How to Promote Compliance Management in the Electricity Market? An Analysis Based on the Evolutionary Game Model
- 1Zhejiang Electricity Trading Center Co., Ltd., Hangzhou, China
- 2Zhejiang Huayun Mdt Info Tech Ltd., Hangzhou, China
- 3China Center for Energy Economics Research, School of Economics, Xiamen University, Xiamen, China
In this paper, we aim to analyze how to effectively promote compliance management in the electricity market. We construct an evolutionary game model under the two different scenarios, i.e., the scenario without governmental supervision and the scenario with governmental supervision, and explicitly describes the strategic behaviors and dynamic evolution process of power enterprises and regulators in the power market. According to the results of the evolutionary stable strategy, we find that, in the absence of governmental supervision, the long-term stable equilibrium of power enterprises' choice of strategy “Compliance” and regulatory agencies' choice of strategy “Not bribery” is hard to be realized. Only if the government effectively supervises the compliance management of the electricity market can the ideal compliance behavior of the two stakeholders be achieved. Furthermore, we conduct a simulation study to analyze the impacts of the various model parameters on the dynamic evolution process. The specific results show that the lower compliance cost, the higher psychological cost, and the larger profit loss of the power enterprises, as well as the lower inspection cost, the higher psychological cost, and the larger rewards of the regulatory agencies, can promote the formation of compliance management. Besides, the larger penalty charged by the government is also conducive to the compliance management of the electricity market.
Introduction
The electricity market is undergoing rapid and dramatic changes (Höhne and Tiberius, 2020). With the emergence of new business modes, the trading modes of the electricity market have been increasingly complex. Moreover, the rapid development and wide application of big data technology and machine learning in China have also brought opportunities and challenges to the power industry (Song et al., 2019). Besides, the electricity market is facing compliance risks brought about by the potential non-standard business operation of enterprises, which leads to additional difficulties and challenges in the management and development of the electricity market. In 2015, the Chinese government pointed out that “it is necessary to ameliorate power regulatory institutions, measures and methods, improve governmental regulatory means, raise the level of scientific supervision over technology, safety, transactions and operations, and further achieve scientific supervision of power system” in the document named “Several Opinions on Further Deepening the Reform of the Power System.” Moreover, to better promote corporate compliance management, the Chinese government has successively promulgated a series of policies such as “Guidelines for the Compliance Management System” and the “Guidelines for the Compliance Management of Central Enterprises” to comprehensively improve the level of compliance for enterprises, including power enterprises.
The requirements of compliance management include three aspects, namely national laws, corporate rules, and professional ethics. Through continuous improvement of market-related laws and rules, the government can discover the market entities' violations of trading rules and regulations timely, thereby safeguarding the overall interests of stakeholders, reducing market risks, and ensuring the sustainable development of the electricity market. The development of compliance management in the electricity market is carried out in conformity with the requirement of the Chinese reform in the field of the electricity market, and catering to the future development demands of the electricity market. Moreover, compliance management is an important guarantee for effectively preventing risks in the electricity market and is of great significance to the business expansion of the electricity market. However, the compliance management of China's electricity market is still in its infancy. At present, there is still a lack of systematic policies to ensure the effective implementation of compliance management in the electricity market. Only by building a complete compliance management system can the electricity market provide better services for society and provide solid and effective support for economic and social development. Therefore, it is very important and necessary to study the compliance management of the electricity market.
Many previous studies have discussed the possible risks in the electricity market from different aspects. Vehviläinen and Keppo (2003) apply the method of financial risk management to the price risk in the electricity market and prove that if the properties of the electricity market are fully considered, the financial method could also be useful for the risk management of the electricity market. Similarly, Deng and Oren (2006) review the applications of various financial derivatives in the electricity market, i.e., electricity derivatives, and analyze the effects of these derivatives in reducing market risks and optimizing hedging strategies. Taking CVaR as the risk measure, Kharrati et al. (2016) construct a model for the decision-making problem of the retailers in the competitive retail power market. The numerical results show that the decisions of rivals will affect the strategies selected by the retailers. Besides, based on the hourly electricity prices of Nord Pool, Uribe et al. (2020) find that the consumers and producers in the power market have asymmetric risks. The tail distribution of the electricity price plays an important role in the risk exposure. Coulon et al. (2013) construct a model for load and price dynamics in the electricity market which aims to reduce the risk of hedging load and price and further find out the optimal hedging strategies. Meunier (2013) tries to analyze the impacts of the risk aversion on the technology mixes of firms in the electricity market and figure out how the uncertainty of fossil fuel prices affects the investment decisions of power producers. The risks that exist in renewable power are also studied. Bahrami and Amini (2018) propose a decentralized energy trading model to reduce the generation risks in the renewable resources. At the same time, the goal of minimizing the cost of load aggregators and maximizing the profit of the generators could be also achieved. Taking wind power as an example, Klessmann et al. (2008) discuss the risks of integrating renewable energy generators into the electricity market in Germany, Spain, and the United Kingdom. The results show that when the market risk is higher, the development of renewable energy needs more financial support. However, higher market risks may also limit indirect costs to society. Aquila et al. (2017) construct a model to analyze the investment decision of wind power generators under different risks in a regulated electricity market and use the method of VaR to make the risk management.
However, the researches on the compliance management risks of the electricity market are limited. Therefore, this paper aims to study the strategic behavior of different stakeholders in the electricity market based on game theory, so as to specifically analyze the influence mechanism of various factors on the compliance management of the electricity market and effectively prevent the compliance management risks in the electricity market.
Compared with the static game, the dynamic game is more suitable to analyze the relationship between different stakeholders, because players' decision-making behavior will change with other participants' strategy to maximize the utility. Therefore, we may prefer the use of the dynamic game model when we want to study sensible countermeasures or propose several policy recommendations (Xie et al., 2017; Jiang et al., 2020). The evolutionary game model, as an important part of the dynamic game, has been widely applied in the analysis of the interactive strategies between different stakeholders. For example, Jiang et al. (2019) analyze the effects of implementing environmental regulation under fiscal decentralization in China; the strategies of three players, i.e., central government, local government, and polluting enterprises, are discussed through the evolutionary game model. Similarly, Sheng et al. (2020) explore the strategy choices of the central government, local government, and firms. The results illustrate that government supervision is important for the implementation of environmental regulation policies. da Silva Rocha and Salomão (2019) analyze the interplay between firms and auditors under the framework of the evolutionary game model as well as well-mixed and structured populations. The results show that high inspecting cost is not conducive to the auditing process. The study of Sun and Zhang (2019) discuss the problem of greenwashing and the role of governmental regulation. Two kinds of firms (dominant and inferior firms) are regarded as the players in the evolutionary game model. The results show that governmental punishment can inhibit the greenwashing behavior of both two kinds of firms, but the governmental tax subsidy can only curb the greenwashing behavior of dominant firms. Zhu et al. (2020) study the renewable portfolio standard through the evolutionary game model. The strategy selection of two different kinds of power sales enterprises and the regulators are analyzed. The results show that tradable green certificate prices, net profit, reward, and punishment are important factors to make different kinds of power sales enterprises comply with quota obligations in the electricity market. Ji et al. (2015) discuss green purchasing in the supply chain based on the evolutionary game model. The cooperation tendency of two players, i.e., suppliers and manufacturers, is studied in detail. Furthermore, some policy suggestions on establishing the green supply chain are obtained. Tian et al. (2014) analyze how to effectively promote green supply chain management from the perspective of subsidy and use the evolutionary game model to study the strategies of three different stakeholders in the automotive manufacturing industry. Based on the evolutionary game model, the work of Gu et al. (2019) mainly focuses on reverse logistics. The interactive strategy between the natural resource-intensive and energy-intensive enterprises is analyzed to discuss how to achieve sustainable development in these industries.
The main contributions of this paper are three-fold. Firstly, in this paper, we construct an evolutionary game model to analyze the dynamic evolution process of stakeholders' strategies in the electricity market. Secondly, we compare and analyze the evolutionary stability strategy under two different scenarios, namely, the scenario without governmental supervision and the scenario with governmental supervision. Moreover, we analyze the specific impacts of different model parameter changes on the realization of compliance management in the electricity market. Thirdly, based on the model results, we further put forward relevant policy recommendations for the promotion of compliance management in the electricity market.
