- 1Biosphere 2, University of Arizona, Oracle, AZ, United States
- 2BIO5 Institute, University of Arizona, Tucson, AZ, United States
- 3Department of Environmental Science, University of Arizona, Tucson, AZ, United States
- 4Aerodyne Research, Inc., Billerica, MA, United States
- 5School of Natural Resources and the Environment, University of Arizona, Tucson, AZ, United States
Soils harbor complex biological processes intertwined with metabolic inputs from microbes and plants. Measuring the soil metabolome can reveal active metabolic pathways, providing insight into the presence of specific organisms and ecological interactions. A subset of the metabolome is volatile; however, current soil studies rarely consider volatile organic compounds (VOCs), contributing to biases in sample processing and metabolomic analytical techniques. Therefore, we hypothesize that overall, the volatility of detected compounds measured using current metabolomic analytical techniques will be lower than undetected compounds, a reflection of missed VOCs. To illustrate this, we examined a peatland metabolomic dataset collected using three common metabolomic analytical techniques: nuclear magnetic resonance (NMR), gas chromatography-mass spectroscopy (GC-MS), and fourier-transform ion cyclotron resonance mass spectrometry (FT-ICR-MS). We mapped the compounds to three metabolic pathways (monoterpenoid biosynthesis, diterpenoid biosynthesis, and polycyclic aromatic hydrocarbon degradation), chosen for their activity in peatland ecosystems and involvement of VOCs. We estimated the volatility of the compounds by calculating relative volatility indices (RVIs), and as hypothesized, the average RVI of undetected compounds within each of our focal pathways was higher than detected compounds (p < 0.001). Moreover, higher RVI compounds were absent even in sub-pathways where lower RVI compounds were observed. Our findings suggest that typical soil metabolomic analytical techniques may overlook VOCs and leave missing links in metabolic pathways. To more completely represent the volatile fraction of the soil metabolome, we suggest that environmental scientists take into consideration these biases when designing and interpreting their data and/or add direct online measurement methods that capture the integral role of VOCs in soil systems.
Introduction
As a complex and heterogeneous ecosystem, soil harbors a myriad of biological processes that are challenging to uncover. Two major contributors to biological soil processes are microbial communities and plant roots. Soil microbial communities are engines of chemical interconversion—microbes produce and consume chemical substrates for metabolism, generating metabolites as waste by-products. Tightly interwoven with the soil microbial network, plant roots also emit metabolites as exudates (Bertin et al., 2003; Vives-Peris et al., 2020). These diverse metabolites form the metabolome, which can be interrogated to provide insight into belowground biological processes. Volatile metabolites, constituting the volatilome (Amann et al., 2014), represent a part of the comprehensive metabolome. Volatile organic compounds (VOCs) have high vapor pressures causing them to enter the gaseous phase depending on environmental conditions. While atmospheric chemists routinely measure VOCs directly using specialized techniques, VOCs are rarely considered in the burgeoning collection of soil microbial metabolomic studies. Could removing bias in sample processing and including more direct measurements of VOCs help fill in a missing, important part of the soil metabolome?
Challenges inherent to most approaches evaluating soil organic matter composition and soil metabolomics make it difficult to simultaneously detect VOCs. First, soil metabolomic field studies start with collecting soil samples to bring back to the lab for sample processing and metabolic measurements. These ex-situ methods overlook VOCs that escape to the atmosphere either prior to sample collection or during disruptive soil sampling (Hewitt, 1996). This artifact may even affect VOC-resolving techniques, such as gas chromatography-mass spectrometry (GC-MS), where VOC loss to the atmosphere prior to sample analysis is a possibility (Eriksson et al., 2001). Second, some metabolomics measurement techniques require an initial liquid chromatography (LC) step, as is the case with LC-mass spectrometry (LC-MS) to characterize compound structure. This process immediately limits the detectable VOCs to those dissolved in the liquid phase. The solvent used to prepare samples in the lab for LC and other direct injection methods can also bias the extracted metabolites from soil samples; methanol preferentially extracts semipolar to nonpolar molecules, whereas water extracts polar molecules (Hollywood et al., 2006). Furthermore, soluble VOCs may partition between the gas and liquid phases making quantification unreliable, while insoluble VOCs will go undetected. Finally, inherent biases, including target size of compounds and ionization mode (positive vs negative), exist for each of the most widely used metabolomic analysis techniques (Table 1). Some of these challenges to measuring the soil volatilome may be addressed by adapting online measurement methods from atmospheric chemistry that directly measure VOCs in the gas phase such as proton-transfer-reaction time of flight MS (PTR-TOF-MS). Increasingly, the soil volatilome is being characterized in its own right using these approaches, either by measuring gases at the soil-atmosphere surface (e.g., using soil incubation chambers [Asensio et al., 2007]), and recently with belowground diffusive probes to measure VOCs in situ (Gil-Loaiza et al., 2020).
VOCs also play important ecological roles in soil and influence atmospheric chemistry. Unlike their non-volatile counterparts, VOCs readily exchange between the soil and atmosphere. In the atmosphere, VOCs are active in photochemical reactions and secondary aerosol formation, and thereby affect air quality (Chameides et al., 1988; Park et al., 2013; Ghirardo et al., 2020), climate (Müller et al., 2017), and precipitation dynamics (Zhao et al., 2016). In the soil, VOCs are important signaling molecules that drive microbe-microbe, plant-microbe, and plant-plant interactions (Penuelas et al., 2014). Specifically, like non-volatile metabolites, microbial VOCs can promote plant growth (Tahir et al., 2017; Tyagi et al., 2018) and plant-root VOCs can either deter or attract microbes (Bitas et al., 2013). But unlike non-volatile metabolites, VOCs diffuse through soil more readily, extending their zone of influence. For example, while the rhizosphere zone influenced by root exudates may be restricted to millimeter-scales for non-volatile compounds, VOCs may diffuse centimeters or farther from roots, thereby extending the reach of the effective rhizosphere (de la Porte et al., 2020). These examples emphasize some of the unique roles of VOCs in the soil and signify that capturing VOCs within the complete soil metabolome is important for resolving belowground processes and aboveground interactions.
