- 1Lethbridge Research and Development Centre, Agriculture and Agri-Food Canada, Lethbridge, AB, Canada
- 2Indian Head Research Farm, Agriculture and Agri-Food Canada, Indian Head, SK, Canada
- 3Saskatoon Research and Development Centre, Agriculture and Agri-Food Canada, Saskatoon, SK, Canada
- 4Department of Soil Science, University of Saskatchewan, Saskatoon, SK, Canada
The shelterbelt component of Canada’s whole-farm model Holos was upgraded from an age-determined to a circumference-determined (at breast height) calculation using a multi-stem averaging approach. The model interface was developed around the idea that a shelterbelt could have multiple rows, and a variable species composition within each row. With this, the model calculates the accumulated aboveground carbon in the standing biomass and a lookup table of modeled tree growth is used to add estimates of the belowground carbon. Going from an initial interface that asks for the current state, the model also incorporates an option of past and future shelterbelt plantings. In order to test the model’s suitability, we measured diverse shelterbelts (evergreen, deciduous, shrub type) in southern Saskatchewan, Canada representing commonly planted woody species. By making use of Caragana, Green Ash, Colorado Spruce, Siberian Elm, and a mixed Caragana/Green Ash tree row, we tested how many tree circumference measurements would be required to yield a representative average. Later, these results were incorporated in the Holos model to estimate the accumulated above- and below-ground carbon in each shelterbelt type.
Introduction
Global food consumption causes roughly one third of the global human induced greenhouse gas emissions (GHG), with agriculture directly contributing 23% (Intergovernmental Panel on Climate Change [IPCC], 2019), with the latter splitting approximately equally into CO2 (from deforestation and other land use change), CH4 (peatlands, rice cropping, and ruminant livestock), and N2O (from crop production). With growing food demand and a still increasing global population at about ∼1% per year in 2015–2020 (United Nations [UN], 2019), these contributions are expected to continually increase (European Environment Agency, 2015). In Canada, the national inventory report (following IPCC guidelines) estimates that agriculture contributes 8.4% to the national GHG budget, with N2O from cropping contributing 5.3% of the total national emissions and CH4 contributing 13% of the total, since the fugitive emission from oil and gas, as well as from landfills, also contribute to the latter share (Baah-Acheamfour et al., 2017; Environment and Climate Change Canada [ECCC], 2019).
Due to the commitments in the Paris climate accord (United Nations Framework Convention on Climate Change [UNFCCC], 2019), Canada is committed to reducing its emissions from 730 Mt CO2 eq. in 2005 to 511 Mt CO2 eq. by 2030 (a reduction of 304 Mt CO2 eq. from an unmitigated emissions scenario of 815 Mt CO2 eq.), with an estimated contribution from agriculture of −2 Mt CO2 eq. (Environment and Climate Change Canada [ECCC], 2020). Yet, the apparent temperature driven decomposition of soil carbon (Gregorich et al., 2017) calls into question the ability of Canadian soils to store more carbon in the future.
Regardless, planting and growing trees is touted as one of the most viable options to capture CO2 from the atmosphere (Bastin et al., 2019), as they pose a longer-term storage of atmospheric carbon with the potential for further processing and carbon sequestration. Canada’s agriculture landscape stretched over 158.7 million acres in 2016 (∼64.2 million ha) (StatsCan, 2019), and 1.7 million acres have planted shelterbelts (Toensmeier, 2016). Udawatta and Jose (2012) reported that shelterbelts could sequester up to 105 Mg C/ha in the aboveground shelterbelt biomass. When belowground biomass and soil carbon are added, shelterbelts have the potential to sequester a significant amount of atmospheric carbon per unit of land compared to other agricultural practices (despite some potential initial losses in soil carbon). In fact, the Canadian federal government once invested heavily into the planting of field, livestock, and farmyard shelterbelts which were intended to reduce wind speed and wind-derived soil erosion (Howe, 1986; Kulshreshtha et al., 2011) and enhance microclimate for crops (Kort, 1988; Kort et al., 2012) and animal production (Prairie Farm Rehabilitation Administration [PFRA], 1980; Poppy, 2003). Most of these shelterbelts have reached the end of their lifetime (Waldron and Hildahl, 1974; Rural Development Institute Shelterbelt Survey, 2014) and are removed for the sake of mechanization and production maximization (Waldron and Hildahl, 1974; Schroeder et al., 2011; Rempel, 2013; Rural Development Institute Shelterbelt Survey, 2014; Ha et al., 2019).
While many scientists have pointed out the various advantages of shelterbelts (Agriculture and Agri-Food Canada [AAFC], 2018), the question remains how Canadian landowners can be encouraged to maintain existing shelterbelts and increase their numbers beyond the current state (Rempel et al., 2017). A common pathway is regulatory or incentivizing policies, but information provision and education are important possibilities as well (Agriculture and Agri-Food Canada [AAFC], Agri-Environment Service Branch [AESB], and Agroforestry Development Centre [ADC] (2010). Stange and Jackson, 2015; Ward and de Gooijer, 2017; Stevenson, 2018). Simulation models are frequently used to test the accuracy and applicability of our gained scientific understanding of natural processes, and yet, such models, when packaged in appropriate software solutions, can be used to educate learners and practitioners in- and outside of academia. This is especially pertinent as the impact of our land use is increasingly felt in the environment (see for instance DiBartolomeis et al., 2019) and the importance of learning the complexities of land use/environment interactions for better decision-making wanes in comparison to perceived economic forcing. However, to gain an understanding of interactions, trade-offs, and the ripple effect of the various greenhouse gas sources and sinks (where the management of one can alter the other), interdisciplinary collaboration is required, to cover each and every aspect of farming systems and their potential interactions with the environment. Subsequently, a systems analysis approach has to be applied to ensure that identified impacts and benefits are not offset through some other, not considered, process or farming system component. For Canada and its farming systems, an initial step has been accomplished with the (whole-farm) Holos model (Little et al., 2008).
The Holos model, Agriculture and Agri-Food Canada’s whole-farm model, is based on the conceptualization of a virtual farm approach proposed by Janzen et al. (2006), and was published in its first iteration as Holos Classic (Little et al., 2008). That first iteration aimed to educate Canadian farmers about the magnitude of GHG emission sources on their farm, and about potential mitigation options they could employ, one of which was the planting of shelterbelts as a way to use sequestered carbon (in tree and shrub biomass and select surface and soil C pools) as an offset against the emissions of other GHG. User feedback triggered the development of a subsequent research version of the model (Holos version 2 and 3), which added management flexibility to the interface, making the model’s results more locally specific.
The Holos model has been utilized in several exemplary whole-farm analyses, ranging from understanding the general GHG impact of representative beef and dairy farm systems (Beauchemin et al., 2010; McGeough et al., 2012), while others tested real farm data (Church et al., 2015; Alemu et al., 2017a), or evaluated the effect of management practices on the whole-farm emissions (Alemu et al., 2017b; Guyader et al., 2017; Little et al., 2017). The model was utilized to investigate it’s capability for calculating tree biomass in farming systems (Amadi et al., 2016; Mayrinck et al., 2019), and was also adopted to assess farming systems in Norway (Bonesmo et al., 2012, 2013; Skjelvåg et al., 2012; Gülzari et al., 2017, 2018; Samsonstuen et al., 2019) and Bulgaria (Petkova, 2012).
A renewed effort in model development followed (Kröbel et al., 2012), and the addition of a new carbon modeling approach (Kröbel et al., 2016) required a ground-up rebuild of the model and thus offered the opportunity to update algorithms and processes in the model, and to redesign the interface in a (non-scientific) user-friendly fashion. As part of this renewal, we started updating the old shelterbelt calculations methods which used allometric calculations based on age (Kort and Turnock, 1999) with the findings of Amichev et al. (2017), who redesigned the allometric calculations based on tree diameter/circumference. These are being incorporated in the new Holos model version 4, together with a stakeholder driven interface design. This paper reports on the practical testing of the interface, parameter requirements, and the underlying equations, which is being conducted using measurements from actual shelterbelts in the vicinity of Indian Head, SK, Canada.
The goal of this manuscript was to:
(i) update the carbon accumulation calculations for tree biomass in the Holos whole-farm model using circumference instead of age driven algorithms, as suggested by Amichev et al. (2017);
(ii) develop a user friendly software interface that is simple to use and yet offers sufficient flexibility to reflect the diversity and complexity of existing shelterbelts;
(iii) test the model’s applicability by estimating the carbon storage of existing (measured) shelterbelts and determine the minimum requirements for model inputs;
(iv) compare the model’s outputs with literature derived measurements or modeled outputs from process models.
Materials and Methods
Site Description
We measured five field shelterbelts consisting of four single and one mixed species near Indian Head, SK, Canada (Figure 1). These sites are part of a larger project where the role of shelterbelts and other field boundary habitats (such as, natural field boundaries and road allowances, wetlands, etc.) on crop yield and quality as well as biodiversity and soil health are examined in large-scale monoculture agricultural landscape in Saskatchewan.
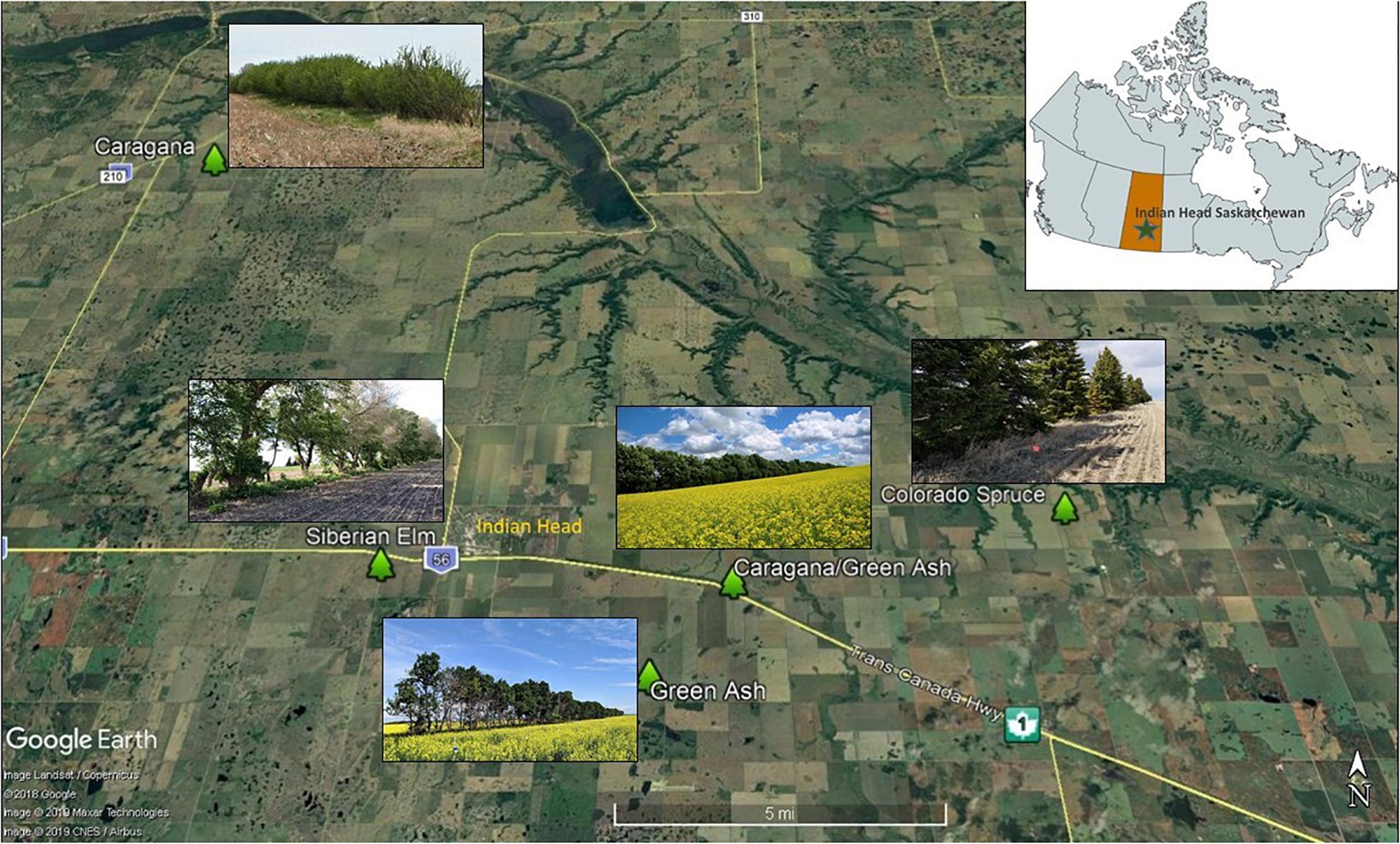
Figure 1. Physical location map of measured shelterbelts near Indian Head, SK, Canada. Inset-map of Canada with a green asterisk showing geographical location of Indian Head, SK, Canada.
