- 1School of Geography and Planning, Sun Yat-sen University, Guangzhou, China
- 2Key Laboratory of the Sustainable Development of Xinjiang’s Historical and Cultural Tourism, Xinjiang University, Urumqi, China
- 3School of Architecture and Urban Planning, Yunnan University, Kunming, China
COVID-19 is a highly infectious disease and public health hazard that has been wreaking havoc around the world; thus, assessing and simulating the risk of the current pandemic is crucial to its management and prevention. The severe situation of COVID-19 around the world cannot be ignored, and there are signs of a second outbreak; therefore, the accurate assessment and prediction of COVID-19 risks, as well as the prevention and control of COVID-19, will remain the top priority of major public health agencies for the foreseeable future. In this study, the risk of the epidemic in Guangzhou was first assessed through logistic regression (LR) on the basis of Tencent-migration data and urban point of interest (POI) data, and then the regional distribution of high- and low-risk epidemic outbreaks in Guangzhou in February 2021 was predicted. The main factors affecting the distribution of the epidemic were also analyzed by using geographical detectors. The results show that the number of cases mainly exhibited a declining and then increasing trend in 2020, and the high-risk areas were concentrated in areas with resident populations and floating populations. In addition, in February 2021, the “Spring Festival travel rush” in China was predicted to be the peak period of population movement. The epidemic risk value was also predicted to reach its highest level at external transportation stations, such as Baiyun Airport and Guangzhou South Railway Station. The accuracy verification showed that the prediction accuracy exceeded 99%. Finally, the interaction between the resident population and floating population could explain the risk of COVID-19 to the highest degree, which indicates that the effective control of population agglomeration and interaction is conducive to the prevention and control of COVID-19. This study identifies and predicts high-risk areas of the epidemic, which has important practical value for urban public health prevention and control and containment of the second outbreak of COVID-19.
Introduction
As of October 15, 2020, there were 38,599,508 confirmed cases of COVID-19 and 1,093,548 deaths worldwide (Fan et al., 2021). The World Health Organization has classified the outbreak as a “global pandemic”. The rapid and extensive spread of COVID-19 requires the consideration of as many factors as possible, and quickly responding to this major public health event poses a great challenge to the scientific community. Therefore, at the intersection of medicine, virology, geography, public administration and other disciplines, there is an urgent need to formulate accurate epidemic prevention policies (Yu et al., 2020).
Although China’s COVID-19 epidemic has been effectively controlled with the joint efforts of the Chinese government and the Chinese people (Zhang et al., 2020a), the number of COVID-19 patients continues to show an upward trend. As the weather becomes cooler and virus activity increases, there are already signs of a second outbreak of COVID-19 (Gosavi and Marley, 2020). Therefore, assessing the risk of COVID-19 and simulating the areas at high risk of future COVID-19 outbreaks can contribute to early prevention and effective containment of a second outbreak of COVID-19 in advance (Thomas et al., 2020).
Since the outbreak of COVID-19, scholars have conducted numerous studies from the perspectives of pathological diagnosis (Xie and Zhu, 2020), drugs and vaccines (Liu et al., 2020), transmission relationships (Heidari et al., 2020), spatiotemporal models (Babac and Mornar, 2020), epidemic prediction (Wang et al., 2020a), transmission simulation (Werth et al., 2021), risk assessment (Jia et al., 2020), and epidemic impact (Du et al., 2020), and all of these studies have played a positive role in the prevention and treatment of COVID-19. In terms of epidemic risk assessment, Jia proposed a risk model of population mobility and conducted risk assessment of an epidemic by analyzing population mobility data (Jia et al., 2020). Du coupled a population mobility accumulation model and an exponential growth model (Xu et al., 2020a) to construct an epidemic model and assessed the epidemic risk using Tencent positioning data. Moreover, Pan divided the infection risk of COVID-19 in various states in the United States on the basis of mobile phone positioning data (Hâncean et al., 2020). Other scholars have evaluated the risk of COVID-19 in different countries and regions based on natural and social environmental factors (Chatterjee et al., 2020), and these evaluations based on the vulnerability of the region itself could also play a positive role in assessing the risk of the COVID-19 epidemic (Xu et al., 2020a). The abovementioned studies are mainly based on population mobility and assess the risk of the COVID-19 epidemic; however, the risk distribution of COVID-19 is determined by multiple urban spatial factors (Ribeiro et al., 2020).
In terms of epidemic prediction, statistical and dynamic models are often used to estimate future cases and infection trends. Statistical models include methods such as linear regression analysis (Chatterjee et al., 2020; Piovella, 2020; Cartenì et al., 2020), time series analysis and statistical process control (Feroze, 2020; Zhang et al., 2020b). Statistical models are generally applied to detect and provide an early warning of COVID-19 outbreaks. Since infectious disease theory is not involved here, only short-term predictive analysis can be performed (Polo et al., 2020). Dynamic models can be divided into several basic types, such as susceptible-infected (SI), susceptible-infected-susceptible (SIS), susceptible-infected-recovered (SIR), and susceptible-exposed-infected-recovered (SEIR) models, based on the characteristics of pathogens, infectious agents, post infection immunity, the source of infection, the route of transmission, and susceptible populations (Yawney and Gadsden, 2020). Moreover, as dynamic models take into account the factors influencing disease transmission and related social factors, they can effectively reveal the trends of the epidemic and change course of the disease (Li et al., 2020). However, these basic dynamic models hardly consider the significant differences among geographical units and dynamic changes in populations, which makes it difficult for these models to support refined risk assessment and simulation by epidemic prevention departments at all levels from single-scale to multiscale coordination (Liu and Mesch, 2020).
In-depth studies have been carried out in different countries and regions on the global spread, modeling and understanding of COVID-19, among which studies from Italy and Romania have demonstrated the necessity to develop new routes between EU countries to contain the spread of the epidemic in the early stages of the outbreak (Hâncean et al., 2020). Studies from Brazil have shown that there are differences in morbidity and mortality between large and small cities and that different age compositions and distributions of health infrastructure all have important effects on COVID-19 (Ribeiro et al., 2020). In Kenya, studies have taken the perspective of household energy and food security during the COVID-19 period, and a sustainable development model during the COVID-19 period has been obtained (Shupler et al., 2021). Norway, on the other hand, has determined national containment strategies depending on the characteristics of a given city during similar crises by analyzing its urban working environment and migration patterns (Venter et al., 2020). India, currently the country with the highest risk of COVID-19, has analyzed the impact of a national lockdown on the urban air quality during COVID-19 (Navinya et al., 2020). Some scholars in the United States have established an early warning and evaluation model based on the responsibility system by using city-related indicators of COVID-19 and performed experimental verification of the epidemic in 17 major cities in the country (Li et al., 2021). Based on the existing models and understanding of the spread of the pandemic in different countries and regions, it can be concluded that developed countries and regions such as the United States and Europe are more concerned about the impact of COVID-19 on the existing urban living environment (Kan et al., 2021), while developing countries and regions such as Southeast Asia and Africa are more concerned about the impact of the pandemic on urban public health resources (Zvobgo and Do, 2020), which illustrates the differences in the level of development among these different countries and regions. Therefore, as China is the largest developing country in the world, studies on the transmission, modeling, and understanding of COVID-19 in China should explore the urban environmental factors that influence the distribution and transmission of COVID-19, taking into account urban public health resources. Such research is likely to be of great regional value (Hou et al., 2021).
In the studies on the early outbreak of COVID-19 and the cross-regional transmission of it, location characteristics of geographical space occupy a large proportion, mainly because there are huge differences in the spatial variability and aggregation degree of COVID-19 infection rate and mortality rate in different countries (Khavarian-Garmsir et al., 2021). However, although some studies have analyzed the heterogeneity of the geospatial distribution of patients with COVID-19, few studies have considered the spatio-temporal variation of confirmed patients with COVID-19 in geospatial space (DuPre et al., 2021). It has been found in previous studies on spatial epidemiology that urban geospatial factors have a strong spatiotemporal effect on the transmission of viruses, including the analysis of the possibility of infectious epidemics from the perspective of the degree of population aggregation in geographic space (Hasselwander et al., 2021). Therefore, the study on the risk distribution of COVID-19 in urban space should carefully consider its spatial and temporal characteristics (Mansour et al., 2021), and analyze the geospatial relationship between communities with different levels of infection and population agglomeration (Hassan et al., 2021), so as to reveal the spatiotemporal changes of COVID-19 in geographical space (Kwok et al., 2021). Spatiotemporal geographic epidemiological data, including cellular signaling data (Xiao et al., 2019), (Zhan et al., 2021), population flow data (He et al., 2020; Zhang and Yuan, 2021), and urban point of interest (POI) data, etc. (He et al., 2021; Mahajan et al., 2021). In a word, these spatiotemporal geographic data can represent the characteristics of epidemic risk in urban space, providing a new research perspective and solution to problems related to epidemic risks in relation to urban geography (Bachir et al., 2019; Sharifi and Khavarian-Garmsir, 2020). Compared with statistical survey data about the epidemic, spatiotemporal geographic epidemiological data have spatiotemporal continuity, and their strong data volume, analysis and processing mode, display capability and other advantages greatly compensate for the insufficient amount of statistical survey data in research on epidemic analysis (Silva et al., 2018 ; Alsunaidi et al., 2021). Therefore, spatiotemporal geographic epidemiological data can play an important auxiliary role in assessing and simulating COVID-19 risk (Hu et al., 2021).
