohbach et al., 2015). To address the reduced economic viability and prevent land abandonment, the European Union's Common Agricultural Policy (CAP) and agricultural policies in European countries outside the EU, such as Switzerland or Norway, have defined plans to support farming activities in these areas (MacDonald et al., 2000; Marriott et al., 2004; Gellrich and Zimmermann, 2007). Since environmental conditions do not allow intensive agricultural production and specific policy measures may limit high-input farming, agricultural land management in areas with natural constraints is often more environmental friendly, with lower fertilizer or pesticide use per hectare (Rudow, 2014). On the other hand, because of the lower productivity of the land, from a life cycle assessment (LCA) perspective, the provision of agricultural goods from these regions is less eco-efficient than in the lowlands. Per unit of product, foods produced in the mountains usually cause higher negative environmental impacts, such as higher global warming potential per kg milk or meat (Hörtenhuber et al., 2010; Ripoll-Bosch et al., 2013). This results in a trade-off between maintaining agricultural production to preserve scenic landscapes with high value semi-natural habitats, and providing agricultural products with a lower environmental impact.
To address this trade-off, the most suitable production systems for such areas have to be identified and, in parallel, environmental impacts of products from these systems must be optimized. An approach focusing only on comparing absolute results of production systems from favorable and less favorable regions could be too narrow, since the chances of identifying a product that is produced more efficiently in areas with natural constraints are rather low. A more promising approach is inspired by the classic economic theory of trade and comparative advantage (Deardorff, 2014). It focuses on possibilities for division of labor between regions with different natural conditions. By considering environmental impacts of a set of products, it is possible to identify those for which the disadvantage of the region with natural constraints is less pronounced than that for other products from this set, i.e., in which mountain farms have a comparative advantage. In consequence, more favorable regions will have a comparative advantage for production activities for which the disadvantages of the region with natural constraints are more pronounced. An example of division of labor between two regions with different climatic and topographic conditions can be found in Switzerland. Swiss lowland farms generally have agriculturally favorable conditions and invest in grassland-based animal production and crop production. In contrast, mountain farms are mainly restricted to grassland based systems due to steep slopes and a shorter vegetation period. Although dairy farming is practiced in both regions, mountain farms do not compete well with lowland farms. Compared to lowland farms, mountain farms have lower income (Roesch, 2012), and milk with higher environmental impacts per kg (Alig et al., 2011). One reason for the lower performance of mountain farms is the lower nutritive quality of home-grown feed, which, when given alone, is not sufficient for today's high-genetic-merit dairy cows (Horn et al., 2013). In contrast, lowland farmers often perceive their forage quality as being too high for their young stock (M. Tanner, 20 October 2015, pers. comm.).
As early as in the 1960s, farmers from the cantons of Thurgau and Grisons, Switzerland, developed a contract rearing plan that took advantage of the different production conditions on mountain and lowland farms. In this plan, dairy farmers from the lowlands sell their female dairy calves to mountain farmers, who then rear them and sell them back to the lowland farmers shortly before calving. Accordingly, the animals spend the less intensive phase of their life on mountain farms, and the more intensive phase, i.e., the productive phase, on lowland farms, which can offer feed of higher quality. The system remains popular, mainly in these two cantons, but it has spread to other regions as well. It is based on a standardized contract, and prices are renegotiated once a year by a delegation of lowland and mountain farmers. This guarantees a fair pricing system and a legal framework that makes the system easily applicable for farmers. In a previous assessment, this collaborative production system was analyzed based on simulated farms that represented typical Swiss lowland and mountain dairy farms (Marton et al., 2016b). It was shown that collaborative production had environmental advantages compared to a system in which dairy farms rear their own young stock. However, this kind of assessment with simulated farms is based on many assumptions that might not reflect the real situation. For example, it was assumed that yields and production efficiency per hectare and per cow were the same on collaborating and non-collaborating farms within each region. Thus, the analysis based on simulated typical farms considered only the benefits due to the comparative advantage of each region. However, collaboration may also affect farm efficiency, since collaboration allows farms to specialize in individual aspects of the dairy production system, e.g., milk production itself or rearing of young stock, which was not considered in simulated farms. For the present study, we tested whether the benefits of the contract rearing system observed for the simulated farms are also valid for real farms, and whether the specialization due to collaboration creates further efficiency gains and reduces the environmental impacts of milk production even more. Besides its effect on environmental impacts of milk, specialization was also expected to affect environmental impacts directly on farms. For instance, by outsourcing the less-intensive young stock and keeping only the more-intensive dairy cows, lowland farms might increase adverse environmental impacts per hectare of usable agricultural area (UAA). On mountain farms, specialization in heifer rearing could have the opposite effect, i.e., reduce environmental impacts per hectare of UAA. Furthermore, it is expected that division of labor has an impact on farmers' workload, since labor constraints are considered an incentive for contract rearing (Olynk and Wolf, 2010). In the present study, we therefore compared environmental impacts and workload of collaborative and non-collaborative dairy production using data from real commercial farms. Specifically, we tested the following hypotheses:
(1) Collaboration leads (a) to intensification and thus to higher environmental impacts per hectare of UAA on lowland farms and (b) to extensification and thus to lower environmental impacts per hectare of UAA on mountain farms. Intensification in this context is defined as an increase in inputs per ha, while extensification corresponds to a reduction in inputs per ha.
(2) Environmental impacts per kg of fat- and protein-corrected milk (FPCM) produced in the overall system is reduced through collaboration.
(3) Workload is lower on collaborating farms than on non-collaborating farms.
Materials and Methods
Farming Systems and Study Region
We compared two farming systems: collaborative (contract rearing) and non-collaborative. Both systems consisted of dairy farms in the lowlands and the mountains. In the collaborative system, lowland farms concentrated on milk production; female dairy calves designated for restocking were sold to mountain farms when weaned. Mountain farms reared the animals and sold them back to lowland farms when the heifers were close to calving. In the non-collaborative system, dairy cows spent their entire lives on the same farm, i.e., both lowland and mountain farms kept productive dairy cows and young stock for restocking.
Following the previous assessment based on simulated typical Swiss dairy farms from the lowland and mountain regions (Marton et al., 2016b), the present study focused on assessing single real farms to verify or disprove the indications obtained from the farm simulations. The farms analyzed were located in the cantons of Thurgau and Grisons, the two cantons that first adopted the contract rearing plan (Figure 1). Both cantons still have many farms that do not participate in contract rearing, which allowed comparison of the collaborative and non-collaborative systems under similar climatic and topographic conditions. Thurgau is a relatively small canton, with ~50% of its area as UAA, mostly in the lowlands, which corresponds to 4.8% of the UAA of Switzerland. Thurgau contains 6.6% of Swiss dairy cows, which produce 7.7% of the milk sold in Switzerland. Grisons is the largest Swiss canton. Due to its location in the center of the Alps, only 8% of its area is used for agriculture, corresponding to 5.2% of the UAA of Switzerland. In addition, 23% of the area of Grisons is considered alpine agricultural area. By law, this area may only be used as pasture during the summer (alpine summer-pasture). Grisons contains 2.9% of Swiss dairy cows, which produce 2.1% of the milk sold in Switzerland (TSM, 2013; Swiss Federal Statistical Office, 2016).
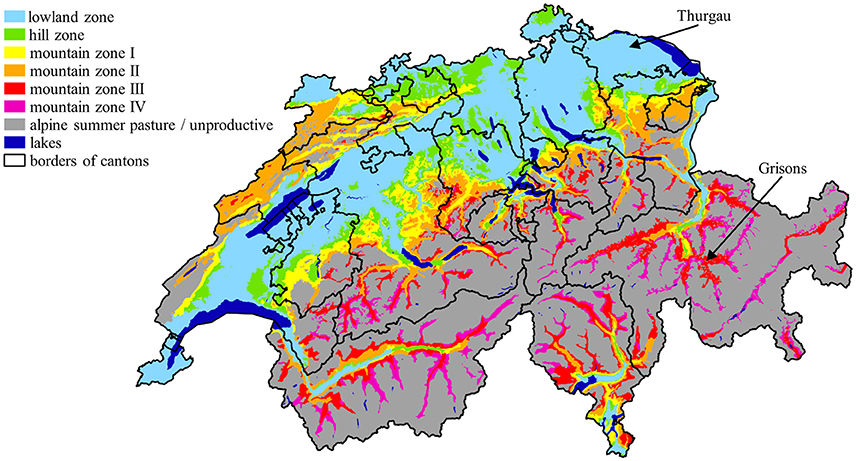
Figure 1. Map of agricultural production zones in Switzerland and locations of the cantons Thurgau and Grisons, where the studied lowland and mountain farms were situated (Federal Office for Agriculture (FOAG), 2015; swisstopo, 2016).
Farm Data and Characteristics
Sixteen dairy farms from the two cantons were assessed; Thurgau represented the lowland and Grisons the mountain region. In each region, four farms were collaborating in contract rearing (hereafter “collaborating farms”), and four farms were not participating in contract rearing (hereafter “non-collaborating farms”). Collaborating and non-collaborating dairy farms were randomly selected from those that offer apprenticeship positions. Since authorization to do so requires additional training for the farmer, we assumed that the farms in the sample were more advanced farms, i.e., farms that apply good management practices and are well-informed about new technical developments.
Data on farm characteristics and agricultural practices were collected during farm visits or provided directly by the farmers. Data collection was based on two datasets developed within the EU FP7 project CANTOGETHER. One set contained data needed for LCA (Teuscher et al., 2014), and the other contained supplementary agronomic and economic data (Regan et al., 2016). Table 1 gives an overview over some farm characteristics. Farms in the sample were larger and had more animals than the average dairy farm in Switzerland, which had (in 2012) 24 ha UAA and 23 dairy cows. Except for one mountain farm, milk yield per cow also lay above the Swiss average of 6000 kg of milk (TSM, 2013). On lowland farms, most forage was home-grown, mainly grass, and whole-crop maize (silage or dried pellets). Most concentrate was purchased; only a small percentage was home-grown. In addition to producing feed, five lowland farms also grew cash crops. Mountain farms were grassland-based, produced mostly home-grown forage, and purchased all concentrate.