For the Holos model interface, we only used planted field shelterbelts to calculate the accumulated carbon in these shelterbelts. The shelterbelts consist of Caragana (Caragana arborescens), Green Ash (Fraxinus pennsylvanica), Colorado Spruce (Picea pungens), Siberian Elm (Ulmus pumila), and mixed Caragana/Green Ash tree plantings. The details including shelterbelt age and characteristics, soil classification, soil texture, and adjacent crop rotations are provided in Table 1.
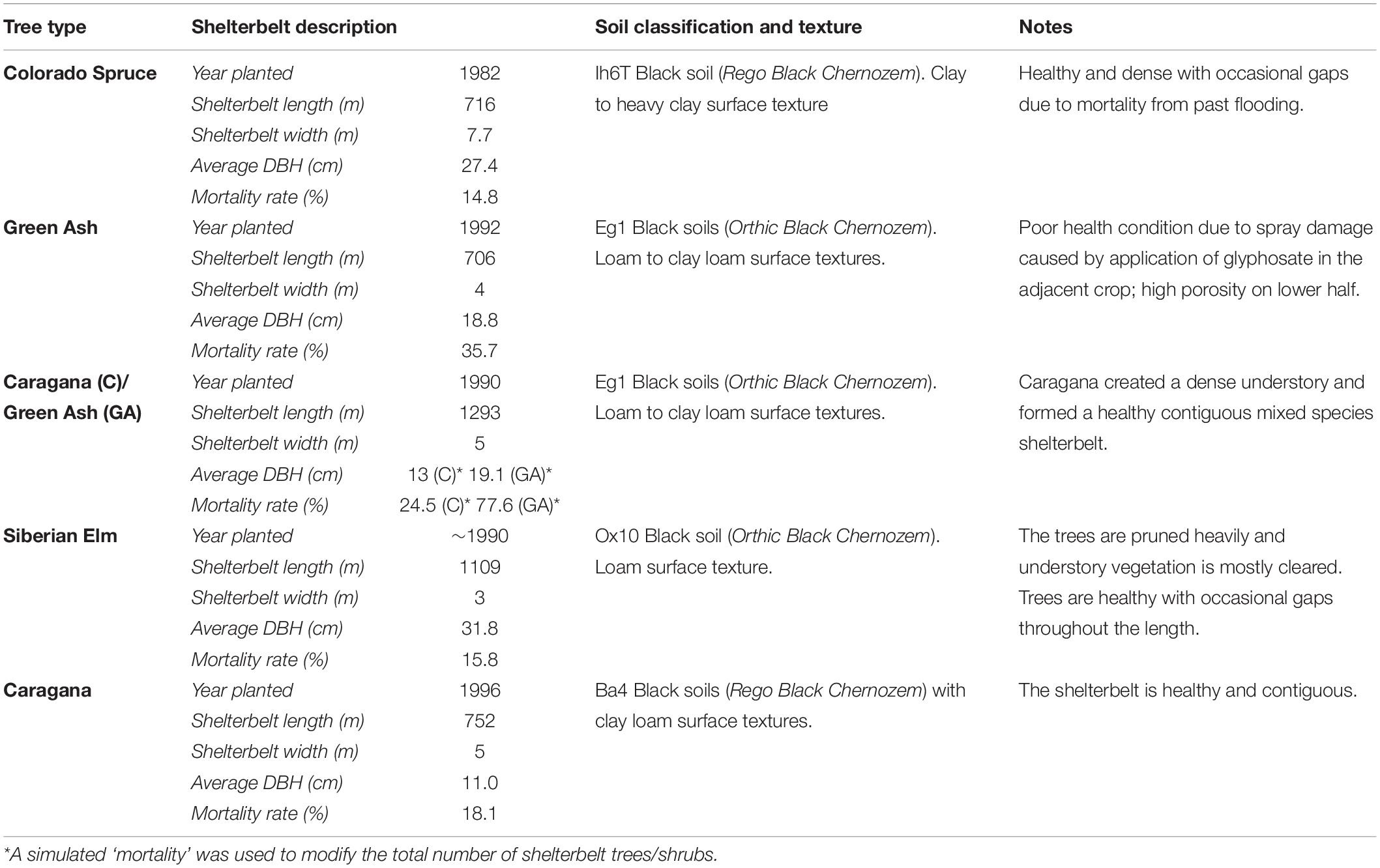
Table 1. Shelterbelt age, characteristics, soil classification and texture of studied shelterbelts near Indian Head, SK, Canada.
Measurement Data
We measured tree stem circumference at 1.3 m height aboveground for single stem trees (Colorado Spruce). When multiple stems are present per single tree (Green Ash and Siberian Elm), a cumulative circumference was estimated using:
where,
Cumulative circumference is the calculated circumference of all stems together (cm).
DBHi is the diameter (cm) of a stem at breast height (1.3 m).
Pi is the mathematical constant 3.14159.
For Caragana, we measured circumference of all stems of the shrub at 30 cm height aboveground and then calculated the cumulative circumference using the same formula as described above. For both trees and Caragana shrubs, we measured the circumference of every tree and/or shrub for 100 m length starting at one end of the shelterbelt. We recorded missing and dead trees for the shelterbelt mortality calculation (Table 2).
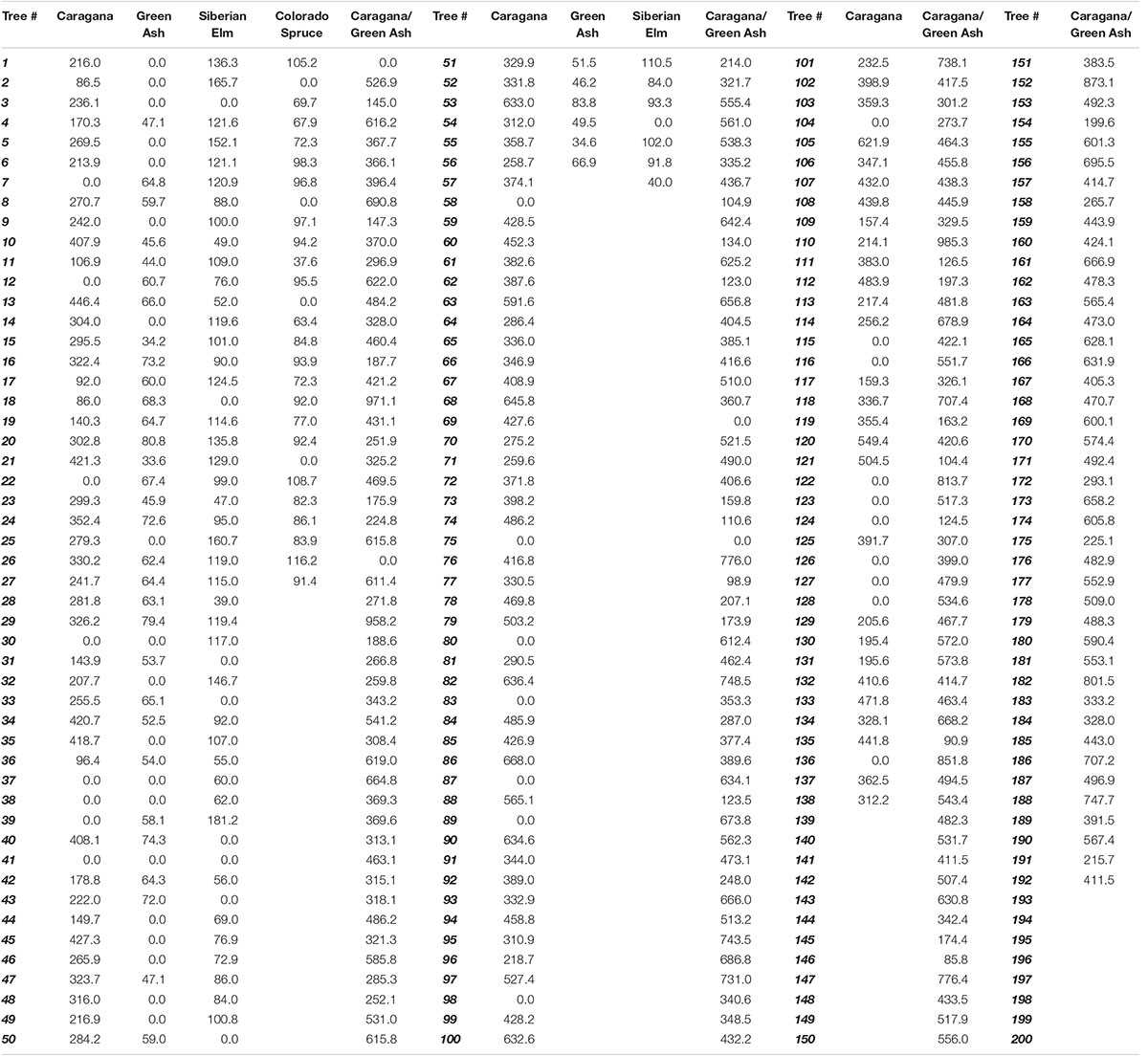
Table 2. Calculated circumference on the basis of cumulative basal area (mm), using measurements from different shelterbelts near Indian Head, SK, Canada.