In recent years, with the development of computer technology, machine learning and deep learning have gradually been applied to relevant research on cities and have achieved good results (Milojevic-Dupont and Creutzig, 2021 ; Wang et al., 2020b). The goal of machine learning is to obtain patterns from existing data samples and to then analyze and predict based on the patterns obtained. Logistic regression (LR) models are among the classic models of machine learning (Cao et al., 2020), and they have advantages related to the objective methods and rigorous calculations involved. Compared with linear regression (Yuchi et al., 2019; Sharifi and Khavarian-Garmsir, 2020), gradient neural network-convergence analysis (GNN-CA) (Aarthi and Gnanappazham, 2018), cellular automata and other simulation algorithms (Zhou et al., 2020), LR is simpler and more efficient in terms of the variables and normality assumptions, and it provides a new solution path for studies on urban decision-making and simulation (Siddiqui et al., 2018).
Accurately assessing and predicting the distribution of high and low risks of COVID-19 is crucial for epidemic prevention and the control of a second outbreak of the epidemic in Guangzhou, which is one of the cities with the largest permanent population and floating population in China (Granella et al., 2021). Taking Guangzhou as an example, this study assesses and simulates the COVID-19 risk from the perspective of geography using machine learning and spatiotemporal geographic epidemiological data. The mechanism and impact of various spatial factors on COVID-19 are discussed, and the assessment and simulation results are verified (Yorio and Moore, 2018). Compared with the existing studies on the epidemic risk, this study has the advantage of smaller scale by evaluating and simulating the high and low risk distribution of the epidemic in Guangzhou through machine learning, which enables the epidemic risk distribution fed back to geographical units more refined, and the epidemic risk analysis based on urban geospatial factors can be greatly conducive to epidemic prevention and control in urban space. At the same time, using geographic detectors to analyze the primary and secondary factors affecting the distribution of epidemic risk level in urban space has important practical significance for the formulation of epidemic prevention and control policies and urban public security.
Materials and Methods
Study Area
The research area is Guangzhou, Guangdong Province, China (Figure 1). As one of the most urbanized and modernized cities in China, Guangzhou has 11 districts with a total area of 7,434.4 square kilometers. According to the Statistical Bulletin of The National Economic and Social Development of Guangzhou 2019 released by the Bureau of Statistics of Guangzhou Municipality on March 6, 2020, the permanent resident population of Guangzhou reached 15.3059 million in 2019. Guangzhou is one of the cities with the largest permanent population and floating population in China; thus, the assessment of the risk of COVID-19 conducted in this study can not only help to understand the areas in Guangzhou at high risk of a COVID-19 epidemic but also provide a decision-making basis for COVID-19 prevention and control nationwide.
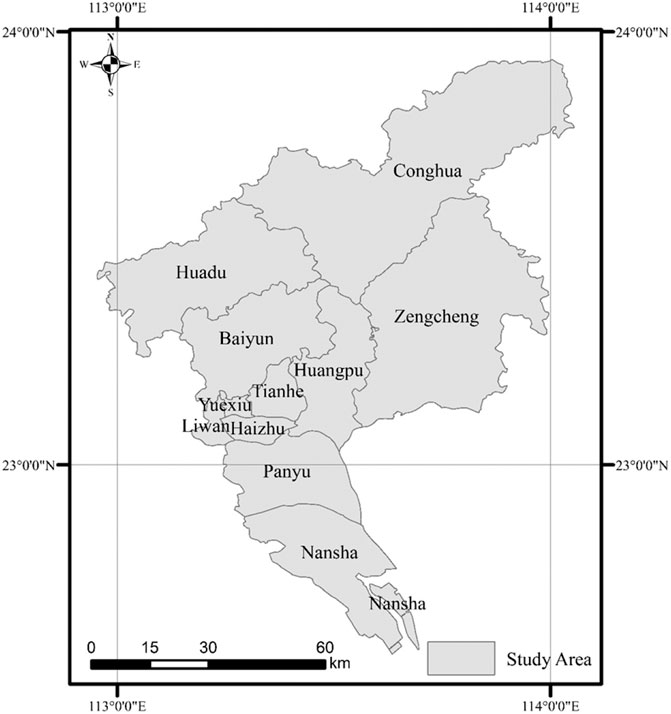
FIGURE 1. Study area (the study area is Guangzhou City, Guangdong Province, China, which is located on the southern coast of China and is one of the cities with the highest level of urbanization and modernization in China).
Study Data
Data Introduction
Spatiotemporal geographic epidemiological data about the epidemic should be directly or indirectly used to monitor and analyze this disease. According to the “triangle” theoretical model of public security (Wang et al., 2020c), public security consists of four parts: the emergency, the disaster carrier, emergency management and disaster elements. The emergency is the disaster itself, the disaster carrier refers to the people and things affected by the emergency when the emergency occurs, and the disaster factor is the factor inducing the occurrence of the emergency. Based on the analysis of the emergency, the disaster carrier and disaster factors, the whole process of an emergency, from occurrence and development to disaster formation and emergency measures, can be controlled.
In this study, the emergency is COVID-19, and the data on COVID-19 come from the National Health Commission of the People’s Republic of China. The data mainly include the number of people infected with COVID-19 in Guangzhou in 2020 and the geographic location of the disease as announced by the committee. The disaster carrier is the entire population of Guangzhou affected by COVID-19, including the floating population and permanent population. Here, the data on the floating population are derived from Guangzhou population heat map data in January, February, and August 2020, combined with the average monthly data from January to August obtained from Tencent-migration data, while the permanent resident data are obtained from the 2019 Statistical Yearbook of Guangdong Province. Disaster factors mainly refer to factors that induce and spread COVID-19, including the main public places where people communicate and gather in cities, such as hospitals, fever clinics, life markets, supermarkets, hotels, restaurants, schools, administrative centers, cultural exchange places, etc. (Stevens et al., 2021), These places play an important role in the flow of urban elements, so they have also become the main places for COVID-19 transmission within cities (Rousseau and Deschacht, 2020). After the outbreak of the COVID-19, Chinese government implements a strict isolation policy by closing schools, administrative units, public services and other places, which restricts the communication and interaction of people in these public places (Liu et al., 2021). In addition, the development of a series of online remote interaction modes such as online teaching and online office has further reduces the level of epidemic risk in these areas (Wu et al., 2021a). Therefore, combining the existing literature and China’s current epidemic prevention policy (Chen et al., 2021), the distance from fever clinic, the distance from living market, the distribution density of supermarket, the density of isolated hotel, the distribution density of catering, and the location distance from traffic station are selected by this study as the disaster factors, which were all screened through and obtained from Guangzhou POIs in 2020.
Based on the “triangle” theoretical model of public security, the following spatiotemporal geographic epidemiological data related to COVID-19 are determined in this study: the fever clinic distance, population flow, supermarket distance, COVID-19 distribution, population density, shopping mall density, restaurant density, public transit station density, and hotel density. Since this study analyzes the risk distribution level of the epidemic based on urban geographic space, the spatial resolution of the data in this study is the study scale unit (the spatial resolution of the data used in this study is unified as 25 × 25 m). The high-precision research unit scale also makes the simulated epidemic risk distribution more refined.
Data Preprocessing
1) After cleaning and duplicate checking of the POI data of Guangzhou obtained from the AMap application programming interface (API), it is found that the total numbers of supermarkets, hotels, shopping malls, public transit stations and restaurants in Guangzhou in 2020 are 27,738, 16,134, 24,686, 57,882 and 15,009, respectively. There are 102 fever clinics announced by the government. The Euclidean distances to fever clinics (Wu et al., 2021b), public transit stations, and shopping malls and the densities of supermarkets, hotels and restaurants are calculated, and the results are shown in Figure 2.
2) Population data preprocessing: The population data are divided into resident population data and floating population data. The floating population data comprise Tencent-migration data as population flow change data. Tencent-migration data can be obtained from Tencent’s positioning big data service window (http://heat.qq.com/index.php). Based on the analysis of the user location information of the user positioning by Tencent’s multiple app programs, Tencent-migration data with a spatial resolution of 25 m × 25 m are obtained. The average monthly Tencent-migration data for January, February and August 2020 are obtained from the Tencent API (Figure 3B–D). The permanent population data come from the 2019 Guangzhou Statistical Yearbook. In 2019, the permanent population of Guangzhou was 15.3059 million, which is consistent with the spatial resolution of the floating population data obtained through resampling (Figure 3A).
3) COVID-19 data: The COVID-19 data come from the National Health Commission of the People’s Republic of China (http://www.nhc.gov.cn/). As of the end of February 2020, there were no significant cumulative new COVID-19 infections in Guangzhou. The cumulative number of COVID-19 infections in January and February 2020 was 137 and 209 cases, respectively, and the spatial resolution was found to be consistent with the floating population data through calibration sampling of their incidence locations; Figure 4 illustrates the results.
