- 1Earth System Science Center, University of Alabama in Huntsville, Huntsville, AL, United States
- 2NASA-SERVIR Science Coordination Office, Huntsville, AL, United States
- 3Centro de Estudios de Atitlán, Universidad del Valle de Guatemala, Solola, Guatemala
- 4Autoridad para el Manejo del Lago de Peten Itza, Flores, Guatemala
- 5Authority for the Sustainable Management of Lake Atitlán Basin and its Environment (AMSCLAE), Solola, Guatemala
- 6Water Resources Center, University of Minnesota, Minneapolis, MN, United States
In this study we evaluated the applicability of a space-borne hyperspectral sensor, Hyperion, to resolve for chlorophyll a (Chl a) concentration in Lake Atitlan, a tropical mountain lake in Guatemala. In situ water quality samples of Chl a concentration were collected and correlated with water surface reflectance derived from Hyperion images, to develop a semi-empirical algorithm. Existing operational algorithms were tested and the continuous bands of Hyperion were evaluated in an iterative manner. A third order polynomial regression provided a good fit to model Chl a. The final algorithm uses a blue (467 nm) to green (559 nm) band ratio to successfully model Chl a concentrations in Lake Atitlán during the dry season, with a relative error of 33%. This analysis confirmed the suitability of hyperspetral-imagers like Hyperion, to model Chl a concentrations in Lake Atitlán. This study also highlights the need to test and update this algorithm with operational multispectral sensors such as Landsat and Sentinel-2.
Introduction
Fresh water bodies provide multiple services ranging from recreation to ecological and economical. In Guatemala, the combination of poor development planning, lack of sewage treatment infrastructure, and overuse of land for agriculture with absent soil protective practices, has led to the degradation of inland water bodies (Perez Gudiel, 2007; Pérez et al., 2011; Romero-Oliva et al., 2014). Lake Atitlán, located in the highlands of Guatemala (14.68 N, 91.16 W) exemplifies a fresh water body subjected to pressures that have increased over the years. Lake Atitlán is the second most visited tourist attraction in the country, as such represents the livelihood of communities located around it. The 15 municipalities in Lake Atitlán's watershed hold about 368,000 inhabitants (INE, n.d.; AMSCLAE, 2017), from which about 170,000 inhabitants are surrounding the lake (INE, n.d.; SIGSA, 2019). In October 2009 the lake experienced a never before seen algal bloom that lasted about 2 months. At its highest point this algal bloom covered about 40% of the 132 square kilometers lake's surface. The bloom was caused by cyanobacteria, first tentatively identified as Lyngbya robusta (Rejmánková et al., 2011) and later as Limnoraphis robusta (Komárek et al., 2013). This bloom affected the local economy as tourism came to a halt. In addition, the lake is a direct source of drinking water, use with no purification, for two of the municipalities around the lake (Romero Santizo, 2009; Dix et al., 2012b).
The data available for the lake show its degradation overtime (Chandra et al., 2013; AMSCLAE, 2017). Lake Atitlán is considered a unique example of an oligotrophic lake, given its unusual transparencies (Secchi disk transparency) that in the 1960s were as high as 20 m (Weiss, 1971) and in 2010 as high as 15 m (Dix et al., 2012a). The scientific data available for the lake, even though existent, is confined to a few monitoring points measured over a few months per year. Due to limited resources the data is not published on the regular basis. The two most complete studies published for Lake Atitlán's water quality are from Weiss (1971) and Dix et al. (2012a) and both are based on in situ standard water quality monitoring methods. Both studies report and provide evidence of the oligotrophic condition of the lake during the dry season. However, there exists a significant time gap between both studies. Weiss and Dix's year long studies showed that the optical properties of Lake Atitlán such as transparency (measured by Secchi disk), vary by season. The rainy season had lower transparencies than the dry season. Weiss found maximum transparencies of 22 m in February 1969, in a station equidistant from San Pablo, San Pedro, and San Marcos towns and Dix et al. (2012a) maximum of 15.5 m in March 2010, at the center of the lake in the station Center Weiss G.
The limited published information available undermines the understanding of the factors promoting the degradation of the lake and the intrinsic behavior and dynamics of Lake Atitlán. Despite recent efforts to invest more in science and technology in Guatemala (UNESCO, 2015), funding for water quality monitoring is minimal. In addition, standard methods to monitor water quality are generally expensive, time consuming and require special equipment and trained personnel, which consequently provides data with limited spatial coverage and temporal frequency (Palmer et al., 2015). Earth observing satellites, on the other hand, can provide a cost-effective solution to Guatemalan authorities and academia to complement water quality measurements on the ground. Several studies exemplified how satellite data can be used for water quality monitoring in inland water bodies, particularly algal bloom monitoring (Vincent et al., 2004; Ogashawara and Moreno-Madriñán, 2014; Watanabe et al., 2015; Page et al., 2018). During Lake Atitlán's algal bloom in 2009 images from NASA Earth observing satellites, such as Landsat, Advance Spaceborne Thermal Emission and Reflection Radiometer (ASTER) and Earth Observing 1 (EO-1) were used to estimate the extent and progression of the algal bloom and provided authorities and general public a complete picture of the event (SERVIR, 2009). In 2011, satellite images were also used to study water surface extent and other minor algal blooms events for the same lake (SERVIR, 2011). Thus far, the common factor among the different analyses using satellite imagery for this region has been their qualitative nature. Still, satellite remote sensing can be more beneficial and actually represent a reliable, quantitative source of water quality parameters that can effectively complement the in situ data collected. In addition, the use of satellite imagery to estimate water quality parameters can increase the timeliness of information and can also provide estimates for the entire water body and not only from single points of measurement. To evaluate if the combination of in situ measurements and satellite imagery can enhance our understanding of lake's Atitlán dynamics, the present study is aimed to evaluate satellite remote sensing to estimate water quality parameters, specifically chlorophyll-a (Chl a) concentration. Chlorophyll concentration is an indirect measurement of phytoplankton biomass (Schalles, 2006). Chl a is a dominant light harvesting pigment and is universally present in eukaryotic algae and cyanobacteria (Rowan, 1989). Therefore, all algae, whether toxic or non-toxic, have Chl a. In this study we evaluate the applicability of a space-borne hyperspectral sensor, Hyperion, to resolve for Chl a concentration. Hyperion was a hyperspectral imager on board of the EO-1 satellite. It is foreseen that this study will contribute to the transition of remote sensing applications from a qualitative to a quantitative nature for algal bloom monitoring and assessment in Guatemala. In addition, this study provides valuable information on the capabilities of hyperspectral satellite data for Chl a concentration retrieval, relevant to ongoing and future hyperspectral satellite missions.
Satellite Remote Sensing and Chlorophyll Algorithms
The Chl a algorithms in ocean waters are based on a simple interaction of phytoplankton density with water, in which usually blue to green band ratios have a robust and sensitive relation to Chl a during low concentrations 1–30 mg/m3 situations. This relationship becomes less sensitive at higher Chl a concentrations (above 30 mg/m3Chla) and is highly compromised by the effects of colored dissolved organic matter (CDOM) in turbid and optically complex waters (Schalles, 2006). According to Schalles (2006) and Mobley (1995) (1) extremely low Chl a concentrations <2 mg/m3Chla show higher reflectances in the blue part of the spectrum (400–500 nm) and reflectance decreases as wavelength increases, with extremely low reflectance values, near to 0, in the NIR (700–800 nm); (2) Chl a concentrations between 2 and 30 mg/m3 show higher reflectances in the green (500–600 nm) and red bands (600–700 nm), with peak reflectance in the green part of the spectrum; and (3) higher Chl a concentrations, > 300 mg/m3, show peak reflectances in the NIR and minimum high in the green part of the spectrum, the blue and red bands show low reflectances. These principles are used to select bands and develop algorithms to retrieve Chl a from satellite images, since it is evident that spectral signature changes depending on the content of Chl a in water. Usually local-based algorithms are needed for inland water bodies, and they vary significantly from one site to another since their development is based on the specific optical constituents of a water body. The measurement of Chl a in water is commonly used (a) as an indicator to monitor water quality programs in coastal and inland waters, (b) in surveillance programs of harmful algal blooms, (c) and in ecological studies of phytoplankton biomass and productivity (Jordan et al., 1991; Morrow et al., 2000). Moreover, Chl a has also been used as an indicator of cyanobacteria (Ogashawara and Moreno-Madriñán, 2014). Satellite remote sensing has been used for decades to estimate Chl a concentrations, most notably with operational applications in the oceans (Mobley, 1995; O'Reilly et al., 1998; Schalles, 2006; Hu et al., 2012). However, significant progress has been made in applying satellite remote sensing in inland water bodies with positive outcomes as described in Palmer et al. (2015) and Bukata (2013). The main challenge to use remote sensing is to isolate the Chl a signal from other cell components and other optically active compounds and the effects of the vertical distribution variation of chlorophyll in the water column.
