- 1Centre for Environmental and Marine Studies (CESAM), Department of Environment and Planning, University of Aveiro, Aveiro, Portugal
- 2MARETEC–Marine, Environment and Technology Centre, Instituto Superior Técnico, LARSyS, Universidade de Lisboa, Lisbon, Portugal
- 3European Commission, Joint Research Centre (JRC), Ispra, Italy
Wildfires are an increasing threat in the Mediterranean region, causing frequent losses of goods and human lives. Not only are wildfires a concern due to their immediate effects on vegetation and soil, but they can also have substantial impacts on surface water quality. Approximately one-third of the world’s largest cities obtain their drinking water from forest catchments. The removal of vegetation and consequent runoff increase with a high concentration of ash and sediment often leads to increased nutrient and contaminant loads to downstream reservoirs, damaging the aquatic ecosystem and threatening human health. This study focused on the post-fire degradation of surface water in Castelo de Bode reservoir, a strategic freshwater supply for Lisbon’s metropolitan area (2,000,000 inhabitants), Portugal. Output data from the catchment model Soil and Water Assessment Tool were used as inputs to the CE-QUAL-W2 reservoir model. CE-QUAL-W2 was then calibrated for water level, temperature, nutrients, total suspended solids, chlorophyll-a, and dissolved oxygen. The post-fire impacts were assessed by adjusting land use features (curve number, crop vegetation management factor), and soil properties (soil erodibility) in the catchment model, considering the different impacts of fire (low, medium, and high severity). The reservoir model was able to perform temperature seasonality and stratification while a weak performance was found for chlorophyll-a probably for having considered only a group of algae. Simulations showed a deterioration of water quality at the dam wall during the first year after the forest fire. Nevertheless, contamination did not appear worrisome with regards to water quality standards likely due to the capability of the reservoir to attenuate inflow concentrations.
Introduction
Forested watersheds are world’s primary source of freshwater supply (Reid et al., 2005). The particularity of the Mediterranean climate, land cover uniformity, and new socio-economic drivers make those areas especially prone to wildfires (Moreira et al., 2001, 2011; Chuvieco, 2012; Turco et al., 2014; Viedma et al., 2015; Nunes J. P. et al., 2018a, Nunes et al., 2018b). Wildfire disturbance can affect the water quality and quantity of water resources by enhancing overland flow, increasing the availability of ash and debris, and disrupting the soil nutrient cycle (Shakesby and Doerr, 2006; Sheridan et al., 2007; Nyman et al., 2010; Emelko et al., 2011; Smith et al., 2011; Bladon et al., 2014). The burning of tree canopies, understory, and the dead mantle layer leave the soil unprotected and susceptible to erosion (Ferreira et al., 2005; Vieira et al., 2014; Shakesby et al., 2016). Degradation of soil properties after forest fires further leads to a decrease of soil infiltration capacity, promoting runoff, and a faster flow of water into streams (Robichaud, 2000; Moody and Martin, 2001; Keizer et al., 2008; Nyman et al., 2010; Shakesby, 2011).
Runoff and atmospheric transportation of ash, together with soil erosion, are the main causes of nutrient enhance in waterbodies (Smith et al., 2011; Bladon et al., 2014). The increase of nutrient concentrations, especially phosphorus and nitrogen, in streams after a wildfire is largely documented in the literature (Spencer and Hauer, 1991; Sheridan et al., 2007; Bladon et al., 2008; Emelko et al., 2011; Smith et al., 2011; Rhoades et al., 2019). Consequently, fires can affect downstream reservoirs, with the magnitude of impact depending on the interplay of several variables such as ground cover, climate, geology, and topography. The size of reservoirs also influences the dissolution capacity of contaminants, with different consequences among studies encountered in the literature. If on one hand, increased amounts of inorganic sediment were reported to be deposited in a reservoir in the aftermath of a wildfire, jeopardizing the correct functioning of water supply treatment processes (White et al., 2006); on the other, studies showed that sediment concentrations remained within the same limits of pre-fire conditions after those disturbances (Lathrop, 1994). Enhanced nutrient concentrations in reservoir/lake waters have also been reported in several other studies (Carignan et al., 2000; Pinel-Alloul et al., 2002; Alexander et al., 2004; Evans et al., 2017), ranging from negligible to 7-fold increases when compared to the pre-fire conditions.
Emmerton et al. (2019) and Alexander et al. (2004) disclosed a greater variation in nutrient concentration in input streams than in lake-waters, presumably reflecting the capacity of large waterbodies to absorb the impacts from contaminated inflows. Still, the impact of wildfires on the quality of downstream water bodies is difficult to assess, mostly due to the lack of adequate tools to address the complex interrelationships between wildfires’ magnitude and water, sediment, and nutrient dynamics at the catchment scale. In such context, the combined use of hydrological and water quality models is a good candidate for creating an integrated study of water quality changes in a reservoir in the aftermath of a wildfire.
The Soil and Water Assessment Tool (SWAT) is a watershed-based model widely used to simulate the impact of land use, land management practices, and climate changes on river flow and constituent transport (Arnold and Fohrer, 2005; Neitsch et al., 2011). The model was previously used to estimate hydrological and water quality changes in the upstream basin of the Castelo de Bode reservoir (Basso et al., 2020). Although SWAT can simulate water quality processes in lakes and reservoirs, the limitation of its steady-state 1-D empirical equations does not make it suitable to adequately describe those processes in large waterbodies. The CE-QUAL-W2 hydrodynamic and water quality model can perform 2-D analysis in large waterbodies, simulating the transformations and interactions of several constituents within the studied volume (Cole and Wells, 2006). In this modeling exercise, the output data from the SWAT model implemented by Basso et al. (2020) was used as inlet values for the CE-QUAL-W2 model (Cole and Wells, 2006) applied to the Castelo de Bode reservoir. The benefit of the combined use of these two models has been already established by few researchers (Flowers et al., 2000; White et al., 2004; Debele et al., 2008; White et al., 2010), confirming the competence of the integrated approach to develop land management and climate changes scenarios.
The present study aims to implement an integrated model and assess the potential post-fire impacts in a large waterbody used to supply drinking water to a great part of the Portuguese population. To do so, this work evaluates the response of a large reservoir to upstream disturbance, assessing the degree of contamination at the water supply tower. The specific objectives of this study are: i) to calibrate and validate the reservoir model to predict water level, nutrients, dissolved oxygen, chlorophyll-a, and sediment concentrations; ii) to model post-fire contaminated inflows impact on reservoir water quality and quantity; and iii) to assess whether the high concentrations observed at the reservoir inlets (Basso et al., 2020) are also maintained at the water intake tower, jeopardizing the proper operation of the treatment processes.
Materials and Methods
Study Area
The reservoir considered in our study was Castelo de Bode (39°54′33″ N, 8°31′88″ W), in central Portugal (Figure 1A). It started to operate as one of the main reservoirs in the country in 1951, fulfilling several functions which included the production of energy, recreational activities, and the supply of water to Lisbon’s metropolitan area (approximately 2,000,000 inhabitants). The reservoir is located in the Zêzere River watershed, one of the main tributaries of the Tagus River. The dam is in the Santarém municipality, approximately 7 km upstream of the confluence with the Tagus river. The Castelo de Bode reservoir has a total capacity of 1,095 hm3 and a useful capacity of 902 hm3. The flooded area covers 3,291 ha when at the maximum storage level (121.5 m). The drainage area of the main body is equal to 2,376 km2, while the two secondary branches have a drainage area of 330 and 290 km2. Following national legislation (Ambiente, 1988), the Castelo de Bode reservoir is classified as protected since the water is used for human consumption. It further plays an important role for ecological defense reasons.
