- 1Department of Knowledge Technologies, Jozef Stefan Institute, Ljubljana, Slovenia
- 2Plant Science Group, Wageningen University and Research, Wageningen, Netherlands
- 3Department for Soil Health and Plant Nutrition, Austrian Agency for Health and Food Safety (AGES), Vienna, Austria
- 4Crops, Environment and Land Use Programme, Teagasc, Wexford, Ireland
- 5Department of Environmental Systems Science, Swiss Federal Institute of Technology, ETH Zürich, Zurich, Switzerland
- 6Centre for Sustainability, Environment and Health, National Institute for Public Health and the Environment, Bilthoven, Netherlands
- 7Soil Biology Group, Wageningen University and Research, Wageningen, Netherlands
- 8Department of Plant and Environmental Sciences, University of Copenhagen, Copenhagen, Denmark
Agricultural decision support systems (DSSs) are mostly focused on increasing the supply of individual soil functions such as, e.g., primary productivity or nutrient cycling, while neglecting other important soil functions, such as, e.g., water purification and regulation, climate regulation and carbon sequestration, soil biodiversity, and habitat provision. Making right management decisions for long-term sustainability is therefore challenging, and farmers and farm advisors would greatly benefit from an evidence-based DSS targeted for assessing and improving the supply of several soil functions simultaneously. To address this need, we designed the Soil Navigator DSS by applying a qualitative approach to multi-criteria decision modeling using Decision Expert (DEX) integrative methodology. Multi-criteria decision models for the five main soil functions were developed, calibrated, and validated using knowledge of involved domain experts and knowledge extracted from existing datasets by data mining. Subsequently, the five DEX models were integrated into a DSS to assess the soil functions simultaneously and to provide management advices for improving the performance of prioritized soil functions. To enable communication between the users and the DSS, we developed a user-friendly computer-based graphical user interface, which enables users to provide the required data regarding their field to the DSS and to get textual and graphical results about the performance of each of the five soil functions in a qualitative way. The final output from the DSS is a list of soil mitigation measures that the end-users could easily apply in the field in order to achieve the desired soil function performance. The Soil Navigator DSS has a great potential to complement the Farm Sustainability Tools for Nutrients included in the Common Agricultural Policy 2021–2027 proposal adopted by the European Commission. The Soil Navigator has also a potential to be spatially upgraded to assist decisions on which soil functions to prioritize in a specific region or member state. Furthermore, the Soil Navigator DSS could be used as an educational tool for farmers, farm advisors, and students, and its potential should be further exploited for the benefit of farmers and the society as a whole.
Introduction
Soil functions are fundamental for the provision of many ecosystem services, as soils contribute to the generation of goods and services beneficial to human society and the environment (Blum, 2005; Schulte et al., 2014; Adhikari and Hartemink, 2016; Baveye et al., 2016). The five main soil functions in agriculture and forestry are primary productivity, water purification and regulation, climate regulation and carbon sequestration, soil biodiversity and habitat provision, and provision and cycling of nutrients (Haygarth and Ritz, 2009; Creamer and Holden, 2010; Bouma et al., 2012; Rutgers et al., 2012; Schulte et al., 2014). If one or more soil functions are impeded, threats to soil functions may arise (e.g., soil sealing, compaction, erosion, loss of biodiversity, loss of organic matter, salinization, contamination, and desertification) (Blum et al., 2004; Creamer and Holden, 2010; Creamer et al., 2010; Stolte et al., 2016) and the rational use and protection of soil would fail (European Commission, 2006; Stankovics et al., 2018).
All soils can perform these functions simultaneously, but the extent and the relative composition of this functionality depend on soil characteristics (physical, chemical, and biological), environmental variables (regimes for temperature, humidity, hydrology, slope), land use (cropland, grassland, forestry), and soil management practices (e.g., drainage and irrigation, tillage, nutrient and pest management, crop choice, and rotation) that reflect the specific demands for soil functions (Schulte et al., 2015; Vogel et al., 2019).
Until now, research and corresponding soil-related policies have mostly focused on increasing the provision of individual soil functions. This has resulted in inconsistent and sometimes even conflicting recommendations (ten Berge et al., 2017). Making correct management decisions for soils is therefore challenging and farmers have to make these decisions on their farm/land daily. Therefore, farmers and farm advisors would greatly benefit from evidence-based decision support systems (DSSs) to support their decision making process. DSS are web-based or app-based software systems and are designed to guide the end-users through different stages of decision making in order to reach a final decision (Dicks et al., 2014). DSS targeted for optimizing the supply of soil functions could be used to provide farmers and farm advisors with information about the potential effects of external physiochemical, biological, and management factors. In addition, DSS could inform stakeholders about whether particular targets for selected soil functions have been reached, and if not, how management could enable them to reach those targets.
The usefulness of DSS has been confirmed in different agricultural domains like pest management, nutrient management planning, farm economy, livestock, and crop management (Jones et al., 2017a,b). The national farm advisory services in several European member states are offering access to DSS as an integrated part of supporting their clients. Examples of such DSS are MarkOnline in Denmark (Bligaard, 2014), Mesp@rcelles in France (APCA, 2019), NMP Online in Ireland (Teagasc, 2016), AgrarCommander in Austria (AGES, 2019), and Web Module Düngung in Germany (LWK Niedersachsen, 2019). Furthermore, in the new 2021–2027 Common Agricultural Policy (CAP) proposal (European Commission, 2018) adopted by the European Commission, member states are suggested to implement nutrient management plans, supported by the use of Farm Sustainability Tools for Nutrients (FaST). This is specifically part of the new framework of standards for good agricultural and environmental condition of land (GAECs). A recent review of app-based DSS in agriculture concludes that there is a demand for and value in systems able to address individual farm management issues for achieving the sustainability goals (Eichler Inwood and Dale, 2019). However, nearly all DSS on the market can be characterized as “single solution” DSS that provide limited data to improve only a specific aspect of farm management practices and lack an integration of sustainability aspects (Eichler Inwood and Dale, 2019). Evaluating several soil functions in the same DSS would overcome this lack of integration. Furthermore, although agricultural DSS are becoming increasingly advanced, the uptake and use of DSS by farmers and farm advisors is still very low compared to the number available and accessible DSS (Rose et al., 2016; Bampa et al., 2019). Several studies show that one of the main reasons for this is the lack of end-user involvement in the design and development of the DSS since the beginning of the process (Rose et al., 2016; Lindblom et al., 2017; Rodela et al., 2017). Rose et al. (2016) argue that a successful uptake of DSS requires end-users to be actively involved in the development of the DSS. In addition, these tools should be designed in such a way that they are easy to use, fit the existing workflow of users, and are trustworthy.
The main goal of the European-founded project LANDMARK (Land Management: Assessment, Research, Knowledge base) is to develop a scientific framework for the quantification and management of the five aforementioned soil functions. Furthermore, it aims to provide guidelines for the optimization of these soil functions at the local, regional, and European scale. In order to quantify the soil functions at the local level, a web-based DSS, the Soil Navigator, was developed. It provides an integrated assessment of the five soil functions, which allows an assessment of trade-offs between soil functions for a specific agricultural management practice. In addition, the DSS proposes a suite of management practices that foster an optimal balance among soil functions, recognizing the different function priorities and requirements across different European pedo-climatic zones (Metzger et al., 2005).