To establish a recommendation in the model’s interface as to how many woody plants would have to be measured by the model user to achieve a representative average, we applied two methodologies. Using the thinnest and thickest stem of each shelterbelt, we calculated the variance and the average for use in the Student’s t-test for each respective shelterbelt. With this, we identified the number of required samples for being within a range of 5, 10, 15, and 20% of the actual measured mean with a probability of 80, 90, 95, and 99%, respectively. However, as potential model users (e.g., landowner) are more likely to measure groups of trees in close vicinity rather than observing statistical necessities, we further investigated how closely a rolling average of 3, 5, 10, 15, and 20 trees would approach the average of all measured trees.
The Holos Model (v. 4)
The Holos model is Agriculture and Agri-Food Canada’s whole-farm model, designed to answer “what if?” questions with respect to a landowner’s management decision effects with regards to the farm’s overall GHG budget. For this purpose, the model includes (in version 3) 18 major crops, as well as detailed estimates for beef, dairy, swine, and poultry, and more rudimentary estimates for other livestock. For the calculations of GHG from the different farm components, IPCC Tier 1 emission factors were employed initially, but for soil carbon, soil N2O, as well as beef and dairy enteric CH4 emissions, Tier 2 factors were implemented based on peer-reviewed publications. The model’s methodology (used equations and publication sources) is freely available upon request1.
The model’s underlying principle of the ‘virtual farm’ was initiated by Janzen et al. (2006), and resulted in the development of Holos Classic (available upon request) and subsequently Holos version 3 (download2). Both models simulate the emissions of a whole farm for 1 year, with the first version offering pre-defined mitigation strategies, and the latter offering a monthly time step for better livestock herd management input. In the outputs, the model lists emissions of N2O, CH4, and CO2, and also converts all emissions into CO2-equivalents. In the CO2 emissions, rough estimates for machinery use and irrigation are incorporated; however, the bulk of CO2 emissions come from upstream emissions (emissions created outside of the systems for inputs that are required for the operation of the system) in fertilizer, pesticide, and electricity production. Agricultural soils are considered to remain in equilibrium until certain management practices occur that cause pre-determined carbon changes (reduction of tillage or summer fallow, and switching from annual to perennial cropping), subsequently output as carbon offsets (negative CO2 emissions). The offsets also include user defined planted shelterbelts [aboveground C accumulation estimates based on Kort and Turnock (1999)].
Kröbel et al. (2012) argued that a whole-farm model should consider more than just GHG emission estimates, as many practices that aim to lower greenhouse gas emissions may inadvertently cause other impacts on the environment that may be as or even more undesirable. Starting with the implementation of a carbon budget module (Kröbel et al., 2016), the model is undergoing a transformation toward multi-year simulations, and many of its components (cropping, pasture, beef, dairy, and shelterbelts) are being updated in the process. The model is being written in C# (C Sharp) using an agile software development approach, and accordingly, the interface development is being stakeholder driven (through online meetings with potential end users who provided feedback on multiple iterations of the interface).
As the model’s primary users are scientists (the model is used in several AAFC and university led projects), farmers (small enterprises have used the model to direct-market their product as carbon neutral), and policy makers (the carbon credit program of Alberta province is based on Holos), a versatile interface is needed to fit the different requirements by the users and their potential knowledge level of the required inputs. For farmers, time requirements and simplicity are important factors. Regardless, for some operations they prefer a lot of detail to represent their farm as best as possible (e.g., beef producers asked for more detailed herd management inputs). The level of details required is similar to scientists who will use specific measurements to feed into the model. In both cases, however, in order to conduct a whole-farm analysis, some input requirements may be missing, which is where the model database attempts to provide representative average values to the model users. These are then also required by policy makers who do not have access to any farm level data, and who use the model to assess the effect of policy initiatives onto average (representative) farm systems.
Therefore, the interface of Holos version 4 is structured into three main stages: a current state, a timeline (for past and future states), and a detail input. The first stage provides an estimate of current GHG emission and potential offsets, while the timeline allows looking back (and forward) to see how patterns evolved over time (Kröbel et al., 2016), this includes the emissions estimates of nitrous oxide, methane, carbon dioxide, and ammonia, as well as leaching of nitrate. Both stages ask for long-term averages to ease user requirements and to better demonstrate the effect of management choices. The last stage allows the input of annually specific values (e.g., based on measurements) for more detailed investigations.
With respect to the shelterbelt component (Figure 2), the model asks for the number of rows, the species within each row, the length of each row, and the number of trees/shrubs in each row to calculate the present carbon storage in the shelterbelt as a way to ‘offset’ other emission sources. In the ‘timeline’ stage, additional input parameters are required: the planting year of the shelterbelt and the number of trees planted. Thus, the user can explore how carbon was accumulated in the shelterbelt over time, and the user can explore which species to plant in a new (renewed) shelterbelt when, for instance, focusing on carbon capture. In the detail input, number of trees, their circumference, and the row length can be adjusted for each individual year, which would allow for more locally specific and accurate estimates (if available).
Throughout the development, model stakeholders were engaged during online meetings to review the progress on the interface development, with a total of 4 online meetings taking place. Only the final results (Figure 2) are shown.
Equations and Calculation Procedures of the New Shelterbelt Component
To update the allometric equation from Kort and Turnock (1999), we adopted the relationships identified by Amichev et al. (2017), derived from a dataset of measured shelterbelts in the province of Saskatchewan, Canada. The updated relationships use the circumference of the tree trunk (measured at 1.30 m height outside tree bark) to calculate the aboveground accumulated carbon in the living tree biomass (Amichev et al., 2017), rather than using the age of the tree (Kort and Turnock, 1999), a much less reliable method and a difficult to ascertain value in hindsight.
where,
Ctree Above-ground C stocks per tree (kg C tree–1).
Carbonconcentration(trees) Carbon concentration of all tree parts (kg kg–1) set to 0.5 kg kg–1 (Kurz et al., 2009).
a Coefficient a (Table 3).
b Coefficient b (Table 3).
tree circumference cumulative tree stem circumference (cm) at 1.30 m tree height (breast height) measured outside tree bark.
circumferencei circumference (cm) at breast height of each individual stem i (i = 1,2,…,n) of a tree with multiple stems.
Using Eqs 2 and 3 to calculate the carbon accumulation in a single tree, row length and planting density will provide the number of trees to be considered (Table 3). To drive the model’s calculations, our team attempted to limit inputs to data that every-day-users can easily obtain. To start assessing the current state, the row length, number of trees (or average spacing), and average circumference (at 1.30 m breast height for trees and 30 cm height for Caragana) are required. With this information, the model calculates the currently accumulated carbon in the aboveground biomass of a single tree, which is used for a series of look-up values in the shelterbelt database.
Lookup Database for Past and Future Shelterbelt Growth and C Additions
As the allometric equations cannot be used to calculate the belowground biomass of a tree, we are relying on previous (3PG) model simulations (Amichev et al., 2016) for an estimate of tree age specific above-/below-ground biomass fractions to estimate the total tree biomass. This total tree biomass is used to calculate the total shelterbelt biomass, and using tree mortality (the ratio, as percentage, of missing/dead over planted trees) and age, are compared to a lookup table of average shelterbelt biomass amounts. The derived fraction of actual (at the time of observation) versus predicted carbon accumulation is used to back-estimate how the carbon accumulation progressed over time in the past, as well to forecast carbon accumulation into the future.
The average shelterbelt biomass and carbon amounts in the lookup table were previously determined with the tree growth (3PG model) and carbon dynamics (CBM-CFS3 model) models which were adapted for shelterbelt systems by Amichev et al. (2016). In that study, historic climate data were used along with extensive field data to parameterize both models for shelterbelt systems (Amichev et al., 2016). For carbon accumulations in the future, a high (A2) climate forcing scenario was used for the period 2016–2075 (Canadian Centre for Climate Modelling and Analysis [CCCMA], 2017, third generation Coupled Global Climate Model). The values in the lookup tables were used to interpolate the biomass and carbon amounts for any farm’s unique shelterbelt design, based on previously generated data for different tree species, ages (1–60 years), spacing (2.0, 3.5, and 5.0 m), and mortality levels (0, 15, 30, and 50%) (Amichev et al., 2017).
The lookup tables in the database also used for mixed shelterbelts through simulated ‘mortalities.’ For example, the correct number of live trees for the first species (N1 = 43) was calculated by modifying the total number of shelterbelt trees (NT = 188) by a simulated ‘mortality’ of 77.6%, and for the second species (N2 = 145) it was modified by a simulated ‘mortality’ of 24.5%. Two of the measured tree species in our measurement dataset are not covered by the database. We hence decided to summarize the estimation methods to create average estimates for coniferous and deciduous trees, respectively. This was done by using data looked up for White Spruce and Scots Pine individually, and then calculate the average between the two for an average coniferous tree (to be used here for Colorado Spruce). Likewise, Manitoba Maple and Green Ash data were looked up and then were averaged for an average deciduous tree (to be used here for Siberian Elm).
The database contains shelterbelt data of each ecodistrict3 of Saskatchewan (EcoRegions Working Group, 1989). We assorted and averaged these data according to the established Canadian plant hardiness zones4 (McKenney et al., 2001) in order to allow the appropriate utilization in other provinces of Canada (with an assumed increase in error that cannot be corrected until more specific data become available). These established averages will serve as a representative growth curve that will be used in estimates over time to (back-) calculate the circumference over time as a fraction determined by the user-supplied current state (e.g., if the current circumference is 50% of the representative growth curve, all past carbon accumulation estimates looked up from the data tables will be reduced accordingly).
Results and Discussion
Model Input Recommendations
In order to calculate the carbon accumulation in a present shelterbelt, the model requires the user to measure circumference(s) of their tree(s) as an input into the model. While measuring the whole length of a shelterbelt would certainly reacquaint anyone with the state and health of their shelterbelt, it appears an overly expansive ask for the use of a model. We hence set out to investigate what is the minimum required number of tree trunks that would be needed to be measured in order to properly assess the carbon accumulation in the shelterbelt with a degree of certainty (Tables 4, 5).
