- 1State Key Laboratory of Water Environment Simulation and School of Environment, Beijing Normal University, Beijing, China
- 2Yellow River Estuary Wetland Ecosystem Observation and Research Station, Ministry of Education, Dongying, China
- 3Research and Development Center for Watershed Environmental Eco‐Engineering, Beijing Normal University, Zhuhai, China
- 4Shandong Yellow River Delta Nature Reserve Administration, Dongying, China
- 5Tang Scholar, Beijing Normal University, Beijing, China
In recent decades, the invasion of saltmarsh plant Spartina alterniflora (S. alterniflora) over a large part of coastal wetlands in China, including the Yellow River Estuary (YRE) as a regional economic hub and global ecosystem services hotspot, has caused increasing concern because of its serious threats to native ecosystems. During the same period, local authorities have implemented a Water-Sediment Regulation Scheme (WSRS) in the Yellow River for flood mitigation and delta restoration purposes. The altered hydrological regime has resulted in unintended changes to estuarine ecosystem. However, the direct consequence of the WSRS on the expansion of S. alterniflora remains unclear. In this study, quantitative relationship between the inter- and intra-annual expansion patterns of S. alterniflora represented by relevant landscape metrics and indicators that quantify the concurrent variations of river and sediment discharges as the proxy of the WSRS impacts were analysed over the period of Year 2011–2018, and the analyses were performed on the YRE as a whole and on five different zones subdivided based on the invasion sequence. The results showed that there was no significant difference in the inter-annual area variation of S. alterniflora between the years with and without WSRS. Compared with the years without WSRS (2016–2017), the intra-annual (monthly) increment of the various landscape metrics [i.e., NP (number of patches), CA (class area), LPI (largest patch index) and AI (aggregation index] were found to be significantly higher in the initial stage of peak growing season (June-July) than in the mid- and late stages (July-September) in the years with WSRS (2011–2015, 2018) in the subregion located close to the south bank of YRE as the most prominent impact zone. In addition, F (mean flow), Ff (number of high flow pulses), Tf (Julian date of maximum flow) and D (duration of WSRS) were identified as the explanatory variables for the intra-annual vegetation landscape pattern changes, and their relative contributions to resultant changes were also assessed. Our results broaden the understanding of estuarine hydrological disturbance as a potential driver regulating the saltmarsh vegetation, and also have implications for S. alterniflora invasion control at estuaries under changing environment.
Introduction
Spartina alterniflora (S. alterniflora), an exotic species native to the East Coast of North America, was introduced to coastal areas of China in 1979 for promoting sediment deposition and erosion control (Li et al., 2009). Ever since then, S. alterniflora has gradually expanded to large areas of estuarine and coastal wetlands due to its high salt tolerance and strong reproductive capacity (Liu et al., 2018; Qiu et al., 2020), and posed serious threats to local native ecosystems (Schwarz et al., 2016). The growth and expansion of S. alterniflora are dependent on a number of key physical factors in estuarine saltmarshes, such as surface elevation (Carey et al., 2017), inundation (Li et al., 2018) and salinity (Elsey-Quirk et al., 2009). Subject to the combined action of terrestrial and marine forcings such as the river and sediment discharges, waves and tides, these physical factors per se are constantly evolving at the estuaries (Snedden et al., 2015; Gao et al., 2018; Han et al., 2018; Poormahdi et al., 2018). Furthermore, it is well established that frequent interventions of human activities such as upstream dam regulation can result in dramatic alterations of river and sediment discharges (Wang et al., 2017; Gao et al., 2018). Therefore, it is necessary to elucidate the roles of the estuarine hydrological alteration to better understand invasion process of S. alterniflora at the estuaries that are crucial for wetland ecosystem management.
A number of studies have examined the interplay between the variations of river and sediment discharges and marsh vegetation on the intra-annual (seasonal) or inter-annual timescale (e.g., Kearney et al., 2011; Carle et al., 2015; Olliver and Edmonds, 2017; Hu et al., 2019). Amongst them, Kearney et al. (2011) analyzed the vegetation cover and marsh area at the freshwater diversion sites at the Louisiana’s coastal wetlands from 1984 to 2005 using Landsat data, and showed that freshwater diversions have not increased vegetation and marsh coverage. However, the input of additional nutrients as a result of the freshwater diversion reduced marsh below-ground biomass and increased its vulnerability to storm damage. Carle et al. (2015) assessed the impact of the historical 2011 Mississippi River flood on the Wax Lake Delta and its vegetation community change using remote sensing and LIDAR data, and found a net delta growth and the conversion between lower-elevation and higher-elevation species in both directions across the delta due to the heterogeneous elevation change. Olliver and Edmonds (2017) confirmed the control of surface elevation and inundation period on the extent of vegetation communities through their analyses of the inter- and intra-annual changes in vegetation cover of Wax Lake Delta using remote sensing and field data.
Based on the monitoring of mudflat elevation and marsh vegetation area at the Yangtze Estuary from 2013 to 2017, Hu et al. (2019) reported that the year with higher river and sediment discharges resulted in significantly higher rates of mudflat accretion and vegetation expansion, which suggested that a short-term increase in river and sediment discharges can stimulate the vegetation expansion due to the positive feedback between vegetation establishment and sedimentary processes. In addition, a number of studies have explored the influences of flow-sediment regime shift on landscape pattern changes of riparian plant species, such as cover of vegetation communities (Caruso et al., 2013), spatial distribution range (Mortenson and Weisberg, 2010) and landscape fragmentation degree (Shen and Ma 2020). By far, the majority of the existing studies focused on assessing the inter-annual change of marsh vegetation coverage and distribution in response to the variable river and sediment discharges using remote sensing data. However, direct relationship between the flow-sediment regime variables and the resultant expansion patterns of saltmarsh plants as represented by the relevant landscape metrics on both inter- and intra-annual timescales remain elusive.
The Yellow River Estuary (YRE) located in the northeast of Shandong Province, China, is a regional economic hub where a major oil field of China (the Shengli Oil Field) is located as well as a global ecosystem services hotspot hosting a key stopover site in the middle of the East Asian-Australasian flyway, which was designated as a Ramsar site in 2013 (Luo et al., 2018). S. alterniflora was purposely introduced to YRE for dike protection and erosion control in 1990, and has since spread to the tidal marshes along the elevation gradient (Ren et al., 2014). Meanwhile, the Yellow River Conservancy Commission (YRCC) initiated the Water-Sediment Regulation Scheme (WSRS) in the Yellow River in 2002 to create artificial flood pulses through dam regulation during a one-month period of the flood season every year to mitigate the siltation both in the Xiaolangdi Reservoir and the lower reaches, and restore ecohydrological conditions downstream (Zhang et al., 2016). As the WSRS resulted in a significant increase in river discharge and sediment load during the operation period, it posed unexpected disturbances on estuarine and coastal ecosystems (Wang et al., 2017). Notably, Ren et al. (2014) found that the expansion of S. alterniflora at the Yellow River Delta was closely related to the fluctuating discharge and sediment load of the Yellow River, which was partly caused by WSRS. Wang et al. (2017) and Bi et al. (2019) demonstrated that coarser suspended sediments induced by WSRS tended to deposit locally at the estuary and stimulate rapid elevation accretion. Ren et al. (2019) studied the inter-annual expansion process of S. alterniflora at the Yellow River Delta, and reported a significant correlation between the expansion area and the improvement in water quality of the Yellow River. Nevertheless, until now, the effects of WSRS on the expansion of S. alterniflora at the YRE are still unclear.
In this study, we explore the effects of WSRS on the spatiotemporal expansion dynamics and distribution patterns of S. alterniflora at the YRE, through assessing the inter- and intra-annual changes of relevant landscape metrics between the years with WSRS (2011–2015, 2018) and the years without WSRS (2016–2017). As a case study, it also sheds some light on the broad question of the effects of flow-sediment regime shift caused by variable river discharge and sediment load on the colonization patterns of estuarine saltmarsh plants. The improved understanding on the invasion process of S. alterniflora can also inform invasion control under changing environment.
Materials and Methods
Study Area
The study area is located at the YRE wetland in Dongying City, China (119°09′E-119°19′E; 37°43′N-37°51′N, Figure 1), which has a typical semi-humid continental monsoon climate with mean annual temperature of 12.1°C and precipitation of 551.6 mm (the rainfall is mostly distributed between July and August) (Song et al., 2018). In 1996, the Yellow River shifted to the Q8 course before emptying into the Bohai Sea after an artificial avulsion, which resulted in the current YRE (Zhang et al., 2016). The Yellow River Delta purposely introduced S. alterniflora near the No. 5 pile to the north of the Gudong oil exploration site in 1990, and S. alterniflora has since spread across the low marshes and spread out to the high marshes gradually (Ren et al., 2014). More recently, some field surveys and remote sensing image analyses have observed that there was a rapid expansion of S. alterniflora communities at the current YRE since 2011 (Ren et al., 2019).