Despite the important roles of soil VOCs, we argue that VOCs have often been overlooked in soil metabolomic studies. These studies typically map metabolites to metabolic pathways to guide expectations for specific biological processes. However, metabolite volatility is rarely considered, even in cases where standard metabolomic analytical techniques capture VOCs. In fact, the volatility of many soil organic compounds is unknown, confounding our current understanding of whether VOCs are underrepresented. Despite a lack of measurements of the volatility of organic compounds, tools that predict volatility from molecular functional groups (Hilal et al., 2007; Nannoolal et al., 2008; Pankow and Asher, 2008) have been widely adopted by the atmospheric chemistry community.
Here, we integrate disciplinary approaches to predict metabolite volatility in three VOC-containing metabolic pathways. We show that compounds with high volatility are disproportionately undetected in a peatland metabolomic dataset derived from three techniques (GC-MS, Fourier-transform ion cyclotron resonance MS [FTICR-MS] by direct injection, and nuclear magnetic resonance [NMR]). The peatland ecosystem is ideal for evaluating the representation of VOCs in metabolomics because we expect high quantities of VOCs (Seewald et al., 2010) as fermentation products of anaerobic metabolism in water-logged, anoxic conditions. This approach establishes a baseline understanding of the soil volatilome and the implications of its underrepresentation in current soil metabolomics studies.
Concept
To predict the volatility of metabolites along metabolic pathways, we adapted tools used to estimate VOC partitioning between the gaseous and condensed aerosol phases under the assumption of standard conditions (i.e., temperature and pressure) and dry sorbent material. Detailed discussions of properties of and factors affecting volatility have been well described elsewhere (Hilal et al., 2003; Nannoolal et al., 2008; Compernolle et al., 2011; Tang et al., 2019). We calculated metabolite vapor pressure (P; atm) using SIMPOL.1 (Pankow and Asher, 2008), which accounts for the impact of functional groups. Specifically, we estimated vapor pressure using the following equation:
for functional groups k = 1, 2, 3…, where b0 is a constant, bk is the functional group contribution term for group k, and v is the number of groups of type k in the compound. For example, hydroxyl (−OH), carboxylic acid (−C[O]OH), ketone (O = O), carbon double bond (C = C), and aromatic ring functional groups each decrease P to a different degree. The method did not specify the impact of phosphate groups, which are common in metabolic pathways, so we used nitrate as a proxy with the assumption that these functional groups have a similar relative contribution to P (Nannoolal et al., 2008).
The volatility of VOCs in a given environment can be expressed by the tendency to partition to the gas vs condensed phase. In the atmosphere, VOC partitioning (ξ) to the gas phase increases with vapor pressure (C∗), which is by convention converted using the ideal gas law to mass-based saturation vapor concentration (μg m–3) that accounts for molecular mass (log10C∗ = log10(PM/RT), where M is molecular mass, R the universal gas constant, and T temperature). Under clean atmospheric conditions, thresholds for nonvolatile, intermediate volatility, and volatile are on the order C∗ = 0.01 μg m–3, 1 μg m–3, and 100 μg m–3, respectively, or more conveniently on a log scale: log10C∗ = −2, 0, and 2 (Donahue et al., 2006). VOC partitioning also depends on the total availability of condensed-phase organic molecules (e.g., total aerosol; CTotal). The same VOC will appear less ‘volatile’ in a polluted atmosphere with greater partitioning on high aerosol loadings [ξi = 1/(1+Ci∗/CTotal)]. Soil contains large quantities of organic matter, but theories linking soil CTotal to thresholds for volatility in the subsurface have not been established. Here, we therefore report a relative volatility index (RVI) using log10C∗ (RVI = log10C∗) as the volatility scale, with the understanding that gas phase partitioning will be dependent upon the environment in soil pores (temperature, moisture, pressure) or at the soil-atmosphere interface. While the RVI does not give an absolute indication of whether a compound is volatile in the soil, it can be used to compare compound volatilities relative to one another.
To provide a reference framework for assessing the extent of detected volatiles, we used metabolic pathway maps that visualize metabolic reactions and their intermediates. Metabolic pathway maps, such as those in the KEGG metabolic database (Kanehisa and Goto, 2000), help soil scientists visualize active soil processes. To illustrate our concept, we assessed a peatland soil metabolomic dataset (generated using typical soil metabolomic analytical techniques that first extracted metabolites from soil (Folch et al., 1957) for analysis by FTICR-MS, 1H NMR, and GC-MS (Wilson et al., 2021) (methods included in Table 1). While FTICR-MS provides compound masses that can be used to predict formulae, 1H NMR, and GC-MS provides m/z values that must be matched to compounds in reference databases. For all metabolite analysis, previously reported methods, standards for peak picking, compound databases, and formula assignment were used (e.g., Weljie et al., 2006; Hiller et al., 2009; Kind et al., 2009; Tolicì et al., 2017; Tfaily et al., 2018).