All mountain farms, and one collaborating, and one non-collaborating lowland farm, sent animals to alpine summer-pasture. To represent this phase (~100 days), we collected data from two alpine summer-pasture farms in Grisons and averaged these data for further calculations.
Most farms collaborated with more than one farm from the other region: mountain farms collaborated with 3–15 lowland farms, which collaborated with 1–5 mountain farms. Since all farms were selected randomly, there was no effective link between collaborating lowland and mountain farms. To simulate collaborative dairy production among the farms in the sample, we combined each collaborating lowland farm with each collaborating mountain farm, resulting in a set of 16 combinations. Farms were combined based on the lowland farm's need for heifers and the mountain farm's production of heifers. For example, if the lowland farm needed four heifers per year and the mountain farm produced 12 heifers per year, then one-third of the mountain farm's heifer rearing enterprise was added to the lowland farm to include the outsourced restocking phase in collaborative dairy production. To compare this collaborative system to non-collaborative dairy production, we combined the non-collaborating dairy farms from the two regions so that the ratio of lowland to mountain UAA corresponded to the median land-use ratio of the two regions in the collaborative system (3.5:1). See Supplementary Tables 1, 2 for details about these combinations. Each non-collaborating lowland farm was combined with each non-collaborating mountain farm, resulting in a set of 16 combinations, which created an equal basis for comparison.
Environmental Assessment
We used LCA to compare environmental impacts of (1) farms within the two regions and (2) milk produced in collaborative and non-collaborative dairy production systems. Life cycle assessment not only considers environmental impacts occurring directly on a farm, it compiles all environmental impacts of products along the whole value chain. This is typically performed in four steps: goal and scope definition, life cycle inventory (LCI), life cycle impact assessment (LCIA), and interpretation of LCIA results (ISO, 2006; Hellweg and Milà i Canals, 2014).
Goal and Scope Definition
To compare environmental impacts within mountain and lowland regions, the functional unit was one hectare of UAA used for the dairy enterprise during 1 year. This functional unit relates to the farm's function as a provider of ecosystem services, such as maintenance of water quality (van der Werf et al., 2009). The dairy enterprise comprised all farm activities linked to dairy production, e.g., management of dairy cows and restocking animals, production of feed for these animals, and use of buildings and machinery. System boundaries were defined as “cradle to farm gate,” including all environmental impacts caused by the dairy enterprise itself and by all upstream processes linked to production and supply of inputs (e.g., fertilizers, purchased feed), infrastructure and machinery (Figure 2). Seven farms in the sample grew cash crops, which connected the cash-crop and dairy enterprises, since the by-product straw from the former was used as bedding in the latter, and some manure produced in the latter was used as fertilizer in the former. For straw, we performed economic allocation between straw and cash crops to allocate part of the cash-crop area to the dairy enterprise. To account for manure spread on areas allocated to the cash-crop enterprise, we used system expansion, since this method has previously been compared to other approaches and was identified as the most suitable to account for interactions between cash-crop and dairy enterprises (Marton et al., 2016a). All emissions related to application of manure to cash crops were attributed to the dairy enterprise, while emissions that a mineral fertilizer would have caused, as well as emissions from producing it, were credited to the dairy system. The amounts of nutrients replaced by manure applied within the cash-crop enterprise were calculated based on crop requirements and the nutrient availabilities of manure and mineral fertilizers. The amount of nitrogen (N) replaced was calculated based on the total ammonium N (TAN) in the applied manure (Flisch et al., 2009) and the ammonia-loss rates of manure and the mineral fertilizer replaced (Hutchings et al., 2009, 2013). When more N was applied to a crop than its theoretical N requirements, only the amount of N required minus the amount of N provided by other fertilizers was credited (Equation 1). We assumed that manure replaced ammonium nitrate, the mineral N fertilizer most commonly used on farms in our sample.
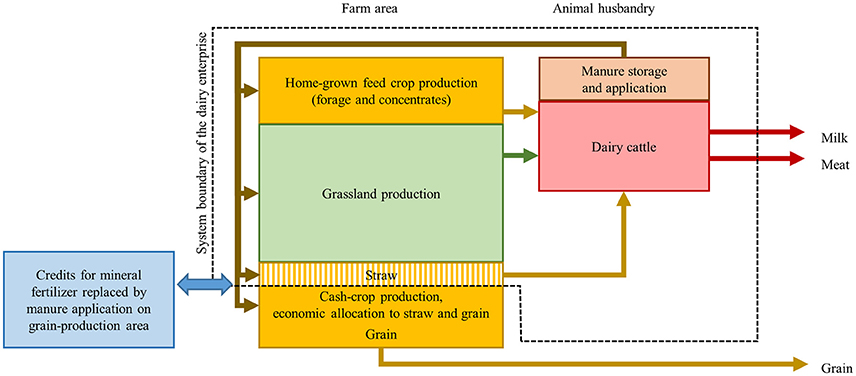
Figure 2. System boundaries of the dairy enterprise. Upstream processes and infrastructure are not illustrated.
For phosphorus (P) and potassium (K), the amount of each contained in manure was calculated to replace the same amount from mineral fertilizers, as long as it did not exceed plant requirements. If the latter was the case, only the amount of nutrients needed by the plant and not covered by other fertilizers was credited. The mineral fertilizers assumed to be replaced were triple-superphosphate and potassium chloride (KCl), respectively.
Farming systems are multifunctional, but their main function is to supply food. Therefore, the collaborative and non-collaborative dairy production systems were compared based on their main output, using the functional unit of 1 kg of FPCM. System boundaries were defined in the same way as for the assessment of the impact per hectare of UAA, i.e., considering all upstream processes and activities on the farm, up to the farm gate. Milk production is a multi-output process, and besides the manure that can be exported from the dairy enterprise (accounted for as described above), meat is also produced from culled animals and surplus calves. We followed the guidelines of the International Dairy Federation, using physical causality to allocate environmental impacts to milk and meat (International Dairy Federation (IDF), 2015).
Life Cycle Inventory
The farmers provided the main data used to calculate the LCI, which included data about yields, animal numbers, purchased inputs such as feed or energy carriers, housing infrastructure and manure management, machinery, and detailed information about field-management practices such as date, type, quantity applied, and application method for each fertilization or crop-protection event. The LCI itself was then calculated with a tool developed for the CANTOGETHER project (CANCalc, Teuscher et al., 2014). In the present study, the LCI consisted of an inventory of farm inputs, linked to upstream processes from ecoinvent v2.2 (ecoinvent Centre, 2010) and the Swiss agricultural LCA (SALCA, Nemecek et al., 2010) database, and farm outputs including direct emissions. The following tools and models were used to calculate direct emissions:
(1) A dataset of organic and mineral fertilizers, with their nutrient contents and availability to plants, based on data from Flisch et al. (2009), Nemecek and Kägi (2007) and fertilizer producers.
(2) A tool developed by project partner SP Technical Research Institute of Sweden (formerly SIK) to predict emissions from enteric fermentation, manure management and application (Berglund and Cederberg, 2014).
(3) The SPACSYS (soil-plant-atmosphere continuum system) model, version 5.1 (Wu et al., 2015), to predict N leaching and runoff, and nitrous oxide (N2O) emissions.
(4) The Universal Soil Loss Equation (Renard et al., 2011) to predict soil erosion.
(5) Elements of the SALCA to predict heavy-metal entry into soil and ammonia emissions from mineral fertilizers.
The LCI was calculated for the entire farm and for its individual farm enterprises, according to the system boundaries defined. Inputs and emissions were grouped into 12 categories: buildings and equipment, machinery, energy carriers, fertilizers and field emissions, pesticides, purchased seeds, purchased concentrate, purchased roughage, purchased animals, animal husbandry, other inputs, and summer pasture. The last category is special, since it comprises all inputs and emissions that occur during the summer-pasture phase, without distinguishing their exact sources. These emissions are mainly caused by the animals, either due to enteric fermentation (corresponding to the category “animal husbandry”) or due to excretion directly on the area (corresponding to the category “fertilizers and field emissions”).
For the credits related to manure application within the cash-crop enterprise, two LCI were calculated, one with manure application, and one in which the mineral fertilizers that had been replaced were applied instead of manure (Equation 1). This was necessary because direct emissions linked to N fertilization, such as nitrate leaching into water or ammonia emissions to the air, are influenced by the type of N source applied. For example, manure has higher ammonia emissions than ammonium nitrate (Hutchings et al., 2009, 2013). If the inventory with manure application had higher direct emissions, the difference was attributed to the dairy enterprise, but if it had lower direct emissions, the difference was credited to the dairy enterprise.
Life Cycle Impact Assessment
We considered impact categories related to three aspects: (1) emissions into the ecosphere, i.e., into the air, soil, or water, (2) land use, and (3) resource use. The impact categories related to emissions into the ecosphere are linked to specific environmental challenges, such as climate change, and thus cover rather narrow topics. Impact categories related to land use are linked to the scarcity of land as a resource, but also to the impact that use of this land could have on biodiversity. The resource-related impact categories, as used in this study, are indicators of both depletion of scarce resources and efficiency of production systems. From these three impact category groups, the following categories were assessed:
• Categories related to impacts caused by emissions into the ecosphere
◦ Acidification (EDIP2003, Hauschild and Potting, 2005).
◦ Ecotoxicity, terrestrial (CML2001, Guinée et al., 2001).
◦ Eutrophication due to N (EDIP2003, Hauschild and Potting, 2005).
