- 1HEPIA Soils and Substrates, University of Applied Sciences and Arts Western Switzerland, Geneva, Switzerland
- 2School of Agricultural, Forest and Food Sciences, Bern University of Applied Sciences, Zollikofen, Switzerland
Increasing soil organic carbon (SOC) content is crucial for soil quality and climate change mitigation. SOC monitoring is indispensable to the corresponding policies and should provide results at farm scale to allow for incentives. In Switzerland, farmers perform mandatory analyses of the SOC content of the 0–20 cm topsoil of every field, based on a composite sample, at least every 10 years. The corresponding results are stored in a database in canton of Geneva. These data may be relevant for topsoil SOC monitoring, in particular for carbon sequestration policies, provided that they show appropriate quality, which is analyzed in this study. The minimum detectable change (MDC) of past results calculated based on the observed SOC changes was 0.013% g g–1 at canton scale (2,700 fields). Based on extended sampling of three representative fields, different sampling strategies were simulated to determine the best future sampling guidelines for farmers. Collecting 20 aliquots with a gouge on the field diagonals was considered the best sampling compromise with field MDC of ∼0.1% g g–1 and a sampling duration of 20 min. Compared to this procedure, former farmers’ sampling was not biased in average but showed a variance of 0.22% g g–1 due to smaller number of aliquots and varying sampling depths. Based on the best sampling results and assumptions on farm-scale SOC variance or SOC differences, the MDCs at farm scale ranged from 0.21 to 0.12% g g–1 (5 fields) and 0.09 to 0.05% g g–1 (30 fields), respectively. These MDCs are small compared to published monitoring networks MDCs and allow determining SOC change rates at farm scale, thus offering perspectives for inexpensive and efficient monitoring in the frame of soil quality or climate mitigation incentives. For the latter, however, additional information with equivalent soil mass and deeper-layer carbon content would be necessary.
Introduction
The 2015 United Nations Climate Change Conference, COP 21, emphasized the urgency to take measures allowing to keep the temperature increase below 2°C (2015 United Nations Climate Change Conference, 2019). The corresponding measures addressed both the reduction in emissions and the sequestration of atmospheric CO2, the so-called negative emission technologies (NET). The recent IPCC report (Intergovernmental Panel on Climate Change, 2018) was extremely alarming with respect to these objectives. “The current failure to reverse the growth in global emissions means that meeting Paris Agreement targets depends increasingly on deployment of NETs” (European Academies Science Advisory Council, 2019). However, the 2018 assessment report from EASAC (European Academies Science Advisory Council, 2018) estimated that NETs “offer only limited realistic potential to remove carbon from the atmosphere and not at the scale envisaged in some climate scenarios.” In this report, carbon sequestration in soils is considered as the most credible NET because it can be performed at large scale without large investments and technology development (European Academies Science Advisory Council, 2019). The 4 per 1,000 initiative (Minasny et al., 2017) assumed that an annual growth rate of 0.4% in the soil organic carbon (SOC) stocks would halt the increase in the CO2 concentration in the atmosphere related to human activities. The potential of agroecology and conservation agriculture to increase SOC stocks is emphasized, although the potential of these cropping systems to meet 4 per 1,000 objectives is largely disputed (De Gryze et al., 2009; Dimassi et al., 2014; Powlson et al., 2014; Baveye et al., 2018).
The potential for atmospheric carbon sequestration in soils is supported by the estimation that conversion of natural to agricultural ecosystems caused depletion of the SOC pool up to 60% in soils of temperate regions and 75% or more in soils of the tropics (Lal, 2004).
Indeed, increasing the soil carbon content is not a matter of climate change mitigation at first. The millennium assessment (Hooper et al., 2005) allowed to understand that soil functions are the cornerstone of ecosystem services. Soil quality, defined as the ability of the soil to function (Karlen et al., 2008), is largely depending on the topsoil SOC content (Bünemann et al., 2018). SOC decrease strongly impacts soil quality (Lal, 2006), in particular its physical resistance and resilience (Kay, 1998). Owing to the multiple benefits of SOC to soil quality, in particular soil fertility in a broad understanding (King et al., 2020), increasing SOC content is an environmental and agronomical emergency regardless of climate questions. The requirements in SOC content with respect to soil physical quality were recently defined by Johannes et al. (2017), who showed that soil structure vulnerability is proportional to the SOC to clay ratio. This ratio should not be smaller than 10% for acceptable structure vulnerability.
There are, therefore, multiple reasons to foster carbon sequestration in soils, which requires to closely monitor SOC content. The minimum detectable change (MDC) of a monitoring network defines a limit of detectability below which changes cannot be statistically assessed. MDC depends on the variance of the considered property and the number of sampling points (Zar, 2007).
Soil organic carbon monitoring networks are usually dedicated to assessing SOC changes at regional scale and are based on a network of sampling sites (Arrouays et al., 2014). Each site usually represents a small area, e.g., 10 × 10 m for the Swiss National Monitoring (Gubler et al., 2015). Aliquots are collected within the sites to form spatial composites for SOC analysis, thus averaging the site-scale spatial variability.
Promoting SOC sequestration in cropland, however, is not only a matter of regional monitoring. Because each farm is the operational management unit, carbon sequestration should be rewarded at farm scale. Therefore, SOC changes must be detected at farm scale with appropriate MDC, which is usually not provided by current monitoring networks. Moreover, it is highly preferable to adopt result-oriented management schemes rewarding effective sequestration than to subsidize the adoption of practices assumed to allow for sequestration as action-oriented schemes do (Burton and Schwarz, 2013). In this review, it is shown that “encouraging farmers to conduct their own monitoring has the added bonus of increasing the social value of expertise in identification and boosting the opportunity for the development of new cultural, symbolic and social capital.” Therefore, “indicators should be sensitive enough to deliver locally appropriate results and be able to entice farmers to participate but, at the same time, be not overly difficult or expensive to administer.” When it is not possible to develop simple, reliable, and self-monitorable indicators, result-oriented schemes are simply not effective in meeting the provision goals. This is particularly true for SOC, whose deficiency is not well perceived by farmers (Hijbeek et al., 2017), while farmers commitment is considered a key for sustainable management (Dal Ferro et al., 2020).
Since 1998, Swiss farmers have to sample their soils to analyze the soil organic matter (SOM) content of the top 0–20 cm layer of every field at least every 10 years to receive the state subsidies for ecological services. The farmers are assumed to provide a composite sample based on 10–15 aliquots taken from the topsoil (0–20 cm depth) of each field, and the analysis must be performed in a certified laboratory. In the Canton of Geneva, these mandatory analyses were performed according to Walkley and Black (1934), and stored in the Geographical Information System of the canton SITG (SITG, 2019) since 1993. In December 2017, Geneva canton has adopted a climate plan aiming, among others, at sequestrating 15,000 tons of CO2 per year in the arable land (Plan climat cantonal-Volet 2, 2017).