The main aim of this paper is to explain the methodological framework for the development of the Soil Navigator DSS. First, we describe the general principles for DSS development and the methodological and theoretical background of the DSS architecture. Then, we present the methodology used for the development of decision support models and their integration into a final DSS, including the active involvement of end-users in the development of the Soil Navigator.
Materials and Methods
Decision Problem
The initial step in the process of decision modeling and developing DSS is to define the decision problem. For farmers and farm advisers, most existing decision models deal with primary productivity, which helps the farmer to achieve crop or livestock production targets and economic revenue. However, in the majority of cases, there are no strong drivers and limited legislation to enhance the multi-functionality of soils (Bünemann et al., 2018). Nevertheless, farmers and farm advisors often try to enhance the multi-functionality of their soils, and are more likely to do so where they have observed reduction in crop yields, due to soil degradation, or due to climate change effects (Olesen et al., 2011). However, information on whether the applied agricultural management practices provide support to the multi-functional performance of their soils or how management needs to be modified in order to achieve better performance are not trivial to find or have access to. Hence, decisions on what agricultural management practices will need to be adopted to achieve better performances of all soil functions remains a complex decision problem.
In our study, the decision problem was defined in two steps:
i) Assessing the performance of the five soil functions under specific management practices, environmental/climatic conditions, and soil characteristics;
ii) Choosing appropriate management practices that will improve the performance of the soil functions under given environmental/climatic conditions and soil characteristics.
Decision Support System
To address this complex decision problem, an integration of existing data and knowledge into a DSS using information technologies is required. We designed our DSS as an interactive computer-based system intended to help farmers and advisors to utilize data, knowledge, and models to make decisions about the management measures that would improve the performance of the soil function (Power, 2019). Furthermore, as recommended by Rose et al. (2016), we involved end-users throughout the development process by consulting farmers and farm advisors in Denmark, Austria, France, Germany, and Ireland. This was done systematically by (i) conducting stakeholder workshops before initiating the development of the DSS, (ii) establishing and consulting National Reference Groups (NRGs) for the Soil Navigator in the development phase, and (iii) organizing workshops with farmers and farm advisors to guide the further development of the DSS prototype.
The developed DSS belongs to the group of cooperative and dynamic DSS (Hättenschwiler, 1999), which allows the decision maker to modify the decision suggestions provided by the system. The process is then repeated until a satisfying solution is generated for the user. Given the nature of the DSS and the complexity of the decision problem, we structured the DSS in accordance with Turban et al. (2004), who suggest that the DSS should include data and knowledge bases, models, and a user interface. The structure thus comprises the following seven parts: knowledge base, database, data transformation, decision models, output, user interface, and user (Figure 1).
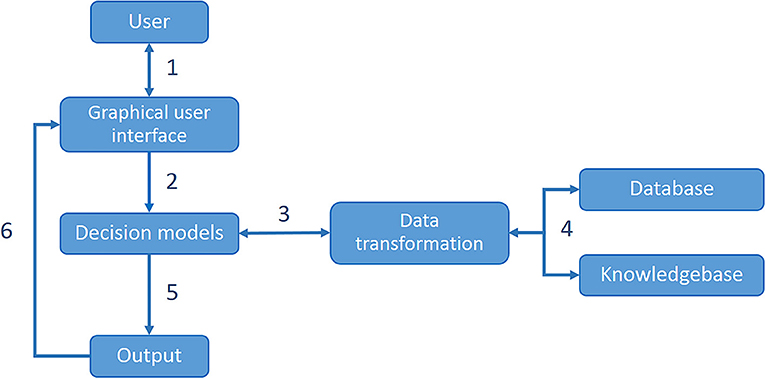
Figure 1. Methodological structure of the DSS for the assessment and management of five soil functions.
The DSS methodological elements are linked through the following types of information and data flows:
(1) User communication channel: The communication channel through which the user inputs the required data about the field of interest and the constraints about the available soil management measures. This information channel provides also the outputs from the DSS to the end-users. The textual and graphical form of the input and output information are provided in a user-friendly form.
(2) Steering information channel: The information entered by the end-user through the interface are sent to the decision support models, where the modeling constraints and operations are set, and the data required for the performance of the demanding modeling tasks are selected.
(3) Data flow: Flow of data that are transformed according to the requirements of the individual decision models.
(4) Raw data flow: Information for the required data are sent to the data and knowledge bases and available data are sent back to the data transformation part for their further formatting in order to be used in the decision support models.
(5) Flow of modeling results: Outputs from the individual decision support models are sent for further meta-analysis and translation into a set of applicable soil management measures.
(6) Output information flow: Information about the proposed management measures are sent back to the user interface and are communicated to the end-users.
Knowledge Base
DSS rely heavily on expert knowledge as their central element (Uusitalo et al., 2015). However, relying on expert knowledge poses several challenges. The first is the acquisition of expert knowledge and its representation in a formalized way for the purposes of decision modeling (Shaw and Woodward, 1990). Another challenge is that the expert knowledge may differ between experts (Tversky and Kahneman, 1974). In addition, finding a sufficient number of experts, which are knowledgeable on the subject matter is often difficult (Shaw and Woodward, 1990). There is also an issue of elicitation of the different opinions of the selected experts.
In our study, groups of scientists from the LANDMARK consortium and stakeholders from the NRGs were involved in participatory modeling approach (Bohanec and Zupan, 2004; Jakku and Thorburn, 2010), where they participated in the development, calibration, and validation of the different soil function decision models. Besides working with experts, we also obtained domain knowledge from empirical data using machine learning and data mining (Trajanov et al., 2015, 2018; Bondi et al., 2018). Machine learning algorithms represent a useful tool to extract knowledge from data and representing it in a format that can be easily used for constructing decision models (Trajanov et al., 2018).
Database
Input data for the DSS came from the end-users that provided specific attributes about their field and the applied management practices. Another part of the data was collected from existing databases (soil, meteorological databases) to which the system is internally connected. Specifically, we used soil, environment, and management data from the LANDMARK project (Micheli et al., 2017; Saby et al., 2018). During the development of the individual decision models, data were used for their verification, calibration, and validation. Later, data were used as an input into the DSS.
Data Transformation
During data transformation, input data are transformed to a format suitable for feeding all models. Transformations performed within this segment included (i) data discretization, (ii) derivation of synthesized input attributes, and (iii) attribute harmonization.
Data discretization is applied to numerical values and discretizes them into nominal (qualitative) values. This step is important, as inputs in the decision models are qualitative values from a predefined scale of values. The discretization process uses thresholds defined in accordance with different ecosystems and climatic zones. The former specify the thresholds regarding the purpose of land use (cropland or grassland). The latter capture the spatial distribution of the thresholds according to six predefined climatic zones (Metzger et al., 2005): Central Atlantic, North Atlantic, Continental, South Alpine, Pannonian, and North Mediterranean region.
The derivation of synthesized input attributes is a process of integration of one or more input attributes through an aggregation function that can be defined as a simple mathematical expression or a set of mathematical expressions and can result in a qualitative or quantitative value. The aggregation is performed using predefined mathematical expression (e.g., functions) for each synthesized attribute.