All metabolomic data were combined, matched to KEGG compound IDs when possible, and mapped to pathways using the KEGG Pathway Mapper (Kanehisa and Sato, 2020). We note that some formulas matched to more than one isomer or compound, and all matches were included in this analysis. We selected three VOC-containing pathways that we expect to be present in peatland ecosystems: (1) monoterpenoid biosynthesis (Mono Bio), describing the formation of monoterpenes which are highly volatile; (2) diterpenoid biosynthesis (Di Bio), describing the formation of diterpenes which include many non-volatile compounds with a few exceptions; and (3) polycyclic aromatic hydrocarbon degradation (PAH Deg), describing the breakdown of hydrocarbons and including many semi-volatile compounds. Along these pathways, we calculated average RVIs for detected and undetected compounds in the peatland dataset and tested for significant differences using non-parametric Mann Whitney U-test.
Overall Patterns
In all our focal pathways, most compounds were detected using FTICR-MS, with only phthalate detected by GC-MS and catechol by NMR (both from the PAH Deg pathway). All these pathways contain mostly secondary metabolites, compounds that tend to exceed the detectable target range of NMR (Table 1). NMR is well suited to measure smaller molecules that are in higher abundance, particularly primary metabolites, some of which are VOCs (i.e., catechol, methanol, and acetone). Indeed, the average RVI of NMR-detected compounds across all three pathways was +2.1 ± 7.2. Therefore, NMR may be a good option for detection of small, primary VOC metabolites, however, the technique suffers from biases that may preclude detection of many VOCs (Table 1). On the other hand, based on the RVIs of the compounds in our focal pathways, the GC-MS and FTICR-MS standard soil metabolomic analysis techniques were better suited to detect compounds with lower volatility.
Biosynthesis Pathways
Diterpenoid Biosynthesis
Diterpenoids are a class of molecular compounds containing four joined isoprene (C5H8) units that include momilactones, oryzalexins, gibberellins, and kaurenes. The capacity to biosynthesize diterpenoids is present in plants, fungi, and select bacteria (Gutiérrez-Mañero et al., 2001; Zi et al., 2014; Tang et al., 2015). The roles of diterpenoids in plants are diverse and include pathogen defense, plant growth effectors, signaling, and abiotic stress responses (Lu et al., 2018; Murphy and Zerbe, 2020). Fungi and bacteria also produce diterpenoids as antimicrobial agents and plant growth promoters (de Boer and de Vries-van Leeuwen, 2012; Zhao et al., 2018).
We elaborated on the Di Bio pathway because a high proportion of metabolites were detected in the peatland dataset (40.8%; Figure 1A), strongly suggesting that this metabolism was active in the peatland. Previous studies in peatland ecosystems examining VOC emissions in situ (PTR-TOF) also detected diterpenes (Li et al., 2020), signifying the presence of volatile diterpenes in peatlands. In our peatland dataset, the average RVI of undetected compounds was significantly higher than those detected (RVIundetected = −0.97 ± 5.7 vs RVIdetected = −4.6 ± 2.8, p < 0.0001; Figure 1A). After removing five undetected outliers with extremely low RVIs (−14, −16, −19, −21, and −26), RVIundetected = +0.04 ± 3.4. This indicates a preference for detection of compounds with lower volatilities by standard metabolomic methods.
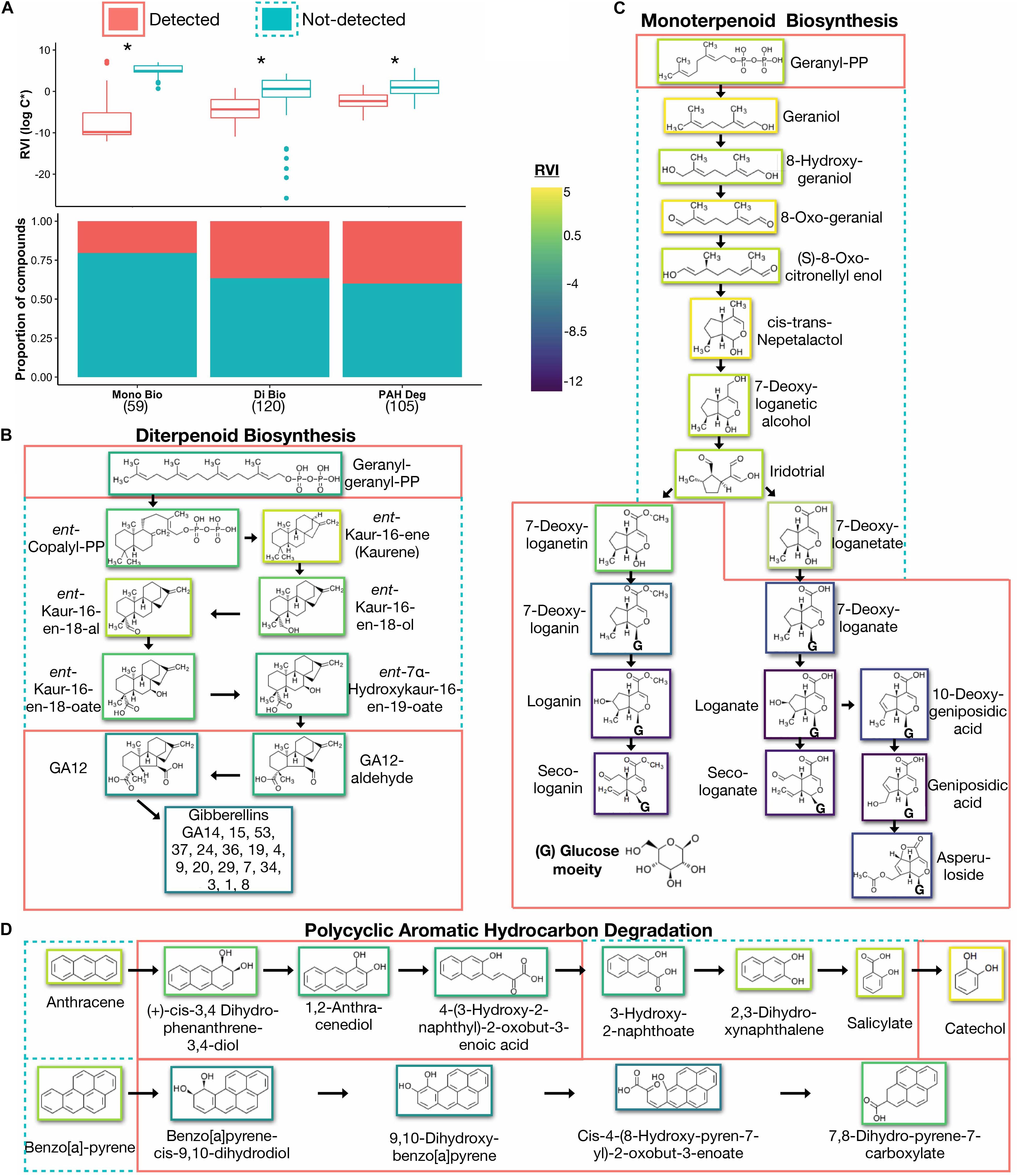
Figure 1. (A) Proportion of detected and undetected compounds across selected VOC-containing pathways (bottom) with average volatilities (top). Asterisk(*) denotes significant differences between volatility (RVI) of detected vs not-detected compounds (Mann Whitney U-test, p < 0.0001). Total number of compounds in each pathway is shown in parentheses. (B) Gibberellin biosynthesis sub-pathway within the Di Bio pathway. (C) Iridoid biosynthesis subpathway within the Mono Bio pathway. (D) Anthracene and Benzo[a]pyrene degradation subpathways within the PAH Deg pathway. Dotted outlines highlight undetected compounds and solid lines detected compounds within the subpathways. The color surrounding each compound represents the volatility (RVI). Mono Bio, monoterpenoid biosynthesis; Di Bio, diterpenoid biosynthesis; PAH Deg, polycyclic aromatic hydrocarbon degradation; RVI, relative volatility index.