This paper aims at analyzing to what extent the mandatory analyses made after farmer’s sampling in the frame of the current Swiss agri-environmental management scheme meet the MDC requirements for SOC content monitoring from regional to field and farm scale. The conversion of SOC content into SOC stocks, which should accompany the implementation of the soil carbon sequestration part of the climate plan, is not considered in this study.
Materials and Methods
The study was conducted in three steps. Three fields with different SOC content and spatial variability, representative of the cultivated fields in the region, were sampled to determine the MDC of field SOC content as a function of the location and number of the aliquots used to make the composite sample. A “best sampling practice” guideline for farmers was derived from these results. Current farmers’ sampling practices were inquired, and the analyses of their composite samples were compared to the “best practice” samples, which allowed to determine the MDC of past analyses stored in the data base. The suitability of farmer’s sampling to meet the SOC content estimation requirements in terms of MDC and SOC error were then discussed from canton to farm and field scale.
Canton of Geneva SOC Database
The Geographical Information System of the canton SITG (SITG, 2019) contains the analyses of all cropland fields since 1993. Arable land in canton of Geneva represents about 9,000 ha with 2,700 fields that were sampled by farmers at least every 10 years since 1993 for mandatory analyses. The most common soil type is Cambisol (Food and Agriculture Organization, 2014) developed on moraines. The composite samples collected by farmers were brought for analysis to the ISO17025 certified Soils and Substrates laboratory of the Swiss University of Applied Sciences, Geneva. The samples were sieved to 2 mm and dried to 40°C, prior to SOC analysis following Walkley and Black (1934). According to these data, the average SOC content of the soils is 1.55% g g–1 with 0.48 standard deviation.
Field Sampling
Three fields representatives of the cultivated soils of the canton (in soil type, surface area, SOC content, and cropping history), constituted of cambisols, with different SOC content and cropping history, were intensively sampled. The fields, denoted J, C, and W covered areas of 2.6, 3.3, and 6.4 ha, respectively, with average SOC content of 1.2, 1.6, and 1.4% g g–1, respectively. Field J was under conventional tillage. Field C was cultivated under conservation agriculture for 10 years and showed the largest SOC content, while on field W, the farmer recently started no-till management.
In each field, 150 samples were collected with a gouge auger (Eijkelkamp® 2.5 cm inside diameter) in the 0–20 cm topsoil layer after removal of the surface residues. After exclusion of wheel tracks and 10-m large strips along the field limits, the sampling points were distributed on a stratified random network (Webster and Oliver, 1992) made of two embedded grids of large and small grid size, respectively. In each large grid cell, 2 points were randomly selected on the nodes of the inner grid. The larger and thinner sampling grid sizes were 20 and 4 m for fields C and J, and 30 and 6 m for W field, respectively. Each sample was analyzed for SOC content according to Walkley and Black (1934) after 2 mm sieving and drying at 40°C.
Simulation of Farmers’ Sampling
We first used the 150 SOC content analyses of each of the three field to create high resolution (1 × 1 m grid) SOC maps with kriging (Journel and Huijbregts, 2004), using the R® package Georob version 0.3–10.
The predicted soil maps (Figure 1) were used to simulate different possible trajectories of sampling to collect composite samples made of 5–25 sampling points. The tested sampling trajectories were single-diagonal, double-parallel transect, and double-diagonal (X shape) trajectories (Figure 2). Sampling points were assigned to each trajectory type with 500 repetitions at random, namely by (i) introducing a random component on the coordinates, which was smaller at the ends of the trajectory than in the middle, to simulate farmer’s walk in the field, and (ii) by adding a random error to the estimated SOC value based on the estimation variance (Figure 2). For each virtual composite sample, we computed mean, and standard deviation of the SOC content extracted from the predicted maps.
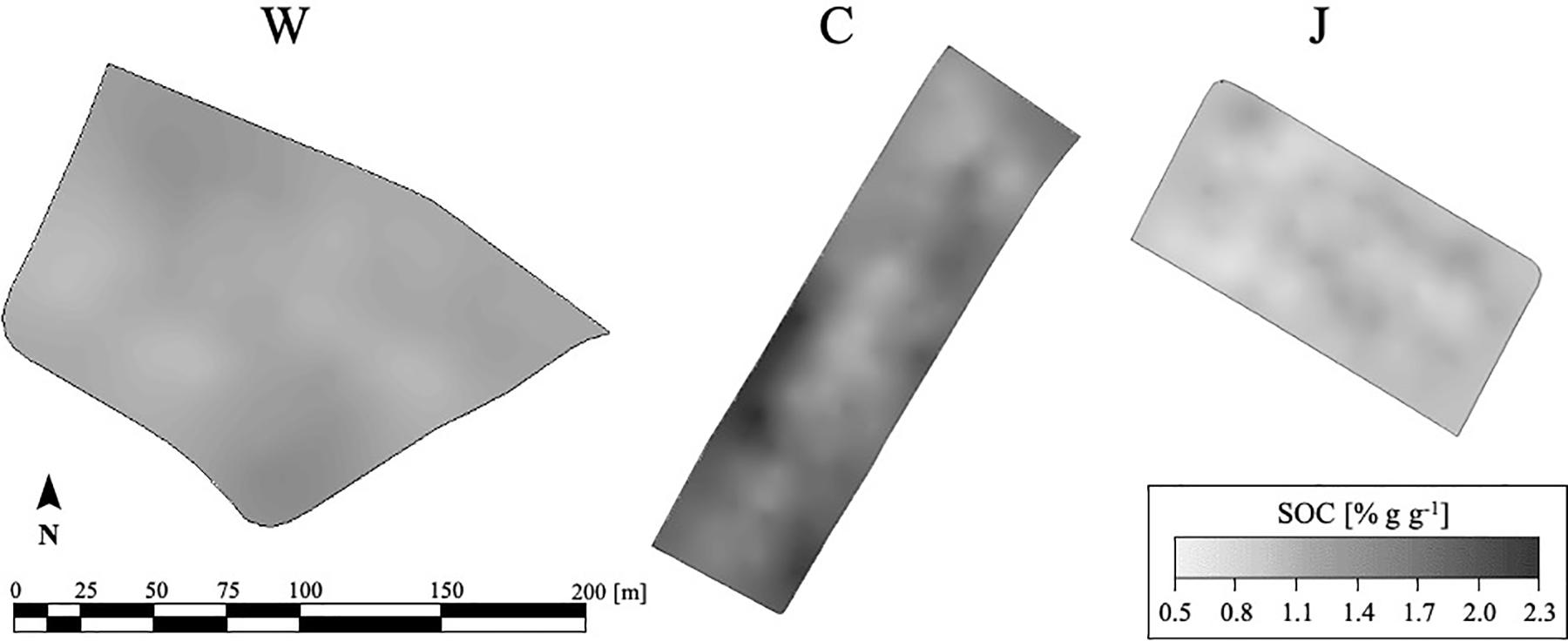
Figure 1. Predicted soil organic carbon (SOC) maps of the three fields. SOC was estimated on a grid of 1 × 1 m by kriging.
