- School of Economics and Management, Shanghai Ocean University, Shanghai, China
As the guarantee for the effective implementation of environmental policies, the spillover of the deterrent effect of environmental administrative penalties is crucial for solving the environmental regulatory dilemma. It is also unclear whether environmental administrative penalties can affect green technology innovation of the peer enterprises and whether they have an impact on both the quantity and quality dimensions. Taking listed firms of heavily polluting industries in China’s Shanghai and Shenzhen A-share from 2016 to 2020 as the sample, this paper analyzes the impact of the environmental administrative penalty on the quantity and quality of corporate green technology innovation based on the perspective of peer effect. The results indicate that: 1) Environmental administrative penalty significantly promotes the quantity and quality of peer enterprises’ green technology innovation. 2) Heterogeneity analysis shows that the diversity of penalties, the competitiveness of the penalized enterprises, and the property rights of peer enterprises all contribute to the different impacts of the environmental administrative penalty on the peer enterprises’ green technology innovation. 3) Further analysis shows that the promotion effect will be weakened when peer enterprises face high financing constraints. The research results expand the related research on direct government regulation and green technology innovation from the perspective of peer effect and provide policy reference for the government to formulate differentiated penalty policies according to the heterogeneity of enterprise.
1 Introduction
Since the 20th century, environmental pollution has become a global issue. Economic growth and urbanization have brought great challenges to resources and the environment (Ali et al., 2022). As the world’s second-largest economy, China is actively exploring ways to balance economic and environmental development. The 20th National Congress of the Communist Party of China proposed to further promote the prevention and control of environmental pollution and realize the green transformation of development mode. It demonstrates China’s determination and efforts in achieving a balance of economic and environmental development once again. At the congress, Chinese leaders repeatedly emphasized, “Innovation is the first driving force that leads development.” Green technology innovation (GTI) takes into account the dual benefits of economic development and environmental protection (Hua and Li, 2022). In the context of enterprises being recognized as one of the main sources of environmental pollution (Shevchenko, 2020), promoting enterprises to carry out GTI is the fundamental way and important driving force for the development of a green economy (Hong et al., 2021). China has been increasing its innovation efforts in recent years. In 2019, China surpassed the United States to become the largest source of international patent applications filed through WIPO, and the number of international patent applications in China once again reached first place in the world in 2020 (PIRS 2021). However, the quality of innovation in China is not high, and the development of most core technologies still lags far behind that of developed countries (Cai and Yu, 2017). The “Stuck Neck” problem of core technology seriously restricts the safe development of China’s economy. Therefore, how encouraging enterprises to improve GTI in quantity and quality is of great significance for China’s transformation into an innovation power.
As a public good, the environment has the characteristics of non-competitive consumption and non-exclusive income, so “free riding” behavior often occurs (Zhang et al., 2022). At the same time, compared with traditional innovation, the spillover effect of GTI will also make the enterprises’ innovation risk not match the innovation return, or even the innovation income is lower than the innovation input (Wang et al., 2020). Therefore, it is difficult for enterprises to independently carry out GTI relying only on the resource allocation role of the market (McGartland et al., 2017). Market failures necessitate government intervention. Governments and relevant organizations have issued a series of policies or treaties related to environmental protection, such as the International Environmental Protection Convention, the Paris Agreement, the Kyoto Protocol, etc. The government requires enterprises to comply with environmental protection laws and regulations by using administrative orders and punishing enterprises that violate the corresponding standards. The deterrent effect of punishment is the guarantee for the effective implementation of those environmental policies (Wang et al., 2018). However, due to information asymmetry and limited government administrative resources, only a part of environmental violations can be detected (Ayres and Braithwaite, 1995), which poses a huge challenge to regulation. Studies have found that the peer enterprises and the penalized enterprises face similar living environments and have similar business structures, so the spillover of the deterrent effect of penalties may also affect the peer enterprises with potential violation motives (Wang et al., 2019). Therefore, deterring enterprises with potential violations is the key to solving the environmental regulatory dilemma and realizing incentives for GTI. Based on this, this article attempts to answer the following questions: Can environmental administrative penalties of penalized enterprises deter peer enterprises? Will it improve the GTI capabilities of peer enterprises? What is the mechanism?
In previous literature, the study of environmental administrative penalties mainly focused on the impact on penalized enterprises. Environmental administrative penalties deter penalized enterprises (Hall, 2022). The penalties would significantly increase corporate audit costs (Xin et al., 2022), reduce corporate cash flows (Ding et al., 2022), increase debt costs (Ding et al., 2021), and promote voluntary disclosure of environmental information (Ding et al., 2019). In addition, self-disclosure of penalty information prevents the decline in the company’s stock market return (Ding et al., 2020). In terms of enterprise environmental management, environmental administrative penalties would inhibit enterprises’ greenwashing behavior (Sun and Zhang, 2019), reduce the number of days of violations (Nadeau, 1997), and improve environmental performance (Earnhart, 2004). With further research, scholars start to study the peer effect of environmental administrative penalties. It is found that environmental administrative penalties will promote environmental protection investment in the peer enterprises (Wang et al., 2020), and can also promote environmental governance in the process and outcome dimensions of other enterprises in the same industry (Chen et al., 2021). In addition, academia has been paying attention to environmental regulation and corporate GTI for a long time. Previous literature mainly focuses on macro-level environmental regulation and tests the Porter Hypothesis (George et al., 2017; Ramanathan et al., 2017). With further research, scholars realize that the innovation incentive effects of different environmental regulations may be different, so they classify environmental regulations and discuss the different innovation incentive effects of different environmental regulations (Ye et al., 2018; Hua et al., 2022). Further, there is literature that classifies GTI (Tao et al., 2021).
According to the review of previous literature, it is found that there are few studies on the innovation incentive effect of environmental administrative penalties, and the mechanism of the impact of environmental administrative penalties on peer enterprises’ GTI is not clear. Moreover, the research on the influencing factors of technological innovation mainly focuses on the quantitative dimension, and there is only a few GTI research involving the quality dimension. After identifying this gap, this paper defines other enterprises in the same industry as the penalized enterprises as peer enterprises and divides the GTI capabilities of enterprises into two dimensions: quantity and quality. The number of green patent applications is used to indicate the quantity of GTI, and the knowledge breadth of green patents is used to indicate the quality of GTI. This study selects the data of Chinese listed companies in the heavy pollution industry in Shanghai and Shenzhen A-shares from 2016–2020 as the research sample. First, a fixed effects model is selected by the Hausman test to empirically analyze the effects of environmental administrative penalties on the quantity and quality of peer enterprises’ GTI, respectively. The findings show that environmental administrative penalty significantly promotes the quantity and quality of peer enterprises’ GTI. Its robustness is confirmed by replacing the explanatory variables, Heckman’s two-stage model, negative binomial model, and panel Tobit model, and it also solves possible endogeneity problems by employing the dynamic system GMM model. After that, the internal and external factors of environmental administrative penalties affecting the GTI capabilities of peer enterprises are analyzed in three aspects: different perspectives of environmental administrative penalties, competitiveness heterogeneity of penalized enterprises, and property rights heterogeneity of peer enterprises. Additionally, we explore the moderating effect of financing constraints on the relationship between the environmental administrative penalties and peer enterprises’ GTI capabilities. And it is found that financing constraints act as a moderator of disincentives. After summarizing the above findings, this study provides policy suggestions.
