- 1Institute of Finance and Economics, Shanghai University of Finance and Economics, Shanghai, China
- 2Institute for Urban-Rural Development, Shanghai University of Finance and Economics, Shanghai, China
The input of chemical fertilizers to save land and the input of agricultural mechanization to save labor have been important driving forces in China’s grain production since the reform and opening-up. In recent years, the existing literature discussed the relationship between the scale of agricultural land and the application of chemical fertilizers but ignored the role of mechanization in agricultural production and management. We used the intermediary effect model to examine how the cropland use scale affected farmers’ fertilization behavior through agricultural mechanization investment. Our dataset contained data from the Fixed Observation Points of the Ministry of Agriculture and Rural Affairs in China conducted between 2015 and 2017. The results showed that the expansion of the cropland scale significantly reduced the fertilizer input per mu and indirectly reduced the fertilizer input of farmers by increasing the input of agricultural mechanization. In a word, agricultural mechanization played a mediation effect. Further heterogeneity results showed that because grain crops are easier to operate mechanically, the mediation effect was more significant; similarly, under the patterns of “south prefer to service, north prefer to self-purchase” and “south grow rice and north grow wheat,” southern areas received a more significant reduction effect in mainland China. These findings defined the role of agricultural mechanization and provided a new perspective for realizing the reduction and efficiency of chemical fertilizers and reducing agricultural non-point source pollution.
1 Introduction
Since the reform and opening-up, China’s grain output has increased from more than 304 million tons in 1978 to more than 669 million tons in 2020, facing the basic national conditions of a large population and limited land, as well as the continuous transfer of labor, which achieved consecutive harvest for 17 years, remaining 650 million tons and above for 6 years. Among all the relevant factors, fertilizers, as an important means of agricultural production, are considered to be a crucial driving force ensuring production, with a contribution rate of over 40%1. However, there has been a long-term problem of the intensive use of cropland and other resources, especially the blindly excessive fertilization in production. According to the China Statistical Yearbook complied by the National Bureau of Statistics of China, the fertilizer intensity has gradually risen from 59 kg/ha to 313 kg/ha from the year of 1978–2020. Although the fertilizer intensity began to decline from 2015 to 2020 due to the fact that China’s government issued the Action Plan for Zero Growth of Fertilizer Use by 2020, it is still much higher than 97.90 kg/ha worldwide excluding China (Zhu et al., 2022).
Research studies have pointed out that the excessive use of chemicals can cause serious environmental damage. This damage is not only agro-ecosystem function (Guo et al., 2010) but also bio-physical including human health (Chen et al., 2020), with economic loss estimates ranging from 7 to 10% of China’s agricultural gross domestic product (Norse and Ju, 2015). To cope with the aforementioned problems for ecological safety and food security, the No. 1 Central Document in 2021 clearly proposes the continuous promotion of fertilizer and pesticide reduction to control agricultural non-point source pollution and promote the sustainable development of agricultural ecology.
An important reason for excessive fertilization in China is the small cropland scale or, precisely speaking, the fragmented operation as China’s agriculture is uniquely characterized by an extremely egalitarian distribution of cultivated land (Wang et al., 2016). The cost and benefit are farmers’ priority in agricultural production and investment decision-making, while the small cropland scale may lead to insufficient incentives for long-term investment, and it is easier to ignore the increase in social cost due to agricultural non-point source pollution. Recent studies have shown that there is a negative relationship between the cropland scale and chemicals like fertilizers and pesticides (Qin and Lu, 2020; Wang et al., 2021). The low level of agricultural production incentives along with insufficiency of restraint results in behindhand ways of fertilization; thus, the amount of fertilizer used by large-scale households is significantly lower than that of small-scale households (Wu et al., 2018). Evidence has been found that increasing the cropland scale can increase the propensity for technology adoption, which applies not only to grain (Hu et al., 2019) but also to economic crops like apple (Ma and Abdulai, 2019), which is conducive to reducing the amount of fertilizer. Therefore, a large number of studies encourage land transfer to achieve moderate scale operations in order to encourage farmers’ long-term investment and adopt fertilizer reduction technologies to reduce fertilizer input (Ju et al., 2016; Gao et al., 2018; Ren et al., 2021). However, there do exist views that the expansion of the cropland scale cannot effectively alleviate the problem of the excessive application of fertilizers, and even aggravate farmers’ short-term production behavior (Bambio and Bouayad, 2018).
In the aforementioned literature on the effect of the cropland scale on fertilizer reduction, these scholars have analyzed different data as well as different methods to study the reduction path of the cropland scale from different perspectives, and they have also captured the differences between the connotation of the operation scale and potential of fertilizer reduction expressed by various land transfer scenarios, but the factor of agricultural mechanization is always somehow excluded, ignoring its deep influence on agricultural production input decisions. As a historic change to China’s small-scale agricultural economy, rather than representing a single transformational change, mechanization’s broad appeal to households results from an accumulation of incremental, overlapping, and complementary advantages (Belton et al., 2021), leading to a profound impact on the replacement of rural labor and agricultural management. Due to the acceleration of industrialization and urbanization, the scarcity and price of labor factors in agricultural production are increasing relative to land, machinery, and other factors. Along with the increasing investment in machinery, the development of mechanization has effectively replaced agricultural labor and promoted productivity (Yang et al., 2013; Zhang et al., 2017). In fact, the comprehensive mechanization rate of cultivation and harvest in China is 71% and that of major crops exceeds 80%. In 2020, the total power of agricultural machinery nationwide is 1.03 billion kilowatts and the number of agricultural machinery is 204 million units (sets); agricultural production has entered a new stage dominated by machinery. It is worth noting that although few studies scientifically and systematically demonstrate whether the substitution of agricultural machinery for labor will improve environmental efficiency, some mechanized measures in agricultural production can help water-saving as well as fertilizer-saving, which may have a certain impact on the application of fertilizers (Ren et al., 2021).