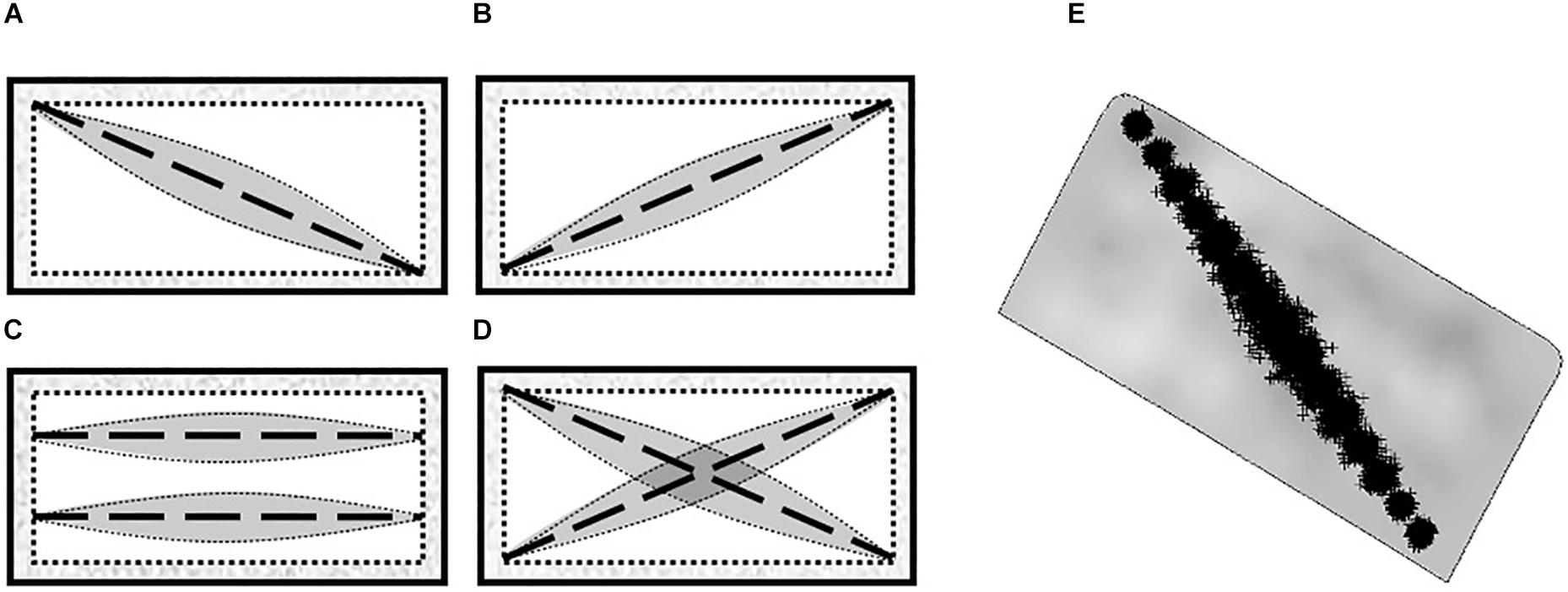
Figure 2. Different types of simulated trajectories: (A,B) single-diagonal, (C) double-parallel transect, and (D) double-diagonal (X-shape), and (E) example of 500 simulated single diagonals with 15 sampling points, J field.
Minimum Detectable Change
The MDC of a sampling network can be calculated with two methods (Saby et al., 2008). If the monitoring network of SOC provides n analyses of the same i sites at different times t0 and t1, the average difference between the SOC analyses is estimated as:
If Sd2 is the estimate of the variance of the differences, the MDC of SOC is:
where zα is the value of the standardized normal distribution at probability α (taken at 5% in the following, zα = 1.96). When differences are not available, the MDC can be estimated with the observed variance of SOC (de Gruijter et al., 2006) as:
where n is the number of points, and S2 is the variance of SOC in the considered area.
Farmers’ Sampling
The sampling error of past SOC analyses of field composite samples stored in the SITG database depends on how the fields were sampled by farmers. This is likely to vary compared to the “best procedure” tested in this study, since poor recommendations were formerly provided to farmers. The following investigation to estimate this “past” error compared to future guidelines was performed. After receiving soil for field mandatory analysis, we contacted the farmer to (i) immediately sample again his field (during the same week and before any operation was carried out) with the selected sampling procedure determined below and (ii) ask him how he proceeded (number of samples in the composite, depth, and sampling strategy), and the reasons for this operating mode. Comparing the SOC content of farmers and “best procedure” composite samples allowed to calculate the farmers’ sampling error to consider for the past SITG SOC data. All contacted farmers accepted the new sampling of their soil and to answer the inquiry. A total of 55 fields cultivated by 49 farmers were sampled a second time after farmer sampling, with 20 samples collected on the X-shape trajectory forming the composite.
Results and Discussion
SOC Properties in the Database and in the Sampled Fields
The mean and standard deviation of SOC as determined from the analyses of the 150 samples from each of the three fields are reported in Table 1.
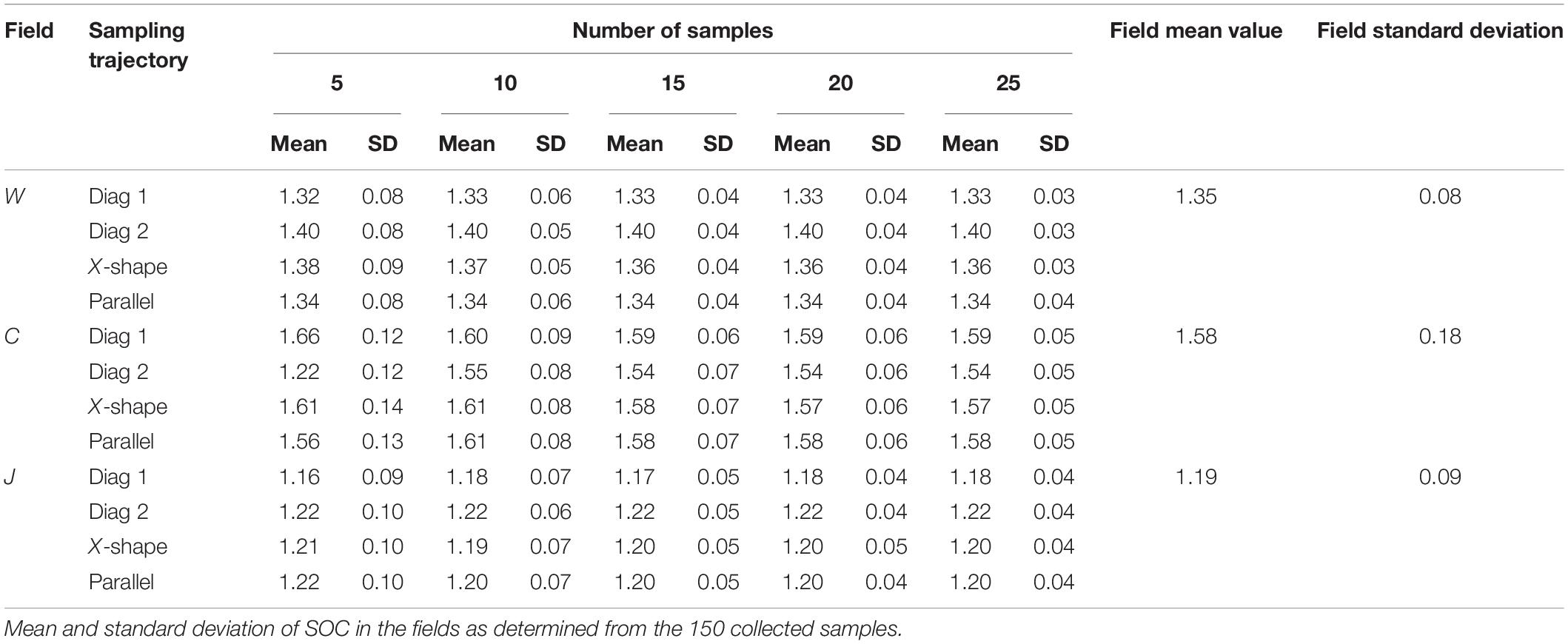
Table 1. Mean value of soil organic carbon (SOC) content (% SOC g g–1) in the simulated composite samples, and standard deviation (SD), for the different simulated sampling trajectories and number of samples to form the composite.