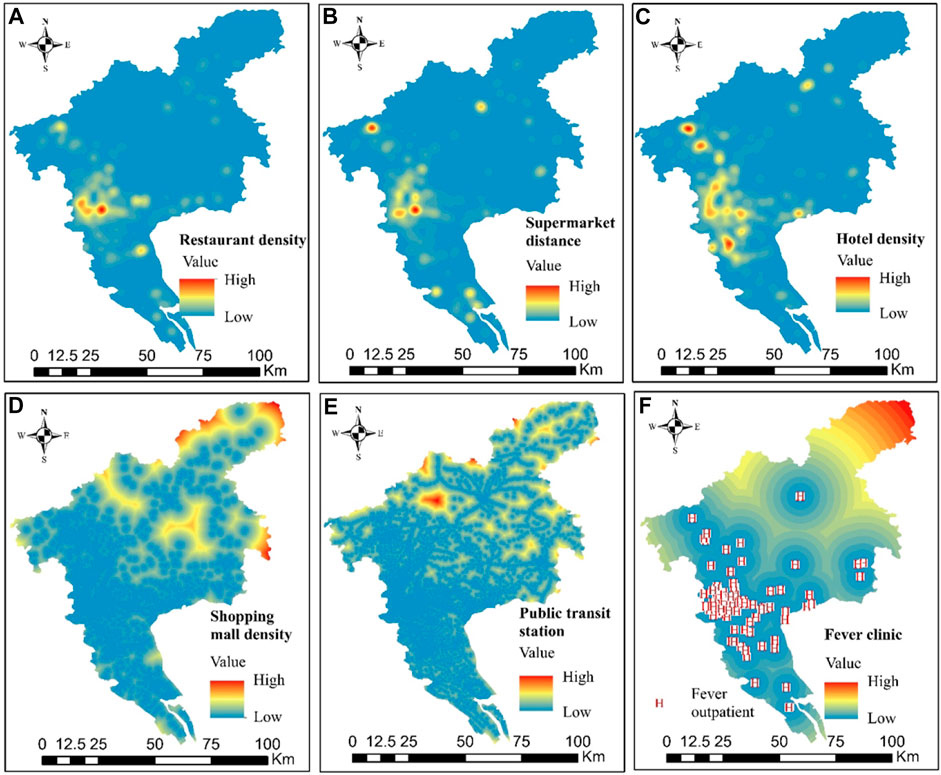
FIGURE 2. POI data preprocessing results [(A–F) are Catering density, Market distance, Hotel density, Quotient hyperdensity, Traffic density, and Fever outpatient distance of the spatiotemporal geographic epidemiological data of Guangzhou; the color in the figure ranges from blue to red, indicating density and distance value ranges from low to high].
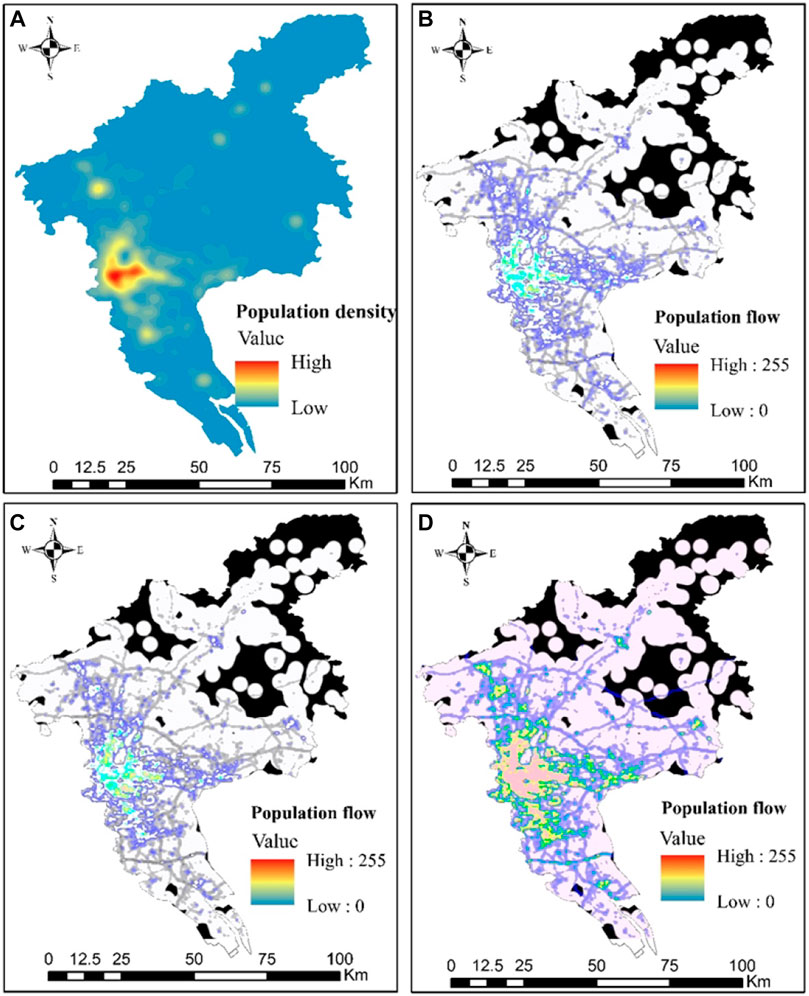
FIGURE 3. Population data preprocessing results [(A) Population density represents the permanent population density of Guangzhou in 2019; (B–D) represent the monthly average data of Tencent population migration in January, February, and August of 2020 in Guangzhou, respectively, and the color in the figure ranges from blue to red, indicating permanent population density and population migration index value ranges from low to high].
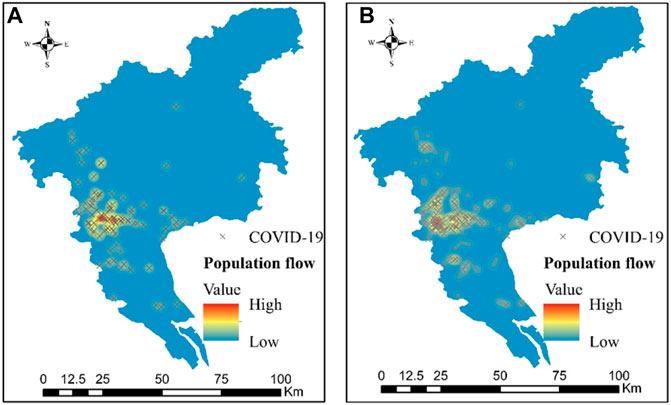
FIGURE 4. COVID-19 data preprocessing results [(A, B) Respectively represent the cumulative number and density distribution of COVID-19 infection in Guangzhou in January 2020, in which COVID-19 represents the incidence location, and the color in the figure ranges from blue to red, indicating the spatial density distribution of COVID-19 patients varies from high to low].
Methods
Logistic Regression
As one of the classic methods of machine learning (Lai et al., 2021), LR can build a linear regression based on the sigmoid function, and with the help of an LR model, it is possible to further explore the relation between independent and dependent variables and to quantitatively analyze the probability of disaster events. Compared with models such as support vector machines (SVMs) and neural networks, LR models have great advantages in training and recognition time, with probability results ranging from 0 to 1, which are easier to interpret (Cheng and Masser, 2003). An LR model is meaningful only when the independent variable is significant. Therefore, the relationship between the occurrence probability of COVID-19 and explanatory factors can be expressed as follows:
where P represents the occurrence probability of COVID-19 on a spatiotemporal geographic scale, which is in the range of [0,1]. The closer the value of P is to 1, the higher the probability of COVID-19 occurring in the area; the closer the value of P is to 0, the lower the probability of COVID-19 occurring in the area. Z stands for a linear combination. Therefore, the fitting equation involved in LR is as follows:
where C stands for the intercept of the model and represents the error value of the occurrence probability of COVID-19 in urban space under the selected indicator factors; B_1, B_2, … B_n stand for the LR coefficient X_1, X_2, … X_n for the index factor.
The technical route of LR model evaluation is shown in Figure 5.
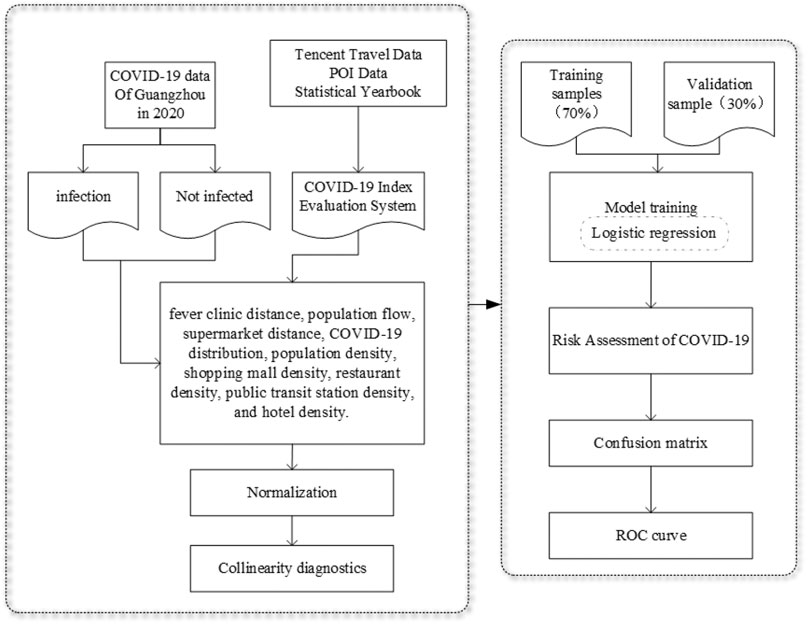
FIGURE 5. Technical route of the LR model (modeling process and verification flow chart of LR model).
Geographic Detectors
According to the first law of geography, everything is interrelated, and the degree of correlation changes with the change in distance (Luo et al., 2019). In geographic space, it can be assumed that if an independent variable has a significant influence on the dependent variable, then the spatial distributions of the independent variable and the dependent variable should be similar in geographic space. A geographic detector is a statistical method based on the spatial variance analysis theory proposed by Wang Jinfeng et al (Fan et al., 2020). The detector can be used to detect the degree of spatial differentiation of different impact factors in geographic space and to verify the coupling of the spatial distribution of two variables as well as the possible causal relationship between the variables (Li et al., 2017).
1) Factor detector
The spatial differentiation degree of COVID-19 detection and the extent to which risk factors explain the spatial differentiation of COVID-19 can be represented by q, and the expression of the factor detector can be expressed as follows:
where
A simple change in the q value satisfies the noncentral F distribution:
where ƛ stands for the noncentral parameter and \overline{Y} stands for the mean value of layer h. Eq. 5 can be used to determine whether the q value is significant.