The first satellite sensor developed to evaluate water properties, particularly Chl a concentration was the Coastal Zone Color Scanner (CZCS) on board Nimbus 7 and launched in late 1978. A two-band ratio of 443–550 nm was calibrated and routinely used for Chl a estimation (O'Reilly et al., 1998). Later, another two operational sensors were also designed to monitor Chl a estimations using bands in the blue and the green regions (Sea-viewing Field of view sensor—SeaWIFS- and Moderate resolution Imaging Spectroradiometer — MODIS-) (O'Reilly et al., 1998; Schalles, 2006). The current operational algorithm used for Chl a estimation has been updated for the sixth time (version 6) and is generated by the NASA Ocean Biology Processing Group (OBPG). These algorithms are based on a multi-band optimization procedure called OC4 (for Ocean Color 4) and their approach is termed Maximum Band Ratio (MBR).
These operational algorithms are based on comparing blue to green ratios. The largest value of the ratios is used in a fourth order polynomial regression equation as the exponential term in a power function equation. These exponential equations best represent the sigmoidal relationship between Chl a and band ratio calculations (O'Reilly et al., 1998).
The operational algorithms for Chl a concentration estimations are based on blue to green band ratios and have been generated for oceanic waters which color is dominated by phytoplankton. The good performance of blue and green ratios in oceanic waters is due to the general tendency that as the phytoplankton concentration increases, reflectance decreases in the blue (400–515 nm) and increases in the green (515–600 nm) (Kirk, 1994).
Evaluating all the algorithms utilized to estimate Chl a concentration, we can deduct that the majority of passive remote sensing chlorophyll algorithms use either (a) blue to green band ratio, or (b) a NIR/red band ratio, or (c) spectral curvature or slope at different regions of the spectrum to estimate Chl a, this last one uses three bands.
In summary, Chl a algorithms develop for: (a) pythoplankton dominated-waters are based on blue to green band ratios, and (b) for optically complex waters are based on either a two-band ratio using NIR and red bands or the spectral curvature approach. Even though these approaches are mostly for oceanic waters, they represent the basis to inland fresh water bodies. Moreover, these approaches can be extrapolated onto inland fresh water bodies, per appropriate calibration and validation with in-situ data. Per previous studies in the lake (Weiss, 1971; Dix et al., 2012a; Chandra et al., 2013), we can deduct that the color of Lake Atitlán's waters is dominated mostly by pythoplankton during the dry season and during the rainy season, given all the runoff and sediment deposited in the lake, the waters become more optically complex, with a mix of constituents affecting the color of the water.
Area of Study
Lake Atitlán is a tropical mountain lake located in the Department of Sololá, Guatemala at 14.70°N, 91.19°W. Its origin is volcanic and is situated within a caldera that was formed 84,000 years ago (Chesner and Halsor, 2010). Located at 1,565 masl, Lake Atitlàn has a volume of 24km3, a maximum depth greater than 300 m with an average of 188 m, and a surface area of about 132 Km2. Lake Atitlàn is surrounded by three volcanoes and forms part of an endorheic basin, where the point of discharge is the lake and there is not an obvious outflow. However, Weiss (1971) proposed the possibility that the lake discharges through subterranean passages into River Madre Vieja, on the Pacific slope drainage, since chemical water characteristics were similar between the lake and river waters. river waters. Figure 1 shows the unique topography of the basin and its location.
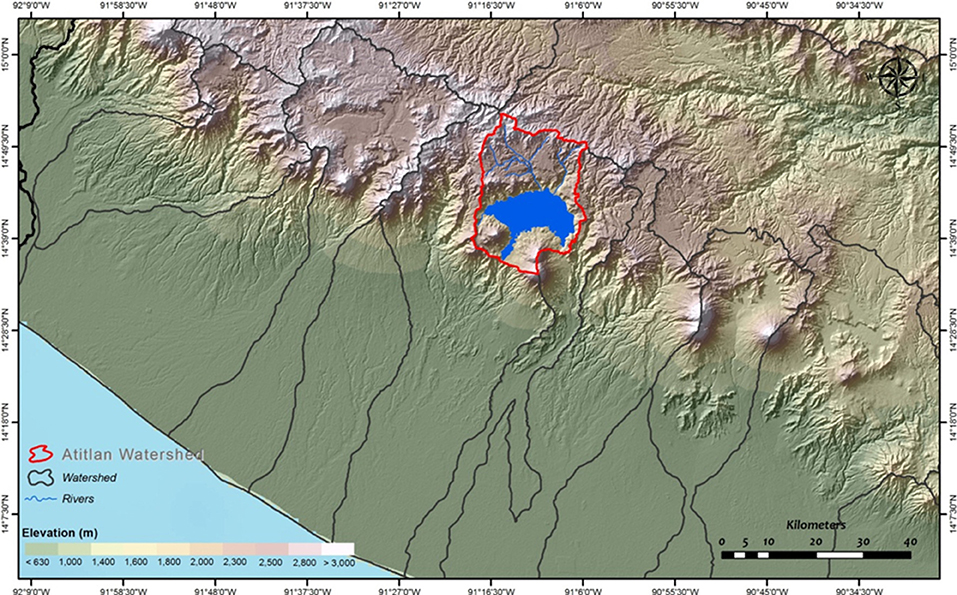
Figure 1. Map of Lake Atitlán Watershed. Topography of the Lake Atitlán watershed located on the highlands of Guatemala. Data source: ASTER GDEM, MAGA. ASTER GDEM is a product of METI and NASA (NASA et al., 2009).
Methods
Remote-sensing spectra was collected from Hyperion satellite images and correlated with in situ measurements of Chl a concentration. Below we explain how in situ measurements were acquired, how satellite data was processed and how the algorithm was developed and tested.
Field Measurements
The Centro de Estudios Atitlán from the Universidad del Valle de Guatemala (UVG) collected 40 in situ measurements of Chl a concentration in synchronization with Hyperion overpasses between January and April 2013. We use the term “in situ” in this paper for data that was collected in the field. These months represent the dry season in Lake Atitlán and consequently the months in which the lake's water is the clearest (Weiss, 1971; Dix et al., 2012b). Samples were collected in the field, placed in a cooler with ice and transported to the laboratory where they were filtered the same day. A standard volume of water (180 ml) was filtered through Whatman GFF filters with 7 micrometer mesh and 25 mm diameter. The filters were individually packaged in aluminum foil and frozen for 24 h. Chlorophyll measurements were carried out using 20 ml methanol to extract the pigment during 12–24 h refrigeration in the dark. Readings were carried out using a Turner fluorometer following Standard Method (Eaton, 2005) and based on Ritchie (2006).