The structure of this paper is as follows. In section Construction of the Evolutionary Game Model, we discuss the evolutionary game model and the specific details. We conduct the simulation study in section Simulation Study. Section Further Discussion is about the discussion. Section Conclusion and Policy Suggestion concludes and proposes relevant policy recommendations.
Construction of the Evolutionary Game Model
Model Description
To study the compliance management in the electricity market, we mainly focus on the strategic behavior of two stakeholders, namely, the power enterprises and regulatory agencies. Hence, the strategy choices and payoffs of these two players in the electricity market need to be fully considered when constructing the evolutionary game model. Moreover, the policy implementation of the central government may also affect the strategy selections and payoffs of the power enterprises and regulatory agencies. For instance, when the central government decides to carry out strict supervision over the compliance management in the electricity market, it will promote the regulatory agencies to strengthen the enforcement of compliance management, thereby forcing the power enterprises to choose compliance in the operation of the business with higher probability. On the contrary, if the central government decides not to conduct strict supervision, the regulatory agencies may not effectively perform their responsibilities, giving enterprises incentives to be non-compliant. Therefore, this paper will analyze the dynamic decision-making process between the two stakeholders in two scenarios: the existence and absence of governmental supervision.
The development of compliance management in China's electricity market is still in its infancy. This paper specifically studies how to effectively control compliance risks from the perspective of evolutionary game theory. The two assumptions, completely rational and complete information in the traditional game theory, are released in the evolutionary game theory. In the evolutionary game theory, decision-makers are assumed to be bounded rationality, which means that decision-makers do not always make decisions to maximize their utility, and their strategic choices may be affected by the environment, i.e., the actions of other players. In this paper, we follow the evolutionary game theory and make the assumptions that there exist many power enterprises and regulatory agencies in the electricity market, and in the process of decision-making, they will learn from others and adjust their choices to obtain a larger payoff.
Power Enterprises
The power enterprises have two possible strategies {Compliance, Not compliance}. We use x to denote the probability that the power enterprises select the strategy of “Compliance.” When the power enterprises choose the strategy of “Compliance,” it means that the power enterprises will make more effort to meet the external (including national and industrial levels) and internal (corporate level) requirements of various laws and regulations in the electricity market. We use c to represent the costs of compliance effort. When the power enterprises choose the strategy of “Not Compliance,” the compliance costs c can be omitted. However, the power enterprises will bear psychological costs pe for fear of being discovered. At the same time, the power enterprises tend to collude with regulatory agencies to avoid exposure of their illegal actions. We use b to represent the bribery costs of the power enterprises, which can also be considered as the amount of money that regulatory agencies earn from the power enterprises. If the power enterprises and the regulatory agencies reach a collusive agreement, both of them will face fines equal to f once the central government finds out. However, if the power enterprises adopt unilateral bribery, they will receive larger fines λf (λ > 1) charged by the central government. Moreover, if the regulatory agencies discover the non-compliance behavior of the power enterprises, the power enterprises' reputation will be damaged. Thence, we assume that the profits of power enterprises will reduce r due to the damaged reputation and the fierce competition in the electricity market.
Regulatory Agencies
The regulatory agencies also have two possible strategies {Not bribery, Bribery}. y denotes the probability that the regulatory agencies select the strategy of “Not bribery.” The strategy of “Not bribery” means that the regulatory agencies will carry out the strict inspection of the behavior of the power enterprises. Moreover, we use s to represent the costs of inspection effort. If the non-compliant behavior of the power enterprises was detected, the regulatory agencies will receive a certain reward e. Conversely, if the regulatory agencies choose the strategy of “Bribery,” they will not scrutinize the behavior of the power enterprises, and the inspection costs s could be ignored. However, the regulatory agencies will bear psychological costs pr for fear of being discovered by the central government. Moreover, they can receive money equals to b from enterprises if they conspire. However, if the central government executes supervision over the compliance management in the electricity market, the bribery behavior will incur fines f charged by the central government. Similar to the power enterprises, the unilateral bribery of the regulatory agencies will be severely punished with fines equals to λf. The notations of all variables used in the evolutionary game model are shown in Table 1.
Payoff Matrix
Scenario Without Governmental Supervision
In the scenario without governmental supervision, the power enterprises will get −c and the regulatory agencies will get −s in the ideal state, namely, the regulatory agencies will earnestly perform their regulatory responsibilities, and the power enterprises will also act in compliance. If the power enterprises choose the strategy “Not compliance” and the regulatory agencies choose the strategy “Not bribery,” the non-compliance behaviors of power companies will be discovered by regulatory agencies. Hence, the power enterprises will get −pe −r and the regulatory agencies will get −s + e. If the power enterprises choose the strategy “Compliance” and the regulatory agencies choose the strategy “Bribery,” the power enterprises will get −c and the regulatory agencies will get −pr. If these two stakeholders collude, the power enterprises will get −pe −b and the regulatory agencies will get −pr + b. The payoff matrix in the scenario without governmental supervision is shown in Table 2.
Scenario With Governmental Supervision
In the scenario with governmental supervision, the payoff matrix is a bit more complicated because we should take into account the fines charged by the central government. In the ideal state, the power enterprises will get −c and the regulatory agencies will get −s, which is exactly the same as in the scenario without governmental supervision. If the power enterprises choose the strategy “Not compliance” and the regulatory agencies choose the strategy “Not bribery,” the non-compliance behaviors of power companies will be discovered by regulatory agencies. Besides, the central government will also punish the non-compliance behaviors of power companies. Hence, the power enterprises will get −pe − λf − r and the regulatory agencies will get −s + e. If the power enterprises choose the strategy “Compliance” and the regulatory agencies choose the strategy “Bribery,” the central government will punish the regulatory agencies. So the power enterprises will get −c and the regulatory agencies will get −pr − λf. If the collusion occurs, the power enterprises will get −pe − b − f and the regulatory agencies will get −pr + b − f. The payoff matrix in the scenario with governmental supervision is shown in Table 3.
Replicator Dynamic System
Scenario Without Governmental Supervision
In the evolutionary game theory, if the payoffs of some players are less than the overall average payoff, then these players will change their strategies. Moreover, if the payoffs of some players are higher than the average, then in the population, the probability of choosing the same strategy will increase (Taylor and Jonker, 1978; Friedman, 1991). Therefore, based on the replicator dynamic system, it is possible to describe the dynamic changes in the probability of players choosing different strategies.
Firstly, we will calculate the expected payoffs under the different strategic behaviors of the power enterprises and regulatory agencies. Using and to represent the strategies of “Compliance” and “Not compliance” of the power enterprises. Then, we have:
Then, we can obtain the expected payoff of the power enterprises, which is shown in Equation (3).
Therefore, the replicator dynamic function of the strategy “Compliance” can be seen in Equation (4).
Similarly, we use the variables and to represent the expected payoffs of the regulatory agencies for choosing the “Not bribery” and “Bribery.” Then, we have:
Then, the expected payoff of the regulatory agencies is shown in Equation (7).
Therefore, the replicator dynamic function of the strategy “Not bribery” is shown in Equation (8).
Scenario With Governmental Supervision
In the same way, we can get the expected payoffs of the power enterprises and regulatory agencies under the situation of governmental supervision. When the central government decides to take some measures to promote compliance management in the electricity market, the government will intensify the supervision on the behavior of power enterprises and regulatory agencies. The expected payoffs under the two different strategies of the power enterprises are:
Then, the expected payoff of the enterprises is shown in Equation (11).
Therefore, the replicator dynamic function of the strategy “Compliance” is Equation (12).
Similarly, the expected payoffs under the two strategies of the regulatory agencies are:
Then, the expected payoff of the regulatory agencies is shown in Equation (15).
Therefore, the replicator dynamic function of the strategy “Not bribery” is shown in Equation (16).
Evolutionary Stable Strategy
Scenario Without Governmental Supervision
Based on the replicator dynamic functions obtained in Equations (4) and (8), we can get the equilibrium points (x, y) ∈ [0, 1] × [0, 1] that satisfy the requirement that the replicator dynamic function should equal to zero due to the evolutionary game theory. Therefore, we have Proposition 1, as shown below.
Proposition 1. The replicator dynamic system in the scenario without governmental supervision has five equilibrium points, i.e., (0, 0), (0, 1), (1, 0), (1, 1), and (x*, y*), where , and , if and 0.
Proof:
(i) Obviously, the four fixed- points (0, 0), (0, 1), (1, 0), and (1, 1) can satisfy the equilibrium conditions.