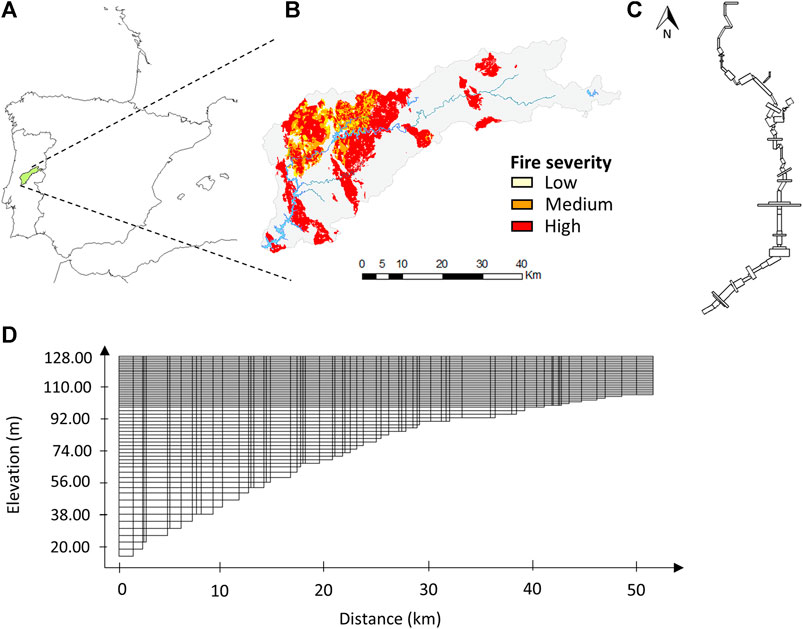
FIGURE 1. (A) Study area location, (B) 2017 burned area extension and fire severity in river Zêzere watershed, (C) plan view and (D) longitudinal profile of Castelo de Bode reservoir.
The climate in the region is Mediterranean, with dry summers and wet winters. The average annual rainfall is less than 1,100 mm, with 75% occurring in the winter season (Ferreira do Zêzere meteorological station 1986-2000; 39°69′20″ N, 8°29′00″ W). The mean air temperature is approximately 14.5°C, with temperatures ranging from a minimum of 6°C in winter and a maximum of 25°C in summer. The average potential annual evapotranspiration is 654 mm (Cernache de Bonjardim meteorological station 1986-2000; 39°81′40″ N, 8°19′00″ W).
Portugal is ranked as having one of the highest burned areas in Europe (San-Miguel-Ayanz et al., 2016), placing wildfires as the main forest disturbance in the country. The national fire hazard map produced by the Institute for the conservation of nature and forests (ICNF), included this region in the high and very high danger classes (ICNF, 2017). The Zêzere River watershed was the scene of several wildfires in the 2017 season, with almost 90,000 ha of the watershed burned that year which lead to several interruptions of the water supply systems due to the elevated concentration of sediments in the streams (Dnoticias., 2017; Leiria, 2017; RTPnotícias, 2017).
Wildfire Scenario
The wildfire scenario considered had the same characteristics of the 2017 season when almost 30% of the area that flows into the reservoir was hit by wildfires. The extension of the burned area, with 88% classified in the high fire severity class, 9% in the moderate, and 3% in the low, are presented in Figure 1B (Basso et al., 2020).
The wildfire scenario was simulated by modifying soil and land use management parameters in the SWAT model. The curve number (CN) of the soil conservation service equation was increased to simulate the enhanced watershed hydrologic response (Nunes et al., 2018a; Nunes et al., 2018b; Havel et al., 2018). To account for fire-induced impacts on soil erosion, the soil erodibility and crop vegetation factors of the USLE equation (Wischmeier and Smith, 1978) were also increased. The magnitude of such model adjustments was monotonically increased according to the fire severity classification, which measures the direct effects on vegetation and soil (Keeley, 2009).
A two-fold increase in the annual runoff was simulated at the subbasin scale, enhancing the discharge peaks that reached the reservoir inlets. Increased CN values limiting the infiltration and the reduced extension of the burned area could explain the small change in the total annual flow characterizing the post-fire scenario. Post-fire scenario simulations showed a high concentration of nutrients and sediment reaching the reservoir, with the phosphate overstepping the national water quality threshold. A decrease in component concentrations was observed between the subbasin and the catchment scale, indicating the variability of processes with spatial scale (Ferreira et al., 2008).
The Hydrodynamic and Water Quality Model CE-QUAL-W2
CE-QUAL-W2 is a two-dimensional, longitudinal and vertical, hydrodynamic, and water quality model (Cole and Wells, 2006). The model assumes negligible lateral variations, making it adequate for studying long and narrow waterbodies. When used in large waterbodies simulations, two-dimensional (2D) models allow the user to obtain results with the same precision as three-dimensional (3D) models (Lima et al., 2009; Hussein and Wells, 2020). The short computational time required by the former makes possible the use of finer meshes, enhancing the quality of the results. The possibility to vary the thickness of layers allows a higher resolution of the simulated processes, improving model performance. Any combination of water quality constituents can be included in the simulations, with over 21 state variables computed by the model. The hydraulic governing equations of the model consist of six laterally averaged equations of the fluid motions to simulate as many unknown variables, that is, free water, pressure, lateral direction velocity, longitudinal velocity, concentration, and density (Cole and Wells, 2006). Water quality parameters are calculated from diffusion, advection, and reactions through constituents as a function of water depth, temperature, and velocity obtained in the hydraulic model.
Model Setup
Reservoir Bathymetry
The capability to simulate multiple branches of a system, as well as the consideration of multiple inlets, made CE-QUAL-W2 appropriate for modeling the Castelo de Bode reservoir. With two narrow branches converging into the main body, the reservoir was dominated by longitudinal gradients throughout the waterbody. The conceptual framework of the study included the base characteristics of the reservoir as the bathymetry, input data as flow and water quality properties, outlet structures, and the weather conditions. The reservoir was represented by 77 longitudinal sections (Figure 1D), all with different widths, lengths, and segment orientations (Figure 1C). Dimensions of the segments were defined by studying the maps of the area before the dam construction (1942), which were obtained from the University of Texas Libraries (US service, 1942). The maps were used to obtain the topography of the valley to find the water depths along the length of the reservoir to build the bathymetry. The segments were established considering the vertical and longitudinal changes of the topography. Therefore, shorter segments were implemented with a rapid change of topography, while longer and wider segments feature a constant terrain. The length of the segments ranged from 100 to 3,000 m, with a width between 50 and 5,000 m. The thickness of the layers was considered variable through depth. Thus, a 1 m thickness layer was chosen for the first 30 m depth, a 2 m thickness layer for the next 34 m depth, and a 3 m thickness for the deepest layers. The Castelo de Bode reservoir was then divided into three branches, one corresponding to the main body and the other two to the tributaries. The bathymetry was validated using the elevation-volume curve provided by the Sistema Nacional de Informação de Recursos Hidricos (SNIRH, 2011), calculating the volumes corresponding to each quota and delineating a cumulative volume curve (Figure 2). An output structure was considered at 70 m below the average water surface level, and an emergency spillway was designed to work when the water level reached the height of full reservoir, equal to 122 m.
Boundary Conditions and Water Quality Inputs
The average daily surface air temperature, dew point temperature, and wind speed were extracted from the meteorological model provided by the National Centers for Environmental Protection (NCEP) (NOAA, 2019) for a 20 years period (1980–2000). These weather data were provided for grid points, equidistant from each other with a global horizontal resolution of 38 km (Fuka et al., 2014). In this study, two points were used. Wind direction (rad) was extracted from Vila de Rei meteorological station (39°67′38″ N, 8°14′99″ W) and was considered homogeneous in the reservoir area. Cloud coverage was provided by the Global Forecast System (GFS 27 km) archive (NOAA, 2019). Solar radiation was computed directly by the model using cloud cover ratios. Outlet flows were obtained from Albufeira de Castelo de Bode hydrological station (39°54′30″ N, 8°31′80″ W), included in the SNIRH network. CE-QUAL-W2 does not consider precipitation as a meteorological variable, but as an inflow value to the reservoir, together with its temperature and constituent concentrations. The hydrological input variables supplied to the system were an underestimation of the total inflow into the reservoir, since potential inputs from runoff, groundwater, and precipitation were not considered due to lack of measurement data. These limitations were adjusted throughout the calibration process by modifying the outlet flow, also to account for potential errors in the outlet discharge.