The Chl a measurements used in this study were collected at the same time than the Secchi disk transparencies. In addition, AMSCLAE supported the collection of in situ data, measuring Secchi disk transparencies. A total of five field campaigns were carried out between UVG and AMSCLAE to collect in situ measurements of Chl a and Secchi disk transparency. Figure 2 shows the locations of these in situ measurements.
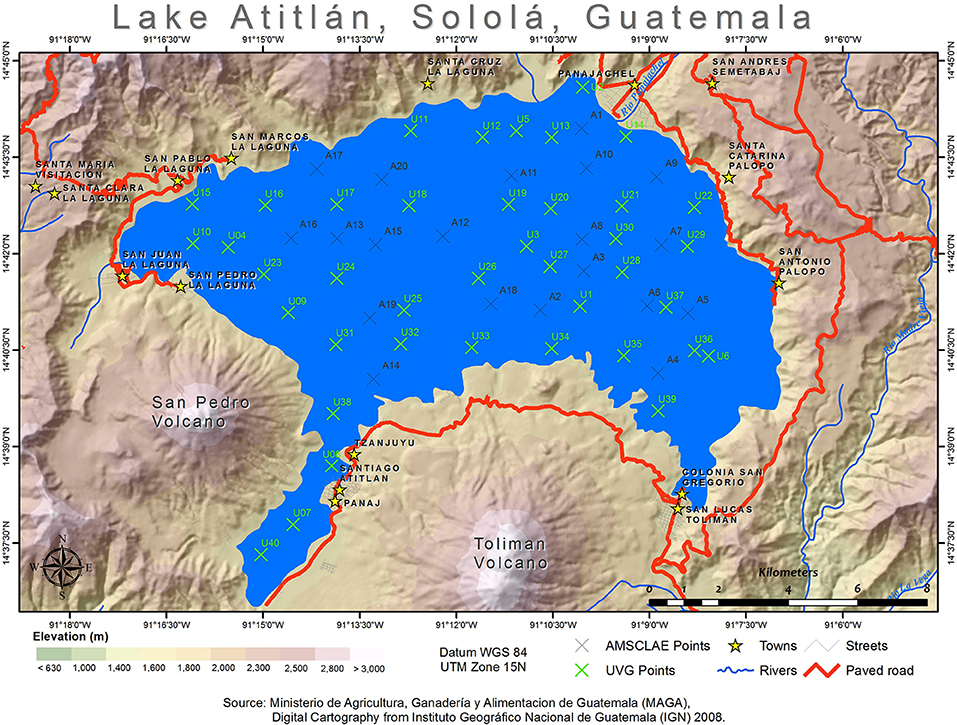
Figure 2. Location of in situ samples. Samples were collected by UVG and AMSCLAE. Topography of the Lake Atitlán watershed. Data source: ASTER GDEM, MAGA. ASTER GDEM is a product of METI and NASA (NASA et al., 2009).
The extremely low values of chlorophyll concentration measured during the dry season in Lake Atitlán at the subsurface level (below limit of detection) provided the initial foundation to determine the depth for the in situ Chl a measurements, which was based on less than the minimum transparency depth, in this study in situ samples of Chl a were measured at 3 m depth. Since there are no profile measurements of chlorophyll concentration or any other optically active components available that corresponded to coinciding overpass Hyperion imagery, it is not possible to determine the influence of the vertical structure of chlorophyll concentration on the surface reflectance measured by the satellite. This is a limitation of this study. However, calculation of Kd490 based on Mueller (2000) derived an average value of 0.3/m, and a median of 0.2/m. These Kd490 values indicate that the intensity of the visible light in the blue to green region of the spectrum will be reduced about 0.3 units per meter. Therefore, at 3m depth there is still available light in the blue and green region of the spectrum in the water column. As described by Stramska and Stramski (2005), even the operational empirical algorithms for chlorophyll retrieval (Ocean color algorithms) are affected to an unknown degree by the nonuniformity of Chl a profiles.
Satellite Data
Hyperion was a hyperspectral imager on board of the satellite Earth Observing-1 (EO-1), launched in 2000 as part of a 1-year technology validation/demonstration mission (U.S. Geological Survey, 2018). The EO-1 mission was undertaken originally to meet the needs of Landsat continuity program. After the baseline mission of EO-1 was accomplished, NASA approved the Extended Mission operations phase, with the objective of maximizing the use of EO-1 data, in December 2001, and this ran through early 2017, when the satellite was decommissioned. Hyperion has continuous spectral bands of about 10 nm width that cover from 0.4 to 2.5 μm of the electromagnetic spectrum, containing 220 spectral bands. The images have 30 m spatial resolution and cover a swath of 7.6 km. The EO-1 satellite flew in formation with the Landsat-7 satellite in a sunsynchronous, 705 km orbit with an equatorial crossing time 1 min later than that of Landsat-7 (Liao et al., 2000). Level 1 Gst data of Hyperion was used for this study. Level 1 Gst is radiometrically corrected and resampled for geometric correction and registration to a geographic map projection (USGS, 2006). There were two main reasons to use Hyperion in this study, first for research purposes, to assess suitability of a hyperspectral sensor to retrieve Chl a concentration and second, due to the ability to task image acquisitions. Hyperion satellites images were tasked at time of in situ data collection during cloud free conditions. A strong coordination effort to acquire in situ data and satellite data at the same time allowed us to generate a sound scientific dataset suitable for algorithm development.
Atmospheric Correction
The digital numbers obtained from the Hyperion satellite imagery were first converted to top of atmosphere (TOA) radiances and then to reflectance. Reflectance is a dimensionless value obtained from the ratio between the upwelling radiance emittance and the incoming radiant flux (irradiance).
The spectral radiance is obtained by radiometrically calibrating the digital number collected in Hyperion Level 1 data. Hyperion data are scaled to limit the amount of saturation and storage space (Beck, 2003). The digital values of the Level 1 product are 16-bit radiances and are stored as a 16-bit signed integer (Beck, 2003). The visible and NIR infrared bands are divided by 40 and the SWIR bands by 80 to de-scale the data and obtain the radiance in W/m2srμm. Then, Hyperion top of the atmosphere (TOA) reflectance was calculated using the following equation:
where: ρTOA= measured spectral radiance in W/m2srμm d = Earth-Sun distance in astronomical units. These values were obtained from the Earth-Sun distance table provided by U.S. Geological Survey (2018) ESUNλ = Mean solar exoatmospheric irradiance phis = Solar zenith angle in degrees, obtained from the Hyperion imagery metadata.
The ρTOA calculated using 1 was transformed to surface reflectance to be compared with in situ values, for this a radiative model was used. The second simulation of satellite signal in the solar spectrum-vector (6SV) was the radiative transfer model used to account for the atmospheric effects on the signal recorded by Hyperion satellite sensor. According to Vermote et al. (2006) the 6SV radiative transfer code “is the most widely used, rigorously validated, and heavily documented radiative transfer code known in the scientific remote-sensing community.” In addition, 6S has been successfully used for remote sensing of water quality (Potes et al., 2012; Shang and Shen, 2016; Markert et al., 2018), which makes it a suitable method for this analysis.
Python code was used to transform ρTOA to surface remote sensing reflectance using 6SV. P6S was run for satellite data accounting for each in situ sample.
The following criteria were used to compare satellite and in situ data following (Le et al., 2013): (1) both measurements (satellite and in situ sample) were acquired within a narrow window of ±3 h (Bailey and Werdell, 2006); and (2) a mean, maximum and median value from a 3 × 3 pixel box centered at the in situ sample site was used to filter sensor and algorithm noise (Hu et al., 2001). All the in situ samples were acquired in locations where the shallow bottom (depths <2 m) was not a problem.