Therefore, it is high time to discuss the effect of the cropland scale on the reduction of fertilizers without missing agricultural mechanization. So, what kind of role does agricultural mechanization play in the reduction of fertilizers in scaled operations? Specifically, does agricultural mechanization have a mediation effect? This study uses the panel data of the Fixed Observation Points from 2015–2017 to conduct empirical tests. The results show that the cropland scale has both direct and indirect effects on fertilizer reduction. A larger cropland scale can significantly increase the mechanization level, while a higher agricultural mechanization level reduces the input of fertilizers per mu. Further analysis shows that the mediation effect of agricultural mechanization is stronger in grain-oriented growers. Moreover, under the patterns of the “South prefer service, north prefer self-purchase” and “South grow rice and north grow wheat,” southern areas receive a more significant reduction effect.
Compared with the existing literature, the marginal contributions of this study are as follows. First, based on the reality of the continuous improvement of agricultural mechanization, agricultural mechanization is included in the analysis framework of “land scale–fertilizer reduction” to explain the reduction effect of mechanization, broadening the relevant research field. The second is to use the Fixed Observation Points data to empirically verify how agricultural mechanization inputs affect farmers’, especially scaled farmers’ fertilization behavior, providing new empirical evidence for fertilizer reduction and agricultural non-point source pollution containment.
The remainder of the paper is organized as follows. In the following part, we present the theoretical mechanism, where we explain the relationship between the cropland scale, mechanization level, and fertilizer reduction in detail. Section 3 formalizes the empirical strategy and introduces data and variables. Section 4 provides the estimated results and interpretation. Section 5 further delves into the stability and heterogeneity discussion. Finally, Section 6 concludes.
2 Theoretical mechanism
Before empirical analysis, we need to sort out the influential mechanism among the cropland scale, agricultural mechanization, and fertilization behavior. In order to clarify the mechanism, we need to delve into two issues: how the cropland scale affects the mechanization level, and how the mechanization level affects the fertilization behavior, as shown in Figure 1.
2.1 Cropland scale and mechanization level
As one of the crucial factors restricting the development of agricultural mechanization, a limited cropland scale comes with inconvenience for agricultural machinery operation while a larger cropland scale increases the need for mechanization (Wang et al., 2016). The cropland scale mainly affects agricultural mechanization through the following aspects: first, limited cropland hinders agricultural economies of scale, which means that it is difficult to effectively share the fixed input required for mobile labor and agricultural machinery, thereby increasing the cost of machinery utilization. Second, small plots and mixed varieties of cropland can increase the difficulty of mechanical operations and reduce the efficiency of agricultural machinery operations. Thus, farmers tend to rely mainly on manual operations supplemented by smaller agricultural machinery, which is not conducive to the full use of large-scale agricultural machinery operations. Third, the decentralized operation increases the transit time of agricultural machinery in various plots and increases the utilization cost of machinery services, which is not conducive to the organization of agricultural machinery services.
As fragmentation of land is not conducive to the effective use of agricultural land, most studies generally encourage the development of agricultural mechanization with large-scale land (Levia and Morris, 2001). Under the background that the rural labor force is generally aging and widespread rural labor shortage, the development of agricultural machinery has effectively replaced the agricultural labor force and promoted the increase of food production. With the continuous increase in the scale of arable land, farmers’ demand for mechanical substitution of labor continues to rise. Mechanization can increase the efficiency of the labor force unit and greatly increase the labor’s ability to cultivate in unit time, which is conducive to increasing farmers’ income (Otsuka, 2013).
2.2 Agricultural mechanization and fertilization behavior
Agricultural mechanization refers to the process of using advanced and applicable agricultural machinery to equip agriculture, improve agricultural production and operation conditions, and continuously improve the agricultural production technology level and economic and ecological benefits. Built on the existing literature and practical experience, agricultural mechanization can mainly affect fertilization behavior through the following paths:
2.2.1 Factor substitution
Studies have pointed out that there is a substitution between fertilizer and land, fertilizer and labor, and fertilizer and machinery (Wu et al., 2018; Zhang et al., 2020; Ren et al., 2021). On one hand, fertilizer and machinery are all capital inputs. As a rational individual, farmers prefer to invest in the least production factors while ensuring the same output. Although some studies have pointed out that compared with higher-cost machinery, farmers are more inclined to use fertilizers to substitute labor (Lu and Xie, 2018); it means that with constrained budget, an increase input of agricultural machinery means a reduction in other capital inputs including fertilizer. On the other hand, as agricultural machinery can significantly replace labor (Liu et al., 2014), fertilization behavior may be affected by labor reduction. At the same time, labor transfer may change households’ budget constraints, thereby simultaneously affecting the operation input (Zheng et al., 2022). Therefore, it can be clearly seen that the magnitude of the effect of agricultural mechanization on fertilizer reduction depends not only on the relative price changes between different factors but also on the degree of substitution between factors in agricultural production, which requires quantitative analysis through empirical evidence.
2.2.2 Planting structure
Agricultural mechanization will affect fertilization behavior by adjusting the planting structure. The operation modes of grains and economic crops show differences: since grains are normally land-intensive, they are easier to be mechanized than economic crops. Therefore, the level of agricultural mechanization would induce the tendency to plant grains. For example, Jiangsu and Shandong provinces in China are mainly plain areas with relatively flat cultivated land, which can the substitution of machinery for labor through mechanization. Farmers will allocate more agricultural resources to grains. Furthermore, there exists heterogeneity in fertilizer consumption within different crops. According to the China Fertilizer Consumption Use Efficiency Evaluation Report (2000–2015), most grains’ fertilizer consumption levels tend to be stable, while those of economic crops such as sugar cane, cotton, and apple have witnessed an obvious rising trend. Particularly, benefit-oriented farmers prefer to use more chemicals in high-valued economic crops to ensure production. Therefore, agricultural mechanization can affect fertilization by changing the planting structure.