The contributions are mainly reflected in the following aspects. First, considering that the effectiveness of environmental administrative penalties on potential non-compliant enterprises has not been explored to a large extent, and the relevant empirical evidence is lacking, this paper studies the incentive effect of environmental administrative penalties on GTI from the perspective of peer effect. It enriches the academic community’s understanding of the peer effect of environmental administrative penalties. Second, considering that the number of patent applications cannot fully represent the GTI capabilities of enterprises, this paper divides the GTI capabilities into two aspects: the quantity and quality of green patent applications, and analyses whether the GTI capabilities of peer enterprises can produce substantial improvement by environmental administrative penalties. It makes the research field of GTI more detailed and provides Chinese suggestions for the strategic deployment of high-quality GTI in the world. Third, considering that most of the previous literature only studies whether enterprises are subject to environmental administrative penalties, and most of the heterogeneity analysis only considers the penalized enterprises, this paper analyzes the heterogeneity from three perspectives: the diversity of penalty, the competitiveness of the penalized enterprises, and the property rights of peer enterprises. In addition, combined with the actual scenario of enterprises carrying out GTI, this paper further tests the moderating effect of financing constraints. Compared with previous literature, this paper has a more detailed research perspective, which provides a theoretical basis for the implementation of environmental administrative penalty policies. In addition, the effective implementation of China’s environmental administrative penalties has important reference value for other countries to formulate environmental policies and improve the GTI capability, especially in developing countries.
The remainder of this paper is organized as follows: Section 2 will carry out a theoretical analysis and put forward hypotheses. The data sources, the measurement of the variables, and the econometric models are introduced in Section 3. The descriptive statistics, the regression results, and the robustness tests are presented in Section 4. The heterogeneity analysis and further analysis are presented in Section 5 and Section 6, respectively. The final section provides the conclusions and suggests some policy implications.
2 Theoretical basis and hypothesis development
The peer effect originated in sociology, which refers to the phenomenon that the behavior of the individual is affected by group behavior to a certain extent and changes with the change of group behavior (Manski, 1993). Early research on peer effects focused on sociology, such as educational production (Zheng, 2015), family financial decisions (Brown et al., 2015), the development of academic achievement in early adolescence (Hou et al., 2018), and criminal behavior (Walters, 2018). With further research, the peer effect has become a hot topic in finance, economics, and management. The research scope of the peer effect extends to enterprises. The behavior of enterprises is not only affected by their economic interests but also by other enterprises with similar status and characteristics, resulting in changes in their decision-making and behavioral results (Zhu et al., 2021). The existing study of the corporate peer effect found that there are significant peer effects in corporate finance and governance decisions such as information disclosure decisions (Seo, 2021), corporate governance (Fairhurst and Nam, 2018), financial decisions (Liu et al., 2022), employee welfare policies (Rind et al., 2021), investment decisions (Wang et al., 2022), and violations (Lu and Chang, 2018). In terms of the peer effect of environmental administrative penalties, Wang Yun et al. (2020) introduce the Deterrent Theory of punishment, empirically analyze the impact of environmental administrative penalties on the environmental protection investment of the peer enterprises, and find that environmental administrative penalties will produce a deterrent effect through the peer influence path. Chen et al. (2021) divide environmental administrative penalties into two aspects: penalty frequency and penalty intensity, and empirically analyze their impact on environmental governance in the process and outcome dimensions of other enterprises in the same industry. It is confirmed that environmental administrative penalties also have a deterrent effect on peer enterprises in the same industry.
In the context that all enterprises in the community are subject to environmental supervision, the peer enterprises will consciously pay attention to and follow the behavior of penalized enterprises to avoid the costs and risks of independent decision-making (Manski, 2000). Lieberman and Asaba (2006) pointed out that the reason for the peer effect of corporate decision-making behavior is to obtain decision-related information and maintain its competitive advantage. Zhu et al. (2021) also believe that compared with traditional decision-making behaviors, technological innovation has the characteristics of high risk, high investment, uncertain return period, and large potential benefits, so its dependence on information and market competition demand is greater. In addition, Li and Zhong (2019), and Zeng et al. (2020) also analyze the internal mechanism of the peer effect of enterprise decision-making from two types of motivations: information acquisition and competitive demand. The impact of environmental administrative penalties on peer enterprises can also be analyzed from these two aspects. On the one hand, environmental administrative penalties for companies that violate environmental regulations send out a deterrent signal through the communities, which may inform peer enterprises about the consequences and costs of engaging in similar environmental behavior (Wang et al., 2019). On the other hand, in order to maintain their reputation and respond to competitive pressures, companies will imitate the behavior of other individuals (Lu et al., 2017). To this end, this paper introduces Deterrence Theory and Competition Theory to analyze the impact of environmental administrative punishment on the GTI capability of peer enterprises.
According to the Deterrence Theory, penalties deter potential offenders by punishing the defendant so that they realize that the costs of crime outweigh the benefits and thus give up committing crimes (Wei and Song, 2006). At the same time, classical criminology also argues that even the most minor punishment will have a deterrent effect when the penalty is determined (Beccaria, 2016). When a penalized enterprise receives an environmental administrative penalty, it sends a deterrent signal to the peer enterprises that the government’s environmental regulation will be more stringent. The business structure and production activities of the peer enterprises are similar to those of the penalized enterprises, so their production and operation processes are also at risk of being penalized. Their perception of the risks and costs of violating environmental regulations increases, and then they will check whether they are complying with environmental regulations based on the deterrent signal, weigh the costs of compliance and violation, and decide whether to carry out GTI. According to Hicks’ Induced Innovation Theory, stricter environmental enforcement will lead to changes in product cost prices and higher environmental costs. When peer enterprises perceive that the sum of the high penalty cost and the cost of reputation loss due to environmental pollution is greater than the reduction of production cost due to risky violation, which means the innovation compensation effect of GTI exceeds the cost of violation (Guo et al., 2018), the peer enterprises cannot obtain competitive advantage through violation, then the optimal choice is the compliance strategy, and GTI will be used to solve the problem. Generally speaking, after the peer enterprises invest successfully in GTI, making pollution meet the environmental standards, they can be exempted from paying the emission exceedance fees or from environmental administrative penalties, thus reducing the economic burden. The government provides tax incentives, financial subsidies, priority procurement, and other policies, which will also partially compensate for the increased costs caused by GTI (Costa-Campi et al., 2017). As the social awareness of green environmental protection increases, consumers are more inclined to choose environmentally friendly products (Li et al., 2016), and GTI by enterprises can not only form a differentiated product advantage but also shape a good social image (Sarkar, 2013) and gain social benefits (Peng and Li, 2005). Therefore, enterprises are more inclined to carry out GTI. Therefore, based on the Deterrence Theory, the peer enterprises will take the initiative to carry out GTI because of the deterrence signal.
According to Competition Theory, in order to maintain a relatively competitive position or to counteract aggressive behavior by their peers, firms will pay close attention to the behavior of other firms (Wu et al., 2022). When firms are subject to environmental administrative penalties, they may engage in GTI (Cai et al., 2020) because of financial penalties, legal liability (Fernando, 2008; Pei et al., 2015), and damage to their social reputation (Polinsky and Shavell, 2000). Enterprises taking the lead in developing a certain technological innovation can protect their legitimate rights and interests by applying for patents, maintain the exclusivity of the technology, consolidate or even expand market share, and further improve their competitiveness (Ambec et al., 2013), so the competitiveness of the penalized enterprises increases. Enterprises in the same industry face a similar market environment, and there is competition for interests and resources between enterprises (Wu et al., 2022). When penalized enterprises carry out GTI to enhance their competitiveness, if the peer enterprises do not follow them, they will be in a backward competitive position, and their market share may decrease, which will adversely affect long-term development. In addition, when the market competition is fierce, commodity prices and profits are easily affected, which in turn leads to market fluctuations. In order to alleviate this situation, the peer enterprises often choose a homogenization strategy to match the penalized enterprises’ behavior (Marvin et al., 2006), and also carry out GTI, thereby alleviating the intensity of competition, calming market volatility and reducing their risks (Marvin et al., 2006). Therefore, based on Competition Theory, the peer enterprises will passively carry out GTI due to competitive pressure.