◦ Eutrophication due to P (EDIP2003, Hauschild and Potting, 2005).
◦ Global warming potential over 100 years (IPCC2013, Myhre et al., 2013).
◦ Ozone depletion (EDIP2003, Hauschild and Potting, 2005), with the addition of the ozone depletion potential of N2O according to Ravishankara et al. (2009).
• Categories related to land use
◦ Deforestation (Frischknecht et al., 2007a).
◦ Land competition (Frischknecht et al., 2007a), excluding alpine summer-pasture land use.
◦ Alpine summer-pasture land use.
◦ Biodiversity on the farm area (Jeanneret et al., 2014).
• Categories related to resource use
◦ Non-renewable energy demand, fossil and nuclear (Frischknecht et al., 2007a).
◦ Resource use, P extraction (based on elementary flow from ecoinvent 2.2, Frischknecht et al., 2007b).
◦ Resource use, K extraction (based on elementary flow from ecoinvent 2.2, Frischknecht et al., 2007b).
◦ Water use (Frischknecht et al., 2007a).
The impact category “ozone depletion potential” was adapted for this study, since currently available life cycle impact assessment methods do not consider the ozone depletion potential of N2O, although it is currently considered the most important ozone-layer-depleting substance (UNEP, 2013). Ravishankara et al. (2009) were the first to publish an ozone depletion potential for N2O: 0.017 kg CFC-11 equivalents per kg N2O. This ozone depletion potential is valid at present, but future change in gas composition in the stratosphere, namely chlorine, CO2 and CH4, may increase the ozone depletion potential of N2O (Revell et al., 2015). We therefore considered the factor of 0.017 as robust enough to be used in our context. For comparison of results with and without consideration of N2O, see Supplementary Figure 1.
The impact category of land competition was also adapted for the purpose of the present study. Alpine summer-pasture land use was treated separately, since it does not compete with other anthropogenic land-use types. The area is not suitable for other agricultural practices and is too remote for other land-use purposes such as urban or industrial use. It does, however, compete with the natural vegetation that would be found on these areas if they were not used as summer pastures. In Europe, alpine summer-pasture areas have declined, and if abandoned, shrubs and forests encroach on them (Anthelme et al., 2001). Although LCA studies usually recommend minimizing land use, other research results indicate that conservation and use of summer pasture is beneficial, especially for biodiversity (Pornaro et al., 2013; Koch et al., 2015). In the present study, more alpine summer-pasture land use was thus considered as beneficial. Similar to land competition, alpine summer-pasture land use is expressed as the area occupied multiplied by the duration of the occupation (square meter years—m2a).
The different impact assessment methods were applied for both functional units, per hectare of UAA and per kg of FPCM, except for biodiversity. Compared to the other LCIA methods used, the method to assess biodiversity is a special case. It relies on estimates of effects of various agricultural land use types (i.e., arable crops, grasslands, semi-natural habitats) and agricultural practices (e.g., plowing, pesticide application, date of first cut in grasslands) on local biodiversity. The LCI provided the data required to apply the biodiversity model and estimate scores for overall species diversity. The model considers the suitability of land use types and the severity of agricultural practices on 11 indicator species groups related to farmland. From the individual scores for each species group, the overall species diversity score is calculated. High overall species diversity scores indicate that a system is beneficial for biodiversity (Jeanneret et al., 2014). Because the method focuses on a farm's agricultural area, its system boundaries exclude upstream processes. Also, since it uses scores instead of quantitative units, these scores cannot be attributed to single products; thus, biodiversity was assessed only per hectare of UAA.
Workload Assessment
Workload related to dairy production was calculated for each farm with the workload budgeting tool ART-AV 2014 (Stark et al., 2014). The tool considers the crop and grassland fields on the farm, and animal numbers of several livestock categories, such as calves, heifers, dairy cows, fattening cattle and pigs. It also considers economies of scale, for instance assuming lower workloads per hectare for larger areas of the same crop produced on a given farm. Using the same allocation rules as for the LCA, we calculated the workload for the dairy enterprise, expressed as hours per hectare of UAA, as well as the hours needed to produce 1 kg of FPCM.
Statistical Tests and Sensitivity Analyses
Differences between collaborative and non-collaborative dairy production systems and between collaborative and non-collaborative farms within each region for the environmental impact categories and workload indicators were tested for significance with a one-sided Mann-Whitney U-test. Comparison of the dairy production systems considered two groups with 16 data points each, a number of observations that is large enough to provide meaningful results. Comparison of the farms within a given region, however, had only two groups with 4 data points each. A one-sided test with such a small sample size will result in a p < 0.05 only if one group contains the first four ranks or the first three and the fifth rank. Since these cases are rare, we therefore also considered tendencies, defined as p ≤ 0.10, when comparing farms within a given region. A value of p = 0.10 would correspond to a situation in which the three best-performing farms are in one group and the worst-performing farm is in the other group, i.e., one group contains ranks 1, 2, 3, and 7, and the other 4, 5, 6, and 8.
Given the sample size, the sample farms do not necessarily represent the entire population of collaborative and non-collaborative dairy farms in Switzerland. For example, while more than 50% of all farms in Grisons are organic (Swiss Federal Statistical Office, 2016), none of the non-collaborating farms in the Grisons sample was organic. Two of them, however, used no mineral fertilizers or pesticides, and thus differed from organic farms only in the type of concentrate purchased. We therefore performed a sensitivity analysis in the comparison of farms, in which these two farms were virtually converted into organic farms by replacing purchased conventional concentrate with organic concentrate. Another sensitivity analysis was performed for credits for mineral fertilizer replaced by manure applied to cash crops. In it, we applied an allocation procedure based on a cut-off principle: all emissions from manure storage were allocated to the dairy enterprise, while those from manure application outside of the dairy enterprise were allocated to the cash-crop enterprise. This allocation procedure corresponds to that in ecoinvent v2.2 (Nemecek and Kägi, 2007) and is also recommended in the IDF's current LCA guidelines, while in the previous version the IDF recommended crediting mineral fertilizers that are replaced (International Dairy Federation (IDF), 2010, 2015).
Results
Environmental Impacts
On lowland farms, environmental impacts per hectare of UAA of collaborating and non-collaborating farms did not differ significantly, not even in tendency (Figures 3–5). In the mountains, collaborating farms had significantly lower environmental impacts for terrestrial ecotoxicity, eutrophication due to N, deforestation, land competition, non-renewable energy demand, P and K resource use, and a tendency for lower emissions for acidification and water use. In addition, there was a tendency for higher alpine summer-pasture land use and higher biodiversity on collaborative mountain farms than on non-collaborative mountain farms.
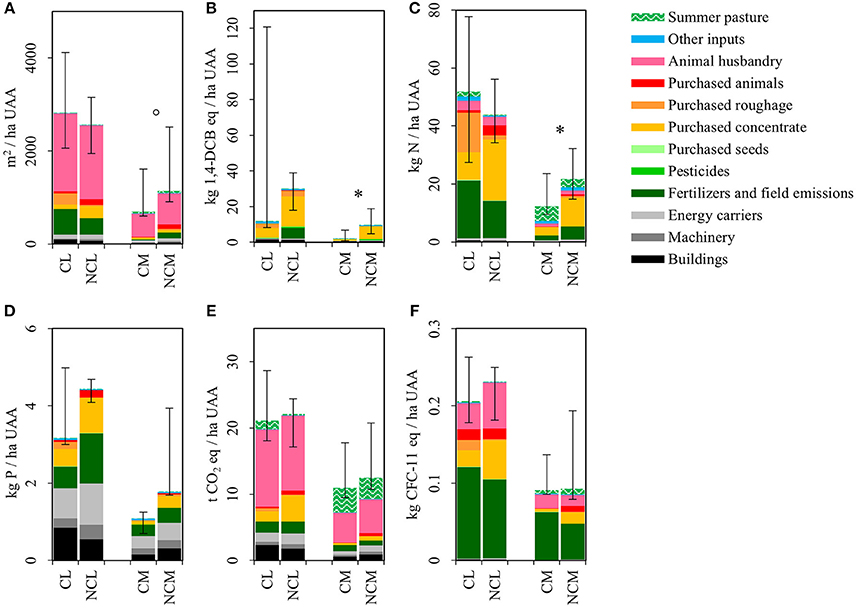
Figure 3. Environmental impact per hectare usable agricultural area (ha UAA) for impact categories related to emissions into the ecosphere: (A) acidification, (B) terrestrial ecotoxicity, (C) eutrophication due to N, (D) eutrophication due to P, (E) global warming potential, (F) ozone depletion potential. Bars indicate the median performance of each farm group (CL, collaborating lowland farms; NCL, non-collaborating lowland farms; CM, collaborating mountain farms; NCM, non-collaborating mountain farms) and sources of impacts. Whiskers indicate the minimum and maximum impact within each group, *indicate significant differences between farm groups within a given region (p < 0.05), °indicate tendencies (p ≤ 0.1).