Attribute harmonization matches the name of each empirical (measured) attribute to the name of the corresponding models' input attribute. This process is required to avoid mismatch in the names and meaning of attributes among different models.
Methodology for Construction of Decision Models
The decision models perform the central tasks of the decision-making process and are at the core of the DSS. In general, they are used for prediction of the outcome of the decision choice that we might make (Mallach, 1994). The decision models help the decision makers to rank a set of decision alternatives and choose the best one according to their preferences. In the Soil Navigator, the ranking of a set of decision alternatives is based on a list of selected criteria, which are relevant for the soil functions. Since we were dealing with a multi-criteria decision problem, we used multi-criteria decision models (MCDM) for the analysis of our decision problem (Kangas et al., 2015). Our approach is based on the application of analytical hierarchical processes (Saaty, 1990) for building decision models. Following this approach, a complex decision problem is decomposed into less complex sub-problems represented by attributes structured into a hierarchy, where hierarchical levels are linked by integrative functions.
The simultaneous assessment of the five soil functions could be addressed by qualitative MCDM (Mendoza and Martins, 2006; Greco et al., 2016). To build qualitative MCDM, we used the DEX (Decision Expert) integrative methodology (Bohanec and Rajkovič, 1990; Bohanec et al., 2013; Bohanec, 2017), which combines the approach of hierarchical MCDM with rule-based expert systems and fuzzy sets. DEX enables the acquisition and the representation of decision knowledge, as well as evaluation and analysis of decision alternatives. DEX is based on attributes with a finite set of nominal values. The integrative functions (integration rules) in DEX are represented with if–then rules, which are given in a tabular form (Figure 2B). These rules are a tabular representation of a mapping from lower-level to higher-level attributes. The DEX methodology enables the construction of transparent and comprehensive models, and it provides mechanisms for presenting aggregation rules in a user-friendly way, i.e., in the form of decision trees.
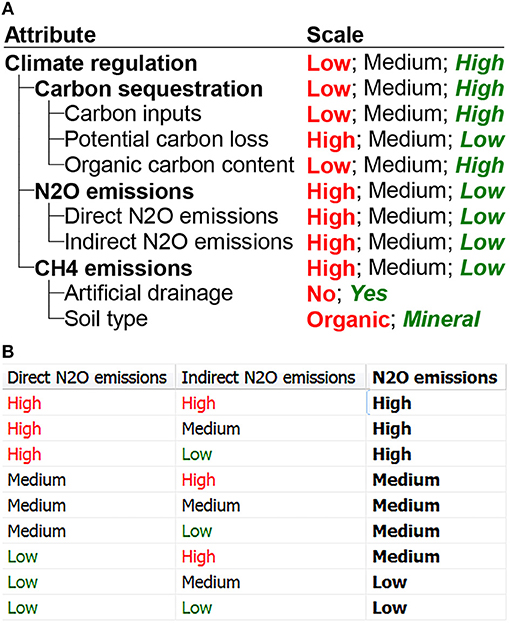
Figure 2. Top part of the DEX model for the climate regulation and carbon sequestration soil function. (A) Hierarchical structure and scale values of attributes. (B) Integration rules for integration of direct and indirect N2O emissions into N2O emissions.
Beside the mere evaluation of alternatives, the DEX methodology provides what-if analysis of alternatives (e.g., effects of changing one or more initial attribute values on model outputs). In addition, DEX is able to handle missing or non-exact data using probabilistic or fuzzy distribution of attributes' values. The evaluation of alternatives was used for the assessment of the performance of all five soil functions, while the what-if analyses were used for the selection of soil management measures that would improve the performance of soil functions if needed. The decision models were built with the software modeling tool DEXi (Bohanec, 2017, 2019).
Output
The output of the developed DSS consists of (i) an assessment of the performance of each soil function and (ii) suggestions of how to improve the performance of the preferred soil function(s). Both outputs utilize the same DEX decision models developed for each soil function. However, they differ in their purpose, format, and the approach of utilization of the DEX tree structures.
The assessment of the performance of the soil functions considers the outputs of the DEX decision models. Each decision model is fed with data prepared in the data transformation step, after which the input basic attributes are aggregated to the upper level of the model structure. Such aggregation to higher level continues until the top node is reached. The aggregated value in the top node is the overall assessment of the performance of a particular soil function. The same approach is applied across all soil functions; thus, the format of the DSS output is a set of qualitative values describing the performance of the five soil functions.
The set of suggestions for improving the performance of the preferred soil function(s) is an output obtained by generative design approach (Lohan et al., 2016) over the DEX decision models. This approach traverses the DEX tree structure from the top to the bottom, i.e., from the top node (output attribute) to the basic (input) attributes. The idea of the generative design approach is to find a suitable combination of input values for a given output of the model. In this case, the input to the DEX decision models represents the current situation of a particular agricultural field represented through a set of qualitative input values. The generative design approach allows identifying attributes that need to be changed in order to achieve the desired performance of a soil function. The generative design can be constrained by users' preferences and only a subset of inputs undergo the generative approach. The same approach is applied for each soil function, which leads to a set of suggestions for improving their performance.
However, each soil function model generates a different set of suggestions of management practices, which leads to a long list of suggestions and they might be sometimes contradicting (some management practices might improve certain soil functions, but decrease other). Thus, an optimal set of management practices is chosen through a combinatorial process, where contradicting management practices are eliminated and only the acceptable sets of suggestions are further propagated to the user interface.
Graphical User Interface
The graphical user interface (GUI) enables the communication between the user and the DSS. The UI of our DSS is divided into two parts. In the first part, the users insert the required data (management, soil, and environmental properties) related to their field or modify the default data obtained from existing databases that the system is connected to.
In the second part, the GUI communicates the results of the DSS to the end-user. The results are represented textually and graphically and show an estimation of the performance/potential of each of the five soil functions in a qualitative way, using three values: low, medium, and high. The GUI also enables the end-users to choose the level of improvement for a certain soil function, as well as to set weights (preferences) to certain soil functions. The system then searches through all possible combinations of management practices that could be taken in order to improve the preferred soil functions and provide the end-user with several suggestions, if they exist.
Users
The target users of the Soil Navigator are farmers and farm advisors. To define the preferred type of communication between our DSS and the users, 32 workshops with 473 stakeholders (farmers, farm advisors, and regional, national, and European stakeholders) were conducted as part of the LANDMARK project (Bampa et al., 2019). The results from these workshops show good to very good understanding among potential end-users of the meaning and need for soil quality and the participants demonstrated their inherent understanding of soil functioning. In addition, in many cases, they showed a reasonable understanding of the four soil functions other than primary production, but found it difficult to assess how multiple soil functions interact and respond to management measures under local conditions. These consultations demonstrated a knowledge gap regarding the existence of soil data and the possible use of such data in decision support tools for assessment and management of soil functions. This knowledge gap exists despite the fact that the importance of having data, providing advice and simple tools to support decisions on soil and land management, was well-recognized. The results of Bampa et al. (2019) showed a strong interest by famers for independent and scientifically supported advice to be provided at field level.