(ii) When we solve Equation (17) which is shown as follows:
We can get the solution specified in Equation (18).
Moreover, the conditions and 0 should be satisfied to ensure (x*, y*) ∈ [0, 1] × [0, 1].
Next, we calculate the Jacobian matrix of the replicator dynamic system to obtain the evolutionary stability strategy (ESS). The Jacobian matrix is specified in Equation (19), and we can further obtain Proposition 2.
Proposition 2.
(i) The equilibrium point (0, 0) is an ESS if pe + b < c and e + pr < s + b.
(ii) The equilibrium point (0, 1) is an ESS if r + pe < c and s + b < e + pr.
(iii) The equilibrium point (1, 0) is an ESS if c < pe + b and pr < s.
(iv) The equilibrium point (1, 1) is an ESS if c < r + pe and s < pr.
(v) The equilibrium point (x*, y*) is not a stable equilibrium point.
Proof:
(i) The Jacobian matrix at the equilibrium point (0, 0) is shown as follows:
When pe + b < c and e + pr < s + b, the two eigenvalues (λ1 = −c + pe + b, λ2 = e − s + pr − b) of the Jacobian matrix J1 are both negative. Also, we have det J1 > 0 and trJ1 < 0. Therefore, the equilibrium point (0, 0) is an ESS if pe + b < c and e + pr < s + b.
(ii) The Jacobian matrix at the equilibrium point (0, 1) is shown as follows:
When pe + b + f < c and e + pr + f < s + b, the two eigenvalues (λ1 = pe + b + f − c, λ2 = e − s + pr − b + f) of the Jacobian matrix J2 are both negative. Moreover, we have detJ1 > 0 and trJ1 < 0. Therefore, the equilibrium point (0, 0) is an ESS if pe + b + f < c and e + pr + f < s + b.
(iii) The Jacobian matrix at the equilibrium point (1, 0) is shown as follows:
When c < pe + b and pr < s, the two eigenvalues (λ1 = c − pe − b, λ2 = pr − s) of the Jacobian matrix J1 are both negative. Therefore, the equilibrium point (1, 0) is an ESS if c < pe + b and pr < s.
(iv) The Jacobian matrix at the equilibrium point (1, 1) is shown as follows:
When c < r + pe and s < pr, the two eigenvalues (λ1 = c − r − pe, λ2 = s − pr) of the Jacobian matrix J1 are both negative. Therefore, the equilibrium point (1, 1) is an ESS if c < r + pe and s < pr.
(v) In the evolutionary game model, an equilibrium point is stable when, and only when, it is a pure-strategy Nash equilibrium (Selten, 1980; Ritzberger and Weibull, 1995). Because the equilibrium point (x*, y*) is a hybrid-strategy Nash equilibrium, it is not a stable equilibrium point (Sheng et al., 2020).
Scenario With Governmental Supervision
Similarly, we could obtain the equilibrium points under the scenario with governmental supervision. Furthermore, we propose Proposition 3 which is shown as follows.
Proposition 3. The replicator dynamic system in the scenario with governmental supervision has five equilibrium points, i.e., (0, 0), (0, 1), (1, 0), (1, 1) and (x*, y*), where , and , if and 0.
Proof:
(i) Obviously, the four fixed points (0, 0), (0, 1), (1, 0), and (1, 1) can satisfy the equilibrium conditions.
(ii) When we solve Equation (24):
We have the solution which is shown in Equation (25).
Moreover, the conditions and 0 should be satisfied to ensure (x*, y*) ∈ [0, 1] × [0, 1].
Then, we calculate the Jacobian matrix of the replicator dynamic system to obtain the ESS in the scenario with governmental supervision. The Jacobian matrix is specified in Equation (26), and we can further obtain Proposition 4.
Proposition 4.
(i) The equilibrium point (0, 0) is an ESS if pe + b + f < c and e + pr + f < s + b.
(ii) The equilibrium point (0, 1) is an ESS if λf + r + pe < c and s + b < e + pr + f.
(iii) The equilibrium point (1, 0) is an ESS if c < pe + b + f and λf + pr < s.
(iv) The equilibrium point (1, 1) is an ESS if c < λf + r + pe and s < λf + pr.
(v) The equilibrium point (x*, y*) is not a stable equilibrium point.
Proof:
(i) The Jacobian matrix at the equilibrium point (0, 0) is shown as follows:
When pe + b + f < c and e + pr + f < s + b, the two eigenvalues (λ1 = pe + b + f − c, λ2 = e − s + pr − b + f) of the Jacobian matrix J2 are both negative. Moreover, we have detJ1 > 0 and trJ1 < 0. Therefore, the equilibrium point (0, 0) is an ESS if pe + b + f < c and e + pr + f < s + b.
(ii) The Jacobian matrix at the equilibrium point (0, 1) is shown as follows:
When λf + r + pe < c and s + b < e + pr + f, the two eigenvalues (λ1 = λf + r − c + pe, λ2 = s − e − pr + b − f) of the Jacobian matrix J2 are both negative. Therefore, the equilibrium point (0, 1) is an ESS if λf + r + pe < c and s + b < e + pr + f.
(iii) The Jacobian matrix at the equilibrium point (1, 0) is shown as follows:
When c < pe + b + f and λf + pr < s, the two eigenvalues (λ1 = c − pe − b − f, λ2 = λf − s + pr) of the Jacobian matrix J2 are both negative. Therefore, the equilibrium point (1, 0) is an ESS if c < pe + b + f and λf + pr < s.
(iv) The Jacobian matrix at the equilibrium point (1, 1) is shown as follows:
When c < λf + r + pe and s < λf + pr, the two eigenvalues (λ1 = c − λf − r − pe, λ2 = s − λf −pr) of the Jacobian matrix J2 are both negative. Therefore, the equilibrium point (1, 1) is an ESS if c < λf + r + pe and s < λf + pr.
(v) Same as the proof in Proposition 2.
Simulation Study
Parameter Setting
In this paper, we aim to analyze how to effectively promote compliance management in the electricity market based on the evolutionary game model. Therefore, in the long-term stable equilibrium, the optimal equilibrium point is (1, 1). As stated in Proposition 2, in the scenario without governmental supervision, the equilibrium point (1, 1) is an ESS if c < r + pe and s < pr. However, the condition s < pr will be hard to satisfy because the psychological costs of the regulatory agencies to select the strategy “Bribery” are usually less than the inspection costs in the reality. This is exactly the reason why the regulatory agencies have the incentive to choose the strategy “Bribery.” Therefore, when the central government does not take effective measures to supervise compliance management in the electricity market, it is difficult for all the stakeholders to choose ideal strategies in long-term stable equilibrium.
Proposition 4 shows that in the scenario with governmental supervision, the equilibrium point (1, 1) is an ESS if c < λf + r + pe and s < λf + pr. This condition can be realized in the reality. Therefore, we mainly focus on the dynamic evolution process in the scenario with governmental supervision and analyze the various influencing factors through simulation. According to the relevant conditions of the parameters in the ESS, we set the initial values of the parameters (randomly generated) in our evolutionary game model as shown in Table 4.
To check the robustness of the results, we set the initial values of x and y to 0.1, 0.3, 0.5, and 0.7, respectively. It can be seen from Figure 1 that no matter what the initial value is set, the probability that the power enterprises choose “Compliance” and the probability that the regulatory agencies choose “Not bribery” both tend to be 1 in the long run. Therefore, we verify the result that the equilibrium point (1, 1) is an ESS if c < λf + r + pe and s < λf + pr.