Input data for the three branches were produced from the output data from three of the fourteen sub-basins characterizing the catchment. Ammonia, nitrate, nitrite, dissolved oxygen, and total suspended solids were directly obtained from the watershed model. The output value of mineral phosphorus was considered as phosphate since it usually occurs in nature as part of the latter (Bhattacharya, 2019). SWAT simulates algae biomass as chlorophyll-a, which is a specific form of chlorophyll that absorbs violet-blue and orange-red light and reflects green-yellow lights. Chlorophyll-a can be used to characterize different algae groups. Johnson et al. (1985) reported percentage of chlorophyll-a compared to dry weight algae biomass ranging from 0.25 to 3, corresponding to a range in algae to chlorophyll-a ratio (mg dry weight organic matter/μg chlorophyll-a) between 0.01 and 0.4. Not subsisting global ratios to differentiate the groups, researchers opted to consider one predominant algae group of the area under study (Debele et al., 2008; Ernst and Owens, 2009). Following this choice, albeit representing a gross approximation, only one group was considered in this study. Following the data collected by Caramujo et al. (2007) in the Zêzere River watershed, diatoms were considered as the main algae group characterizing the Castelo de Bode reservoir.
Organic matter transport in streams was obtained using the stoichiometric ratio between organic-N and organic matter (OM) or organic-P and OM. Assuming a constant ratio of 0.08 between organic-N and OM (Cole and Wells, 2006; Debele et al., 2008), the fraction of organic-P in OM was estimated using the following equation:
where ORGN and ORGP are the stoichiometric fractions between OM and the organic-N and -P, respectively. The second member of the equation represents the cumulative organic-N and organic-P loads estimated by the SWAT model over the simulation period (1986-2000), equal to 5.86 × 107 and 1.19 × 107 kg, respectively. Solving Eq. 1, the stoichiometric ratio for organic-P was equal to 0.0162. OM was then calculated using the following equation:
Organic matter in CE-QUAL-W2 was modeled by considering four different pools such as labile dissolved organic matter (LDOM), refractory dissolved organic matter (RDOM), labile particulate organic matter (LPOM), and refractory particulate organic matter (RPOM). When sediment concentration was smaller than 100 mg L−1, under low and base flow conditions, 40% of the OM was considered as in particulate form. Whereas, under storm flow conditions, with sediment concentration greater or equal to 100 mg L−1, 75% of the OM was assumed to be in particulate form. The correct assignment between labile and refractory form is not unique, based almost always on an educated guess (Debele et al., 2008). To divide the OM into the two categories, it was assumed that the majority reaching the reservoir (75%) was in the refractory form (Debele et al., 2008).
Calibration and Validation
Flow and water quality data at the reservoir inlet were provided by the SWAT model. Input data were previously calibrated for a 12-year period (1984–1996) and then validated for a 4-year period (1996-2000) (Basso et al., 2020). Inlet data from the upstream basins flowing to each branch were inserted into the model. CE-QUAL-W2 was then calibrated (1988–1996) and validated (1996–2000) using water level data measured at the Albufeira de Castelo de Bode hydrological station (39°45′50″ N, 8°32′30″ W), and the superficial water temperature data measured at Albufeira de Castelo de Bode surface water quality station (39°54′46″ N, 8°31′71″ W). Calibration followed an “iterative trial and error” procedure, which consisted of adjusting model parameters one at a time until deviations between measured and simulated properties were minimized. Model calibration was performed by changing different parameters related to both physical and ecological processes in the reservoir (Table 1). Several parameters controlling the algal life cycle, like growth or nutrients and temperature preference, were user-defined. Control file parameters related to algae were chosen from the range of values for freshwater diatoms given in the literature. Other coefficients were shaped around existing values from studies in analog systems. A warm-up period of four years was considered to ensure model stability. Data on water surface level were available daily for the first 4 years (1988–1991) and monthly for the following 8 years. Besides a graphical analysis, model performance for water level was evaluated using statistical parameters as the coefficient of determination (R2), the Nash-Sutcliffe model efficiency coefficient (NSE), and the percentage bias (PBIAS).
Water quality parameters were calibrated using measured data at the Albufeira de Castelo de Bode surface water quality station (39°54′46″ N, 8°31′71″ W). The study was calibrated for nutrient, nitrate and phosphate, total suspended sediment, chlorophyll-a, and dissolved oxygen. Due to the insufficient data for meaningful statistical analyses, model performance relied mostly on the comparison between simulated and measured average, standard deviation, median, the root-mean-square error (RMSE), and PBIAS for the period 1989–2000. PBIAS limits for water quality parameters differed from the streamflow ones. For flow data, values from 0 to 5% were considered excellent and until 15% were considered satisfactory (Moriasi et al., 2015). For water quality, values smaller than 20% for sediment and 30% for nutrients were considered satisfactory. The minimum value detected for phosphate concentration was 0.0087 mgPO4-P L−1, imposed by the sensor measurement limits. This threshold was thus also considered for model calibration while in the fire scenario all simulated values were taken into consideration.
The effects of fires were studied in the surface layer closer to the dam wall, where the water supply tower was located. The year 1998 was assumed to be representative of precipitation long term average in the region (1980–2000) (PORDATA, 2019) and was thus considered for the comparison between the two scenarios. Scenario analysis was performed for the first year after wildfires by simulating only the parameters that best fitted the measurements during model calibration and validation. Thus, water level, TSS concentration, nitrate, and phosphate were considered.
Results
Model Calibration and Validation
A good agreement was achieved between simulated water level and field data (Figure 3) suggesting that the model was able to reproduce observed water level variation. The model statistics parameters supported these findings, with statistical performance measures scoring good for both calibration and validation periods (Table 2).
Simulated surface temperature presented the same seasonal pattern as observed field measurements (Figure 4) but was generally underestimated during the spring periods. When considering the RMSE and PBIAS indicators, the model showed the capacity to correctly simulate temperature (Table 3). The water temperatures simulated the typical summer thermal stratification, with higher temperatures in the epilimnion and a gradual decrease with depth (metalimnion), leading to the lowest temperatures at the bottom layers (hypolimnion). The year-round pattern of reservoir temperature stratification cycle was adequately simulated, as seen by the comparison with the observed data at four depths (surface, 5, 15, and 30 m) in winter (Figure 5A), spring (Figure 5B), and summer (Figure 5C).
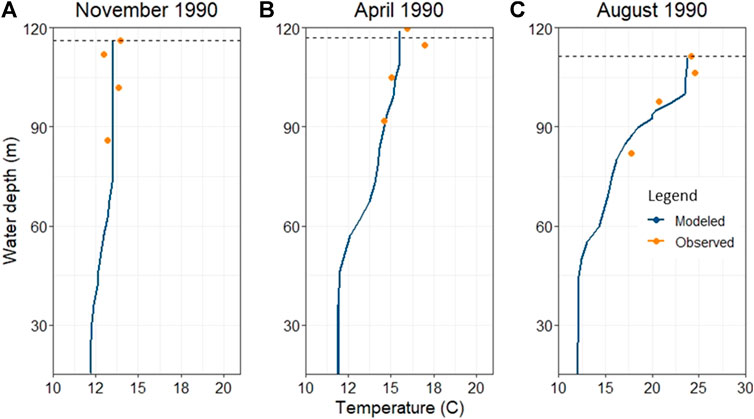
FIGURE 5. Water temperature comparison through depth for different seasons (A) autumn, (B) spring, and (C) summer. Y axis represent the elevation from the bottom of the dam.
Average estimations of TSS concentration showed a correspondence between modeled and observed data, the RMSE-value revealed an acceptable reproduction of measured data. Standard deviation indicated that simulated concentrations deviated more from the average compared to measurements, as seen by the maximum in Figure 6A. Chlorophyll-a simulated values presented an average, standard deviation of the same order of magnitude of the observed data (Figure 6B) while high RMSE-value showed a weak correlation between the data. The model presented an agreement with dissolved oxygen values (Figure 6C), low values of RMSE showed a good correlation between data (Table 3).