The C field, under conservation agriculture for 10 years, was not only the most heterogeneous but also showed largest SOC content. As expected, the field J managed with conventional tillage showed the smallest SOC content and variability. The experimental semivariograms were calculated for each field, and their fitted properties are reported in Table 2.
Best Field Sampling Procedure
The average and standard deviation of the SOC composites estimated from the different trajectories and different number of samples in the composite for each of the three fields are reported in Table 1. Mean values and standard deviations are close between trajectories, the closest estimation to the observed means being provided by parallel and X-shape sampling trajectories, compared to single diagonals. The decrease in standard deviation is marked until 20 samples, and the difference is smaller between 20 and 25 samples (Table 1). Therefore, we considered the X-shape trajectory with 20 subsamples forming the composite as the best practical compromise, taking into account that two parallel lines involve a risk to sample a former manure reclamation line. This sampling procedure takes ∼20 min for the experimented field size. The corresponding standard deviations were 0.04, 0.06, and 0.05% g g–1 of SOC for W, C, and J fields, respectively, (Table 1). The average error on the SOC value for 20 subsamples collected following an X-shape sampling to form the composite is smaller than 0.03% g g–1 compared to observed SOC mean value for the different fields.
However, in these simulations, the analytical variance of SOC analysis (determined as 0.0017 by the laboratory) is not taken into account because of the large number of trajectories simulated. In the case of a single composite sampling, only one analysis is performed. Therefore, the variances reported in Table 1 can be considered as the sampling variance to which the analytical variance should be added, as presented in (Ramsey, 1998).
Farmers’ Sampling
On average, the difference between SOC estimated by farmers and SOC estimated with the second sampling was -0.026% g g–1 and was not significant. The variance of the difference was 0.05 corresponding to a standard deviation of 0.22% g g–1. This variance is, therefore, an estimation of the field sampling variance for past analyses. Farmers made composite samples from seven aliquots on average. Forty-two out of 49 farmers sampled randomly following a single diagonal or double diagonal (X-shape), and 29 out of 49 sampled in the 0–20 cm layer. Although other sampling depths (e.g., 0–10, 10–25, etc.) were denoted, the sampled layer always belonged to the plow layer except one case. Since the earliest conversions to Conservation Agriculture occurred in 2008 only in the canton, most fields show a relatively homogeneous SOC content down to the plow layer, the depth of which was 30 cm in general, thus explaining the small observed error despite the various sampling depths used.
All farmers that took part in the survey stated that their sampling strategy followed recommendations they received from different sources, which we could verify. This is very encouraging, since we may expect a large majority of the farmers to apply an officially recommended best sampling practice in the future.
Minimum Detectable Change at Regional, Field and Farm Scales
At canton scale, the standard deviation of the SOC content of the arable land (0.48% g g–1) allowed to calculate the MDC using Eq. (3). However, the database also allowed to calculate the standard deviation of the difference between two consecutive sampling dates. We selected 390 fields whose second sampling time occurred in the last decade and whose field area and shape were unchanged at the two sampling dates. The average time lag between the sampling dates was 9.9 years because, in some cases, the mandatory analysis was performed earlier than required. The standard deviation of the difference was calculated as 0.35% g g–1 (variance, 0.12). We used Eq. (2) to calculate the MDC on the network formed by the 2,700 cropped fields (arable land) based on this standard deviation. The corresponding MDCs are of 0.013 [Eq. (2)] and 0.026% g g–1 [Eq. (3)], respectively. The two estimations are close, which supports the assumption made by de Gruijter et al. (2006) to calculate the MDC based on the observed variance [Eq. (3)]. This was also verified in the case of SOC content for England and Wales soils by Saby et al. (2008), although in both cases the standard estimation and MDC were overestimated by the latter method. According to these results, the SOC monitoring based on farmer’s sampling and mandatory analyses at canton scale provides smaller MDC than those reported for different European countries networks by Saby et al. (2008). Note that if the two consecutives dates were closer than 10 years, the variance of the difference could be smaller, hence resulting in a smaller MDC as calculated with Eq. (2), and vice versa. This could be further investigated, if rewarding carbon sequestration on shorter time lag than 10 years was considered.
At field scale, the MDC of X-shape trajectory for the different number of samples in the composite at a level of 95% confidence can be calculated as 1.96∗S, with S2 the variance of this sampling trajectory calculated as the sum of the sampling variance of the considered trajectory (Table 2) and the laboratory SOC analysis variance (0.0017; Ramsey, 1998). The MDCs were also calculated with Eq. (3) for each of the three fields and from the average variance of these fields. The results yielded by the two methods are reported in Table 3. Average values of MDCs are unsurprisingly similar with the two calculation modes. For X-shape trajectory and 20 subsamples in the composite, and based on the simulated composites, the average MDC was 0.10% g g–1, with values of 0.07, 0.15, and 0.08% g g–1 for W, C, and J fields, respectively. Assuming a bulk density of 1.35 g cm–3 (average value for the cropland topsoil of the canton) and an average SOC of 1.55 g g–1, the 0.1% g g–1 MDC corresponds to 2.7 t ha–1 of C in the 20-cm topsoil layer and 6.5% of the C stock in this layer.