In 2002, the YRCC began to implement the Water-Sediment Regulation Scheme (WSRS) in the Yellow River to mitigate the siltation both in the lower river reaches and the Xiaolangdi Reservoir by flood pulses created by dam regulation. The WSRS has been operated every year since 2002, except 2016 and 2017, which resulted in significant increases in river and sediment discharges during a short period (∼20 days) from mid-June to early July (Zhang et al., 2016; Wang et al., 2017). Notably, the WSRS has posed substantial changes on estuarine and coastal ecosystems, such as shift of sedimentation dynamics and changes in delta morphology (Wang et al., 2010; Bi et al., 2014).
In this study, the entire YRE was subdivided into five sub-regions from a to e based on the sequence of the land formation and invasion (colonization) of the S. alterniflora (Figure 2). Specifically, sub-region a and sub-region d were created by sediments from the Yellow River during the 2011 flood, where S. alterniflora began to spread on a large scale around the same period. Sub-regions b and c were subsequently formed in 2013, and sub-region b was colonized by S. alterniflora in 2014, while S. alterniflora did not emerge in sub-region c until 2016. Further away from the river mouth, sub-region e was formed in 2007, where S. alterniflora began to spread on a large scale in 2013.
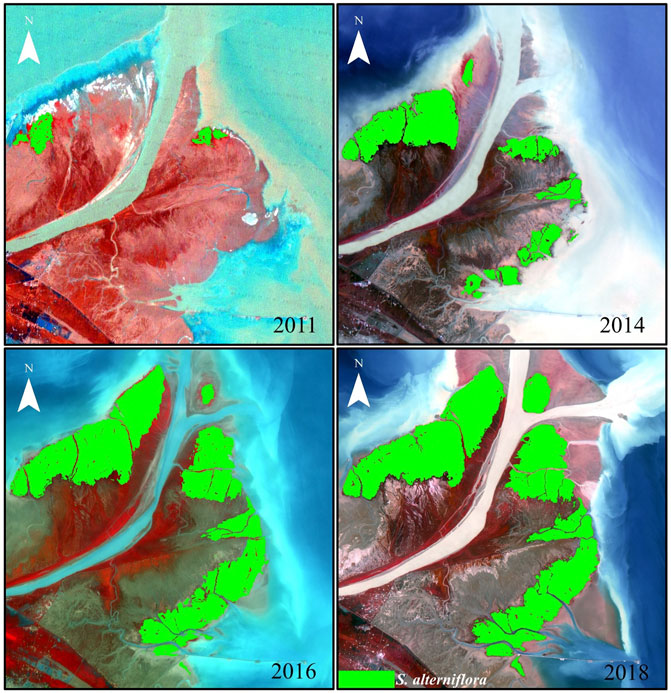
FIGURE 2. S. alterniflora spatial distribution in December 11, 2011, September 22, 2014, September 11, 2016 and September 17, 2018, respectively.
Remote Sensing Data and Image Processing
The WSRS usually takes place in June-July every year since 2002, except 2016 and 2017, and S. alterniflora began to spread rapidly at the YRE since 2011 (Zhang et al., 2016). Moreover, the peak growing season of S. alterniflora is from June to September, and it almost stops growing at the end of September at the YRE. According to previous studies, temporally adjacent dataset can yield a time series to estimate the effect of external disturbances to ecosystems (Walker et al., 2015). Considering its short return cycle (16 days) and moderate resolution, the Landsat satellite images were suitable for observing seasonal dynamics of S. alterniflora over the study period. Therefore, 32 good-quality (i.e., cloud-free, low tide level) Landsat 7/8 remote sensing images of YRE with temporally adjacent mean 30 days (1 image per month) from June to September during 2011 to 2018 were acquired to examine the spatiotemporal patterns of S. alterniflora invasion in our study. These images were downloaded from the United States Geological Survey (USGS). Table 1 lists the features of the images used in this study.
Following previous studies (Liu et al., 2018; Mao et al., 2019), we chose maximum likelihood classifier to interpret S. alterniflora at the YRE based on its spectrum characteristics. Firstly, all images were reprojected into universe transverse Mercator world geodetic system-84 (UTM/WGS 84) coordinate system. Then, the Fast Line-of-sight Atmospheric Analysis of Hypercubes (FLAASH) model was applied to conduct atmospheric corrections in the ENVI 5.3 software (Harris Geospatial Solutions, Inc., United States). In order to improve classification accuracy, the panchromatic band with a spatial resolution of 15 m and the multispectral bands with a spatial resolution of 30 m for all Landsat 7/8 images were fused using Gram-Schmidt Pan-sharpening fusion method to exhibit higher sharpness and spectral quality. Additionally, the spectral enhancement methods of the tasseled cap transform and false color composition were used to initialize the data for efficient interpretation (Aiazzi et al., 2006). To further assess the spatial distribution of S. alterniflora, S. alterniflora and other land covers (i.e., water, mudflats, other vegetation patches such as Suaeda salsa, Tamarix chinensis and Phragmites australis) were identified as different classes and selected as training samples, and a supervised classification was carried out using the Support Vector Machine (SVM) (Mountrakis et al., 2011). Based on the field verification data and historical images, some misclassifications of unvegetated and vegetated habitats were further corrected.
Quantifying the Invasion of Spartina alterniflora
In order to quantitatively assess the landscape pattern change of S. alterniflora at the YRE, we reviewed relevant studies (Kelly et al., 2011; Almeida et al., 2016; Liu et al., 2017) and chose the landscape metrics with relevance to our study and easiness to interpret. These metrics mainly focus on composition and spatial configuration of landscape. Specifically, composition refers to the diversity and abundance of patch types within the landscape, and the composition metrics are the most basic and important measures of landscape patterns. In this study, we calculated CA (class area), NP (number of patches) and LPI (largest patch index) (McGarigal 2014). CA and NP are the metrics commonly used to measure landscape composition. LPI quantifies the percentage of total landscape area comprised by the largest patch, which is a simple measure of dominance. Spatial configuration is related to spatially explicit characteristics, which contains more complicated information about the landscape structural and functional features, and is closely related to landscape fragmentation degree (Leitão et al., 2012; Almeida et al., 2016). Here, AI (aggregation index) was chosen to assess landscape configuration, which is an effective index to measure the aggregation degree of spatial patterns (McGarigal 2014; Almeida et al., 2016). Finally, the monthly increment of the various landscape metrics (i.e., the difference of the landscape metrics between two adjacent months) was used to quantify the intra-annual difference of S. alterniflora invasion at the YRE. All landscape metrics are listed in Table 2, and they were calculated using Fragstats 4.2 software (McGarigal et al., 2002).
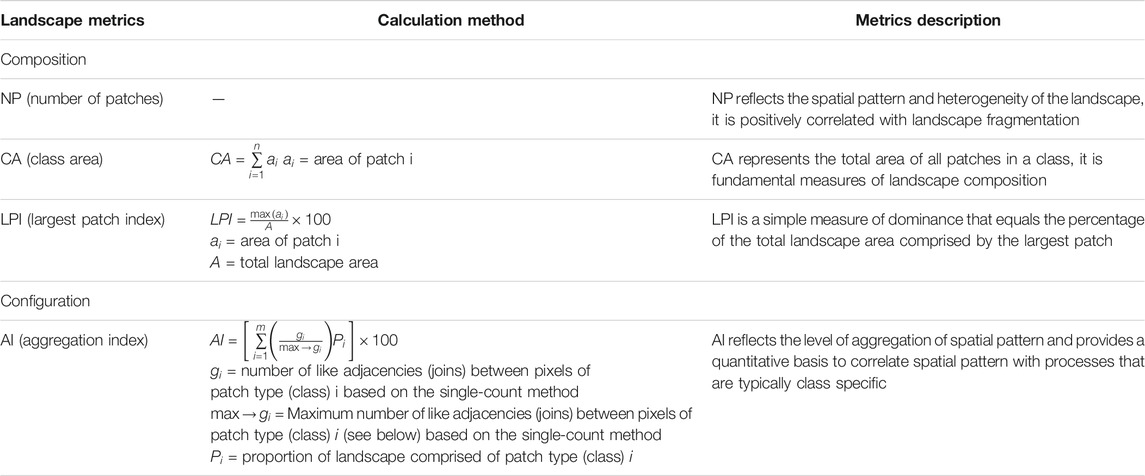
TABLE 2. List and description of selected landscape metrics. Metrics description adapted from McGarigal et al. (2002).
Hydrological Data Acquisition and Quantification With Flow-Sediment Regime Indicators
River and sediment discharges to the YRE were acquired from the records at the Lijin hydrological gauge station, which is the station closest to the YRE and located approximately 100 km upstream. Both daily runoff and sediment load data recorded at Lijin station from 2011 to 2018 have been made available by the YRCC of China.