Following these initial stakeholder workshops, NRGs for the Soil Navigator were established with farmer and farm advisors in Denmark, Austria, France, Germany, and Ireland in order to involve them in the development of the Soil Navigator. The NRGs were consulted using an online survey and a follow-up interview to ensure that the Soil Navigator DSS was designed in such a way that it (i) is easy to use; (ii) provides trustworthy, relevant, and valuable information; and (iii) uses terms that are recognized and meaningful for both farmers and farm advisors. In the survey, the NRG members were asked about (i) the most meaningful terms for describing the five soil functions, (ii) the availability of data required for running the DSS, (iii) their preferences for the functionality and design of the DSS, and (iv) what would make the DSS trustworthy, relevant, and valuable for them. After the first DSS prototype was developed, we subsequently organized hands-on evaluation workshops with farmers and farm advisors in Denmark, Austria, France, Germany, and Ireland to get feedback and expertise for the further development of the DSS. This participatory approach taken toward continuously involving end-users along the development process has proved successful in creating a sense of ownership and trust toward the tool finalization.
Construction of the Decision Models
In our study, DEX decision models were developed for all five soil functions using the following five standard steps of building ecological models: construction of the models, verification, sensitivity analysis and calibration in an iterative way, and validation (Jørgensen and Fath, 2011).
The construction of the DEX models started by breaking down the concept of each soil function into smaller and less complex parts using the software DEXi (Bohanec, 2019). The structure of the model is given as a hierarchy of attributes (Figure 2A). It consists of basic attributes (input data), aggregated attributes (internal nodes), which provide the assessment of the alternatives at various hierarchical levels, and the root attribute (top attribute), which gives the overall assessment of the alternatives and presents the final output of the model. The same initial or aggregated attribute can participate in several integration rules and such attributes are named linked attributes.
The involved experts assigned a finite set of qualitative (nominal) values (e.g., low, medium, high; suitable, not suitable; wet, dry) to each attribute in the model. Their value scales were ordered preferentially from “bad to good” or were left unordered in cases when the attributes' values could not be ordered (Figure 2A). The integration from basic attributes (e.g., soil pH, salinity or tillage) to the soil function (the attribute at the top of the hierarchy) was defined by integration rules given in a form of decision tables that were formulated by the involved experts (Figure 2B).
An example of the structure of a DEX model is presented in Figure 2A, where the first three hierarchical levels of the climate regulation and carbon sequestration soil function are shown. Each attribute has an ordered scale of values, which are integrated in a decision table as presented in Figure 2B. The rows in the table represent integration rules, which map the values of lower-level attributes into an integrated (higher-level) attribute. A detailed description of the DEX model for the climate regulation and carbon sequestration soil function is explained in Van de Broek et al. (unpublished)1.
When the models were constructed, a model verification was performed in order to test their internal operational logic and behavior. The verification was performed by domain experts and end-users (farm advisors and farmers) who designed several theoretical case study scenarios, covering a wide spectrum of possible evaluation alternatives (e.g., variability of soil samples). The outputs from the models were compared to the results of a ranking made by experts and end-users of the soil function performance. If the experimental results were not as expected, the integration rules were re-examined. If this did not make a significant change, the model structure was modified as well.
When the verification of all soil function decision models was completed, a sensitivity analyses was carried out. This was used to find input attributes whose values had a negligible impact on the model behavior. These attributes were removed from the models to reduce the model complexity. The sensitivity analysis of the DEX models was based on the contribution of a corresponding attribute to the final evaluation result. Because the attributes had different value scales (some attributes have more values than others), the weights had to be normalized to the same unit interval. In our study, we used global normalized weights, which take into account the structure of the entire model and the relative importance of every part (Bohanec, 2019). In cases when the weights of the basic attributes were negligible (<1%), the attributes were removed from the model structure and the integration rules in the corresponding integration table were modified accordingly. After that, the verification process was repeated.
To adjust the sensitivity of the decision models to specific pedo-climatic conditions, calibration of the models was performed. Since we developed qualitative multi-attribute models where tables with integration rules are used (Figure 2B), the sensitivity analysis was performed by the variation of the integration rules. When data were available, we applied data mining to obtain additional knowledge about the integration rules in order to improve the model sensitivity and performance (Sandén et al., 2019).
To check how well the model outputs fit real-world data, a validation of the decision models was performed. First, an estimation of the real performance of the soil function on a certain field was calculated from empirical data or was estimated by experts and end-users. Subsequently, the model's output was compared to the estimated level of the soil function performance. Finally, the ratio between the number of correctly predicted soil function performance levels and the total number of estimations was calculated. Different validation criteria were formulated for each soil function due to the differences in the availability and quality of empirical data (Rutgers et al., 2019; Sandén et al., 2019; Delgado et al., unpublished2; Van de Broek et al., unpublished1). The data used for validation were not used for model calibration.
Soil Navigator
Conceptual Structure of the DSS Soil Navigator
The conceptual structure of the DSS Soil Navigator (Figure 3) consists of two parts. In the first part, the assessment of the soil functions was carried out, while the second part searches for appropriate soil management practices to improve the performance of the soil functions in accordance with the expectations and goals of the user.
The assessment of the performance of all five soil functions is based on the inputs to the DSS, which comprises data describing the properties of the assessed field. There are three categories of input data. The first category describes environmental conditions (climatic and orographic data), the second category describes soil properties of the assessed field (e.g., water pathways, physical, chemical, and biological soil properties), and the last category provides data about the current soil and agronomic management activities (crop management, fertilization, water management, pest management, harvest) for that field. Once the input data are pre-processed, they are sent to the soil function decision models, which provide an assessment of the performances of the five soil functions.
If the performance of the assessed soil functions is not in accordance with the expected levels, the DSS proposes appropriate changes in management practices that will increase the performance of these soil functions. This is performed in the second part of the system. The mechanism of iterative what-if analysis searches through all theoretical combinations of the values of input attributes to find combinations that provide the accepted performance level of the soil functions. The number of suitable combinations of mitigation measures could theoretically be very large. Therefore, the selection is based on the collection of mitigation options that are actually criteria representing the end-users' management preferences or constraints. The final output from the DSS is a list of mitigation measures that end-user could apply on the field in order to achieve the desired performances of the soil functions.
Soil Function Decision Models
In this section, we provide a brief overview of the individual soil function decision models. Since the decision models of soil functions should address both cropland and grassland soils, some of the decision models have been split into two separate decision models, one for cropland and one for grassland. By doing so, the sensitivity of the outputs for changes in the input data has been increased. The detailed descriptions of each model are provided in separate papers in this issue (Rutgers et al., 2019; Sandén et al., 2019; Delgado et al., unpublished2; Van de Broek et al., unpublished1). The model for nutrient cycling was developed earlier and published by Schröder et al. (2016).
The primary productivity decision model consists of sub-models describing the environmental conditions (E), inherent soil conditions (S) (physical: structure, groundwater table depth; chemical: micro- and macro-elements; biological: pH, C/N ratio, soil organic matter), soil management (M), and crop properties (C). Primary productivity, as the top attribute, integrates the sub-models, which leads to an assessment of the capacity of a soil to produce biomass. A detailed description of the primary productivity model is given in Sandén et al. (2019).
The structure of the nutrient cycling decision model consists of three sub-models, integrated into the top attribute, describing the ability of a soil to provide and cycle nutrients. The first sub-model comprises nutrient fertilizer replacement value, which describes the extent to which nutrients, particularly those in left or applied organic residues, are as available to plants as manufactured mineral fertilizers. The second part of the model describes the extent to which plant-available nutrients are effectively taken up by crops and the last part addresses the harvest index describing the extent to which the nutrients taken up by crops are eventually leaving the field in the form of successful harvests (Schröder et al., 2018).