A closer examination of the gibberellin biosynthesis sub pathway within the Di Bio pathway shows that almost all of the gibberellins were detected in the peatland dataset; however, there were a series of undetected intermediate compounds on the pathway to their production (Figure 1B). Gibberellins are plant hormones that promote growth and root elongation (Tanimoto, 2005), and even some plant growth promoting bacteria can also produce gibberellins (Gutiérrez-Mañero et al., 2001; Bottini et al., 2004). Within this sub pathway, five compounds between ent-Copalyl-PP and GA12-aldehyde were undetected (Figure 1B) and, furthermore, had higher average RVIs than the detected compounds (RVIundetected = +0.34 ± 2.2 vs RVIdetected = −5.4 ± 2.1, p < 0.0001). To support the SIMPOL.1 prediction that these undetected kaurene compounds had high volatility, previous research has found kaurene to be volatile and emitted by plant flowers, stems, leaves, and roots (Yáñez-Serrano et al., 2018). The capacity to synthesize kaurene compounds within this sub pathway is shared between plants, fungi, and bacteria (Salazar-Cerezo et al., 2018). Therefore, kaurene should be produced regardless of source, but may remain undetected due to its higher volatility.
Monoterpenoid Biosynthesis
Monoterpenoids are molecules with two joined isoprene units that include α-pinene, linalool, camphor, and iridoids. Plants are the primary producers of monoterpenoids, and there are very few reports of bacteria or fungi capable of this synthesis (Penuelas et al., 2014). It is well established that plants emit monoterpenes from flowers to attract pollinators (Barragán-Fonseca et al., 2020) and from their roots to attract beneficial microbes and small eukaryotes like nematodes (Ali et al., 2011). This release of monoterpenes also deters pathogens using their antimicrobial and anti-fungicidal attributes (Lee et al., 2016; Reis et al., 2016).
We focused on the Mono Bio pathway map because, unlike diterpenes, most monoterpenes are volatile, and we therefore expected fewer compounds from this pathway to be present in our peatland dataset. Indeed, we detected only 20% of the compounds in the Mono Bio pathway (Figure 1A). As expected, the overall RVIs were higher in the Mono Bio than the Di Bio pathway, signifying a higher overall volatility of the monoterpene compounds (+2.7 vs. −1.6, respectively). Similar to the Di Bio pathway, the average volatility of the compounds detected were significantly lower than those undetected (RVIundetected = +5.4 vs. RVIdetected = −1.3, p < 0.001).
The sub pathway to iridoid compound biosynthesis contained a majority of the detected compounds including loganin, secologanin, and laganate (Figure 1C). These detected iridoids had a lower volatility than the seven missing compound intermediates stemming from Gereanyl-PP (RVIundetected = +2.79 vs. RVIdetected = −8.94, p < 0.001; Figure 1C). This represents another example of intermediate metabolites that should be present in the soil but are absent from the dataset and have a significantly higher volatility.
Degradation Pathway
Polycyclic Aromatic Hydrocarbon Degradation
Polycyclic aromatic hydrocarbons (PAHs), a class of chemicals with two or more benzene rings fused together, occur naturally in coal, oil, and gasoline or can be produced through the incomplete combustion of these biomasses. PAHs in soils are often from anthropogenic sources as fossil fuel combustion creates atmospheric emissions that deposit on land (Malawska et al., 2006), but natural PAHs can also form, for example, from microbial decomposition of plant residues in aeration-exposed peatlands that go through seasonal thaws (Gabov et al., 2020). Soils, particularly peatlands, are the main reservoir for PAHs in the environment (Wilcke and Amelung, 2000) where PAHs are persistent and difficult to break-down due to their hydrophobic properties causing them to strongly bind to soil particles (Gabov et al., 2020). Some bacteria (Déziel et al., 1996; Ghosal et al., 2016) and fungi (Hammel, 1995; Kadri et al., 2017) are capable of PAH degradation, however, in peatlands, PAHs accumulate at fast rates due to low degradation rates in the highly anaerobic conditions with high organic content. Yet, some peatlands are capable of higher degradation rates; for example, Ledum peatlands can degrade PAHs at a greater rate than Sphagnum peatlands (Wang et al., 2019).
