- 1School of Architecture and Civil Engineering, Xihua University, Chengdu, China
- 2School of Architecture, Southwest Jiaotong University, Chengdu, China
Abstract: Geological disasters have long been a constant threat to socioeconomic development and human life and property. Many studies focus on the occurred geological disasters and ignore the potential risk events. Fine-scale multi-type analysis of Potential Geological Disasters Spots (PGDSs) is a necessary but insufficiently explored research field in China’s mountainous areas. Taking Meigu County (Sichuan Province, Southwest China), a typical disaster-prone region, as the study area. Average nearest neighbor (ANN), standard deviational ellipse (SDE), kernel density estimation (KDE), and ordinary least squares (OLS) regression are used to assess the spatial distribution of potential geological disasters spots and their influencing factors. The findings show that: potential geological disasters spots have a significant spatial heterogeneity and exhibited a prominent clustering characteristic. They were distributed in an elevation range of 1,500–2000 m, which receives an average monthly rainfall of more than 240 mm, as well as in highly foliated (0.6 < Normalized Difference Vegetation Index (NDVI) < 0.8), west-facing (247.5–292.5°), and slopes of 10–20°. In addition, slope, aspect, population density, and rainfall were found as the main influencing factors. The results provide practical rural development measures with support for potential geological disasters spots avoidance and preparedness.
1 Introduction
In recent years, frequent climate change and geological disasters seriously obstructed socioeconomic development and posed a serious threat to achieving sustainable development goals worldwide (Fedeski and Gwilliam, 2007; Zhuang et al., 2020; Wan et al., 2021; Wang et al., 2021). China is one of the countries with the highest incidence and frequency of geological disasters in the world (Li et al., 2015; Hou et al., 2016; Xiao et al., 2022). Geological disasters have long been a persistent threat to the development of urban and rural construction in China (Bulte et al., 2018; Ao et al., 2021; Zhao Z. et al., 2021; Zeng et al., 2021; Zhou et al., 2021; Pu et al., 2022), especially in mountainous rural areas that are more disaster-prone (Xu et al., 2020; Lin J et al., 2021; Qing et al., 2021; Gan et al., 2022). According to a report released by the Ministry of Natural Resources of China, a total of 7,840 geological disasters occurred in China in 2020, resulting in 139 deaths (missing), 58 injuries, and direct economic losses of 50.2 billion yuan (http://vod.mnr.gov.cn/). Over the past decades, China’s rural society and economy have developed rapidly (Li K. et al., 2021). All the rural villages were lifted out of extreme poverty at the end of 2020. However, in the vast mountainous regions of China, people’s lives and properties are still under serious threat due to frequent geological disasters, accompanied by the risk of returning to poverty due to disasters, and the sustainable development of the rural areas is seriously hindered. In addition, with rapid urbanization, the land use cover of the mountainous rural areas has changed dramatically. Many infrastructures, such as roads and gas engineering, have been put into construction, which means that human activities are disturbing the ecological environment more and more. The risk of geological disasters will gradually increase (Jiang et al., 2016). Therefore, research on the risk of geological disasters in mountainous rural areas must be addressed.
The concept of “Potential Geological Disaster Spots (PGDSs)” refers to a broad range of point and partial non-point events (Gan et al., 2022), which are potentially dangerous to occur or have already occurred but still are unstable. There are many PGDSs, including landslides, debris flows, unstable slopes, avalanches, ground subsidence, etc. The different causes of different types of geological disasters lead to significant variability in their spatial distribution and time of occurrence. In addition, the PGDSs are widely distributed, sudden, and temporally erratic, resulting in severe threats to people’s lives and property (Xu D. et al., 2018; Lin J et al., 2021; Wang et al., 2021; Yao et al., 2022). Most studies on geological disasters mainly focus on the spatiotemporal characteristics of different types of geological disasters (Zuo et al., 2009; Pu et al., 2022), assessment of severity and susceptibility (Chang et al., 2022; Kim et al., 2022), impact on human lives and production (Wu et al., 2022), etc. However, few studies focus on potential geological disasters. Related studies have confirmed that PGDSs are more hidden and sudden than those that have occurred or have been managed. Therefore, in the prevention of geological disasters, special attention needs to be paid to identifying PGDSs where no disaster has occurred. According to a notice issued by the Sichuan provincial government on a geological disaster prevention program, by the end of 2020, there are about 36,000 geological disaster potential sites in Sichuan, posing varying degrees of threat to the lives of nearly 1.5 million people and 850 billion yuan of property (https://www.sc.gov.cn/). In addition, the abovementioned studies primarily focus on the macro scale, such as city level, province level, and river basin level, ignoring the fine-scale analysis at the county or town level. Notably, China’s mountainous rural areas, often geological disaster-prone areas, have faced increasing risk from PGDSs caused by global climate change and increasing human engineering activities (Qing et al., 2021). The residents in mountainous rural areas are more profoundly affected by PGDSs due to impaired transportation and delayed rescue operations compared to the plain areas (Pan, 2016). Therefore, to better characterize the risk and undertake precautionary measures, scientific insight into pre-disaster preparedness is required by identifying the spatial distribution characteristics, patterns, and influencing factors of PGDSs.
Meigu County, the case study area of this research, is increasingly affected by a variety of homogeneously distributed PGDSs in Sichuan Province, Southwest China. We select Meigu County as the case study for two reasons: 1) The case representation. Meigu County is one of the specific areas in China most prone to geological disasters, with a large number and widely distributed PGDSs. 2) The urgency of studies. Due to the unique topographical complexity, rainfall regime, and intensive human engineering activities, the PGDSs have been remarkable and have shown an increasing trend in recent years. As a result, most local communities are constantly affected by PGDSs, and people’s living and production are seriously threatened. Thus, Meigu County is an ideal case to assess the PGDSs spatial distribution and influencing factors in China’s mountainous rural areas.
The contributions of this paper are as follows: 1) analyzed the spatial distribution characteristics and fine-scale spatial heterogeneity mapping of PGDSs; 2) Identified the main PGDSs contributing factors using the ordinary least squares (OLS) model; and 3) tailored PGDSs-based planning recommendations for better pre-disaster planning and preparedness.
The remainder of this paper is structured as follows: Section 2 reviews the existing literature on the PGDS spatial distribution and the influencing factors. Section 3 introduces the study area, methods, and datasets. Section 4 shows the results. Section 5 discusses the results and highlights recommendations for disaster avoidance in mountainous rural areas. Finally, Section 6 provides the conclusion drawn from the findings.