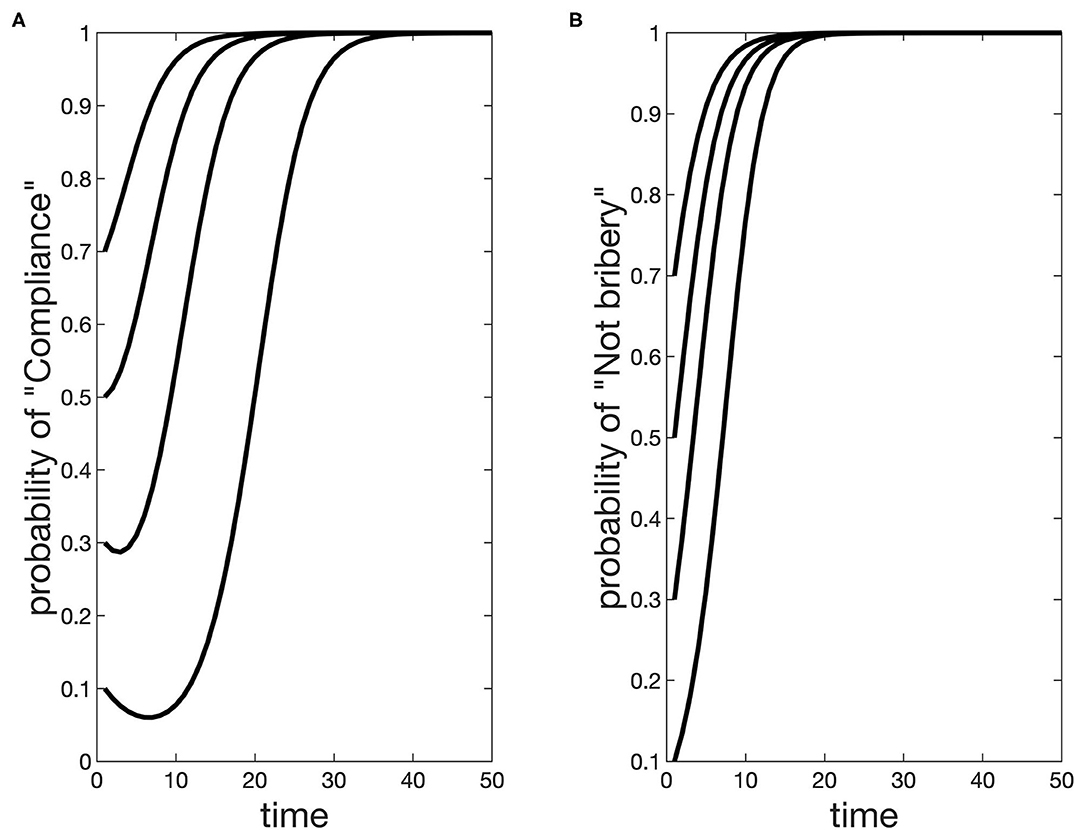
Figure 1. Evolutionary process of the two stakeholders' strategies. (A) is the dynamic strategy of the enterprises, and (B) is the dynamic strategy of the regulatory agencies.
Sensitivity Analysis
To analyze the impacts of various parameters on the dynamic evolutionary process specifically, we further make the sensitivity analysis for each model parameter.
The heterogeneous impacts of the compliance costs c of the power enterprises are shown in Figure 2. It can be seen that changes in compliance costs c exhibit greater impacts on the strategic choices of power enterprises. When the value of compliance costs increases, the probability evolution speed of power enterprises in choosing “Compliance” will decrease, which leads to the persistent choice of “Not compliance” in the electricity market. However, changes in compliance costs have little impact on the strategic choices of the regulatory agencies, and the influence is indirect according to the replicator dynamic system. In other words, the impacts of the changes in compliance costs on the strategic behavior of the regulatory agencies are totally based on the changes in the strategic behavior of power enterprises.
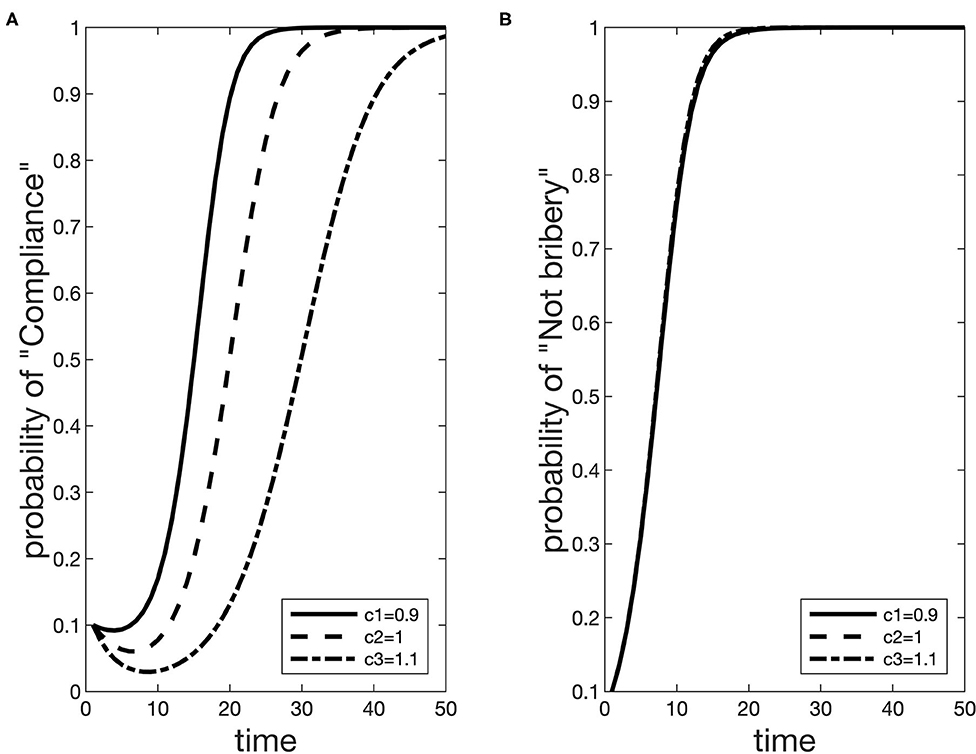
Figure 2. Evolutionary process of the two stakeholders' strategies with different values of c. (A) is the dynamic strategy of the enterprises, and (B) is the dynamic strategy of the regulatory agencies.
As shown in Figure 3, for power enterprises, the impact of psychological cost pe on the probability of choosing the strategy “Compliance” is just the opposite of the impact of compliance costs c. That is to say, the greater the psychological pressure that the power enterprises will face when choosing the strategy “Not compliance,” the lower the probability that the power enterprises will choose the strategy “Not compliance.” This result illustrates that higher psychological costs pe can force the power enterprises to be more likely to make decisions that comply with relevant laws and regulations. Similar to the costs of compliance c, the psychological costs of power enterprises pe have little influence on the decision-making process of the regulatory agencies. It mainly affects the strategic behavior of the power enterprises and then plays role on the selection of the regulatory agencies.
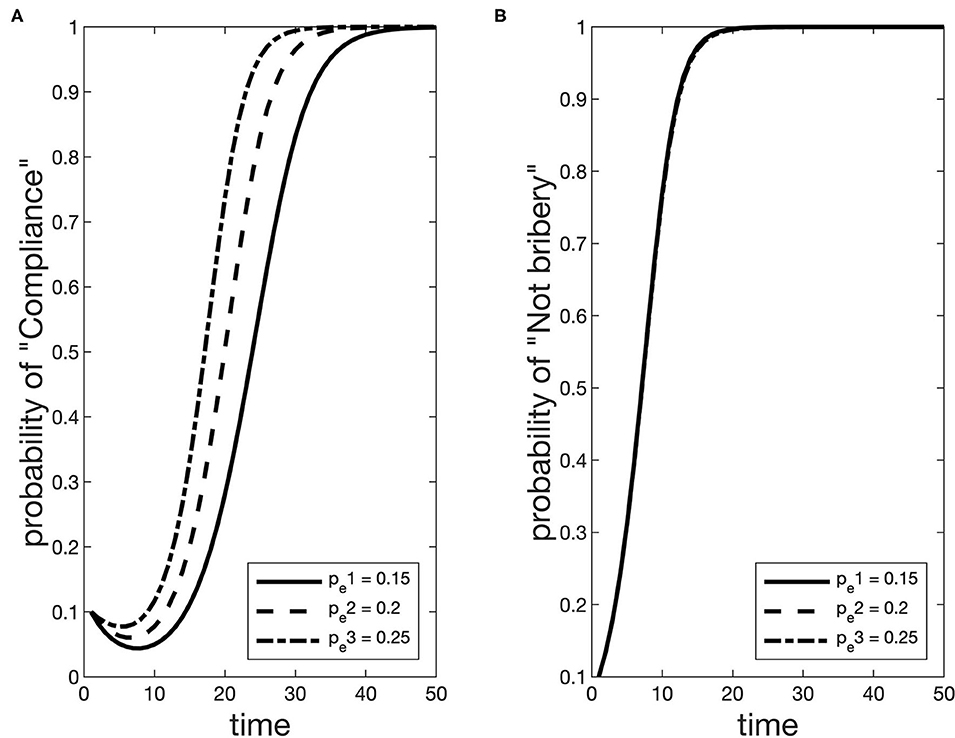
Figure 3. Evolutionary process of the two stakeholders' strategies with different values of pe. (A) is the dynamic strategy of the enterprises, and (B) is the dynamic strategy of the regulatory agencies.