The climate regulation and carbon sequestration decision model integrates carbon sequestration, N2O emissions and CH4 emissions. The carbon sequestration sub-model is determined by the magnitude of carbon inputs, carbon losses, and the soil organic carbon concentration. The N2O emissions sub-model makes a distinction between direct N2O emissions occurring on agricultural fields, and indirect N2O emissions, after reactive N species have been transported through the landscape. The part of the model addressing CH4 emissions are determined by the extent to which artificial drainage is applied on organic soils. Detailed information about the model are given in Van de Broek et al. (unpublished)1.
The water regulation and purification soil function decision model integrates three sub-models describing the prevailing soil water pathways: water storage, water runoff, and water percolation. Water storage is determined by the attributes used for assessing the water holding capacity and soil moisture deficit. Water runoff is determined by the attributes used for assessing the water-, sediment-, and nutrient-related runoff. The water percolation sub-model is determined by the attributes used for assessing the resulting drainage of excess of water above that potentially stored in the soil and the resulting nutrient leaching and losses (Wall et al., 2018).
The soil biodiversity and habitat provisioning decision model integrates four sub-models describing soil nutrients (status, trends, turnover, and nutrients availability), soil biology (available information on diversity, biomass, and activity of soil organisms), soil structure [structure and density, ranging from mesoscale (coarse fractions, soil particles, organic matter, air, and water-filled space) to macroscale (soil layers, terrain, slope)], and soil hydrology (soil humidity and the soil water flow pathways) (Rutgers et al., 2019).
The structural properties of the DEX decision models of all five soil functions are given in Table 1. All decision models have similar hierarchical structure (number of hierarchical levels), as well as the number of basic attributes. From the number of integration rules, it is evident that the water regulation and purification and the biodiversity and habitat models are more complex than the others, because of the total number of attributes and their scales of values. However, the decision models for all five soil functions use the same subset of basic attributes, so the total number of distinctive input attributes for all decisions models is 75.
Graphical User Interface
The GUI enables the interactions between the user and the DSS through a series of steps: (i) data entry, (ii) specification of soil function preferences, and (iii) selection of the changes of factors or states in an agricultural field. The development and testing of the GUI was based on end-user preferences indicated in the surveys and follow-up interviews with the members of NRGs for the Soil Navigator.
The data entry form (Figure 4) allows the user to provide all available data for a particular agricultural field. The required set of input data includes data about the agroecosystem, environment, soil, and management (rightmost column, Figure 4). The middle panel shows the input forms for the required attributes within each of the data categories, while the field “Scenario” (at the top of the page) allows the user to specify a name for the particular scenario under consideration. The DSS has an option of importing data from the external corresponding databases.
The user is required first to specify his preferred soil function (Figure 5) that is based on the outputs from the assessment of the soil functions (given on the right side of the screen) and includes an input form for specifying a preference for improvement the performance of one or more soil functions (bottom panel). The preferences can be specified through the given sliders that have a value corresponding to the initially assessed level of performance.
The final step/output that is presented through the Soil Navigator GUI is the proposed set of suggestions for the improvement of the performance of a soil function (Figure 6). In this step, the user can also request to find other suggestions if the ones that are offered do not satisfy the user's expectations and possibilities. All input data for an assessed field and the suggestions for the improvement of its soil functions can be saved and later used for the purpose of validation of the results, as well as its re-evaluation in the future.
In Figures 4–6, we present the Soil Navigator GUI using a scenario that involves an agricultural field located in Germany, within the Central Atlantic climatic zone. The purpose of the land use is crop production within a mixed farm type (crop and livestock production). Figure 4 shows some of the input data for the soil from the selected field.
In the second step (Figure 5), the system provides an initial assessment of the performance of all five soil functions. The soil functions primary productivity and water regulation and purification are assessed as most efficient (high performance capacity), climate regulation and carbon sequestration as medium-scaled performance, and cycling of nutrients along with soil biodiversity and habitat provision as lowest level of performance (low performance). Consequently, the overall improvement can be achieved by improving the performance of the last three functions. In the given example (Figure 5), the user selected the function Nutrient Cycling as the only function of interest and target/level for improvement of the capacity of this soil function to medium performance level (visually given on the bar chart with violet color with reduced opacity).
In the final step, the system performs a search of all possible combinations of values of the input attributes in order to identify a smaller number of combinations that would improve the capacity of the soil to deliver the nutrient cycling function. When a suitable combination is found, the bar chart in the top right corner shows more colors (Figure 6). The inner rectangles with bold colors represent the initial assessment. The outer rectangles show the user's preferences, stated in the previous step, the borders of which are bolded only to those that are successfully improved. In our case, the system found a solution, which would improve the performance of the function cycling of nutrients, without compromising the other functions. The solution shows that the share of legumes needs to be increased drastically in order to achieve the desired performance of the soil functions in this particular field.
Discussion
Jones et al. (2017a,b) highlighted the lack of integrated DSS for farm system management. They envisioned a DSS platform that connects various models, databases, analyses, and information synthesis tools in an easy-to-use interface to enable analyses and outputs to answer questions relating to the management of particular farming “systems” biophysical resources and/or socio-economic situations. Jones et al. (2017a,b) concluded that such DSS are required, but still not developed. The Soil Navigator DSS encompasses the above-listed components, performs similar tasks, and communicates with the end-users through user-friendly graphical interface designed according to Rose et al. (2016). Furthermore, the Soil Navigator meets the documented needs for a DSS that will assist farmers and advisors to achieve sustainability of the agricultural landscape (Eichler Inwood and Dale, 2019), by enabling field-specific assessment and the enhancement of five soil functions simultaneously while integrating sustainability concerns from multiple dimensions or themes. In addition, the Soil Navigator DSS has the potential to complement the FaST tools required by the proposal on the 2021–2027 CAP (European Commission, 2018). As part of the GAESs framework, farmers will be required by Member States to use FaST tools in order to establish nutrient management plans and support the agronomic and the environmental performance on their farms. The tool should provide on-farm decision support featuring minimum nutrient management functionalities. However, the capacity of a soil to provide and recycle nutrients is determined not only by nutrient management practices but also by environmental or climatic/weather conditions and farm- or soil-related management practices. This implies that for the same level of functioning, if attainable at all, soils will require different managements under different pedo-climatic conditions.
Another consequence of the interplay of factors is that some environments are better suited to perform certain functions and deliver specific services than others, regardless of management efforts. Decisions favoring nutrient cycling may compromise one or more other functions, as for example increased cycling of phosphorus (P) nutrient may have negative consequences for the quality of water (water purification function) even if losses from the soil are relatively small. This complicates the decision making process even further. Consequently, there is no such thing as a one size (or soil) fits all soil strategy, which is in line with the findings of Sandén et al. (2018). Decisions must therefore be based on careful considerations accounting for local demands, their soils' potential to deliver functions and even ecosystem services, as well as synergies and trade-offs between soil functions and the weightings of alternative options for achieving these services.