2 Literature review
2.1 Spatial distribution of geological disasters in China
China has a wide distribution of geological disasters due to its many mountainous areas and complex terrain, and different types of geological disasters cover almost all areas of inland China. The spatiotemporal distribution pattern of geological disasters is influenced by the natural geographical environment and human activities. In the beginning, scholars have spared no effort to study disaster risk zoning and visualization maps (van Westen et al., 2000). Geological disaster risk zones are divided into different classes according to their susceptibility and hazardousness, which is a method to reveal the spatial distribution characteristics of geological disasters. Then, more and more scholars realize that the distribution of geological disasters is closely related to their causes, so the spatial distribution characteristics of different types of geological disasters have significant differences (Jiang et al., 2016). In recent years, many related studies have further confirmed that the spatial distribution of different types of geological disasters varies in China. For instance, landslide disasters are mainly gathered in North and Southern China, while debris flow disasters are often distributed in Southeastern China (Liu et al., 2012). Regarding distribution, the vulnerability to geological disasters in China is generally the lowest in eastern China, the highest in western China, and gradually decreasing from western to eastern (Li et al., 2015).
However, on a fine scale, the distribution of geological disasters is closely related to their elevation, slope, rainfall, and other natural environmental features (Lin J et al., 2021; Chang et al., 2022). For instance, a study in the Fujian delta region, South China, showed that the incidence of geological disasters is higher at an altitude of 600–800 m, a slope of 6–15°, a southwest orientation, and a distance of 200 m from the river than other regions (Lin J et al., 2021). But another study showed that 90.2% of the total geological disasters are distributed at altitudes over 1,000 m in mountainous rural areas (Jiang et al., 2016). The abovementioned studies indicate that the subdivision of the spatial distribution of geological disasters is determined by local topographic features. Furthermore, some scholars argue that the disturbance of human activities is essential in inducing geological disasters. Therefore, the geological disaster-prone areas are primarily distributed in areas with dense populations and economic activities (Zhao D. et al., 2021; Wang et al., 2021). In mountainous rural areas, due to the disturbance of the rapid construction of engineering infrastructure, geological disasters are often distributed in a linear pattern along traffic arteries, and in a cluster pattern along townships and settlements (Qin et al., 2021). In addition, the distribution of geological disasters spots is also related to other influencing factors. For instance, geological disasters are often distributed in areas with low vegetation coverage because the root system of vegetation can improve soil stability.
In summary, the spatial distribution of geological disasters is essential to disaster prevention research. A clear understanding of the distribution pattern of geological disasters is of great significance for formulating effective countermeasures. Previous studies have established a cognitive system of the spatial distribution characteristics of geological disasters in China, revealing the heterogeneity of the spatial distribution of geological disasters and enabling us to have a macroscopic understanding of the distribution of geological disasters. However, as mentioned above, the distribution of geological disasters is closely related to the local geographical and natural environment and human activities, and micro-scale research can help to fine-grained knowledge of the distribution characteristics of regional geological disasters, which in turn can help in disaster prevention and mitigation planning, resource allocation optimization, etc.
2.2 Influencing factors of geological disasters
The influencing factors of geological disasters are divided into two categories, natural environment and human activities (Lin J et al., 2021). In recent years, geological risk assessment has attracted increasing scholarly attention, especially regarding the factors influencing geological disasters. Numerous studies have shown that a complex interaction of global and functionally region-specific factors governs geological disasters (Li et al., 2016; Wang et al., 2020; Tang et al., 2021), such as elevation, slope, rainfall, population density, distance from faults, etc. (Youssef et al., 2012; Li et al., 2013; Wang et al., 2021; Siddique et al., 2022; Wang et al., 2022). For instance, Qin et al. (2010) examined the relationship between landslides and the topography in Wenchuan County, which showed that the number of landslides is positively correlated with the slope and elevation, while negatively associated with aspect. Another study also in the mountainous areas in southern China showed that elevation, distance from faults and slope are the main influencing factors causing geological disasters (Lin J et al., 2021). Furthermore, according to the time of high frequency of geological disasters, debris flows and landslides primarily occur in the summer months of June to August, when there is very heavy and prolonged rainfall. Therefore, the water system and rainfall are crucial factors inducing geological disasters.
The abovementioned studies provide the basis for a deeper understanding of geological disasters. However, most studies focused on single-type disasters such as debris flow (Chiou et al., 2015), landslides (Bai et al., 2010; Chen et al., 2017; Li et al., 2017; Deng et al., 2022; Lv et al., 2022), rockfall (Chiessi et al., 2010), flood hazard (Wang et al., 2015), etc., or adopted macro-regional scale investigations such as coastal areas (China) (Pan, 2016), Pearl River Delta (China) (Li H. et al., 2021), urban agglomerations (Lin J et al., 2021), and river basin (Chiou et al., 2015; Dong et al., 2021; Lv et al., 2022). Most micro-scale studies at the county level also lacked multi-type disaster investigations (Zhao Z. et al., 2021). In summary, to have a more precise view of geological disasters in China’s mountainous rural areas, fine-scale multi-type disaster analysis procedures are needed to provide a more robust interpretation and analysis regarding the distribution, preparedness, and avoidance of geological disasters.
3 Materials and methods
3.1 Study area
Meigu County, located in Southwestern China (102°53′-103°21′E, 28°02′-28°54′N), covers a total area of approximately 2,573 km2. At the end of 2020, the county had a population of 0.26 million distributed in one town and 35 townships according to official statistics (Figure 1) (http://www.meigu.gov.cn/). The region is geologically or geomorphologically complex and is characterized by a typical four-season pattern with an annual temperature of 11.4°C (Song et al., 2020). The rainy season with a mean yearly rainfall of approximately 814.6 mm, stretches between June and September, with heavy rainstorms occurring in the summer. The complex geological, geomorphological, and climatic characteristics of the region trigger an increasing occurrence of geological human-affecting disasters (Tang et al., 2020), which primarily include landslides, desire flows, and collapse (Figure 2). Xinhua News Agency reported that a continuous heavy rainfall turned into severe debris flow in Meigu County on 26 June 2016, resulting in 79 people injured, six missing, 20 ha of arable lands and 16 houses damaged, including ten partially affected, five collapsed and one buried house (www.xinhuanet.com). In addition, according to the statistics of the Megu County government, 75 PGDSs were identified from 2016–2020, resulting in 722 rural households and 68.21 million yuan in the property at serious risk (http://www.meigu.gov.cn/). In recent years, with increasing extreme weather conditions and continuous progress of human engineering activities, the region’s rural settlements have faced heightened challenges and threats of geological disasters.