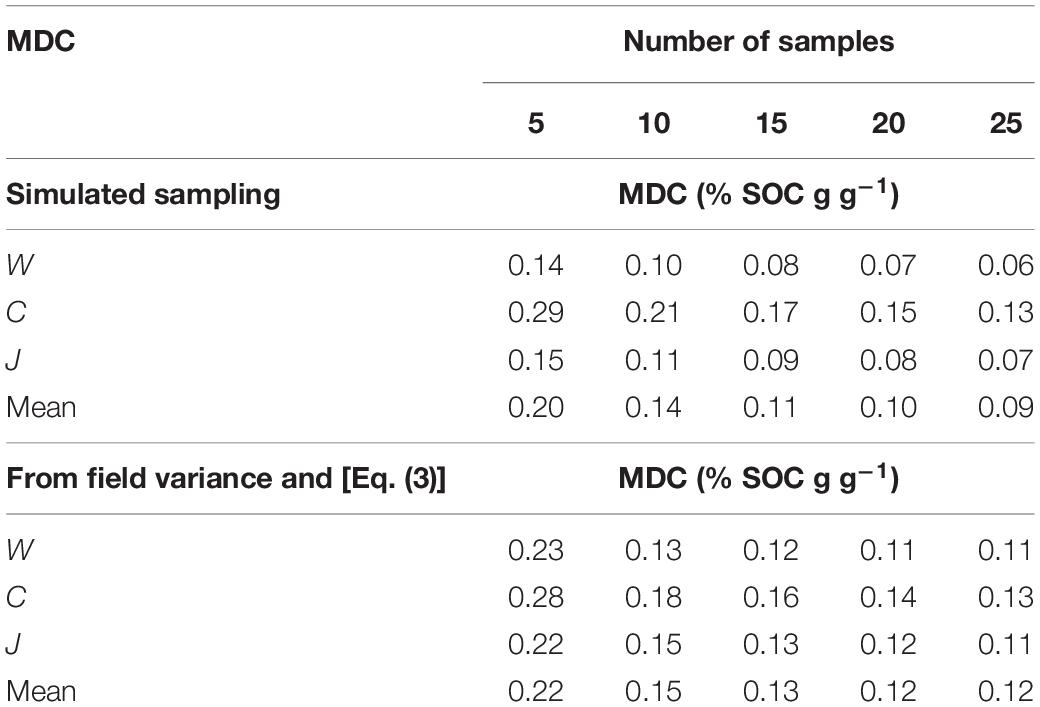
Table 3. Minimum detectable change of soil organic carbon (SOC) content (% g g–1) as determined (i) from simulated repeated sampling on the two diagonals using the variances in Table 1 and (ii) using the observed average variance for each experimental field, and average values.
At farm scale, the MDC of SOC depends on the number of fields in the farm. Most Swiss farms have 10–30 fields, and the average number in Geneva is 20 (HSSO, 2019). To cover a wide range of cases, we considered farms with 5–30 fields, using 20 samples per field to form the composite. MDCs were calculated in two ways: (i) using Eq. (3) and considering the variance of SOC at farm scale and n the total number of samples collected to calculate the average SOC content, namely 20∗(number of fields) or (ii) using Eq. (2), considering the variance of the difference between two consecutive field sampling at farm scale, with n taken as the number of fields. In the first case, an assumption must be made on the variance of SOC at farm scale. In the second one, an assumption on the farm-scale variance of the differences in SOC values between two sampling campaigns must be made. We assumed a SOC variance of 0.2 at farm scale (reminding that it is 0.3 at canton scale), and we assumed that the variance of the differences at farm scale was 0.06, namely, half of the variance of the differences between consecutive sampling at canton scale. Both assumptions are likely to overestimate the variance and the resulting MDCs, which should be further investigated, however. The corresponding calculated MDCs are reported in Figure 3. The calculated MDCs with the two approaches are very close and decrease with the number of fields, from 0.21 to 0.09% g g–1 [Eq. (2)] and 0.12 to 0.05% g g–1 [Eq. (3)] for 5–30 fields, respectively. In a small farm with 5 fields, an MDC of 0.12% g g–1 SOC means that a yearly SOC change of 0.024% g g–1 would be detectable after 5 years, and in a mid-size farm (15 fields), an MDC of 0.07% g g–1 SOC means that a yearly SOC change of 0.014% g g–1 would be detectable after 5 years, which correspond to annual sequestration rates of 16 and 9‰, respectively, at the observed mean SOC content of Geneva fields. According to the dataset used to calculate the standard deviation of the differences between two consecutive sampling, the annual rates of SOC change observed in Geneva range between −35 and + 40‰ (Figure 4). These SOC changing rates are in the range of values reported for conservation agriculture in the review of (Minasny et al., 2017).
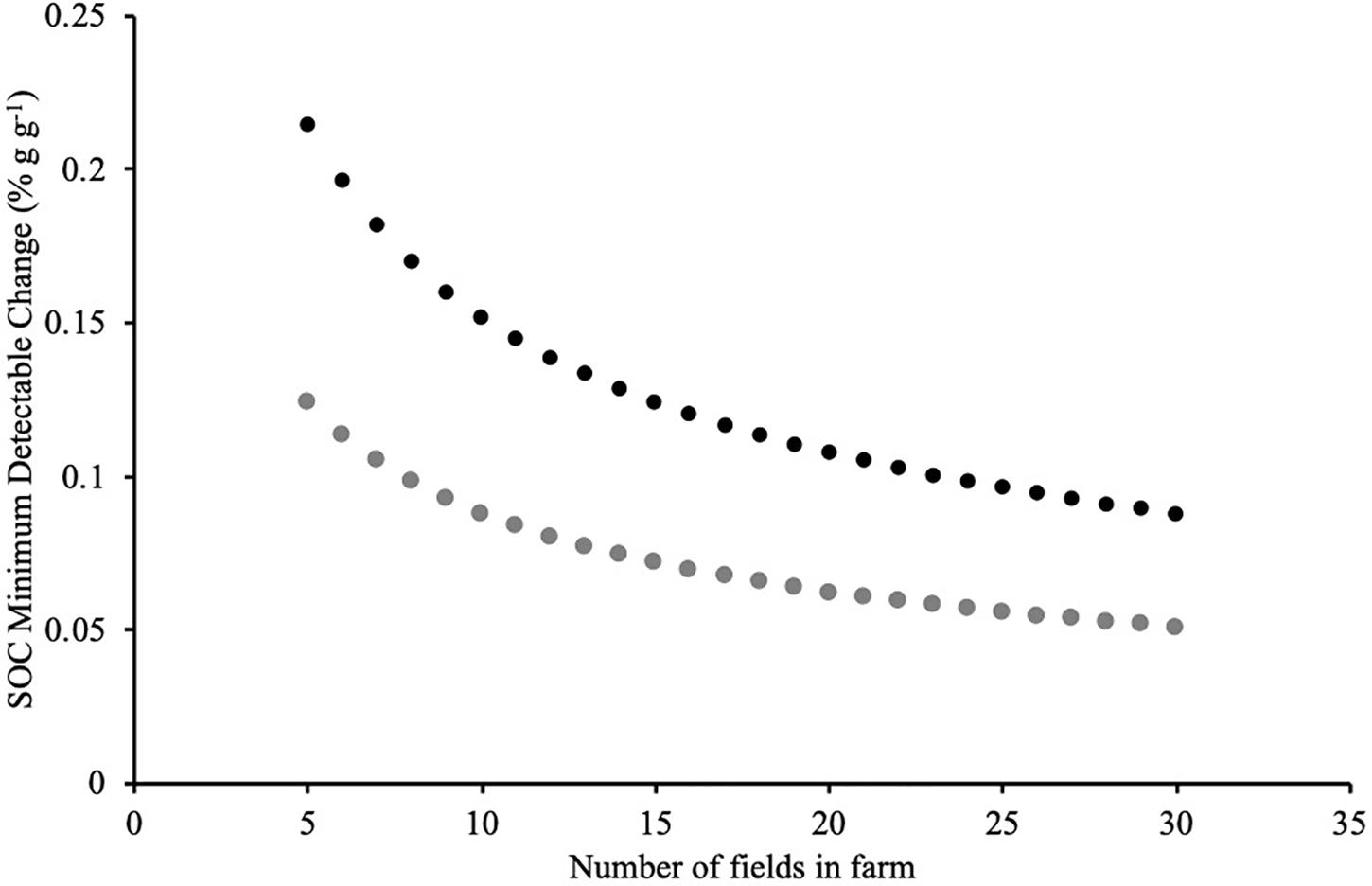
Figure 3. Minimum detectable change of soil organic carbon (SOC) at farm scale, as a function of the number of fields. Black dots: calculated using Eq. (2) and the estimated variance of the difference between two consecutive analyses at farm scale; gray dots: calculated using Eq. (3) and the estimated variance of SOC content at farm scale.