It is in this space that the Soil Navigator DSS could support the objectives of the CAP post-2020. Based on the European Commission commitment to make FaST interoperable and modular, it should be possible to couple the Soil Navigator DSS with FaST. Whereas, FaST is focusing on nutrients, the Soil Navigator DSS could make it possible for the farmer to perform a combined assessment and optimization of nutrient cycling, primary productivity, biodiversity and habitat provisioning, water regulation and purification, and climate regulation and carbon sequestration. In addition, farmers will be able to assess the potential change in GHG emission as a consequence of the management they apply, and to make them aware of trade-offs between, e.g., C sequestration and N2O emissions. Obvious trade-offs occur, e.g., between application of fertilizer and manure, leading to increased carbon sequestration on one side and potentially leading to increased N2O emissions on the other side, if not managed correctly (Tubiello et al., 2015; Zhou et al., 2017; Lugato et al., 2018). Thus, the Soil Navigator could facilitate activities that will reduce the impact of agricultural sector on climate change and provide support actions to achieve the European Union commitments under the Paris Agreement (United Nations/Framework Convention on Climate Change, 2015).
Besides the potential to integrate the Soil Navigator in the CAP post-2020, there is also potential to use the DEX models at larger spatial scales (e.g., regional or European) in order to improve the provision of soil functions in a spatially explicit context. Such an application of the developed DEX models could be used to indicate which soil functions should be prioritized by a specific region or member state. However, in order to produce reliable results, the different DEX models would have to be adjusted to match the specific scale. This can be handled easily, since the embedded DEX models can be improved upon request (e.g., for a higher tier assessment, other systems, such as forestry). By applying a set of harmonized models, it is possible to use the available data and knowledge as efficient as possible.
The Soil Navigator DSS also has the potential to function as an educational tool for farmers, farm advisors, and students. The Soil Navigator DSS presents an opportunity to gain knowledge about different soil functions and how they are affected by management strategies under certain soil and environmental conditions. The tool could potentially guide discussions between the farmers and farm advisors and demonstrate that primary productivity is closely linked with other soil functions. The stakeholders would be able to visualize the effect of the implementation of a specific management practice not only toward primary productivity but also toward the performance of other soil functions. Such demonstrations may incentivize farmers to obtain the data needed to run more specific Soil Navigator scenarios for particular farms or soil conditions in order to obtain more reliable results (e.g., soil pH, organic matter content, or soil texture). The Soil Navigator DSS could also be linked to regional soil maps and thereby educate the farmers about new sources of information. Finally, it can be used as a tool to assess the influence of the global climatic changes on the soil functions, which will enable experts to perform risk assessment and risk management and to propose practical and effective climate adaptation measures for farmers and other stakeholders.
As outlined in this paper, the integrated field-scale assessment and optimization of soil functions delivered by the Soil Navigator DSS have many different potential applications that should be further exploited for the benefit of farmers and the society as a whole.
Data Availability
The datasets for this manuscript are not publicly available because they contain personal data about applied soil management practices. Requests to access the datasets should be directed to RC, cmFjaGVsLmNyZWFtZXJAd3VyLm5s.
Author's Note
This article resulted from cooperation with soil functions' task groups in the LANDMARK H2020 project.
Author Contributions
JS, TS, HS, DW, MV, and MR contributed to the development of the soil function decision models. MD, AT, VK, and CH developed the decision support system, while FB and RC contributed to reading and structuring of the paper. MD and AT wrote most of the paper with major inputs from VK, CH, JS, FB, TS, HS, MV, MR, and RC.
Funding
This research was conducted as part of the LANDMARK (LAND Management: Assessment, Research, Knowledge Base) project. This project has received funding from the project LANDMARK within the European Union's Horizon 2020 research and innovation program under the Grant Agreement 635201.
Conflict of Interest Statement
The authors declare that the research was conducted in the absence of any commercial or financial relationships that could be construed as a potential conflict of interest.
Footnotes
1. ^Van de Broek, M., Henriksen, C. B., Ghaley, B. B., Lugato, E., Kuzmanovski, V., Trajanov, A., et al. (unpublished). Assessing the climate regulation potential of agricultural soils using a decision support tool adapted to stakeholders’ needs and possibilities. Front. Environ. Sci. 7.
2. ^Delgado, A., O’Sullivan, L., Debeljak, M., Creamer, R. E., Henriksen, C. B., Wall, D. P. (unpublished). Farming systems targeted to water regulation and purification in agricultural soils. Front. Environ. Sci.
References
Adhikari, K., and Hartemink, A. E. (2016). Linking soils to ecosystem services—A global review. Geoderma 262, 101–111. doi: 10.1016/j.geoderma.2015.08.009
AGES (2019). AgrarCommander. Available online at: https://dev.moneysoft.at/cgi-bin/agrar/ages/acages.cgi
APCA (2019). Mes Parcelles. Available online at: https://chambres-agriculture.fr/chambres-dagriculture/nos-missions-et-prestations/nos-marques/mes-parcelles/
Bampa, F., Creamer, R. E., O'Sullivan, L., Madena, K., Sandén, T., Spiegel, H., et al. (2019). Harvesting European knowledge on soil functions and land management using multi criteria decision analysis. Soil Use Manag. 35, 6–20. doi: 10.1111/sum.12506
Baveye, P. C., Baveye, J., and Gowdy, J. (2016). Soil “ecosystem” services and natural capital: critical appraisal of research on uncertain ground. Front. Environ. Sci. 4:e00041. doi: 10.3389/fenvs.2016.00041
Bligaard, J. (2014). Mark online, a full scale GIS-based Danish farm management information system. Int. J. Food Syst. Dyn. 5, 190–195. doi: 10.18461/ijfsd.v5i4.544
Blum, W. E. H. (2005). Functions of soil for society and the environment. Rev. Environ. Sci. Biotechnol. 4, 75–79. doi: 10.1007/s11157-005-2236-x
Blum, W. E. H., Büsing, J., and Montanarella, L. (2004). Research needs in support of the European thematic strategy for soil protection. Trends Analyt. Chem. 23, 680–685. doi: 10.1016/j.trac.2004.07.007
Bohanec, M. (2017). “Multi-criteria DEX model: an overview and analysis,” in SOR ‘17 Proceedings (Ljubljana: Slovenian Society Informatika, Section for Operational Research).
Bohanec, M. (2019). DEXi: A Program for Multi-attribute Decision Making. Available online at: http://kt.ijs.si/MarkoBohanec/dexi.html
Bohanec, M., and Rajkovič, V. (1990). DEX: an expert system shell for decision support. Sistemica 1, 145–157.
Bohanec, M., Žnidaršič, M., Rajkovic, V., Bratko, I., and Zupan, B. (2013). DEX methodology: three decades of qualitative multi-attribute modeling. Informatica 37, 49–54.