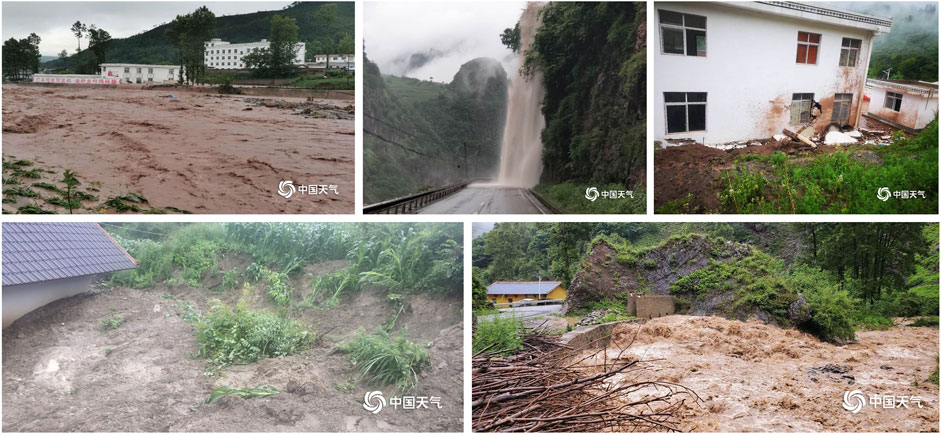
FIGURE 2. Geological disasters in the study area. (Photo source: China Weather, http://www.weather.com.cn/).
3.2 Methods
We analyzed the PGDSs spatial distribution characteristics using Average Nearest Neighbor Ratio (ANN), Kernel Density Estimation (KDE) and Standard Deviational Ellipse (SDE) models. Then, we constructed OLS regression models to identify the main influencing factors to PGDSs. The analytical framework is shown in Figure 3.
3.2.1 Spatial distribution analysis of potential geological disasters spots
3.2.1.1 Standard deviational ellipse
SDE explores the spatial distribution direction and trend characteristics of point elements (Xu F. et al., 2018; Yang et al., 2021). Its standard basic parameters include the center, long axis, short axis and azimuth of the ellipse. The center indicates the relative position of its distribution, the long axis characterizes its dispersion in the direction of the primary trend, and the azimuth reflects the direction of the primary distribution trend (Zhang et al., 2022).
Where θ refers to the azimuth of the ellipse and the standard deviation along the major axis x and minor axis y of the SDE.
3.2.1.2 Average nearest neighbor
ANN measures the spatial distribution characteristics of point-like elements by using a criterion.
Where R is the ratio, D0 is the actual nearest neighbor distance, and D is the theoretical closest neighbor distance. A is the area of the study area, and N is the number of point-like elements. The spatial distribution characteristics of PGDSs are evaluated according to the value of R: R = 1 indicates random distribution, R < 1 indicates agglomerative distribution, R > 1 indicates discrete distribution.
3.2.1.3 kernel density estimation
KDE is a common spatial analysis method (Dong et al., 2020; Song and Li, 2020; Xu et al., 2022), used to visualize the spatial clustering status of PGDSs.
Where, k is the kernel function, h is the bandwidth with a value greater than 0, n is the number of spots within the bandwidth, di is the distance of the location from the ith observed location, and a fixed bandwidth value is used in this study.
3.2.2 Ordinary least squares regression model
3.2.2.1 Ordinary least squares model
The OLS method involves a global linear regression model and is a multivariate linear function between the dependent and independent variables (Yang L et al., 2020a; Yang L et al., 2020b; Guo et al., 2020; Yang et al., 2022).
Where Yi is the dependent variable, β0, βk and ε represent a constant, the regression coefficient, and a random error term, respectively.
3.2.2.2 Dependent and explanatory variables
The identification of influencing factors is critical for the construction of the regression model. We selected the density of PGDSs in each unit as the dependent variable. As described, the natural environment and human activities are the two main influencing factors inducing geological disasters. Therefore, for the explanatory variables, we divided the eight variables into four groups based on the previous studies and field investigation: terrain, human activities, climate, and ecology. Steep terrain and slopes are more likely to form landslides and debris flows, so the terrain category must represent the complex and variable terrain conditions. Elevation, slope, and aspect are selected by referencing relevant studies. For the human activities category, we choose population density and GDP as the variables representing the density of populations and economic activities. Furthermore, frequent climate change affects the distribution of rainfall and vegetation growth, to a certain extent, can lead to the potential occurrence of geological disasters. Thus, rainfall and temperature are selected as the proxy of the climate category. The event of geological disasters is usually concentrated in the summer months of June to October in the study area; therefore, rainfall and temperature data are collected for the same periods and are averaged. Previous studies have shown that vegetation coverage is an essential factor induce geological disaster. High vegetation coverage can enhance soil stability, reduce erosion, and prevent landslides and debris flow geological disasters. Previously, we tried to use the biological abundance index and soil erosion intensity to reflect the ecological environment. But in the remote mountainous rural areas of China, it is difficult to obtain fine data to support micro-scale studies. Therefore, we attempt to represent the fragile ecological environment of Meigu County using NDVI. Compared with the relevant studies, we did not select the variables such as stratigraphic lithology, distance from water, or distance from fault (Xi et al., 2021; Wang et al., 2022), because the topography of the study area is highly undulating and significantly different from the two-dimensional planar analysis, and the above factors can hardly reflect the variability of the variables in the vertical dimension. Finally, the explanatory variables include elevation, slope, aspect, normalized difference vegetation index (NDVI), gross domestic product (GDP), population density, rainfall, and temperature (Table 1; Figure 4).
3.3 Data
The data source used in this research are summarized as follows:
(1) The PGDSs data, including 137 spots, were obtained from the geospatial data cloud (China, http://www.gscloud.cn/).
(2) The vector data were obtained from the national 1:1 million public versions topographic data (2021) provided by the National Catalogue Service for Geographic Information in China (https://www.webmap.cn/main.do?method=index).
(3) Digital elevation model (DEM) data was obtained from a geospatial data cloud (China, http://www.gscloud.cn/) and used to calculate elevation, aspect, and slope; population density data were obtained from World POP (https://www.worldpop.org/). GDP, rainfall data, and NDVI data were obtained from resource and environmental science and data center (China, https://www.resdc.cn/Default.aspx). The data of rainfall and temperature were averaged over July-September data.