We focused on the PAH Deg pathway because, as expected, our peatland dataset contained a high number of compounds this pathway (40%, Figure 1A). Furthermore, PAHs and their degradation products are semi-volatile (Ghosal et al., 2016), therefore, have the potential to be missed using standard metabolomic techniques. Consistent with our above findings, the average volatility of the detected compounds was significantly lower than those that were undetected (RVIundetected = +0.88 ± 2.4 vs RVIdetected = −2.2 ± 2.1, p < 0.001).
Compounds from sub-pathways within the PAH Deg pathway were detected in the peatland dataset to different degrees. From the benzo[a]pyrene degradation sub-pathway, almost all compounds were detected, except for benzo[a]pyrene itself. Benzo[a]pyrene is the largest PAH with six rings, and the compounds from this sub pathway had the lowest volatilities overall (average RVI = −3.5 ± 2.3). In contrast, compounds from the other three sub-pathways for pyrene, anthracene, phenanthrene, and fluorene have more patchy detection, but also higher RVIs on average. For example, anthracene, a PAH with three rings, and its degradation products have on average RVI of +0.20 ± 2.6 (for comparison of Benzo[a]pyrene and anthracene degradation see Figure 1D).
Conclusion
Here, we provide compelling evidence that typical soil metabolomic analytical techniques miss some soil VOCs and therefore underestimate the role of the volatilome in the total soil metabolome and make it difficult to conclude which pathways are active. We showed that compounds undetected in a peatland dataset had significantly higher estimated volatilities than those detected within the context of three important VOC-containing metabolic pathway. There are several reasons that a compound could be undetected, including low steady state concentrations, chemical instability, short lifetime, and fast metabolism. While these other processes could be affecting compound detectability, we argue that non-volatile compounds are just as susceptible as volatile compounds, and, therefore, do not affect our conclusions. Given the plethora of known and currently unknown metabolic pathways in soil, these results only begin to unearth the potential for a missing volatilome in current soil metabolomic research projects.
Already, researchers often use more than one measurement method because no single technique can capture all metabolites in a sample. While existing techniques can be tuned to target specific compounds, there is currently no global method that can provide molecular identification of all chemicals in a system at high time resolution. Each technique is specialized to target different sizes and classes of compounds and comes with its own biases depending on how samples were processed and data analyzed (Table 1). Furthermore, biases inherent in sample collection, extraction, and measurement can compound, therefore, the methodology for each step should be carefully considered. Adjustments in sample processing (i.e., collecting samples in air-tight containers or capturing VOCs in adsorptive cartridges) and selection of analysis methods could help gear soil metabolomic measurement techniques to capture more VOCs. Additionally, in situ VOC measuring techniques, such as PTR-TOF-MS or GC-CI-MS (Table 1), could be added to the soil metabolomic repertoire to directly target volatile metabolites. Moreover, online VOC analytical techniques yield faster measurements than FT-ICR-MS, GC/LC-EI-MS, and NMR, with near continuous (1Hz) data for uninterrupted analysis by PTR-TOF-MS, and longer sample measurement time for GC-CI-MS, but with greater structural information. New efforts to integrate VOC measuring techniques to capture and characterize the complete soil metabolome will provide a deeper understanding of the complex biological processes occurring belowground.
Data Availability Statement
Publicly available datasets were analyzed in this study. This data can be found here: SPRUCE long-term repository (doi: https://doi.org/10.25581/spruce.083/1647173).
Author Contributions
LM, LH, JK, and MT conceived of the concept and approach. LH and LM wrote the manuscript. MT provided peatland dataset. LH curated the data and chose metabolic pathways. KG and LH calculated compound RVIs. JK contributed his expertise in volatility predictive models. LH produced the figure. All authors contributed to editing the manuscript.
Funding
LH was supported by Biosphere 2 through the office of the Senior Vice President for Research Innovation and Impact at the University of Arizona. This material is based upon work supported by the National Science Foundation under Grant No. 2034192.
Conflict of Interest
JK was employed by the company Aerodyne Research, Inc.
The remaining authors declare that the research was conducted in the absence of any commercial or financial relationships that could be construed as a potential conflict of interest.
Acknowledgments
Thanks to our summer research interns Kelly Rushford, Steven Persaud, and Rafael Viana Furer for their preliminary data analysis.
Abbreviations
Mono Bio, monoterpenoid biosynthesis; Di Bio, diterpenoid biosynthesis; PAH Deg, polycyclic aromatic hydrocarbon degradation; RVI, relative volatility index; NMR, nuclear magnetic resonance; GC-CI-MS, gas chromatography coupled to chemical ionization mass spectrometry; GC-EI-MS, gas chromatography electron-ionization mass spectrometry; LC-EI-MS, liquid chromatography electron-ionization mass spectroscopy; FTICR-MS, fourier-transform ion cyclotron resonance mass spectrometry; PTR-TOF-MS, proton-transfer-reaction time-of-flight mass spectrometry.