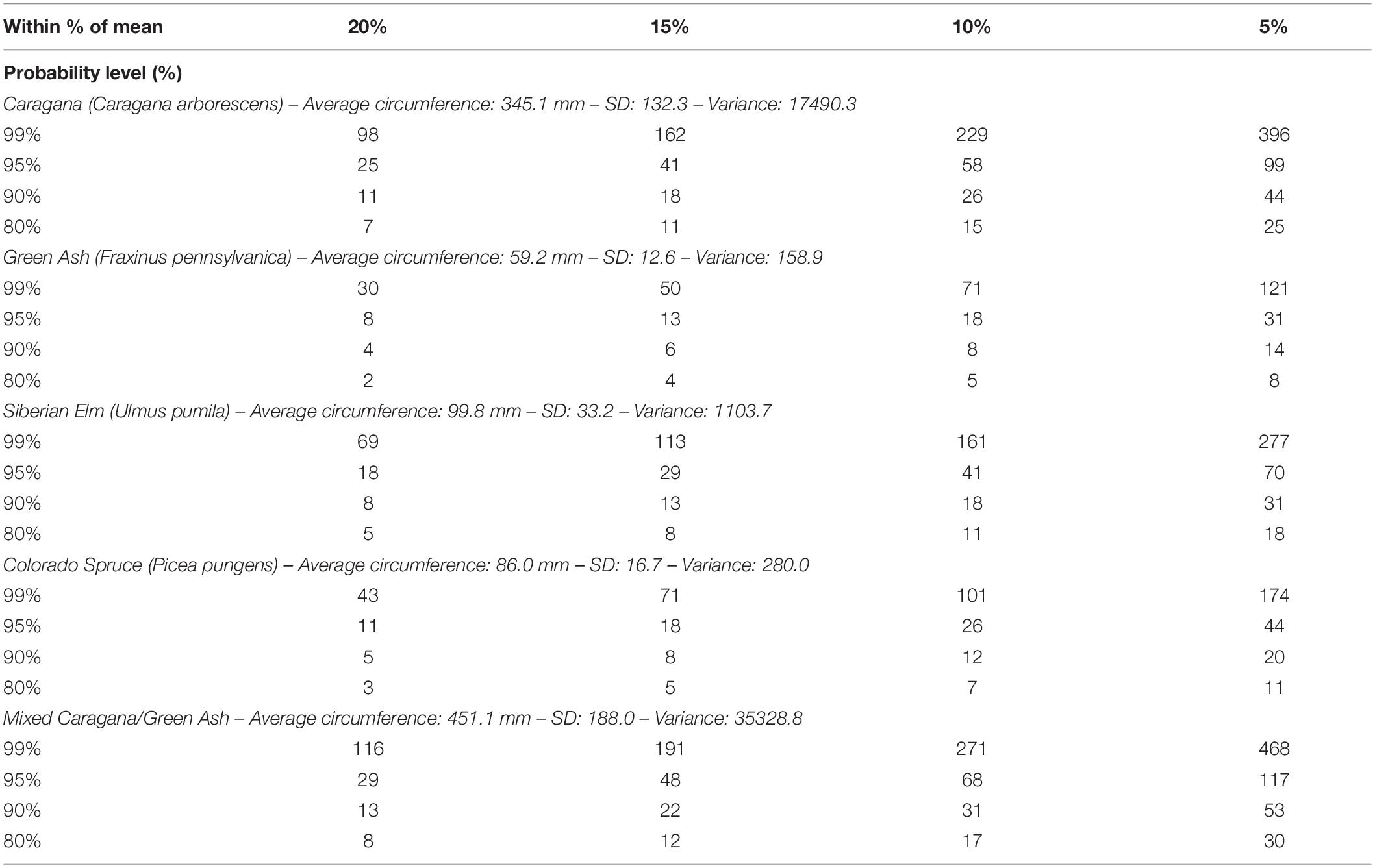
Table 4. t-Test determined required number of samples for a representative estimation of average circumference for different shelterbelt species, based on measured shelterbelts from near Indian Head, SK, Canada.
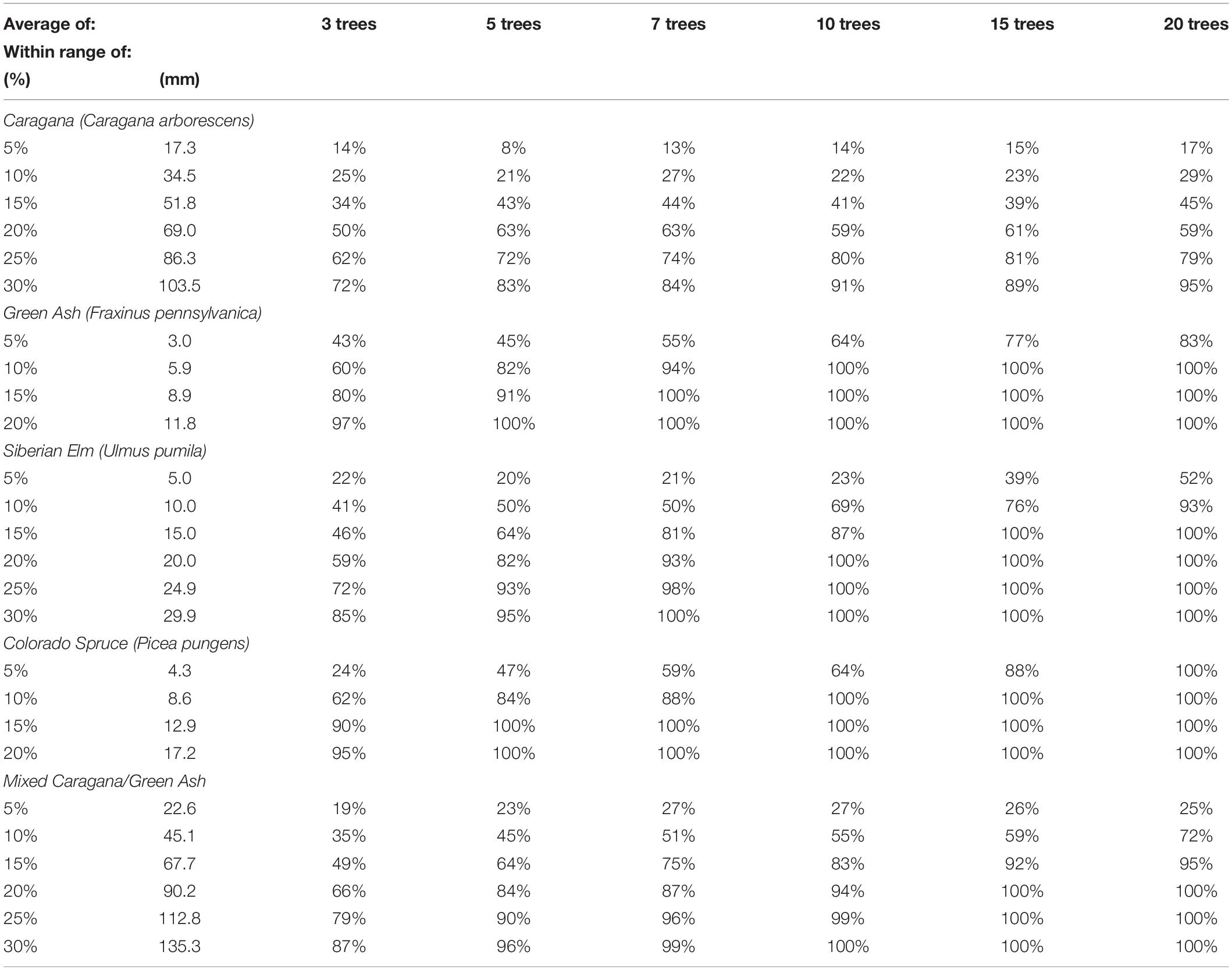
Table 5. Estimating the accuracy of representative measurements of circumference (and derived averages) for different shelterbelt species, using measurements from near Indian Head, SK, Canada.
The statistical analysis suggests that the inherent variability of tree growth within shelterbelts would require a large number of tree measurements to create a close estimate to the real average with a high confidence (Table 4). The requirements were much higher for Caragana and Siberian Elm, but considerably lower for Colorado Spruce and Green Ash. Thus, with an expectation that a maximum of 10 trees would be measured by a user on their own volition, an 80% probability would be achieved to be within 15 and 10% of the average for Caragana and Siberian Elm, respectively, while for Green Ash and Colorado Spruce the same number of measurements would give a 90% confidence estimate that is within 10 and 15% of the real mean (Table 4).
Assuming that a user would rather measure groups of trees than properly random sampled trees of within a shelterbelt, we calculated the rolling mean of cumulative circumferences within each shelterbelt dataset to see how often a randomly selected group would approximate the real average. Rolling means met the average of the measured tree shelterbelts within a 15% error range quite reliably with seven trees measured (Green Ash, Siberian Elm, and Colorado Spruce in 100, 81, and 100% of the cases), while for Caragana the error range increased to 30% (Table 5). Increasing the number of measured trees from 7 to 10 allowed to fit within the above mentioned error ranges more reliably, but did not effectively decrease the error range of the circumference. By increasing the sample size to measuring 15 or 20 trees for an estimation of the average shelterbelt circumference, would decrease the error range to 10% for Green Ash, Siberian Elm, and Colorado Spruce, while for Caragana, the error range would stay at 25%. Based on the findings observed in this study, we recommend that a user would need at least seven trees to measure circumferences of their trees as an input into the model.
Carbon Accumulation Estimates
When assessing the accumulation of carbon in the planted trees and their respective environment, it needs to be taken into account that their respective age is unequal (Caragana shelterbelt being the youngest at 24 years, and the Colorado Spruce shelterbelt being the oldest at 38 years). Furthermore, the single tree growth has to be put into the context of the complete shelterbelt, which requires considering trees that have not survived, carbon deposited through leaf litter, as well as the continuous loss of carbon from the soil (see Figure 5). Furthermore, different species have different growth rate patterns and their management (or lack thereof) determines how much of that potential can be realized (Table 6). In this sense, however, the results of these estimates are not representative, but rather meant to demonstrate the models capability to assess individual landowner’s shelterbelts with sufficient certainty.
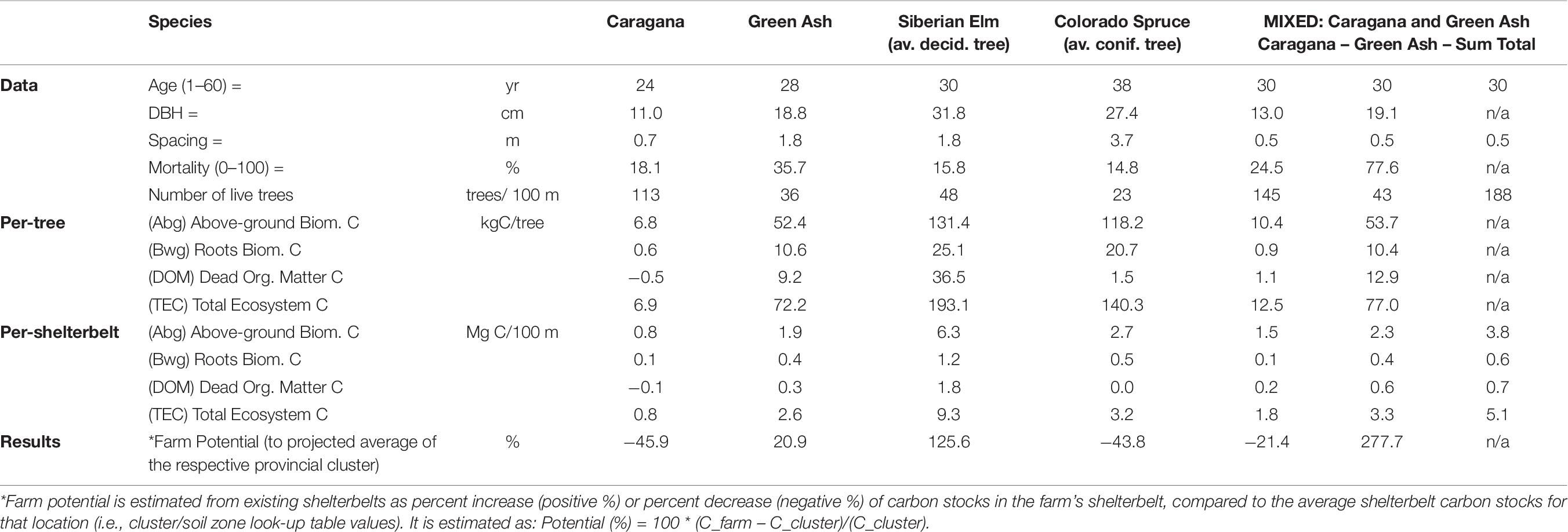
Table 6. Carbon accumulation estimates for different shelterbelt of varying age and mortality on a 100 m row length, using measurements from near Indian Head, SK, Canada (Note: the mixed shelterbelt has simulated “mortalities” used to avoid double-counting of live trees of the two species for one and the same planting location).