In addition, the quantity of GTI cannot fully represent the level of independent innovation capabilities of peer enterprises (Zhang and Zheng, 2018). Low-quality GTI not only occupies the funds of peer enterprises, consumes a lot of scientific research resources, but also has difficulty meeting environmental supervision standards and improving market competitiveness. When the penalized enterprise is subject to an environmental administrative penalty, peer enterprises face external competitive pressure and deterrent signals, and their internal management must still follow the principle of profit maximization. However, the speculative behavior of lower-quality innovation cannot relieve the pressure of competition and the threat of penalties for peer enterprises. Therefore, the optimal strategy is to carry out high-quality patent research and development, improve the efficiency of patent technology transformation and application, maximize the use of limited resources to exert the compensation effect of technological innovation, and enhance their long-term competitive advantage (Jin et al., 2022). The theoretical analysis framework diagram is shown in Figure 1. Thus, the hypothesis is proposed as follows:
Hypothesis. The environmental administrative penalty of the punished enterprises can positively promote the quantity and quality of the peer enterprises’ GTI.
3 Methodology
3.1 Sample and data collection
According to the 16 types of heavily polluting industries defined in The Guidelines for Environmental Information Disclosure of Listed Companies (Draft for Comment) issued by China in 2010, the listed companies in the heavily polluting industries in Shanghai and Shenzhen A-shares from 2016 to 2020 are selected as research samples. Then we omit listed firms that are marked “Special Treatment” (ST), marked “Particular Transfer,” (PT) suspended listing, data missing, and subject to environmental administrative penalties. At last, we obtain 3,278 firm-year observations. The environmental administrative penalty data comes from the websites of the IPE, which is obtained through manual collection, and the enterprise GTI data comes from the Chinese Research Data Services (CNRDS) database, and the enterprise financial data comes from the China Stock Market and Accounting Research (CSMAR) database.
3.2 Variables and the measurement
3.2.1 Explained variable: Enterprises’ GTI level
Two indicators are established to measure the enterprise GTI capabilities: quantity of GTI (ANGP) and quality of GTI (AQGP).
Quantity of GTI (ANGP): It is measured by the number of corporate green patent applications.
Quality of GTI (AQGP): It is measured by the quality of enterprise green patent applications. Drawing on the practice of previous research (Akcigit et al., 2016; Zhang and Zheng, 2018), this paper uses the complexity of knowledge contained in a patent to measure the quality of green patent applications, which is defined as the knowledge breadth method. It refers to the calculation idea of industrial concentration, and weights the patent classification numbers at the group level. The bigger the variance of classification, the higher the quality of GTI.
3.2.2 Explanatory variable: Environmental administrative penalty
The environmental administrative penalty variable (Pind) refers to the methods of Kedia et al. (2015) and Valerie (2016) to measure the financial irregularities of enterprises in the industry. And it is expressed by the ratio of the number of penalized enterprises to the total number of enterprises in the industry.
3.2.3 Control variables
The corporate factors that affect GTI are mainly divided into three categories: corporate characteristics (Vogel, 2002), corporate financial performance (Cai and Li, 2017), and corporate governance characteristics (Qi et al., 2018), for which the following control variables are set. In terms of company characteristics, three variables are set: enterprise size (SIZE), listing age (AGE), and nature of property rights (SOE). In terms of the company’s financial performance, four variables are set: asset-liability ratio (DAR), return on total assets (ROTA), operating cash flow (OCF), and growth rate of operating profit (SGR). In terms of corporate governance characteristics, two variables are set: the largest shareholder shareholding ratio (FBSR) and agency fees (AFEE). The specific index calculation method is shown in Table 1.
3.3 Econometric model
This study uses multi-year and multi-enterprise panel data for panel regression. The fixed-effect model is finally selected through the Hausman test. In order to test the impact of environmental administrative penalties on the quantity and quality of peer enterprises’ GTI, model 1) for the number of green patent applications and model 2) for the knowledge width of green patent applications are established. Since the GTI may be affected by the previous period, the explanatory variables
4 Empirical results and analysis
4.1 Descriptive statistics
The results of descriptive statistics are shown in Table 2. The average, median and maximum value of ANGP for the number of green patent applications are 2.977, 0.000 and 606.000, and the standard deviation is 14.451. The average, median and maximum values of AQGP for the quality of green patent apply are 1.309, 0.000, 237.703, and the standard deviation is 6.220, indicating that most companies have a low level of GTI, and the GTI levels of different companies vary significantly. So differentiated data makes research feasible. The average and median value of Pind for the environmental administrative penalty of penalized enterprises are similar, the average value is 0.336, indicating that in most industries, companies that are punished account for a minority. The minimum value is 0.000, indicating that there is an industry in which no company has been penalized throughout the year.
4.2 Test results
Table 3 shows the estimated results using the fixed-effect model based on model 1) and model (2). The core explanatory variable is the environmental administrative penalty variable of the penalized enterprises with a lag of one period
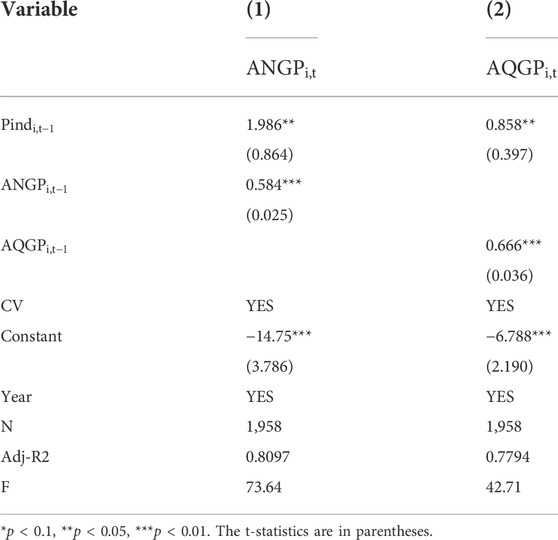
TABLE 3. Test results for the impact of the environmental administrative penalty on the peer enterprises’ GTI.