2.2.3 Technological progress
The reason why agricultural mechanization can improve fertilization behavior through the path of technological progress is that some mechanical measures can help water-saving as well as fertilizer-saving. On one hand, mechanized application in agriculture is more efficient than manual fertilization. Although manual fertilization may have the advantage of greater mobility on small-scaled land, it is unavoidably spread uneven on the surface. In particular, in Southern China where it is rainy, farmers often need to overfertilize to avoid loss due to rain. Agricultural mechanization can realize deep application through transformation from manual fertilization to deep tillage, thus reducing losses and improving fertilizer efficiency. Mechanization can also save manpower to realize straw returning and root fertilizer application, and improve the fertilizer utilization rate and soil nutrient characteristics combined with the progress of agricultural techniques such as mechanical deep application, integration of water and fertilizers, and simultaneous sowing of fertilizers. On the other hand, one special nature of agricultural production is that in addition to fertilization, fine field management is required as well, which requires the use of tractors, rototillers, sprayers, and other agricultural machinery to assist in transportation, land leveling, and fertilization. Therefore, croplands close to roads are convenient for mechanized field consolidation, which is conducive to lower fertilizer use intensity.
It needs to be noted that there are mainly two ways to realize agricultural mechanization: self-purchased machinery and agricultural machinery services. Based on the current general discussion on the effects of agricultural mechanization on fertilizer use, the effects of different types of agricultural machinery inputs may not be consistent. In the context of the continuous transfer of the rural labor force and the aging and feminization of the agricultural labor force in China, agricultural service has increased (Yang et al., 2013; Wang et al., 2021). Many elderly farmers no longer work by themselves but purchase service to manage their farmland. The cost of purchasing agricultural machinery from agricultural cooperatives is normally lower than that of individuals, and the price of renting agricultural machinery is often lower than that of buying agricultural machinery by oneself. Farmers tend to purchase services instead of directly purchasing agricultural machinery when the cropland does not reach to a certain scale, so as to reduce production costs and improve operating efficiency. Therefore, from the perspective of the income effect, the purchase of agricultural machinery services is often cheaper than that of agricultural machinery and has less impact on the budget constraints of productive consumption. From the perspective of the planting structure, there is another special reason for the significant impact of agricultural machinery service on the planting structure compared with the self-purchase of agricultural machinery: agricultural machinery cooperatives tend to shrink the types of machinery and update specific effective machineries in order to ensure profit. Disguisedly, the types of crops that the farmers can choose are relatively fixed, and farmers need to adjust their planting structure according to the agricultural service provider’s menu. Considering the different fertilizer consumption of different crops, this will undoubtedly affect the fertilization application. Similarly, in terms of factor substitution and technical progress, compared with self-used machinery input, agricultural machinery services tend to be more specialized and scaled, the information cost of machinery cooperative docking with the dealer’s is lower, and agricultural machinery updates more conveniently. Agricultural machinery service personnel have generally received agricultural technology education and training, and specialized agricultural fertilization service organizations can effectively reduce farmers’ transaction risks and promote fertilizer reduction by their advantages in obtaining agricultural material quality information. Above all, the effects of different types of agricultural mechanization inputs may not be consistent.
In conclusion, the cropland scale may indirectly affect farmers’ fertilization behavior through agricultural mechanization in multiple paths. With the continuous expansion of cropland scale and the continuous improvement of the agricultural mechanization level, the fertilization mode can be changed positively and the utilization efficiency of fertilizers will be higher. Thus, farmers can put less fertilizer per area, and fertilizer intensity decreases with the expansion of the cropland scale and the improvement of the agricultural mechanization level. For this reason, this study will further verify with empirical tests.
3 Empirical strategy, data, and variables
3.1 Empirical strategy
3.1.1 Causal steps approach
This paper focuses on how the cropland scale affects fertilization through the mechanization level. Under Baron and Kenny’s framework (1986), the following regression equations can be used to describe the relationship between the three variables:
where coefficient
Furthermore, in order to solve endogeneity problems such as omitted variables, this study combined the two-way fixed-effect model with Baron and Kenny’s procedure. The specific steps are described as follows. First, we investigate the influence of the cropland scale on fertilizer input per mu, where its model is set as follows:
where
Second, we consider the mediator mechanism of agricultural mechanization:
among which
To fully take endogeneity into consideration, this study tries to control the influential variables mentioned in the theoretical mechanism into the empirical model as much as possible to avoid omitted variables. At the same time, the two-way fixed-effect model controlling time and individual also helps weaken endogeneity to some extent. Also, according to the statistical yearbook, the national application amount of fertilizer began to drop from 2015, which may be due to the fact that China implemented the “action plan for zero fertilizer growth by 2020." Therefore, we choose to use data after 2015 in the period of 2015–2017 on purpose in order to ensure the consistency of the political environment. In addition, the empirical analysis part will provide the results under OLS, random-effect model, and fixed-effect models for stability.
3.1.2 Effectiveness test
The Sobel test is generally used as a supplement to the causal step regression method for mediation effects (Baron and Kenny, 1986). The idea of the Sobel test is to compare the resulting z statistic with a critical value from the standard normal distribution, where the z-value of
where
As the assumption necessary for the Sobel test is that the sampling distribution of
3.2 Data source and variables
Our analysis used data mainly from the Fixed Observation Rural Survey, which was set up in 1984 with the approval of the Secretariat of the CPC Central Committee, conducted by the Ministry of Agriculture and Rural Affairs of the People’s Republic of China. Samples from 2015–2017 were selected in this study and sorted into the form of panel data. In addition to the main variables, the individual control variables and household control variables were included as well. We chose the family decision-maker as the representative of family members. After ruling out the outliers and eliminating the samples without farmland or fertilization, the final sample used is 53,260, involving 21,037 independent households. The concerned variables are as follows.