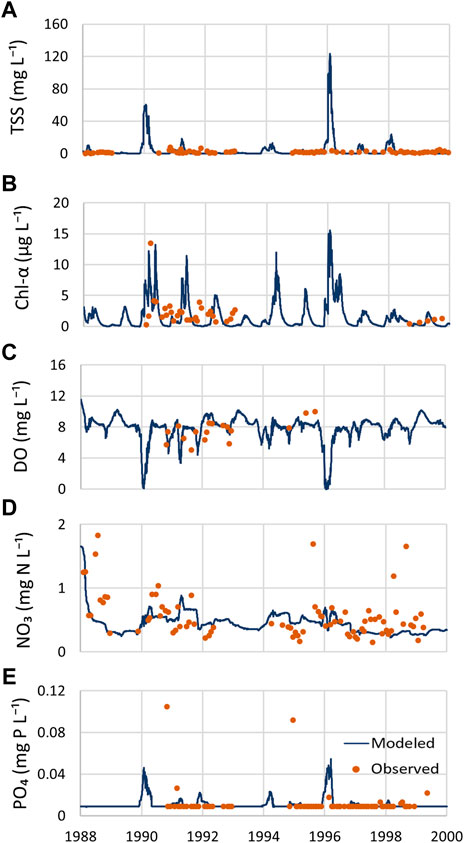
FIGURE 6. Modeled and observed data comparison for (A) sediment, (B) oxygen, (C) phosphate, (D) nitrate, and (E) chlorophyll-a.
Model performance improved for nutrient concentrations, attaining small RMSE values for the nitrate/nitrite and phosphate, respectively. Simulated concentrations of nutrients (Figures 6D,E) showed consistency when considering the average and the median values the same median as field observations (Table 3). Considering the PBIAS value, all water quality parameters presented a “very good” or “good” model performance (Table 3).
Wildfire Scenario
The wildfire scenario showed a small decrease in the water level from 118 m in the reference scenario (without wildfire) to 117 m after the impacts of fire. The changes induced by the wildfire also led to the decrease of the minimum water level from 114 to 113.2 m, and the maximum water level from 121 m in the reference conditions to 120 (Figure 7).
The wildfire scenario presented a considerable increase in the TSS median, 4.7 times the reference value, and minimum value which increase from 0.011 to 0.16 mg L−1. The maximum value also endured an increase, but of lesser significance. The total annual yield per liter was 1.5 times the reference scenario, moving from 1,192 to 1791 mg L−1. The increase in the TSS along the simulated period followed the peaks in inlet flow rates.
Nitrate concentration also presented a considerable increase when compared with the reference scenario. A total yield of 390 mg N-NO3 L−1 was simulated in the fire scenario and the maximum concentration increased 4 times from 0.30 mg N- NO3 L−1 to 1.49 N- NO3 L−1. Unlike what was observed for the TSS, a continuous increase during the simulated period interested the nitrate.
The changes in the maximum phosphate concentration matched those in nitrate, increasing four times (from 0.014 to 0.06 mg P-PO4 L−1 in the fire scenario). The total yield in the fire scenario resulted almost 4-fold greater in comparison to the reference scenario (Figure 8). The increase in phosphate concentration followed the TSS trend being soil erosion a major source of phosphorus in the waters.
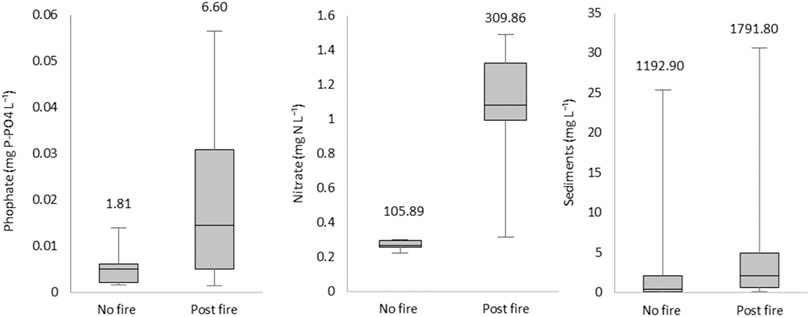
FIGURE 8. Comparison between the scenario post-fire and the reference scenario for the year 1998. The total concentration per liter is present on top of each box-plot.
Discussion
Model Performance
Model simulations produced a satisfactory estimation of the two most relevant physical properties controlling the dynamic of the reservoir, namely, the water level and temperature. Only slight deviations were observed for water level (Figure 4), most probably generated by the model domain geometry having small differences from the real bathymetry. Simulated water levels correctly followed the observed data trend as seen in the performed statistical analysis (Table 2). A more detailed determination of the error between observed and modeled data was possible when daily observed data were available. A difference of 15 cm between the observed and the simulated data was considered acceptable and all statistical parameters confirmed a “very good” model efficiency (Moriasi et al., 2015).
It is important to stress that a complete statistical analysis in water quality calibration was not possible, due to data limitation and non-homogeneity. Differences between observed and measured data were expected since instantaneous measures (observed data) were compared to daily simulated values. Model results denoted a correct depiction of the seasonal temperature fluctuations and thermal amplitude, suggesting that the forcing prescribed for air temperature, solar radiations, and wind action was adequate (Figure 4). Figure 5 showed that the model was able to simulate the vertical thermal structure of the reservoir, characterized by the summer stratification and the winter homogeneous profile. Still, a better agreement with the observed data corresponded to the availability of daily water level data. CE-QUAL-W2 includes the water temperature in the hydrodynamic subroutine of the model. The transport of heat in the river is described by an advection-diffusion equation, function of the heat flux penetrating through the depth, the density, and the volume of the fluid. The minor detail caused by the lower resolution of the flow data may have caused a greater fluctuation of the hydrograph with a lower resolution of the vertical discretization of the model (Cole and Wells, 2006; Norton and Bradford, 2009). This may have had repercussions in the simulation of the surface temperature, causing the peaks to be underestimated in the validation period, where only monthly water level data were available.
Total suspended sediment concentration simulated by the model presented two peaks coincident with greater rainfall events, which transported deposited material that had accumulated in the watershed (Figure 6A). These two peaks were also present for the dissolved oxygen (Figure 6C) and the phosphate trends (Figure 6E). Phosphorus is inherently bound to sediment particles, thus the increase of suspended sediment concentration led to an increase of phosphorus related compounds. The loss of oxygen leads to the release to the water column of phosphorus previously trapped in the sediments, enhancing its availability in the dissolved form. These peaks were not detected by observed data, and the absence of such observations contributed to reduce the predictability of the model. The phosphate measurement sensor did not detect values lower than 0.0087 mgPO4-P L−1 raising difficulties during the calibration process. Average concentrations lower than 0.017 mgPO4-P L−1 were detected in Castelo de Bode reservoir (INAG, 2011) and lower than 0.02 mgPO4-P L−1 for Enxoé reservoir in Portugal (Brito et al., 2018), confirming that the CE-QUAL-W2 model correctly simulates surface phosphate concentration. In what concerned nitrate/nitrite concentrations, the model seemed to underestimate this parameter. Debele et al. (2008) attributed the underestimation of nitrate/nitrite concentration in the reservoir to the low loads from the watershed estimated by SWAT. CE-QUAL-W2 underestimation of nitrate/nitrite was already discussed for another Portuguese reservoir (Brito et al., 2018). From Figure 6D it can be observed that measured data presented three outlier values, which could have a significant weight in the final validation.
The simulated surface dissolved oxygen was well represented, including the sudden decrease during algae blooms measured in the field and modeled (Figure 6B). The average values correctly depicted the usual trend of dissolved oxygen throughout the year (INAG, 2011). However, the scarcity of observed data prevented the use of this parameter to evaluate the effects of the fires. Even if the statistical analysis showed good results for chlorophyll-a (Table 3), poor graphical replication of the parameter is depicted in Figure 6B. The temporal series of observed data had a considerable year-round variation, unlike model results that showed significant seasonal variation. Water residence time in the dam might be influenced by the discharges to produce electric energy, conditioning the normal growth of algae. Moreover, representing the algae community as one group may also have contributed to the poor performance of CE-QUAL-W2 (Debele et al., 2008). Nevertheless, the model was able to simulate the oligotrophic behavior of Castelo de Bode (INAG, 2011). Finally, given the complexity of both models used in this study, particularly regarding their number of parameters, there is a high degree of uncertainty in the results. For instance, the use of limited observed data for the model calibration could lead to uncertainty in parameters estimates (McIntyre et al., 2002). Besides the models themselves, additional sources of uncertainty are found on the measuring methodologies and the input data (Pechlivanidis et al., 2011). Consequently, the uncertainty in model inputs can have a very large impact on the modeling results, and although most of the water quality variables have been satisfactorily simulated by the models, the equifinality is inevitable.