In the green patent application quantity model 1) and the green patent application quality model 2), the coefficients of the core explanatory variable of environmental administrative penalty for penalized enterprises
Besides, in the regression results, the explanatory variables of the GTI level lagging one period (
4.3 Robust tests and endogenous test
4.3.1 Variable substitution
4.3.1.1 Z-score normalization
The main explanatory variable of this paper
4.3.1.2 Dummy variable setting
Since the selection of different proxy variables to measure the GTI level of enterprises may have different effects on the estimation results, this paper refers to Zhang et al. (2022), setting dummy variables based on the median number of the quantity and quality of enterprise GTI to test the robustness. If the quantity of GTI of peer enterprises is greater than or equal to the median of all samples in the year, the dummy variable of the quantity of GTI (ANGP_M) is assigned to 1, which indicates that the number of green patent applications is high, otherwise it is assigned to 0, which indicates that the number of green patent applications is low. If peer enterprises’ quality of GTI is greater than or equal to the median of all samples in the year, the dummy variable of the quality of GTI (AQGP_M) is assigned to 1, which means the enterprise has high GTI quality, otherwise it is assigned to 0, which means the enterprise has low GTI quality. After replacing the variables, the benchmark regression is conducted again, and the results are shown in columns (3)–(4) of Table 4. The coefficient of the core explanatory variable
4.3.2 Model substitution
4.3.2.1 Heckman two-stage selection model
There may be sample selectivity bias in this paper. On the one hand, the green patent application data are all from the CNRDS database, but the CNRDS database only includes the green patent data of Shanghai and Shenzhen A-share listed firms, and it is difficult to obtain green patent data of heavily polluting enterprises other than Shanghai and Shenzhen A-shares. On the other hand, the sample data contains both high-quality GTI observations and low-quality GTI observations, but a high-quality GTI observation of zero does not cause bias only when it occurs randomly, but whether to carry out high-quality GTI activity is a decision made by the firm after considering the internal and external environment. Therefore, both quantitative and qualitative GTI variables are subject to selective bias. The Heckman two-stage selection model can be used to solve the sample selectivity bias problem, so it is used for the robust test. Firstly, the first stage green patent decision model is constructed for Probit regression, which mainly tests the correlation between environmental administrative penalties of penalized enterprises and the level of GTI of peer enterprises, and then calculates the inverse Mills ratio (IMR) according to the first-stage model. The second stage is a regular regression equation with the additional inverse Mills ratio (IMR). When Probit>0, ANGP and AQGP will only be observed, so enterprises with
4.3.2.2 Negative binomial regression model
As one of the explained variables in this paper, the quantity of GTI is measured by the number of green patents applied by enterprises in the year, which is a count variable and conforms to the Poisson distribution. However, the indicator of the number of green patent applications is excessively scattered and its expectation and variance have large differences, for which a negative binomial model is chosen for estimation. Meanwhile, the number of green patent applications has a large number of zero values in the year, and the zero-inflated negative binomial model should be used theoretically, but the p-value of the Vuong test statistic is not significant, so the zero-inflated negative binomial regression is rejected, and the standard negative binomial regression model is selected for robustness testing. The test results are shown in column (5) of Table 5. The parameter estimate of α is 2.55, while the 95% confidence interval of α is (2.13,3.06), so it is appropriate to use the standard negative binomial regression. Meanwhile, the estimate of the core explanatory variable
4.3.2.3 Panel Tobit model
Since one of the explained variables, the GTI quality index, is calculated by the knowledge breadth method, and its value is positive and approximately continuously distributed, but there are a large number of zero values, which is a lower bounded problem, so the panel Tobit regression is used to test the robustness of the GTI quality model. The results of the test are shown in column (6) of Table 5, and the p-value of the LR test is 0.000, indicating that the use of the panel Tobit model is appropriate. In addition, the coefficient of the core explanatory variable
4.3.3 Endogenous test
There is a mutually causal relationship between GTI and the profitability of enterprises. On the one hand, capital is an important factor for enterprises to carry out GTI, and enterprises with good profitability can obtain more capital from the profit, while the level of profitability is also a factor that external investors need to consider when investing in enterprises. On the other hand, it is known from the theory of technological innovation that technological innovation is a strong guarantee for the core competitiveness of enterprises. Green patents generated by enterprises’ high-quality GTI activities are easy to be examined by patent examiners, and their exclusivity and practicality are strong, which are more conducive to converting it into a product to obtain technology monopoly profits, thereby improving enterprises’ profitability. Given that the dynamic system GMM model can solve the endogeneity problem caused by reverse causality, the one-period lag of the SGR variable is selected as an instrumental variable for testing, and the IMR is added to correct the sample selectivity bias. The results are shown in Table 6. From the regression results of the test, the p-values of AR 1) are both less than 0.05 and the p-values of AR 2) are both greater than 0.1, indicating that the original hypothesis of “all instrumental variables are valid” (p-value greater than 0.1) cannot be rejected, which proves that the instrumental variables do not have over-identification problem and pass the over-identification test. In column (1), the
5 Heterogeneity analysis
5.1 The impact of the heterogeneous environmental administrative penalty on the peer enterprises’ GTI
The environmental administrative penalty is diverse in frequency, severity, and administrative level of the penalty implementers, and the deterrent force produced by different penalty results varies. To this end, the environmental administrative penalty variables of penalized enterprises are constructed according to these three dimensions to study the impact on the quantity and quality of peer enterprises’ GTI when penalized enterprises are subject to heterogeneous environmental administrative penalties.
The construction of the frequency variable of environmental administrative penalty for penalized enterprises (Pind_num) refers to the study of Valerie (2016). Taking the median of the frequency of environmental administrative penalties imposed on penalized enterprises in the industry, Pind_num is represented by the ratio of the number of companies with penalty frequency greater than the median to the total number of companies in the industry. The construction of the severity variable of environmental administrative penalty for penalized enterprises (Pind_str) refers to the study of Chen et al. (2021). Assign corresponding points to each environmental administrative penalty method, and then add the total score to get the severity of the environmental administrative penalty for each enterprise. The higher the score, the stronger the severity of the environmental administrative penalty the enterprise is subjected to. Taking the median of the penalized enterprises in the industry, Pind_str is represented by the ratio of the number of companies with penalty severity greater than the median to the total number of companies in the industry. A similar method is used to construct the administrative level variable of the penalty implementers (Pind_dep). Assign points to the penalty imposed on enterprises from different levels of administration. The prefecture-level city and below administrations are counted as 1 point, the provincial-level administrations are counted as 2 points, and the provincial-level administrations are counted as 3 points. And then add up to get the administrative level of the penalty implementers for each enterprise. Taking the median of the penalized enterprises in the industry, Pind_dep is represented by the ratio of the number of companies with a penalty administrative level greater than the median to the total number of companies in the industry. Substitute the above three variables for the variable
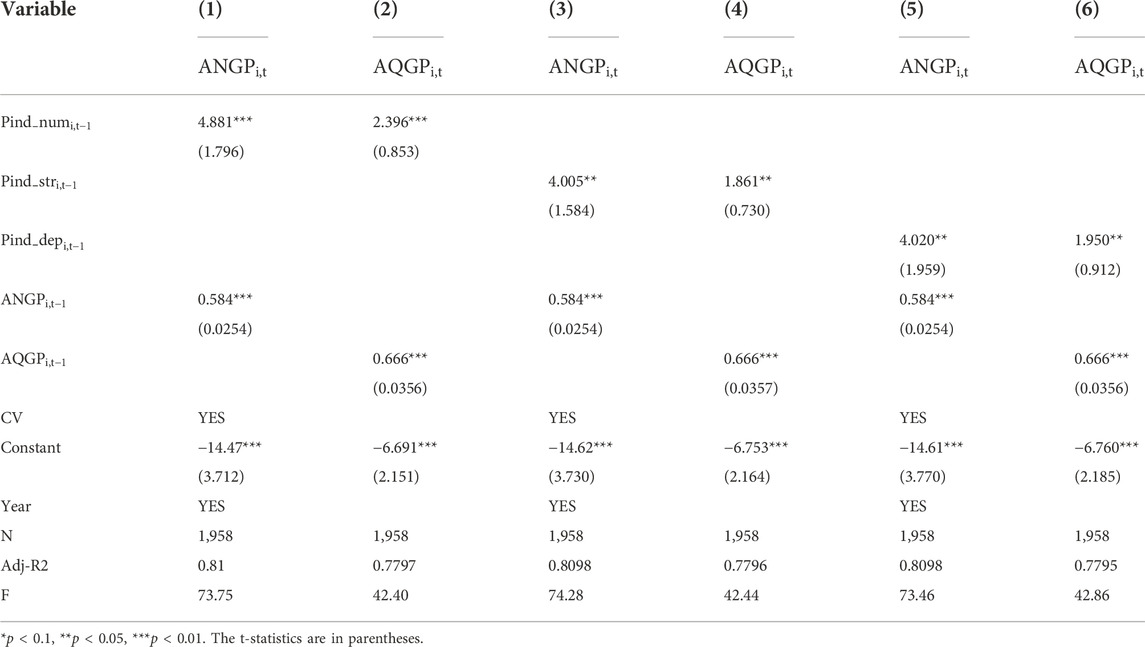
TABLE 7. Test results for the impact of heterogeneous environmental administrative penalty on the peer enterprises’ GTI.