Testing Chlorophyll a Algorithms
Existing operational algorithms were tested, including the default blue to green ratio OCx algorithms (O'Reilly et al., 1998; Werdell and Bailey, 2005), the red to NIR band ratios and the three-band approach for spectral shape, specifically the Fluorescence Line Height (FLH) and Maximum Chlorophyll Index (MCI), mathematical formulations are presented in Table 1. FLH as described by Gower et al. (1999, 2005) and Wynne et al. (2008) uses a central band at 685nm and it measures the fluorescence of Chl a, which produces a narrow peak at this part of the spectrum for Chl a concentrations up to 30 mg/m3. Above this concentration the absorption by water and Chl a pigments combine to shift the peak to longer wavelengths (706 nm at 300 mg/m3) (Gower et al., 2005), which is the central band used in MCI. These last two algorithms use the reflectance height relative to a baseline formed linearly between two neighboring bands which are distributed evenly, hence the priority on the central band. The continuous bands of Hyperion were evaluated in an iterative manner to determine optimal position that would be used in the band ratio approach. Linear, power and polynomial equations were tested to find the best statistical correlations between in situ Chl a and satellite reflectance. For the algorithm development 75% of the in situ measurements (30 samples) were used and for the algorithm evaluation the remaining 25% (10 samples) were used.
Regression Analysis
From the total (40) in situ Chl a samples one was diskarded since it did not match the correspondent Hyperion image. From the resulting 39 samples, 30 were selected randomly for the algorithm development. The least square method was used for the regression analysis which was tested using linear and polynomial fits. To further select the best algorithm the analysis of variance (ANOVA) was used.
Inference of Best Fit and Algorithm Evaluation
This explains the methodology used to select and validate final algorithm. To validate the resulting algorithm a cross-validation resampling procedure was employed. With a data set of n data stations, the data will be randomly resampled n times, leaving out one station each time, following Chernick (2012). The predictive power of the algorithm will be evaluated using different statistical parameters. The best results obtained from the linear and polynomial regression are evaluated further with other statistical parameters such as the analysis of variance (ANOVA). In the ANOVA analysis the F-test (or F-ratio) and p-value (or p(F), significance of F) were evaluated. The F-test is used to evaluate the hypothesis that all predictor variables under consideration have no explanatory power and that all regression coefficients are zero (Chatterjee et al., 2000).
Results and Discussion
Field Measurements
Chl a measurements collected in situ were relatively low, in a range of 1.01−10.91mg/m3 (see Table 2). Secchi disk transparency and Chl a display modes values of 6 m and 7 mg/m3, respectively. The datasets represent low optically complex waters, as expected for the dry season, when these datasets were acquired on the field. Forty samples were collected for Chl a concentration and 60 samples for Secchi disk transparency. These samples were acquired on January 16, 24, 29; February 22 and April 05, 2013.
Linear Regression
The coefficient of determination, R2 and the Standard error of estimate were used to assess the results of the linear regression analysis based on band ratios and spectral shape models (FLH and MCI) described in Table 1. First, simple surface reflectance (SR) band ratios were assessed and then log-transformed ratios were used. Log-transformation datasets are used in the development of ocean color algorithms (O'Reilly et al., 1998). Extremely low R2 were obtained using linear regressions for both approaches, the highest R2 was 0.302, representing not good fit. However, given the multiple bands assessed in this study, this step was used to pre-select band ratios that will be further tested in polynomial regressions. The hyperion bands assessed were in the blue and red-edge part of the spectrum.
Polynomial Regression
Polynomial regressions using a third order polynomial fit following the methods of the ocean color algorithms (O'Reilly et al., 1998; Werdell and Bailey, 2005) were tested. See OCx model in Table 1.
O'Reilly et al. (1998) and Werdell and Bailey (2005) reported the SR443/SR555 ratio maximal to be at Chl a < 0.3 mg/m3, SR490/SR555 ratio was maximal between 0.3 and 2.0 mg/m3 and the SR510/SR555 was maximal above 2.0 mg/m3 and below 30 mg/m3. Given the Chl a concentration values obtained in the study (1–11 mg/m3), in theory the analogous band ratios used to represent SR490/SR555 and SR510/SR555 (SR487, SR498/SR559 and SR508/SR559, respectively) should have performed better to simulate the Chl a. Nonetheless, our best fit was obtained with SR467/SR555, using all datasets. The regression was tested using different combinations of bands. Two datasets, one with all stations containing the whole range of Chl a concentration (a), and another with Chl a concentrations <9 mg/m3 (b) were assessed. Overall, group b had better fits, this is due to the nature of the data, since most of the observations were in this range. This also implies that the algorithm resolves better real Chl a conditions that are lower than 9 mg/m3. Band ratios SR487/SR559 and SR498/SR559 provided good R2, between 0.65 and 0.66, using mean reflectance values, but were outperformed by SR467/SR555 with an R2 of 0.7. The 3-band algorithm, FLH, did not improve significantly in the polynomial regression, R2 of 0.52. Given the overall good performance of the polynomial regression, the band ratios with the best R2 in this step were selected for further evaluation.
Inference of Best Fit
A summary of the results for the analysis of variance (ANOVA) for the polynomial regressions that have the higher R2 are shown in Table 3. All the algorithms have larger F-ratios than the one tabulated for the significant level of 0.01. Therefore, it can be stated that the results are significant at level 0.01. Consequently the hypothesis that the predictor variables have no explanatory power can be rejected. This means that the Chl a concentration can be modeled using a blue to green band ratio. A blue to green band ratio satisfactorily explains the behavior of Chl a in the waters of Lake Atitlán at a significant level of 0.01. Evaluating the results from the mean and median values it can be deducted that the third order polynomial of the ratios 467/559 and 467/548 have the best results. The F-ratio is larger than the tabulated one and the ρ-values are significantly smaller than 0.01, see Table 3. Figures 3, 4 show the polynomial regression graphs for band ratio 467/559, both using the median and mean values datasets respectively.
This is the algorithm selected in this study for chlorophyll a concentration estimation in Lake Atitlán.
Evaluation of the Algorithm
A cross-validation resampling technique was performed to generate a data set that will be used to assess the adequacy of the model. The leave-one-out method was used for this purpose (Chernick, 2012). In this method the complete data set (n = 39) conformed by the data points used for the algorithm development (30) together with the other points that were left out of the algorithm development (9) are randomly resampled n times leaving one point out (n−1). Then the accuracy of the model is tested on the data point left out. This method was used since the number of samples for validation was too small (9 points) which can result in a very large bias (Chernick, 2012). Multiple statistical parameters were used to evaluate the model performance. The Mean relative Error (MRE) is the ratio of the absolute error to the real observed measurement, which is assumed to be error free. The MRE provides an estimate of how relevant is the absolute error. MRE is presented as a percentage. The Root mean square error (RMSE) is a common measure to evaluate model performance (Willmott et al., 1985; Moriasi et al., 2007). RMSE has the advantage of indicating an error in the units of the constituent of interest, in this case mg/m3. However, its disadvantage lies in that large errors are weighted heavily, producing a large RMSE even if there are small errors in a good portion of the data. RMSE values of 0 indicate a perfect fit and the general interpretation is that the smaller the RMSE the better the model performance. Given the uncertainty of what is considered a low RMSE another model evaluation statistic is used to aid the interpretation of RMSE, the RMSE-observations standard deviation ratio (RSR). RSR standardizes RMSE using the observations standard deviation and is calculated as the ratio of the RMSE and standard deviation of observations (Moriasi et al., 2007). The closer the RSR is to zero (0) the better the model performance. The bias error (BIAS) indicates an average model “bias”; that is average over-or under prediction (Willmott and Matsuura, 2005). The percent bias (PBIAS) measures the average tendency of the simulated data to be larger or smaller than their observed counterparts (Gupta et al., 1999). The optimal value of PBIAS is 0.0. Positive values indicate model underestimation bias, and negative values indicate model overestimation (Gupta et al., 1999; Moriasi et al., 2007). PBIAS is the deviation of the data being evaluated, expressed as a percentage (Moriasi et al., 2007). The Nash-Sutcliffe efficiency is a normalized statistic that determines the relative magnitude of the residual variance (“noise”) compared to the measured data variance (“information”) (Nash and Sutcliffe, 1970; Moriasi et al., 2007).