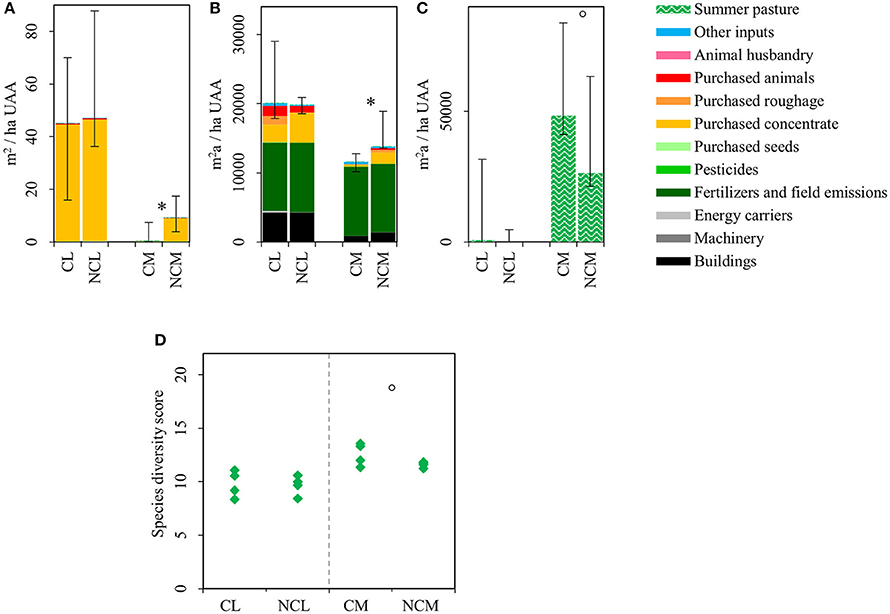
Figure 4. Environmental impacts per hectare usable agricultural area (ha UAA) for impact categories related to land use. Top: (A) deforestation, (B) land competition, (C) alpine summer-pasture land use. Bars indicate median performance of each farm group and sources of impacts. Whiskers indicate minimum and maximum impacts within each group (CL, collaborating lowland farms; NCL, non-collaborating lowland farms; CM, collaborating mountain farms; NCM, non-collaborating mountain farms). Bottom: (D) Biodiversity of the UAA expressed as overall species diversity scores, *indicates significant differences between farm groups within the same region (p < 0.05), and °indicates tendencies (p ≤ 0.1).
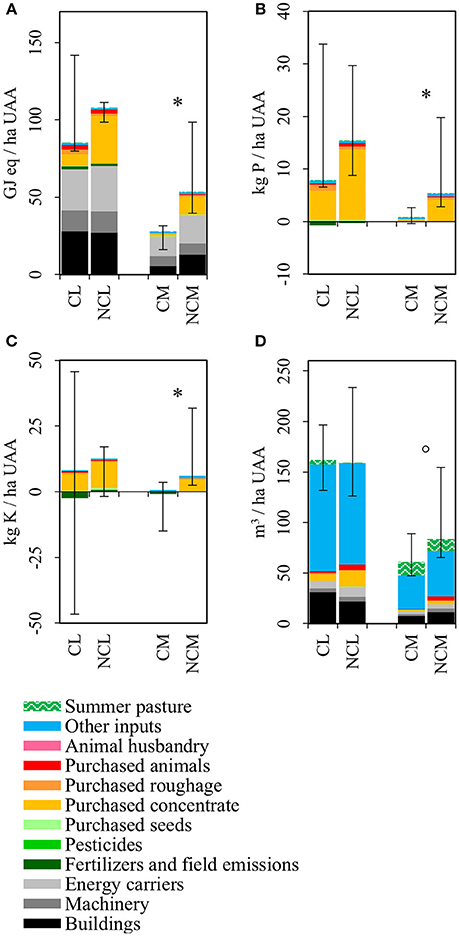
Figure 5. Environmental impact per hectare usable agricultural area (ha UAA) for impact categories related to resource use: (A) non-renewable energy demand, (B) resource use, P extraction, (C) resource use, K extraction, (D) water use. Bars indicate the median performance of each farm group (CL, collaborating lowland farms; NCL, non-collaborating lowland farms; CM, collaborating mountain farms; NCM, non-collaborating mountain farms) and sources of impacts. Whiskers indicate the minimum and maximum impact within each group, *indicate significant differences between farm groups within a given region (p < 0.05), °indicate tendencies (p ≤ 0.1).
In both regions, variability in environmental impacts within a group of farms was often higher than differences between the groups. This was most prominent for ecotoxicity of collaborating lowland farms (Figure 3B), one of which had much higher emissions than non-collaborating lowland farms. The high ecotoxicity on this farm was due to a relatively large amount of potatoes in the feed ration, and potato production was linked to high pesticide use. Two further impact categories with high variability were P and K resource use (Figures 5B,C), which both depended highly on the fertilizer strategy and the crops grown on individual farms. Negative impacts (i.e., a positive effect on the environment) resulted from credits due to manure applied to cash crops. Two of the collaborating lowland farms grew sugar beets, a crop with high K demand. Application of manure on this crop led to high credits, which in one case were much higher than the K resource use linked to the inputs of the farm's dairy enterprise. In contrast, one collaborating lowland farm had relatively high K resource use, since it was the only lowland farm that applied KCl to grassland. The same was true for one non-collaborating mountain farm, which applied KCl to both grassland and maize.
Per kg of FPCM, collaborative production caused lower or equal environmental impacts compared to non-collaborative production (Figure 6), except for alpine summer-pasture land use, for which collaborative production used more area. Differences were significant for terrestrial ecotoxicity, eutrophication due to P, ozone depletion, deforestation, alpine summer-pasture land use, non-renewable energy demand, P resource use, and water use. As for individual farms, variability among farm combinations was high. For some farm combinations, the credits attributed to the dairy enterprise due to manure applied to cash crops led to negative K resource use, especially when two farms were combined that both had a negative K resource use.
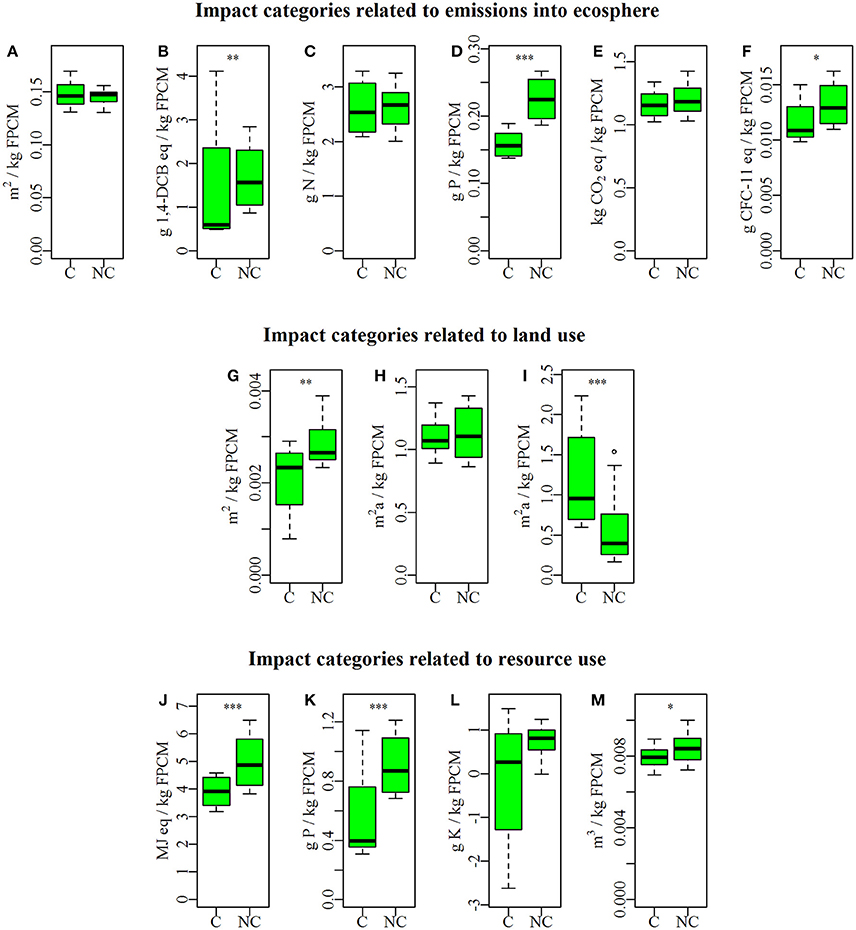
Figure 6. Environmental impacts per kg of fat- and protein-corrected milk (FPCM) from collaborative (C; n = 16) and non-collaborative (NC; n = 16) production systems for impact categories (A) acidification, (B) terrestrial ecotoxicity, (C) eutrophication due to N, (D) eutrophication due to P, (E) global warming potential, (F) ozone depletion potential, (G) deforestation, (H) land competition, (I) alpine summer-pasture land use, (J) non-renewable energy demand, (K) resource use, P extraction, (L) resource use, K extraction, and (M) water use. Whiskers indicate 1.5 times the standard deviation, and asterisks indicate significance level (*p < 0.05, **p < 0.01, ***p < 0.001).
Sensitivity Analyses
In the first sensitivity analysis (two non-collaborating mountain farms virtually converted to organic farms), impacts for terrestrial ecotoxicity, deforestation, and P and K resource use decreased (Table 2). Nonetheless, non-collaborating mountain farms still had significantly higher impacts for eutrophication due to N, land competition, non-renewable energy demand, and a tendency for higher impacts for acidification, ecotoxicity, and water use. In contrast, deforestation was no longer significantly higher on non-collaborating farms. For P and K resource use, the formerly significant differences were reduced, but a tendency for higher use of these elements on non-collaborating mountain farms remained. Per kg of FPCM, the conversion had no substantial influences. Differences between collaborative and non-collaborative production remained significant for terrestrial ecotoxicity, eutrophication due to P, ozone depletion, deforestation, alpine summer-pasture land use, non-renewable energy demand, P resource use, and water use.
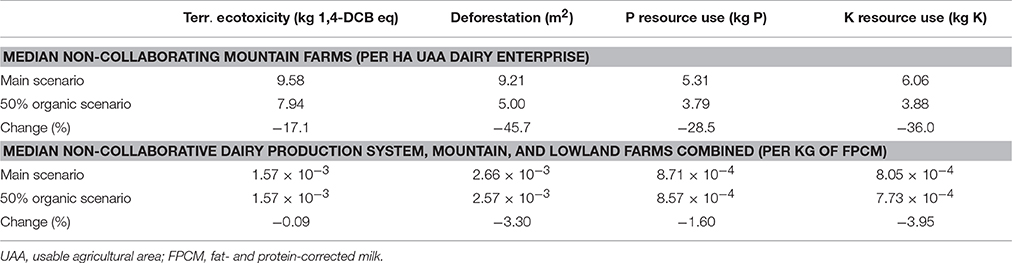
Table 2. Sensitivity analysis: conversion of two non-collaborative mountain farms into organic farms (50% organic scenario) compared to the original scenario, in which all non-collaborative mountain farms were conventional farms (main scenario) for the impact categories affected the most by this change (terrestrial ecotoxicity, deforestation, P resource use, K resource use).