Wildfire Scenario
The increase of peak flows due to the post-fire enhanced runoff has already been acknowledged (Shakesby and Doerr, 2006). When an event with relatively large precipitation occurs, the mass of surface water displaced to water bodies substantially increases (Campbell et al., 1977; Stoof et al., 2011; Lourenço et al., 2012; Bladon et al., 2014). However, the post-fire water yield is also dependent on the size of the burned area and the meteorological conditions following the wildfire (DeBano et al., 1998; Lane et al., 2006; Bart, 2016; Wine and Cadol, 2016). The decrease of soil infiltration capacity increases the overland flow rates as well as decreases the subsurface flow (Taufik et al., 2017; Rodrigues et al., 2019). Reduced subsurface flows could then cause water shortage during dry periods. Basso et al. (2020) observed a considerable increase in the runoff and peak flows in the post-fire scenario. This increase, however, was not noticeable on the total water yield in the inlet of Castelo de Bode reservoir, where only a slight increase was observed when compared to the reference scenario. As observed in Rodrigues et al. (2019), the reduction of flow during the dry period led to a minimal change in the total water yield. The decrease of the average water level of one meter, albeit small, could be explained by the sensitivity that the model seemed to have to small variations in input flow.
The scarce number of studies addressing water quality deterioration in reservoirs after wildfires limited the comparison of the results in the present study. There were, however, some major outcomes that agree with published data on the topic so far. The elevated concentration of nutrients at Castelo de Bode inlet suggested a possible threat to aquatic life and human health (Basso et al., 2020). A considerable dilution of 50 and 90% for nitrate and phosphate, respectively, was observed at the dam wall when compared to the reservoir inlet. These figures were in agreement with Emmerton et al. (2019) measures, which detected strong and constant retention of nutrients in a lake after a wildfire, suggesting little potential for downstream transport. A study conducted in the large reservoir of Dartmouth, Australia, did not notice evident changes in nutrients concentrations although high nutrient waters were delivered after a wildfire (Alexander et al., 2004). An increase from 2 to 7 fold was instead detected in shallow lakes after a wildfire disturbance (Carignan et al., 2000; Pinel-Alloul et al., 2002). The results of the simulation of CE-QUAL-W2 indicated an increase in nitrate until a maximum value of 1.49 mg N-NO3 L−1, below the 11.3 mg N-NO3 L−1 threshold for surface water intended for human consumption in Portugal (Ambiente, 1998). With an increase of concentration in the post-fire scenario, phosphate also did not overstep either the limit for drinking water, being 0.17 mg P-PO4 L−1 (Ambiente, 1998) or the environmental quality limit of 0.1 mg P-PO4 L−1 (USEPA, 1986). The additional input of nutrients into lakes and reservoirs could have important implications for increased occurrence of harmful algal blooms, being a serious issue in both the aquatic ecosystem and drinking water treatment. Total suspended solids overcame the maximum recommended value (VMR) of 25 mg L−1, considered as the quality standard to be respected or not exceeded (Ambiente, 1998).
A high concentration of TSS might reduce the efficiency of water treatment processes as well as making drinking water unpalatable (Emelko et al., 2011). The findings of White et al. (2006); Bladon et al. (2014) revealed an elevated concentration of sediments, which made the water unfit for reticulation. Nevertheless, in this model exercise, the average concentration of TSS slightly increased in the fire scenario, abiding within the range of the reference scenario. The same behavior was observed during a measurement campaign in Yellowstone Lake, where turbidity levels did not change after a wildfire (Lathrop, 1994). Studies conducted in lakes with smaller capacities, however, measured high levels of sediments/turbidity following an event (Pinel-Alloul et al., 2002; Tecle and Neary, 2014). The ability of large water bodies to dilute high concentrations of contaminants might explain the contradictory results found in the literature (Smith et al., 2011). The contrast with the high concentration recorded at the reservoir inlet (Basso et al., 2020) could reflect the capacity of the reservoir to dilute contaminated inflows.
Notwithstanding, several interruptions of the treatment plant located in the Ribeira de Alge, a tributary of Castelo de Bode, were recorded in the period after the forest fires. According to local and national news media, the impact of a flood in recently burned areas on water quality led to an interruption of water supply systems (Leiria, 2017; Dnoticias, 2017; RTPnoticias, 2017). However, and contrary to what was recorded upstream, there were no interruptions of the water supply in Castelo de Bode, confirming the system capacity to reduce the high concentrations recorded at its inlets. In this particular study, it was predicted an increase of phosphate and sediment concentrations during wet periods in the region. These increases presented a slow but continuous trend and then returned to pre-fire values over a 20-days interval. Conversely, the increase in nitrate concentration in the post-fire scenario never reverted to pre-fire values. Future studies could assess the time and the distance from the inlet after which the reservoir is able to dilute the polluted inflow, thus making a comprehensive assessment of the waterbody’s ability to mitigate the impacts of wildfires. The capability of a reservoir to attenuate water quality contaminations from streams is of great relevance in post-fire scenario management (Lathrop, 1994; Alexander et al., 2004). The findings of this work support the conclusion of Smith et al. (2011) and Evans et al. (2017) that post-fire concentrations increase of most constituents is improbable to violate drinking water guidelines.
To strengthen the use of reservoir models to simulate post-fire scenarios, the study would benefit from observed data for model performance validation. A calibration of those conditions could help the stakeholders in the decision-making adapting to climate change scenarios, and to prioritize fuel reduction for water supply protection. The simple analysis of the extension of the burned area contributing area, or to test if such wildfire scenario under more demanding climate conditions could compromise water quality at the reservoir, would by itself provide valuable information to land and water managers. Output for simulations in extreme climate conditions could differ greatly from one conducted in an average rainfall year. Such conditions can be droughts (Hoegh-Guldberg et al., 2019) that could attenuate the dilution effect from the reservoir or extreme rainfall events (Hoegh-Guldberg et al., 2019) in which greater hydrological and erosive response should be expected. This is particularly relevant when considering the expected increase in burn area worldwide (Seidl et al., 2017), and changes in rainfall regimes (Hoegh-Guldberg et al., 2019). This combination of factors has already revealed a significant increase in sedimentation in 388 of the 471 analyzed watersheds in the western United States, compromising water quality and quantity until 2050 (Sankey et al., 2017).
The literature on the impacts of wildfires on water quality focuses heavily on watercourses and only limited studies have been conducted at the reservoir level, mostly focused on the inlet of the latter. As such, more precise monitoring of in-lake and reservoir outlet post-fire impacts for several years after the event would improve the knowledge of water quality variation in a large waterbody.
Conclusion
The results of this modeling study highlight the relevance of combining watershed models with reservoir models to assess the impact of wildfires on water quality. Despite the limited amount of field data available for the modeling exercise, most of the water quality variables have been satisfactorily simulated by the combined use of the two models. The use of only one group of algae may have reduced the ability of the model to correctly reproduced the water quality variables that have a relation with chlorophyll-a concentrations.
An overall degradation of the water quality at the dam wall was observed in the fire scenario simulation. Nevertheless, the modeling exercise concluded that 2017 forest fires did not pose any danger to human health or aquatic life. This outcome can be attributed to the size of Castelo de Bode reservoir, storing a volume of water capable of diluting the high concentration of nutrients detected at the inlet of the reservoir. However, the high concentration of TSS detected on the water surface may require an adjustment in the water treatment processes.
Future works could take benefits from observed pre- and post-fire data to endorse the reliability of the model for both scenarios. Moreover, climate change scenarios should be considered to examine the dependence of water contaminant concentrations to extreme meteorological conditions after a wildfire. Increases in extreme precipitation events or dry periods are just some of the effects of climate changes that may enhance water quality deterioration.
Data Availability Statement
The raw data supporting the conclusions of this article will be made available by the authors, without undue reservation.
Author Contributions
MB implemented the model, create the simulations and performed the statistical analysis. DV, MM, and MB contributed to the design of the study. DV helped to design the post-fire scenario. MM helped on the calibration of the model. TR provided the databases and help on the drafting of the manuscript. All authors contributed to manuscript revision, read, and approved the submitted version.