The study area was divided into 36 units consisting of one town and 35 townships. Descriptive statistics of the explanatory variables are given in Table 2.
4 Results
4.1 Spatial distribution of potential geological disasters spots
As seen in Figure 5, Meigu county suffers from a multitude of PGDSs, among which debris flow is the primary type of disaster, with a number of 69, accounting for 50.36% of the total; followed by landslide, with a number of 61 and a proportion of 44.53%. These two PGDSs are densely distributed along the Meigu river. The number of unstable slopes is only 7, accounting for 5.11% of the total, mainly distributed in Jiukou Township and Erqi Township in the south of the study area.
Combined with the SDE result (Figure 6), PGDSs present a north-to-south direction distribution pattern except for unstable slopes and landslides which were unevenly distributed across the study area.
The results of the ANN model showed that the spatial distribution of geological disasters has a significant accumulation characteristic except for collapses (Table 3). Regarding accumulation, desire flows were the highest, with an R of 0.512, followed by landslides (R = 0.3854). Only the collapse reflected a dispersed distribution characteristic (R = 1.60).
The kernel density map was divided into five levels using the natural break-point method in ArcGIS (version 10.5): low, medium-low, medium, medium-high, and high (Figure 7). The results showed that: (i)The spatial distribution of landslides formed four agglomeration areas, in which Caihong (CH) township, Equgu (EQG) township, Jingyetexi Township (JYTX) and Bapu (BP) Township were categorized as core areas (Figure 7A). 2) The spatial distribution of mudslides formed two belt-like shapes (Figure 7B). (3) The collapse was mainly concentrated in Jiukou Township (JK), and the junction of the Lamuajue Township (LMQJ) and Erqi Township (EQ) (Figure 7C). In summary, the spatial distribution of PGDSs has a significant heterogeneity with cluster formations (Figure 7D).
4.2 Patterns of potential geological disasters spots incorporating explanatory variables
(1) The elevation of the study area ranged from 650 to 4100 m and was divided into 500 m interval classes referencing the previous studies (Li et al., 2013), then we superimposed PGDSs. Through the statistical analysis, we found that approximately 95.65% of the PGDSs distribute below 2500m, and the largest percentage of PGDSs distribute between 1500m and 2000m (Figure 8A).
(2) The slope ranges from 0–69.5° and can also be divided into seven grades at 10°intervals. It is shown that 92.70% of the population density occur below the slope of 30°. The largest percentage of PGDSs distribute between 10° and 20°; the ratio was 48.91% (Figure 8B).
(3) The aspect is divided into eight grades at 45° intervals, which represent eight common directions, namely north (0–22.5°,337.5–360°), northeast (22.5–67.5°), east (67.5–112.5°), southeast (112.5–157.5°), south (157.5–202.5°), southwest (202.5–247.5°), west (247.5–292.5°), northwest (292.5–337.5°). We found that most PGDSs distribute in the west direction (accounting for 25.55% of the total) (Figure 8C).
(4) The population density was divided into five grades at 500 pcs/km2 intervals. Most PGDSs distribute in the 500–1,000 pcs/km2; the ratio is 29.20% (Figure 8D).
(5) The GDP was also divided into five grades at 20 thousand CNY/1 km2 intervals. Most PGDSs distribute in the 1.20–1.40 million CNY/km2; the ratio is 27.74% (Figure 8E).
(6) The NDVI was also divided into five grades at 0.2 intervals. Most PGDSs distribute in the range of 0.6–0.8; the ratio is 60.85% (Figure 8F).
(7) The rainfall was divided into five grades at 20 mm intervals. Most PGDSs distribute in the range of over 240 mm; the ratio is 41.61% (Figure 8G).
(8) The temperature was divided into five grades at 2°C. We found most PGDSs distribute in the range of 19–21°C; the ratio is 40.88% (Figure 8H).
4.3 Correlation coefficient and the impacts of determinants
Table 4 shows the results of the OLS model. The VIF of the explanatory variables are all lower than the threshold of 10 except for temperature (the variable will be removed in the OLS model), indicating no obvious collinearity. It can be seen in Table 4 that the PGDSs are significantly correlated with slope, aspect, population density, and rainfall. The coefficient values are 1.398, 0.137, 0.010, and 1.979, respectively (p < 0.05). The adjusted R-squared is 0.5493, which means the model has a good explanatory. The model could be shown as follows: YPGDSs = −439.318 + 1.398 X1 + 0.1367 X2 + 0.010 X3 + 1.979 X4, where YPGDSs was the density of PGDSs in each unit, X1 is slope, X2 is aspect, X3 is population density, and X4 is rainfall.
5 Discussion
This study attempts to identify the PGDSs spatial distribution characteristics and its influencing factors in the geological disaster-prone mountainous rural areas, the results reveal that rainfall and slope are the main contributing factors to PGDSs occurrence.
5.1 Spatial distribution of potential geological disasters spots in mountainous rural areas
Due to their remote location and sparse population, mountainous rural areas have received little scholarly attention (Klein et al., 2003; Chen et al., 2008). We emphasize the importance of conducting research on mountainous rural areas for two reasons: First, most mountainous rural areas are always geologically disaster-prone in China, thus hindering its development (Xu et al., 2017), where Geological Disaster Induced Poverty (GDIP; yin zai zhi pin) is a frequent problem (Xu et al., 2020). Second, there is an essential need to investigate the spatial distribution and influencing factors of PGDSs on a fine scale (Wang et al., 2020). We found that PGDSs are distributed westwardly (247.5–292.5°) in an elevation range of 1,500–2000 m, the surface slope of 10–20°, high vegetation cover of 0.6 < NDVI <0.8, and high average monthly rainfall of >240 mm which is approximately in agreement with the findings of Lin J et al., 2021 who showed that PGDSs are distributed in areas with low elevation (200–400 m), gentle slopes (5–15°), and high rainfall (annual average 1500–1600 mm). The results, however, contradict those of previous studies (Chiessi et al., 2010; Li et al., 2013; Lin J et al., 2021), probably due to differences in topographical attributes and highlight the importance of geographical differences when analyzing the PGDSs spatial distribution and its influencing factors.