3.2.1 Cropland scale
The independent variable was cropland scale. To be specific, it is added up by the grain2 area and economic crop area at the end of the year. The former referred to the total farmland area actually managed by families at the end of the year, including private farmland, forage land, and sporadic reclamation land, corresponding to grain. The latter referred to the total economic crop area actually managed by the end of the year, which corresponds to economic crops such as mulberry, tea, and fruit trees. In our analysis, the cropland scale was regarded as the actual field applied fertilizer which was measured in mu.
3.2.2 Agricultural mechanization level
The mediator variable in our analysis was agricultural mechanization input. According to the relevant variables provided in the questionnaire, this study intended to use the sum of the original value of agricultural input and machinery service cost at the end of the year. The former represents the stock of the household while the latter represents the cost of renting others’ machinery for operation. The sum of the aforementioned was closest to the actual agricultural mechanization input. In accordance with most literature reports, this variable takes a logarithmic form with added 1 during regression.
3.2.3 Fertilizer application intensity
The dependent variable studied in this work was the intensity of fertilizer use, which represents the fertilizer input per mu and was measured in kilograms per mu. In order to obtain the specific amount of fertilizer per mu, we first calculate the average price of fertilizer by dividing the total fee of the purchased fertilizer by the total amount of the purchased fertilizer within each family. Then, we use the total fee of the used fertilizer to divide by the aforementioned average price to obtain the total used fertilizer. In the end, we can divide the total used fertilizer to the cultivated area in order to obtain the fertilizer application intensity.
3.2.4 Other control variables
The selection of other control variables followed the criterion of exogenous as much as possible, including individual and family levels as they may affect the input degree of agricultural mechanization and fertilization behavior of households to a certain extent (Zhang et al., 2019; Ren et al., 2021; Yang et al., 2022). First of all, at the individual level, we choose the age, education level, agricultural training, and health status of household decision-makers in order to control an individual’s stock of experience and human capital quality (Yang et al., 2022). Specifically, age represented the householder’s age. The education level was measured by the educated years. Agricultural training was obtained by combining the questions “have you received agricultural technical education” and “have you received agricultural training;” if the individual participated in any of them, it will be assigned as 1, otherwise, it is 0. Health status was a dummy variable (1 for healthy and 0 for unhealthy). On the family level, the proportion of the labor force was obtained by dividing the number of labor force by the permanent residents to control the family’s labor structure (Gao et al., 2021). The planting structure was obtained by dividing the grain area by the total cropland to make sure all analyses were under controlling crop types. The family income was lagged by one period to control the economic level, where the total income has been adjusted by the rural consumer price index published by the National Bureau of Statistics of China based on the year 2014 and took the logarithmic form in regression. We also used the number of pieces of cropland in order to control land fragmentation (Ren et al., 2021). Finally, time and individual dummy variables are used to control the influence of each year and individual effects which contain the influence of regional factors such as topographical and natural characteristics and other human factors like personal preferences that cannot be measured in the questionnaire.
3.3 Descriptive analysis
Table 1 presents the descriptive analysis of the sample data. On the total level, the average scale of the agricultural land was 11.82 mu, reflecting a basic situation of small-scale management. In terms of dependent variables, the average input of fertilizers is about 108.96 kg/mu, among which the fertilizer used for grains is lower than that for economic crops. As for mediator variables, the mean of the agricultural input is around 4,048.32 yuan. When it comes to the controlled variables, the average age is between 54 and 55 years old; the average health status is 83.80%; the proportion of labor force accounts for 66.75% for each family; the average income is around 64,630.6 yuan; and the average planting structure is 80.13%, which means that grains are dominant crops. The aforementioned descriptive statistical results basically meet the expectations where each variable’s mean and variance in different years are very close, reflecting the reliability of the data.
4 Empirical analysis
Table 2 shows the first step of the step regression test, that is, the estimated results of the impact of the agricultural land scale on the average fertilizer input per mu. In order to ensure the stability of the results, this study uses OLS, random-effect model, fixed-effect model, and two-way fixed-effect model. After fixing the time and individual effects, when agricultural land scale increases by a unit, the average fertilizer input per mu will decrease by about 0.0388 kg, which is significant at the statistical level of 10%. In terms of other control variables, the proportion of labor force has a significant negative impact on the input of fertilizer, reflecting that there is a certain substitution relationship between fertilizer and labor factors. With the decline of the proportion of labor force, farmers need to increase fertilizer input to ensure production. The previous year’s income level does not have a significant effect on fertilizer input, possibly indicating that fertilization behavior is an empirical fixed habit. The planting structure significantly affects the input of fertilizer, which is due to the characteristics of labor-intensive and high fertilizer consumption of economical crops compared with grain crops in agricultural production activities.
Moreover, this study selected the contracted land area of farmers as the instrument variable of the cropland scale (Wu et al., 2018). Theoretically, the contracted land is usually distributed evenly within the collective in the early stage according to the cultivated land resource endowment and population of the village collective (Adamopoulos and Restuccia, 2014), which means that the contracted land area is basically irrelevant to the disturbance term of the model, thus meeting the exogenous requirements. Second, although farmers can transfer or adjust the cropland scale, under the restrictions of many factors, farmers with large initial contracted land may still have a larger cropland scale (correlation coefficient >0.77). Compared with the aforementioned results, the coefficient of the cropland scale is actually larger using the instrumental variable method, which means that the actual reduction effect from the cropland scale is underestimated to some extent.
To sum up, the regression results under different models show that the scale of agricultural land has a significant negative impact on the average fertilizer input per mu, reflecting the robustness of the results.