The second sensitivity analysis concerned allocation of emissions from manure that was applied outside of the dairy enterprise, i.e., on cash crops (credits or cut-off approach). The choice of allocation method influenced mainly the results for P and K resource use (Table 3). For farms that applied manure outside of the dairy enterprise, P and K resource use of the dairy enterprise was higher under the cut-off approach. However, the choice of allocation method affected only absolute results and not differences between farms within a given region. In the lowlands, differences between collaborating and non-collaborating farms remained insignificant. In the mountains, collaborating farms still had significantly lower P and K resource use per ha UAA than non-collaborating farms. Per kg of FPCM, on the other hand, the choice of allocation method influenced differences in K resource use: under the cut-off approach, differences between collaborative and non-collaborative production were no longer significant. For the other impact categories, the choice of allocation method did not influence the significance of differences between collaborative and non-collaborative production.
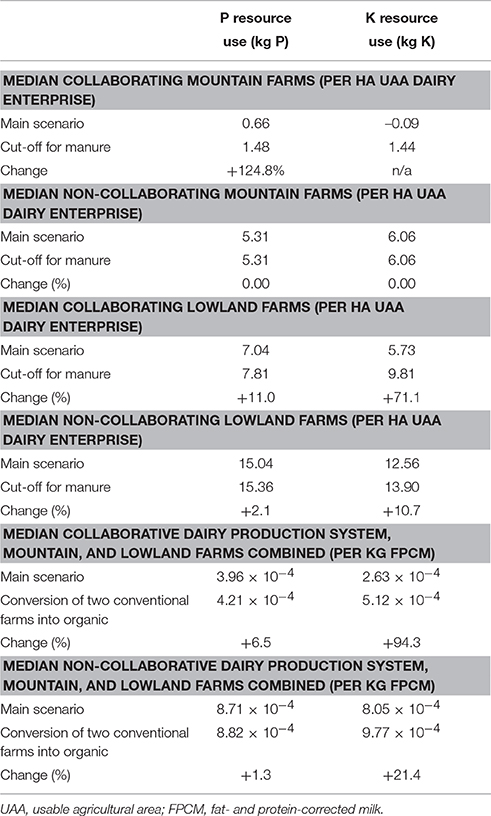
Table 3. Sensitivity analysis: cut-off approach for manure applied outside of the dairy enterprise, in which emissions from manure application lay outside of the scope of the dairy enterprise (cut-off for manure), compared to the original scenario, in which credits for mineral fertilizers replaced by manure were applied (main scenario) for the impact categories affected the most by this change (P resource use, K resource use).
Workload
In the lowlands, collaborating farms had slightly higher (but not significantly so) median workload per hectare of UAA than non-collaborating farms. In the mountains, however, collaborating farms had significantly lower workload per hectare of UAA (median of 64 h/ha UAA) than non-collaborating farms (median of 134 h/ha UAA). Workload per kg of FPCM was significantly lower for collaborating farms (Figure 7).
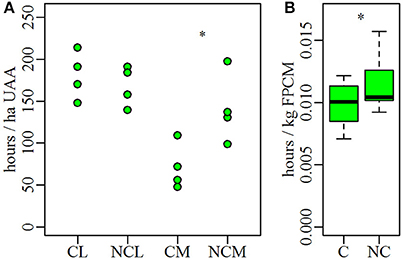
Figure 7. Comparison of workload (A) per hectare usable agricultural area (ha UAA) of the dairy enterprise on collaborating lowland (CL, n = 4), non-collaborating lowland (NCL, n = 4), collaborating mountain (CM, n = 4), and non-collaborating mountain (NCM, n = 4) farms; and (B) per kg fat- and protein-corrected milk (FPCM) in collaborative (C, n = 16) and non-collaborative (NC, n = 16) dairy production systems. Whiskers indicate 1.5 times the standard deviation, and *indicates significant differences between farm groups (p < 0.05).
Discussion
Effects of Collaboration on Environmental Performance of Lowland Farms
We hypothesized that collaborative production would lead to intensification of lowland farms due to outsourcing of the less-intensive heifer rearing activity and an increase in environmental impacts per hectare of UAA. Overall, this hypothesis was not confirmed. There were no significant differences between the collaborating and non-collaborating farms. Variability of the results within each group was higher than differences between the two groups. Concentrate use explained most of the differences in environmental impacts among lowland farms. For most impact categories, differences between individual farms were strongly influenced by the amount of concentrate purchased, although concentrate was not always the main contributor to environmental impacts in absolute terms. For example, enteric fermentation from animals (animal husbandry) contributed most to global warming potential, but it varied little among farms. Concentrate contributed only moderately to global warming potential but had high variability, making it responsible for differences among farms.
Livestock density (expressed as livestock units/ha UAA) was similar for both collaborating and non-collaborating farms, but most animals on collaborating farms were dairy cows, which usually depend more on external inputs such as concentrate. However, concentrate use per hectare was not higher on collaborating than on non-collaborating farms. Per cow, three of the collaborating farms used less concentrate than any of the non-collaborating farms, while achieving similar milk yields. These farms were able to produce more milk with home-grown feed, which indicated higher quality or more efficient use of home-grown forages. We presume that this was an effect of farm specialization, which reduces complexity of farm management, often increasing efficiency (Kingwell, 2011). More efficient use of farm resources may decrease environmental impacts. On the other hand, one of the four collaborating lowland farms used more concentrate per cow than any other farm in the sample and it was also the farm with the highest milk yield per cow. Consequently, it had the highest impacts per hectare of UAA for most of the impact categories studied. Our findings indicate that collaboration does not necessarily lead to intensification on lowland farms. It depends more on other farm strategy decisions whether a farm intensifies or not.
Effects of Collaboration on Environmental Performance of Mountain Farms
For mountain farms, we hypothesized that due to reduced intensity, collaborating farms would have lower environmental impacts per hectare of UAA than non-collaborating farms. Our results supported this hypothesis. But a lower intensity was not the only reason for the better environmental performance of collaborating farms; organic farming led to additional benefits. Organic production is relatively common for collaborating mountain farms, since a special exception in the directive for organic production allows them to purchase animals from conventional farms in the case of contract rearing (Bio Suisse, 2016). Since collaborating farms rely on relatively low amounts of external inputs, conversion to an organic farm has relatively low cost. In addition, organic farms receive higher subsidies, making organic farming more attractive.
On the other hand, organic farming was also a source of uncertainty in the present study. This farming practice was well-represented in the sample of collaborating mountain farms but underrepresented in the sample of non-collaborating mountain farms. The sensitivity analysis showed that organic farming practices influenced impacts of mountain farms, especially those related to mineral fertilization (P and K resource use), pesticide application (terrestrial ecotoxicity) and concentrate use (deforestation). Accordingly, for these impact categories, net differences between the population of collaborating and non-collaborating mountain farms in Switzerland may be smaller than apparent from the sample of farms investigated. For deforestation, virtual conversion of the two conventional non-collaborating farms into organic farms reduced impact to the point that non-collaborating farms no longer differed from collaborating farms. This was due to only one feed ingredient: soybean meal. Soybean meal from Brazil is considered to be the main source for deforestation in conventional concentrate. The organic concentrate used in this study from the SALCA database (Nemecek et al., 2010) contained no soybean meal from deforested areas. In contrast, the conventional concentrate included soybean meal from the global market and thus also from Brazil. Consequently, use of organic concentrate led to much lower deforestation. However, this result is valid only as long as indirect land-use change is excluded from the assessment (Meyfroidt et al., 2013), since land competition itself did not decrease in the scenario with more organic farms.
Alpine Summer-Pastures
Mountain farms generally practice more alpine summer-grazing, but due to the steep slopes and relatively poor feed quality on these pastures and the higher feed requirements of today's dairy cows, the traditional practice of summer pasture is becoming less attractive to dairy farmers (Penati et al., 2011). It is therefore not surprising that collaborating mountain farms used more summer pastures than non-collaborating mountain farms, since it is still convenient to use these pastures for less demanding animals. In this study, we considered greater use of these areas as a benefit, especially for biodiversity. Still, the effective biodiversity value of summer pasture also depends on its management. The biodiversity assessment method used in the present study (Jeanneret et al., 2014) considers management practices at the farm level, but so far it is not applicable to summer pasture. Therefore, we can discuss effects of possible changes in summer-pasture management only qualitatively. Alpine summer-pasture land use by non-collaborating and collaborating mountain farms differs in the type of animals they send to summer pasture, the former sending both dairy cows and heifers, the latter sending almost only heifers. Since cows and heifers may differ in their grazing patterns, the question arises whether dairy cows or heifers are best suited to maintain optimal summer-pasture vegetation. In the study of Homburger et al. (2015), dairy cows showed different grazing and land-use patterns than suckler beef cows. Dairy cows tended to avoid steeper slopes more than suckler beef cows did. During the night, dairy cows were housed either in a shed or on a small paddock near the farm buildings where animals were milked, while suckler beef cows were kept on the same paddocks as during the day. Homburger et al. (2015) did not study heifers, but we expect that they behave more like suckler beef cows than dairy cows. Since suckler beef cows and heifers are not milked, they can be sent to summer pastures without the need to keep them close to farm buildings. Because they weigh less, heifers are also more suited to steeper slopes and cause less treading damage than heavier animals (Greenwood and McKenzie, 2001). We therefore expect that heifers are better suited to management that optimizes and maintains high biodiversity on summer pasture; thus, collaborative mountain farms have not only greater, but potentially more beneficial, use of summer pastures.