Bohanec, M., and Zupan, B. (2004). A function-decomposition method for development of hierarchical multi-attribute decision models. Decis. Support Syst. 36, 215–233. doi: 10.1016/s0167-9236(02)00148-3
Bondi, G., Creamer, R., Ferrari, A., Fenton, O., and Wall, D. (2018). Using machine learning to predict soil bulk density on the basis of visual parameters: tools for in-field and post-field evaluation. Geoderma 318, 137–147. doi: 10.1016/j.geoderma.2017.11.035
Bouma, J., Broll, G., Crane, T. A., Dewitte, O., Gardi, C., Schulte, R. P. O., et al. (2012). Soil information in support of policy making and awareness raising. Curr. Opin. Environ. Sustain. 4, 552–558. doi: 10.1016/j.cosust.2012.07.001
Bünemann, E. K., Bongiorno, G., Bai, Z., Creamer, R. E., De Deyn, G., de Goede, R., et al. (2018). Soil quality—A critical review. Soil Biol. Biochem. 120, 105–125. doi: 10.1016/j.soilbio.2018.01.030
Creamer, R., and Holden, N. (2010). Special issue: soil quality. Soil Use Manag. 26, 197–197. doi: 10.1111/j.1475-2743.2010.00299.x
Creamer, R. E., Brennan, F., Fenton, O., Healy, M. G., Lalor, S. T. J., Lanigan, G. J., et al. (2010). Implications of the proposed Soil Framework Directive on agricultural systems in Atlantic Europe—A review. Soil Use Manag. 26, 198–211. doi: 10.1111/j.1475-2743.2010.00288.x
Dicks, L. V., Walsh, J. C., and Sutherland, W. J. (2014). Organising evidence for environmental management decisions: a ‘4S’ hierarchy. Trends Ecol. Evol. 29, 607–613. doi: 10.1016/j.tree.2014.09.004
Eichler Inwood, S. E., and Dale, V. H. (2019). State of apps targeting management for sustainability of agricultural landscapes. A review. Agron. Sustain. Dev. 39:8. doi: 10.1007/s13593-018-0549-8
European Commission (2006). Proposal of a Directive of the European Parliament and of the Council 2006 Establishing a Framework for the Protection of Soil and Amending Directive 2004/35/EC. Brussels: Commission of the European Communities.
European Commission (2018). Proposal for a Regulation of the European Parliament and of the Council Establishing Rules on Support for Strategic Plans to be Drawn up by Member States under the Common Agricultural Policy (CAP Strategic Plans) and financed by the European Agricultural Guarantee Fund (EAGF) and by the European Agricultural Fund for Rural Development (EAFRD)—COM/2018/392 final−2018/0216 (COD). Brussels: Commission of the European Communities.
Greco, S., Ehrgott, M., and Figueira, J. (eds.). (2016). “Multiple criteria decision analysis: state of the art surveys,” in International Series in Operations Research & Management Science, Vol. 233 (New York, NY: Springer), 1347.
Hättenschwiler, P. (1999). “Neues anwenderfreundliches Konzept der Entscheidungsunterstützung,” in Gutes Entscheiden in Wirtschaft, Politik und Gesellschaft (Zurich: vdf Hochschulverlag AG), 189–208.
Haygarth, P. M., and Ritz, K. (2009). The future of soils and land use in the UK: soil systems for the provision of land-based ecosystem services. Land Use Policy 26, S187–S197. doi: 10.1016/j.landusepol.2009.09.016
Jakku, E., and Thorburn, P. J. (2010). A conceptual framework for guiding the participatory development of agricultural decision support systems. Agric. Syst. 103, 675–682. doi: 10.1016/j.agsy.2010.08.007
Jones, J. W., Antle, J. M., Basso, B., Boote, K. J., Conant, R. T., Foster, I., et al. (2017a). Brief history of agricultural systems modeling. Agric. Syst. 155, 240–254. doi: 10.1016/j.agsy.2016.05.014
Jones, J. W., Antle, J. M., Basso, B., Boote, K. J., Conant, R. T., Foster, I., et al. (2017b). Toward a new generation of agricultural system data, models, and knowledge products: state of agricultural systems science. Agric. Syst. 155, 269–288. doi: 10.1016/j.agsy.2016.09.021
Jørgensen, S. E., and Fath, B. D. (2011). Fundamentals of Ecological Modelling: Applications in Environmental Management and Research. Amsterdam: Elsevier.
Kangas, A., Kurttila, M., Hujala, T., Eyvindson, K., and Kangas, J. (2015). Decision Support for Forest Management. Heildeberg: Springer.
Lindblom, J., Lundström, C., Ljung, M., and Jonsson, A. (2017). Promoting sustainable intensification in precision agriculture: review of decision support systems development and strategies. Precis. Agric. 18, 309–331. doi: 10.1007/s11119-016-9491-4
Lohan, D. J., Dede, E. M., and Allison, J. T. (2016). Topology optimization for heat conduction using generative design algorithms. Struct. Multidiscipl. Optim. 55, 1063–1077. doi: 10.1007/s00158-016-1563-6
Lugato, E., Leip, A., and Jones, A. (2018). Mitigation potential of soil carbon management overestimated by neglecting N2O emissions. Nat. Clim. Chang. 8, 219–223. doi: 10.1038/s41558-018-0087-z
LWK Niedersachsen (2019). Web Module Düngung. Available online at: https://www.lwk-niedersachsen.de/index.cfm/portal/2/nav/342/article/11632.html
Mallach, E. G. (1994). Understanding Decision Support Systems and Expert Systems. Boston, MA: Irwin.
Mendoza, G. A., and Martins, H. (2006). Multi-criteria decision analysis in natural resource management: a critical review of methods and new modelling paradigms. For. Ecol. Manag. 230, 1–22. doi: 10.1016/j.foreco.2006.03.023
Metzger, M. J., Bunce, R. G. H., Jongman, R. H. G., Mücher, C. A., and Watkins, J. W. (2005). A climatic stratification of the environment of Europe. Global Ecol. Biogeogr. 14, 549–563. doi: 10.1111/j.1466-822X.2005.00190.x
Micheli, E., Szegi, T., Csorba, A., Bardy, M., Saby, N., Drufin, S., et al. (2017). Definition of Required Minimum Set of Data. Available online at: https://landmark2020.bitrix24.com/~jIqmm
Olesen, J. E., Trnka, M., Kersebaum, K. C., Skjelvåg, A. O., Seguin, B., Peltonen-Sainio, P., et al. (2011). Impacts and adaptation of European crop production systems to climate change. Eur. J. Agron. 34, 96–112. doi: 10.1016/j.eja.2010.11.003
Power, D. J. (2019). Free Decision Support Systems Glossary. Available online at: http://dssresources.com/dssbook/glossary.pdf
Rodela, R., Bregt, A. K., Ligtenberg, A., Pérez-Soba, M., and Verweij, P. (2017). The social side of spatial decision support systems: investigating knowledge integration and learning. Environ. Sci. Policy 76, 177–184. doi: 10.1016/j.envsci.2017.06.015
Rose, D. C., Sutherland, W. J., Parker, C., Lobley, M., Winter, M., Morris, C., et al. (2016). Decision support tools for agriculture: towards effective design and delivery. Agric. Syst. 149, 165–174. doi: 10.1016/j.agsy.2016.09.009
Rutgers, M., Van Leeuwen, J. P., Creamer, R. E., Cluzeau, D., Debeljak, M., Gatti, F., et al. (2019). Modelling of soil functions for assessing soil quality: soil biodiversity and habitat provisioning. Front. Environ. Sci. 7:00113. doi: 10.3389/fenvs.2019.00113
Rutgers, M., van Wijnen, H. J., Schouten, A. J., Mulder, C., Kuiten, A. M., Brussaard, L., et al. (2012). A method to assess ecosystem services developed from soil attributes with stakeholders and data of four arable farms. Sci. Total Environ. 415, 39–48. doi: 10.1016/j.scitotenv.2011.04.041
Saaty, T. L. (1990). Multicriteria Decision Making: The Analytic Hierarchy Process. Pittsburgh, PA: RWS Publications.