Some unexpected findings are obtained. First, the density of PGDSs is a surrogate for its susceptibility in mountainous areas, consistent with the findings of Jiang et al. (2016). More clearly, the spatial distribution of PGDSs is a simpler and more efficient path to identifying PGDSs susceptibility than constructing a causal relationship with topographical and climatic explanatory variables. Second, in this research, we found that the spatial distribution of landslide and debris flow seems to have possible correlations that merit further investigation.
5.2 Geographical differentiation of influencing factors
In comparison with previous studies, we found that rainfall and slope are the main factors affecting PGDSs incidence (Table 4), which differs from those of Wang et al. (2021). Furthermore, another study suggested that human activities and rainfall are the most prominent factors in similar mountainous areas (Wang et al., 2020). Aiming to characterize the magnitude effect of these factors, we found that rainfall and slope have a stronger correlation with PGDS occurrence than other factors. Consistent with these findings, Smyth & Royle (2000) confirmed that heavy rainfall and natural environment features are the primary triggers of PGDS incidence. Consistent with the findings, natural factors had a more profound impact on PGDSs in mountainous areas than human activities, possibly because of the low population density and their insignificant effects in mountainous rural areas. Overall, it can be concluded that there exist similarities in the forming mechanism of micro-scale PGDSs in mountainous rural areas. We also found a non-strong correlation between NDVI and PGDS; however, PGDSs are mainly distributed in high vegetation areas, primarily in steep slope regions.
5.3 Recommendation for potential geological disasters spots avoidance
Arguably, based on most previous studies, human engineering activities are always the main but unavoidable influencing factors increasing the susceptibility of PGDSs in mountainous rural areas (Tselios and Tompkins, 2020; Tan et al., 2021; Khan et al., 2022). These factors are a consequence of rural development and can potentially be used for PGDS prevention. Additionally, although settlement relocation policies have proven to be an effective strategy for PGDSs prevention in disaster-proven areas (Xu et al., 2020), these strategies have limited practical effectiveness in mountainous rural areas which suffer from widespread low development suitability (Liu et al., 2022). Under these conditions, a good understanding of the PGDSs spatial distribution and influencing factors is essential in PGDS preparedness.
As concluding recommendations, a careful suitability analysis and planning considering topographical and climatic features are needed to identify low PGDSs affected areas for settlement relocation and future developments. Second, a meteorological risk warning system can be established for the region’s rural communities against ubiquitous and recurrent PGDSs, such as continuous heavy rainfalls, which is a critical factor that induces PGDS in mountainous areas. Finally, a combination of prevention and preparedness strategies and practical planning measures, such as the engineering of unfavorable terrains or relocation, should be constructed to avoid high-risk PGDSs (Li H. et al., 2021).
5.4 Research limitations
In this research, we analyzed the PGDSs spatial pattern and influencing factors to reflect on the PGDSs distribution characteristics and possible planning recommendations. However, due to limited data accuracy, this study neglected to include the PGDSs extent (e.g., large, medium, small) and level (e.g., minor, moderate, severe). Furthermore, this study only explored the PGDSs potentialities in the region and suggested holistic susceptibility and vulnerability assessments and the construction of evaluation indicator systems to gain practical insights. Moreover, the study area is a mountainous rural area, while the soil geological map is two-dimensional that does not truly reflect the current situation. Therefore, we did not consider the influence of soil properties on PGDSs. In the future, we will combine field surveys to obtain more fine-grained data.
6 Conclusion
Taking Meigu County as the study case, the paper analyzed the spatial distribution characteristics, patterns, and influencing factors of PGDS using GIS technology. Compared with the previous studies, we focused on mountainous rural areas to perform a fine-scale multi-type PGDS analysis using multiple analysis methods (e.g., ANN, KDE, SDE, etc.). We found PGDSs are distributed in an elevation range of 1,500–2000 m which receives a mean monthly rainfall of more than 240 mm and in highly foliated (0.6 < NDVI <0.8) west-facing slopes. We also found that PGDSs significantly correlate with slope, aspect, population density, and rainfall. The results can provide important implications for land use planning of resource allocation for disaster prediction and prevention.
Data availability statement
The original contributions presented in the study are included in the article/Supplementary Material, further inquiries can be directed to the corresponding author.
Author contributions
BS: conceptualization, funding acquisition, supervision, and writing—original draft. YC: formal analysis and writing—review and editing. MA: writing—review and editing. RZ: writing—review and editing.
Funding
This work was supported by the Science and Technology Department of Sichuan Province (No. 2020YFS0309), Si Chuan Research Center of Yi Culture (No. w202311).
Conflict of interest
The authors declare that the research was conducted in the absence of any commercial or financial relationships that could be construed as a potential conflict of interest.
Publisher’s note
All claims expressed in this article are solely those of the authors and do not necessarily represent those of their affiliated organizations, or those of the publisher, the editors and the reviewers. Any product that may be evaluated in this article, or claim that may be made by its manufacturer, is not guaranteed or endorsed by the publisher.