Effect of Collaboration on Environmental Impacts per Kg of Fat- and Protein-Corrected Milk
We hypothesized that collaborative production would reduce environmental impacts per kg of FPCM. This was supported by the LCA results and confirmed the tendencies already observed at the farm level. Our results were also consistent with those of a previous assessment based only on simulated farms, for which collaborative production was calculated to have lower non-renewable energy demand and lower resource use both for P and K (Marton et al., 2016b). Compared to those of the simulated farms, the differences found in the present assessment of real farms were larger. We assume that the real farms not only benefited from effects of the principle of comparative advantage observed under farm simulations but were also able to improve the system further by increasing efficiency gains via specialization, as observed for the collaborating lowland farms.
Still, our assessment is based on a small and possibly biased sample. We identified two possible causes for bias. First, none of the non-collaborating mountain farms was organic. At the farm level, virtually converting half of them into organic farms reduced certain impacts of the group, but not enough to influence results per kg of FPCM. This was because mountain farms in the non-collaborative system contributed only 11% of total milk production, due to two reasons: the ratio of lowland to mountain land use was set to 3.5:1 (the median ratio in the collaborative system), and mountain farms produced less milk per ha than lowland farms. The second possible bias came from sampling only dairy farms that offered apprenticeship positions. To hire apprentices, farmers need to have additional training. We assume that most farmers who are willing to help train the next generation are more motivated to keep themselves updated about agricultural developments. If so, their farms may have above-average environmental performance. In any case, direct comparison with other LCA studies is always challenging, since system boundaries and allocation procedures vary, as do the methods used to calculate direct emissions (de Vries and de Boer, 2010). Therefore, we have no evidence for the magnitude of bias in the samples. If bias does exist, it is reasonable to assume that it affects all samples equally and thus does not change the conclusions drawn.
Effects of Collaboration on Workload
Our hypothesis that collaboration would reduce workload was partially confirmed. The reduction in workload on collaborating mountain farms was the most distinct, since heifers require less labor to care for than dairy cows. On lowland farms, the most important influences on workload were economies of scale, which were considered by the workload estimation tool. By outsourcing heifers, collaborating lowland farms increased the number of their dairy cows, obtaining economies of scale. However, non-collaborating dairy farms from our sample tended to be larger. Consequently, they kept more dairy cows than collaborating dairy farms and thus could also profit from economies of scale. The effect of larger size was at least as beneficial to non-collaborating farms as the effect of having fewer animal categories was to collaborating farms. Our results confirm those of Regan et al. (2016), who analyzed workload based on self-declaration by farmers in the same farm sample. Their approach considered all farming activities combined (i.e., dairy and cash-crop enterprises), since it was not possible for farmers to indicate workload for each enterprise on the farm, but this likely had no major influence on the results.
Combining workloads of farms from the two regions, collaboration decreased workload per kg of FPCM. This was caused mainly by lower workload on mountain farms under collaboration, but also by higher output of milk per ha UAA on lowland farms under collaboration. Like environmental impacts, higher work efficiency in the collaborative production system was assumed to be a combination of benefits from focusing on an activity with comparative advantage and from specialization.
Contribution of the System to Preservation of High Nature Value Farmland in the Mountain Region
The contract rearing system increased eco- and work-efficiency of dairy production in our sample, but the question remains if it also helps to preserve high-nature-value farmland in the mountains. High nature value farmland in the mountains is threatened by two developments: intensification and abandonment. Both result mostly from the lower economic viability of traditional mountain farming systems (Strohbach et al., 2015). Regarding the intensification issue, replacing dairy cows with heifers would maintain agricultural use and, at the same time, reduce intensity of farmland use. Land on collaborating mountain farms was managed less intensively than that on non-collaborating mountain dairy farms, since the former used fewer external inputs and had a lower stocking rate. This low-input farming system thus corresponds more to the relatively low intensity of traditional farming systems in the mountain area, which shaped the mountain farming area's high nature value.
An important constraint, however, is that contract rearing alone might not be sufficient to prevent farmers from abandoning their land, because it would reduce not only the workload of mountain farms but also the income. Therefore, compensating the income loss with off-farm labor would be necessary to make the system viable (Marton et al., 2016b). In Switzerland, off-farm labor usually pays better than on-farm labor (Hoop and Schmid, 2014; Swiss Federal Statistical Office, 2015), but jobs are not always available in the remote mountain regions. The sampled contract rearing mountain farms effectively gained a large percentage of their family income from off-farm labor. Furthermore, and in contrast to results from simulated farms, these farms also managed to achieve incomes that were at the same levels as those of non-collaborating mountain dairy farms (Regan et al., 2016). Collaboration decreased the risk of land abandonment due to economic reasons for the farms in the sample, since it provided farmers with a reasonable income.
The contract rearing system can keep mountain areas in production and may even increase the high nature value of mountain farmland through its less intensive management. Since high-nature-value farming provides provisioning, regulating and cultural ecosystem services (Plieninger and Bieling, 2013), the system is also expected to contribute to the important role of mountainous regions in providing such services.
From the Cantonal to National Level
The sample farms in this study were located in Thurgau and Grisons, since the system is most popular in these two cantons, but farms in other cantons also practice contract rearing. The contract rearing mountain farms in the sample collaborated not only with lowland farms from Thurgau, but also from Lucerne, Aargau and Zurich. The collaborating lowland farms in the sample cooperated either with farms from Grisons or St. Gall. In most cantons, agricultural extension services offer a brokering service for farmers to facilitate collaboration (Agridea, 2016). Whether results based on data from farms in only two cantons are valid for the entire country depends on the degree to which they represent Switzerland's lowland and mountain zones. Classification of Switzerland into mountain, hill and lowland zones is based on climatic and topographic characteristics (Federal Office for Agriculture, 2016). Production conditions for farms from Thurgau and Grisons should therefore be similar to those of farms in other lowland and mountain regions, respectively. Furthermore, results from the present study were in line with those from the study with simulated average Swiss farms (Marton et al., 2016b). It is therefore probable that the benefits observed for the sample farms are also valid for farms from other cantons of Switzerland. Still, it is unlikely that the system will be extended to all Swiss mountain dairy farms. Even if all lowland farms outsourced their young stock to mountain farms, the demand for contract rearing would involve only approximately one-third of all mountain dairy farms (Marton et al., 2016b). The resulting reduction in mountain milk production would most likely not affect production of traditional mountain cheese, since farms producing milk designated for cheese production receive an extra price premium (Finger et al., 2015), which acts as an incentive to continue dairy production. Farms that produce milk designated for other purposes and not marketed as a mountain product are more likely to abandon milk production.
Conclusion
Using the example of a contract rearing system between farms in a favorable region and a region with natural constraints, we demonstrated how collaboration can help decrease environmental impacts of agricultural production while keeping the areas with natural constraints productive and thus preserve these high nature value areas. We identified two effects responsible for the improvement. The first was associated with comparative advantages, in which each collaboration partner focused on an activity with lower opportunity costs. The collaborative system allowed both mountain and lowland farms to concentrate on the phase within the life of a dairy cow that corresponded best to the resources available on their land. The steeper land and lower energy content of mountain grass can fulfill the needs of young stock, while lowland farms can produce high-quality grass, other forages such as maize, and the concentrate needed to meet the higher energy requirements of lactating dairy cows. The second effect responsible for lower impacts under collaboration was specialization. Specialization can reduce management complexity of farms, and focusing on fewer activities helps to increase the skills necessary to perform them. Limitations of our study included the relatively small sample size and the potential overrepresentation of more advanced dairy farms. Further, research is needed to test whether the effects also apply to average dairy farms. We are confident, however, that reduction in management complexity will be especially beneficial for more average collaborating farms. Furthermore, we believe that this example of contract rearing involving favorable and less favorable regions could encourage development of other collaborative production systems. It would be of great interest to study the applicability of this principle to other agricultural production systems with a regional division of labor or to other geographic and climatic regions.
Author Contributions
SM is the corresponding author and main researcher. GL performed the biodiversity assessment. MC performed some of the LCA calculations. MK is project collaborating partner and supervisor. GG is project leader and co-supervisor. GL, MC, MK, and GG contributed to finalizing the manuscript.
Conflict of Interest Statement
The authors declare that the research was conducted in the absence of any commercial or financial relationships that could be construed as a potential conflict of interest.
Acknowledgments
We wish to express our gratitude to the farmers who provided the data for our assessment. We also thank Rémy Teuscher, Peter Koch, Hisko Baas, and Jens Lansche for the development of the CANTOGETHER LCA tools, and Lianhai Wu for his support with SPACSYS. We would also like to thank Daniel U. Baumgartner for his support and proofreading of the manuscript, and Thomas Nemecek for his methodological advice during the project. This work was funded under the EU Seventh Framework Program by the CANTOGETHER project (no. 289328): Crops and ANimals TOGETHER. The views expressed in this work are the sole responsibility of the authors and do not necessarily reflect the views of the European Commission.
Supplementary Material
The Supplementary Material for this article can be found online at: http://journal.frontiersin.org/article/10.3389/fenvs.2016.00074/full#supplementary-material
Abbreviations
CFC-11, Trichlorofluoromethane; FPCM, Fat and protein corrected milk; IDF, International Dairy Federation; KCl, potassium chloride; LCA, Life cycle assessment; LCI, Life cycle inventory; LCIA, Life cycle impact assessment; SALCA, Swiss agricultural life cycle assessment; TAN, Total ammonia nitrogen; UAA, Usable agricultural area.