It is seen from Figure 4 that the bribery costs of the power enterprises b have a certain impact on the strategy selections of the two stakeholders. When the bribery costs b are greater, the power enterprises will choose the strategy “Compliance” instead of the strategy “Not compliance” early, because the power enterprises need to pay more money to the regulatory agencies. As far as the regulatory agencies are concerned, if the amount of bribes is larger, the regulatory agencies are more inclined to collude with power enterprises, which will slow down the dynamic changes of the regulatory agencies' decision to “Not bribery.”
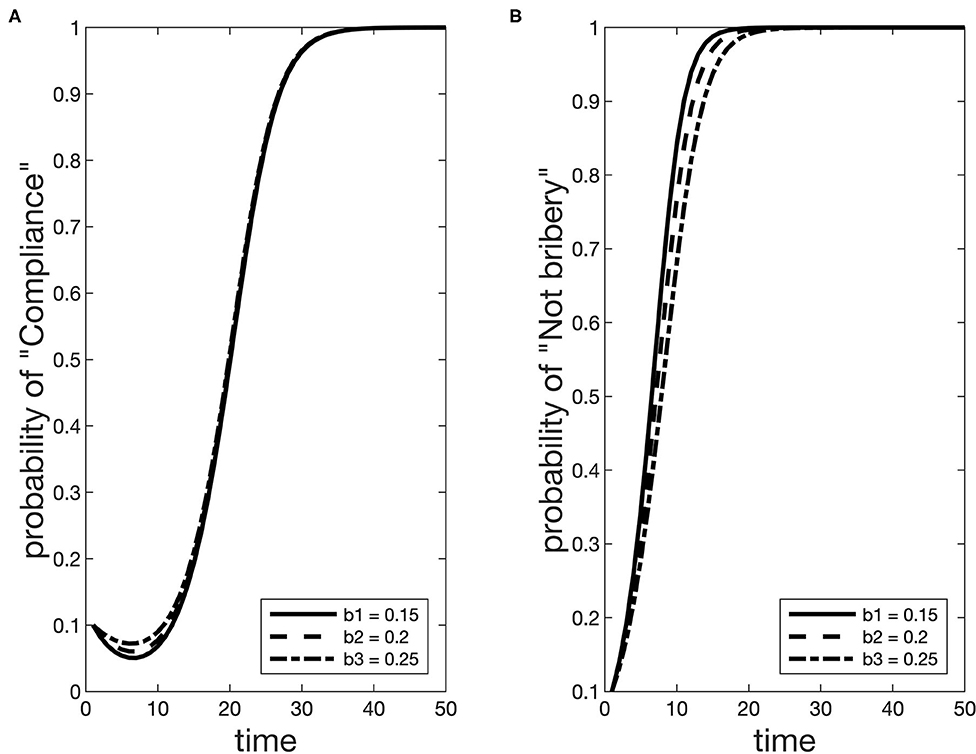
Figure 4. Evolutionary process of the two stakeholders' strategies with different values of b. (A) is the dynamic strategy of the enterprises, and (B) is the dynamic strategy of the regulatory agencies.
The impacts of profit loss r on the strategic choices of the power enterprises are similar to the impacts of psychological cost pe. When the non-compliance of the power enterprises is discovered, the corporate reputation will be damaged, thereby reducing the profits of the power enterprises. The greater the impact on corporate profits, the more likely the power enterprises are to choose strategy “Compliance,” which makes the proportion of compliance companies in the electricity market higher. Besides, it is seen from Figure 5 that the change in a profit loss of the power enterprises r has less impact on the decision choices of the regulatory agencies.
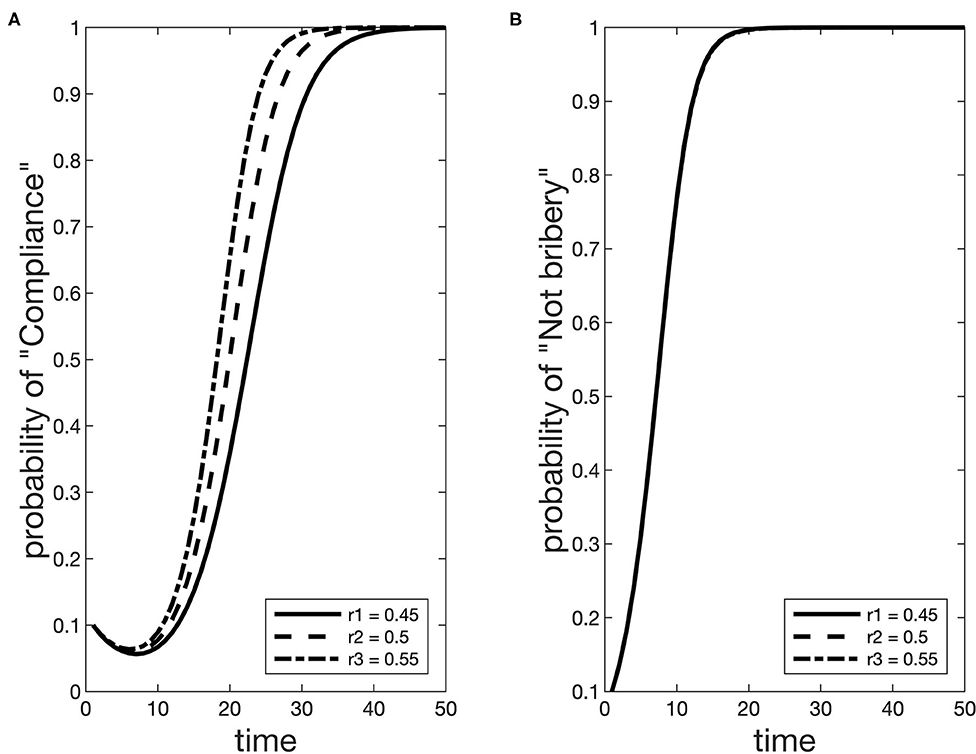
Figure 5. Evolutionary process of the two stakeholders' strategies with different values of r. (A) is the dynamic strategy of the enterprises, and (B) is the dynamic strategy of the regulatory agencies.
The results in Figure 6 show that the inspection costs of the regulatory agencies s exhibit similar impacts on the decision-making of power enterprises and regulatory agencies. When the inspection costs of the regulatory agencies s are higher, the probability of power enterprises choosing strategy “Compliance” and the probability of regulatory agencies choosing strategy “Not bribery” will approach 1 more slowly in the long run. This illustrates that higher costs of inspection are not conducive to implement compliance management and effective supervision in the electricity market.
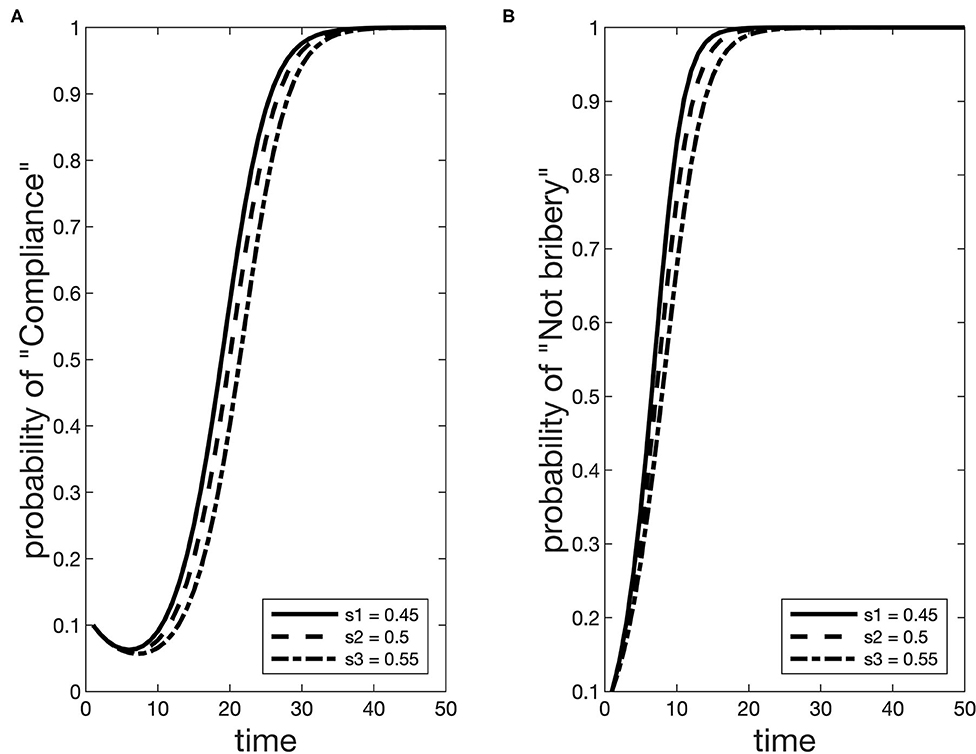
Figure 6. Evolutionary process of the two stakeholders' strategies with different values of s. (A) is the dynamic strategy of the enterprises, and (B) is the dynamic strategy of the regulatory agencies.