The Siberian Elm shelterbelt accumulated the most carbon of all shelterbelts, double than the mixed Caragana/Green Ash shelterbelt, triple of the Colorado Spruce shelterbelt, and more than 10 times the carbon accumulation of the pure Caragana shelterbelt (Figure 3). The growth rate of the Siberian Elm also caused to diminish the early growth carbon loss seen for other trees, thus turning the system quickly into a carbon sink after already 3 years (Figures 4, 5). It is remarkable in this sense that the Siberian Elm had, in the total budget, the smallest aboveground fraction contributing to the total (68% of TEC), and the largest dead organic matter accumulation (19% of TEC).
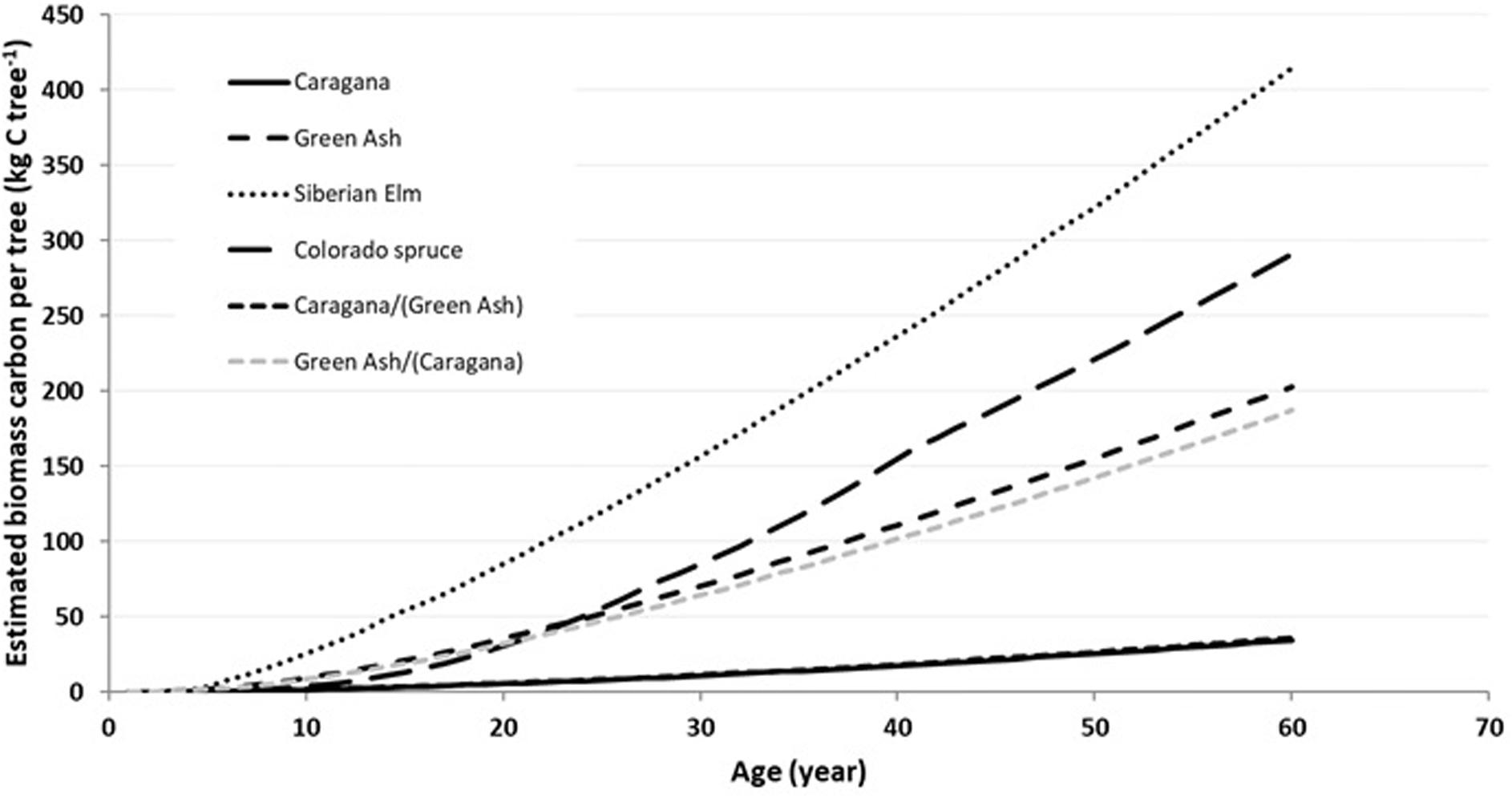
Figure 3. Estimated carbon accumulation in the living biomass of trees grown near Indian Head, SK, Canada, using adjusted values from the representative estimates of Cluster BLK 3 (Amichev et al., 2017).
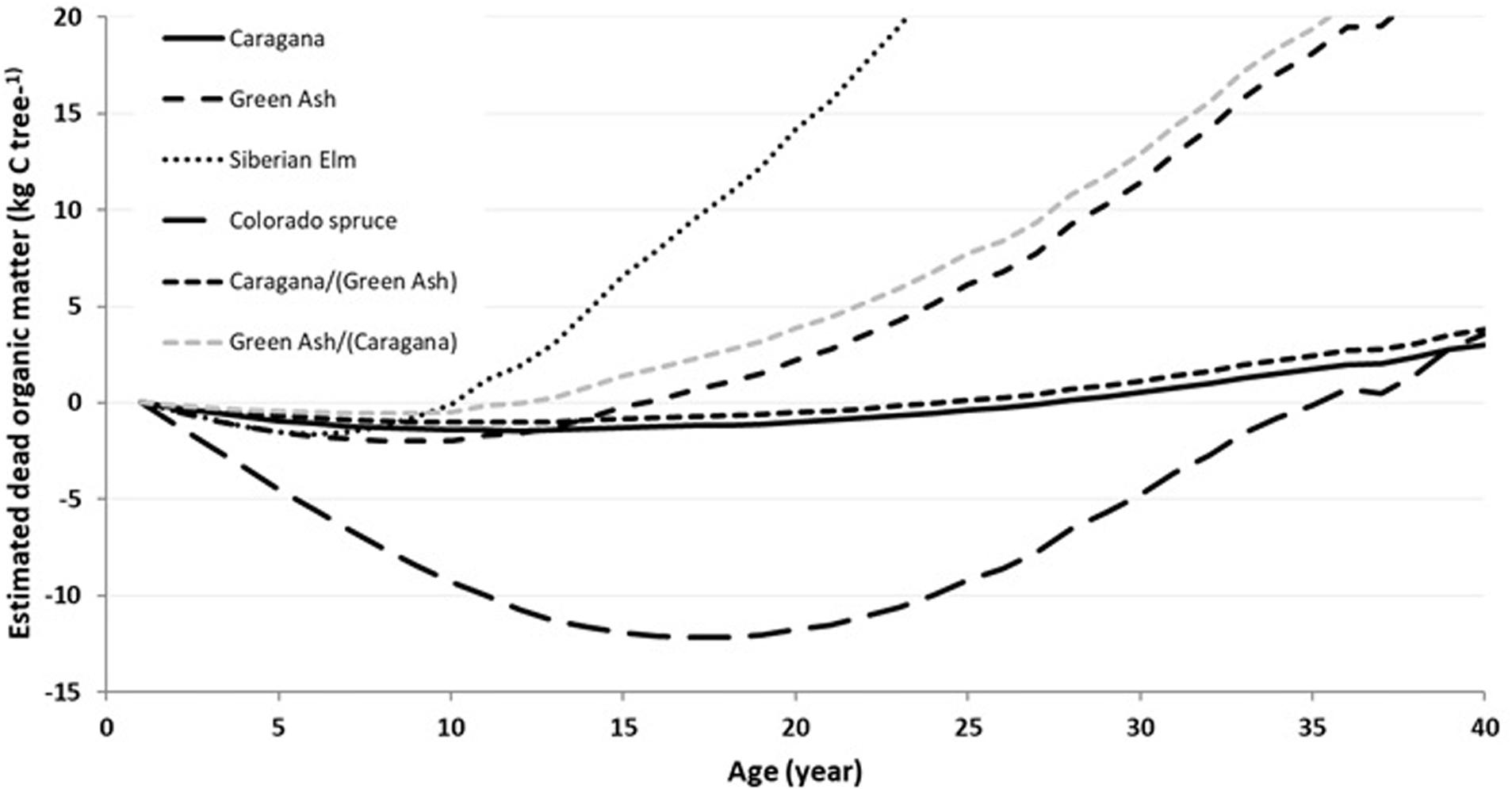
Figure 4. Estimated carbon change in the dead organic matter underneath trees grown near Indian Head, SK, Canada, using adjusted values from the representative estimates of Cluster BLK 3 (Amichev et al., 2017).