2) Interaction detector
To identify the interactions between different risk factors,
3) Risk detector
Whether there is a significant difference between the mean value of the attributes of the two subintervals is detected, and the t\ statistic is used for testing:
where
It is assumed that if
4) Ecological detector
Whether the two impact factors
where
Results
Logistic Regression Model Training
On the basis of COVID-19 data from January and February 2020 and floating population data from January, February and August 2020, COVID-19 infection areas were divided, and positive and negative sample construction data sets were built. Since the nine spatial factors used in this study may show multicollinearity, which will cause a serious deviation in the operation results of the LR model, collinearity diagnosis of different factors should be carried out first (Saedi et al., 2020). The product of tolerance (TOL) and the variance inflation factor (VIF) is equal to 1, which is also a common indicator that reflects the degree of collinearity of factors. In general, when the VIF is greater than or equal to 10 or the value of TOL is less than or equal to 0.1, there is a high degree of collinearity among factors, which does not satisfy the modeling conditions (Zhang et al., 2020c). In this study, multicollinearity analysis of nine factors was carried out based on Python, and the results are shown in Figure 6. The VIF and TOL of all factors are 1, which meets the modeling conditions. Therefore, the nine spatial factors should be imported for model training.
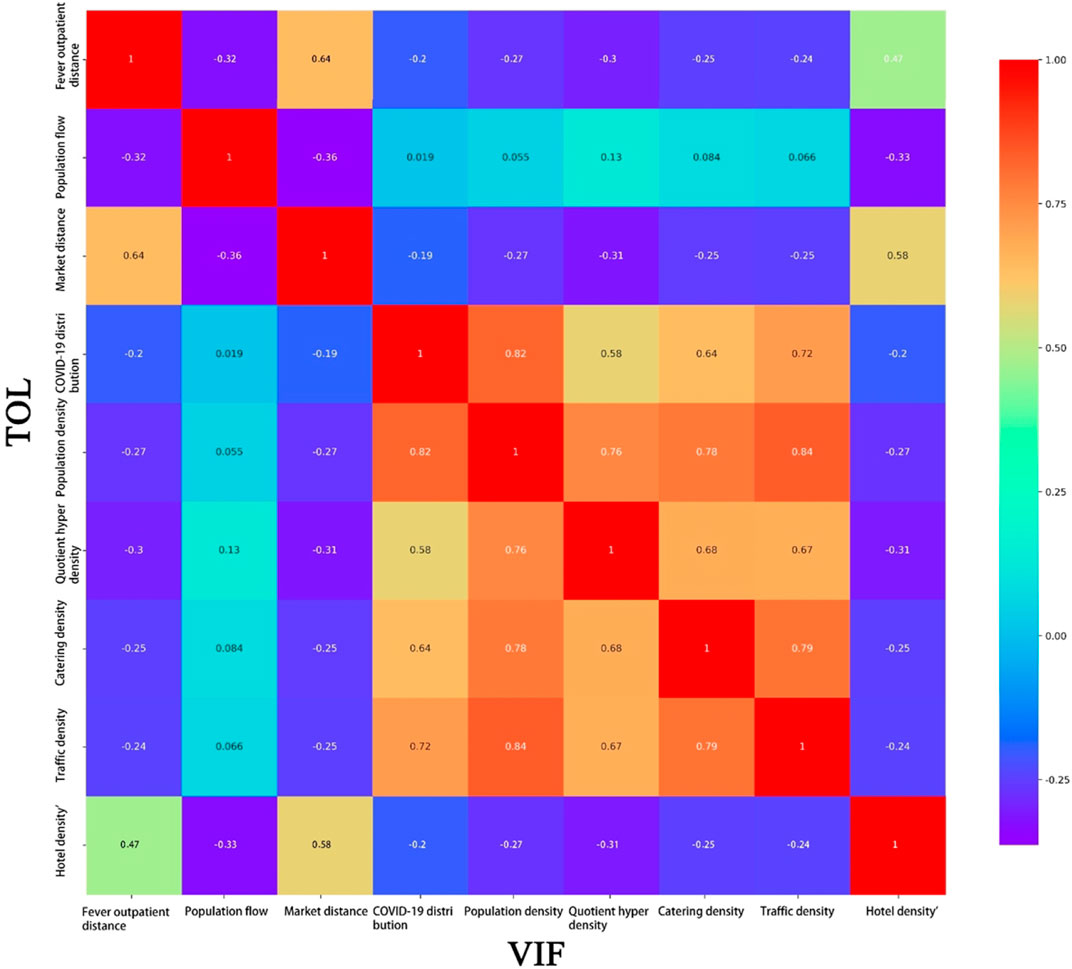
FIGURE 6. Heat map of the collinearity diagnosis of influencing factors (Collinearity diagnosis of different factors is shown in the figure: the TOL of different factors is on the left, the VIF of different factors is on the bottom; the value in the figure is the product value of TOL and VIF between different factors; the color in the figure ranges from blue to red, indicating the corresponding value of TOL and VIF ranges from 0 to 1).
Assessment and Prediction of COVID-19 Risk
Assessment of COVID-19 Risk
Based on the model training results, the higher the risk level is, the higher the probability of COVID-19 occurrence. Incorporating actual geographical locations, a distribution map of the risk level of COVID-19 in Guangzhou in January (Figure 7A), February (Figure 7B), and August (Figure 7C) 2020 is obtained.
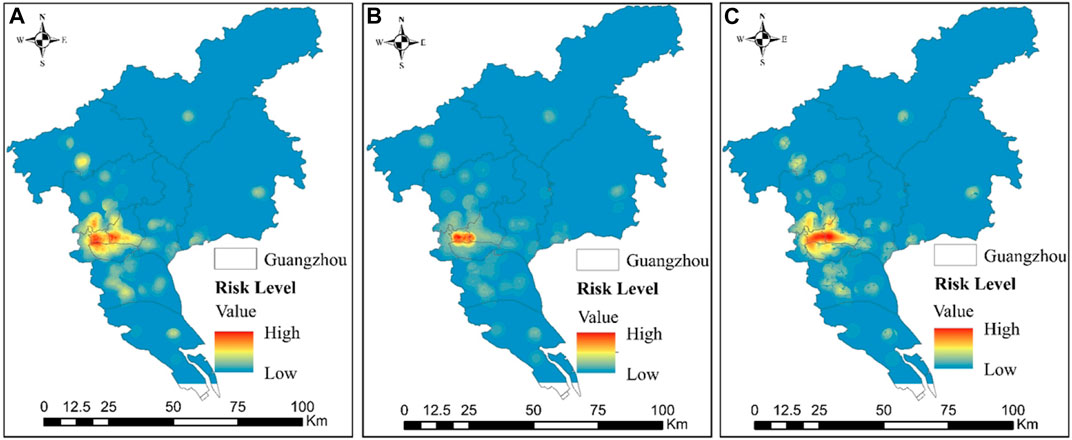
FIGURE 7. Distribution map of the risk level of COVID-19 in (A) January, (B) February, and (C) August 2020 [(A–C) are the high- and low-risk distributions of COVID-19 in Guangzhou in January, February and August of 2020, respectively. The color in the figure ranges from blue to red, indicating that the risk level of COVID-19 ranges from low to high. In addition, the areas with high risk in January, February and August of 2020 are more concentrated].
The distribution map (Figure 7A) shows that the areas at high risk of a COVID-19 epidemic in January 2020 were mainly concentrated in the Yuexiu, Haizhu, Tianhe and Liwan Districts. Comparing Figure 2 and Figure 4 reveals that in January, there was a large number of new COVID-19 patients in these regions. Guangzhou is a city with a high concentration of the floating population and permanent resident population, and Guangzhou is also an area with a relatively high distribution density of other spatial factors, such as hotels, shopping malls, and supermarkets. All of these factors increase the risk of COVID-19 outbreaks in these four regions.
The areas at high risk of a COVID-19 epidemic in February 2020 were mainly concentrated in Yuexiu District and Tianhe District. Figure 2 shows that although the Yuexiu and Tianhe Districts are relatively densely populated with permanent residents, the “home quarantine” policy not only greatly restricted the mobility and interaction of people but also reduced the transmission routes and pathways of COVID-19. The “home quarantine” policy effectively curbed the spread of the virus, bringing the cumulative number of new COVID-19 infections under control.
The areas at high risk of a COVID-19 epidemic in August 2020 were mainly concentrated in the Yuexiu, Haizhu, Liwan, Baiyun and Panyu Districts as well as external transportation hubs, including Baiyun Airport and high-speed railway stations. The COVID-19 epidemic was effectively controlled after February, and population activities and urban interactions began to return to normal starting in May 2020. However, with the large-scale mobility and interaction of the population, the risk areas of the epidemic changed from the previous low-risk areas to high-risk areas.
Comparing the high-risk distribution map of the COVID-19 epidemic in January, February and August 2020 reveals that in general, the level of risk experienced a rapid decline and then a slow rise, with the risk reaching its lowest point in February. In addition, in terms of the cumulative number of new patients, there were basically no new local patients after February, which suggests that the “home quarantine” policy was a positive and effective means of epidemic prevention. Additionally, comparing the distribution of regions with a high risk of an epidemic in the 3 months above shows that in February, the areas with a high risk of an epidemic were mainly concentrated in the areas with a dense permanent population, while in January and August, these areas were mainly concentrated in areas with a dense floating population and a dense permanent population, demonstrating that controlling the flow and interaction of the population is the best means of epidemic prevention.
Prediction of COVID-19 Risk
The COVID-19 risk levels in January, February and August 2020 were reintroduced into the model to simulate and predict the COVID-19 risk distribution in February 2021. As shown in Figure 8, the distribution of COVID-19 risk in February 2021 is roughly similar to that in August 2020; that is, the high-risk areas are mainly concentrated in the Yuexiu, Haizhu, Tianhe, Liwan, Baiyun and Panyu Districts, but the epidemic risk value is higher than that in August 2020. Since these areas have always been areas where the resident population and the floating population are highly concentrated, without corresponding epidemic prevention measures, the mobility and interaction of the population will continuously promote the spread of COVID-19. Therefore, in the event of a second COVID-19 outbreak, these areas will be more likely to spread the virus.