References
Ao, Y., Zhang, H., Yang, L., Wang, Y., Martek, I., and Wang, G. (2021). Impacts of earthquake knowledge and risk perception on earthquake preparedness of rural residents. Nat. Hazards (Dordr) 107 (2), 1287–1310. doi:10.1007/s11069-021-04632-w
Bai, S., Wang, J., Lu, G., Zhou, P., Hou, S., and Xu, S. (2010). GIS-based logistic regression for landslide susceptibility mapping of the Zhongxian segment in the Three Gorges area, China. Geomorphology 115 (1-2), 23–31. doi:10.1016/j.geomorph.2009.09.025
Bulte, E., Xu, L., and Zhang, X. (2018). Post-disaster aid and development of the manufacturing sector: Lessons from a natural experiment in China. Eur. Econ. Rev 101, 441–458. doi:10.1016/j.euroecorev.2017.10.019
Chang, M., Dou, X., Tang, L., and Xu, H. (2022). Risk assessment of multi-disaster in mining area of guizhou, China. Int. J. Disaster Risk Reduct 78, 103128. doi:10.1016/j.ijdrr.2022.103128
Chen, S., Ferng, J., Wang, Y., Wu, T., and Wang, J. (2008). Assessment of disaster resilience capacity of hillslope communities with high risk for geological hazards. Eng. Geol 98 (3-4), 86–101. doi:10.1016/j.enggeo.2008.01.008
Chen, W., Xie, X., Wang, J., Pradhan, B., Hong, H., Bui, D., et al. (2017). A comparative study of logistic model tree, random forest, and classification and regression tree models for spatial prediction of landslide susceptibility. Catena 151, 147–160. doi:10.1016/j.catena.2016.11.032
Chiessi, V., D'Orefice, M., Mugnozza, G. S., Vitale, V., and Cannese, C. (2010). Geological, geomechanical and geostatistical assessment of rockfall hazard in San Quirico Village (Abruzzo, Italy). Geomorphology 119 (3-4), 147–161. doi:10.1016/j.geomorph.2010.03.010
Chiou, I., Chen, C., Liu, W., Huang, S., and Chang, Y. (2015). Methodology of disaster risk assessment for debris flows in a river basin. Stoch. Environ. Res. Risk Assess 29 (3), 775–792. doi:10.1007/s00477-014-0932-1
Deng, N., Li, Y., Ma, J., Shahabi, H., Hashim, M., de Oliveira, G., et al. (2022). A comparative study for landslide susceptibility assessment using machine learning algorithms based on grid unit and slope unit. Front. Environ. Sci 10. doi:10.3389/fenvs.2022.1009433
Dong, J., Peng, J., Liu, Y., Qiu, S., and Han, Y. (2020). Integrating spatial continuous wavelet transform and kernel density estimation to identify ecological corridors in megacities. Landsc. Urban Plan 199, 103815. doi:10.1016/j.landurbplan.2020.103815
Dong, Z., Wang, G., Amankwah, S. O. Y., Wei, X., Hu, Y., and Feng, A. (2021). Monitoring the summer flooding in the Poyang Lake area of China in 2020 based on Sentinel-1 data and multiple convolutional neural networks. Int. J. Appl. Earth Observation Geoinformation 102, 102400. doi:10.1016/j.jag.2021.102400
Fedeski, M., and Gwilliam, J. (2007). Urban sustainability in the presence of flood and geological hazards: The development of a GIS-based vulnerability and risk assessment methodology. Landsc. Urban Plan. 83 (1), 50–61. doi:10.1016/j.landurbplan.2007.05.012
Gan, L., Wang, L., Hu, Z., Lev, B., Gang, J., and Lan, H. (2022). Do geologic hazards affect the sustainability of rural development? Evidence from rural areas in China. J. Clean. Prod 339, 130693. doi:10.1016/j.jclepro.2022.130693
Guo, A., Yang, J., Sun, W., Xiao, X., Cecilia, J., Jin, C., et al. (2020). Impact of urban morphology and landscape characteristics on spatiotemporal heterogeneity of land surface temperature. Sustain. Cities Soc 63, 102443. doi:10.1016/j.scs.2020.102443
Hou, J., Lv, J., Chen, X., and Yu, S. (2016). China’s regional social vulnerability to geological disasters: Evaluation and spatial characteristics analysis. Nat. Hazards (Dordr). 84 (1), 97–111. doi:10.1007/s11069-015-1931-3
Jiang, W., Deng, Y., Tang, Z., Cao, R., Chen, Z., and Jia, K. (2016). Adaptive capacity of mountainous rural communities under restructuring to geological disasters: The case of Yunnan Province. J. Rural Stud. 47, 622–629. doi:10.1016/j.jrurstud.2016.05.002
Khan, I., Ali, A., Waqas, T., Ullah, S., Ullah, S., Shah, A. A., et al. (2022). Investing in disaster relief and recovery: A reactive approach of disaster management in Pakistan. Int. J. Disaster Risk Reduct. 75, 102975. doi:10.1016/j.ijdrr.2022.102975
Kim, D., Won, J., Lee, E., Park, K. R., Kim, J., Park, S., et al. (2022). Disaster assessment using computer vision and satellite imagery: Applications in detecting water-related building damages. Front. Environ. Sci 10. doi:10.3389/fenvs.2022.969758
Klein, R. J. T., Nicholls, R. J., and Thomalla, F. (2003). Resilience to natural hazards: How useful is this concept? Environ. Hazards 5 (1), 35–45. doi:10.1016/j.hazards.2004.02.001
Kong, Y., He, W., Zhang, Z., Shen, J., Yuan, L., Gao, X., et al. (2022). Spatial-temporal variation and driving factors decomposition of agricultural grey water footprint in China. J. Environ. Manag 318, 115601. doi:10.1016/j.jenvman.2022.115601
Li, H., Xu, E., and Zhang, H. (2021). Examining the coupling relationship between urbanization and natural disasters: A case study of the Pearl River delta, China. Int. J. Disaster Risk Reduct 55, 102057. doi:10.1016/j.ijdrr.2021.102057
Li, K., Geng, H., Yue, L., Li, K., and Huang, L. (2021). Spatial differentiation characteristics and driving mechanism of rural settlements transformation in the metropolis: A case study of pudong district, shanghai. Front. Environ. Sci. 9. doi:10.3389/fenvs.2021.755207
Li, L., Lan, H., Guo, C., Zhang, Y., Li, Q., and Wu, Y. (2017). A modified frequency ratio method for landslide susceptibility assessment. Landslides 14 (2), 727–741. doi:10.1007/s10346-016-0771-x
Li, M., Lv, J., Chen, X., and Jiang, N. (2015). Provincial evaluation of vulnerability to geological disaster in China and its influencing factors: A three-stage DEA-based analysis. Nat. Hazards (Dordr) 79 (3), 1649–1662. doi:10.1007/s11069-015-1917-1