In Table 7, the coefficients of
Specifically, in terms of penalty frequency, as penalized enterprises receive more environmental administrative penalties, the cost of breaking the law will also increase, forcing them to innovate green technologies (Cai et al., 2020) and creating competitive pressure on peer enterprises. At the same time, these penalties are transmitted to society as risk information again and again. The stronger the deterrent signal received by peer enterprises, the stronger their perception of the risk of being penalized and the cost of noncompliance (Ling et al., 2022), forcing them to check whether they can meet regulatory requirements and improve their GTI. In terms of the severity of the environmental administrative penalty, the deterrent effect of the penalty will vary depending on the means of penalties (Shimshack, 2014). The more severe the environmental administrative penalty, the greater the deterrent effect on the penalized enterprises. According to the strong Porter Hypothesis, penalized enterprises will innovate green technology (Miao et al., 2019), and improve their competitiveness. The competitive pressure on peer enterprises increases as the severity of the environmental administrative penalties increases. The disclosure of penalty information also increases the deterrent signals received by peer enterprises as the severity of environmental administrative penalties increase and reduces the information asymmetry between enterprises (Yan et al., 2022). For long-term development, the peer enterprises will also implement green strategies in the face of increasingly strong competitive pressure and deterrent signals, and improve their own GTI level. Regarding the administrative level of the penalty implementer of environmental administrative penalty, the environmental administration at all levels has different powers to impose fines and penalties. The higher the administrative level, the higher the amount of fine that the department can directly make, so the greater its deterrent effect (Xu et al., 2020). Besides, the information disclosure of enterprises that are subject to environmental administrative penalties by the environmental protection department at or above the provincial level is mandatory. The greater the information spreads, the greater the impact. Therefore, the higher the administrative level of the penalty implementer, the greater the deterrent effect on peer enterprises (Ling et al., 2022). In addition to receiving deterrent signals, peer enterprises will also be subject to competitive pressure from penalized enterprises, so peer enterprises will also be forced to improve their GTI level. All in all, the more frequent the penalty, the more severe the penalty, and the higher the administrative level of the penalty implementer, it will all send a signal to the society that environmental law enforcement is intensified, and the deterrent signal and competitive pressure received by peer enterprises will be stronger. When the compensation effect of GTI exceeds the cost caused by illegal activities, it provides the impetus for the sustainable innovation activities of peer enterprises, thereby improving their own GTI level (Porter, 1991).
5.2 The impact of the heterogeneous penalized enterprises on the peer enterprises’ GTI
The deterrent effect of environmental administrative penalties will vary due to the different competitiveness of the penalized enterprises. Using the method of Wang et al. (2020), the market share is used to measure the market competitiveness of the enterprise, where the market share is equal to the ratio of the company’s operating income to the total operating income of the industry. The ratio of the number of punished enterprises in the top 10% and the bottom 10% of the market share in the industry to the total number of enterprises in the same industry is taken to represent the environmental administrative penalty variables of highly competitive (Pcpt_B) and weakly competitive (Pcpt_S) companies, respectively. Replace the
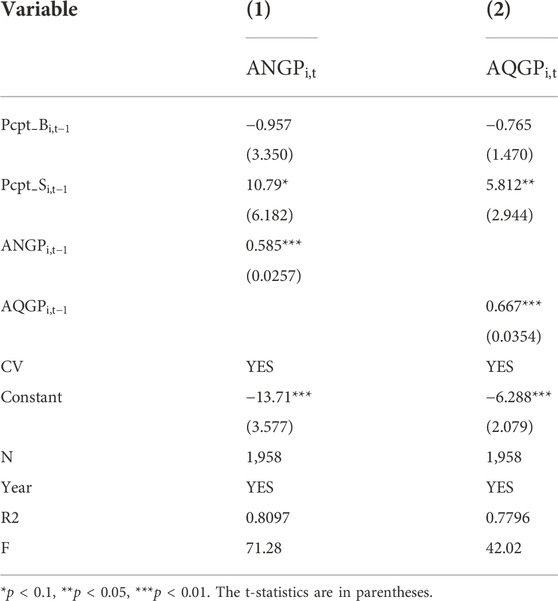
TABLE 8. Test results for the impact of the heterogeneous penalized enterprises on the peer enterprises’ GTI.
In columns (1) and (2), the coefficient of the
5.3 The impact of the environmental administrative penalty on the GTI of heterogeneous peer enterprises
For peer enterprises with potential violations, the innovation behaviors of state-owned enterprises and non-state-owned enterprises are quite different. Therefore, it is necessary to consider the property rights of peer enterprises. According to the study by Wang et al. (2021), classify the sample according to state-owned enterprises and non-state-owned enterprises to form two subsamples, and the property rights variable (SOE) in the control variable is deleted. Test the differences in the sensitivity to environmental administrative penalties of the peer enterprises with heterogeneous property rights. The test results are shown in Table 9.
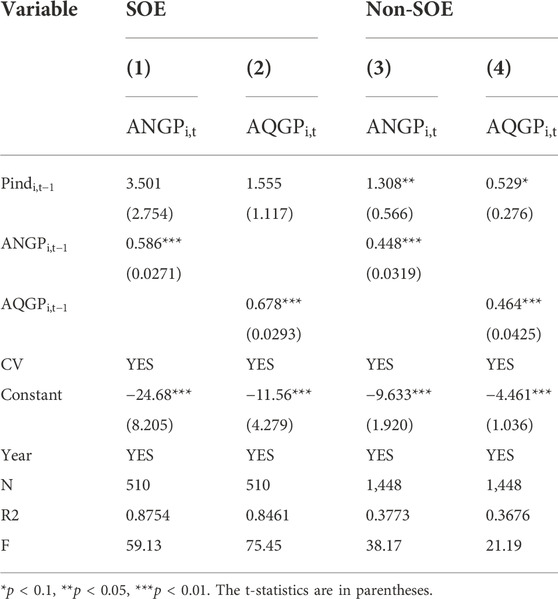
TABLE 9. Test results for the impact of the environmental administrative penalty on the GTI of heterogeneous peer enterprises.
According to Table 9, the coefficients of the variable
6 Further analysis: The moderating effect of peer enterprises’ financing constraints
The GTI of peer enterprises is easily restricted by their financing constraints in the process of development (Ji, 2018). The capital use cycle of innovation activities is long, the capital demand is large, and the profitability is also unstable. At the same time, the incompleteness of the capital market, the asymmetry of information, and the existence of agency problems make peer enterprises face serious financing constraints, which in turn affects the GTI. This section examines the moderating effect of financing constraints in the process of environmental administrative penalty affecting the peer enterprises’ GTI.
Among the measurement methods of financing constraints (FC), the SA index constructed by Hadlock and Pierce (2010) can fully reflect the level of financing constraints of enterprises. The SA index does not contain endogenous variables, which can avoid measurement bias and subjectivity problems existing in other methods, and is widely used in various studies (Wu and Huang, 2017).