Funding
This work received financial support from FCT/MCTES (PIDDAC) through project LARSyS - FCT Pluriannual funding 2020-2023 (UIDB/50009/2020), CESAM from FCT/MCTES national funds (PIDDAC) through projects UIDP/50017/2020 and UIDB/50017/2020, the co-funding by the FEDER, within the PT2020 Partnership Agreement and Compete 2020, and by the FEMME project (PCIF/MPG/0019/2017). MB was funded by Fundação para a Ciência e a Tecnologia (FCT) through the contract SFRH/BD/146356/2019. MM was funded by FCT/MCTES (PIDDAC) through project LARSyS - FCT Pluriannual funding 2020-2023 (UIDB/50009/2020). TR was supported by FCT by contract CEECIND/01152/2017. DV was funded by national funds (OE), through FCT in the scope of the framework contract foreseen - DL57/2016 (CDL-CTTRI-97-ARH/2018 - REF.191-97-ARH/2018).
Conflict of Interest
The authors declare that the research was conducted in the absence of any commercial or financial relationships that could be construed as a potential conflict of interest.
References
Alexander, S. J., Grace, M., and Mckelvie, I. (2004). Effect of Bushfires on Receiving Waters, Eastern Victoria. Water Stud: First Interim Report to the Department of Sustainability and EnvironmentCentre, Sch. Chem. Monash Univ. Aust.
Ambiente, M. (1988). Decreto Regulamentar n.o 2/88. Diário da República Série I. Portugal: Governo de Portugal, 219–222.
Ambiente, M. (1998). Decreto-Lei n.o 236/98. Diário da República Série I-A. Portugal: Governo de Portugal, 3676–3722.
Arnold, J. G., and Fohrer, N. (2005). SWAT2000: Current Capabilities and Research Opportunities in Applied Watershed Modelling. Hydrol. Process. 19, 563–572. doi:10.1002/hyp.5611
Bansal, M. K. (1976). Nitrification in Natural Streams. J. Water Pollut. Control. Fed. 48, 2380–2393.
Bart, R. R. (2016). A Regional Estimate of Postfire Streamflow Change in California. Water Resour. Res. 52, 1465–1478. doi:10.1002/2014WR016553
Basso, M., Vieira, D. C. S., Ramos, T. B., and Mateus, M. (2020). Assessing the Adequacy of SWAT Model to Simulate Postfire Effects on the Watershed Hydrological Regime and Water Quality. Land Degrad. Dev. 31, 619–631. doi:10.1002/ldr.3476
Bhattacharya, A. (2019). Changing Environmental Condition and Phosphorus-Use Efficiency in Plants. Chapter 5 - Changing Environmental Condition and Phosphorus-Use Efficiency in Plants, in: Bhattacharya, A.B.T.-C.C. and R.U.E. in P. (Ed.),. Academic Press, pp. 241–305. doi:10.1016/B978-0-12-816209-5.00005-2
Bladon, K. D., Emelko, M. B., Silins, U., and Stone, M. (2014). Wildfire and the Future of Water Supply. Environ. Sci. Technol. 48, 8936–8943. doi:10.1021/es500130g
Bladon, K. D., Silins, U., Wagner, M. J., Stone, M., Emelko, M. B., Mendoza, C. A., et al. (2008). Wildfire Impacts on Nitrogen Concentration and Production from Headwater Streams in Southern Alberta's Rocky Mountains. Can. J. For. Res. 38, 2359–2371. doi:10.1139/x08-071
Brito, D., Ramos, T. B., Gonçalves, M. C., Morais, M., and Neves, R. (2018). Integrated Modelling for Water Quality Management in a Eutrophic Reservoir in South-Eastern Portugal. Environ. Earth Sci. 77, 40. doi:10.1007/s12665-017-7221-5
Campbell, R. E., Baker, J., Ffolliott, P. F., Larson, F. R., and Avery, C. C. (1977). Wildfire Effects on a Ponderosa pine Ecosystem: an Arizona Case Study, Agric. For. Serv. Rocky Mt. For. Range Exp. Station., 12. Fort Collins: USDA ForCO US Dep, 191.
Caramujo, M.-J., Mendes, C. R. B., Cartaxana, P., Brotas, V., and Boavida, M.-J. (2007). Influence of Drought on Algal Biofilms and Meiofaunal Assemblages of Temperate Reservoirs and Rivers. Hydrobiologia 598, 77–94. doi:10.1007/s10750-007-9141-y
Carignan, R., D'Arcy, P., and Lamontagne, S. (2000). Comparative Impacts of Fire and forest Harvesting on Water Quality in Boreal Shield Lakes. Can. J. Fish. Aquat. Sci. 57, 105–117. doi:10.1139/f00-125
Chuvieco, E. (2012). Remote Sensing of Large Wildfires: In the European Mediterranean Basin. Berlin: Springer Science & Business Media.
Cole, T. M., and Wells, S. A. (2006). CE-QUAL-W2: A Two-Dimensional, Laterally Averaged, Hydrodynamic and Water Quality Model, Version 3.5. Instruction Report EL-06-1. US Army Engineering and Research Development Center: Vicksburg, MS.
DeBano, L. F., Neary, D. G., and Ffolliott, P. F. (1998). Fire Effects on Ecosystems. New York, NY: John Wiley & Sons.
Debele, B., Srinivasan, R., and Parlange, J.-Y. (2008). Coupling upland Watershed and Downstream Waterbody Hydrodynamic and Water Quality Models (SWAT and CE-QUAL-W2) for Better Water Resources Management in Complex River Basins. Environ. Model. Assess. 13, 135–153. doi:10.1007/s10666-006-9075-1
Dnoticias, pt (2017). Enxurradas obrigam município de Ansião a interromper captação de água.Available at: https://www.dnoticias.pt/pais/enxurradas-obrigam-municipio-de-ansiao-a-interromper-captacao-de-agua-BM1680322# (accessed 12.15.19).
Emelko, M. B., Silins, U., Bladon, K. D., and Stone, M. (2011). Implications of Land Disturbance on Drinking Water Treatability in a Changing Climate: Demonstrating the Need for “Source Water Supply and protection” Strategies. Water Res. 45, 461–472. doi:10.1016/j.watres.2010.08.051
Emmerton, C. A., Beaty, K. G., Casson, N. J., Graydon, J. A., Hesslein, R. H., Higgins, S. N., et al. (2019). Long-Term Responses of Nutrient Budgets to Concurrent Climate-Related Stressors in a Boreal Watershed. Ecosystems 22, 363–378. doi:10.1007/s10021-018-0276-7
Eppley, R. W., Rogers, J. N., and McCarthy, J. J. (1969). Half-saturation Constants for Uptake of Nitrate and Ammonium by Marine Phytoplankton1. Limnol. Oceanogr. 14, 912–920. doi:10.4319/lo.1969.14.6.0912
Ernst, M. R., and Owens, J. (2009). Development and Application of a WASP Model on a Large Texas Reservoir to Assess Eutrophication Control. Lake Reservoir Management 25, 136–148. doi:10.1080/07438140902821389
Evans, C. D., Malcolm, I. A., Shilland, E. M., Rose, N. L., Turner, S. D., Crilly, A., et al. (2017). Sustained Biogeochemical Impacts of Wildfire in a Mountain Lake Catchment. Ecosystems 20, 813–829. doi:10.1007/s10021-016-0064-1
Ferreira, A. J. D., Coelho, C. O. A., Ritsema, C. J., Boulet, A. K., and Keizer, J. J. (2008). Soil and Water Degradation Processes in Burned Areas: Lessons Learned from a Nested Approach. Catena. 74, 273–285. doi:10.1016/j.catena.2008.05.007
Ferreira, A. J. D., Silva, J. S., Maia, M. J., Catry, F., and Moreira, F. (2005). Gestão Pós-Fogo. Extracção de madeira queimada e proteção da floresta contra a erosão do solo. Lisboa, Portugal: Direcção-Geral dos Recursos Florestais
Finenko, Z. Z., and Krupatkina-Akinina, D. K. (1974). Effect of Inorganic Phosphorus on the Growth Rate of Diatoms. Mar. Biol. 26, 193–201. doi:10.1007/bf00389251
Flowers, J. D., Santhi, C., and Hauck, L. M. (2000). The Use of an Integrated Modeling Approach for TMDL Development in the lake Waco/Bosque River Watershed. Wisconsin, USA: ASAE Annu. Int. Meet. Milwaukee, 9–12.