Saby, N. P. A., Csorba, A., Szegi, T., Micheli, E., Trajanov, A., Debeljak, M., et al. (2018). Data Management Plan. Available online at: https://landmark2020.bitrix24.com/~K601p
Sandén, T., Spiegel, H., Stüger, H. P., Schlatter, N., Haslmayr, H. P., Zavattaro, L., et al. (2018). European long-term field experiments: knowledge gained about alternative management practices. Soil Use Manag. 34, 167–176. doi: 10.1111/sum.12421
Sandén, T., Trajanov, A., Spiegel, H., Kuzmanovski, V., Saby, N. P. A., Picaud, C., et al. (2019). Development of an agricultural primary productivity decision support model: a case study in France. Front. Environ. Sci. 7:58. doi: 10.3389/fenvs.2019.00058
Schröder, J. J., Schulte, R. P. O., Creamer, R. E., Delgado, A., van Leeuwen, J., Lehtinen, T., et al. (2016). The elusive role of soil quality in nutrient cycling: a review. Soil Use Manag. 32, 476–486. doi: 10.1111/sum.12288
Schröder, J. J., Schulte, R. P. O., Sandén, T., Creamer, R. E., van Leeuwen, J., Rutgers, M., et al. (2018). Project Glossary: Definition of Common Terms and Concepts in Relation to Soil Functions and Soil Quality. LANDMARK Report 1.1.
Schulte, R. P. O., Bampa, F., Bardy, M., Coyle, C., Creamer, R. E., Fealy, R., et al. (2015). Making the most of our land: managing soil functions from local to continental scale. Front. Environ. Sci 3:e00081. doi: 10.3389/fenvs.2015.00081
Schulte, R. P. O., Creamer, R. E., Donnellan, T., Farrelly, N., Fealy, R., O'Donoghue, C., et al. (2014). Functional land management: a framework for managing soil-based ecosystem services for the sustainable intensification of agriculture. Environ. Sci. Policy 38, 45–58. doi: 10.1016/j.envsci.2013.10.002
Shaw, M. L. G., and Woodward, J. B. (1990). Modeling expert knowledge. Knowl. Acquis. 2, 179–206. doi: 10.1016/S1042-8143(05)80015-9
Stankovics, P., Tóth, G., and Tóth, Z. (2018). Identifying gaps between the legislative tools of soil protection in the EU member states for a common European soil protection legislation. Sustainability 10:2886. doi: 10.3390/su10082886
Stolte, J. M., Tesfai, L., Øygarden, S., Kværnø, J., Keizer, F., Verheijen, P., et al. (2016). Soil threats in Europe. EU Joint Research Centre.
Teagasc (2016). NMP Online User Manual. Available online at: https://www.teagasc.ie/media/website/environment/soil/NMP_User_Manual_2016__D5.pdf
ten Berge, H. F. M., Schröder, J. J., Oleson, J. E., and Giraldez-Cervera, J. V. (2017). Preserving Agricultural Soils in the EU. Report for European Parliament Committee for Agriculture and Rural Development, Directorate-General for Internal Policies. Bruxelles: Policy Department for Structural and Cohesion Policies.
Trajanov, A., Kuzmanovski, V., Leprince, F., Real, B., Dutertre, A., Maillet-Mezeray, J., et al. (2015). Estimating drainage periods for agricultural fields from measured data: data-mining methodology and a case study (La JailliÈRe, France). Irrig. Drain. 64, 703–716. doi: 10.1002/ird.1933
Trajanov, A., Kuzmanovski, V., Real, B., Perreau, J. M., Dzeroski, S., and Debeljak, M. (2018). Modeling the risk of water pollution by pesticides from imbalanced data. Environ. Sci. Pollut. Res. Int. 25, 18781–18792. doi: 10.1007/s11356-018-2099-7
Tubiello, F. N., Salvatore, M., Ferrara, A. F., House, J., Federici, S., Rossi, S., et al. (2015). The contribution of agriculture, forestry and other land use activities to global warming, 1990–2012. Glob. Chang. Biol. 21, 2655–2660. doi: 10.1111/gcb.12865
Turban, E., Aronson, J. E., and Liang, T. P. (2004). Decision Support Systems and Intelligent Systems. 7th Edn. Upper Saddle River, NJ: Prentice-Hall Inc.
Tversky, A., and Kahneman, D. (1974). Judgment under uncertainty: heuristics and biases. Science 185:1124. doi: 10.1126/science.185.4157.1124
United Nations/Framework Convention on Climate Change (2015). “Adoption of the Paris Agreement,” in 21st Conference of the Parties (Paris).
Uusitalo, L., Lehikoinen, A., Helle, I., and Myrberg, K. (2015). An overview of methods to evaluate uncertainty of deterministic models in decision support. Environ. Modell. Softw. 63, 24–31. doi: 10.1016/j.envsoft.2014.09.017
Vogel, H.-J., Wollschläger, U., Helming, K., Heinrich, U., Willms, M., Wiesmeier, M., et al. (2019). “Assessment of soil functions affected by soil management,” in Atlas of Ecosystem Services, eds M. Schröter, A. Bonn, S. Klotz, R. Seppelt, and C. Baessler (Cham: Springer), 77–82. doi: 10.1007/978-3-319-96229-0_13
Wall, D., O'Sullivan, L., Debeljak, M., Trajanov, A., Schröder, J. E., Bugge Henriksen, C., et al. (2018). Key Indicators and Management Strategies for Water Purification and Regulation. Available online at: https://landmark2020.bitrix24.com/~FdtnT
Keywords: soil functions, field scale, decision support system, multi-criteria decision models, method DEX, soil management
Citation: Debeljak M, Trajanov A, Kuzmanovski V, Schröder J, Sandén T, Spiegel H, Wall DP, Van de Broek M, Rutgers M, Bampa F, Creamer RE and Henriksen CB (2019) A Field-Scale Decision Support System for Assessment and Management of Soil Functions. Front. Environ. Sci. 7:115. doi: 10.3389/fenvs.2019.00115
Received: 21 February 2019; Accepted: 04 July 2019;
Published: 05 August 2019.
Edited by:
Philippe C. Baveye, AgroParisTech Institut des Sciences et Industries du Vivant et de L'environnement, FranceReviewed by:
Juan José Jiménez, Instituto Pirenaico de Ecología (CSIC), SpainZamir Libohova, United States Department of Agriculture (USDA), United States
Copyright © 2019 Debeljak, Trajanov, Kuzmanovski, Schröder, Sandén, Spiegel, Wall, Van de Broek, Rutgers, Bampa, Creamer and Henriksen. This is an open-access article distributed under the terms of the Creative Commons Attribution License (CC BY). The use, distribution or reproduction in other forums is permitted, provided the original author(s) and the copyright owner(s) are credited and that the original publication in this journal is cited, in accordance with accepted academic practice. No use, distribution or reproduction is permitted which does not comply with these terms.
*Correspondence: Marko Debeljak, bWFya28uZGViZWxqYWtAaWpzLnNp