This paper uses the absolute value of the SA index to measure the level of financing constraints of peer enterprises. When the absolute value of the SA index is larger, it indicates that the financing constraints of peer enterprises are greater (Gu and Zhu, 2021). In order to examine the moderating effect of financing constraints on environmental administrative penalty and peer enterprises’ GTI, the following model is constructed:
The test results are shown in Table 10. Columns (1) and (2) are the test results of the moderating effect of financing constraints on the relationship between environmental administrative penalty and the quantity and quality of peer enterprises’ GTI. The results show that the coefficient of the interaction term (
This is mainly because as the main influencing factor of technology innovation, capital is essential for enterprises to carry out GTI (Gu et al., 2021). Like other innovation activities, GTI activities are characterized by high investment, low return, and high risk, which require sufficient and stable financial support (Yu et al., 2021). The financing funds for enterprises’ innovation activities mainly come from external funds provided by financial markets and internal funds generated from production and operation activities. Due to the information asymmetry between enterprises and external investors, investors are likely to set a large number of restrictive clauses for enterprises while investing funds, increasing the hidden financing costs of enterprises (Xu et al., 2021), which makes it more difficult for enterprises to obtain external funds for investment in GTI than other types of investments. In addition, when peer enterprises face high external financing constraints, they may turn to internal financing such as their funds to carry out GTI, and if their funds cannot meet the financial needs of GTI, it will also adversely affect GTI. When the lack of capital restricts the GTI decision of the peer enterprises, it also weakens their motivation to improve the quality of GTI (Deng et al., 2022). The peer enterprises will reduce the research and development of GTI projects (Yang and Xi, 2019), so both the quantity and quality of GTI will be affected, which leads to the weakened role of financing constraints in environmental administrative penalties promoting the quantity and quality of GTI.
7 Conclusion and policy implications
Based on the existing research results, this paper takes the listed companies of heavily polluting industries in Shanghai and Shenzhen A-share from 2016 to 2020 as the research sample, divides the GTI level into quantity and quality, and studies the impact of the environmental administrative penalty on the peer enterprises’ GTI. Combined with empirical results, it is found that: 1) Environmental administrative penalty can significantly promote the quantity and quality of peer enterprises’ GTI. 2) The diversity of environmental administrative penalties makes the deterrent effect of different penalties vary. The frequency of the penalty, the severity of the penalty, and the administrative level of the penalty implementer can positively promote the quantity and quality of peer enterprises’ GTI. 3) The impact of the environmental administrative penalties on peer enterprises will vary due to the different characteristics of the penalized enterprises. Environmental administrative penalties of penalized enterprises with weak competitiveness can significantly promote the quantity and quality of peer enterprises’ GTI, while the penalty of highly competitive companies has no significant effect. 4) The different property rights of peer enterprises also make their sensitivity to environmental administrative penalties different. The environmental administrative penalty can significantly promote the quantity and quality of GTI in non-state-owned peer enterprises but have no significant impact on state-owned peer enterprises. 5) When peer enterprises face high financing constraints, the effect of the environmental administrative penalties on the quantity and quality of peer enterprises’ GTI will be weakened.
These findings provide the following policy implications: Governments should increase the mandatory disclosure of information on corporate environmental administrative penalties. Also considering that the frequency, severity, and administrative level of the penalty implementer of environmental administrative penalties have a significant contribution to the quantity and quality of GTI in the peer enterprises, the government should implement more severe environmental administrative penalties to maximize their deterrent effect. But the government’s policymaking should not be “one size fits all.” They should assess the competitiveness and the nature of the property rights of enterprises, and formulate more targeted policies. In addition, the government should also standardize and improve the financing mechanism of enterprises’ GTI, alleviate the problem of capital demand in the process of transformation and upgrading of enterprises’ green industries, stimulate the vitality of enterprise innovation, and avoid the occurrence of the “patent bubble” phenomenon.
This study may have some limitations as follows. First, the sample only includes listed companies, but environmental administrative penalties are not only imposed on listed companies, and environmental administrative penalties for non-listed companies can also be observed by the peer enterprises. Besides, the listed companies are easy to be monitored, so their impact on peer enterprises may not be the same as that of non-listed companies. Therefore, in future research, case studies can be used to analyze the impact of environmental administrative penalties imposed on non-listed companies, making the scope of the study as comprehensive as possible. Second, the period of the sample is 2016–2020, but enterprise GTI from research and development to patent application generally takes 3 years or even longer. What’s more, China has implemented a stricter environmental protection law since 2015 and proposed to resolutely fight the nationwide battle to prevent and control pollution in 2018. Environmental supervision is increasing year by year, so the 5-year period can hardly reflect the long-term mechanism of the environmental administrative penalty. For this reason, the period of the sample should be broadened in future studies to better study the long-term governance mechanism of environmental administrative penalties.
Data availability statement
Publicly available datasets were analyzed in this study. This data can be found here: The Chinese Research Data Services (CNRDS) database, the China Stock Market and Accounting Research (CSMAR) database and the websites of the IPE.
Author contributions
All authors contributed to the study’s conception and design. XC put forward the idea, designed the study, and reviewed the manuscript. Material preparation, data collection and analysis, and the first draft of the manuscript were performed by MZ. All authors read and approved the final manuscript.
Funding
This work was supported by The National Social Science Fund of China (Grant number: 15BGL067).
Acknowledgments
The authors would like to thank the reviewers who provided valuable comments on the earlier version of this manuscript.
Conflict of interest
The authors declare that the research was conducted in the absence of any commercial or financial relationships that could be construed as a potential conflict of interest.
Publisher’s note
All claims expressed in this article are solely those of the authors and do not necessarily represent those of their affiliated organizations, or those of the publisher, the editors and the reviewers. Any product that may be evaluated in this article, or claim that may be made by its manufacturer, is not guaranteed or endorsed by the publisher.
Abbreviations
GTI, green technology innovation.
References
Akcigit, U., Baslandze, S., and Stantcheva, S. (2016). Taxation and the international mobility of inventors. Am. Econ. Rev. 106 (10), 2930–2981. [J]. doi:10.1257/aer.20150237
Ali, M. (2021). Imitation or innovation: To what extent do exploitative learning and exploratory learning foster imitation strategy and innovation strategy for sustained competitive advantage? Technol. Forecast. Soc. Change 165, 120527. [J]. doi:10.1016/j.techfore.2020.120527
Ali, S., Jiang, J., Ahmad, M., Usman, O., and Ahmed, Z. (2022). A path towards carbon mitigation amidst economic policy uncertainty in BRICS: An advanced panel analysis. Environ. Sci. Pollut. Res. 29, 62579–62591. [J]. doi:10.1007/s11356-022-20004-8
Ali, S., Zhang, J., Usman, M., Khan, F. U., Ikram, A., and Anwar, B. (2019). Sub-national institutional contingencies and corporate social responsibility performance: Evidence from China. Sustainability 11, 5478. doi:10.3390/su11195478
Ambec, S., Cohen, M. A., Elgie, S., and Lanoie, P. (2013). The porter hypothesis at 20: Can environmental regulation enhance innovation and competitiveness? Rev. Environ. Econ. Policy 7 (1), 2–22. [J]. doi:10.1093/reep/res016
Ayres, I., and Braithwaite, J. (1995). Responsive regulation: Transcending the deregulation debate. Oxford, NY, USA: Oxford University Press.
Bi, Q., Li, X. Y., and Yu, L. C. (2018). Research on the heterogeneity of quantiles between environmental tax and enterprise competitiveness——panel quantile regression analysis. Collect. Essays Finance Econ. 235 (7), 37–47. [J]. doi:10.13762/j.cnki.cjlc.2018.07.003
Brown, S., Ghosh, P., and Taylor, K. (2015). Household finances and social interaction: Bayesian analysis of household panel data. Rev. Income Wealth 62 (3), 467–488. [J]. doi:10.1111/roiw.12174
Cai, S. H., and Yu, L. P. (2017). Innovation quantity, innovation quality and firm benefit. China Soft Sci. 48 (5), 30–37. [J].