References
Agridea (2016). Erläuterungen zum Aufzuchtvertrag – Anhang 2016 (German Version); Contrat d'élevage et de rachat (French Version). Available online at: https://www.agridea.ch/en/publications/publication/animal-production/cattle/contrat-delevage-et-de-rachat/ (Accessed August 26, 2016).
Alig, M., Baumgartner, D. U., Mieleitner, J., and Nemecek, T. (2011). “Environmental impacts of Swiss milk production in the mountain region,” in 16th Symposium of the European Grassland Federation, eds E. M. Pötsch, B. Krautzer, and A. Hopkins. (Gumpenstein, AT: Wallig Ennstaler Druckerei und Verlag). Available online at: http://www.europeangrassland.org/fileadmin/media/EGF2011.pdf (Accessed April, 2 2016).
Anthelme, F., Grossi, J.-L., Brun, J.-J., and Didier, L. (2001). Consequences of green alder expansion on vegetation changes and arthropod communities removal in the northern French Alps. Forest Ecol. Manag. 145, 57–65. doi: 10.1016/S0378-1127(00)00574-0
Berglund, M., and Cederberg, C. (2014). SIKtool-EFM–Enteric Fermentation and Manure Management. Borås: SIK–The Swedish Institute for Food and Biotechnology.
Bio Suisse (2016). Richtlinien für die Erzeugung, Verarbeitung und den Handel von Knospe-Produkten. Available online at: http://www.bio-suisse.ch/media/VundH/Regelwerk/2016/DE/rl_2016_d_auszug_ii_14.12.2015.pdf (Accessed June 9, 2016).
Deardorff, A. V. (2014). Local comparative advantage: trade costs and the pattern of trade. Int. J. Econ. Theory 10, 9–35. doi: 10.1111/ijet.12025
de Vries, M., and de Boer, I. J. M. (2010). Comparing environmental impacts for livestock products: a review of life cycle assessments. Livest. Sci. 128, 1–11. doi: 10.1016/j.livsci.2009.11.007
ecoinvent Centre (2010). ecoinvent Data - The Life Cycle Inventory Data V2.2. Dübendorf: Swiss Centre for Life Cycle Inventories. Available online at: http://www.ecoinvent.org/database/older-versions/ecoinvent-version-2/ecoinvent-version-2.html (Accessed June 13, 2016).
Federal Office for Agriculture (2016). Weisungen und Erläuterungen zur Verordung über den landwirtschaftlichen Produktionskataster und die Ausscheidung von Zonen. Available online at: https://www.blw.admin.ch/blw/de/home/instrumente/grundlagen-und-querschnittsthemen/landwirtschaftliche-zonen/ausscheiden-der-zonen.html (Accessed October 12, 2016).
Federal Office for Agriculture (FOAG) (2015). Landwirtschaftliche Zonengrenzen. Available online at: https://www.blw.admin.ch/blw/de/home/politik/datenmanagement/geografisches-informationssystem-gis/download-geodaten.html (Accessed October 12, 2016).
Finger, R., Listorti, G., and Tonini, A. (2015). “The cheese processing aid in Switzerland: ex-post and ex-ante evaluations,” in International Conference of Agricultural Economists (Milan: International Association of Agricultural Economists). Available online at: http://EconPapers.repec.org/RePEc:ags:iaae15:211633 (Accessed June 29, 2016).
Flisch, R., Sinaj, S., Charles, R., and Wichner, W. (2009). Grundlagen für die Düngung im Acker- und Futterbau. Agrarforschung 16, 1–97. Available online at: http://www.agrarforschungschweiz.ch/archiv_11de.php?jahr=2009&band=16&heft=02
Frischknecht, R., Jungbluth, N., Althaus, H.-J., Bauer, C., Doka, G., Dones, R., et al. (2007a). “Implementation of life cycle impact assessment methods,” in Ecoinvent Report (Dübendorf: Swiss Centre for Life Cycle Inventories). Available online at: http://www.ecoinvent.org/files/201007_hischier_weidema_implementation_of_lcia_methods.pdf (Accessed May13, 2016).
Frischknecht, R., Jungbluth, N., Althaus, H.-J., Doka, G., Dones, G., and Heck, T. (2007b). “Overview and methodology,” in Ecoinvent Report (Dübendorf: Swiss Centre for Life Cycle Inventories). Available online at: http://www.ecoinvent.org/files/200712_frischknecht_jungbluth_overview_methodology_ecoinvent2.pdf (Accessed May 13, 2016).
Gellrich, M., and Zimmermann, N. E. (2007). Investigating the regional-scale pattern of agricultural land abandonment in the Swiss mountains: a spatial statistical modelling approach. Landscape Urban Plan. 79, 65–76. doi: 10.1016/j.landurbplan.2006.03.004
Greenwood, K. L., and McKenzie, B. M. (2001). Grazing effects on soil physical properties and the consequences for pastures: a review. Aust. J. Exp. Agr. 41, 1231–1250. doi: 10.1071/EA00102
Grêt-Regamey, A., Brunner, S. H., and Kienast, F. (2012). Mountain ecosystem services: who cares? Mt. Res. Dev. 32, S23–S34. doi: 10.1659/mrd-journal-d-10-00115.s1
Guinée, J. B., Gorrée, M., Heijungs, R., Huppes, G., Kleijn, R., de Koning, A., et al. (2001). Life Cycle Assessment–An Operational Guide to the ISO Standards. Part 2b: Operational Annex. Den Haag; Leiden: Ministry of Housing, Spatial Planning and Environment (VROM) and Centre of Environmental Science (CML). Available online at: http://cml.leiden.edu/research/industrialecology/researchprojects/finished/new-dutch-lca-guide.html (Accessed May 13, 2016).
Hauschild, M. Z., and Potting, J. (2005). “Spatial differentiation in life cycle impact assessment–The EDIP2003 methodology,” in Environmental News (Copenhagen: The Danish Ministry of the Environment, Environmental Protection Agency). Available online at: http://www2.mst.dk/udgiv/publications/2005/87-7614-579-4/pdf/87-7614-580-8.pdf (Accessed July 4, 2016).
Hellweg, S., and Milà i Canals, L. (2014). Emerging approaches, challenges and opportunities in life cycle assessment. Science 344, 1109–1113. doi: 10.1126/science.1248361
Homburger, H., Lüscher, A., Scherer-Lorenzen, M., and Schneider, M. K. (2015). Patterns of livestock activity on heterogeneous subalpine pastures reveal distinct responses to spatial autocorrelation, environment and management. Mov. Ecol. 3, 1–15. doi: 10.1186/s40462-015-0053-6
Hoop, D., and Schmid, D. (2014). Zentrale Auswertung von Buchhaltungsdaten – Grundlagenbericht 2013. Ettenhausen: Agroscope INH. Available online at: http://www.grundlagenbericht.ch (Accessed July 4, 2016).
Horn, M., Steinwidder, A., Gasteiner, J., Podstatzky, L., Haiger, A., and Zollitsch, W. (2013). Suitability of different dairy cow types for an Alpine organic and low-input milk production system. Livest. Sci. 153, 135–146. doi: 10.1016/j.livsci.2013.01.011
Hörtenhuber, S., Lindenthal, T., Amon, B., Markut, T., Kirner, L., and Zollitsch, W. (2010). Greenhouse gas emissions from selected Austrian dairy production systems – model calculations considering the effects of land use change. Renew. Agr. Food Syst. 25, 316–329. doi: 10.1017/S1742170510000025
Hutchings, N., Amon, B., Dämmgen, U., and Webb, J. (2009). “4.B Manure management,” in EMEP/EEA Air Pollutant Emission Inventory Guidebook 2009, Vol. 73 (Copenhagen: European Environment Agency). Available online at: http://www.eea.europa.eu//publications/emep-eea-emission-inventory-guidebook-2009">http://www.eea.europa.eu//publications/emep-eea-emission-inventory-guidebook-2009 (Accessed April 15, 2016).
Hutchings, N., Webb, J., and Amon, B. (2013). “3.D Crop production and agricultural soils,” in EMEP/EEA Air Pollutant Emission Inventory Guidebook 2013, Vol. 43 (Copenhagen: European Environment Agency). Available online at: http://www.eea.europa.eu//publications/emep-eea-guidebook-2013 (Accessed April 15, 2016).
International Dairy Federation (IDF) (2010). “A common carbon footprint approach for dairy–The IDF guide to standard lifecycle assessment methodology for the dairy sector,” in Bulletin of the International Dairy Federation (Brussels: International Dairy Federation). Available online at: http://www.idf-lca-guide.org/Files/media/Documents/445-2010-A-common-carbon-footprint-approach-for-dairy.pdf (Accessed July 4, 2016).
International Dairy Federation (IDF) (2015). “A common carbon footprint approach for the dairy sector - The IDF guide to standard life cycle assessment methodology,” in Bulletin of the International Dairy Federation (Brussels: International Dairy Federation). Available online at: http://www.fil-idf.org/Public/Download.php?media=41396 (Accessed May 13, 2016).
ISO (2006). ISO14040:2006. Environmental Management – Life Cycle Assessment – Principles and Framework. Geneva: International Organization for Standardization.