Table 4 displays the results of the model evaluation statistics. In general the results obtained from the validation and cross-validation data sets agree. It is important to mention that in this analysis the algorithm development and evaluation is being done with satellite data. Meanwhile, the majority of the algorithms developed for water quality parameters had been generated using in situ measured reflectance (O'Reilly et al., 1998; Schalles, 2006). The evaluation of these algorithms performances is also commonly done using in situ measured reflectance (Le et al., 2013). Our analysis portrays a different approach for algorithm development and evaluation, for an understudied area where, in situ reflectance is not available, which can be more easily replicated using a variety of satellite remote sensing data in future research projects.
The algorithm assessed had a MRE error of about 33%. Le et al. (2013) reported its lowest MRE value of 25.66% for a 2-band algorithm using in situ reflectance Rrs(λ) in the Tampa Bay area. Le et al. (2013) also reported higher MRE values for 3-band and 4-band algorithms with 50.68% and 48.05%, respectively, using in situ reflectance as well. Le et al. (2013) evaluated algorithm performance using real satellite data from MODIS and MERIS. There was no meaningful statistical relationship between in situ measurements of Chl-a and coincident MODIS reflectance. This was attributed to atmospheric correction errors in MODIS data and abscence of appropiate spectral bands. The algorithm evaluation performed by Le et al. (2013) using real satellite data from MERIS provided better statistical results, with a MRE of 35.33% for the 2-band algorithm and 46.93%, and 69.15% for the 3-band and 4-band algorithm, respectively. The 2-band algorithm evaluated by Le et al. (2013) was a NIR-red band ratio generated for optically complex waters with Chl a concentrations that ranged between 2 and 80 mg/m3.
However, the MRE was degraded when using the MERIS satellite data (35.33%) compared to using the in situ reflectance (25.66%) due to spatial patchiness, differences in Chl a concentrations between in situ measurement and satellite over pass (Chen et al., 2010; Le et al., 2013) and even atmospheric correction errors (Le et al., 2013). All of these sources of error apply for the analysis performed in Lake Atitlán, and the MRE obtained for the Hyperion data (33%) is very similar to that observed by Le et al. (2013) with MERIS data (35.33%). The algorithm performance results obtained by Le et al. (2013) provide a source of reference for our analaysis, since they are reported for both in situ reflectance measurements and satellite-derived reflectance measurements. The RMSE obtained for the algorithm evaluated in this study had an approximate value of 2.0 mg/m3, which is very similar to the optimal obtained by Le et al. (2013) using MERIS-derived data, 2.01 mg/m3. To further support the interpretation of the RMSE, the RSR statistic was calculated. An optimal value of RSR would be zero, which indicates zero residual variation, and therefore perfect model simulation (Moriasi et al., 2007). The average RSR value obtained for the algorithm performance evaluation is of 0.77 (see Table 4) which is close to zero. The BIAS and PBIAS measurements indicate that overall the model evaluated is overestimating by 0.26 mg/m3 the simulated Chl a concentration values. Finally, the NSE statistic value of 0.46 (see Table 4) falls into the range to determine that the model has an acceptable performance.
Discussion
The data set utilized for algorithm development represented a small range of Chl-a concentration (1–10 mg/m3), which limits the application of the algorithm generated within this concentration range. The final algorithm selected to simulate Chl a concentration follows the form of the Ocean color algorithms (OCx), of a polynomial regression fit using blue and green bands. Band ratios of blue and green bands are used for low Chl a concentrations, such as the measured in Lake Atitlán in which the major constituent driving the color of water is chlorophyll. The latter was also true for the conditions under which the algorithm was developed in Lake Atitlán. Even though the overall performance of the algorithm selected provided an overestimation of the simulated Chl a, the algorithm also presents an under estimation of Chl a concentrations when in situ Chl a was higher than 9 mg/m3, Figure 5 portrays this. It is recommended to use a larger calibration and validation data set to fit and test the algorithm to a broader Chl a concentration range.
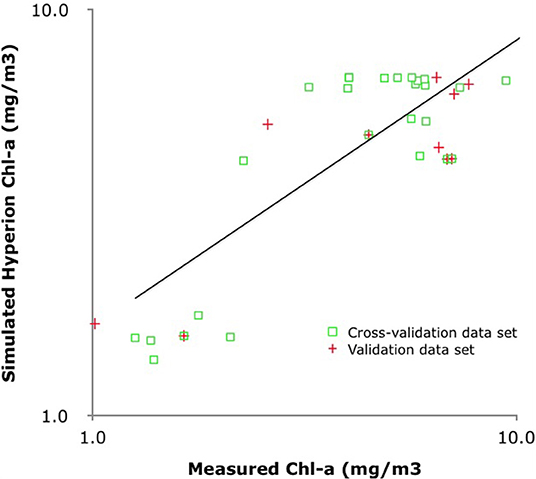
Figure 5. Scatter plot of in situ Chl-a from the data sets used for model evaluation and algorithm using satellite reflectance Rrs(λ).
The blue to green ratio selected in this study represents the theoretical behavior of the water dominated by Chl a, in which the Chl a absorption and scattering characteristics strongly influence the spectral signature of the water. For example, in these waters Chl a absorbs in the blue part of the spectrum (400–500 nm) and scatter in the green part of the spectrum (500–600 nm) with an evident reflectance peak at about 550 nm (Schalles, 2006). The band ratio selected in this study SR467/SR559 represents these spectral regions. As recorded by Schalles (2006) this absorption in the blue and scatter in the green is consistent from low (3.3 mg/m3) to high (more than 60 mg/m3) Chl a concentrations. In theory these blue to green ratios are applicable for water dominated by Chl a in concentrations that range from low to high Chl a above 60 mg/m3. However, the evaluation performance of the algorithm in this analysis exhibited larger errors especially in low and high Chl a concentrations, for our case the low was defined <2–4 mg/m3 and high > 9 mg/m3, see Table 2. This is due to the low representation of these values for the algorithm development and it can be deduced that the majority of data sets are between 6 and 8 mg/m3.
Conclusions and Future Perspectives
After evaluating the different wavelengths and band ratios used in previous studies to model Chl a in Lake Atitlán, the best result was obtained by a blue to green band ratio. The final algorithm obtained had relative error of 33% and assumes that the color of the water in Lake Atitlán is mainly driven by phytoplankton. Given that the data sets used to generate and evaluate this algorithm reflect a small range of Chl a concentration (1–10 mg/m3) it is expected that the relative error will increase when the algorithm is applied in Chl a concentrations > 10 mg/m3. In addition, the analyses performed confirmed the suitability of Hyperion satellite images to model Chl a concentrations in Lake Atitlán. The developed algorithm was applied to experimentally estimate Chl a in Lake Atitlán during a bloom event, using Landsat OLI data in August 2015. The water quality parameters of Lake Atitlán present a seasonal pattern that is related to the dry and rainy season of the area. At the end of the rainy season lower transparencies and higher Chl a concentrations are recorded. Meanwhile, at the end of the dry season higher transparencies and lower Chl a concentrations are recorded. Thermal stratification and mixing processes take place seasonally in Lake Atitlàn but given the limited measurements in the lake this seasonality is not well characterized yet (Dix et al., in preparation).