Li, S., Zhou, Z., Li, L., Lin, P., Xu, Z., and Shi, S. (2016). A new quantitative method for risk assessment of geological disasters in underground engineering: Attribute Interval Evaluation Theory (AIET). Tunn. Undergr. Space Technol 53, 128–139. doi:10.1016/j.tust.2015.12.014
Li, X., Liu, X., Li, J., Wang, Q., Liao, W., and Zhang, L. (2013). Factor analysis of earthquake-induced geological disasters of the M7. 0 Lushan earthquake in China. Geodesy Geodyn. 4 (2), 22–29. doi:10.3724/SP.J.1246.2013.02022
Lin, J., Chen, W., Qi, X., and Hou, H. (2021). Risk assessment and its influencing factors analysis of geological hazards in typical mountain environment. J. Clean. Prod. 309, 127077. doi:10.1016/j.jclepro.2021.127077
Lin, J., Lin, M., Chen, W., Zhang, A., Qi, X., and Hou, H. (2021). Ecological risks of geological disasters and the patterns of the urban agglomeration in the Fujian Delta region. Ecol. Indic. 125, 107475. doi:10.1016/j.ecolind.2021.107475
Liu, J., Jin, X., Xu, W., and Zhou, Y. (2022). Evolution of cultivated land fragmentation and its driving mechanism in rural development: A case study of jiangsu province. J. Rural Stud. 91, 58–72. doi:10.1016/j.jrurstud.2022.02.011
Liu, X., Yu, C., Shi, P., and Fang, W. (2012). Debris flow and landslide hazard mapping and risk analysis in China. Front. Earth Sci. 6 (3), 306–313. doi:10.1007/s11707-012-0328-9
Lv, L., Chen, T., Dou, J., and Plaza, A. (2022). A hybrid ensemble-based deep-learning framework for landslide susceptibility mapping. Int. J. Appl. Earth Observation Geoinformation 108, 102713. doi:10.1016/j.jag.2022.102713
Oliveira, S., Pereira, J. M. C., San-Miguel-Ayanz, J., and Lourenco, L. (2014). Exploring the spatial patterns of fire density in southern europe using geographically weighted regression. Appl. Geogr 51, 143–157. doi:10.1016/j.apgeog.2014.04.002
Pan, A. P. (2016). Study on mobility-disadvantage group' risk perception and coping behaviors of abrupt geological hazards in coastal rural area of China. Environ. Res. 148, 574–581. doi:10.1016/j.envres.2016.04.016
Pu, C., Xu, Q., Zhao, K., Chen, W., Wang, X., Li, H., et al. (2022). Spatiotemporal evolution and surface response of land subsidence over a large-scale land creation area on the Chinese Loess Plateau. Int. J. Appl. Earth Observation Geoinformation 111, 102835. doi:10.1016/j.jag.2022.102835
Qi, S., Xu, Q., Lan, H., Zhang, B., and Liu, J. (2010). Spatial distribution analysis of landslides triggered by 2008.5.12 Wenchuan Earthquake. China. Eng. Geol 116 (1), 95–108. doi:10.1016/j.enggeo.2010.07.011
Qin, Y., Wu, J., Zhan, H., Xiong, C., Jia, C., Bai, X., et al. (2021). Discussion on the correlation between active fault and geological disaster distribution in the Ganzi area, Western Sichuan province, China. J. Geomechanics 27 (3), 463–474. doi:10.12090/j.issn.1006-6616.2021.27.03.042
Qing, C., Guo, S., Deng, X., and Xu, D. (2021). Farmers' disaster preparedness and quality of life in earthquake-prone areas: The mediating role of risk perception. Int. J. Disaster Risk Reduct 59, 102252. doi:10.1016/j.ijdrr.2021.102252
Siddique, T., Haris, P. M., and Pradhan, S. P. (2022). Unraveling the geological and meteorological interplay during the 2021 Chamoli disaster, India. Nat. Hazards Res 2 (2), 75–83. doi:10.1016/j.nhres.2022.04.003
Smyth, C. G., and Royle, S. A. (2000). Urban landslide hazards: Incidence and causative factors in niterói, rio de Janeiro state, Brazil. Appl. Geogr 20 (2), 95–118. doi:10.1016/S0143-6228(00)00004-7
Song, W., and Li, H. (2020). Spatial pattern evolution of rural settlements from 1961 to 2030 in Tongzhou District, China. Land Use Policy 99, 105044. doi:10.1016/j.landusepol.2020.105044
Song, Y., Jarvis, D. I., Bai, K., Feng, J., and Long, C. (2020). Assessment of the resilience of a tartary buckwheat (fagopyrum tataricum) cultivation system in Meigu, southwest China. Sustainability 12 (14), 5683. doi:10.3390/su12145683
Tan, Q., Bai, M., Zhou, P., Hu, J., and Qin, X. (2021). Geological hazard risk assessment of line landslide based on remotely sensed data and GIS, 169. Measurement doi:10.1016/j.measurement.2020.108370
Tang, F., Lin, F., Jin, C., Deng, G., Zhao, H., Tie, Y., et al. (2020). Practical geological technologies facilitating poverty alleviation in Wumeng Mountain area. China Geol. 3 (3), 504–508. doi:10.31035/cg2020055
Tang, M., Liu, P., Chao, X., and Han, Z. (2021). The performativity of city resilience for sustainable development of poor and disaster-prone regions: A case study from China. Technol. Forecast. Soc. Change 173, 121130. doi:10.1016/j.techfore.2021.121130
Tselios, V., and Tompkins, E. L. (2020). Can we prevent disasters using socioeconomic and political policy tools? Int. J. Disaster Risk Reduct 51, 101764. doi:10.1016/j.ijdrr.2020.101764
van Westen, C. J., Soeters, R., and Sijmons, K. (2000). Digital geomorphological landslide hazard mapping of the Alpago area, Italy. Int. J. Appl. Earth Observation Geoinformation 2 (1), 51–60. doi:10.1016/S0303-2434(00)85026-6
Wan, F., Guo, H., Li, J., Gu, M., Pan, W., and Ying, Y. (2021). A scheduling and planning method for geological disasters. Appl. Soft Comput 111, 107712. doi:10.1016/j.asoc.2021.107712
Wang, H., Wang, X., Zhang, C., Wang, C., and Li, S. (2022). Analysis on the susceptibility of environmental geological disasters considering regional sustainable development. Environ. Sci. Pollut. Res. Int. doi:10.1007/s11356-022-22778-3
Wang, J., Zhu, S., Luo, X., Chen, G., Xu, Z., Liu, X., et al. (2020). Refined micro-scale geological disaster susceptibility evaluation based on UAV tilt photography data and weighted certainty factor method in Mountainous Area. Ecotoxicol. Environ. Saf 189, 110005. doi:10.1016/j.ecoenv.2019.110005
Wang, X., Zhang, C., Wang, C., Liu, G., and Wang, H. (2021). GIS-based for prediction and prevention of environmental geological disaster susceptibility: From a perspective of sustainable development. Ecotoxicol. Environ. Saf 226, 112881. doi:10.1016/j.ecoenv.2021.112881
Wang, Z., Lai, C., Chen, X., Yang, B., Zhao, S., and Bai, X. (2015). Flood hazard risk assessment model based on random forest. J. Hydrology 527, 1130–1141. doi:10.1016/j.jhydrol.2015.06.008