Jeanneret, P., Baumgartner, D. U., Freiermuth Knuchel, R., Koch, B., and Gaillard, G. (2014). An expert system for integrating biodiversity into agricultural life-cycle assessment. Ecol. Indic. 46, 224–231. doi: 10.1016/j.ecolind.2014.06.030
Kingwell, R. (2011). Managing complexity in modern farming. Aust. J. Agric. Resour. Econ. 55, 12–34. doi: 10.1111/j.1467-8489.2010.00528.x
Koch, B., Edwards, P. J., Blanckenhorn, W. U., Walter, T., and Hofer, G. (2015). Shrub encroachment effects the diversity of plants, butterflies, and grasshoppers on two Swiss subalpine pastures. Arct. Antarct. Alp. Res. 47, 345–357. doi: 10.1657/AAAR0013-093
Lomba, A., Guerra, C., Alonso, J., Honrado, J. P., Jongman, R., and McCracken, D. (2014). Mapping and monitoring high nature value farmlands: challenges in European landscapes. J. Environ. Manage. 143, 140–150. doi: 10.1016/j.jenvman.2014.04.029
MacDonald, D., Crabtree, J. R., Wiesinger, G., Dax, T., Stamou, N., Fleury, P., et al. (2000). Agricultural abandonment in mountain areas of Europe: environmental consequences and policy response. J. Environ. Manage. 59, 47–69. doi: 10.1006/jema.1999.0335
Marriott, C. A., Fothergill, M., Jeangros, B., Scotton, M., and Louault, F. (2004). Long-term impacts of extensification of grassland management on biodiversity and productivity in upland areas. A review. Agronomie 24, 447–462. doi: 10.1051/agro:2004041
Marton, S. M. R. R., Zimmermann, A., Kreuzer, M., and Gaillard, G. (2016a). Comparing the environmental performance of mixed and specialised dairy farms: the role of the system level analysed. J. Clean. Prod. 124, 73–83. doi: 10.1016/j.jclepro.2016.02.074
Marton, S. M. R. R., Zimmermann, A., Kreuzer, M., and Gaillard, G. (2016b). Environmental and socioeconomic benefits of a division of labour between lowland and mountain farms in milk production systems. Agric. Syst. 149, 1–10. doi: 10.1016/j.agsy.2016.07.015
Meyfroidt, P., Lambin, E. F., Erb, K.-H., and Hertel, T. W. (2013). Globalization of land use: distant drivers of land change and geographic displacement of land use. Curr. Opin. Env. Sustain. 5, 438–444. doi: 10.1016/j.cosust.2013.04.003
Myhre, G., Shindell, D., BreÌĄon, F.-M., Collins, W., Fuglestvedt, J., Huang, J., et al. (2013). “Anthropogenic and natural radiative forcing,” in Climate Change 2013: The Physical Science Basis. Contribution of Working Group I to the Fifth Assessment Report of the Intergovernmental Panel on Climate Change, eds T. F. Stocker, D. Qin, G.-K. Plattner, M. Tignor, S. K. Allen, J. Boschung, A. Nauels, Y. Xia, V. Bex, and P. M. Midgley (Cambridge; New York, NY: Cambridge University Press), 659–740. Available online at: http://www.climatechange2013.org.
Nemecek, T., Freiermuth Knuchel, R., Alig, M., and Gaillard, G. (2010). “The advantage of generic LCA tools for agriculture: examples SALCAcrop and SALCAfarm,” in 7th International Conference on Life Cycle Assessment in the Agri-food Sector, eds B. Notarnicola, E. Settanni, G. Tassielli, and P. Giungato (Bari).
Nemecek, T., and Kägi, T. (2007). “Life cycle inventories of agricultural production systems,” in Ecoinvent Data V2.0 - Ecoinvent Report No. 15 (Dübendorf: Swiss Centre for Life Cycle Inventories). Available online at: http://www.ecoinvent.ch (Accessed September 4, 2015).
Olynk, N. J., and Wolf, C. (2010). Aligning incentives for contract dairy heifer growth. J. Agric. Resour. Econ. 35, 489–502. Available online at: http://www.jstor.org/stable/23243068
Penati, C., Berentsen, P. B. M., Tamburini, A., Sandrucci, A., and de Boer, I. J. M. (2011). Effect of abandoning highland grazing on nutrient balances and economic performance of Italian Alpine dairy farms. Livest. Sci. 139, 142–149. doi: 10.1016/j.livsci.2011.03.008
Plieninger, T., and Bieling, C. (2013). Resilience-based perspectives to guiding high-nature-value farmland through socioeconomic change. Ecol. Soc. 18:20. doi: 10.5751/es-05877-180420
Pornaro, C., Schneider, M. K., and Macolino, S. (2013). Plant species loss due to forest succession in Alpine pastures depends on site conditions and observation scale. Biol. Conserv. 161, 213–222. doi: 10.1016/j.biocon.2013.02.019
Ravishankara, A. R., Daniel, J. S., and Portmann, R. W. (2009). Nitrous oxide (N2O): the dominant ozone-depleting substance emitted in the 21st century. Science 326, 123–125. doi: 10.1126/science.1176985
Regan, J. T., Marton, S. M. R. R., Barrantes, O., Ruane, E., Hanegraaf, M., Berland, J., et al. (2016). Does the recoupling of dairy and crop production at the district scale generate environmental benefits? A case-study approach in Europe. Eur. J. Agron. doi: 10.1016/j.eja.2016.08.005
Renard, K. G., Yoder, D. C., Lightle, D. T., and Dabney, S. M. (2011). “Universal soil loss equation and revised universal soil loss equation,” in Handbook of Erosion Modelling, eds R. P. C. Morgan and M. A. Nearing (Blackwell Publishing Ltd.), 137–167. Available online at: http://www.tucson.ars.ag.gov/unit/publications/PDFfiles/2122.pdf.
Revell, L. E., Tummon, F., Salawitch, R. J., Stenke, A., and Peter, T. (2015). The changing ozone depletion potential of N2O in a future climate. Geophys. Res. Lett. 42, 10047–10055. doi: 10.1002/2015gl065702
Ripoll-Bosch, R., de Boer, I. J. M., Bernués, A., and Vellinga, T. V. (2013). Accounting for multi-functionality of sheep farming in the carbon footprint of lamb: a comparison of three contrasting Mediterranean systems. Agric. Syst. 116, 60–68. doi: 10.1016/j.agsy.2012.11.002
Roesch, A. (2012). Factors affecting the situation of economically weak farms in Switzerland. Agr. Econ. Rev. 13, 23–38. Available online at: http://www.eng.auth.gr/mattas/13_1_2.pdf
Rudow, K. (2014). Less favoured area payments – impacts on the environment, a German perspective. Agr. Econ. 60, 260–272. Available online at: http://www.agriculturejournals.cz/web/agricecon.htm?type=article&id=74_2013-AGRICECON
Stark, R., Stehle, T., and Schick, M. (2014). Arbeitsvoranschlag & Modellkalkulationssystem, 2014 Edn. Tänikon: Forschungsanstalt Agroscope Reckenholz-Tänikon ART.
Strohbach, M. W., Kohler, M. L., Dauber, J., and Klimek, S. (2015). High nature value farming: from indication to conservation. Ecol. Indic. 57, 557–563. doi: 10.1016/j.ecolind.2015.05.021
Swiss Federal Statistical Office (2015). Wages and Income from Employment – Indicators. Wage level – By Branch. Available online at: http://www.bfs.admin.ch/bfs/portal/en/index/themen/03/04/blank/key/lohnstruktur/nach_branche.html (Accessed June13, 2016).
Swiss Federal Statistical Office (2016). STAT-TAB. Available online at: https://www.pxweb.bfs.admin.ch/default.aspx?px_language=de (Accessed May 13, 2016).
swisstopo (2016). swissBOUNDARIES3D. Available online at: https://shop.swisstopo.admin.ch/de/products/landscape/boundaries3D (Accessed October 12, 2016).
Teuscher, R., Lansche, J., Nemecek, T., and Gaillard, G. (2014). “Optimizing the LCA data processing for food products in the context of life cycle sustainability assessment: challenges and opportunities,” in 9th International Conference on Life Cycle Assessment in the Agri-Food Sector (LCA Food 2014), eds R. Schenck and D. Huizenga (San Francisco, CA: ACLCA).
TSM (2013). Milchstatistik der Schweiz - Statistique latière de la Suisse 2012. Berne. Available online at: http://www.swissmilk.ch/de/produzenten/milchmarkt/zahlen-fakten-milchmarkt/statistiken/-dl-/fileadmin/filemount/publikation-milchstatistik-der-schweiz-2012-de.pdf (Accessed 13 June 2016).
UNEP (2013). Drawing Down N2O to Protect Climate and the Ozone Layer. A UNEP Synthesis Report. United Nations Environment Programme (UNEP), Nairoby. Available online at: http://www.unep.org/pdf/UNEPN2Oreport.pdf [Accessed 11 May 2016].
van der Werf, H. M. G., Kanyarushoki, C., and Corson, M. S. (2009). An operational method for the evaluation of resource use and environmental impacts of dairy farms by life cycle assessment. J. Environ. Manage. 90, 3643–3652. doi: 10.1016/j.jenvman.2009.07.003
Keywords: contract rearing, less favored areas, natural constraints, dairy, life cycle assessment, biodiversity, workload
Citation: Marton SMRR, Lüscher G, Corson MS, Kreuzer M and Gaillard G (2016) Collaboration between Mountain and Lowland Farms Decreases Environmental Impacts of Dairy Production: The Case of Swiss Contract Rearing. Front. Environ. Sci. 4:74. doi: 10.3389/fenvs.2016.00074
Received: 16 July 2016; Accepted: 31 October 2016;
Published: 16 November 2016.
Edited by:
Maria Tsiafouli, Aristotle University of Thessaloniki, GreeceReviewed by:
Ângela Cristina Lomba, Research Centre in Biodiversity and Genetic Resources (CIBIO), PortugalAndrey S. Zaitsev, Justus Liebig University, Germany and Interim Panel of Eminent Experts (RAS), Russia
Copyright © 2016 Marton, Lüscher, Corson, Kreuzer and Gaillard. This is an open-access article distributed under the terms of the Creative Commons Attribution License (CC BY). The use, distribution or reproduction in other forums is permitted, provided the original author(s) or licensor are credited and that the original publication in this journal is cited, in accordance with accepted academic practice. No use, distribution or reproduction is permitted which does not comply with these terms.
*Correspondence: Silvia M. R. R. Marton, cy5tYXJ0b25AbXV1dWguY2g=