The results of this study represent an early effort, known to the authors, to use satellite images to quantitatively monitor water quality parameters in an inland water body in Central America. The only existing applications of satellite remote sensing for water quality monitoring has been limited to qualitative applications in this region. Therefore, the results of this sutdy demonstrate that Chl a can be estimated from hyperspectral imagers like the now-defunct Hyperion, with a relative error of 33%. The algorithm developed is relevant for the current Venμs satellite, DLR Earth Sensing Imaging Spectrometer (DESIS) and the upcoming Hyspiri mission, all hyperspectral, that are or will capture data useful to monitor Chl a concentration in Lake Atitlan using the developed algorithm. Given the rapid eutrophication of fresh water bodies world wide (Ho et al., 2019), it is imperative to develop cost-effective methods, such as the one presented in this work, that allow local water managers monitor water quality of their fresh water resources.
The error obtained is slightly below the desired error set by NASA's Ocean Biology and Biogeochemistry Program of 35% (McClain et al., 2006). This confirms the adequacy of the results. Other studies that retrieve Chl a concentration in fresh water bodies using multispectral sensors show low degree of certainty (Boucher et al., 2018). However, better performance was obtained when time window of multispectral satellite data acquisition was closer to acquisition of in situ data used in correlation. This highlights two issues, (1) the high applicability of hyperspectral sensors and use of narrow spectral bands to retrieve Chl a concentration and (2) the relevance of methodologies encompassing same time/date acquisition of satellite data and in situ observation to develop algorithms to retrieve water quality parameters from satellite images.
The main sources of error for this algorithm stem from the estimation of surface reflectance obtained from the satellite image and the optical variability presented in the in situ data set that was used to generate the algorithm. The latter is related to the in situ Chl a data set used for the algorithm development, which in this case represented a range of 1–10 mg/m3. Under higher Chl a concentrations (>10 mg/m3) it is expected that the current algorithm will underperform given the data sets used for its generation. Our validation shows that locations with maximum Chl a concentrations (> or = to 10 mg/m3) retrieved simulated Chl a concentration lower than real. Additional testing of applicable band combinations would be required. The significant conclusions of this study can be summarized as follows:
• Hyperion satellite images can be successfully used to model Chl a concentrations in Lake Atitlán.
• A semi-empirical algorithm that uses a blue to green band ratio is suitable to model Chl a concentrations in Lake Atitlán during the dry season. This algorithm has a relative error of 33%.
The Chl a concentrations described in this study are relatively low (1.0 – 10 mg/m3), but the demonstration of Hyperion's suitability to model such low concentrations (represented by low reflectance values) is promising. It is reasonable to expect that Hyperion can be applied to distinguish Chl a in lakes with more variable or higher Chl a concentrations. This could be achieved with further in situ sampling in more varied conditions and would require fine tuning. The methodology used in this work can also be replicated in other lakes of the region to generate ad-hoc algorithms that represent the unique water quality characteristics of those individual water bodies.
Future research should be oriented toward determining the performance of ALI and other multispectral satellite images, such as Landsat MSS, ETM+, and OLI, in estimating Chl a concentration re-calibrating this algorithm. This will expand the applicability of the results obtained in this study. The accuracy of the algorithm should be very straightforward to calculate using ALI because images are available for the same dates on which the in situ Chl a data were collected. The original bands selected from Hyperion will need to be replaced by the closest ALI bands. Landsat 8 and Copernicus, Sentinel 2 is the other logical next step in testing the performance of multispectral imagers, but this will only be possible through the careful coordination of field sampling efforts with satellite overpasses and clear-sky conditions.
Multi-algorithm approaches that combine indices and look-up table approaches are promising (Salem et al., 2017) developments that can expand applicability of locally-tuned algorithms and should be explored when using hyperspectral datasets.
Since the development of this algorithm to the release of the peer-reviewed article, EO-1 has been decommissioned. Hence, it is critical to test and calibrate algorithms using operational sensors, such as Landsat and Sentinel-2.
As a final conclusion we would like to stress the local impact that this research has had in the area of Atitlán and Guatemala. The results of study have provided with new tools to the Lake Authorities (AMSCLAE, CONAP) and academia (UVG, San Carlos) to monitor Chl a, such as in the case of the algal bloom in 2015. Satellite remote sensing applications like this will allow for the creation of a systematic record of Chl a concentration in the lake that will document the progress of the water quality conditions. At the same time it will be possible to determine the more critical factors that are affecting the water quality of the lake and evaluate the impacts that the implemented policies or conservation actions are having in the lake's water quality.
Data Availability Statement
The datasets analyzed in this article are not publicly available. Requests to access the datasets should be directed to Africa Flores, YWZyaWNhLmZsb3Jlc0B1YWguZWR1LnI=.
Author Contributions
AF-A developed research idea, performed all remote sensing analysis, and lead article writing. RG provided guidance for analysis and supported article writing. MD collected in-situ datasets used in this analysis and supported article writing. Led section for in situ Chl a sampling methods. CR-O, JS-A, BP, BH, EC, and FB provided critical feedback and updates related to current state of science, lake conditions and cyanobacteria found in lake. In addition, all co-authors from AMSCLAE were involved in in situ data collection, that was used in this analysis.
Conflict of Interest
The authors declare that the research was conducted in the absence of any commercial or financial relationships that could be construed as a potential conflict of interest.
Acknowledgments
First and foremost, we would like to thank SERVIR leadership, Dan Irwin, Ashutosh Limaye, and NASA Applied Sciences, Capacity Building Program Manager, Nancy Searby, for their constant support to this work. Thanks also to USAID Climate Change office for their support to SERVIR, Jenny Frankle-Reed, Pete Epanchin, Carry Stokes, and Kevin Coffey. This work was based on the SERVIR thesis research, done by Africa Flores (Flores, 2013) under NASA-UAH Cooperative Agreement NNM11AA01A.
The authors would like to thank Stu Frye from NASA Goddard Space Flight Center Greenbelt, MD, who tasked EO-1 to acquire images at the same time that in situ sampling was being carried out. We would also like to recognize the invaluable support provided by researchers in Guatemala, Estuardo Bocel, Juan Carlos Bocel, Isabel Arriola, Cesar Arriola, and the rest of the AMSCLAE team in 2013 who collaborated to collect in situ samples for this study. Thank you to Sundar Christopher who provided crucial guidance to perform this analysis. Thanks also to UAH faculty Tom Sever, Udaysankar Nair, Quingyan Han, John Christy, staff Michele Kennedy, Jan Williamson, Linda Berry, David Corredor, and David Cook. Additional support for subsequent research in this area provided by National Geographic and Microsoft AI grant.
References
Bailey, S. W., and Werdell, P. J. (2006). A multi-sensor approach for the on-orbit validation of ocean color satellite data products. Remote Sens. Environ. 102, 12–23. doi: 10.1016/j.rse.2006.01.015
Boucher, J., Weathers, K. C., Norouzi, H., and Steele, B. (2018). Assessing the effectiveness of Landsat 8 chlorophyll a retrieval algorithms for regional freshwater monitoring. Ecol. Appl. 28, 1044–1054. doi: 10.1002/eap.1708
Bukata, R. P. (2013). Retrospection and introspection on remote sensing of inland water quality: “Like Déjà Vu All Over Again”. J. Great Lakes Res. 39, 2–5. doi: 10.1016/j.jglr.2013.04.001
Chandra, S., Rejmánková, E., Dix, M., Girón, N., Mosquera, V., Komárek, J. J K, et al. (2013). Estado del Lago Atitlan, Informe 2013. Technical report.
Chatterjee, S., Hadi, A. S., and Price, B. (2000). Regression Analysis by Example, 3rd Edn. New York, NY: Wiley-Interscience.
Chen, Z., Hu, C., Muller-Karger, F. E., and Luther, M. (2010). Short-term variability of suspended sediment and phytoplankton in Tampa Bay, Florida: observations from coastal oceanographic tower and ocean color satellites. Estuar. Coast. Shelf Sci. 89, 62–72. doi: 10.1016/j.ecss.2010.05.014
Chernick, M. R. (2012). Resampling methods. Wiley Interdiscip. Rev. Data Min. Knowl. Discov. 2, 255–262. doi: 10.1002/widm.1054
Chesner, C. A., and Halsor, S. P. (2010). “A bathymetric survey of Lake Atitlan, Guatemala,” in American Geophysical Union, Fall Meeting 2010 (San Francisco, CA).