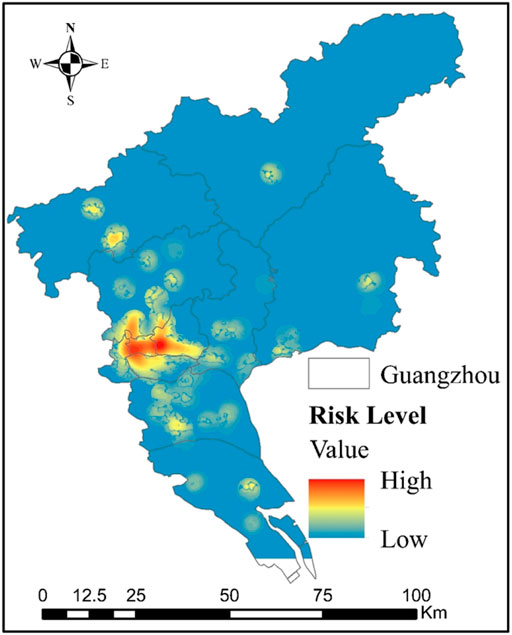
FIGURE 8. Distribution map of the risk level of COVID-19 in February 2021 (the forecast of COVID-19 risk level in February 2021 is shown in the figure, in which the color ranges from black to white, indicating the predicted value of COVID-19 risk level ranging from low to high).
Compared with August 2020, external transportation hubs such as Baiyun Airport and the Guangzhou South Railway Station, which have been important regions for population mobility and interaction, have a significantly higher risk of an outbreak in February 2021. The permanent population of Guangzhou will not increase significantly in February 2021; however, February 14, 2021, is the Chinese Lunar New Year. Thus, the whole month falls within the Spring Festival travel season. During the 2019 Chinese Lunar New Year, the population mobility across all of China exceeded 3,000,000,000 individual trips (Zhang et al., 2020c). Therefore, during the Spring Festival travel season of 2021, the population mobility in Guangzhou is bound to reach a new peak, and a large number of population movements are likely to exacerbate the risk of COVID-19 transmission.
Analyzing the risk distribution of COVID-19 between February 2021 and 2020 intuitively shows that the risk of COVID-19 is most directly related to the population concentration and mobility. Therefore, the risk of COVID-19 transmission can be greatly reduced if the population concentration and mobility can be inhibited to a certain extent.
Preliminary Accuracy Test Model
Verification of the risk level of COVID-19 is an important condition for the generalization of research results. Therefore, in order to test the accuracy of the risk assessment of COVID-19 based on spatio-temporal geoepidemiological data, confusion matrix and ROC curve verification are used in this study to verify the accuracy of the results (Shu et al., 2020). Firstly, the dataset of epidemiological data is classified into training data and validation data through the Sklearn module, in which the training data accounts for 70% and validation data accounts for 30% (Abedini et al., 2017). Then, cross-validation is conducted for training data and verification data of different classifications, and the obtained verification indexes are accuracy, precision and recall. Finally, the verification indexes obtained from the training data and test data of different classifications are returned in the form of array to get the final accuracy verification results.
Verification of the Confusion Matrix
The preliminary accuracy test is a crucial step in verifying the reliability and predictability of the model (Kranji et al., 2019). In this study, a confusion matrix (the average value of verification indexes obtained from different training data and verification data) is used to conduct a preliminary accuracy test of the prediction of COVID-19 in February 2021. Confusion matrix test results are shown in Figure 9. The preliminary accuracies of the risk areas and risk-free areas are 0.9932 and 0.8949, respectively. Both of these values are greater than 0.85, demonstrating that the model has high accuracy in its prediction of epidemic risk, but the accuracy of the risk-free areas is relatively low, which may be due to the smaller number of risk-free areas and samples. The precision and recall are 0.9439 and 0.8995 for the risk areas and 0.9392 and 0.8849 for the risk-free areas, respectively. From the perspective of precision, recall and accuracy, the LR model for COVID-19 prediction has relatively high accuracy.
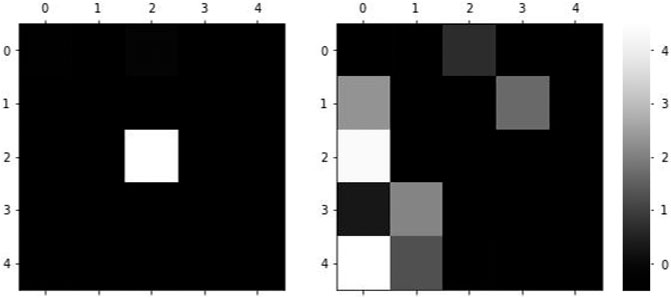
FIGURE 9. Heat map of the verification of the confusion matrix of the logistic regression model (the verification result of the logistic regression confusion matrix is shown in the heat map. The color in the figure ranges from black to white, indicating that the verification result value ranges from 0 to 5. The higher the thermal value is, the more accurate the verification result of the confusion matrix).
Verification of the Receiver Operating Characteristic Curve
The area under the curve (AUC) value was used to comprehensively test and evaluate the predictive accuracy of the LR model for receiver operating characteristic (ROC) curve validation (Chirisa et al., 2020). When the AUC value is greater than 0.5, the closer it is to 1, the higher the predictive accuracy of the model. Figure 10 shows that the after logistic regression, the average AUC values of the cross-checking of training samples, verification samples and all data of different categories are 0.9934, 0.9932 and 0.9933, respectively. All of these values are higher than 0.99 and close to 1, showing that the model has fairly high predictive accuracy and further illustrating the important role of LR models in predicting the COVID-19 risk distribution.
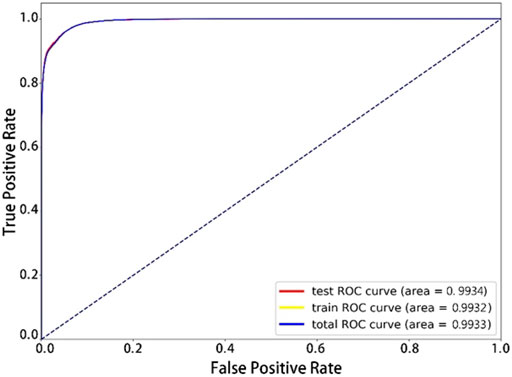
FIGURE 10. ROC curve verification results (the verification results of the ROC curve include the test ROC curve, train ROC curve and total ROC curve, and the three values together determine the accuracy of the results. The closer the area under different curves is to 1, the higher the accuracy will be).
Analysis of Influencing Factors
1) Risk factor detector
The factor test results in Table 2 show that in COVID-19 risk assessment, population mobility is the most important factor determining COVID-19 infections in cities, followed by the density of the resident population. This finding is not only consistent with previous COVID-19 risk assessments and predictions but also demonstrates that the most effective way to prevent COVID-19 is to avoid the mobility and excessive agglomeration of people. On the other hand, the densities of public transit stations, shopping malls, and restaurants and the distance to supermarkets have similar influences. That is, the influences of these factors are all slightly lower than those of population mobility, indicating that to prevent the population from being exposed to the public environment for a long period of time, reducing population mobility and interaction in population agglomeration areas is a reasonable means of epidemic prevention. The factors that have the lowest impact on the risk level of COVID-19 are the distance to fever clinics and hotel density because, on the one hand, even if someone tests positive for COVID-19, he or she can be promptly transferred to a fever clinic for treatment; on the other hand, hotels mainly play a role in isolation. During an epidemic, more people choose home isolation, and there is less time to go to a hotel, which makes the population density of the hotel very low; as a result, hotels have little influence as a spatial factor.
2) Interaction detector
The results of the interaction test of different factors are shown in Table 3. The results showed that the risk level of the epidemic in Guangzhou could be best explained by the interaction between the permanent population density and the floating population. When the Q value is 0.67, the effect of the epidemic risk is interpreted to be greater than that of a single impact factor after the interaction of the two indicators, illustrating that epidemic prevention and control can achieve the maximum effect if the floating population and permanent resident population can be effectively controlled.
The ecological test results (Table 4) are obtained based on the assumption that the test value of F is 0.05, where Y represents a significant difference and N represents no significant difference. In the risk distribution of a COVID-19 epidemic, the results for the densities of the permanent population and floating population are significantly different from the results for other influencing factors, indicating that the densities of the permanent population and floating population are the most important factors affecting the risk of COVID-19. Moreover, the floating population has a greater impact on the risk of COVID-19 than the permanent population. Compared with the population factor, there is no significant difference in other factors, including the distributions of supermarkets, hotels and shopping malls. Therefore, under the premise of reasonably and safely controlling population factors, the distribution of other urban facilities can reasonably provide basic life services for urban residents.
In order to avoid the interaction between influencing factors caused by repeated calculation of the algorithm, this study carries out a second cross-validation of the results obtained by the Interaction Detector and Ecological Detector, which shows that there are no significant differences between final verification result and the first one. In other words, the index calculation results between Interaction Detector and Ecological Detector are reasonableness and interdependency.
Discussion
This study proposes a risk assessment and prediction model of COVID-19 based on spatiotemporal geographic epidemiological data, an LR model and geographic detectors. The risk levels of COVID-19 in January, February and August 2020 are obtained, and the areas at high risk of COVID-19 in February 2021 are predicted. The spatial variability and attribute associations among different influencing factors are also analyzed to identify the main factors influencing the spread of COVID-19.