Table 3 shows the second step in the step regression test, that is, the estimated results of the impact of land size on the mediator variable mechanization input. The results of OLS, random-effect, fixed-effect, and two-way fixed-effect models are reported to ensure the robustness as well. After fixing the time and individual effects, as well as other control variables, the cropland scale has a positive effect on farmers’ mechanization input, which is significant at the statistical level of 1%. The reason for the relatively small economic coefficient may be that, as pointed out in the descriptive analysis, the agricultural land scale is about 11.82 mu on average, which restricts its scale effect to some extent. In terms of other variables, agricultural mechanization input is positively affected by the previous income level, as the increase in income can relax the budget constraints of farmers. The input of agricultural machinery was positively affected by the planting structure, and it was significant at the statistical level of 1%. Based on the definition of the planting structure in this paper, it can be seen that the production of economical crops is comparatively difficult to be mechanized in China due to a higher demand for labor force. Therefore, food crops are more conducive to agricultural mechanization than economical crops. All in all, the regression results under different models show that the mediator is significantly positively affected by the independent variable and is relatively robust.
Table 4 shows the third step in the step regression test, that is, the estimation result of the impact of cropland scale on per mu fertilizer input after controlling the intermediate variable of agricultural mechanization input. The results showed that first, after controlling the mediator variables, cropland scale still has a negative impact on fertilizer input, and it was significant at the statistical level of 1%. Second, the absolute values of the coefficients of farmland scale in columns (10)–(13) are lower than those in columns (1)–(4) in Table 2. Third, the results of OLS and random effect show that the input of agricultural machinery negatively affected the input of fertilizers, and it was significant at the statistical level of 1%. The simultaneous satisfaction of the aforementioned conditions indicates that part of the mediation effect exists significantly. We notice that the negative impact under the fixed effect is not significant. According to Baron and Kenny (1986), if at least one of A and B is found not to be significant in the step regression test, the Sobel test can be supplemented. In order to ensure the validity of the results, validity tests will be supplemented in the next section.
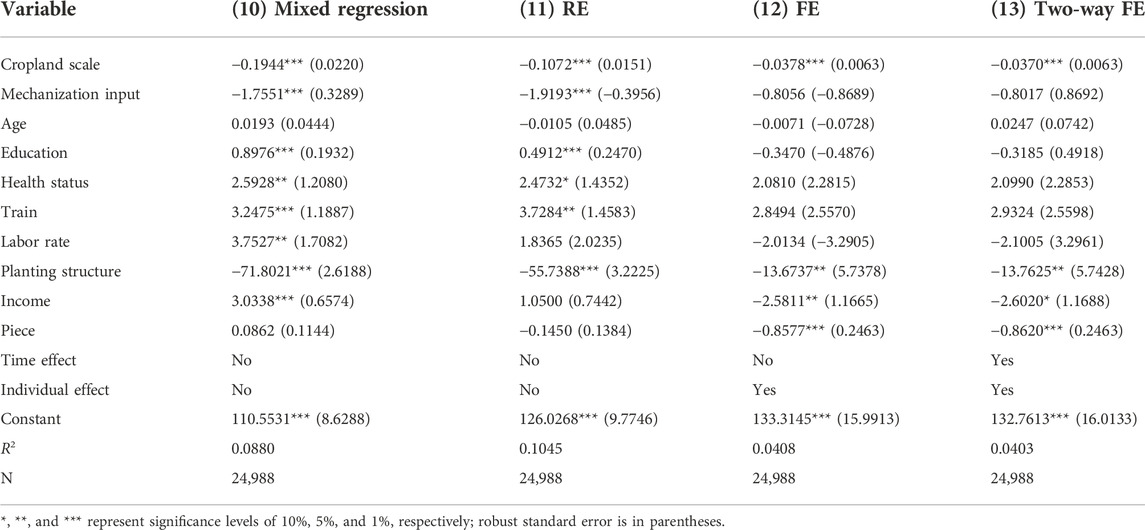
TABLE 4. Impact of farmland scale on fertilization behavior: the mediating effect of agricultural mechanization input.
5 Validation and further discussion
5.1 Effectiveness test
Table 5 shows the results of the Sobel test. The results show that the direct negative effect of agricultural land scale on fertilizer input is −0.1947, with an indirect effect of −0.0249 and the total effect of −0.2196. The calculated mediating effect accounts for 11.34% of the total effect. The Sobel test’s p value is less than 0.05, indicating that the null hypothesis should be rejected and the mediating effect exists significantly. However, the disadvantage of the Sobel test is that it assumes
In order to ensure the robustness of the results, we conducted the bootstrap test as shown in Table 6, which was repeated 200, 500, and 1,000 times, respectively. The estimated results of different repeated sampling times show that the mediating effect is −0.0249. Whether it is a nonparametric percentile method or bias-corrected percentile bootstrap method, the confidence interval does not include 0, indicating that the intermediary effect exists significantly and the result is robust.
Another way of the stability test is altering different samples. Our first way is to obtain the sub-sample corresponding to the householder’s age between 16 and 64 years old, accounting for around 75% of the total sample. The reason why we chose the aforementioned span of the total sample is because the working labor is required to be older than 15 and younger than 65 according to the National Bureau of Statistics of China. Table 7 shows the two-step regression result for this part of the samples, which is close to the step regression method only without the first step. In Table 7, OLS, random-effect, and fixed-effect models are used, respectively; (14)–(16) list the impact of the agricultural land scale on agricultural mechanization, and (17)–(19) report the impact of the land scale on fertilization behavior controlling agricultural mechanization. The results show that the expansion of the cropland scale significantly increased the investment in agricultural mechanization. Moreover, the cropland scale and mechanization have a significantly negative impact on fertilizer intensity, which shows the existence of the mediation effect. Furthermore, as shown in Table 8, the results of the Sobel test and bootstrap test show that the mediating effect exists significantly, and the coefficient is −0.0249, accounting for 11.78% of the total effect, which has passed the effectiveness test.