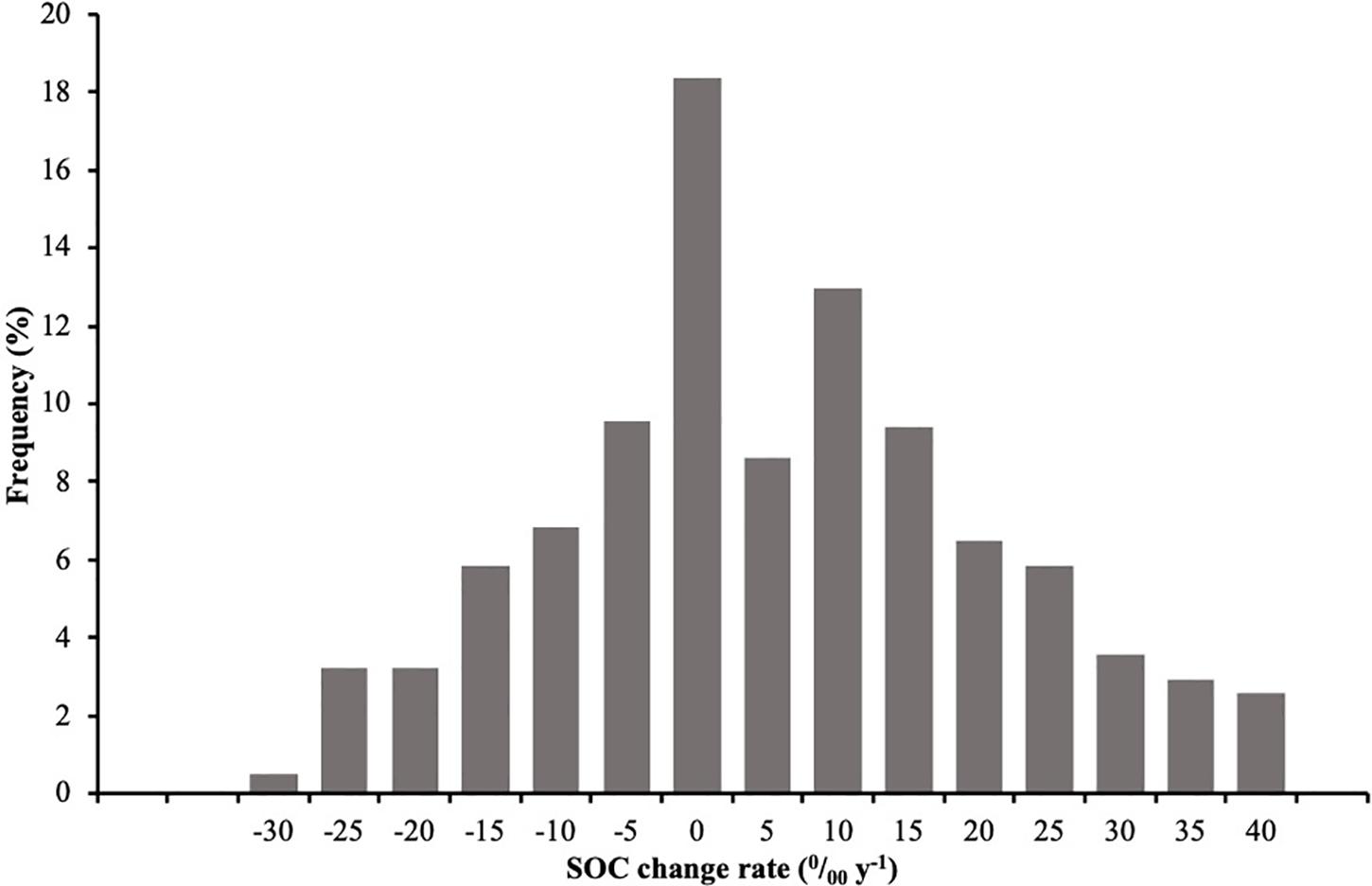
Figure 4. Histogram of the observed annual soil organic carbon (SOC) change rate for 390 fields of arable land from canton of Geneva. Time lag between two SOC analyses 10 years and sampling performed by farmers.
The SOC variances observed at field and canton scale are in the range of values commonly observed for arable land, after exclusion of organic soils and for fields of <10 ha surface area as reported in the meta-analysis of Saby et al. (2008). Therefore, our results may apply in a wide range of situations, keeping in mind that the variance at field scale is increasing with field area (Saby et al., 2008) and that the variances of SOC and SOC differences at farm scale were assumed a priori and are likely to be overestimated, which should be further determined.
For fields ranging from 2.5 to 6.5 ha fields, the X-shape trajectory with 20 sampling points was the most effective and easy to apply method, thus our favorite for best practices guidelines. Our results show that former sampling by farmers was neither standardized nor optimized in sampling depth, trajectory, and number of samples forming the composites. However, they followed some sampling guidelines they received, which is very encouraging for the future application of sampling guidelines.