When regulatory agencies choose not to implement effective inspection and tend to collude with power enterprises, they will bear a certain psychological cost pr. As shown in Figure 7, the psychological costs of the regulatory agencies pr also display the same influences on the strategic choices of the power enterprises and the regulatory agencies, which is similar to the inspection costs s. When the psychological costs pr are higher, the possibility of the regulatory agencies choosing strategy “Bribery” will decrease. Moreover, the higher psychological costs pr can more effectively promote the regulatory agencies to perform the duties of inspection effectively. Furthermore, as the regulatory agencies are less likely to accept bribes, power enterprises are also more inclined to conduct “Compliance.” Therefore, the higher psychological cost pr of the regulatory agencies will better promote the compliance management of the electricity market.
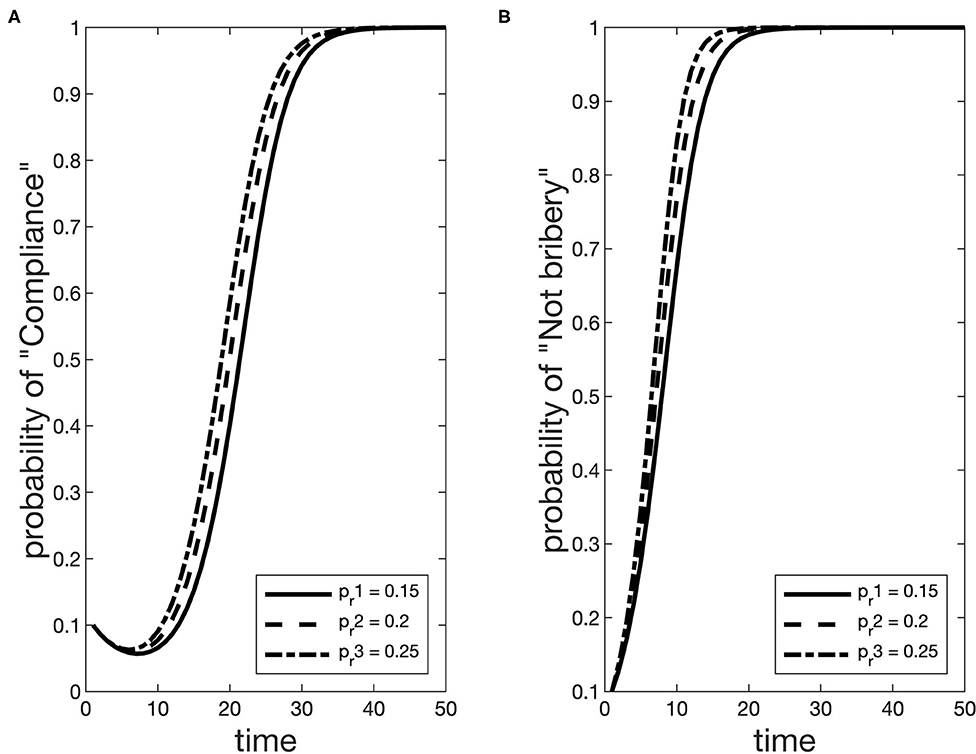
Figure 7. Evolutionary process of the two stakeholders' strategies with different values of pr. (A) is the dynamic strategy of the enterprises, and (B) is the dynamic strategy of the regulatory agencies.
As shown in Figure 8, the role of the rewards e to the regulatory agencies in detecting the non-compliant behavior in the electricity market is similar to the role of the psychological cost of the regulatory agencies pr. If the regulatory agencies will get higher rewards e when they find the non-compliance behavior of the power enterprises, the regulatory agencies have more incentive to inspect the behavior of power enterprises strictly. As a result, the probability that regulatory agencies choose the strategy “Not bribery” will approach to 1 faster. Besides, as the power enterprises know that their actions will be strictly supervised by the regulatory agencies, the probability of choosing strategy “Compliance” for power enterprises will be higher.
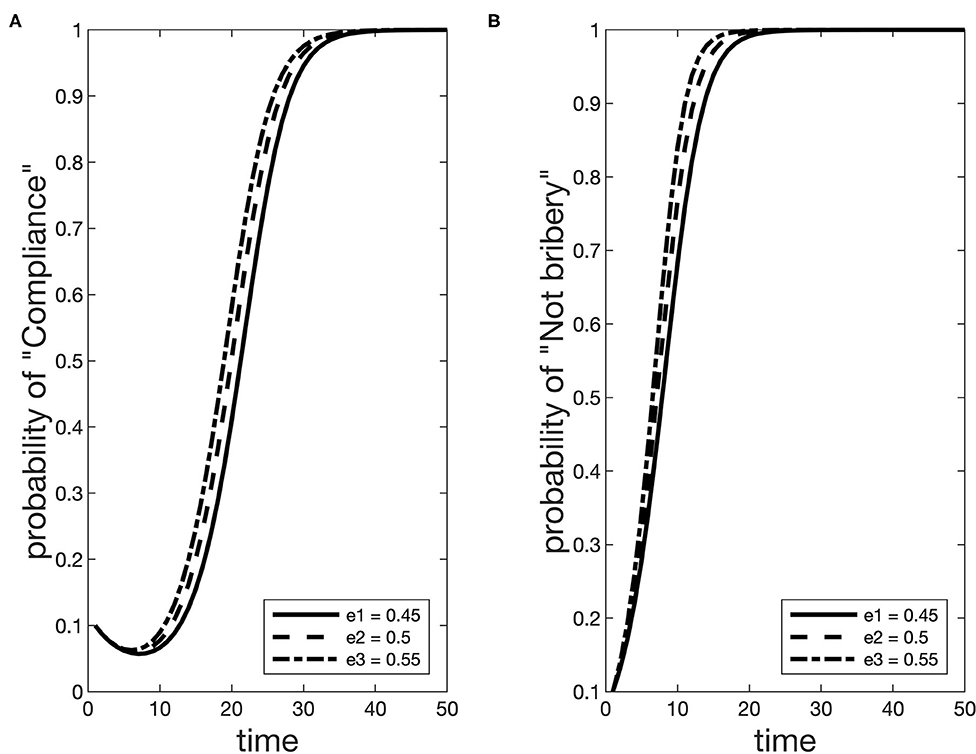
Figure 8. Evolutionary process of the two stakeholders' strategies with different values of e. (A) is the dynamic strategy of the enterprises, and (B) is the dynamic strategy of the regulatory agencies.
The impact of the fines f charged by the central government for non-compliance behaviors on the two stakeholders' strategy selection process is specifically shown in Figure 9. We can find that the change of the fines charged by the central government f has a greater impact on the decision-making of power enterprises, but less on the strategic behavior of regulatory agencies. In addition, the higher the fine charged by the central government, the more effective it can be to promote the power enterprises and regulatory agencies to choose the strategy “Compliance” and the strategy “Not bribery,” respectively.