The Indicators of Hydrologic Alteration (IHA) framework proposed by Richter et al. (1996) is one of the most widely used methods to evaluate the variations of flow regime and their impact on ecosystems. Wohl et al. (2015) broadened the flow regime concept into a more inclusive paradigm to also incorporate sediment regime. Xu and Li (2019) used the upgraded flow-sediment regime indicators of IHA to study the impact of flow-sediment regime changes on floodplain ecosystem. In this study, we adopted the IHA framework and focused on the indicators which are particularly relevant to the hydrological disturbances caused by the WSRS as well as the key life history stages of S. alterniflora (see Table 3 for a complete list of the chosen indicators).
Specifically, we chose the duration of annual WSRS as the time period for all hydrological indicators. As increases in runoff and sediment load over a short period had been demonstrated to affect the coastal ecosystem and saltmarsh plant growth (Hu et al., 2019), mean flow and sediment load during the WSRS period each year were selected. Moreover, the magnitude of flow and sediment load caused by the WSRS are far beyond the natural flow-sediment regime, and the hydrological extremes can most interfere with the phenology and growth of saltmarsh plants (Hu et al., 2019; Jones et al., 2019). As such, we chose indicators represent the magnitude and occurrence time of peak flow and sediment load. The 7 days/3 days/1 day maximum flow and sediment load were selected to best capture the peak value, and the Julian date of maximum flow and sediment load during the WSRS period each year represented the time when the peak flow and sediment load occurred. In addition, Kettenring et al. (2015) and Balke et al. (2014) found that a certain degree of frequency variation of sediment accretion/erosion and flooding can create windows of opportunity for invasion of exotic saltmarsh plants. Therefore, the number of high flow and sediment load pulses during the WSRS period each year were also selected. Besides, as the degree of fluctuation in flow and sediment regime have proven effects on establishment and survival of saltmarsh plants (Seabloom et al., 2001; Cao et al., 2018), we chose the number of flow and sediment reversals to assess the potential effects of rate of change of flow-sediment regime.
In summary, the above-chosen indicators include magnitude, timing, duration, frequency and rate of change of flow-sediment regime shift, which provides a holistic quantification of the variations of flow and sediment load that may impact the saltmarsh plants (Poff et al., 1997; Elsey-Quirk et al., 2019). These indicators were calculated using IHA software (Smythe Scientific Software, Boulder, CO, United States).
Statistical Analysis
A student’s t test (Winter 2013) was used to analyze the significance of differences in inter-annual area variation of S. alterniflora invasion between the years with and without WSRS. A repeated-measures ANOVA (Keselman et al., 2001) was used to examine the significance of trend differences in intra-annual variation of S. alterniflora landscape patterns between the years with and without WSRS. All these statistical analyses were performed in SPSS 22 software (SPSS Inc., Chicago, IL, United States).
Furthermore, we employed generalized linear models (GLMs) using Gaussian errors to estimate how flow-sediment regime variations during the WSRS affect the landscape patterns of S. alterniflora. Spearman’s rank correlation coefficients (rs) with correlation values rs < |0.75| and variance inflation factors (VIF) < 4 (R package ‘‘car’‘) were adopted as the criteria to reduce multicollinearity among the explanatory variables (Dormann et al., 2013; Fox and Weisberg 2018). The models were simplified using stepwise analysis (forward and backward) with the R package ‘‘MASS’’ (Crawley 2012). In order to assess the goodness-of-fit of the GLMs and obtain the optimal regression models, the adjusted determination coefficient (Radj2, a higher value indicates better fit), significance (p < 0.05 or 0.01) and Akaike Information Criterion (AIC, a smaller value indicates better fit) were used to compare multiple models (Burnham and Anderson 2004). Additionally, to further calculate the relative importance of all explanatory variables contained in the optimal GLMs and measure the individual contribution of each explanatory variable to the explained variables, the Lindeman, Merenda and Gold (LMG) metric was used with the R package ‘‘relaimpo’’ in the optimal models (Grömping 2006). We ranked relative importance values of each explanatory variable according to the LMG values to quantify their contribution to the intra-annual landscape pattern changes of S. alterniflora. All these modeling analyses were performed in R version 3.5.2, and the R Package “car” ‘‘MASS’’ and ‘‘relaimpo’’ were used to run the GLMs (R Core Team, 2017). In addition, the Durbin-Watson statistic (DW value: 0–4) was introduced to check the autocorrelation between residuals of optimal models (Penda et al., 2012). A value near 2 indicates non-autocorrelation between residuals, whereas values below (above) 2 indicate positive (negative) autocorrelation between residuals. Moreover, the normal probability plots of regression standardized residuals were introduced to check the normality between residuals of optimal models (Wisniak and Polishuk 1999). If the resulting plot is approximately linear, we can assume that the residuals are normally distributed. All these post-robustness tests were performed in SPSS 22 (SPSS Inc., Chicago, IL, United States).
Results
Spatiotemporal Dynamics of Spartina alterniflora Invasion
Figure 3 shows the evolution of S. alterniflora cover area and spatial distribution throughout the entire estuary and sub-regions in September each year from 2011 to 2018. In general, the S. alterniflora continued to invade from 2011 to 2018 with the total area increasing from 205.04 to 3,394.29 ha at the YRE, and the invasion of S. alterniflora followed sequence in different sub-regions. Specifically, the S. alterniflora expanded rapidly in sub-region a and increased from 146.4 ha in 2011 to 910.2 ha in 2018. Sub-regions b and c, which were newly formed in 2013, were the youngest regions at the YRE. Due to the suitable environmental conditions, S. alterniflora quickly invaded and occupied 689.5 ha in sub-region b and 143.5 ha in sub-region c in 2018. Moreover, the S. alterniflora showed continuous expansion in sub-region d throughout the study period, and the area of S. alterniflora expanded from 58.6 ha to 527.4 ha. Sub-region e hosted the largest S. alterniflora communities in the south bank of YRE, and the area of S. alterniflora covered 1,123.67 ha in 2018. In addition, we also analyzed the significance of differences in inter-annual variation of S. alterniflora cover area in September between the years with WSRS (2011–2015, 2018) and without WSRS (2016–2017) for the entire YRE and all sub-regions. The results consistently showed no significant difference throughout the study area and study period.
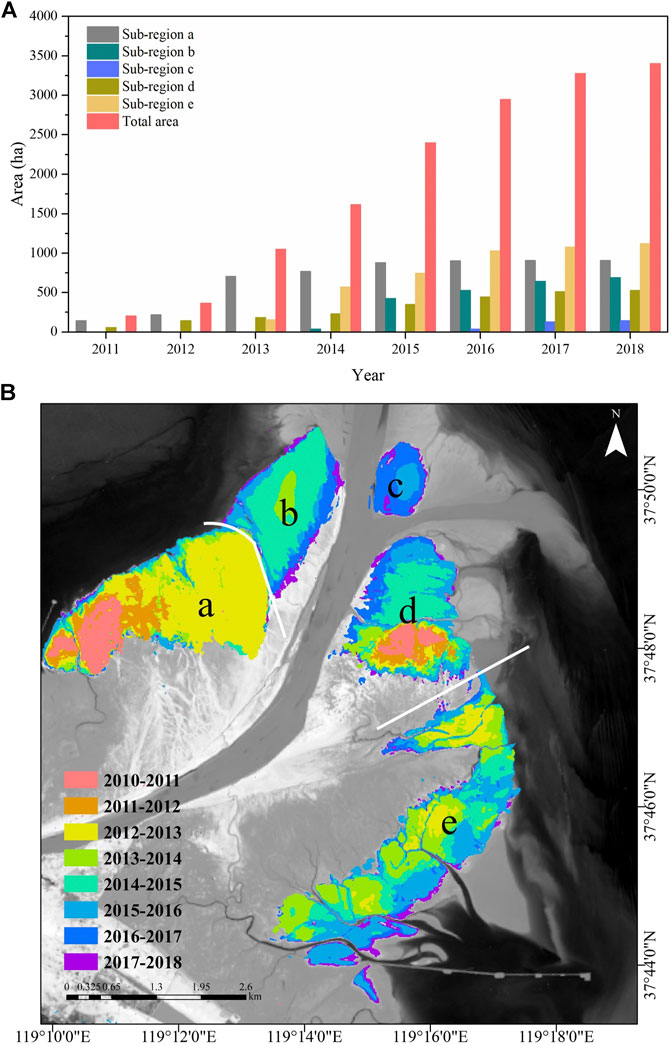
FIGURE 3. (A) Temporal evolution of S. alterniflora cover area for the entire Yellow River Estuary and the sub-regions from 2011 to 2018 and (B) Spatial variation of S. alterniflora at the Yellow River Estuary from 2011 to 2018.