The corresponding MDCs are small compared to published values according to the meta-analysis of Saby et al. (2008). From this point of view, farmers’ sampling for mandatory analyses represent a high-quality SOC monitoring at regional scale, even in a small canton such as Geneva with a relatively small number of fields. It would be even smaller for larger cantons or at country scale. Moreover, in Switzerland, this monitoring is available without additional expenses since it is based on mandatory analyses automatically stored in the canton GIS. Such a result was not expected by experts, who tend to trust on experts sampling or technology despite the many drawbacks in terms of cost and limited use.
Indeed, the major advantage of such a monitoring is not the small MDC at regional scale, however. First, the results are available at field and farm scale, which is not the case for published monitoring networks, usually performed on repeated sampling of small areas, thus neither representing the field containing the site nor the farm. Such a monitoring allows to know if SOC has increased or decreased at network scale but would not allow rewarding farmer’s results. Second, because the sampling is performed by farmers, the resulting SOC estimation is much more reliable to farmer’s eyes than any expert-operated measurement. Therefore, the corresponding SOC satisfies a fundamental indicator requirement in the perspective of result-oriented management scheme (Burton and Schwarz, 2013), which is a guarantee of farmers commitment and motivation, and is a requisite for the implementation of carbon sequestration incentives in arable land in the frame of soil quality management.
Monitoring carbon stocks for climate plans, however, requires additional information, such as soil bulk density, and should consider SOC stock on the 0–30 cm depth layer at least (Eggleston et al., 2008). The simplified Equivalent Soil Mass procedure proposed by Wendt and Hauser (2013) applies to composites samples collected with a gouge and could be adapted to the present case to meet the requirements for the Geneva climate plan, as currently investigated. If so, little additional efforts would be expected from farmers, which is important since complicated procedures may jeopardize their willingness to follow the corresponding guidelines. Collecting the 20–30 cm composite would allow both complying with international standards and apply the method proposed by Wendt and Hauser (2013). This should be considered since, under conservation agriculture, a decrease in SOC content below 10–15 cm depth compared to conventional tillage was reported after long-term experiments, which may balance the increase observed in the top layer (e.g., Balesdent et al., 2000; Hernanz et al., 2002; Dimassi et al., 2014).
Conclusion
This study allowed to propose sampling guidelines to farmers, namely, collect a composite made of 20 aliquots collected on the two field diagonals, for optimal SOC content estimation in the 0–20 cm depth layer of a field, which is the required sampling depth in the Swiss mandatory soil analysis system. It was performed on fields of <10 ha surface area and average SOC content of 1.5% g g–1. A composite sample made of 20 aliquots collected using a gouge along the two diagonals of the field was a good compromise in terms of minimum detectable change (0.17% g g–1), mean SOC estimation error (<0.03% g g–1), quickness (20 min), and ease of sample collection. Farmers past sampling practices were less accurate. Nevertheless, with an additional field sampling variance estimated to 0.22% g g–1, on average, these results were good in a perspective of SOC monitoring. At canton scale, the proposed field sampling scheme provides an MDC smaller than 0.03% g g–1 for SOC, which is small compared to published MDCs of monitoring networks, with almost no cost since these analyses are already available. The small MDC is explained by the large number of sampling sites in the monitoring, namely, the 2,700 cropland fields in Geneva. At farm scale, an MDC of 0.07% g g–1 is expected for 15 fields, which means that annual increases of < 0.02% g g–1 SOC would be detectable after 5 years.
The major strengths of this monitoring are with respect to farm scale and farmers’ commitment. Contrarily to classical SOC monitoring networks, determining the SOC content of each field with farmers’ sampling allows monitoring SOC changes at farm scale, thus opening the door to result-oriented management schemes, such as expected for rewarding carbon sequestration in the frame of climate plans.
Data Availability Statement
The raw data supporting the conclusions of this article will be made available by the authors, without undue reservation.
Author Contributions
CD performed the field and statistical work. MN supervised the geostatistical section. KG and OS collaborated to the interpretation of the results. PB supervised the work. All authors contributed to the article and approved the submitted version.
Conflict of Interest
The authors declare that the research was conducted in the absence of any commercial or financial relationships that could be construed as a potential conflict of interest.
Acknowledgments
We acknowledge M. Jotterand, Wegmuller, and Chollet, who allowed us to extensively sample their fields, and all the farmers who contributed to the inquiry.
References
2015 United Nations Climate Change Conference (2019). Wikipedia. Available online at: https://en.wikipedia.org/w/index.php?title=2015_United_Nations_Climate_Change_Conference&oldid=908345407 (accessed August 3, 2019).
Arrouays, D., Marchant, B. P., Saby, N. P. A., Meersmans, J., Jolivet, C., Orton, T. G., et al. (2014). “On soil carbon monitoring networks,” in Soil Carbon Progress in Soil Science, eds A. E. Hartemink and K. McSweeney (Berlin: Springer International Publishing), 59–68. doi: 10.1007/978-3-319-04084-4_6
Balesdent, J., Chenu, C., and Balabane, M. (2000). Relationship of soil organic matter dynamics to physical protection and tillage. Soil Tillage Res. 53, 215–230. doi: 10.1016/s0167-1987(99)00107-5
Baveye, P. C., Berthelin, J., Tessier, D., and Lemaire, G. (2018). The “4 per 1000” initiative: a credibility issue for the soil science community? Geoderma 309, 118–123. doi: 10.1016/j.geoderma.2017.05.005
Bünemann, E. K., Bongiorno, G., Bai, Z., Creamer, R. E., De Deyn, G., de Goede, R., et al. (2018). Soil quality - a critical review. Soil Biol. Biochem. 120, 105–125. doi: 10.1016/j.soilbio.2018.01.030
Burton, R. J. F., and Schwarz, G. (2013). Result-oriented agri-environmental schemes in Europe and their potential for promoting behavioural change. Land Use Policy 30, 628–641. doi: 10.1016/j.landusepol.2012.05.002
Dal Ferro, N., Camarotto, C., Piccoli, I., Berti, A., Mills, J., and Morari, F. (2020). Stakeholder perspectives to prevent soil organic matter decline in Northeastern Italy. Sustainability 12:378. doi: 10.3390/su12010378
de Gruijter, J. J., Bierkens, M. F. P., Brus, D. J., and Knotters, M. (2006). Sampling for Natural Resource Monitoring. Berlin: Springer.
De Gryze, S., Albarracin, M. V., Catala-Luque, R., Howitt, R. E., and Six, J. (2009). Modeling shows that alternative soil management can decrease greenhouse gases. Calif. Agric. 63, 84–90. doi: 10.3733/ca.v063n02p84
Dimassi, B., Mary, B., Wylleman, R., Labreuche, J., Couture, D., Piraux, F., et al. (2014). Long-term effect of contrasted tillage and crop management on soil carbon dynamics during 41 years. Agric. Ecosyst. Environ. 188, 134–146. doi: 10.1016/j.agee.2014.02.014
Eggleston, H. S., Miwa, K., Ngara, T., and Tanabe, K. (2008). Guidelines for National Greenhouse Gas Inventories - A Primer, Prepared by the National Greenhouse Gas Inventories Programme. IPCC. Japan: IGES. Available online at: https://www.ipcc-nggip.iges.or.jp/support/Primer_2006GLs.pdf (accessed April 17, 2020).