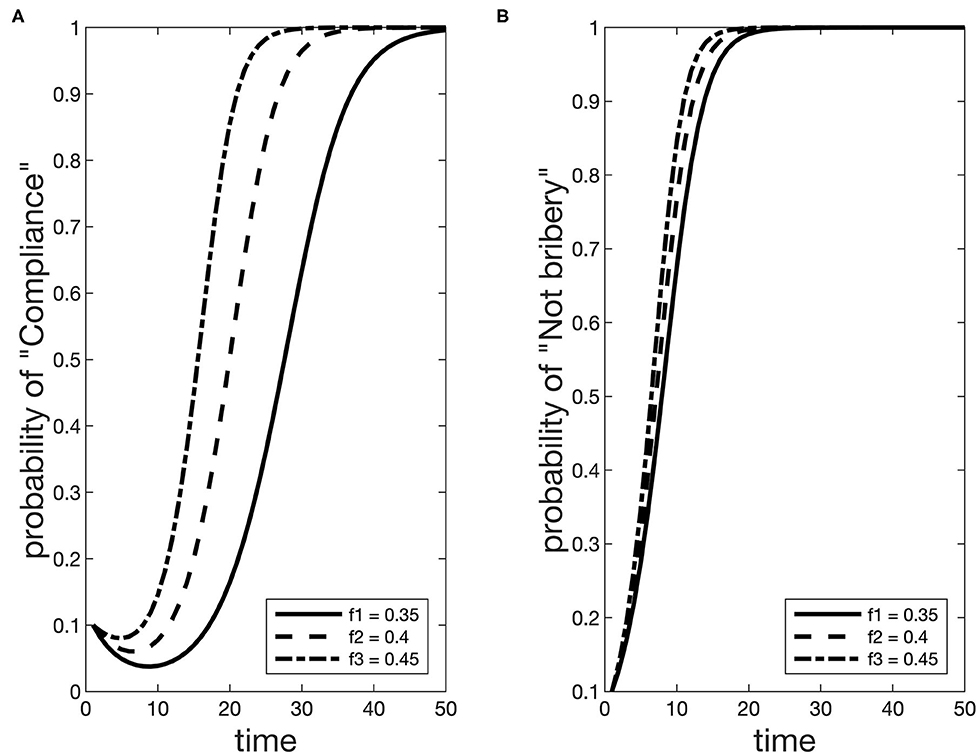
Figure 9. Evolutionary process of the two stakeholders' strategies with different values of f. (A) is the dynamic strategy of the enterprises, and (B) is the dynamic strategy of the regulatory agencies.
In Figure 10, we describe the influence of the coefficient λ of fine in the unilateral bribery on the evolutionary process of the two stakeholders' strategies. It can be seen that compared with the impact on the regulatory agencies, the change of the coefficient λ of fines in the unilateral bribery has a greater impact on power enterprises. If the power enterprises carry out unilateral bribery, the punishment of the central government will be greater, and the power enterprises are more inclined to implement the strategy “Compliance” and avoid heavier fines.
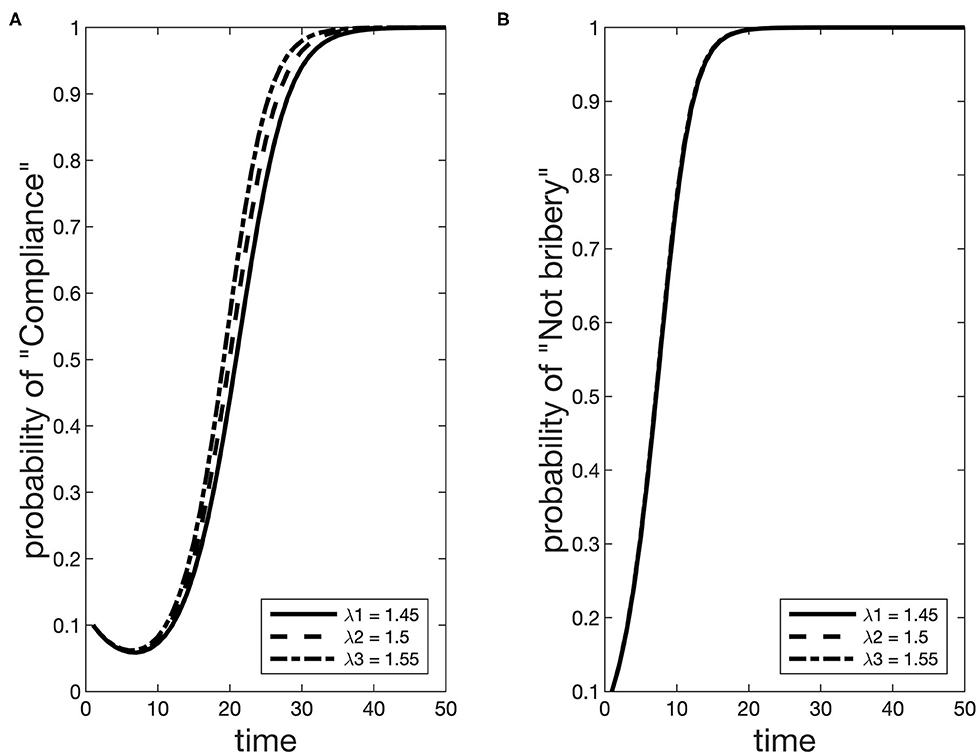
Figure 10. Evolutionary process of the two stakeholders' strategies with different values of λ. (A) is the dynamic strategy of the enterprises, and (B) is the dynamic strategy of the regulatory agencies.
Further Discussion
From the results of sensitivity analysis, we can find that the impacts of different model parameters on the dynamic process of power enterprises and regulatory agencies' strategy selections are inconsistent. From the perspective of power enterprises, the compliance costs c, psychological cost pe, and profit loss r are vital to the dynamic evolutionary process. The increase in compliance costs is not conducive to the implementation of compliance management in the electricity market. When the costs of compliance increase, power enterprises tend to choose the strategy “No compliance,” which leads to the slow evolutionary process of power enterprises to the choice of compliance. Moreover, if the psychological cost of strategy “No compliance” is large enough, the power enterprises will tend to choose the strategy “Compliance.” Besides, once the non-compliance behavior of power enterprises is disclosed, their reputation and profit will be seriously damaged. The higher the profit loss of power enterprises, the more likely they are to choose the strategy “Compliance.” From the perspective of regulatory agencies, the bribery profits b, inspection costs s, psychological cost pr, and rewards e are important. When the regulatory agencies face the greater temptation of bribery, they are more inclined not to seriously perform their regulatory duties. The higher inspection costs are not conducive to the implementation of the compliance management of the power market. The regulatory agencies, as well as power enterprises, would decrease the probability of choosing the strategies of compliance management. The psychological costs of regulatory agencies when they choose the strategy “Bribery” will also influence their choices. The higher psychological costs can promote the compliance management of the electricity market. Moreover, once the regulatory agencies discover the non-compliance behavior of the power enterprises, the government will give rewards to the regulatory agencies, and the higher rewards are conducive to the compliance management of the electricity market. From the perspective of the central government, the fines f and the coefficient λ of fine will help to affect the dynamic evolutionary process. The amount of penalty imposed by the government on power enterprises and regulatory agencies can affect the decision-making of these two stakeholders. When the fines are higher, the power enterprises are more inclined to choose the strategy “Compliance,” and the regulatory agencies will tend to choose the strategy “No bribery.”
Conclusion and Policy Suggestion
This paper attempts to analyze how to effectively promote compliance management in the electricity market. By constructing the evolutionary game model of two stakeholders (power enterprises and regulatory agencies) in the electricity market, the ESS under different scenarios is analyzed, i.e., the scenario without governmental supervision and the scenario with governmental supervision. Moreover, we further make a comparative analysis with different scenarios; the results show that, in the absence of government supervision, the long-term stable equilibrium of power enterprises' choice of strategy “Compliance” and regulatory agencies' choice of strategy “Not bribery” cannot be realized. Only if the government effectively supervises the compliance management of the electricity market can the ideal compliance management of the electricity market be realized. Moreover, we also analyze the roles of different model parameters in the dynamic evolutionary process. The relevant results show that the lower compliance cost, the higher psychological cost, and the larger profit loss of the power enterprises, the lower inspection cost, the higher psychological cost, and the larger rewards of the regulatory agencies, as well as the larger penalty charged by the government, are conducive to the compliance management of the electricity market.
Therefore, to promote the effective implementation of compliance management in the electricity market, the central government should focus on the following aspects. (1) The government should consider taking some measures to reduce the compliance costs of power enterprises and encourage the power enterprises to choose compliance management in the business operation. (2) The government should strengthen the recognition and implementation of compliance management in the electricity market, to make sure that there will be greater psychological pressure if the power enterprises choose the strategy “No compliance,” so as to promote the compliance behavior of power enterprises more validly. (3) The government can disclose information of non-compliance power enterprises publicly, so as to give full play to the effective supervision role of the society on compliance management and jointly resist the occurrence of non-compliance in the electricity market. (4) The government should take some measures to reduce the inspection costs of the regulatory agencies to perform their duties and encourage the regulatory agencies to actively inspect the behavior of the power enterprises, rather than colluding with power enterprises. (5) The government should consider conducting stricter supervision on the works of the regulatory agencies, which can stimulate the regulatory agencies to perform their duties strictly. (6) Besides, the government should increase the incentive payments to the regulatory agencies to stimulate their motivation. (7) Last but not least, the government should increase the punishment of non-compliance behavior in the electricity market.