We further analyzed the trend differences in the intra-annual area variation of S. alterniflora invasion between the years with and without WSRS (Figure 4). The results showed that there was no significant difference for the entire YRE (p = 0.248). However, when breaking up into the sub-regions, monthly increment of area in sub-region d in the years with WSRS was significantly different from years without WSRS (p = 0.037). In addition, in the years with WSRS, the monthly area increment of S. alterniflora was significantly higher in the initial stage of the peak growing season (June-July) than in the mid- and late stages (July-September), compared to the years without WSRS. In contrast to sub-region d, the intra-annual variation of S. alterniflora area in sub-region b (p = 0.114) and sub-region e (p = 0.318) showed no significant trend differences between the years with and without WSRS. It is noteworthy that S. alterniflora hardly spread in sub-region a since 2016, and S. alterniflora did not appear in sub-region c until 2016 (Figure 3). As such, there were not enough and accurate data to analyze the differences of S. alterniflora in years with and without WSRS, and thus we excluded these two sub-regions as well as sub-region b and e, and focus on sub-region d in our subsequent analysis.
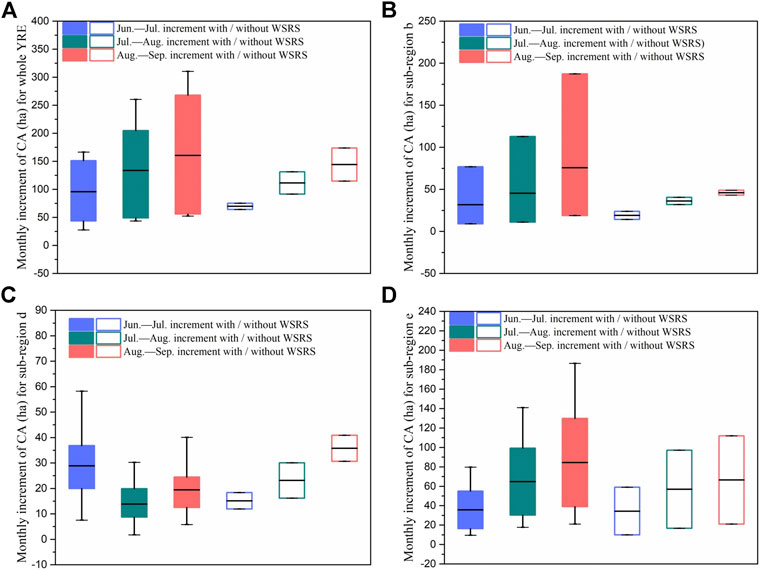
FIGURE 4. Ranges and means of monthly increment of CA (class area) of S. alterniflora for (A) the whole YRE (B) sub-region b (C) sub-region d (D) sub-region e from June to September during 2011 to 2018.
We extended the above analysis to other landscape pattern metrics in sub-region d (Figure 5), and significant differences were also found in the monthly increment trends of NP (number of patches) (p = 0.039), LPI (largest patch index, p = 0.035) and AI (aggregation index, p = 0.027) between the years with and without WSRS. This suggests that the intra-annual growth and colonization behaviors of S. alterniflora in sub-region d are very likely to be affected by the WSRS.
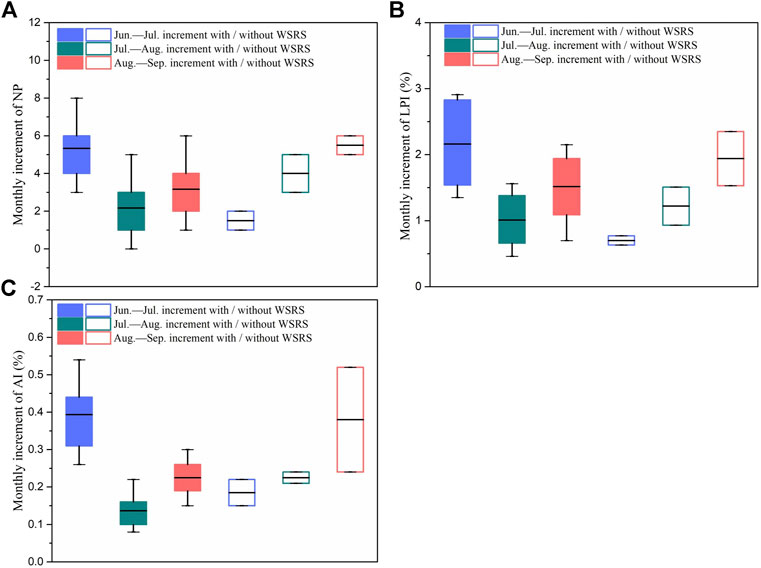
FIGURE 5. Ranges and means of monthly increment of (A) NP (number of patches) (B) LPI (largest patch index) and (C) AI (aggregation index) of S. alterniflora for sub-region d from June to September during 2011 to 2018.
Spartina alterniflora Invasion in Relation to Variation of Flow-Sediment Regime Caused by Water-Sediment Regulation Scheme
Table 4 shows all 15 flow-sediment regime variables during the WSRS at the YRE. F (mean flow) was chosen as the basic variable for ease of interpretability and applicability in calculating the Spearman’s rank correlation coefficients (rs) and variance inflation factors (VIF) for other variables (Richter et al., 1996). Based on the correlation analysis, the variables Ff (rs = 0.64), Tf (rs = 0.27), RS (rs = 0.56) and D (rs = 0.7) were selected to analyze VIF, and the associated VIF values (1.341, 2.643 and 1.933, respectively) all satisfied the criteria set to reduce multicollinearity (< 4). Finally, F (mean flow), Ff (number of high flow pulses), Tf (Julian date of maximum flow) and D (duration of WSRS) were retained after checking the multicollinearity among all explanatory variables using Spearman’s rank correlation coefficients and VIF. In order to assess the influence of WSRS on the S. alterniflora expansion, we analyzed the relationship between the identified key explanatory variables and the increment of the landscape metrics (i.e., CA, NP, LPI and AI) during annual peak growing season of S. alterniflora in the years with WSRS (2011–2015, 2018) in sub-region d (Table 5). In addition, the robustness of optimal GLMs were further tested to ensure the non-accidental character of the results, the DW value (Table 5) and normal probability plots of regression standardized residuals (Figure 6) showed that the obtained optimal models met the robustness requirements.
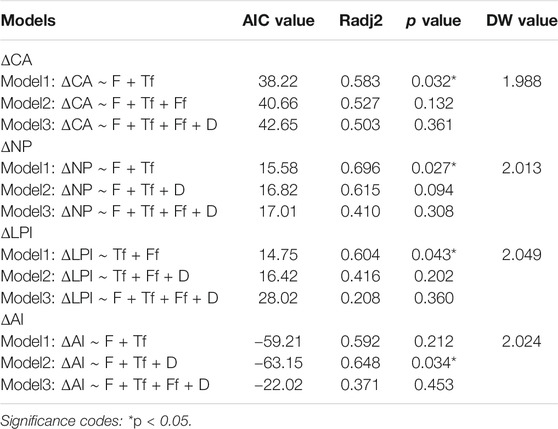
TABLE 5. Comparison and selection of the optimal general linear models (GLMs) analyzing the influences on changes of landscape metrics of S. alterniflora during annual peak growing season.
In addition, we further analyzed the relative contribution of the identified explanatory variables in the optimal GLMs to landscape pattern changes of S. alterniflora (Figure 7; Table 5). Results showed that optimal models explained approximately 58% of CA (class area) change (R2 = 0.583), 70% of NP (number of patches) change (R2 = 0.696), 60% of LPI (largest patch index) change (R2 = 0.604) and 65% of AI (aggregation index) change (R2 = 0.648), suggesting that the identified flow-sediment regime variables were able to explain a large number of changes in intra-annual landscape pattern of S. alterniflora. Specifically, mean flow (F, p = 0.032) was the most important explanatory variable for the change of class area (CA), and Julian date of maximum flow (Tf, p = 0.045) also had significant effect on the change of class area. Similarly, change of number of patches (NP) was mainly affected by mean flow (F, p = 0.012) and Julian date of maximum flow (Tf, p = 0.019). The change of largest patch index (LPI) was mainly affected by number of high flow pulses (Ff, p = 0.033), followed by Julian date of maximum flow (Tf, p = 0.037). As for the aggregation index (AI), the mean flow (F, p = 0.029), Julian date of maximum flow (Tf, p = 0.023) and duration of WSRS (D, p = 0.047) were found to play significant role (Figure 7).