After the outbreak of COVID-19, the assessment of COVID-19 risk transmission based on the geographical perspective were initially mainly focused on the macro scale, including regional, national and global epidemic assessments (Chakraborty and Maity, 2020). With the popularization of epidemiological data applications for population mobility, the risk assessment of COVID-19 has taken the meso and micro perspectives. That is, studies have started to explore the reasons for the spread of the epidemic from the perspective of the population mobility between communities (Ouyang et al., 2020; Yan et al., 2021). However, such studies continue to place greater emphasis on discussing the impact of population mobility on epidemic risk, and they do not objectively assess and predict the current epidemic risk from the spatiotemporal perspective (Chen et al., 2021). In this study, using multisource spatiotemporal geographic epidemiological data, machine learning-based simulations were conducted, taking into account the resident population, the floating population and all urban spatial factors that may affect the spread of the epidemic in geographical space. Finally, the primary and secondary factors affecting the risk of an epidemic are discussed, and the verification results show that the simulation method is quite accurate.
The areas at high risk of COVID-19 are mainly concentrated in areas with resident populations and floating populations, and this result is basically similar to that of previous studies on COVID-19 (Cokun et al., 2021). Since humans are the main carriers of COVID-19 and other infectious diseases, the mobility and interaction of the population are the most important factors contributing to the high risk of COVID-19 (Nguyen et al., 2020; Xu et al., 2020b). Compared with current studies related to epidemic risk assessment and prediction, this study focuses on the analysis of the impact of urban spatial factors on epidemic risk from the perspective of spatial-temporal geography, allowing the spread of the epidemic to be expressed in terms of geographical location, which is conducive to preventing and controlling the epidemic in the community at the micro scale.
Finally, this study leaves some areas that require further exploration. Guangzhou, China, was selected as the case for analysis in this study (Peirlinck et al., 2020). To better prevent and control the global pandemic, it is necessary to conduct further assessments and simulations of specific epidemics in cities with severe outbreaks around the world.
Conclusion
Risk assessment and prediction of the COVID-19 epidemic and analysis of the main influencing factors hold great practical value for the construction of urban public health safety spaces. In this study, spatiotemporal geographic epidemiological data such as Tencent-migration data and POI data as well as LR and geographical detector models are used to assess the risk of COVID-19 in Guangzhou in January, February and August 2020 and to predict the risk distribution of COVID-19 in February 2021. In addition, the main factors affecting the areas at high risk of COVID-19 are analyzed, and the following conclusions are drawn:
1) The risk of COVID-19 in 2020 mainly exhibited a downward trend and then an upward trend. Although the “home quarantine” policy implemented by the Chinese government has effectively contained the spread of COVID-19 and further reduced the risk of the epidemic for a short time, with the increase in population mobility and interaction degree as well as the recovery of production and the activities of daily life, regional epidemic risk is beginning to show an upward trend.
2) The prediction results of the epidemic situation in February 2021 show that the COVID-19 risk of major external transport hubs in Guangzhou increased significantly due to the arrival of the Spring Festival travel rush, except for areas with dense population movement and interaction. The accuracy of the risk prediction of COVID-19 is greater than 99%, which indicates that the prediction of COVID-19 is highly reliable.
3) The main factors affecting the epidemic risk level are the distribution of the floating population and resident population, and the interaction between the floating population and the resident population also explains the risk distribution of the epidemic to the greatest extent. Therefore, if population agglomeration is limited, then the rational distribution of other urban spatial factors will not have an important impact on the risk of the epidemic.
On the basis of using spatiotemporal geographic epidemiological data, the risk assessment and prediction models for COVID-19 are highly practical and accurate. This study objectively and accurately assesses and predicts areas at high risk of COVID-19, which is conducive to not only preventing and controlling a second outbreak but also providing solutions to urban public security problems for epidemic prevention agencies.
Data Availability Statement
The original contributions presented in the study are included in the article/supplementary files, further inquiries can be directed to the corresponding author.
Author Contributions
XH and CZ: Conceptualization; YW: Methodology; XY: Software; XH and CZ: Validation; XH: Formal analysis; XH: Investigation; CZ: Resources; YW: Data curation; XH: Writing—original draft preparation; XH and CZ: Writing—review and editing.
Conflict of Interest
The authors declare that the research was conducted in the absence of any commercial or financial relationships that could be construed as a potential conflict of interest.
Publisher’s Note
All claims expressed in this article are solely those of the authors and do not necessarily represent those of their affiliated organizations, or those of the publisher, the editors and the reviewers. Any product that may be evaluated in this article, or claim that may be made by its manufacturer, is not guaranteed or endorsed by the publisher.
References
Aarthi, A. D., and Gnanappazham, L. (2018). Urban Growth Prediction Using Neural Network Coupled Agents-Based Cellular Automata Model for Sriperumbudur Taluk, Tamil Nadu, India. Egypt. J. Remote Sensing Space Sci. 21, 353–362. doi:10.1016/j.ejrs.2017.12.004
Abedini, M., Ghasemyan, B., and Mogaddam, M. H. R. (2017). Landslide Susceptibility Mapping in Bijar City, Kurdistan Province, Iran: a Comparative Study by Logistic Regression and AHP Models. Environ. Earth Sci. 308, 76. doi:10.1007/s12665-017-6502-3
Alsunaidi, S. J., Almuhaideb, A. M., Ibrahim, N. M., Shaikh, F. S., Alqudaihi, K. S., Alhaidari, F. A., et al. (2021). Applications of Big Data Analytics to Control COVID-19 Pandemic. Sensors (Basel) 21. doi:10.3390/s21072282
Babac, M. B., and Mornar, V. (2020). Resetting the Initial Conditions for Calculating Epidemic Spread: COVID-19 Outbreak in Italy. IEEE Access 8, 148021–148030. doi:10.1109/access.2020.3015923
Bachir, D., Khodabandelou, G., Gauthier, V., El Yacoubi, M., and Puchinger, J. (2019). Inferring Dynamic Origin-Destination Flows by Transport Mode Using mobile Phone Data. Transportation Res. C: Emerging Tech. 101, 254–275. doi:10.1016/j.trc.2019.02.013
Cao, Y., Zhang, X., Fu, Y., Lu, Z., and Shen, X. (2020). Urban Spatial Growth Modeling Using Logistic Regression and Cellular Automata: A Case Study of Hangzhou. Ecol. Indic 113. doi:10.1016/j.ecolind.2020.106200
Cartenì, A., Di Francesco, L., and Martino, M. (2020). How Mobility Habits Influenced the Spread of the COVID-19 Pandemic: Results from the Italian Case Study. Sci. Total Environ. 741, 140489. doi:10.1016/j.scitotenv.2020.140489
Chakraborty, I., and Maity, P. (2020). COVID-19 Outbreak: Migration, Effects on Society, Global Environment and Prevention. Sci. Total Environ. 728, 138882. doi:10.1016/j.scitotenv.2020.138882
Chatterjee, A., Gerdes, M. W., and Martinez, S. G. (2020). Statistical Explorations and Univariate Timeseries Analysis on COVID-19 Datasets to Understand the Trend of Disease Spreading and Death. SENSORS-BASEL 20. doi:10.3390/s20113089
Chen, J., Guo, X., Pan, H., and Zhong, S. (2021). What Determines City's Resilience against Epidemic Outbreak: Evidence from China's COVID-19 Experience. SUSTAIN. CITIES SOC. 70. doi:10.1016/j.scs.2021.102892
Cheng, J., and Masser, I. (2003). Urban Growth Pattern Modeling: a Case Study of Wuhan City, PR China. Landscape Urban Plann. 62, 199–217. doi:10.1016/s0169-2046(02)00150-0
Chirisa, I., Mutambisi, T., Chivenge, M., Mabaso, E., Matamanda, A. R., and Ncube, R. (2020). The Urban Penalty of COVID-19 Lockdowns across the globe: Manifestations and Lessons for Anglophone Sub-saharan Africa. GeoJournal, 1–14. doi:10.1007/s10708-020-10281-6
Cokun, H., Yildirim, N., and Gunduz, S. (2021). The Spread of COVID-19 Virus through Population Density and Wind in Turkey Cities. SCI. TOTAL ENVIRON. 751. doi:10.1016/j.scitotenv.2020.141663