Cai, W. G., and Li, G. P. (2018). The drivers of eco-innovation and its impact on performance: Evidence from China. J. Clean. Prod. 176, 110–118. doi:10.1016/j.jclepro.2017.12.109
Cai, X., Zhu, B., Zhang, H., Li, L., and Xie, M. (2020). Can direct environmental regulation promote green technology innovation in heavily polluting industries? Evidence from Chinese listed companies. Sci. Total Environ. 746, 140810. [J]. doi:10.1016/j.scitotenv.2020.140810
Chen, X. Y., Xiao, H., and Zhang, G. Q. (2021). Does environmental administrative penalty promote corporate environmental governance? An analysis based on the process and outcome dual dimensions. Bus. Manag. J. 43 (6), 136–155. [J].
Costa-Campi, T., García-Quevedo, J., and Martínez-Ros, E. (2017). What are the determinants of investment in environmental R&D?. Energy Policy 104, 455–465.
Deng, H., Li, C., and Wang, L. (2022). The impact of corporate innovation on environmental performance: The moderating effect of financing constraints and government subsidies. Sustainability 14, 11530. doi:10.3390/su141811530
Ding, X., Appolloni, A., and Shahzad, M. (2021). Environmental administrative penalty, corporate environmental disclosures and the cost of debt. J. Clean. Prod. 332, 129919. [J]. doi:10.1016/j.jclepro.2021.129919
Ding, X., Qu, Y., and Shahzad, M. (2020). Stock market's reaction to self-disclosure of environmental administrative penalties: An empirical study in China. Pol. J. Environ. Stud. 29 (6), 4029–4039. [J]. doi:10.15244/pjoes/118584
Ding, X., and Shahzad, M. (2022). Environmental administrative penalty, environmental disclosures, and the firm’s cash flow: Evidence from manufacturing firms in China. Environ. Sci. Pollut. Res. 29, 36674–36683. [J]. doi:10.1007/s11356-021-18145-3
Ding, X., Ying, Q., and Shahzad, M. (2019). The impact of environmental administrative penalties on the disclosure of environmental information. Sustainability 11 (20), 5820. [J]. doi:10.3390/su11205820
Du, W. Q., and Guo, S. J. (2021). Firm heterogeneity, R&D investment and innovation performance: An empirical study based on GPS. Sci. Technol. Manag. Res. 41 (23), 124–132. [J].
Earnhart, D. (2004). Regulatory factors shaping environmental performance at publicly-owned treatment plants. J. Environ. Econ. Manag. 48 (1), 655–681. [J]. doi:10.1016/j.jeem.2003.10.004
Fairhurst, D., and Nam, Y. (2018). Corporate governance and financial peer effects. Financ. Manag. 49, 235–263. [J]. doi:10.1111/fima.12240
Fernando, S., and Fernando, C. S. (2008). Environmental risk management and the cost of capital. Strateg. Manag. J. 29 (6), 569–592. [J]. doi:10.1002/smj.678
George van, L., and Mohnen, P. (2017). Revisiting the porter hypothesis: An empirical analysis of green innovation for The Netherlands. Econ. Innovation New Technol. 26, 63–77. [J]. doi:10.1080/10438599.2016.1202521
Gu, H. F., and Zhu, H. P. (2021). Does executive pay gap promote enterprises innovation investment? ——based on evidence from Chinese A-share listed companies. Account. Res. 12, 107–120. [J].
Guo, Y. Y., Zhang, S., and Zhang, D. P. (2018). The impacts of environmental regulation and governmental R&D funding on green technology innovation: Suppressing or promoting? A literature review. East China Econ. Manag. 32 (07), 40–47. [J]. doi:10.19629/j.cnki.34-1014/f.170926016
Hadlock, J., and Pierce, R. (2010). New evidence on measuring financial constraints: Moving beyond the KZ index. Rev. Financ. Stud. 23 (5), 1909–1940. [J]. doi:10.1093/rfs/hhq009
Hall, J. (2022). Facing the music through environmental administrative penalties: Lessons to be learned from the implementation and impact of section 24G? Potchefstroom Electron. Law J. 25. [J]. doi:10.17159/1727-3781/2022/v25ia13321
Hong, M., Zhenghui, L., and Drakeford, B. (2021). Do the green credit Guidelines affect corporate green technology innovation? Empirical research from China. Int. J. Environ. Res. Public Health 18 (4), 1682. [J]. doi:10.3390/ijerph18041682
Hou, K., Zhang, Y. Y., Xiang, X. P., and Ren, P. (2018). Peer group socialization on academic achievement during early adolescence: Moderating effects of perceptions of school climate. Psychol. Dev. Educ. 34 (03), 294–303. [J]. doi:10.16187/j.cnki.issn1001-4918.2018.03.05
Hua, S. M., and Li, J. Z. (2022). Can environmental regulation tools improve the quantity and quality of enterprise green technology innovation under the digital economy condition? Sci. Technol. Prog. Policy 1-10. [J/OL][-11-13] Available at: http://kns.cnki.net/kcms/detail/42.1224.g3.20220822.0803.002.html.
Ji, L. Y. (2018). Impact of technological innovation on upgrading of China's manufacturing industry structure: Based on adjustment of financing constraint. Technol. Econ. 37 (11), 30–36. [J].
Jin, Y., Wen, W., and He, Y. (2022). Impact of digital transformation on corporate green innovation: Evidence from China’s manufacturing listed companies. Finance Trade Res. 33 (7), 69–83. [J]. doi:10.19337/j.cnki.34-1093/f.2022.07.006
Kedia, S., Koh, K., and Rajgopal, S. (2015). Evidence on contagion in earnings management. Account. Rev. 90 (6), 2337–2373. [J]. doi:10.2308/accr-51062
Li, J. N., and Zhong, T. L. (2019). Peer effects in corporate investment: Empirical study based on Chinese listed firms. Chin. J. Manag. Sci. 27 (12), 22–31. [J]. doi:10.16381/j.cnki.issn1003-207x.2019.12.003
Li, S., Jayaraman, V., Paulraj, A., and Shang, K. c. (2016). Proactive environmental strategies and performance: Role of green supply chain processes and green product design in the Chinese high-tech industry. Int. J. Prod. Res. 54 (7), 2136–2151. [J]. doi:10.1080/00207543.2015.1111532
Lieberman, M. B., and Asaba, S. (2006). Why do firms imitate each other? Acad. Manage. Rev. 31 (2), 366–385. [J]. doi:10.5465/amr.2006.20208686
Ling, H., Zhong, T., Gan, S., Liu, J., and Xu, C. (2022). Penalties vs. Subsidies: A study on which is better to promote corporate environmental governance. Front. Environ. Sci. 10, 10. [J]. doi:10.3389/fenvs.2022.859591
Liu, L. Q., Chen, Z. R., and Wang, G. (2020). Market signal, technical characteristics and the international high-quality patents in China. Bus. Manag. J. 42 (02), 23–39. [J]. doi:10.19616/j.cnki.bmj.2020.02.002
Liu, Y. D., Padgett, Carol, and Yin, Chao (2022). Internal information quality and financial policy peer effects. Int. Rev. Financial Analysis 84, 102357. [J]Vol. doi:10.1016/j.irfa.2022.102357
Lu, D., Wang, Y. C., and Fu, P. (2014). Do CEO incentives improve the effectiveness of internal control? Empirical evidence from listed state-owned enterprises. Account. Res. 6, 66–72+97. [J].