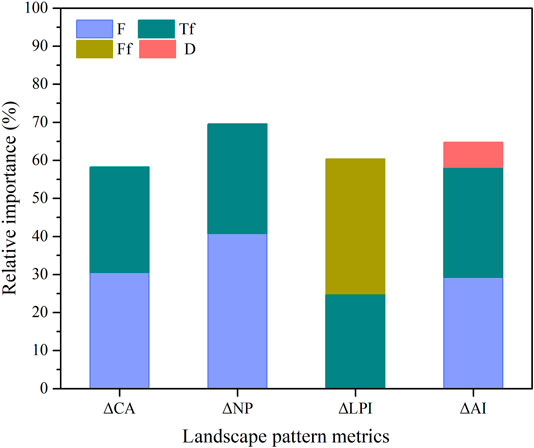
FIGURE 7. The relative contribution of flow-sediment regime variables in the optimal GLMs to landscape pattern changes of S. alterniflora.
Figure 8 further shows the correlation of key flow-sediment regime variables in the optimal GLMs with landscape pattern changes of S. alterniflora. Overall, when the runoff increased, the class area (CA) and aggregation index (AI) significantly increased. However, the number of patches (NP) was significantly lower under high runoff. In addition, when the occurrence time of high pulse was delayed, the number of patches (NP) decreased, whereas the class area (CA), largest patch index (LPI) and aggregation index (AI) of S. alterniflora increased. Furthermore, the aggregation index (AI) decreased with increasing duration of high pulses, and the largest patch index (LPI) decreased with increasing high pulse frequency.
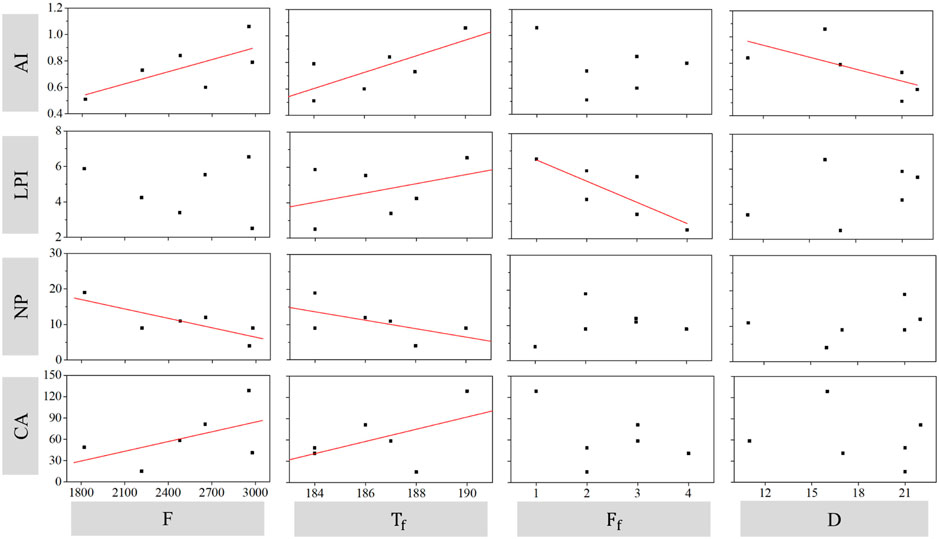
FIGURE 8. The correlation of flow-sediment regime variables with landscape pattern change of S. alterniflora. Regression lines were presented when flow-sediment regime variables are contained in the optimal GLMs.
Discussion
Many studies have analyzed the effects of marine forcings such as tides and waves on saltmarsh plants (Silinski et al., 2016; Carus et al., 2017). Moreover, some studies have shown that frequent hydrodynamic and sedimentation disturbances can restrict the saltmarsh plants growth (Balke et al., 2013; Aguiar et al., 2018). However, a general understanding of whether the landscape patterns of the saltmarsh vegetation are affected by the intra-annual changes of river and sediment discharges is lacking. In this study, our results showed that the variations of estuarine river and sediment discharges caused by an annual hydrological regime regulation operation, i.e., the WSRS, significantly altered intra-annual expansion patterns of S. alterniflora in part of the estuarine saltmarsh close to the mouth (sub-region d) (Figure 4 and Figure 5). Our study further analyzed the relative importance of identified flow-sediment regime variables to the change of intra-annual distribution patterns of S. alterniflora (Figure 7; Table 5), and demonstrated that the increase of high pulse magnitude and the delay of high pulse occurrence time can be beneficial to S. alterniflora invasion, whereas the increase of high pulse frequency and duration can mitigate S. alterniflora invasion (Figure 8).
River regulation such as WSRS results in biogeomorphic variation of estuarine saltmarshes in surface elevation, inundation and salinity (Han et al., 2018; Yang et al., 2020), and the resultant response of plant species to changing environments is complicated (Carle et al., 2015; Snedden et al., 2015). The artificial controls on water and sediment input may cause regime shifts in morphological evolution of estuaries and deltas, such as progradation of delta lobes and crevasse splays vs. avulsions (Nienhuis et al., 2018; Gao et al., 2019), which could further affect estuarine biogeomorphic environments (Xu et al., 2016). In previous studies, Keogh et al. (2019) and Nienhuis et al. (2018) have reported that the crevasse splays were conducive to generation of new land and variations of biogeomorphic conditions in estuaries, due to the water and sediment channeled along the crevasse splays. In sub-region d of the YRE, a crevasse splay was formed and developed since 2011, and S. alterniflora began to spread along the crevasse splay ever since then (Figure 9). The WSRS usually takes place in the initial stage of the peak growing season (June-July) of S. alterniflora, and the additional freshwater and sediment supply channeled along the crevasse splay from the Yellow River into sub-region d during the WSRS may contribute to the enhanced expansion and landscape pattern changes. Notably, Han et al. (2018) reported that compared with the regions only affected by tides, in the regions remained inundated all the time after annual WSRS including sub-region d, the soil salinity and soil moisture were significantly changed after the WSRS. Xie et al. (2019) further demonstrated from field survey that freshwater irrigation was beneficial to the S. alterniflora growth because of the reduced soil salinity and increased moisture content caused by freshwater input.
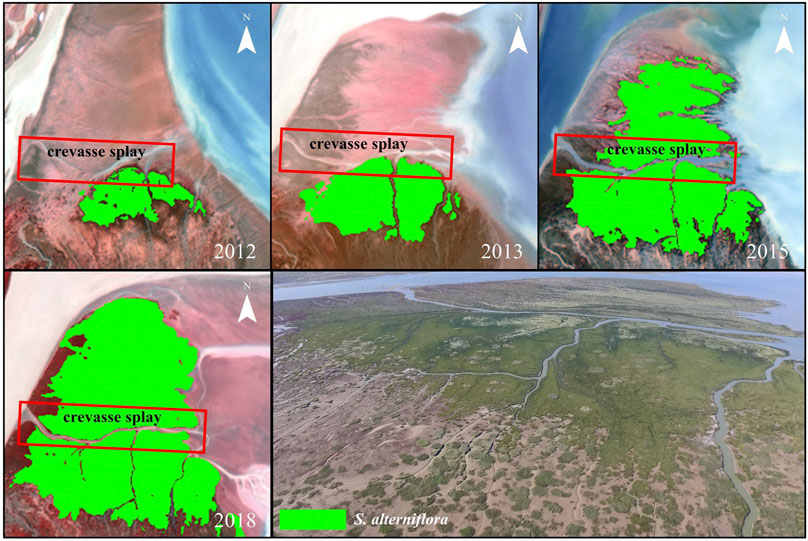
FIGURE 9. Landsat 7/8 remote sensing images acquired in September 24, 2012, September 19, 2013, September 25, 2015 and September 17, 2018, and field observation image acquired in 2018.09 showing S. alterniflora invading along the crevasse splay in sub-region d at the Yellow River Estuary. Photo credit: X. Ma.
On the landscape scale, our results verified that the flow-sediment regime changes caused by WSRS can interfere with the intra-annual expansion process of S. alterniflora in sub-region d. Compared with the years without WSRS (2016–2017), the monthly increment of S. alterniflora landscape metrics (i.e., CA, NP, LPI and AI) were significantly higher in the initial stage of the peak growing season (June-July), which was also the WSRS period, than in the mid- and late stages of the peak growing season (July-September) in the years with WSRS (2011–2015, 2018) (Figures 4, 5). In contrast to sub-region d, there are no crevasse splays to supply additional freshwater and sediment from the Yellow River to saltmarshes during the WSRS in the other sub-regions, which may partly lead to the insignificant effects of the WSRS on the S. alterniflora expansion in these areas.