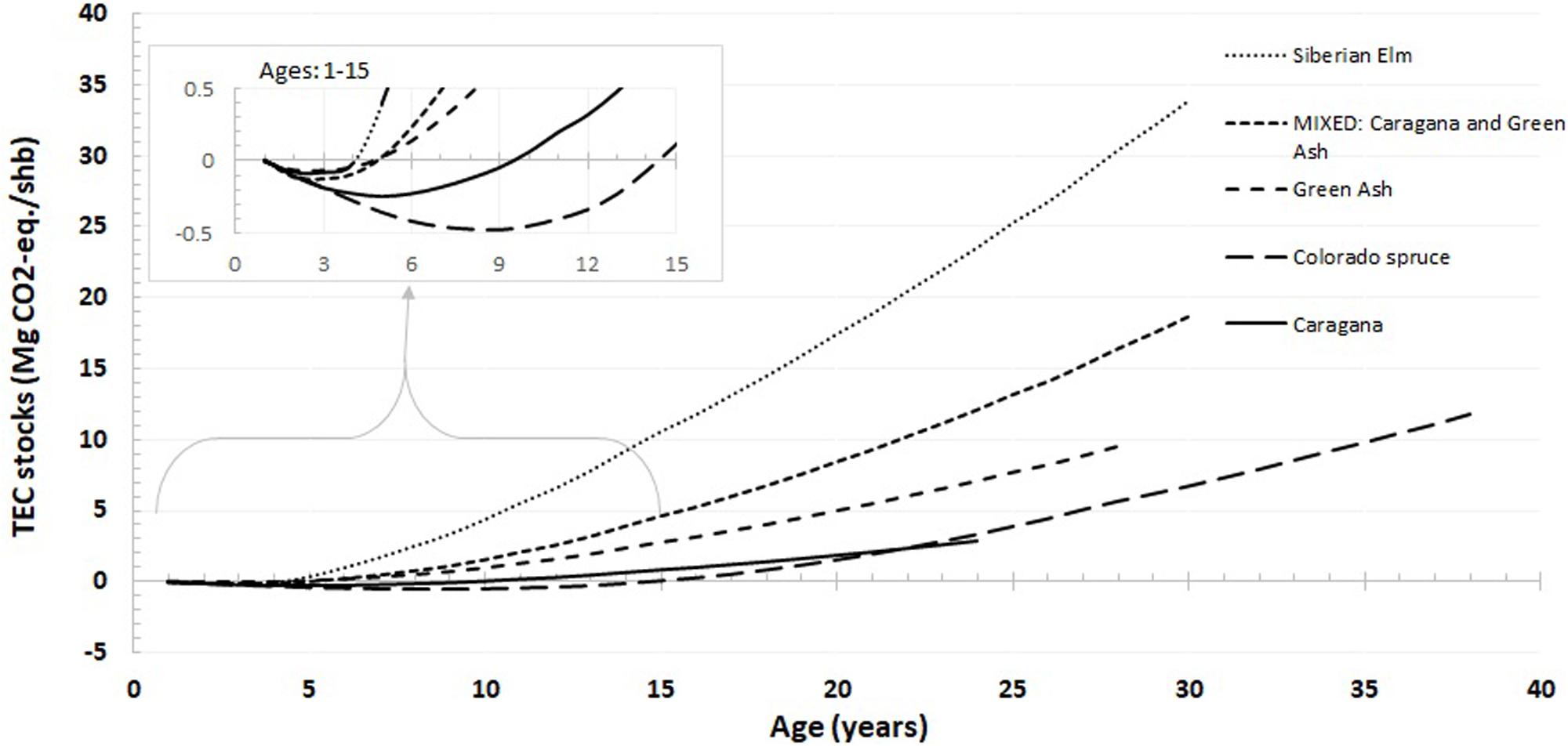
Figure 5. Estimated Total Ecosystem Carbon (TEC) accumulation through different shelterbelts (of different age) near Indian Head, SK, Canada, using adjusted values from the representative estimates of Cluster BLK 3 (Amichev et al., 2017).
The only shelterbelt somewhat competing with the Siberian Elm was the Caragana/Green Ash shelterbelt (Table 6), even though better management (tree survival) and thus realized growth potential meant that the Caragana in mix with Green Ash accumulated double the carbon of the pure Caragana shelterbelt (albeit with six additional growth years, and closer spacing), while the Green Ash accumulated roughly 30% more than the pure shelterbelt stand (Table 6). For Caragana, this difference was purely on the basis of surviving shrubs, as the actual carbon accumulation per shrub was equal (Figure 3). With respect to TEC, the Green Ash showed similar contributions patterns as the Siberian Elm (70 and 17% for aboveground and dead organic matter), but for the Caragana, a distinctly larger fraction of carbon is in the aboveground biomass (84%) and a much smaller fraction in the dead organic matter (9%).
For the Colorado Spruce shelterbelt, which was the oldest, but also the shelterbelt with the fewest trees, a considerable amount of carbon was still accumulated (Table 6). However, downscaled on a per tree basis, a Siberian Elm tree stored about 25% more carbon than a Colorado Spruce (which had 8 years more to grow) (Figure 3). The Colorado Spruce also requires 15 years to become a carbon sink (Figure 5). This may be due to the fact that almost all the TEC is located in the living above- and below-ground biomass (84 and 15%, respectively), while there is almost no dead organic matter accumulated (Figure 4). In general, it takes a much longer time for dead organic matter of coniferous shelterbelts (i.e., fallen needles, branches, bark) to decompose and be added into the soil carbon pool, compared to deciduous shelterbelts; this prolonged time for the soil under a Colorado Spruce shelterbelt to act as carbon source is reflected in Figure 4.
All the shelterbelts measured in this study were from black soil zones in Saskatchewan, Canada, and were within 100 km distance. Crop management practices are representative of the region; however, there are noticeable differences in the management of shelterbelts among the sites studied. Based on the TEC measured per shelterbelts, Siberian Elm showed the highest potentials of carbon sequestration in this study. If carbon sequestration is the sole objective of a user, then this species would be the best candidate among the species studied. However, shelterbelts provide many other benefits that should not be ignored. While Caragana shelterbelt is found the least potential in terms of TEC, the species provides added benefits by fixing atmospheric nitrogen and a dense vegetation boundary line to protect the crops and soils from wind damage. Another shelterbelt, Colorado Spruce, is a tall evergreen tree and not only protects crops from wind damage but also provides essential habitat for wildlife. Regardless, based on the findings of this study, we are confident of the capability of the model to assess individual landowner’s shelterbelts with sufficient certainty.
Biomass Contributions and Other Benefits of Shelterbelts
Historically shelterbelts were planted in the Canadian Prairies since 1903 to protect the soils and crops from wind damage and wind erosion as well as to provide shelter for livestock and farmyards from strong wind during cold winter and hot summer months (Mayrinck et al., 2019). However, with the changes in production technologies, such as adoption of zero tillage and cover crops, the emphasis on the benefits of shelterbelts has declined. Many large landowners view shelterbelts as a barrier in maneuvering large machinery. Large equipment takes a longer time to go around these non-crop areas during the short window of spring and fall farm operations (seeding, spraying, and harvesting). Small landowners, though more likely to retain shelterbelts than larger landowners, may view these areas as non-productive areas and often cleared and converted them into croplands to increase production areas. Such activities are responsible for decreasing shelterbelts in the prairie region.
Although shelterbelts may not seem important to many landowners for protecting soils and crops from strong winds, there is still a need to examine other benefits provided by these areas before removing them from farmlands. In a recent study, shelterbelts are shown to improve crop yield by modifying microclimate in the adjacent crops (Osorio et al., 2019). The increase in yield compensated for the footprint of the shelterbelt and yet boosted yield in soybeans and wheat. Shelterbelts provide critical semi-natural habitats to pollinators and other beneficial insects, birds, mammals, and other wildlife within large monoculture fields of agricultural crops (reviewed in Dix et al., 1995; Mize et al., 2008). Alongside the benefits mentioned above, shelterbelts can have a significant effect on mitigating GHG emissions from Canadian agricultural activity (Ward and de Gooijer, 2017). For agroforestry to be successful as a mitigation tool, the plant materials comprising the agroforestry practice must themselves have adaptive capacity to future shifts in conditions due to climate change (Lengnick, 2015). To optimize the potential of agroforestry as a GHG mitigation tool, species selection (e.g., growth speed and lifespan) will be important (Amadi et al., 2016).
Tree species currently used and potentially available for use in agroforestry have potential to be susceptible to erratic and extreme weather events, as well as climate-induced fluctuations in insects and pathogens (Fuhrer, 2003; Allen et al., 2010). For example, a primary species that was historically used in agroforestry plantings, Siberian Elm, is no longer recommended because it is a host for the banded elm bark beetle (Scolytus schevyrewi) (Negron et al., 2005) while the recommendation of Green Ash is becoming questionable with the emergence of the emerald ash borer (Agrilus planipennis) moving into new ecosystems across Canada.
On the other hand, hybrid poplar (Populus spp.) are widely planted as shelterbelts in the Canadian prairies (>5.68 million trees, 4144 km in length; Amichev et al., 2017) selected to be cold hardy, drought tolerant, pest and disease resilient. At age 60 years, hybrid poplar attain 15–17 m in height with a mean aboveground biomass ranging from 397 to 634 OD Mg km–1 and DBH of 52–63 cm. In the current study, we did not measure the circumference of hybrid poplar for use in the Holos model due to the reason that all the shelterbelts included are field shelterbelts. The hybrid poplar shelterbelts available in the region are farmyard shelterbelts that serve a different function, such as protect farmhouse and livestock from wind and cold. For consistency purposes, we compared five field shelterbelts in this study. In the future we plan to include hybrid poplar in a broader context because when compared with the five shelterbelt tree/shrub species tested in the Holos model, hybrid poplar display inherent capacity to grow fast with the largest potential to sequester carbon (average aboveground biomass for Green Ash – 32 Mg km–1; White Spruce – 41 Mg km–1; hybrid poplar – 105 Mg km–1; Kort and Turnock, 1999).
It will be essential to determine vulnerability of tree species under modeled climate change scenarios in order to position the necessary production and delivery of suitable plant materials and to provide science-based guidance for plant selection (Ward, 2016). Therefore, a key need is to test a range of woody plant germplasm to identify sources of germplasm that is adapted to both current and future conditions in Canada (Silim, 2004; Johnston et al., 2009). However, since research on climate change adapted plant materials is limited and due to the longevity of woody species one is at the mercy of using diversity as a key principle in developing climate change adapted agroforestry plantings (Schoeneberger et al., 2012) which is fundamentally selecting a variety of plant species that will succeed under shifting weather and climate change conditions. Equally, tree breeding programs can expand selection options, such as the trait-assisted selection from diverse set of germplasm collection (Soolanayakanahally, 2010; Keller et al., 2011) to generate woody feedstocks with high resource-use efficiencies (particularly, water and nutrients) for present and future climates.
Conclusion
The whole-farm model Holos was updated with a new allometric equation for its shelterbelt component to more accurately estimate the carbon accumulation in Canadian shelterbelts. Using measured shelterbelts near Indian Head, SK, Canada, the model calculated that a Siberian Elm shelterbelt accumulated most carbon, followed by a mixed Caragana/Green Ash shelterbelt, while single stands of Caragana, Green Ash or Colorado Spruce had poor carbon accumulation in comparison. However, these results are in dependence of actual shelterbelt management (and age) more so than the species selection.
Trees that have excelled in carbon accumulation in the past, may not perform as well in a changing climate, and are already under threat due to invading species. If shelterbelts are to be an active component in our Canadian climate commitments, investment will be needed both to build the genetic potential for continuing tree growth and the distribution of new cultivars across the Canadian landscape. The Holos model can be of assistance to showcase the carbon storage potential to model users, either in farming or policy making.