Moreover, we tried other sub-samples by truncating the independent variable at the 5th percentile and 10th percentile. Specifically, the first sub-sample group is generated by cutting off 5% both from the top and the end of the independent variable while the second sub-sample group is generated by cutting 10% from both sides. The aforementioned selection can not only help us further reduce the potential impact of extreme values but also obtain sub-samples. In Table 9, OLS, random-effect, and fixed-effect models are used, respectively. The results show that the expansion of the cropland scale significantly decreased the fertilizer intensity in both sub-samples. Furthermore, as shown in Table 10, the results of the Sobel test and bootstrap test show that the mediating effect exists significantly, and the coefficient of the indirect effect is −0.0274 and 0.0283 within each sub-sample, accounting for 11.18% and 13.12% of the total effect, respectively.
5.2 Heterogeneous analysis
As the mediating effect may vary within different cropping systems, we attempted to further analyze the heterogeneity of grain growers and economic crop growers. We divided samples into two groups, the grain-oriented group and economic crop-oriented group; farmers whose planting structure3 is above 50% are considered grain-oriented growers, while the opposite are considered economic crop-oriented. Table 11 shows the results of OLS, random-effect, and two-way fixed-effect models. The results of the causal step regression show that, after controlling the cropland, the significant negative impact of agricultural mechanization on fertilizer intensity mainly exists in grain-oriented samples. But for the economic crop-oriented group, after controlling the cropland scale, the impact of agricultural mechanization on fertilizer intensity is not significant. Combined with the results shown in Table 12, the economic crop-oriented group failed the Sobel test and bootstrap test as well, while the grain-oriented group witnessed a significant mediating effect from the mechanization level. The reasons for this result may be diverse: on one hand, grains are relatively easier to operate mechanically due to the difference in production methods, while economic crops are labor-intensive and, therefore, agricultural machinery operations are difficult to perform; on the other hand, from the perspective of equipment manufacturing, the development of economical crops’ mechanization is lower than that of grains; in addition, the fertilizer consumption of economical crops is generally higher than that of grains in terms of biological characteristics.
5.3 Further analysis
As there are many differences in the grain structure, terrain, and climate between the southern part and northern part of China, based on the aforementioned discussion, this paper further distinguishes grain-oriented farmers into two groups according to geographic characteristics. Specifically, we regard samples from provinces south of the Qinling Moutain–Huaihe River line as the southern group, and vice versa as the northern group. Table 13 shows the results of the step regression test as well as the Sobel and bootstrap test repeated 500 times for the two different groups. The results show that there are significant partial mediation effects in agricultural mechanization in both southern and northern samples. Among them, the mediation effect coefficient of the southern sample is −0.1413, accounting for 28.07% of the total effect, while that of the northern part is −0.0083, accounting for only 6.10% of the total effect. On the whole, the mediation effect of grain-oriented farmers in the south is stronger than that in the north.
The possible reasons for this result are as follows: first, there are differences in the selection of agricultural machinery investment between the south and the north. Restricted by the endowment of agricultural land scale in the north and south, compared with the self-purchase of agricultural machinery, southern farmers prefer to choose agricultural machinery services, while northern farmers prefer self-purchased machinery. According to the descriptive analysis of the north and south samples given in Table 14, the average proportion of “agricultural machinery service/self-purchased agricultural machinery” in the southern sample is greater than 1, while that in the northern sample is less than 1. This means that the agricultural machinery service investment of farmers in the south is greater than that of self-purchased agricultural machinery, and vice versa in the north. As mentioned previously, agricultural machinery service providers generally have received agricultural technology education and training and can effectively reduce the transaction risk of farmers and promote the reduced application of fertilizers by virtue of their advantages in obtaining agricultural material quality information. Second, from the perspective of the grain planting structure, based on the dual factors of history and climate, southern China has become rice-oriented while northern China has become wheat-oriented overall. From the descriptive analysis given in Table 14, the southern samples mainly plant rice and corn, while northern samples mainly plant corn and wheat. The samples show an obvious pattern of “rice in the south and wheat in the north.” In addition to the difference in fertilizer consumption between the two types of food crops, the labor input of rice is about twice that of wheat in the same area (Buck, 1931), which means that the demand for agricultural machinery input is more urgent. Third, the south is rainier than the north, and the fertilizer on the land surface is easily washed by rain, so farmers have to add fertilizer input, and the input of agricultural machinery can change the fertilization method from manual surface application to deep tillage and deep application, which is conducive to increase the efficiency and reduce the fertilizer. To sum up, the southern samples benefit more from the mediation variable of agricultural mechanization overall.

TABLE 14. Agricultural mechanization and planting structure of grain farmers in northern China and southern China.
6 Conclusion and policy implications
At present, balancing environmental protection and agricultural production has become an unavoidable issue in the process of China’s agricultural modernization. This paper focuses on how the agricultural land scale affects farmers’ fertilization behavior through agricultural mechanization input. Based on the Fixed Observation Points Data of the Ministry of Agriculture and Rural Affairs in China between 2015 and 2017, this study uses different methods to investigate how the agricultural land scale affects fertilization behavior through agricultural mechanization input. The main conclusion of this paper is that agricultural land scale significantly improves the level of agricultural mechanization and significantly reduces the average fertilizer input per mu through the mediating effect. The results of the follow-up analysis show that this effect mainly exists in grain crop farmers, and the effect is stronger in the south sample. Compared with previous studies, on the basis of verifying the reduction effect of agricultural land scale, this paper defines the role of agricultural mechanization and discusses in detail by distinguishing different crops and north–south differences, which provides a new perspective for reducing fertilizers and reducing agricultural non-point source pollution. Based on the aforementioned analysis, this paper puts forward the following suggestions.