As for the specific roles of the flow-sediment regime shifts in S. alterniflora invasion, our results shed some light on the relative importance of the identified flow-sediment regime variables (Figures 7, 8). The distribution pattern of S. alterniflora is related to the gradient of the various biogeomorphic factors (e.g., elevation, inundation, salinity etc.), which are subject to physical disturbances such as flooding (Kettenring et al., 2015). Our results show that when the runoff increased, the grow and expansion of S. alterniflora were enhanced and the degree of fragmentation decreased as indicated by the increasing class area (CA) and aggregation index (AI) and decreasing number of patches (NP). This may be attributed to the increased soil moisture and reduced salinity due to the WSRS, which stimulated the S. alterniflora expansion (Han et al., 2018). Furthermore, delayed disturbance was shown to produce more benefits to S. alterniflora growth and expansion through the analysis with the delay of high pulse occurrence time (Figure 8). Notably, Richter et al. (1996) indicated that the timing of extreme flood conditions provides measure of environmental disturbances, which may be intimately linked to the key life-cycle phases of plants, and the degree of physical disturbances (i.e., hydrodynamic forces and associated sedimentation dynamics) defines the lethal thresholds of seedling establishment (Hu et al., 2015; Cao et al., 2018). The initial stage of the peak growing season is the key period of S. alterniflora seedling establishment at the YRE, and the delay of high pulse occurrence time, which created disturbance-free or low-disturbance periods for seedling establishment and recruitment of S. alterniflora, can be beneficial to S. alterniflora growth and expansion (Balke et al., 2011). On the contrary, the effects of the increase in high pulse frequency and duration tend to be negative, e.g., the former led to increased fragmentation as manifested by the decreasing largest patch index (LPI), and the latter led to decreasing aggregation index (AI). This intricate interplay is consistent with the previous findings that the survival and growth of S. alterniflora seedlings were closely related to the life-history-dependent stress tolerance and hence the adaptability of the plant species to external disturbances (Balke et al., 2013; Balke et al., 2014; Bruckner et al., 2019).
Conclusion
Our study explored the effects of the annual Water-Sediment Regulation Scheme on S. alterniflora expansion at the Yellow River Estuary as a case study of the broad question of the effects of flow-sediment regime shift caused by variable river discharge and sediment load on the colonization patterns of estuarine saltmarsh plants. In line with previous studies (Ren et al., 2019), our analysis based on remote sensing images confirmed that the S. alterniflora had a dramatic expansion at the YRE since 2011. The results showed that compared with the years without WSRS (2016–2017), the intra-annual (monthly) increment of S. alterniflora landscape metrics (i.e., CA, NP, LPI and AI) were significantly higher in the initial stage of the peak growing season (June-July) than in the mid- and late stages of the peak growing season (July-September) in the years with WSRS (2011–2015, 2018) in the subregion located close to the south bank of YRE as the most prominent impact zone.
Furthermore, our study identified the key flow-sediment regime variables affecting the intra-annual landscape pattern changes of S. alterniflora, namely, F (mean flow), Ff (number of high flow pulses), Tf (Julian date of maximum flow) and D (duration of WSRS). Our study further analyzed the relative importance of identified flow-sediment regime variables to the change of intra-annual distribution patterns of S. alterniflora, and demonstrated that the increase of high pulse magnitude and the delay of high pulse occurrence time can be beneficial to S. alterniflora invasion, whereas the increase of high pulse frequency and duration can mitigate S. alterniflora invasion. Our results broaden the understanding of estuarine hydrological disturbance as a potential driver regulating the saltmarsh vegetation at the landscape scale, and also have implications for S. alterniflora invasion control at estuaries under changing environment.
It is worth pointing out that there are limited data in the years without WSRS (2016–2017), which may affect the accuracy of statistical results. In addition, S. alterniflora only started spreading in the YRE from 2011, by then the WSRS has been implemented for 9 years. As such, we cannot establish a rigorous control group to compare the inter-annual landscape pattern changes of S. alterniflora before and after the implementation of WSRS. In spite of this, our study divided the entire YRE into five sub-regions based on the sequence of the land formation and invasion (colonization) of the S. alterniflora and explored the expansion characteristics of S. alterniflora in different regions in the years with/without WSRS. Furthermore, our study focused on the change of vegetation landscape patterns through remote sensing image based spatial analysis, and future studies can be extended to examine the underlying mechanisms of the vegetation landscape changes in response to changing environmental factors, such as hydrodynamic disturbance, sediment load and nutrients supply, induced by the WSRS.
Data Availability Statement
The original contributions presented in the study are included in the article/supplementary materials, further inquiries can be directed to the corresponding author.
Author Contributions
Conceptualization, SF and DS; Methodology, SF, LS, and XM; Validation, DS, SZ, and WG; Investigation, SF, AW and XM; Data Curation, SF; Writing—Original Draft Preparation, SF; Writing—Review and Editing, DS; Supervision, DS and TS; Funding Acquisition, TS. All authors have read and agreed to the published version of the manuscript.
Funding
This work was supported by the Joint Funds of the National Natural Science Foundation of China (grant U1806217), the Key Project of National Natural Science Foundation of China (grant 51639001), and the Interdisciplinary Research Funds of Beijing Normal University. WG acknowledges support from the fellowship of China Postdoctoral Science Foundation (grant 2020M680438).
Conflict of Interest
The authors declare that the research was conducted in the absence of any commercial or financial relationships that could be construed as a potential conflict of interest.
References
Aguiar, F. C., Segurado, P., Martins, M. J., Bejarano, M. D., Nilsson, C., Portela, M. M., et al. (2018). The Abundance and Distribution of Guilds of Riparian Woody Plants Change in Response to Land Use and Flow Regulation. J. Appl. Ecol. 55, 2227–2240. doi:10.1111/1365-2664.13110
Aiazzi, B., Baronti, S., Selva, M., and Alparone, L. (2006). “Enhanced Gram-Schmidt Spectral Sharpening Based on Multivariate Regression of MS and Pan Data,” in IEEE International Symposium on Geoscience and Remote Sensing, Denver, CO, July 31–August 4, 2006 (Luciano Alparon), 1–8.
Almeida, D., Rocha, J., Neto, C., and Arsénio, P. (2016). Landscape Metrics Applied to Formerly Reclaimed Saltmarshes: A Tool to Evaluate Ecosystem Services? Estuarine, Coastal Shelf Sci. 181, 100–113. doi:10.1016/j.ecss.2016.08.020
Balke, T., Bouma, T., Horstman, E., Webb, E., Erftemeijer, P., and Herman, P. (2011). Windows of Opportunity: Thresholds to Mangrove Seedling Establishment on Tidal Flats. Mar. Ecol. Prog. Ser. 440, 1–9. doi:10.3354/meps09364
Balke, T., Herman, P. M. J., and Bouma, T. J. (2014). Critical Transitions in Disturbance-Driven Ecosystems: Identifying Windows of Opportunity for Recovery. J. Ecol. 102, 700–708. doi:10.1111/1365-2745.12241
Balke, T., Webb, E. L., den Elzen, E., Galli, D., Herman, P. M. J., and Bouma, T. J. (2013). Seedling Establishment in a Dynamic Sedimentary Environment: a Conceptual Framework Using Mangroves. J. Appl. Ecol. 50, 740–747. doi:10.1111/1365-2664.12067
Bi, N., Sun, Z., Wang, H., Wu, X., Fan, Y., Xu, C., et al. (2019). Response of Channel Scouring and Deposition to the Regulation of Large Reservoirs: A Case Study of the Lower Reaches of the Yellow River (Huanghe). J. Hydrol. 568, 972–984. doi:10.1016/j.jhydrol.2018.11.039
Bi, N., Wang, H., and Yang, Z. (2014). Recent Changes in the Erosion-Accretion Patterns of the Active Huanghe (Yellow River) delta Lobe Caused by Human Activities. Continental Shelf Res. 90, 70–78. doi:10.1016/j.csr.2014.02.014
Brückner, M. Z. M., Schwarz, C., Dijk, W. M., Oorschot, M., Douma, H., and Kleinhans, M. G. (2019). Salt Marsh Establishment and Eco‐Engineering Effects in Dynamic Estuaries Determined by Species Growth and Mortality. J. Geophys. Res. Earth Surf. 124, 2962–2986. doi:10.1029/2019jf005092
Burnham, K. P., and Anderson, D. R. (2004). Multimodel Inference. Sociological Methods Res. 33, 261–304. doi:10.1177/0049124104268644
Cao, H., Zhu, Z., Balke, T., Zhang, L., and Bouma, T. J. (2018). Effects of Sediment Disturbance Regimes on Spartina Seedling Establishment: Implications for Salt Marsh Creation and Restoration. Limnol. Oceanogr. 63, 647–659. doi:10.1002/lno.10657
Carey, J. C., Raposa, K. B., Wigand, C., and Warren, R. S. (2017). Contrasting Decadal-Scale Changes in Elevation and Vegetation in Two Long Island Sound Salt Marshes. Estuaries and Coasts 40, 651–661. doi:10.1007/s12237-015-0059-8
Carle, M. V., Sasser, C. E., and Roberts, H. H. (2015). Accretion and Vegetation Community Change in the Wax Lake Delta Following the Historic 2011 Mississippi River Flood. J. Coastal Res. 313, 569–587. doi:10.2112/jcoastres-d-13-00109.1
Carus, J., Heuner, M., Paul, M., and Schröder, B. (2017). Plant Distribution and Stand Characteristics in Brackish Marshes: Unravelling the Roles of Abiotic Factors and Interspecific Competition. Estuarine, Coastal Shelf Sci. 196, 237–247. doi:10.1016/j.ecss.2017.06.038
Caruso, B. S., Edmondson, L., and Pithie, C. (2013). Braided River Flow and Invasive Vegetation Dynamics in the Southern Alps, New Zealand. Environ. Manage. 52, 1–18. doi:10.1007/s00267-013-0070-4
Dormann, C. F., Elith, J., Bacher, S., Buchmann, C., Carl, G., Carré, G., et al. (2013). Collinearity: a Review of Methods to deal with it and a Simulation Study Evaluating Their Performance. Ecography 36, 27–46. doi:10.1111/j.1600-0587.2012.07348.x
Elsey-Quirk, T., Graham, S. A., Mendelssohn, I. A., Snedden, G., Day, J. W., Twilley, R. R., et al. (2019). Mississippi River Sediment Diversions and Coastal Wetland Sustainability: Synthesis of Responses to Freshwater, Sediment, and Nutrient Inputs. Estuarine, Coastal Shelf Sci. 221, 170–183. doi:10.1016/j.ecss.2019.03.002
Elsey-Quirk, T., Middleton, B. A., and Proffitt, C. E. (2009). Seed Flotation and Germination of Salt Marsh Plants: the Effects of Stratification, Salinity, And/or Inundation Regime. Aquat. Bot. 91, 40–46. doi:10.1016/j.aquabot.2009.02.001
Fox, J., and Weisberg, S. (2018). An R Companion to Applied Regression. Los Angeles: Sage Publications.