Du, Z., Wang, L., Cauchemez, S., Xu, X., Wang, X., Cowling, B. J., et al. (2020). Risk for Transportation of Coronavirus Disease from Wuhan to Other Cities in China. Emerg. Infect. Dis. 26, 1049–1052. doi:10.3201/eid2605.200146
DuPre, N. C., Karimi, S., Zhang, C. H., Blair, L., Gupta, A., Alharbi, L. M. A., et al. (2021). County-level Demographic, Social, Economic, and Lifestyle Correlates of COVID-19 Infection and Death Trajectories during the First Wave of the Pandemic in the United States. Sci. Total Environ. 786, 147495. doi:10.1016/j.scitotenv.2021.147495
Fan, D., Li, Y., Liu, W., Yue, X. G., and Boustras, G. (2021). Weaving Public Health and Safety Nets to Respond the COVID-19 Pandemic. Saf. Sci. 134, 105058. doi:10.1016/j.ssci.2020.105058
Fan, P., Yue, W., Zhang, J., Huang, H., Messina, J., Verburg, P. H., et al. (2020). The Spatial Restructuring and Determinants of Industrial Landscape in a Mega City under Rapid Urbanization. Habitat Int. 95. doi:10.1016/j.habitatint.2019.102099
Feroze, N. (2020). Forecasting the Patterns of COVID-19 and Causal Impacts of Lockdown in Top Five Affected Countries Using Bayesian Structural Time Series Models. Chaos Solitons Fractals 140, 110196. doi:10.1016/j.chaos.2020.110196
Gosavi, A., and Marley, R. J. (2020). Public Policy in a Pandemic: A Hazard-Control Perspective and a Case Study of the BCG Vaccine for COVID-19. IEEE Eng. Manag. Rev. 48, 111–117. doi:10.1109/emr.2020.3010438
Granella, F., Reis, L. A., Bosetti, V., and Tavoni, M. (2021). COVID-19 Lockdown Only Partially Alleviates Health Impacts of Air Pollution in Northern Italy. Environ. Res. Lett. 16. doi:10.1088/1748-9326/abd3d2
Hâncean, M. G., Perc, M., and Lerner, J. (2020). Early Spread of COVID-19 in Romania: Imported Cases from Italy and Human-To-Human Transmission Networks. R. Soc. Open Sci. 7, 200780. doi:10.1098/rsos.200780
Hassan, M. S., Bhuiyan, M. A. H., Tareq, F., Bodrud-Doza, M., Tanu, S. M., and Rabbani, K. A. (2021). Relationship between COVID-19 Infection Rates and Air Pollution, Geo-Meteorological, and Social Parameters. Environ. Monitor. Assess. 193, 29. doi:10.1007/s10661-020-08810-4
Hasselwander, M., Tamagusko, T., Bigotte, J. F., Ferreira, A., Mejia, A., and Ferranti, E. J. S. (2021). Building Back Better: The COVID-19 Pandemic and Transport Policy Implications for a Developing Megacity. Sustain. Cities Soc. 69. doi:10.1016/j.scs.2021.102864
He, X., Zhou, C., Zhang, J., and Yuan, X. (2020). Using Wavelet Transforms to Fuse Nighttime Light Data and POI Big Data to Extract Urban Built-Up Areas. Remote Sense-Basel. 12, 1–20. doi:10.3390/rs12233887
He, X., Yuan, X., Zhang, D., Zhang, R., Li, M., and Zhou, C. (2021). Delineation of Urban Agglomeration Boundary Based on Multisource Big Data Fusion-A Case Study of Guangdong-Hong Kong-Macao Greater Bay Area (GBA). Remote Sensing 13, 1801. doi:10.3390/rs13091801
Heidari, M., Mirniaharikandehei, S., Khuzani, A. Z., Danala, G., Qiu, Y., and Zheng, B. (2020). Improving the Performance of CNN to Predict the Likelihood of COVID-19 Using Chest X-ray Images with Preprocessing Algorithms. Int. J. Med. Inform. 144, 104284. doi:10.1016/j.ijmedinf.2020.104284
Hou, X., Gao, S., Li, Q., Kang, Y., Chen, N., Chen, K., et al. (2021). Intracounty Modeling of COVID-19 Infection with Human Mobility: Assessing Spatial Heterogeneity with Business Traffic, Age, and Race. P Natil. Acad. Sci. USA, 118. doi:10.1073/pnas.2020524118
Hu, S., Xiong, C., Yang, M., Younes, H., Luo, W., and Zhang, L. (2021). A Big-Data Driven Approach to Analyzing and Modeling Human Mobility Trend under Non-pharmaceutical Interventions during COVID-19 Pandemic. Transportation Research Part C: Emerging Technologies 124. doi:10.1016/j.trc.2020.102955
Jia, J. S., Lu, X., Yuan, Y., Xu, G., Jia, J., and Christakis, N. A. (2020). Population Flow Drives Spatio-Temporal Distribution of COVID-19 in China. Nature 582, 389–394. doi:10.1038/s41586-020-2284-y
Kan, Z., Kwan, M. P., Wong, M. S., Huang, J., and Liu, D. (2021). Identifying the Space-Time Patterns of COVID-19 Risk and Their Associations with Different Built Environment Features in Hong Kong. Sci. Total Environ. 772, 145379. doi:10.1016/j.scitotenv.2021.145379
Khavarian-Garmsir, A. R., Sharifi, A., and Moradpour, N. (2021). Are High-Density Districts More Vulnerable to the COVID-19 Pandemic? Sustain. Cities Soc. 70. doi:10.1016/j.scs.2021.102911
Kranji, N., Medak, D., Upan, R., and Rezo, M. (2019). Support Vector Machine Accuracy Assessment for Extracting green Urban Areas in Towns. Remote Sense-Basel 11. doi:10.3390/rs11060655
Kwok, C. Y. T., Wong, M. S., Chan, K. L., Kwan, M. P., Nichol, J. E., Liu, C. H., et al. (2021). Spatial Analysis of the Impact of Urban Geometry and Socio-Demographic Characteristics on COVID-19, a Study in Hong Kong. Sci. Total Environ. 764, 144455. doi:10.1016/j.scitotenv.2020.144455
Lai, Y., Tang, B., Chen, X., and Zheng, X. (2021). Spatial Determinants of Land Redevelopment in the Urban Renewal Processes in Shenzhen, China. Land Use Policy 103. doi:10.1016/j.landusepol.2021.105330
Li, C., Wang, X., Wu, X., Liu, J., Ji, D., and Du, J. (2017). Modeling and Projection of Dengue Fever Cases in Guangzhou Based on Variation of Weather Factors. Sci. Total Environ. 605-606, 867–873. doi:10.1016/j.scitotenv.2017.06.181
Li, Q., Tang, Z., Coleman, N., and Mostafavi, A. (2021). Detecting Early-Warning Signals in Time Series of Visits to Points of Interest to Examine Population Response to COVID-19 Pandemic. IEEE Access 9, 27189–27200. doi:10.1109/access.2021.3058568
Li, S., Song, K., Yang, B., Gao, Y., and Gao, X. (2020). Preliminary Assessment of the COVID-19 Outbreak Using 3-Staged Model E-ISHR. J. Shanghai Jiaotong Univ. (Sci.) 25, 157–164. doi:10.1007/s12204-020-2169-0
Liu, M., Liu, M., Li, Z., Zhu, Y., Liu, Y., Wang, X., et al. (2021). The Spatial Clustering Analysis of COVID-19 and its Associated Factors in mainland China at the Prefecture Level. Sci. Total Environ. 777. doi:10.1016/j.scitotenv.2021.145992
Liu, Q., Sha, D., Liu, W., Houser, P., Zhang, L., Hou, R., et al. (2020). Spatiotemporal Patterns of COVID-19 Impact on Human Activities and Environment in Mainland China Using Nighttime Light and Air Quality Data. Remote Sense-Basel 12. doi:10.3390/rs12101576
Liu, X. J., and Mesch, G. S. (2020). The Adoption of Preventive Behaviors during the COVID-19 Pandemic in China and Israel. Int. J. Environ. Res. Public Health 17, 17. doi:10.3390/ijerph17197170
Luo, L., Mei, K., Qu, L., Zhang, C., Chen, H., Wang, S., et al. (2019). Assessment of the Geographical Detector Method for Investigating Heavy Metal Source Apportionment in an Urban Watershed of Eastern China. Sci. Total Environ. 653, 714–722. doi:10.1016/j.scitotenv.2018.10.424
Mahajan, V., Cantelmo, G., and Antoniou, C. (2021). Explaining Demand Patterns during COVID-19 Using Opportunistic Data: a Case Study of the City of Munich. Eur. Transp Res. Rev. 13. doi:10.1186/s12544-021-00485-3
Mansour, S., Al Kindi, A., Al-Said, A., Al-Said, A., and Atkinson, P. (2021). Sociodemographic Determinants of COVID-19 Incidence Rates in Oman: Geospatial Modelling Using Multiscale Geographically Weighted Regression (MGWR). Sustain. Cities Soc. 65. doi:10.1016/j.scs.2020.102627
Milojevic-Dupont, N., and Creutzig, F. (2021). Machine Learning for Geographically Differentiated Climate Change Mitigation in Urban Areas. Sustain. Cities Soc. 64. doi:10.1016/j.scs.2020.102526
Navinya, C., Patidar, G., and Phuleria, H. C. (2020). Examining Effects of the COVID-19 National Lockdown on Ambient Air Quality across Urban India. Aerosol Air Qual. Res. 20, 1759–1771. doi:10.4209/aaqr.2020.05.0256
Nguyen, Q. C., Huang, Y., Kumar, A., Duan, H., Keralis, J. M., Dwivedi, P., et al. (2020). Using 164 Million Google Street View Images to Derive Built Environment Predictors of COVID-19 Cases. Int. J. Environ. Res. Public Health 17, 17. doi:10.3390/ijerph17176359
Ouyang, X., Huo, J., Xia, L., Shan, F., Liu, J., Mo, Z., et al. (2020). Dual-Sampling Attention Network for Diagnosis of COVID-19 from Community Acquired Pneumonia. IEEE Trans. Med. Imaging 39, 2595–2605. doi:10.1109/tmi.2020.2995508
Peirlinck, M., Linka, K., Sahli Costabal, F., and Kuhl, E. (2020). Outbreak Dynamics of COVID-19 in China and the United States. Biomech. Model. Mechanobiol 19, 2179–2193. doi:10.1007/s10237-020-01332-5
Piovella, N. (2020). Analytical Solution of SEIR Model Describing the Free Spread of the COVID-19 Pandemic. Chaos Solitons Fractals 140, 110243. doi:10.1016/j.chaos.2020.110243
Polo, D., Quintela-Baluja, M., Corbishley, A., Jones, D. L., Singer, A. C., Graham, D. W., et al. (2020). Making Waves: Wastewater-Based Epidemiology for COVID-19 - Approaches and Challenges for Surveillance and Prediction. Water Res. 186, 116404. doi:10.1016/j.watres.2020.116404