Going forward in an attempt to utilize shelterbelts as a potential ‘negative emissions’ sink for Canadian GHG reduction targets, the selection of species needs to be reassessed based on other factors than just the potential carbon accumulation. There has been little documented research on the ecological and economic benefits of shelterbelts in promoting crop productivity and ecological diversity in intensely cropped agricultural landscapes in Saskatchewan. There is a need to measure the benefits or services provided by field shelterbelts, such as increased pollination from native bees and predation of harmful pests by beneficial insects and birds, to determine whether it is advantageous for landowners to maintain these habitat areas on the landscape.
Data Availability Statement
All datasets generated for this study are included in the article.
Author Contributions
RK and FA were the main authors. JM and AM conceived the software development. YN and LP conducted the shelterbelt measurements. BA guided and reviewed the model updated and provided data access. RS, TW, CL, and KV provided feedback and discussion throughout the work and for the manuscript. All authors contributed to the article and approved the submitted version.
Funding
This study was provided by Agriculture and Agri-Food Canada’s (AAFC) Canadian Agriculture Partnership (CAP) program for the accomplishment of this work, while underlying data were originally funded through the Agricultural Greenhouse Gas Program (AGGP), also financed through AAFC’s CAP program.
Conflict of Interest
The authors declare that the research was conducted in the absence of any commercial or financial relationships that could be construed as a potential conflict of interest.
Acknowledgments
We would like to acknowledge funding that contributed to the writing to this manuscript.
Footnotes
- ^ aafc.holos.aac@canada.ca
- ^ https://www.agr.gc.ca/eng/scientific-collaboration-and-research-in-agriculture/agricultural-research-results/holos-software-program/?id=1349181297838
- ^ https://open.canada.ca/data/en/dataset/fe9fd41c-1f67-4bc5-809d-05b62986b26b
- ^ http://sis.agr.gc.ca/cansis/nsdb/climate/hardiness/index.html
References
Agriculture and Agri-Food Canada [AAFC] (2018). Agriculture and Agri-Food Canada. Benefits of agroforestry. Available online at: http://www.agr.gc.ca/eng/science-and-innovation/agricultural-practices/agroforestry/benefits-of-agroforestry/?id=1344633257343 (accessed August 15, 2019).
Agriculture and Agri-Food Canada [AAFC], Agri-Environment Service Branch [AESB], and Agroforestry Development Centre [ADC] (2010). Prairie Shelterbelt Program: Frequently Asked Questions. Ottawa: Government of Canada, 26.
Alemu, A. W., Amiro, B. D., Bittman, S., MacDonald, D., and Ominski, K. H. (2017a). Greenhouse gas emission of Canadian cow-calf operations: a whole-farm assessment of 295 farms. Agricult. Syst. 151, 73–83. doi: 10.1016/j.agsy.2016.11.013
Alemu, A. W., Janzen, H. H., Little, S., Hao, X. Y., Thompson, D. J., Baron, V., et al. (2017b). Assessment of grazing management on farm greenhouse gas intensity of beef production systems in the Canadian Prairies using life cycle assessment. Agricult. Syst. 158, 1–13. doi: 10.1016/j.agsy.2017.08.003
Allen, C. D., Macalady, A. K., Chenchouni, H., Bachelet, D., McDowell, N., Vennetier, M., et al. (2010). A global overview of drought and heat-induced tree mortality reveals emerging climate change risks for forests. Forest Ecol. Manag. 259, 660–684. doi: 10.1016/j.foreco.2009.09.001
Amadi, C. C., Van Rees, K. C. J., and Farrell, R. E. (2016). Greenhouse gas mitigation potential of shelterbelts: estimating farm-scale emission reductions using the Holos model. Can. J. Soil Sci. 97, 353–367. doi: 10.1139/CJSS-2016-2017
Amichev, B. Y., Bentham, M. J., Kulshreshtha, S. N., Laroque, C. P., Piwowar, J. M., and Van Rees, K. C. J. (2017). Carbon sequestration and growth of six common tree and shrub shelterbelts in Saskatchewan, Canada. Can. J. Soil Sci. 97, 368–381. doi: 10.1139/cjss-2016-0107
Amichev, B. Y., Bentham, M. J., Kurz, W., Laroque, C. P., Kulshreshtha, S. N., Piwowar, J. M., et al. (2016). Carbon sequestration by white spruce shelterbelts in Saskatchewan, Canada: 3PG and CBM-CFS3 model simulations. Ecol. Model. 325, 35–46. doi: 10.1016/j.ecolmodel.2016.01.003
Baah-Acheamfour, M., Chang, S. X., Bork, E. W., and Carlyle, C. N. (2017). The potential of agroforestry to reduce atmospheric greenhouse gases in Canada Insight from pairwise comparisons with traditional agriculture, data gaps and future research. Forest. Chronicle 93, 180–189. doi: 10.5558/tfc2017-024
Bastin, J. F., Finegold, Y., Garcia, C., Mollicone, D., Rezende, M., Routh, D., et al. (2019). The global tree restoration potential. Science 365, 76–79. doi: 10.1126/science.aax0848
Beauchemin, K. A., Janzen, H. H., Little, S. M., McAllister, T. A., and McGinn, S. M. (2010). Life cycle assessment of greenhouse gas emissions from beef production in western Canada: a case study. Agricult. Syst. 103, 371–379. doi: 10.1016/j.agsy.2010.03.008
Bonesmo, H., Beauchemin, K. A., Harstad, O. M., and Skjelvåg, A. O. (2013). Greenhouse gas emission intensities of grass silage based dairy and beef production: a systems analysis of Norwegian farms. Livestock Sci. 152, 239–252. doi: 10.1016/j.livsci.2012.12.016
Bonesmo, H., Little, S. M., Harstad, O. M., Beauchemin, K. A., Skjelvåg, A. O., and Sjelmo, O. (2012). Estimating farm-scale greenhouse gas emission intensity of pig production in Norway. Acta Agricult. Scand. Sec. A 62, 318–325. doi: 10.1080/09064702.2013.77091
Canadian Centre for Climate Modelling and Analysis [CCCMA] (2017). The Third Generation Coupled Global Climate Model. British Columbia: CCCMA.
Church, J. S., Raymond, A. F., Moote, P. E., Van Hamme, J. D., and Thompson, D. J. (2015). Investigating the carbon footprint of cattle grazing the Lac du Bois Grasslands of British Columbia. J. Ecosyst. Manag. 15, 1–14.
DiBartolomeis, M., Kegley, S., Mineau, P., Radford, R., and Klein, K. (2019). An assessment of acute insecticide toxicity loading (AITL) of chemical pesticides used on agricultural land in the United States. PLoS One 14:e0220029. doi: 10.1371/journal.pone.0220029
Dix, M. E., Johnson, R. J., Harrell, M. O., Case, R. M., Wright, R. J., Hodges, L., et al. (1995). Influence of trees on abundance of natural enemies of insect pests: a review. Agrofor. Syst. 29, 303–311. doi: 10.1007/bf00704876
Environment and Climate Change Canada [ECCC] (2019). Pollutant Inventories and Reporting Division. National Inventory Report 1990–2017: Greenhouse Gas Sources and Sinks in Canada. Cat. No.: En81-4/1E-PDF. Atlantic: ECCC.
Environment and Climate Change Canada [ECCC] (2020). Canadian Environmental Sustainability Indicators: Progress towards Canada’s greenhouse gas emissions reduction target. Cat. No.: En4-144/48- 2019E-1-PDF. Available online at: https://www.canada.ca/en/environment-climate-change/services/environmental-indicators/progress-towards-canada-greenhouse-gas-emissions-reduction-target.html (accessed August 14, 2019).
EcoRegions Working Group (1989). Ecoclimatic Regions of Canada, First Approximation. Ecological Land Classification Series, No. 23. Ottawa: Environment Canada.
European Environment Agency (2015). Agriculture and Climate Change. Available online at: https://www.eea.europa.eu/signals/signals-2015/articles/agriculture-and-climate-change (accessed January 30, 2015).
Fuhrer, J. (2003). Agroecosystem responses to combinations of elevated CO2, ozone, and global climate change. Agricult. Ecosyst. Environ. 97, 1–20. doi: 10.1016/s0167-8809(03)00125-7
Gregorich, E. G., Janzen, H. H., Ellert, B. H., Helgason, B. L., Qian, B., Zebarth, B. J., et al. (2017). Litter decay controlled by temperature, not soil properties, affecting future soil carbon. Glob. Change Biol. 23, 1725–1734. doi: 10.1111/gcb.13502
Gülzari, ŞÖ, Åby, B. A., Persson, T., Höglind, M., and Mittenzwei, K. (2017). Combining models to estimate the impacts of future climate scenarios on feed supply, greenhouse gas emissions and economic performance on dairy farms in Norway. Agricult. Syst. 157, 157–169. doi: 10.1016/j.agsy.2017.07.004
Gülzari, ŞÖ, Ahmadi, B. V., and Stott, A. W. (2018). Impact of subclinical mastitis on greenhouse gas emissions intensity and profitability of dairy cows in Norway. Prevent. Vet. Med. 150, 19–29. doi: 10.1016/j.prevetmed.2017.11.021
Guyader, J., Little, S., Kröbel, R., Benchaar, C., and Beauchemin, K. A. (2017). Comparison of greenhouse gas emissions from corn-and barley-based dairy production systems in Eastern Canada. Agricult. Syst. 152, 38–46. doi: 10.1016/j.agsy.2016.12.002
Ha, T. V., Amichev, B. Y., Belcher, K. W., Bentham, M. J., Kulshreshtha, S. N., Laroque, C. P., et al. (2019). Shelterbelt Agroforestry Systems Inventory and Removal Analyzed by Object-based Classification of Satellite Data in Saskatchewan, Canada. Can. J. Remote Sens. 45, 246–263. doi: 10.1080/07038992.2018.1540297
Intergovernmental Panel on Climate Change [IPCC] (2019). IPCC Special Report on Climate Change, Desertification, Land Degradation, Sustainable Land Management, Food Security, and Greenhouse gas fluxes in Terrestrial Ecosystems. Geneva: IPCC.
Janzen, H. H., Angers, D. A., Boehm, M., Bolinder, M., Desjardins, R. L., Dyer, J. A., et al. (2006). A proposed approach to estimate and reduce net greenhouse gas emissions from whole farms. Can. J. Soil Sci. 86, 401–418. doi: 10.4141/s05-101
Johnston, M. H., Campugna, M., Gray, P. A., Kope, H. H., Loo, J. A., Ogden, A. E., et al. (2009). Vulnerability of Canada’s Tree Species to Climate Change and Management Options for Adaptation: An Overview for Policy Makers And Practitioners. Ottawa, ON: Canadian Council of Forest Ministry.