Gao, W. L., Shao, D. D., Wang, Z. B., Nardin, W., Yang, W., Sun, T., et al. (2018). Combined Effects of Unsteady River Discharges and Wave Conditions on River Mouth Bar Morphodynamics. Geophys. Res. Lett. 45, 12903–12911. doi:10.1029/2018gl080447
Gao, W., Shao, D., Wang, Z. B., Nardin, W., Rajput, P., Yang, W., et al. (2019). Long‐Term Cumulative Effects of Intra‐Annual Variability of Unsteady River Discharge on the Progradation of Delta Lobes: A Modeling Perspective. J. Geophys. Res. Earth Surf. 124, 960–973. doi:10.1029/2017jf004584
Grömping, U. (2006). Relative Importance for Linear Regression in R: the Package Relaimpo. J. Stat. Softw. 17, 1–27. doi:10.18637/jss.v017.i01
Han, L., Bai, J., Ye, X., Zhang, G., Zhuang, T., and Liu, S. (2018). Effects of Freshwater Restoration on Dynamics of Water and Salt Information in Salt Marsh Soils of the Yellow River Estuary,China. J. Beijing Normal UniversityNatural Sci. 54, 42–47. doi:10.16360/j.cnki.jbnuns.2018.01.006
Hu, M.-Y., Ge, Z.-M., Li, Y.-L., Li, S.-H., Tan, L.-S., Xie, L.-N., et al. (2019). Do short-term Increases in River and Sediment Discharge Determine the Dynamics of Coastal Mudflat and Vegetation in the Yangtze Estuary?. Estuarine, Coastal Shelf Sci. 220, 176–184. doi:10.1016/j.ecss.2019.03.004
Hu, Z., van Belzen, J., van der Wal, D., Balke, T., Wang, Z. B., Stive, M., et al. (2015). Windows of Opportunity for Salt Marsh Vegetation Establishment on Bare Tidal Flats: The Importance of Temporal and Spatial Variability in Hydrodynamic Forcing. J. Geophys. Res. Biogeosci. 120, 1450–1469. doi:10.1002/2014jg002870
Jones, S. F., Yando, E. S., Stagg, C. L., Hall, C. T., and Hester, M. W. (2019). Restoration Affects Sexual Reproductive Capacity in a Salt Marsh. Estuaries and Coasts 42, 976–986. doi:10.1007/s12237-019-00552-y
Kearney, M. S., Riter, J. A., and Turner, R. E. (2011). Freshwater River Diversions for Marsh Restoration in Louisiana: Twenty‐six Years of Changing Vegetative Cover and Marsh Area. Geophys. Res. Lett. 38, 45–50. doi:10.1029/2011gl047847
Kelly, M., Tuxen, K. A., and Stralberg, D. (2011). Mapping Changes to Vegetation Pattern in a Restoring Wetland: Finding Pattern Metrics that Are Consistent across Spatial Scale and Time. Ecol. Indicators 11, 263–273. doi:10.1016/j.ecolind.2010.05.003
Keogh, M. E., Kolker, A. S., Snedden, G. A., and Renfro, A. A. (2019). Hydrodynamic Controls on Sediment Retention in an Emerging Diversion-Fed delta. Geomorphology 332, 100–111. doi:10.1016/j.geomorph.2019.02.008
Keselman, H. J., Algina, J., and Kowalchuk, R. K. (2001). The Analysis of Repeated Measures Designs: a Review. Br. J. Math. Stat. Psychol. 54, 1–20. doi:10.1348/000711001159357
Kettenring, K. M., Whigham, D. F., Hazelton, E. L. G., Gallagher, S. K., and Weiner, H. M. (2015). Biotic Resistance, Disturbance, and Mode of Colonization Impact the Invasion of a Widespread, Introduced Wetland Grass. Ecol. Appl. 25, 466–480. doi:10.1890/14-0434.1
Leitão, A. B., Miller, J., Ahern, J., and McGarigal, K. (2012). Measuring Landscapes: A Planner's Handbook. Washington, DC: Island press.
Li, B., Liao, C.-h., Zhang, X.-d., Chen, H.-l., Wang, Q., Chen, Z.-y., et al. (2009). Spartina Alterniflora Invasions in the Yangtze River Estuary, China: An Overview of Current Status and Ecosystem Effects. Ecol. Eng. 35, 511–520. doi:10.1016/j.ecoleng.2008.05.013
Li, R., Yu, Q., Wang, Y., Wang, Z. B., Gao, S., and Flemming, B. (2018). The Relationship between Inundation Duration and Spartina Alterniflora Growth along the Jiangsu Coast, China. Estuarine, Coastal Shelf Sci. 213, 305–313. doi:10.1016/j.ecss.2018.08.027
Liu, M., Mao, D., Wang, Z., Li, L., Man, W., Jia, M., et al. (2018). Rapid Invasion of Spartina Alterniflora in the Coastal Zone of Mainland China: New Observations from Landsat OLI Images. Remote Sensing 10, 1933. doi:10.3390/rs10121933
Liu, M. Y., Li, H. Y., Li, L., Man, W. D., Jia, M. M., Wang, Z. M., et al. (2017). Monitoring the Invasion of Spartina Alterniflora Using Multi-Source High-Resolution Imagery in the Zhangjiang Estuary, China. Remote Sens. 9, 18. doi:10.3390/rs9060539
Luo, S., Shao, D., Long, W., Liu, Y., Sun, T., and Cui, B. (2018). Assessing 'coastal Squeeze' of Wetlands at the Yellow River Delta in China: A Case Study. Ocean Coastal Manage. 153, 193–202. doi:10.1016/j.ocecoaman.2017.12.018
Mao, D., Liu, M., Wang, Z., Li, L., Man, W., Jia, M., et al. (2019). Rapid Invasion of Spartina Alterniflora in the Coastal Zone of Mainland China: Spatiotemporal Patterns and Human Prevention. Sensors 19. doi:10.3390/s19102308
McGarigal, K., Cushman, S. A., Neel, M. C., and Ene, E. (2002). FRAGSTATS: Spatial Pattern Analysis Program for Categorical Maps. Amherst: University of Massachusetts.