References
Ali, J. G., Alborn, H. T., and Stelinski, L. L. (2011). Constitutive and induced subterranean plant volatiles attract both entomopathogenic and plant parasitic nematodes. J. Ecol. 99, 26–35. doi: 10.1111/j.1365-2745.2010.01758.x
Amann, A., Costello, B., de, L., Miekisch, W., Schubert, J., Buszewski, B., et al. (2014). The human volatilome: volatile organic compounds (VOCs) in exhaled breath, skin emanations, urine, feces and saliva. J. Br. Res. 8:034001. doi: 10.1088/1752-7155/8/3/034001
Asensio, D., Peñuelas, J., Filella, I., and Llusià, J. (2007). On-line screening of soil VOCs exchange responses to moisture, temperature and root presence. Plant Soil 291, 249–261. doi: 10.1007/s11104-006-9190-4
Barragán-Fonseca, K. Y., Van Loon, J. J. A., Dicke, M., and Lucas-Barbosa, D. (2020). Use of visual and olfactory cues of flowers of two brassicaceous species by insect pollinators. Ecol. Entomol. 45, 45–55. doi: 10.1111/een.12775
Bertin, C., Yang, X., and Weston, L. A. (2003). The role of root exudates and allelochemicals in the rhizosphere. Plant Soil 256, 67–83. doi: 10.1023/a:1026290508166
Bitas, V., Kim, H.-S., Bennett, J. W., and Kang, S. (2013). Sniffing on microbes: diverse roles of microbial volatile organic compounds in plant health. Mol. Plant. Microbe. Interact. 26, 835–843. doi: 10.1094/mpmi-10-12-0249-cr
Bottini, R., Cassán, F., and Piccoli, P. (2004). Gibberellin production by bacteria and its involvement in plant growth promotion and yield increase. Appl. Microbiol. Biotechnol. 65, 497–503.
Chameides, W. L., Lindsay, R. W., Richardson, J., and Kiang, C. S. (1988). The role of biogenic hydrocarbons in urban photochemical smog: atlanta as a case study. Science 241, 1473–1475. doi: 10.1126/science.3420404
Compernolle, S., Ceulemans, K., and Müller, J.-F. (2011). Evaporation: a new vapour pressure estimation methodfor organic molecules including non-additivity and intramolecular interactions. Atmos. Chem. Phys. 11, 9431–9450. doi: 10.5194/acp-11-9431-2011
de Boer, A. H., and de Vries-van Leeuwen, I. J. (2012). Fusicoccanes: diterpenes with surprising biological functions. Trends Plant Sci. 17, 360–368. doi: 10.1016/j.tplants.2012.02.007
de la Porte, A., Schmidt, R., Yergeau, É, and Constant, P. (2020). A gaseous milieu: extending the boundaries of the rhizosphere. Trends Microbiol. 28, 536–542. doi: 10.1016/j.tim.2020.02.016
Déziel, É, Paquette, G., Villemur, R., Lépine, F., and Bisaillon, J. (1996). Biosurfactant production by a soil Pseudomonas strain growing on polycyclic aromatic hydrocarbons. Appl. Environ. Microbiol. 62, 1908–1912. doi: 10.1128/aem.62.6.1908-1912.1996
Donahue, N. M., Robinson, A. L., Stanier, C. O., and Pandis, S. N. (2006). Coupled partitioning, dilution, and chemical aging of semivolatile organics. Environ. Sci. Technol. 40, 2635–2643. doi: 10.1021/es052297c
Eriksson, M., Fäldt, J., Dalhammar, G., and Borg-Karlson, A.-K. (2001). Determination of hydrocarbons in old creosote contaminated soil using headspace solid phase microextraction and GC–MS. Chemosphere 44, 1641–1648. doi: 10.1016/s0045-6535(00)00371-4
Folch, J., Lees, M., and Stanley, G. H. S. (1957). A simple method for the isolation and purification of total lipides from animal tissues. J. Biol. Chem. 226, 497–509. doi: 10.1016/s0021-9258(18)64849-5
Gabov, D., Yakovleva, E., and Vasilevich, R. (2020). Vertical distribution of PAHs during the evolution of permafrost peatlands of the European arctic zone. Appl. Geochem. 123:104790. doi: 10.1016/j.apgeochem.2020.104790
Ghirardo, A., Lindstein, F., Koch, K., Buegger, F., Schloter, M., Albert, A., et al. (2020). Origin of volatile organic compound emissions from subarctic tundra under global warming. Glob. Chang. Biol. 26, 1908–1925. doi: 10.1111/gcb.14935
Ghosal, D., Ghosh, S., Dutta, T. K., and Ahn, Y. (2016). Current state of knowledge in microbial degradation of polycyclic aromatic hydrocarbons (PAHs): a review. Front. Microbiol. 7:1369. doi: 10.3389/fmicb.2016.01369
Gil-Loaiza, J., Roscioli, J. R., Shorter, J. H., Volkmann, T. H. M., Ng, W.-R., Krechmer, J. E., et al. (2020). Versatile soil gas concentration and isotope monitoring: optimization and integration of novel soil gas probes with online trace gas detection. Biogeosciences Discuss. [preprint] doi: 10.5194/bg-2020-401
Gutiérrez-Mañero, F. J., Ramos-Solano, B., Probanza, A., Mehouachi, J., Tadeo, F. R., and Talon, M. (2001). The plant-growth-promoting rhizobacteria Bacillus pumilus and Bacillus licheniformis produce high amounts of physiologically active gibberellins. Physiol. Plant. 111, 206–211. doi: 10.1034/j.1399-3054.2001.1110211.x
Hammel, K. E. (1995). Mechanisms for polycyclic aromatic hydrocarbon degradation by ligninolytic fungi. Environ. Health Perspect. 103(Suppl. 5) 41–43. doi: 10.2307/3432477
Hewitt, A. D. (1996). “Effect of collection and handling practices on concentrations of volatile organic compounds detected in soil subsamples,” in Volatile Organic Compounds in the Environment, (West Conshohocken, PA: ASTM International).