Keller, S. R., Soolanayakanahally, R. Y., Guy, R. D., Silim, S., Olson, M. S., and Tiffin, P. (2011). Climate-driven local adaptation of ecophysiology and phenology in balsam poplar, Populus balsamifera L. (Salicaceae). Am. J. Bot. 98, 99–108. doi: 10.3732/ajb.1000317
Kort, J. (1988). Benefits of windbreaks to field and forage crops. Agricult. Ecosyst. Environ. 22–23, 165–190. doi: 10.1016/0167-8809(88)90017-90015
Kort, J., Bank, G., Pomeroy, J., and Fang, X. (2012). Effects of shelterbelts on snow distribution and sublimation. Agrofor. Syst. 86, 335–344. doi: 10.1007/s10457-011-9466-9464
Kort, J., and Turnock, R. (1999). Carbon reservoir and biomass in Canadian prairie shelterbelts. Agroforestry Systems 44, 175–186.
Kröbel, R., Bolinder, M. A., Janzen, H. H., Little, S. M., Vandenbygaart, A. J., and Kätterer, T. (2016). Canadian farm-level soil carbon change assessment by merging the greenhouse gas model Holos with the Introductory Carbon Balance Model (ICBM). Agricult. Syst. 143, 76–85. doi: 10.1016/j.agsy.2015.12.010
Kröbel, R., Janzen, H. H., Beauchemin, K. A., Bonesmo, H., Little, S. M., and McAllister, T. A. (2012). A proposed approach to estimate and reduce the environmental impact from whole farms. Acta Agricult. Scand. Sec. A 62, 225–232. doi: 10.1080/09064702.2013.770912
Kulshreshtha, S., Van Rees, K., Hesseln, H., Johnston, M., and Kort, J. (2011). “Issues in agroforestry development on the canadian prairies,” in Handbook on Agroforestry: Management Practices and Environmental Impact, Ed. L. Kellimore (Hauppauge, NY: Nova Science Publishers), 91–127.
Kurz, W. A., Dymond, C. C., White, T. M., Stinson, G., Shaw, C. H., Rampley, G. J., et al. (2009). CBM-CFS3: a model of carbon-dynamics in forestry and land-use change implementing IPCC standards. Ecol. Model. 220, 480–504. doi: 10.1016/j.ecolmodel.2008.10.018
Lengnick, L. (2015). Resilient Agriculture: Cultivating Food Systems for A Changing Climate. Gabriola Island, BC: New Society Publishers, 288.
Little, S. M., Benchaar, C., Janzen, H. H., Kröbel, R., McGeough, E. J., and Beauchemin, K. A. (2017). Demonstrating the Effect of Forage Source on the Carbon Footprint of a Canadian Dairy Farm Using Whole-Systems Analysis and the Holos Model: Alfalfa Silage vs. Corn Silage. Climate 5:87. doi: 10.3390/cli5040087
Little, S. M., Lindeman, J., Maclean, K., and Janzen, H. H. (2008). Holos - A Tool to Estimate and Reduce GHGs from Farms. Methodology and Algorithms for Version 1.1.x. Ottawa, ON: Agriculture & Agri-Food Canada, 158.
Mayrinck, R. C., Laroque, C. P., Amichev, B. Y., and Van Rees, K. (2019). Above- and below-ground carbon sequestration in shelterbelt trees in canada: a review. Forests 10:922. doi: 10.3390/f10100922
McGeough, E. J., Little, S. M., Janzen, H. H., McAllister, T. A., McGinn, S. M., and Beauchemin, K. A. (2012). Life cycle assessment of greenhouse gas emissions from dairy production in Eastern Canada: a case study. J. Dairy Sci. 95, 5164–5175. doi: 10.3168/jds.2011-5229
McKenney, D. W., Hutchinson, M. F., Kesteven, J. L., and Venier, L. A. (2001). Canada’s plant hardiness zones revisited using modern climate interpolation techniques. Can. J. Plant Sci. 81, 129–143.
Mize, C. W., Brandle, J. R., Schoeneberger, M. M., and Bentrup, G. (2008). “Ecological Development and Function of Shelterbelts in Temperate North America,” in Toward Agroforestry Design-An Ecological Approach, eds S. Jose and A. M. Gordon (New York, NY: Springer), 27–54. doi: 10.1007/978-1-4020-6572-9_3
Negron, J. F., Witcosky, J. J., Cain, R. J., LaBonte, J. R., Duerr, D. A., et al. (2005). The banded elm bark beetle: a new threat to elms in North American. Am. Entomol. 51, 84–94. doi: 10.1093/ae/51.2.84
Osorio, R. J., Barden, C. J., and Ciampitti, I. A. (2019). GIS approach to estimate windbreak crop yield effects in Kansas-Nebraska. Agroforest Syst. 93, 1567–1576. doi: 10.1007/s10457-018-0270-2
Petkova, M. (2012). Bulgarian Research Program for Reducing Methane Production from Ruminants Through Innovation in Their Nutrition. Bulgaria: Institute of Animal Science Kostinbrod 2232.
Poppy, L. (2003). Shelter Your Livestock With Trees. Indian Head, SK: Agriculture and Agri-Food Canada (PFRA).
Prairie Farm Rehabilitation Administration [PFRA] (1980). Development evaluation study: PFRA Tree Nursery Program1902–1972. Regina, SK: Government of Canada.
Rempel, J. C. (2013). Costs, Benefits, and Barriers to the Adoption and Retention of Shelterbelts in Prairie Agriculture as Identified by Saskatchewan producers. Master Thesis, University of Saskatchewan, Saskatchewan.
Rempel, J. C., Kulshreshtha, S. N., Amichev, B. Y., and Van Rees, K. C. J. (2017). Costs and benefits of shelterbelts: a review of producers’ perceptions and mind map analyses for Saskatchewan. Canada. Can. J. Soil Sci. 97, 341–352.
Rural Development Institute Shelterbelt Survey (2014). Prairie Producers’ Use of and Attitudes Towards Shelterbelts. Manitoba: Brandon University.
Samsonstuen, S., Åby, B. A., Crosson, P., Beauchemin, K. A., Bonesmo, H., and Aass, L. (2019). Farm scale modelling of greenhouse gas emissions from semi-intensive suckler cow beef production. Agricult. Syst. 176:102670. doi: 10.1016/j.agsy.2019.102670
Schoeneberger, M., Bentrup, G., de Gooijer, H., Soolanayakanahally, R., Sauer, T., Brandle, J., et al. (2012). Branching out: agroforestry as a climate change mitigation and adaptation tool for agriculture. J. Soil Water Conserv. 67:128A0136A.
Schroeder, B., Walker, D., Inouye, G., Poppy, L., and Lutz, J. (2011). “Innovative agroforestry designs: ecobuffers,” in Agroforestry: A Profitable Land Use. Proceedings, 12th North American Agroforestry Conference, eds S. F. Ashton, S. W. Workman, W. G. Hubbard, and D. J. Moorhead (Athens, GA: University of Georgia), 46.
Silim, S. (2004). Ensuring High Productivity of Alberta’s Poplar Plantation Under a Changing Climate. A Progress Report Prepared for the Forest Management Branch Alberta Lands and Forests Division. Indian Head, SK: Agriculture and Agri-Food Canada, 14.
Skjelvåg, A. O., Arnoldussen, A. H., Klakegg, O., and Tveito, O. E. (2012). Farm specific natural resource base data for estimating greenhouse gas emissions. Acta Agricult. Scand. Sec. A 62, 310–317. doi: 10.1080/09064702.2013.777092
Soolanayakanahally, R. (2010). Latitudinal gradients in adaptive traits of Populus. PhD Dissertation, University of British Columbia, Vancouver.
Stange, C., and Jackson, M. (2015). Windbreak Renovation Video. Fargo: North Dakota State University-Fargo Extension.
StatsCan (2019). Statistics Canada. 2016 Census of Agriculture. Available online at: https://www150.statcan.gc.ca/n1/daily-quotidien/170510/dq170510a-eng.htm (accessed August 15, 2019).
Stevenson, L. (2018). Renovated windbreak for farmer education. Manitoba Co-operator. Available online at: https://www.manitobacooperator.ca/news-opinion/news/local/renovate-shelterbelts-dont-remove-to-make-them-more-effective/ (accessed August 7, 2019).
Toensmeier, E. (2016). The carbon farming solution. White River Junction. Vermont: Chelsea Green Publishing.
Udawatta, R. P., and Jose, S. (2012). Agroforestry strategies to sequester carbon in temperate North America. Agroforestry Syst. 86, 225–242. doi: 10.1007/s10457-012-9561-1
United Nations [UN] (2019). Department of Economic and Social Affairs, Population Division (2019). World Population Prospects 2019: Highlights (ST/ESA/SER.A/423). New York, NY: United Nations.
United Nations Framework Convention on Climate Change [UNFCCC] (2019). National Determined Contributions. NDC. Canada’s 2017nationally determined contribution submission to the united nations framework Convention on Climate Change. Rio de Janeiro: UNFCCC.
Waldron, R. M., and Hildahl, V. (1974). Deterioration of Shelterbelts in Southwestern Saskatchewan. Edmonton: Canadian Forestry Service, Northern Forest Research Centre.
Ward, T. (2016). Agroforestry: A land management option for building resilient Canadian agricultural systems in a changing climate. Unpublished Master’s Thesis, University of Missouri, Columbia Missouri.
Ward, T., and de Gooijer, H. (2017). “Expanding the North American Perspective – Canada,” in Agroforestry: Enhancing Resiliency in U.S. Agricultural Landscapes Under Changing Conditions, eds M. M. Schoeneberger, G. Bentrup, and T. Patel-Weynand (Washington, DC: USDA Forest Service Gen. Tech. Report WO-96), 101–112.
Keywords: shelterbelts, agroforestry, Holos model, carbon sequestration, allometric modeling of carbon
Citation: Kröbel R, Moore J, Ni YZ, McPherson A, Poppy L, Soolanayakanahally RY, Amichev BY, Ward T, Laroque CP, Van Rees KCJ and Akhter F (2020) Demonstration and Testing of the Improved Shelterbelt Component in the Holos Model. Front. Environ. Sci. 8:149. doi: 10.3389/fenvs.2020.00149
Received: 31 October 2019; Accepted: 04 August 2020;
Published: 08 September 2020.
Edited by:
Thomas Barfoed Randrup, Swedish University of Agricultural Sciences, SwedenReviewed by:
Michael W. Strohbach, Technische Universität Braunschweig, GermanyEdward W. Bork, University of Alberta, Canada
Copyright © 2020 Kröbel, Moore, Ni, McPherson, Poppy, Soolanayakanahally, Amichev, Ward, Laroque, Van Rees and Akhter. This is an open-access article distributed under the terms of the Creative Commons Attribution License (CC BY). The use, distribution or reproduction in other forums is permitted, provided the original author(s) and the copyright owner(s) are credited and that the original publication in this journal is cited, in accordance with accepted academic practice. No use, distribution or reproduction is permitted which does not comply with these terms.
*Correspondence: Roland Kröbel, cm9sYW5kLmtyb2ViZWxAY2FuYWRhLmNh; Fardausi Akhter, ZmFyZGF1c2kuYWtodGVyQGNhbmFkYS5jYQ==