Wu, S., Zhang, R., Wang, C., and Feng, D. (2022). The impact of natural disasters on rural household wealth: Micro evidence from China. Front. Environ. Sci 10. doi:10.3389/fenvs.2022.993722
Xi, X., Wang, S., Yao, L., Zhang, Y., Niu, R., and Zhou, Y. (2021). Evaluation on geological environment carrying capacity of mining city – a case study in Huangshi City, Hubei Province, China. Int. J. Appl. Earth Observation Geoinformation 102, 102410. doi:10.1016/j.jag.2021.102410
Xiao, Y., Tang, X., Li, Y., Huang, H., and An, B.-W. (2022). Social vulnerability assessment of landslide disaster based on improved TOPSIS method: Case study of eleven small towns in China. Ecol. Indic 143, 109316. doi:10.1016/j.ecolind.2022.109316
Xu, D., Liu, E., Wang, X., Tang, H., and Liu, S. (2018). Rural households, livelihood capital, risk perception, and willingness to purchase earthquake disaster insurance: Evidence from southwestern, China. Int. J. Environ. Res. Public Health 15 (7), 1319. doi:10.3390/ijerph15071319
Xu, D., Peng, L., Liu, S., Su, C., Wang, X., and Chen, T. (2017). Influences of migrant work income on the poverty vulnerability disaster threatened area: A case study of the three gorges reservoir area, China. Int. J. Disaster Risk Reduct 22, 62–70. doi:10.1016/j.ijdrr.2017.03.001
Xu, F., Li, H., and Bao, H. (2018). Performance comparisons of land institution and land regulation systems on water area decrease. Habitat Int. 77, 12–20. doi:10.1016/j.habitatint.2017.12.009
Xu, G., Jiang, Y. H., Wang, S., Qin, K., Ding, J. C., Liu, Y., et al. (2022). Spatial disparities of self-reported COVID-19 cases and influencing factors in Wuhan, China. Sustain. Cities Soc 76, 103485. doi:10.1016/j.scs.2021.103485
Xu, Y., Qiu, X., Yang, X., Lu, X., and Chen, G. (2020). Disaster risk management models for rural relocation communities of mountainous southwestern China under the stress of geological disasters. Int. J. Disaster Risk Reduct 50, 101697. doi:10.1016/j.ijdrr.2020.101697
Yang, L., Chau, K. W., Szeto, W. Y., Cui, X., and Wang, X. (2020a). Accessibility to transit, by transit, and property prices: Spatially varying relationships. Transp. Res. Part D Transp. Environ. 85, 102387. doi:10.1016/j.trd.2020.102387
Yang, L., Chu, X., Gou, Z., Yang, H., Lu, Y., and Huang, W. (2020b). Accessibility and proximity effects of bus rapid transit on housing prices: Heterogeneity across price quantiles and space. J. Transp. Geogr. 88, 102850. doi:10.1016/j.jtrangeo.2020.102850
Yang, L., Liang, Y., He, B., Lu, Y., and Gou, Z. (2022). COVID-19 effects on property markets: The pandemic decreases the implicit price of metro accessibility. Tunn. Undergr. Space Technol 125, 104528. doi:10.1016/j.tust.2022.104528
Yang, Z., Wu, D., and Wang, D. (2021). Exploring spatial path dependence in industrial space with big data: A case study of Beijing, Cities, 108. doi:10.1016/j.cities.2020.102975
Yao, J., Zhang, X., Luo, W., Liu, C., and Ren, L. (2022). Applications of Stacking/Blending ensemble learning approaches for evaluating flash flood susceptibility. Int. J. Appl. Earth Observation Geoinformation 112, 102932. doi:10.1016/j.jag.2022.102932
Youssef, A. M., Pradhan, B., Sabtan, A. A., and El-Harbi, H. M. (2012). Coupling of remote sensing data aided with field investigations for geological hazards assessment in Jazan area, Kingdom of Saudi Arabia. Environ. Earth Sci 65 (1), 119–130. doi:10.1007/s12665-011-1071-3
Zeng, X., Guo, S., Deng, X., Zhou, W., and Xu, D. (2021). Livelihood risk and adaptation strategies of farmers in earthquake hazard threatened areas: Evidence from sichuan province, China. Int. J. Disaster Risk Reduct 53, 101971. doi:10.1016/j.ijdrr.2020.101971
Zhang, X., Zhang, B., Yao, Y., Wang, J., Yu, F., Liu, J., et al. (2022). Dynamics and climatic drivers of evergreen vegetation in the Qinling-Daba Mountains of China. Ecol. Indic 136, 108625. doi:10.1016/j.ecolind.2022.108625
Zhao, D., Lancuo, Z., Hou, G., Xu, C., and Li, W. (2021). Assessment of geological disaster susceptibility in the hehuang valley of qinghai province. J. Geomechanics 27 (1), 83–95. doi:10.12090/j.issn.1006-6616.2021.27.01.009
Zhao, Z., Chen, J., Xu, K., Xie, H., Gan, X., and Xu, H. (2021). A spatial case-based reasoning method for regional landslide risk assessment. Int. J. Appl. Earth Observation Geoinformation 102, 102381. doi:10.1016/j.jag.2021.102381
Zhou, W., Guo, S., Deng, X., and Xu, D. (2021). Livelihood resilience and strategies of rural residents of earthquake-threatened areas in Sichuan Province, China. Nat. Hazards (Dordr). 106 (1), 255–275. doi:10.1007/s11069-020-04460-4
Zhuang, L., He, J., Yong, Z., Deng, X., and Xu, D. (2020). Disaster information acquisition by residents of China's earthquake-stricken areas. Int. J. Disaster Risk Reduct 51, 101908. doi:10.1016/j.ijdrr.2020.101908
Keywords: potential geological disasters spots, spatial distribution, mountainous rural areas, rainfall, disaster-prone region
Citation: Shu B, Chen Y, Amani-Beni M and Zhang R (2022) Spatial distribution and influencing factors of mountainous geological disasters in southwest China: A fine-scale multi-type assessment. Front. Environ. Sci. 10:1049333. doi: 10.3389/fenvs.2022.1049333
Received: 20 September 2022; Accepted: 09 November 2022;
Published: 24 November 2022.
Edited by:
Jun Yang, Northeastern University, ChinaCopyright © 2022 Shu, Chen, Amani-Beni and Zhang. This is an open-access article distributed under the terms of the Creative Commons Attribution License (CC BY). The use, distribution or reproduction in other forums is permitted, provided the original author(s) and the copyright owner(s) are credited and that the original publication in this journal is cited, in accordance with accepted academic practice. No use, distribution or reproduction is permitted which does not comply with these terms.
*Correspondence: Bo Shu, shubo@swjtu.edu.cn, shubo@mail.xhu.edu.cn