Fuka, D. R., Walter, M. T., MacAlister, C., Degaetano, A. T., Steenhuis, T. S., and Easton, Z. M. (2014). Using the Climate Forecast System Reanalysis as Weather Input Data for Watershed Models. Hydrol. Process. 28, 5613–5623. doi:10.1002/hyp.10073
Havel, A., Tasdighi, A., and Arabi, M. (2018). Assessing the Hydrologic Response to Wildfires in Mountainous Regions. Hydrol. Earth Syst. Sci. 22, 2527–2550. doi:10.5194/hess-22-2527-2018
Hoegh-Guldberg, O., Jacob, D., Taylor, M., Bolaños, T. G., Bindi, M., Brown, S., et al. (2019). The Human Imperative of Stabilizing Global Climate Change at 1.5° C. Sci. 365, eaaw6974. doi:10.1126/science.aaw6974
Hoogenhout, H., and Amesz, J. (1965). Growth Rates of Photosynthetic Microorganisms in Laboratory Cultures. Archiv. Mikrobiol. 50, 10–25. doi:10.1007/bf00439783
Hussein, A. M., and Wells, S. A. (2020). Comparison of a 2D and 3D Hydrodynamic and Water Quality Model for Lake Systems. World Environ. Water Resour. Congr.Proceedings. doi:10.1061/9780784481400.007
Inag, I. P. (2011). Modelação matemática da qualidade da água em Albufeiras com planos de ordenamento. V. Efeito de fogos florestais no regime de escoamento e na qualidade da áhua de rios e albufeiras. Ministério da Agric. mar, Ambient. Lisbon, Portugal: e Ordenam. do Territ. Inst. da Água, I.P
Johnson, P., Chan, W. H., Gherini, S. A., and Chamberlin, C. E. (1985). Rates, Constants, and Kinetics Formulations in Surface Water Quality Modeling. Athens, GA: US Environmental Protection AgencyEnvironmental Research Lab.EPA/600/3-85/040
Keeley, J. E. (2009). Fire Intensity, Fire Severity and Burn Severity: a Brief Review and Suggested Usage. Int. J. Wildland Fire 18, 116–126. doi:10.1071/wf07049
Keizer, J. J., Doerr, S. H., Malvar, M. C., Prats, S. A., Ferreira, R. S. V., Oñate, M. G., et al. (2008). Temporal Variation in Topsoil Water Repellency in Two Recently Burnt Eucalypt Stands in north-central Portugal. CATENA 74, 192–204. doi:10.1016/j.catena.2008.01.004
Lane, P. N. J., Sheridan, G. J., and Noske, P. J. (2006). Changes in Sediment Loads and Discharge from Small Mountain Catchments Following Wildfire in South Eastern Australia. J. Hydrol. 331, 495–510. doi:10.1016/j.jhydrol.2006.05.035
Lathrop, R. j. (1994). Impacts of the 1988 Wildfires on the Water-Quality of Yellowstone and Lewis Lakes, Wyoming. Int. J. Wildland Fire 4, 169–175. doi:10.1071/wf9940169
Leiria, J. de. (2017). Chuvas contaminam águas de Ansião com cinzas dos incêndios. Available at: https://www.jornaldeleiria.pt/noticia/chuvas-contaminam-aguas-de-ansiao-com-cinzas-dos-incendios-7799 (accessed 12.15.19).
Lima, L., Mangiavacchi, N., Soares, C., and Fontoura, J. (2009). Latelly Averaged 2D Model for Thermal Stratification Simulations in Reservoir Environments. Turbulence Heat and Mass Transfer 6. Proceedings of the Sixth International Symposium On Turbulence Heat and Mass Transfer, Rome, Italy, September 14–18, 2009 (Begel House Inc). doi:10.1615/ichmt.2009.turbulheatmasstransf.1600
Lourenço, L., Nunes, A., Bento-Gonçalves, A., and Vieira, A. (2012). Soil Erosion after Wildfires in Portugal: What Happens when Heavy Rainfall Events Occur. Res. Soil Erosion, 65–88. doi:10.5772/50447
McIntyre, N., Wheater, H., and Lees, M. (2002). Estimation and Propagation of Parametric Uncertainty in Environmental Models. J. Hydroinformatics 4, 177–198. doi:10.2166/hydro.2002.0018
Moody, J. A., and Martin, D. A. (2001). Initial Hydrologic and Geomorphic Response Following a Wildfire in the Colorado Front Range. Earth Surf. Process. Landforms 26, 1049–1070. doi:10.1002/esp.253
Moreira, F., Rego, F. C., and Ferreira, P. G. (2001). Temporal (1958–1995) Pattern of Change in a Cultural Landscape of Northwestern Portugal: Implications for Fire Occurrence. Landsc. Ecol. 16, 557–567. doi:10.1023/A:1013130528470
Moreira, F., Viedma, O., Arianoutsou, M., Curt, T., Koutsias, N., Rigolot, E., et al. (2011). Landscape - Wildfire Interactions in Southern Europe: Implications for Landscape Management. J. Environ. Manage. 92, 2389–2402. doi:10.1016/j.jenvman.2011.06.028
Moriasi, D. N., Gitau, M. W., Pai, N., and Daggupati, P. (2015). Hydrologic and Water Quality Models: Performance Measures and Evaluation Criteria. Trans. ASABE 58, 1763–1785. doi:10.13031/trans.58.10715
Neitsch, S. L., Arnold, J. G., Kiniry, J. R., and Williams, J. R. (2011). Soil and Water Assessment Tool Theoretical Documentation Version 2009. College Station, TX: Texas Water Resources Institute.
NOAA (2019). GFS Global Forecast System [WWW Document]. Available at: https://www.ncdc.noaa.gov/data-access/model-data/model-datasets/global-forcast-system-gfs (Accessed 20.9.19).
Norton, G. E., and Bradford, A. (2009). Comparison of Two Stream Temperature Models and Evaluation of Potential Management Alternatives for the Speed River, Southern Ontario. J. Environ. Manage. 90, 866–878. doi:10.1016/j.jenvman.2008.02.002
Nunes, J. P., Doerr, S. H., Sheridan, G., Neris, J., Santín Nuño, C., Emelko, M. B., et al. (2018a). Assessing Water Contamination Risk from Vegetation Fires: Challenges, Opportunities and a Framework for Progress. Hydrol. Process.
Nunes, J. P., Naranjo Quintanilla, P., Santos, J. M., Serpa, D., Carvalho-Santos, C., Rocha, J., et al. (2018b). Afforestation, Subsequent Forest Fires and Provision of Hydrological Services: A Model-Based Analysis for a Mediterranean Mountainous Catchment. Land Degrad. Develop. 29, 776–788. doi:10.1002/ldr.2776
Nyman, P., Sheridan, G., and Lane, P. N. J. (2010). Synergistic Effects of Water Repellency and Macropore Flow on the Hydraulic Conductivity of a Burned forest Soil, South-East Australia. Hydrol. Process. 24, 2871–2887. doi:10.1002/hyp.7701
Pechlivanidis, I. G., Jackson, B. M., McIntyre, N. R., and Wheater, H. S. (2011). Catchment Scale Hydrological Modelling: a Review of Model Types, Calibration Approaches and Uncertainty Analysis Methods in the Context of Recent Developments in Technology and Applications. Glob. NEST J. 13, 193–214.
Pinel-Alloul, B., Prepas, E., Planas, D., Steedman, R., and Charette, T. (2002). Watershed Impacts of Logging and Wildfire: Case Studies in Canada. Lake Reservoir Management 18, 307–318. doi:10.1080/07438140209353937
Pordata, (2019). Total Rainfall. Base de Dados Portugal Contemporâneo. Available at https://www.pordata.pt/en/Portugal/Total+rainfall-1070 (Accessed 20.9.19)
Reid, W., Mooney, H., Cropper, A., Capistrano, D., Carpenter, S., Chopra, K., et al. (2005). Millenium Ecosystem Assessment Synthesis Report. Washington, DC
Rhoades, C. C., Chow, A. T., Covino, T. P., Fegel, T. S., Pierson, D. N., and Rhea, A. E. (2019). The Legacy of a Severe Wildfire on Stream Nitrogen and Carbon in Headwater Catchments. Ecosystems 22, 643–657. doi:10.1007/s10021-018-0293-6
Robichaud, P. R. (2000). Fire Effects on Infiltration Rates after Prescribed Fire in Northern Rocky Mountain Forests, USA. J. Hydrol. 231-232, 220–229. doi:10.1016/s0022-1694(00)00196-7
Rodrigues, E. L., Jacobi, C. M., and Figueira, J. E. C. (2019). Wildfires and Their Impact on the Water Supply of a Large Neotropical metropolis: A Simulation Approach. Sci. Total Environ. 651, 1261–1271. doi:10.1016/j.scitotenv.2018.09.289
RTPnotícias, (2017). Há água contaminada no concelho de Ansião [WWW Document]. Available at: https://www.rtp.pt/noticias/pais/ha-agua-contaminada-no-concelho-de-ansiao_a1045797 (accessed 12.15.19).