McGarigal, K. (2014). Landscape Pattern Metrics. Washington, DC: Wiley StatsRef: Statistics Reference Online. doi:10.1002/9781118445112.stat07723
Mortenson, S. G., and Weisberg, P. J. (2010). Does River Regulation Increase the Dominance of Invasive Woody Species in Riparian Landscapes?. Glob. Ecol. Biogeogr. 19, 562–574. doi:10.1111/j.1466-8238.2010.00533.x
Mountrakis, G., Im, J., and Ogole, C. (2011). Support Vector Machines in Remote Sensing: A Review. Isprs J. Photogrammetry Remote Sens. 66, 247–259. doi:10.1016/j.isprsjprs.2010.11.001
Nienhuis, J. H., Törnqvist, T. E., and Esposito, C. R. (2018). Crevasse Splays versus Avulsions: A Recipe for Land Building with Levee Breaches. Geophys. Res. Lett. 45, 4058–4067. doi:10.1029/2018gl077933
Olliver, E. A., and Edmonds, D. A. (2017). Defining the Ecogeomorphic Succession of Land Building for Freshwater, Intertidal Wetlands in Wax Lake Delta, Louisiana. Estuarine, Coastal Shelf Sci. 196, 45–57. doi:10.1016/j.ecss.2017.06.009
Penda, S., Djellout, H., and Proïa, F. (2012). Moderate Deviations for the Durbin-Watson Statistic Related to the First-Order Autoregressive Process. Esaim Probab. Stat. 18, 308–331. doi:10.1051/ps/2013038
Poff, N. L., Allan, J. D., Bain, M. B., Karr, J. R., Prestegaard, K. L., Richter, B. D., et al. (1997). The Natural Flow Regime. Bioscience 47, 769–784. doi:10.2307/1313099
Poormahdi, S., Graham, S. A., and Mendelssohn, I. A. (2018). Wetland Plant Community Responses to the Interactive Effects of Simulated Nutrient and Sediment Loading: Implications for Coastal Restoration Using Mississippi River Diversions. Estuaries and Coasts 41, 1679–1698. doi:10.1007/s12237-018-0390-y
Qiu, S., Liu, S., Wei, S., Cui, X., Nie, M., Huang, J., et al. (2020). Changes in Multiple Environmental Factors Additively Enhance the Dominance of an Exotic Plant with a Novel Trade‐off Pattern. J. Ecol. 108, 1989–1999. doi:10.1111/1365-2745.13386
R CoreTeam (2017). R: A Language and Environment for Statistical Com‐puting. Vienna, Austria: R Foundation for Statistical Computing.
Ren, G., Liu, Y., Ma, Y., and Zhang, J. (2014). Spartina Alterniflora Monitoring and Change Analysis in Yellow River Delta by Remote Sensing Technology. Acta Laser Biol. Sin. 23, 596.
Ren, G.-B., Wang, J.-J., Wang, A.-D., Wang, J.-B., Zhu, Y.-L., Wu, P.-Q., et al. (2019). Monitoring the Invasion of Smooth Cordgrass Spartina Alterniflora within the Modern Yellow River Delta Using Remote Sensing. J. Coastal Res. 90, 135–145. doi:10.2112/si90-017.1
Richter, B. D., Baumgartner, J. V., Powell, J., and Braun, D. P. (1996). A Method for Assessing Hydrologic Alteration within Ecosystems. Conservation Biol. 10, 1163–1174. doi:10.1046/j.1523-1739.1996.10041163.x
Schwarz, C., Ysebaert, T., Vandenbruwaene, W., Temmerman, S., Zhang, L., and Herman, P. M. J. (2016). On the Potential of Plant Species Invasion Influencing Bio-Geomorphologic Landscape Formation in Salt Marshes. Earth Surf. Process. Landforms 41, 2047–2057. doi:10.1002/esp.3971
Seabloom, E. W., Moloney, K. A., and Van der Valk, A. G. (2001). Constraints on the Establishment of Plants along a Fluctuating Water-Depth Gradient. Ecology 82, 2216–2232. doi:10.1890/0012-9658(2001)082[2216:coteop]2.0.co;2
Shen, Q., and Ma, Y. (2020). Did Water Diversion Projects lead to Sustainable Ecological Restoration in Arid Endorheic Basins? Lessons from Long-Term Changes of Multiple Ecosystem Indicators in the Lower Heihe River Basin. Sci. Total Environ. 701, 11. doi:10.1016/j.scitotenv.2019.134785
Silinski, A., Heuner, M., Troch, P., Puijalon, S., Bouma, T. J., Schoelynck, J., et al. (2016). Effects of Contrasting Wave Conditions on Scour and Drag on pioneer Tidal Marsh Plants. Geomorphology 255, 49–62. doi:10.1016/j.geomorph.2015.11.021
Snedden, G. A., Cretini, K., and Patton, B. (2015). Inundation and Salinity Impacts to above- and Belowground Productivity in Spartina Patens and Spartina Alterniflora in the Mississippi River Deltaic plain: Implications for Using River Diversions as Restoration Tools. Ecol. Eng. 81, 133–139. doi:10.1016/j.ecoleng.2015.04.035
Song, H., Liu, X., Yu, W., and Wang, L. (2018). Seasonal and Spatial Variation of Nitrogen Oxide Fluxes from Human-Disturbance Coastal Wetland in the Yellow River Estuary. Wetlands 38, 945–955. doi:10.1007/s13157-018-1043-4
Walker, J., de Beurs, K., and Wynne, R. (2015). Phenological Response of an Arizona Dryland forest to Short-Term Climatic Extremes. Remote Sens. 7, 10832–10855. doi:10.3390/rs70810832
Wang, H., Bi, N., Saito, Y., Wang, Y., Sun, X., Zhang, J., et al. (2010). Recent Changes in Sediment Delivery by the Huanghe (Yellow River) to the Sea: Causes and Environmental Implications in its Estuary. J. Hydrol. 391, 302–313. doi:10.1016/j.jhydrol.2010.07.030
Wang, H., Wu, X., Bi, N., Li, S., Yuan, P., Wang, A., et al. (2017). Impacts of the Dam-Orientated Water-Sediment Regulation Scheme on the Lower Reaches and delta of the Yellow River (Huanghe): A Review. Glob. Planet. Change 157, 93–113. doi:10.1016/j.gloplacha.2017.08.005
Winter, J. D. (2013). Using the Student's T-Test with Extremely Small Sample Sizes. Pract. Assess. Res. Eval. 18, 12. doi:10.4121/UUID:7BA3F2CB-7FF6-4531-8AE4-D44462DADEF5
Wisniak, J., and Polishuk, A. (1999). Analysis of Residuals - a Useful Tool for Phase Equilibrium Data Analysis. Fluid Phase Equilibria 164, 61–82. doi:10.1016/s0378-3812(99)00246-0
Wohl, E., Bledsoe, B. P., Jacobson, R. B., Poff, N. L., Rathburn, S. L., Walters, D. M., et al. (2015). The Natural Sediment Regime in Rivers: Broadening the Foundation for Ecosystem Management. Bioscience 65, 358–371. doi:10.1093/biosci/biv002
Xie, T., Cui, B., Li, S., and Zhang, S. (2019). Management of Soil Thresholds for Seedling Emergence to Re-establish Plant Species on Bare Flats in Coastal Salt Marshes. Hydrobiologia 827, 51–63. doi:10.1007/s10750-018-3589-9
Xu, B., Yang, D., Burnett, W. C., Ran, X., Yu, Z., Gao, M., et al. (2016). Artificial Water Sediment Regulation Scheme Influences Morphology, Hydrodynamics and Nutrient Behavior in the Yellow River Estuary. J. Hydrol. 539, 102–112. doi:10.1016/j.jhydrol.2016.05.024
Xu, C., and Li, Y. (2019). Effect of Flow-Sediment Regime on Benthic Invertebrate Communities: Long-Term Analysis in a Regulated Floodplain lake. Sci. Total Environ. 649, 201–211. doi:10.1016/j.scitotenv.2018.08.308
Yang, H., Zhang, X. Y., Cai, H. Y., Hu, Q., Liu, F., and Yang, Q. S. (2020). Seasonal Changes in River-Tide Dynamics in a Highly Human-Modified Estuary: Modaomen Estuary Case Study. Mar. Geology 427, 11. doi:10.1016/j.margeo.2020.106273
Keywords: saltmarsh, Spartina alterniflora invasion, landscape patterns, remote sensing, Yellow River Estuary, water-sediment regulation scheme
Citation: Fu S, Zheng S, Gao W, Wang A, Ma X, Sun L, Sun T and Shao D (2021) Effects of the Water-Sediment Regulation Scheme on the Expansion of Spartina alterniflora at the Yellow River Estuary, China. Front. Environ. Sci. 9:642442. doi: 10.3389/fenvs.2021.642442
Received: 16 December 2020; Accepted: 11 June 2021;
Published: 28 June 2021.
Edited by:
Ligang Xu, Nanjing Institute of Geography and Limnology (CAS), ChinaReviewed by:
Elzbieta Antczak, University of Łódź, PolandNaishuang Bi, Ocean University of China, China
Copyright © 2021 Fu, Zheng, Gao, Wang, Ma, Sun, Sun and Shao. This is an open-access article distributed under the terms of the Creative Commons Attribution License (CC BY). The use, distribution or reproduction in other forums is permitted, provided the original author(s) and the copyright owner(s) are credited and that the original publication in this journal is cited, in accordance with accepted academic practice. No use, distribution or reproduction is permitted which does not comply with these terms.
*Correspondence: Dongdong Shao, ZGRzaGFvQGJudS5lZHUuY24=