Hilal, S. H., Karickhoff, S. W., and Carreira, L. A. (2003). Prediction of the vapor pressure boiling point, heat of vaporization and diffusion coefficient of organic compounds. QSAR Comb. Sci. 22, 565–574. doi: 10.1002/qsar.200330812
Hilal, S. H., Saravanaraj, A. N., Whiteside, T., and Carreira, L. A. (2007). Calculating physical properties of organic compounds for environmental modeling from molecular structure. J. Comput. Aided Mol. Des. 21, 693–708. doi: 10.1007/s10822-007-9134-y
Hiller, K., Hangebrauk, J., Jäger, C., Spura, J., Schreiber, K., and Schomburg, D. (2009). Metabolite detector: comprehensive analysis tool for targeted and nontargeted GC/MS based metabolome analysis. Anal. Chem. 81, 3429–3439. doi: 10.1021/ac802689c
Hollywood, K., Brison, D. R., and Goodacre, R. (2006). Metabolomics: current technologies and future trends. Proteomics 6, 4716–4723. doi: 10.1002/pmic.200600106
Kadri, T., Rouissi, T., Kaur Brar, S., Cledon, M., Sarma, S., and Verma, M. (2017). Biodegradation of polycyclic aromatic hydrocarbons (PAHs) by fungal enzymes: a review. J. Environ. Sci. 51, 52–74. doi: 10.1016/j.jes.2016.08.023
Kanehisa, M., and Goto, S. (2000). KEGG: kyoto encyclopedia of genes and genomes. Nucleic Acids Res. 28, 27–30.
Kanehisa, M., and Sato, Y. (2020). KEGG mapper for inferring cellular functions from protein sequences. Protein Sci. 29, 28–35. doi: 10.1002/pro.3711
Kind, T., Wohlgemuth, G., Lee, D. Y., Lu, Y., Palazoglu, M., Shahbaz, S., et al. (2009). FiehnLib: mass spectral and retention index libraries for metabolomics based on quadrupole and time-of-flight gas chromatography/mass spectrometry. Anal. Chem. 81, 10038–10048. doi: 10.1021/ac9019522
Lee, G. W., Chung, M.-S., Kang, M., Chung, B. Y., and Lee, S. (2016). Direct suppression of a rice bacterial blight (Xanthomonas oryzae pv. oryzae) by monoterpene (S)-limonene. Protoplasma 253, 683–690. doi: 10.1007/s00709-015-0904-4
Li, H., Väliranta, M., Mäki, M., Kohl, L., Sannel, A. B. K., Pumpanen, J., et al. (2020). Overlooked organic vapor emissions from thawing arctic permafrost. Environ. Res. Lett. 15:104097. doi: 10.1088/1748-9326/abb62d
Lu, X., Zhang, J., Brown, B., Li, R., Rodríguez-Romero, J., Berasategui, A., et al. (2018). Inferring roles in defense from metabolic allocation of rice diterpenoids. Plant Cell 30, 1119–1131. doi: 10.1105/tpc.18.00205
Malawska, M., Ekonomiuk, A., and Wiłkomirski, B. (2006). Polycyclic aromatic hydrocarbons in peat cores from southern Poland: distribution in stratigraphic profiles as an indicator of PAH sources. Mires Peat 1, 1–14.
Müller, A., Miyazaki, Y., Tachibana, E., Kawamura, K., and Hiura, T. (2017). Evidence of a reduction in cloud condensation nuclei activity of water-soluble aerosols caused by biogenic emissions in a cool-temperate forest. Sci. Rep. 7:8452.
Murphy, K. M., and Zerbe, P. (2020). Specialized diterpenoid metabolism in monocot crops: Biosynthesis and chemical diversity. Phytochemistry 172:112289. doi: 10.1016/j.phytochem.2020.112289
Nannoolal, Y., Rarey, J., and Ramjugernath, D. (2008). Estimation of pure component properties: Part 3. estimation of the vapor pressure of non-electrolyte organic compounds via group contributions and group interactions. Fluid Phase Equilib. 269, 117–133.
Pankow, J. F., and Asher, W. E. (2008). SIMPOL.1: a simple group contribution method for predicting vapor pressures and enthalpies of vaporization of multifunctional organic compounds. Atmos. Chem. Phys. 8, 2773–2796. doi: 10.5194/acp-8-2773-2008
Park, J.-H., Goldstein, A. H., Timkovsky, J., Fares, S., Weber, R., Karlik, J., et al. (2013). Active atmosphere-ecosystem exchange of the vast majority of detected volatile organic compounds. Science 341, 643–647. doi: 10.1126/science.1235053
Penuelas, J., Asensio, D., Tholl, D., Wenke, K., Rosenkranz, M., Piechulla, B., et al. (2014). Biogenic volatile emissions from the soil. Plant Cell Environ. 37, 1866–1891. doi: 10.1111/pce.12340
Reis, S. L., Mantello, A. G., Macedo, J. M., Gelfuso, E. A., da Silva, C. P., Fachin, A. L., et al. (2016). Typical monoterpenes as insecticides and repellents against stored grain pests. Molecules 21:258. doi: 10.3390/molecules21030258
Salazar-Cerezo, S., Martínez-Montiel, N., García-Sánchez, J., Pérez-Y-Terrón, R., and Martínez-Contreras, R. D. (2018). Gibberellin biosynthesis and metabolism: a convergent route for plants, fungi and bacteria. Microbiol. Res. 208, 85–98. doi: 10.1016/j.micres.2018.01.010
Seewald, M. S. A., Bonfanti, M., Singer, W., Knapp, B. A., Hansel, A., Franke-Whittle, I., et al. (2010). Substrate induced VOC emissions from compost amended soils under aerobic and anaerobic incubation. Biol. Fertil. Soils 46, 371–382. doi: 10.1007/s00374-010-0445-0