This paper also has some shortcomings. Due to the limitation of data, this paper uses randomly generated data rather than the real data in the simulation. Thus, it can only analyze the dynamic strategy changes of the compliance management in the electricity market, not the real situation of China's electricity market. With the increasing development of compliance management in China's electricity market, we aim to make a detailed analysis of compliance management in the electricity market through real data in future research.
Data Availability Statement
The datasets generated in this study are available directly from the corresponding author.
Author Contributions
LJ and YL: conceptualization, methodology, and writing—original draft. XW: software, data curation, and writing—review and editing. CC and YC: conceptualization, methodology, writing—original draft, writing—review and editing, and supervision. All authors have read and agreed to the published version of the manuscript.
Funding
This work was supported by the project of Zhejiang Electricity Trading Center Co., Ltd. (Research and Design of Power Market Compliance Management System, Contract number SGZJDJ00JSJS1900024), National Natural Science Foundation of China (grant numbers 71874149, 71934001), and National Social Science Fund of China (grant number 20ZDA084).
Conflict of Interest
LJ, CC, and XW were employed by the company Zhejiang Electricity Trading Center Co., Ltd. YL was employed by the company Zhejiang Huayun Mdt Info Tech Ltd.
The remaining author declares that the research was conducted in the absence of any commercial or financial relationships that could be construed as a potential conflict of interest.
References
Aquila, G., Junior, P. R., Pamplona, E. D. O., and de Queiroz, A. R. (2017). Wind power feasibility analysis under uncertainty in the Brazilian electricity market. Energy Econ. 65, 127–136. doi: 10.1016/j.eneco.2017.04.027
Bahrami, S., and Amini, M. H. (2018). A decentralized trading algorithm for an electricity market with generation uncertainty. Appl. Energy 218, 520–532. doi: 10.1016/j.apenergy.2018.02.157
Coulon, M., Powell, W. B., and Sircar, R. (2013). A model for hedging load and price risk in the Texas electricity market. Energy Econ. 40, 976–988. doi: 10.1016/j.eneco.2013.05.020
da Silva Rocha, A. B., and Salomão, G. M. (2019). Environmental policy regulation and corporate compliance in evolutionary game models with well-mixed and structured populations. Eur. J. Oper. Res. 279, 486–501. doi: 10.1016/j.ejor.2019.05.040
Deng, S. J., and Oren, S. S. (2006). Electricity derivatives and risk management. Energy 31, 940–953. doi: 10.1016/j.energy.2005.02.015
Friedman, D. (1991). Evolutionary games in economics. Econometrica 59, 637–666. doi: 10.2307/2938222
Gu, W., Wei, L., Zhang, W., and Yan, X. (2019). Evolutionary game analysis of cooperation between natural resource- and energy-intensive companies in reverse logistics operations. Int. J. Prod. Econ. 218, 159–169. doi: 10.1016/j.ijpe.2019.05.001
Höhne, S., and Tiberius, V. (2020). Powered by blockchain: forecasting blockchain use in the electricity market. Int. J. Energy Sect. Manag. 14, 1221–1238. doi: 10.1108/IJESM-10-2019-0002
Ji, P., Ma, X., and Li, G. (2015). Developing green purchasing relationships for the manufacturing industry: an evolutionary game theory perspective. Int. J. Prod. Econ. 166, 155–162. doi: 10.1016/j.ijpe.2014.10.009
Jiang, B., Wang, X., Xue, H., Li, J., and Gong, Y. (2020). An evolutionary game model analysis on emission control areas in China. Mar. Policy 118:104010. doi: 10.1016/j.marpol.2020.104010
Jiang, K., You, D., Merrill, R., and Li, Z. (2019). Implementation of a multi-agent environmental regulation strategy under Chinese fiscal decentralization: an evolutionary game theoretical approach. J. Clean. Prod. 214, 902–915. doi: 10.1016/j.jclepro.2018.12.252
Kharrati, S., Kazemi, M., and Ehsan, M. (2016). Equilibria in the competitive retail electricity market considering uncertainty and risk management. Energy 106, 315–328. doi: 10.1016/j.energy.2016.03.069
Klessmann, C., Nabe, C., and Burges, K. (2008). Pros and cons of exposing renewables to electricity market risks—a comparison of the market integration approaches in Germany, Spain, and the UK. Energy Policy 36, 3646–3661. doi: 10.1016/j.enpol.2008.06.022
Meunier, G. (2013). Risk aversion and technology mix in an electricity market. Energy Econ. 40, 866–874. doi: 10.1016/j.eneco.2013.10.010
Ritzberger, K., and Weibull, J. W. (1995). Evolutionary selection in normal-form games. Econometrica 63, 1371–1399. doi: 10.2307/2171774
Selten, R. (1980). A note on evolutionarily stable strategies in asymmetric animal conflicts. J. Theor. Biol. 84, 93–101. doi: 10.1016/S0022-5193(80)81038-1
Sheng, J., Zhou, W., and Zhu, B. W. (2020). The coordination of stakeholder interests in environmental regulation: lessons from China's environmental regulation policies from the perspective of the evolutionary game theory. J. Clean. Prod. 249:119385. doi: 10.1016/j.jclepro.2019.119385
Song, M., Fisher, R., and Kwoh, Y. (2019). Technological challenges of green innovation and sustainable resource management with large scale data. Technol. Forecast. Soc. Change 144, 361–368. doi: 10.1016/j.techfore.2018.07.055
Sun, Z., and Zhang, W. (2019). Do government regulations prevent greenwashing? an evolutionary game analysis of heterogeneous enterprises. J. Clean. Prod. 231, 1489–1502. doi: 10.1016/j.jclepro.2019.05.335
Taylor, P. D., and Jonker, L. B. (1978). Evolutionary stable strategies and game dynamics. Math. Biosci. 40, 145–156. doi: 10.1016/0025-5564(78)90077-9
Tian, Y., Govindan, K., and Zhu, Q. (2014). A system dynamics model based on evolutionary game theory for green supply chain management diffusion among Chinese manufacturers. J. Clean. Prod. 80, 96–105. doi: 10.1016/j.jclepro.2014.05.076
Uribe, J. M., Mosquera-López, S., and Guillen, M. (2020). Characterizing electricity market integration in Nord Pool. Energy 208:118368. doi: 10.1016/j.energy.2020.118368
Vehviläinen, I., and Keppo, J. (2003). Managing electricity market price risk. Eur. J. Oper. Res. 145, 136–147. doi: 10.1016/S0377-2217(01)00399-X
Xie, H., Cheng, L., and Lv, T. (2017). Factors influencing farmer willingness to fallow winter wheat and ecological compensation standards in a groundwater funnel area in Hengshui, Hebei Province, China. Sustainability 9:839. doi: 10.3390/su9050839
Keywords: compliance management, electricity market, evolutionary game model, governmental supervision, strategy behavior
Citation: Jin L, Chen C, Li Y, Wang X and Cheng Y (2021) How to Promote Compliance Management in the Electricity Market? An Analysis Based on the Evolutionary Game Model. Front. Environ. Sci. 9:655080. doi: 10.3389/fenvs.2021.655080
Received: 18 January 2021; Accepted: 23 February 2021;
Published: 03 May 2021.
Edited by:
Yongping Sun, Hubei University of Economics, ChinaReviewed by:
Chang Liu, Southwestern University of Finance and Economics, ChinaJianghua Liu, Shanghai University of Finance and Economics, China
Copyright © 2021 Jin, Chen, Li, Wang and Cheng. This is an open-access article distributed under the terms of the Creative Commons Attribution License (CC BY). The use, distribution or reproduction in other forums is permitted, provided the original author(s) and the copyright owner(s) are credited and that the original publication in this journal is cited, in accordance with accepted academic practice. No use, distribution or reproduction is permitted which does not comply with these terms.
*Correspondence: Yuanyuan Cheng, yycheng_xmu@sina.com