First of all, small farmers should adhere to the guidance of appropriate scale management. The small scale of agricultural land will increase the difficulty of agricultural mechanization and the substitution of machinery for labor. As far as the findings of this study are concerned, the average agricultural land scale is about 11 mu, meaning most farmers still belong to small-scale operation. The current situation of agricultural land scale will hinder mechanization and become the restriction of fertilizer reduction. Therefore, small farmers should be actively guided to carry out land transferring and integration, and help the standardized construction of high-quality farmland, so as to break through the constraints of land scale matching and mechanical utilization efficiency faced by fertilizer reduction.
Second, the development of agricultural mechanization ought to be emphasized as well. On one hand, adhere to the orientation of vigorously developing the socialization and service of agricultural machinery. For example, the government should facilitate the cross-regional operation of China’s agricultural machinery and improve the availability of agricultural machinery services for farmers. On the other hand, according to the conclusion of this paper, the mediating effect of agricultural mechanization of grain crops is more significant. Therefore, it is necessary to further strengthen the independent innovation ability of agricultural machinery and equipment manufacturing of economical crops, and realize the reduction and efficiency of economical crop fertilization by integrating agricultural machinery and agronomic measures. Finally, by improving the subsidy incentive system, such as agricultural machinery subsidies and strengthening agricultural technology education and training, we can form a joint force to improve farmers’ production technology and organization mode.
Finally, it should be pointed out that although this study has verified that agricultural mechanization plays a certain indirect role in fertilization reduction on the agricultural land scale, there are also some deficiencies and space to be expanded: for example, due to data limitations, this study fails to conduct further analysis on the types of agricultural reduction. What is the impact of agricultural mechanization on different types of fertilizers such as nitrogen, phosphorus, and potassium, as well as pesticides, agricultural films, and carbon emission? Clarifying the aforementioned questions will help us further evaluate the scaled effect of agricultural land, understand the role of agricultural mechanization investment in improving production efficiency and controlling agricultural non-point source pollution, and provide important theoretical support for realizing the multiple policy objectives of ecological protection and ensuring agricultural production, especially food security. Therefore, future research may focus on the aforementioned issues, which are also the main research directions of this paper in the coming future.
Data availability statement
The raw data supporting the conclusion of this article will be made available by the authors, without undue reservation.
Author contributions
JL and QX contributed to the conception and design of the study and funding acquisition. TZ contributed to the data analysis and the main manuscript text. JL and QX supervised and revised the manuscript. All authors read and agreed to the published version of the manuscript.
Funding
This study was funded by grants from the National Social Science Foundation of China (20AZD046; 21ZDA060; and 22ZDA030), the Program of China Institute for Rural Studies of Tsinghua University (CIRS2022-3), and the Program for Innovative Research Team of Shanghai University of Finance and Economics (2018110693).
Conflict of interest
The authors declare that the research was conducted in the absence of any commercial or financial relationships that could be construed as a potential conflict of interest.
Publisher’s note
All claims expressed in this article are solely those of the authors and do not necessarily represent those of their affiliated organizations, or those of the publisher, the editors, and the reviewers. Any product that may be evaluated in this article, or claim that may be made by its manufacturer, is not guaranteed or endorsed by the publisher.
Footnotes
1Ministry of Agriculture and Rural Affairs in China, Action Plan for Zero Growth of Fertilizer Application by 2020, http://www.moa.gov.cn/ztzl/mywrfz/gzgh/201509/t20150914_4827907.htm.
2According to the questionnaire, the crops can be divided into grain and economic crops. The former refers to wheat, rice, corn, bean, and potato, and the latter refers to cotton, oil crops, sugar crops, hemp, tobacco, sericulture, vegetables, and fruits.
3As mentioned previously, the planting structure is obtained by dividing the grain area by the total cropland.
References
Adamopoulos, T., and Restuccia, D. (2014). The size distribution of farms and international productivity differences. Am. Econ. Rev. 104 (6), 1667–1697. doi:10.1257/aer.104.6.1667
Bambio, Y., and Bouayad, A. S. (2018). Land tenure security and investment: Does strength of land right really matter in rural Burkina Faso? World Dev. 111 (6), 130–147. doi:10.1016/j.worlddev.2018.06.026
Baron, R. M., and Kenny, D. A. (1986). The moderator–mediator variable distinction in social psychological research: Conceptual, strategic, and statistical considerations. J. Personality Soc. Psychol. 51 (6), 1173–1182. doi:10.1037/0022-3514.51.6.1173
Belton, B., Win, M. T., Zhang, X., and Filipski, M. (2021). The rapid rise of agricultural mechanization in Myanmar. Food Policy 101 (9), 102095. doi:10.1016/j.foodpol.2021.102095
Chen, X. X., Liu, Y. M., Zhao, Q. Y., Cao, W. Q., and Zou, C. Q. (2020). Health risk assessment associated with heavy metal accumulation in wheat after long-term phosphorus fertilizer application. Environ. Pollut. 262, 114348. doi:10.1016/j.envpol.2020.114348