European Academies Science Advisory Council (2018). Negative Emission Technologies: What Role In Meeting Paris Agreement Targets?. Brussels: European Academies Science Advisory Council.
European Academies Science Advisory Council (2019). Forest Bioenergy, Carbon Capture And Storage, And Carbon Dioxide Removal: An Update. Brussels: European Academies Science Advisory Council.
Food and Agriculture Organization (2014). World Reference Base For Soil Resources 2014 International Soil Classification System For Naming Soils And Creating Legends For Soil Maps. Rome: FAO.
Gubler, A., Schwab, P., Wächter, D., Meuli, R. G., and Keller, A. (2015). Observatoirev National des Sols (NABO) 1985 á 2009. Berne: Office fédéral de l’environnement OFEV.
Hernanz, J. L., Lopez, R., Navarrete, L., and Sanchez-Giron, V. (2002). Long-term effects of tillage systems and rotations on soil structural stability and organic carbon stratification in semiarid central Spain. Soil Tillage Res. 66, 129–141. doi: 10.1016/s0167-1987(02)00021-1
Hijbeek, R., Cormont, A., Hazeu, G., Bechini, L., Zavattaro, L., Janssen, B., et al. (2017). Do farmers perceive a deficiency of soil organic matter? A European and farm level analysis. Ecol. Indic. 83, 390–403. doi: 10.1016/j.ecolind.2017.08.023
Hooper, D. U., Chapin, F. S., Ewel, J. J., Hector, A., Inchausti, P., Lavorel, S., et al. (2005). Effects of biodiversity on ecosystem functioning: a consensus of current knowledge. Ecol. Monogr. 75, 3–35. doi: 10.1890/04-0922
HSSO (2019). Données/I.39b Exploitations Parcellisées Par Canton - Statistique Historique de la Suisse. Available online at: https://hsso.ch/fr/2012/i/39b (accessed August 21, 2019).
Intergovernmental Panel on Climate Change (2018). Global Warming of 1.5°C. Geneva: Intergovernmental Panel on Climate Change.
Johannes, A., Matter, A., Schulin, R., Weisskopf, P., Baveye, P. C., and Boivin, P. (2017). Optimal organic carbon values for soil structure quality of arable soils. does clay content matter? Geoderma 302, 14–21. doi: 10.1016/j.geoderma.2017.04.021
Journel, A. G., and Huijbregts, C. J. (2004). Mining Geostatistics. Caldwell, NJ: The Blackburn Press.
Karlen, D., Andrews, S., Weinhold, B., and Zobeck, T. (2008). Soil quality assessment: past, present and future. Electron. J. Integr. Biosci. 6, 3–14.
Kay, B. D. (1998). “Soil structure and organic carbon: a review,” in Soil Processes And The Carbon Cycle Advances In Soil Science, ed. J. M. K. R. Lal (Boca Raton, FL: CRC Press), 169–197. doi: 10.1201/9780203739273-13
King, A. E., Ali, G. A., Gillespie, A. W., and Wagner-Riddle, C. (2020). Soil organic matter as catalyst of crop resource capture. Front. Environ. Sci. 8:50. doi: 10.3389/fenvs.2020.00050
Lal, R. (2004). Soil carbon sequestration impacts on global climate change and food security. Science 304, 1623–1627. doi: 10.1126/science.1097396
Lal, R. (2006). Enhancing crop yields in the developing countries through restoration of the soil organic carbon pool in agricultural lands. Land Degrad. Dev. 17, 197–209. doi: 10.1002/ldr.696
Minasny, B., Malone, B. P., McBratney, A. B., Angers, D. A., Arrouays, D., Chambers, A., et al. (2017). Soil carbon 4 per mille. Geoderma 292, 59–86. doi: 10.1016/j.geoderma.2017.01.002
Plan climat cantonal-Volet 2 (2017). GECH - Répub. Cant. Genève. Available online at: https://www.ge.ch/document/plan-climat-cantonal-volet-2 (accessed August 3, 2019).
Powlson, D. S., Stirling, C. M., Jat, M. L., Gerard, B. G., Palm, C. A., Sanchez, P. A., et al. (2014). Limited potential of no-till agriculture for climate change mitigation. Nat. Clim. Chang. 4, 678–683. doi: 10.1038/nclimate2292
Ramsey, M. H. (1998). Sampling as a source of measurement uncertainty: techniques for quantification and comparison with analytical sources. J. Anal. Spectrom. 13, 97–104. doi: 10.1039/a706815h
Saby, N. P. A., Bellamy, P. H., Morvan, X., Arrouays, D., Jones, R. J. A., Verheijen, F. G. A., et al. (2008). Will European soil-monitoring networks be able to detect changes in topsoil organic carbon content? Glob. Chang. Biol. 14, 2432–2442. doi: 10.1111/j.1365-2486.2008.01658.x
SITG (2019). SITG | Le Territoire Genevois à la Carte. Available online at: https://ge.ch/sitg/ (accessed August 3, 2019).
Walkley, A., and Black, I. A. (1934). An examination of the degtjareff method for determining soil organic matter, and a proposed modification of the chromic acid titration method. Soil Sci. 37, 29–38. doi: 10.1097/00010694-193401000-00003
Webster, R., and Oliver, M. A. (1992). Sample adequately to estimate variograms of soil properties. J. Soil Sci. 43, 177–192. doi: 10.1111/j.1365-2389.1992.tb00128.x
Wendt, J. W., and Hauser, S. (2013). An equivalent soil mass procedure for monitoring soil organic carbon in multiple soil layers. Eur. J. Soil Sci. 64, 58–65. doi: 10.1111/ejss.12002
Keywords: topsoil, soil organic carbon content, minimum detectable change, sampling method, composite sampling
Citation: Deluz C, Nussbaum M, Sauzet O, Gondret K and Boivin P (2020) Evaluation of the Potential for Soil Organic Carbon Content Monitoring With Farmers. Front. Environ. Sci. 8:113. doi: 10.3389/fenvs.2020.00113
Received: 17 April 2020; Accepted: 29 June 2020;
Published: 30 July 2020.
Edited by:
Maria Luz Cayuela, Spanish National Research Council, SpainReviewed by:
Daniel Plaza-Bonilla, Universitat de Lleida, SpainNicola Dal Ferro, University of Padua, Italy
Copyright © 2020 Deluz, Nussbaum, Sauzet, Gondret and Boivin. This is an open-access article distributed under the terms of the Creative Commons Attribution License (CC BY). The use, distribution or reproduction in other forums is permitted, provided the original author(s) and the copyright owner(s) are credited and that the original publication in this journal is cited, in accordance with accepted academic practice. No use, distribution or reproduction is permitted which does not comply with these terms.
*Correspondence: Pascal Boivin, pascal.boivin@hesge.ch