Dix, M., Dix, M., Cabrera, D., Symonds, E., Orozco, E., Toledo, A., et al. (2012a). Estado Fisico, Quimico y Biologico del Lago Atitlan. Octubre 2009 a Diciembre 2010. Technical report, Centro de Estudios Atitlan, Laboratorio de Analisis y Monitoreo, Universdiad del Valle de Guatemala.
Dix, M., Orozco, M., Cabrera, D., Bocel, E., Toledo, A., and Symonds, E. (2012b). El Lago de Atitlan, Guatemala: su estado ecologico octubre 2009-diciembre 2011. Revista de la Universidad del Valle de Guatemala 24, 35–50.
Eaton, A. D. (2005). Standard Methods for the Examination of Water and Wastewater, 21st Edn. Washington, DC: APHA-AWWA-WEF.
Flores, A. (2013). Hyperspectral remote sensing of water quality in Lake Atitlán, Guatemala. (M.S. thesis). The University of Alabama in Huntsville, Huntsville, AL, 156.
Gitelson, A. A., Gurlin, D., Moses, W. J., and Barrow, T. (2009). A bio-optical algorithm for the remote estimation of the chlorophyll- a concentration in case 2 waters. Environ. Res. Lett. 4:045003. doi: 10.1088/1748-9326/4/4/045003
Gower, J., King, S., Borstad, G., and Brown, L. (2005). Detection of intense plankton blooms using the 709 nm band of the MERIS imaging spectrometer. Int. J. Remote Sens. 26, 2005–2012. doi: 10.1080/01431160500075857
Gower, J. F. R., Doerffer, R., and Borstad, G. A. (1999). Interpretation of the 685 nm peak in water-leaving radiance spectra in terms of fluorescence, absorption and scattering, and its observation by MERIS. Int. J. Geomat. Geosci. 20, 1771–1786. doi: 10.1080/014311699212470
Gupta, H. V., Sorooshian, S., and Yapo, P. O. (1999). Status of automatic calibration for hydrologic models: comparisson with multilevel expert calibration. J. Hydrol. 4, 135–143. doi: 10.1061/(ASCE)1084-0699(1999)4:2(135)
Ho, J. C., Michalak, A. M., and Pahlevan, N. (2019). Widespread global increase in intense lake phytoplankton blooms since the 1980s. Nature 574, 667–670. doi: 10.1038/s41586-019-1648-7
Hu, C., Carder, K. L., and Muller-karger, F. (2001). How precise are SeaWIFS ocean color estimates? Implications of digitization-noise errors. Remote Sens. Environ. 76, 239–249. doi: 10.1016/S0034-4257(00)00206-6
Hu, C., Lee, Z., and Franz, B. (2012). Chlorophyll a algorithms for oligotrophic oceans: a novel approach based on three-band reflectance difference. Geophys. Res. 117, 1–25. doi: 10.1029/2011JC007395
INE (Instituto Nacional de Estadistica) (n.d.). Guatemala: Estimaciones de la Población total por municipio. Período 2008–2020. Retrieved from: http://www.oj.gob.gt/estadisticaj/reportes/poblacion-total-por-municipio(1).pdf
Jordan, T. E., Cornell, J., and Miklas Weller, D. E. (1991). Long-term trends in estuarine nutrients and chlorophyll, and short term effects of variation in watershed discharge. Mar. Ecol. Prog. Ser. 75, 121–132. doi: 10.3354/meps075121
Kirk, J. T. (1994). Light and Photosynthesis in Aquatic Ecosystems, 2nd Edn. Cambridge: Cambridge University Press.
Komárek, J., Zapomělová, E., Šmarda, J., Kopecký, J., Rejmánková, E., Woodhouse, J., et al. (2013). Polyphasic evaluation of Limnoraphis robusta, a water-bloom forming cyanobacterium from Lake Atitlán, Guatemala, with a description of Limnoraphis gen. nov. Fottea 13, 39–52. doi: 10.5507/fot.2013.004
Le, C., Hu, C., Cannizzaro, J., English, D., Muller-Karger, F., and Lee, Z. (2013). Evaluation of chlorophyll-a remote sensing algorithms for an optically complex estuary. Remote Sens. Environ. 129, 75–89. doi: 10.1016/j.rse.2012.11.001
Liao, L., Jarecke, P., Gleichauf, D., and Hedman, T. (2000). “Performance characterization of the Hyperion Imaging spectometer instrument,” in Proceedings of the SPIE 4135, Earth Observing Systems (San Diego, CA), Vol. 264, 12.
Markert, K. N., Schmidt, C. M., Griffin, R. E., Flores, A. I., Poortinga, A., Saah, D. S., et al. (2018). Historical and operational monitoring of surface sediments in the lower mekong basin using landsat and Google earth engine cloud computing. Remote Sens. 10:909. doi: 10.3390/rs10060909
McClain, C., Hooker, S., Feldman, G., and Bontempi, P. (2006). Satellite data for ocean biology, biogeochemestry, and climate research. EOS Trans. 87, 337–343. doi: 10.1029/2006EO340002
Mobley, C. D. (1995). “The optical properties of water,” in Handbook of Optics, Vol. 1, ed M. Bass (New York, NY: McGraw-Hill), 43.3–43.56.
Moriasi, D. N., Arnold, J. G., Liew, M. W. V., Bingner, R. L., Harmel, R. D., and Veith, T. L. (2007). Model Evaluation guidelines for systematic quantification of accuracy in watershed simulations. Am. Soc. Agricult. Biol. Eng. 50, 885–900. doi: 10.13031/2013.23153
Morrow, J. H., White, B. H., Chimiente, M., and Hubler, S. (2000). A bio-optical approach to reservoir monitoring in Los Angeles. Limnoology and Lake Management. Adv. Limnol. 55, 171–191.
Mueller, J. L. (2000). “SeaWiFS algorithm for the diffuse attenuation coefficient, K(490), using water-leaving radiances at 490 and 555 nm,” in Sea-WiFS Postlaunch Calibration and Validation Analyses, Part 3, SeaWiFS Postlaunch Technical Report Series, Vol. 11, eds S. B. Hooker and E. R. Firestone (Greenbelt, MD: NASA Goddard Space Flight Center), 24–27
NASA, METI, AIST, Japan Space Systems, and U.S./Japan ASTER Science Team (2009). ASTER Global Digital Elevation Model V003 [Data set]. NASA EOSDIS Land Processes DAAC. doi: 10.5067/ASTER/ASTGTM.003
Nash, J. E., and Sutcliffe, J. V. (1970). River flow forecasting through conceptual models: Part 1. A discussion of principles. J. Hydrol. 10, 282–290. doi: 10.1016/0022-1694(70)90255-6
Ogashawara, I., and Moreno-Madriñán, M. (2014). Improving inland water quality monitoring through remote sensing techniques. ISPRS Int. J. Geo-Informat. 3, 1234–1255. doi: 10.3390/ijgi3041234
O'Reilly, J. E., Maritorena, S., Mitchell, B. G., Siegel, D. A., Carder, K. L., Garver, S. A., et al. (1998). Ocean color chlorophyll algorithms for SeaWiFS. J. Geophys. Res. 103, 24937–24953. doi: 10.1029/98JC02160
Page, B. P., Kumar, A., and Mishra, D. R. (2018). Int J Appl Earth Obs Geoinformation A novel cross-satellite based assessment of the spatio-temporal development of a cyanobacterial harmful algal bloom. Int. J. Appl. Earth Obs Geoinformat. 66, 69–81. doi: 10.1016/j.jag.2017.11.003
Palmer, S. C., Kutser, T., and Hunter, P. D. (2015). Remote sensing of inland waters: challenges, progress and future directions. Remote Sens. Environ. 157, 1–8. doi: 10.1016/j.rse.2014.09.021
Perez Gudiel, D. B. (2007). Evaluacion del efecto de la aireacion artificial para mejorar la calidad del agua en el lago de Amatitlan (Ph.D. thesis). Universidad de San Carlos de Guatemala, Guatemala.