Tahir, H. A. S., Gu, Q., Wu, H., Raza, W., Hanif, A., Wu, L., et al. (2017). Plant growth promotion by volatile organic compounds produced by Bacillus subtilis SYST2. Front. Microbiol. 8:171. doi: 10.3389/fmicb.2017.00171
Tang, H.-Y., Yin, X., Zhang, C.-C., Jia, Q., and Gao, J.-M. (2015). Structure diversity, synthesis, and biological activity of cyathane diterpenoids in higher fungi. Curr. Med. Chem. 22, 2375–2391. doi: 10.2174/0929867322666150521091333
Tang, J., Schurgers, G., and Rinnan, R. (2019). Process understanding of soil BVOC fluxes in natural ecosystems: a review. Rev. Geophys. 57, 966–986. doi: 10.1029/2018rg000634
Tanimoto, E. (2005). Regulation of root growth by plant hormones—roles for auxin and gibberellin. CRC Crit. Rev. Plant Sci. 24, 249–265. doi: 10.1080/07352680500196108
Tfaily, M. M., Wilson, R. M., Cooper, W. T., Kostka, J. E., Hanson, P., and Chanton, J. P. (2018). Vertical stratification of peat pore water dissolved organic matter composition in a peat bog in Northern Minnesota. J. Geophys. Res. Biogeosci. 123, 479–494. doi: 10.1002/2017jg004007
Tolicì, N., Liu, Y., Liyu, A., Shen, Y., Tfaily, M. M., Kujawinski, E. B., et al. (2017). Formularity: software for automated formula assignment of natural and other organic matter from ultrahigh-resolution mass spectra. Anal. Chem. 89, 12659–12665. doi: 10.1021/acs.analchem.7b03318
Tyagi, S., Mulla, S. I., Lee, K.-J., Chae, J.-C., and Shukla, P. (2018). VOCs-mediated hormonal signaling and crosstalk with plant growth promoting microbes. Crit. Rev. Biotechnol. 38, 1277–1296. doi: 10.1080/07388551.2018.1472551
Vives-Peris, V., de Ollas, C., Gómez-Cadenas, A., and Pérez-Clemente, R. M. (2020). Root exudates: from plant to rhizosphere and beyond. Plant Cell Rep. 39, 3–17. doi: 10.1007/s00299-019-02447-5
Wang, Z., Li, H., and Liu, S. (2019). Different distribution of polycyclic aromatic hydrocarbons (PAHs) between sphagnum and ledum peat from an ombrotrophic bog in Northeast China. J. Soils Sediments 19, 1735–1744. doi: 10.1007/s11368-018-2178-x
Weljie, A. M., Newton, J., Mercier, P., Carlson, E., and Slupsky, C. M. (2006). Targeted profiling: quantitative analysis of 1H NMR metabolomics data. Anal. Chem. 78, 4430–4442. doi: 10.1021/ac060209g
Wilcke, W., and Amelung, W. (2000). Persistent organic pollutants in native grassland soils along a climosequence in North America. Soil Sci. Soc. Am. J. 64, 2140–2148. doi: 10.2136/sssaj2000.6462140x
Wilson, R. M., Malak, M. T., Max, K., Eric, J., Caitlin, P., Cassandra, A. Z., et al. (2021). Soil metabolome response to whole ecosystem warming at the Spruce and Peatland Responses Under Changing Environments experiment. PNAS. (in press).
Yáñez-Serrano, A. M., Fasbender, L., Kreuzwieser, J., Dubbert, D., Haberstroh, S., Lobo-do-Vale, R., et al. (2018). Volatile diterpene emission by two mediterranean Cistaceae shrubs. Sci. Rep. 8, 6855.
Zhao, C., Huang, M., Fast, J. D., Berg, L. K., Qian, Y., Guenther, A., et al. (2016). Sensitivity of biogenic volatile organic compounds to land surface parameterizations and vegetation distributions in California. Geosci. Mod. Dev. 9, 1959–1976. doi: 10.5194/gmd-9-1959-2016
Zhao, J.-C., Wang, Y.-L., Zhang, T.-Y., Chen, Z.-J., Yang, T.-M., Wu, Y.-Y., et al. (2018). Indole diterpenoids from the endophytic fungus Drechmeria sp. as natural antimicrobial agents. Phytochemistry 148, 21–28. doi: 10.1016/j.phytochem.2018.01.010
Keywords: soil metabolome, volatilome, volatile organic compounds, soil microbial processes, GC-MS, FT-ICR-MS, PTR-TOF-MS, metabolomics
Citation: Honeker LK, Graves KR, Tfaily MM, Krechmer JE and Meredith LK (2021) The Volatilome: A Vital Piece of the Complete Soil Metabolome. Front. Environ. Sci. 9:649905. doi: 10.3389/fenvs.2021.649905
Received: 05 January 2021; Accepted: 25 March 2021;
Published: 23 April 2021.
Edited by:
Monika Mortimer, China Jiliang University, ChinaReviewed by:
David Amouroux, UMR 5254 Institut des Sciences Analytiques et de Physico-Chimie pour l’Environnement et les Matériaux (IPREM), FranceSaraswati Saraswati, University of Waterloo, Canada
Peng Cai, Huazhong Agricultural University, China
Copyright © 2021 Honeker, Graves, Tfaily, Krechmer and Meredith. This is an open-access article distributed under the terms of the Creative Commons Attribution License (CC BY). The use, distribution or reproduction in other forums is permitted, provided the original author(s) and the copyright owner(s) are credited and that the original publication in this journal is cited, in accordance with accepted academic practice. No use, distribution or reproduction is permitted which does not comply with these terms.
*Correspondence: Laura K. Meredith, bGF1cmFtZXJlZGl0aEBhcml6b25hLmVkdQ==