Sadeghian, A., Chapra, S. C., Hudson, J., Wheater, H., and Lindenschmidt, K.-E. (2018). Improving in-lake Water Quality Modeling Using Variable Chlorophyll A/algal Biomass Ratios. Environ. Model. Softw. 101, 73–85. doi:10.1016/j.envsoft.2017.12.009
San-Miguel-Ayanz, J., Durrant, T., Boca, R., Libertà, G., Branco, A., de Rigo, D., et al. (2016). Forest Fires in Europe, Middle East and North Africa 2015. Luxembourg: Publ. Off. Eur. Union. doi:10.2788/914
Sankey, J. B., Kreitler, J., Hawbaker, T. J., McVay, J. L., Miller, M. E., Mueller, E. R., et al. (2017). Climate, Wildfire, and Erosion Ensemble Foretells More Sediment in Western USA Watersheds. Geophys. Res. Lett. 44, 8884–8892. doi:10.1002/2017gl073979
Seidl, R., Thom, D., Kautz, M., Martin-Benito, D., Peltoniemi, M., Vacchiano, G., et al. (2017). Forest Disturbances under Climate Change. Nat. Clim Change 7, 395–402. doi:10.1038/nclimate3303
Shakesby, R. A., Moody, J. A., Martin, D. A., and Robichaud, P. R. (2016). Synthesising Empirical Results to Improve Predictions of post-wildfire Runoff and Erosion Response. Int. J. Wildland Fire 25, 257–261. doi:10.1071/wf16021
Shakesby, R. A. (2011). Post-wildfire Soil Erosion in the Mediterranean: Review and Future Research Directions. Earth-Science Rev. 105, 71–100. doi:10.1016/j.earscirev.2011.01.001
Shakesby, R., and Doerr, S. (2006). Wildfire as a Hydrological and Geomorphological Agent. Earth-Science Rev. 74, 269–307. doi:10.1016/j.earscirev.2005.10.006
Sheridan, G., Lane, P., Noske, P., Feikema, P., Sherwin, C., and Grayson, R. (2007). Impact of the 2003 Alpine Bushfires on Streamflow: Estimated Changes in Stream Exports of Sediment, Phosphorus and Nitrogen Following the 2003 Bushfires in Eastern Victoria. Canberra: Murray-Darling Basin Comm.
Smith, E. A., Kiesling, R. L., Galloway, J. M., and Ziegeweid, J. R. (2014). Water Quality and Algal Community Dynamics of Three deepwater Lakes in Minnesota Utilizing CE-QUAL-W2 Models. U.S. Geol. Surv. Scientific Invest. Rep. 73, 5066. doi:10.3133/sir20145066
Smith, H. G., Sheridan, G. J., Lane, P. N. J., Nyman, P., and Haydon, S. (2011). Wildfire Effects on Water Quality in forest Catchments: A Review with Implications for Water Supply. J. Hydrol. 396, 170–192. doi:10.1016/j.jhydrol.2010.10.043
SNIRH (2011). Serviço Nacional de Informação sobre Recursos Hídricos [WWW Document]. Available at: https://snirh.apambiente.pt/(accessed 9.9.19).
Spencer, C. N., and Hauer, F. R. (1991). Phosphorus and Nitrogen Dynamics in Streams during a Wildfire. J. North Am. Benthological Soc. 10, 24–30. doi:10.2307/1467761
Stoof, C. R., Moore, D., Ritsema, C. J., and Dekker, L. W. (2011). Natural and Fire-Induced Soil Water Repellency in a Portuguese Shrubland. Soil Sci. Soc. America J. 75, 2283–2295. doi:10.2136/sssaj2011.0046
Talling, J. F. (1955). The Relative Growth Rates of Three Plankton Diatoms in Relation to Underwater Radiation and Temperature. Ann. Bot. 19, 329–341. doi:10.1093/oxfordjournals.aob.a083432
Taufik, M., Torfs, P. J. J. F., Uijlenhoet, R., Jones, P. D., Murdiyarso, D., and Van Lanen, H. A. J. (2017). Amplification of Wildfire Area Burnt by Hydrological Drought in the Humid Tropics. Nat. Clim Change 7, 428–431. doi:10.1038/nclimate3280
Tecle, A., and Neary, D. (2014). Water Quality Impacts of forest Fires. Arizona-Nevada Academy of Science. doi:10.4172/2375-4397.1000140
Turco, M., Llasat, M.-C., von Hardenberg, J., and Provenzale, A. (2014). Climate Change Impacts on Wildfires in a Mediterranean Environment. Climatic Change 125, 369–380. doi:10.1007/s10584-014-1183-3
Us service, A. M. (1942). University of Texas Libraries [WWW Document]. Available at: http://legacy.lib.utexas.edu/maps/portugal.html (accessed 4.5.18).
USEPA (1986). Quality Criteria for Water: EPA/440/5-86/001. Washington, DC: United States Environmental Protection Agency.
Viedma, O., Moity, N., and Moreno, J. M. (2015). Changes in Landscape Fire-hazard during the Second Half of the 20th century: Agriculture Abandonment and the Changing Role of Driving Factors. Agric. Ecosyst. Environ. 207, 126–140. doi:10.1016/j.agee.2015.04.011
Vieira, D. C. S., Prats, S. A., Nunes, J. P., Shakesby, R. A., Coelho, C. O. A., and Keizer, J. J. (2014). Modelling Runoff and Erosion, and Their Mitigation, in Burned Portuguese forest Using the Revised Morgan-Morgan-Finney Model. For. Ecol. Management 314, 150–165. doi:10.1016/j.foreco.2013.12.006
White, I., Wade, A., Worthy, M., Mueller, N., Daniell, T., and Wasson, R. (2006). The Vulnerability of Water Supply Catchments to Bushfires: Impacts of the January 2003 Wildfires on the Australian Capital Territory. Australas. J. Water Resour. 10, 179–194. doi:10.1080/13241583.2006.11465291
White, J. D., Prochnow, S. J., Filstrup, C. T., Scott, J. T., Byars, B. W., and Zygo-Flynn, L. (2010). A Combined Watershed-Water Quality Modeling Analysis of the Lake Waco Reservoir: I. Calibration and Confirmation of Predicted Water Quality. Lake Reservoir Management 26, 147–158. doi:10.1080/07438141.2010.495315
White, K. L., Chaubey, I., and Haggard, B. E. (2004). Linking Watershed and Reservoir Models. ASAE Annual Meeting. Ottawa, ON, Canada: American Society of Agricultural and Biological Engineers, 1.
Keywords: water contamination, lake, CE-QUAL-W2, hydrodynamic model, model integration
Citation: Basso M, Mateus M, Ramos TB and Vieira DCS (2021) Potential Post-Fire Impacts on a Water Supply Reservoir: An Integrated Watershed-Reservoir Approach. Front. Environ. Sci. 9:684703. doi: 10.3389/fenvs.2021.684703
Received: 23 March 2021; Accepted: 25 May 2021;
Published: 11 June 2021.
Edited by:
Gordon O’Brien, University of Mpumalanga, South AfricaCopyright © 2021 Basso, Mateus, Ramos and Vieira. This is an open-access article distributed under the terms of the Creative Commons Attribution License (CC BY). The use, distribution or reproduction in other forums is permitted, provided the original author(s) and the copyright owner(s) are credited and that the original publication in this journal is cited, in accordance with accepted academic practice. No use, distribution or reproduction is permitted which does not comply with these terms.
*Correspondence: Marta Basso, bWFydGFiYXNzb0B1YS5wdA==