Gao, J., Gai, Q., Liu, B., and Shi, Q. (2021). Farm size and pesticide use: Evidence from agricultural production in China. China Agric. Econ. Rev. 13 (4), 912–929. doi:10.1108/caer-11-2020-0279
Gao, L., Zhang, W., Mei, Y., Sam, A. G., Song, Y., and Jin, S. (2018). Do farmers adopt fewer conservation practices on rented land? Evidence from straw retention in China. Land Use Policy 79, 609–621. doi:10.1016/j.landusepol.2018.08.026
Guo, J. H., Liu, X. J., Zhang, Y., Shen, J. L., Han, W. X., Zhang, W. F., et al. (2010). Significant acidification in major Chinese croplands. Science 327 (5968), 1008–1010. doi:10.1126/science.1182570
Hu, Y., Li, B., Zhang, Z., and Wang, J. (2019). Farm size and agricultural technology progress: Evidence from China. J. Rural Stud. 93 (7), 417–429. doi:10.1016/j.jrurstud.2019.01.009
Ju, X., Gu, B., Wu, Y., and Galloway, J. N. (2016). Reducing China’s fertilizer use by increasing farm size. Glob. Environ. Change 41, 26–32. doi:10.1016/j.gloenvcha.2016.08.005
Leiva, F. R., and Morris, J. (2001). PH—postharvest technology: Mechanization and sustainability in arable farming in england. J. Agric. Eng. Res. 79, 81–90. doi:10.1006/jaer.2000.0686
Liu, Y., Hu, W., Jetté-Nantel, S., and Tian, Z. (2014). The influence of labor price change on agricultural machinery usage in Chinese agriculture. Can. J. Agric. Economics/Revue Can. d'agroeconomie. 62 (2), 219–243. doi:10.1111/cjag.12024
Lu, H., and Xie, H. (2018). Impact of changes in labor resources and transfers of land use rights on agricultural non-point source pollution in Jiangsu Province, China. J. Environ. Manag. 207, 134–140. doi:10.1016/j.jenvman.2017.11.033
Ma, W., and Abdulai, A. (2019). IPM adoption, cooperative membership and farm economic performance: Insight from apple farmers in China. China Agric. Econ. Rev. 11 (2), 218–236. doi:10.1108/caer-12-2017-0251
Mackinnon, D. P., Lockwood, C. M., and Williams, J. (2004). Confidence limits for the indirect effect: Distribution of the product and resampling methods. Multivar. Behav. Res. 39 (1), 99–128. doi:10.1207/s15327906mbr3901_4
Norse, D., and Ju, X. (2015). Environmental costs of China’s food security. Agric. Ecosyst. Environ. 209, 5–14. doi:10.1016/j.agee.2015.02.014
Otsuka, K. (2013). Food insecurity, income inequality, and the changing comparative advantage in world agriculture. Agric. Econ. 44, 7–18. doi:10.1111/agec.12046
Preacher, K. J., and Hayes, A. F. (2004). SPSS and SAS procedures for estimating indirect effects in simple mediation models. Behav. Res. Methods Instrum. Comput. 36 (4), 717–731. doi:10.3758/bf03206553
Qin, S. L., and Lu, X. Y. (2020). Do large-scale farmers use more pesticides? Empirical evidence from rice farmers in five Chinese provinces. J. Integr. Agric. 19 (2), 590–599. doi:10.1016/s2095-3119(19)62864-9
Ren, C., Jin, S., Wu, Y., Zhang, B., Kanter, D., Wu, B., et al. (2021). Fertilizer overuse in Chinese smallholders due to lack of fixed inputs. J. Environ. Manag. 293, 112913. doi:10.1016/j.jenvman.2021.112913
Wang, S., Bai, X., Zhang, X., Reis, S., Chen, D., Xu, J., et al. (2021). Urbanization can benefit agricultural production with large-scale farming in China. Nat. Food 2 (3), 183–191. doi:10.1038/s43016-021-00228-6
Wang, X., Yamauchi, F., and Huang, J. (2016). Rising wages, mechanization, and the substitution between capital and labor: Evidence from small scale farm system in China. Agric. Econ. 47 (3), 309–317. doi:10.1111/agec.12231
Wu, Y., Xi, X., Tang, X., Luo, D., Gu, B., Lam, S. K., et al. (2018). Policy distortions, farm size, and the overuse of agricultural chemicals in China. Proc. Natl. Acad. Sci. U. S. A. 115 (27), 7010–7015. doi:10.1073/pnas.1806645115
Yang, J., Huang, Z., Zhang, X., and Reardon, T. (2013). The rapid rise of cross-regional agricultural mechanization services in China. Am. J. Agric. Econ. 95 (5), 1245–1251. doi:10.1093/ajae/aat027
Yang, Y., Li, Z., and Jin, M. (2022). How do chemical fertilizer reduction policies work?—empirical evidence from rural China. Front. Environ. Sci. 10, 1346. doi:10.3389/fenvs.2022.955278
Zhang, X., Yang, J., and Thomas, R. (2017). Mechanization outsourcing clusters and division of labor in Chinese agriculture. China Econ. Rev. 43, 184–195. doi:10.1016/j.chieco.2017.01.012
Zhang, J., Wang, J., and Zhou, X. (2019). Farm machine use and pesticide expenditure in maize production: Health and environment implications. Int. J. Environ. Res. and Public Health 16 (10), 1808. doi:10.3390/ijerph16101808
Zhang, Y., Long, H., Li, Y., Ge, D., and Tu, S. (2020). How does off-farm work affect chemical fertilizer application? Evidence from China’s mountainous and plain areas. Land Use Policy 99, 104848. doi:10.1016/j.landusepol.2020.104848
Zheng, H., Ma, W., Guo, Y., and Zhou, X. (2022). Interactive relationship between non-farm employment and mechanization service expenditure in rural China. China Agric. Econ. Rev. 14 (1), 84–105. doi:10.1108/caer-10-2020-0251
Keywords: agricultural mechanization, cropland scale, fertilizer use, mediation effect, rural China
Citation: Liu J, Xu Q and Zhou T (2022) Role of mechanization: The impact of the cropland use scale on fertilizer reduction. Front. Environ. Sci. 10:1053715. doi: 10.3389/fenvs.2022.1053715
Received: 26 September 2022; Accepted: 14 November 2022;
Published: 25 November 2022.
Edited by:
Zhen Wang, Huazhong Agricultural University, ChinaCopyright © 2022 Liu, Xu and Zhou. This is an open-access article distributed under the terms of the Creative Commons Attribution License (CC BY). The use, distribution or reproduction in other forums is permitted, provided the original author(s) and the copyright owner(s) are credited and that the original publication in this journal is cited, in accordance with accepted academic practice. No use, distribution or reproduction is permitted which does not comply with these terms.
*Correspondence: Jin Liu, bGl1LmppbkBtYWlsLnNodWZlLmVkdS5jbg==