Ribeiro, H. V., Sunahara, A. S., Sutton, J., Perc, M., and Hanley, Q. S. (2020). City Size and the Spreading of COVID-19 in Brazil. PLOS ONE 15, e0239699. doi:10.1371/journal.pone.0239699
Rousseau, S., and Deschacht, N. (2020). Public Awareness of Nature and the Environment during the COVID-19 Crisis. Environ. Resource Econ. 76, 1149–1159. doi:10.1007/s10640-020-00445-w
Saedi, R., Verma, R., Zockaie, A., Ghamami, M., and Gates, T. J. (2020). Comparison of Support Vector and Non-linear Regression Models for Estimating Large-Scale Vehicular Emissions, Incorporating Network-wide Fundamental Diagram for Heterogeneous Vehicles. Transportation Res. Rec. 2674, 70–84. doi:10.1177/0361198120914304
Sharifi, A., and Khavarian-Garmsir, A. R. (2020). The COVID-19 Pandemic: Impacts on Cities and Major Lessons for Urban Planning, Design, and Management. Sci. Total. Environ. 749, 142391. doi:10.1016/j.scitotenv.2020.142391
Shu, B., Zhu, S., Qu, Y., Zhang, H., Li, X., and Carsjens, G. J. (2020). Modelling Multi-Regional Urban Growth with Multilevel Logistic Cellular Automata. Compute. Environ. Urban 80. doi:10.1016/j.compenvurbsys.2019.101457
Shupler, M., Mwitari, J., Gohole, A., Anderson De Cuevas, R., Puzzolo, E., Čukić, I., et al. (2021). COVID-19 Impacts on Household Energy & Food Security in a Kenyan Informal Settlement: The Need for Integrated Approaches to the SDGs. Renew. Sustain. Energ. Rev 144, None. doi:10.1016/j.rser.2021.111018
Siddiqui, A., Siddiqui, A., Maithani, S., Jha, A. K., Kumar, P., and Srivastav, S. K. (2018). Urban Growth Dynamics of an Indian Metropolitan Using CA Markov and Logistic Regression. Egypt. J. Remote Sensing Space Sci. 21, 229–236. doi:10.1016/j.ejrs.2017.11.006
Silva, B. N., Khan, M., Jung, C., Seo, J., Muhammad, D., Han, J., et al. (2018). Urban Planning and Smart City Decision Management Empowered by Real-Time Data Processing Using Big Data Analytics. Sensors (Basel) 18. doi:10.3390/s18092994
Stevens, N. J., Tavares, S. G., and Salmon, P. M. (2021). The Adaptive Capacity of Public Space under COVID‐19: Exploring Urban Design Interventions through a Sociotechnical Systems Approach. Hum. Factors Man. 31, 333–348. doi:10.1002/hfm.20906
Thomas, L. J., Huang, P., Yin, F., Luo, X. I., Almquist, Z. W., Hipp, J. R., et al. (2020). Spatial Heterogeneity Can lead to Substantial Local Variations in COVID-19 Timing and Severity. Proc. Natl. Acad. Sci. USA 117, 24180–24187. doi:10.1073/pnas.2011656117
Venter, Z. S., Barton, D. N., Gundersen, V., Figari, H., and Nowell, M. (2020). Urban Nature in a Time of Crisis: Recreational Use of green Space Increases during the COVID-19 Outbreak in Oslo, Norway. Environ. Res. Lett. 15. doi:10.1088/1748-9326/abb396
Wang, B., Sun, Y., Duong, T. Q., Nguyen, L. D., and Hanzo, L. (2020). Risk-Aware Identification of Highly Suspected COVID-19 Cases in Social IoT: A Joint Graph Theory and Reinforcement Learning Approach. IEEE Access 8, 115655–115661. doi:10.1109/access.2020.3003750
Wang, Y., Li, Z., Zeng, C., Xia, G., and Shen, H. (2020). An Urban Water Extraction Method Combining Deep Learning and Google Earth Engine. IEEE J-Stars 13, 768–781. doi:10.1109/jstars.2020.2971783
Wang, Y., Sha, Z., Tan, X., Lan, H., Liu, X., and Rao, J. (2020). Modeling Urban Growth by Coupling Localized Spatio-Temporal Association Analysis and Binary Logistic Regression. Comput. Environ. Urban Syst. 81. doi:10.1016/j.compenvurbsys.2020.101482
Werth, A., Gravino, P., and Prevedello, G. (2021). Impact Analysis of COVID-19 Responses on Energy Grid Dynamics in Europe. Appl. Energ. 281, 116045. doi:10.1016/j.apenergy.2020.116045
Wu, J., Wang, K., He, C., Huang, X., and Dong, K. (2021). Characterizing the Patterns of China's Policies against COVID-19: A Bibliometric Study. Inform. Process Manag. 58. doi:10.1016/j.ipm.2021.102562
Wu, Y., Chen, F., Sun, Z., Zhang, Y., Song, Y., Feng, W., et al. (2021). Impact of the Pandemic of COVID-19 on Emergency Attendance for Stroke and Acute Myocardial Infarction in Beijing, China. Theromb. Thromblysis. Journal of Thrombosis and Thrombolysis. doi:10.1007/s11239-021-02385-8
Xiao, Y., Wang, D., and Fang, J. (2019). Exploring the Disparities in Park Access through mobile Phone Data: Evidence from Shanghai, China. Landscape Urban Plann. 181, 80–91. doi:10.1016/j.landurbplan.2018.09.013
Xie, J., and Zhu, Y. (2020). Association between Ambient Temperature and COVID-19 Infection in 122 Cities from China. Sci. Total Environ. 724, 138201. doi:10.1016/j.scitotenv.2020.138201
Xu, J., Ge, X., Zhang, X., Zhao, W., Zhang, R., and Zhang, Y. (2020). COVID-19 Impact on the Concentration and Composition of Submicron Particulate Matter in a Typical City of Northwest China. Geophys. Res. Lett. 47, e2020GL089035. doi:10.1029/2020GL089035
Xu, X., Wen, C., Zhang, G., Sun, H., Liu, B., and Wang, X. (2020). The Geographical Destination Distribution and Effect of Outflow Population of Wuhan when the Outbreak of COVID-19. Dianzi Keji Daxue Xuebao/Journal of the University of Electronic Science and Technology of China, 324–329.
Yan, L., Li, J., Liu, M., Hu, M., Xu, Z., and Xue, K. (2021). Heating Behavior Using Household Air-Conditioners during the COVID-19 Lockdown in Wuhan: An Exploratory and Comparative Study. Build Environ. 195, 107731. doi:10.1016/j.buildenv.2021.107731
Yawney, J., and Gadsden, S. A. (2020). A Study of the COVID-19 Impacts on the Canadian Population. IEEE Access 8, 128240–128249. doi:10.1109/access.2020.3008608
Yorio, P. L., and Moore, S. M. (2018). Examining Factors that Influence the Existence of Heinrich's Safety Triangle Using Site-specific H&S Data from More Than 25,000 Establishments. Risk Anal. 38, 839–852. doi:10.1111/risa.12869
Yu, X., Qi, G., and Hu, J. (2020). Analysis of Second Outbreak of COVID-19 after Relaxation of Control Measures in India. Nonlinear Dynam. doi:10.1007/s11071-020-05989-6
Yuchi, W., Gombojav, E., Boldbaatar, B., Galsuren, J., Enkhmaa, S., Beejin, B., et al. (2019). Evaluation of Random forest Regression and Multiple Linear Regression for Predicting Indoor fine Particulate Matter Concentrations in a Highly Polluted City. Environ. Pollut. 245, 746–753. doi:10.1016/j.envpol.2018.11.034
Zhan, Z., Wei, Q., and Hong, J. (2021). Cellphone Addiction during the Covid-19 Outbreak: How Online Social Anxiety and Cyber Danger Belief Mediate the Influence of Personality. Comput. Hum. Behav. 121. doi:10.1016/j.chb.2021.106790
Zhang, C., Pei, Y., Li, J., Qin, Q., and Yue, J. (2020). Application of Luojia 1-01 Nighttime Images for Detecting the Light Changes for the 2019 spring Festival in Western Cities, China. Remote Sens-Basel 12. doi:10.3390/rs12091416
Zhang, J., and Yuan, X. (2021). COVID-19 Risk Assessment: Contributing to Maintaining Urban Public Health Security and Achieving Sustainable Urban Development. Sustainability 13, 4208. doi:10.3390/su13084208
Zhang, L., Chen, K., Jiang, H., and Zhao, J. (2020). How the Health Rumor Misleads People's Perception in a Public Health Emergency: Lessons from a Purchase Craze during the COVID-19 Outbreak in China. Int. J. Environ. Res. Public Health 17. doi:10.3390/ijerph17197213
Zhang, X., Lin, H., Zhu, C., Wang, J., Li, M., Xu, C., et al. (2020). Spatiotemporal Characteristics and Dynamic Process Analysis of Epidemic Diagnosis Time of COVID-19 at Early Stage in Chinese Mainland, 45. Geomatics and Information Science of Wuhan University, 45, 791–797. doi:10.13203/j.whugis20200255
Zhou, L., Dang, X., Sun, Q., and Wang, S. (2020). Multi-scenario Simulation of Urban Land Change in Shanghai by Random forest and CA-Markov Model. Sustain. Cities Soc., 55. doi:10.1016/j.scs.2020.102045
Keywords: Guangzhou, logistic regression, influencing factors, interaction, spatiotemporal geography
Citation: He X, Zhou C, Wang Y and Yuan X (2021) Risk Assessment and Prediction of COVID-19 Based on Epidemiological Data From Spatiotemporal Geography. Front. Environ. Sci. 9:634156. doi: 10.3389/fenvs.2021.634156
Received: 07 December 2020; Accepted: 20 July 2021;
Published: 30 July 2021.
Edited by:
Marco Casazza, University of Naples Parthenope, ItalyReviewed by:
Chaowei Yang, George Mason University, United StatesYuhao Kang, University of Wisconsin-Madison, United States
Copyright © 2021 He, Zhou, Wang and Yuan. This is an open-access article distributed under the terms of the Creative Commons Attribution License (CC BY). The use, distribution or reproduction in other forums is permitted, provided the original author(s) and the copyright owner(s) are credited and that the original publication in this journal is cited, in accordance with accepted academic practice. No use, distribution or reproduction is permitted which does not comply with these terms.
*Correspondence: Chunshan Zhou, emhvdWNzQG1haWwuc3lzdS5lZHUuY24=