Pérez, L., Bugja, R., Lorenschat, J., Brenner, M., Curtis, J., Hoelzmann, P., et al. (2011). Aquatic ecosystems of the Yucatán Peninsula (Mexico), Belize, and Guatemala. Hydrobiologia 661, 407–433. doi: 10.1007/s10750-010-0552-9
Potes, M., Costa, M. J., and Salgado, R. (2012). Satellite remote sensing of water turbidity in Alqueva reservoir and implications on lake modelling. Hydrol. Earth Syst. Sci. 16, 1623–1633. doi: 10.5194/hess-16-1623-2012
Rejmánková, E., Komárek, J., Dix, M., Komárková, J., and Girón, N. (2011). Cyanobacterial blooms in Lake Atitlan, Guatemala. Limnologica 41, 296–302. doi: 10.1016/j.limno.2010.12.003
Ritchie, R. J. (2006). Consistent sets of spectrophotometric chlorophyll equations for acetone, methanol and ethanol solvents. Photosynth. Res. 89, 27–41. doi: 10.1007/s11120-006-9065-9
Romero Santizo, M. A. (2009). Valoración económica del lago de Atitlán, Sololá (M.S. thesis). Universidad de San Carlos de Guatemala, Guatemala.
Romero-Oliva, C. S., Contardo-Jara, V., Block, T., and Pflugmacher, S. (2014). Accumulation of microcystin congeners in different aquatic plants and crops - A case study from lake Amatitlán, Guatemala. Ecotoxicol. Environ. Safety 102, 121–128. doi: 10.1016/j.ecoenv.2014.01.031
Salem, S. I., Higa, H., Kim, H., Kazuhiro, K., Kobayashi, H., Oki, K., et al. (2017). Multi-algorithm indices and look-up table for chlorophyll-a retrieval in highly turbid water bodies using multispectral data. Rem. Sens. 9. doi: 10.3390/rs9060556
Schalles, J. (2006). “Optical remote sensing techniques to estimate phytoplankton chlorophyll a concentrations in coastal,” in Remote Sensing of Aquatic Coastal Ecosystem Processes. Remote Sensing and Digital Image Processing, Vol. 9, eds L. Richardson and E. Ledrew (Dordrecht: Springer), 27–79.
SERVIR (2009). Pollution in Lake Atitlán, Sololá, Guatemala - Nov 2009. Retrieved from: https://servirglobal.net/Global/Articles/Article/1082/reappearance-of-pollution-in-lake-atitln-solol-guatemala-may-2011?page=2
SERVIR (2011). Cambios en la Superficie de Area del Lago Atitlan, Guatemala 1986-2011. Retrieved from: https://servirglobal.net/Global/Articles/Article/1088/servir-analyzes-changes-in-lake-atitlans-surface-extent-1986-2011
Shang, P., and Shen, F. (2016). Atmospheric correction of satellite GF-1/WFV imagery and quantitative estimation of suspended particulate matter in the yangtze estuary. Sensors 16:E1997. doi: 10.3390/s16121997
SIGSA (Sistema de Información Gerencial de Salud) (2019). Sistema de Información Gerencial de Salud. Retrieved from: https://sigsa.mspas.gob.gt/datos-de-salud
Stramska, M., and Stramski, D. (2005). Effects of a nonuniform vertical profile of chlorophyll concentration on remote-sensing reflectance of the ocean. Appl. Opt. 44, 1735–1747.
U.S. Geological Survey (2018). Earth Observing 1 (EO-1). Retrieved from: https://archive.usgs.gov/archive/sites/eo1.usgs.gov/index.html
USGS (2006). Hyperion Level 1GST (L1GST) Producto Output Files Data Format. Technical report, Department of Interior, USGS.
Vermote, E., Tanre, D., Deuze, J. L., Herman, M, Morcrette, J. J, and Kotchenova, S. (2006). Second Simulation of a Satellite Signal in the Solar Spectrum - Vector (6SV). Technical report.
Vincent, R. K., Qin, X., McKay, R. L., Miner, J., Czajkowski, K., Savino, J., et al. (2004). Phycocyanin detection from LANDSAT TM data for mapping cyanobacterial blooms in Lake Erie. Remote Sens. Environ. 89, 381–392. doi: 10.1016/j.rse.2003.10.014
Watanabe, F., Alcântara, E., Rodrigues, T., Imai, N., Barbosa, C., and Rotta, L. (2015). Estimation of chlorophyll-a concentration and the trophic state of the barra bonita hydroelectric reservoir using OLI/Landsat-8 images. Int. J. Environ. Res. Public Health 12, 10391–10417. doi: 10.3390/ijerph120910391
Weiss, C. (1971). Water Quality Investigations, Lake Atitlan 1968-1970. Technical report, University of North Carolina at Chapel Hill.
Werdell, P. J., and Bailey, S. W. (2005). An improved in-situ bio-optical data set for ocean color algorithm development and satellite data product validation. Remote Sens. Environ. 98, 122–140. doi: 10.1016/j.rse.2005.07.001
Willmott, C. J., Ackleson, S. G., Davis, R. E., Feddema, J. J., Klink, K. M., Legates, D. R., et al. (1985). Statistics for the evaluation and comparison of models. J. Geophys. Res. 90:8995. doi: 10.1029/JC090iC05p08995
Willmott, C. J., and Matsuura, K. (2005). Advantages of the mean absolute error (MAE) over the root mean square error (RMSE) in assessing average model performance. Climate Res. 30, 79–82. doi: 10.3354/cr030079
Wynne, T. T., Stumpf, R. P., Tomlinson, M. C., Warner, R. A., Tester, P. A., Dyble, J., et al. (2008). Relating spectral shape to cyanobacterial blooms in the laurentian great lakes. Int. J. Remote Sens. 29, 3665–3672. doi: 10.1080/01431160802007640
Appendix
Keywords: hyperspectral remote sensing, water quality, chlorophyll a concentration, Lake Atitlán, Guatemala
Citation: Flores-Anderson AI, Griffin R, Dix M, Romero-Oliva CS, Ochaeta G, Skinner-Alvarado J, Ramirez Moran MV, Hernandez B, Cherrington E, Page B and Barreno F (2020) Hyperspectral Satellite Remote Sensing of Water Quality in Lake Atitlán, Guatemala. Front. Environ. Sci. 8:7. doi: 10.3389/fenvs.2020.00007
Received: 15 June 2019; Accepted: 19 January 2020;
Published: 05 February 2020.
Edited by:
Niall Patrick Hanan, New Mexico State University, United StatesReviewed by:
Marc Peipoch, Stroud Water Research Center, United StatesQiuyan Yu, New Mexico State University, United States
Copyright © 2020 Flores-Anderson, Griffin, Dix, Romero-Oliva, Ochaeta, Skinner-Alvarado, Ramirez Moran, Hernandez, Cherrington, Page and Barreno. This is an open-access article distributed under the terms of the Creative Commons Attribution License (CC BY). The use, distribution or reproduction in other forums is permitted, provided the original author(s) and the copyright owner(s) are credited and that the original publication in this journal is cited, in accordance with accepted academic practice. No use, distribution or reproduction is permitted which does not comply with these terms.
*Correspondence: Africa I. Flores-Anderson, YWZyaWNhLmZsb3Jlc0BuYXNhLmdvdg==