Lu, N., Wu, J., and Liu, Z. (2022). How does green finance reform affect enterprise green technology innovation? Evidence from China. Sustainability 14, 9865. doi:10.3390/su14169865
Lu, R., Wang, C., and Deng, M. M. (2017). Peer effect” in capital structure of China's listed firms. Bus. Manag. J. 39 (1), 181–194. [J]. doi:10.19616/j.cnki.bmj.2017.01.012
Machokoto, M., Gyimah, D., and Ntim, G. (2021). Do peer firms influence innovation?. Br. Account. Rev. 53, 100988. [J]. doi:10.1016/j.bar.2021.100988
Manski, F. (2000). Economic analysis of social interactions. J. Econ. Perspect. 14 (3), 115–136. [J]. doi:10.1257/jep.14.3.115
Manski, F. (1993). Identification of endogenous social effects: The reflection problem. Rev. Econ. Stud. 60 (3), 531. [J]. doi:10.2307/2298123
McGartland, A., Revesz, R., Axelrad, A., Dockins, C., Sutton, P., and Woodruff, T. J. (2017). Estimating the health benefits of environmental regulations. Science 357 (6350), 457–458. [J]. doi:10.1126/science.aam8204
Miao, M., Su, Y. D., Zhu, X., Jiang, Y. S., and Zhang, H. Y. (2019). The influence of environmental regulation on corporate technological innovation——based on the test of mediating effect of financing constraints. Soft Sci. 33 (12), 100–107. [J]. doi:10.13956/j.ss.1001-8409.2019.12.16
Nadeau, L. W. (1997). EPA effectiveness at reducing the duration of plant-level noncompliance. J. Environ. Econ. Manag. 34 (1), 54–78. [J]. doi:10.1006/jeem.1997.1003
Pei, H., Chang, Y., Zeng, Q., and Pei, H. (2015). Do lenders applaud corporate environmental performance? Evidence from Chinese private-owned firms. J. Bus. Ethics 143, 179–207. [J]. doi:10.1007/s10551-015-2758-2
Peng, F., and Li, B. D. (2005). Analysis of environment protection investment. Environ. Sci. Technol. 3, 72–74+119. [J]. doi:10.19672/j.cnki.1003-6504.2005.03.031
PIRS (2021). 2021 blue book of scientific and technological innovation of Chinese enterprises. Shenzhen city, China: Prospective Industry Research Institute. Available at: https://bg.qianzhan.com/report/detail/2111101550234741.html.
Polinsky, A. M., and Shavell, S. (2000). The economic theory of public enforcement of law. J. Econ. Literature 38 (1), 45–76. [J]. doi:10.1257/jel.38.1.45
Qi, S. Z., Lin, S., and Cui, J. B. (2018). Do environmental rights trading schemes induce green innovation? Evidence from listed firms in China. Econ. Res. J. 53 (12), 129–143. [J].
Qi, Y., Lu, H. Y., and Zhang, N. C. (2016). Can environmental regulation achieve win-win of “reducing pollution” and “increasing performance”? ——evidence from key environmental protection cities with “compliance” and “non-compliance” quasi-experimental. Finance Trade Econ. 9, 126–143. [J]. doi:10.19795/j.cnki.cn11-1166/f.2016.09.010
Ramanathan, R., Qile, H., Black, A., Ghobadian, A., and Gallear, D. (2017). Environmental regulations, innovation and firm performance: A revisit of the porter hypothesis. J. Clean. Prod. 155, 79–92. [J]. doi:10.1016/j.jclepro.2016.08.116
Rind, A., Akbar, S., Boubaker, S., Lajili-Jarjir, S., and Mollah, S. (2021). The role of peer effects in corporate employee welfare policies. Br. J. Manag. 33, 1609–1631. [J]. doi:10.1111/1467-8551.12513
Sarkar, A. N. (2013). Promoting eco-innovations to leverage sustainable development of eco-industry and green growth. Eur. J. Sustain. Dev. 2 (1), 171–224. [J].
Seo, Hojun (2021). Peer effects in corporate disclosure decisions. J. Account. Econ. 71 (1), 101364. [J]Vol. doi:10.1016/j.jacceco.2020.101364
Shevchenko, A. (2020). Do financial penalties for environmental violations facilitate improvements in corporate environmental performance? An empirical investigation. Bus. Strategy Environ. 30, 1723–1734. [J]. doi:10.1002/bse.2711
Shimshack, P. (2014). The economics of environmental monitoring and enforcement. Annu. Rev. Resour. Econ. 6 (1), 339–360. [J]. doi:10.1146/annurev-resource-091912-151821
Sun, Z., and Zhang, W. (2019). Do government regulations prevent greenwashing? An evolutionary game analysis of heterogeneous enterprises. J. Clean. Prod. 231, 1489–1502. [J]. doi:10.1016/j.jclepro.2019.05.335
Tao, F., Zhao, J. Y., and Zhou, H. (2021). Does Environmental Regulation Improve the Quantity and Quality of Green Innovation—Evidence from the Target Responsibility System of Environmental Protection. China Industrial Economics 2021 (02), 136–154. doi:10.19581/j.cnki.ciejournal.2021.02.016
Valerie, L. (2016). Do false financial statements distort peer firms' decisions? Account. Rev. 91 (1), 251–278. [J]. doi:10.2308/accr-51096
Vogel, D. (2002). Globalization and environmental reform: The ecological modernization of the global economy by arthur P. J. Mol. Political Sci. Q. 117, 691–693. [J]. doi:10.2307/798155
Walters, G. D. (2018). Resistance to peer influence and crime desistance in emerging adulthood: A moderated mediation analysis. Law Hum. Behav. 42 (6), 520–530. [J]. doi:10.1037/lhb0000293
Wang, L., Kong, D. M., and Dai, Y. H. (2018). Politicians' promotion pressure and firm innovation. J. Manag. Sci. China 21 (1), 111–126. [J].
Wang, L., Yang, X. Q., Zhang, P. C., Li, W. Y., and Zhang, S. M., (2022). Hear all parties: Executive social networks and peer effects in firm’s investment decisions. J. Manag. Sci. China 25 (6), 81–99. [J]. doi:10.19920/j.cnki.jmsc.2022.06.005
Wang, R., Wijen, F., and Heugens, P. P. M. A. R. (2018). Government's green grip: Multifaceted state influence on corporate environmental actions in China. Strateg. Manag. J. 39 (2), 403–428. [J]. doi:10.1002/smj.2714
Wang, L. P., Li, C., and Li, S. Q. (2020). Can environmental information disclosure regulate the relationship between environmental cost and enterprise value. Int. J. Environ. Pollut.y 67 (2–4), 95–110.
Wang, W., Li, N., Wang, Z. R., and Qi, X. H. (2020). Study on the relationship between government subsidies willingness to explore innovation and market competitiveness of enterprises. Sci. Technol. Manag. Res. 40 (5), 15–22. [J].
Wang, Y., Li, Y. X., Ma, Z., and Song, J. B. (2020). Can the penalty for environmental violation act as a deterrent to peers? The evidence from the peer effect of environmental regulation. J. Manag. Sci. China 23 (1), 77–95. [J].
Wang, Y., Yanxi, L., Zhuang, M., and Song, J. (2019). The deterrence effect of a penalty for environmental violation. Sustainability 11 (15), 4226. [J]. doi:10.3390/su11154226
Wang, Z. Y., Cao, Y., and Lin, S. L. (2021). The characteristics and heterogeneity of environmental regulation's impact on enterprises' green technology innovation——based on green patent data of listed firms in China. Stud. Sci. Sci. 39 (5), 909–919+929. [J]. doi:10.16192/j.cnki.1003-2053.20200916.001
Wei, J., and Song, Y. K. (2006). Deterrent theory of punishment: Past, now and future——analysis of economics of punishment. Study & Explor. 4, 193–197. [J].
Wu, N., Bai, Y. X., and An, Y. (2022). Active imitation or passive reaction: Research on the peer effect on trade credit. Nankai Bus. Rev. 1-31. [J/OL][-04-13] Available at: http://kns.cnki.net/kcms/detail/12.1288.F.20220315.1615.003.html.
Wu, Q. S., and Huang, X. H. (2017). The function display of finance company and financing constraints relieve of the group's listed companies. China Ind. Econ. 9, 156–173. [J]. doi:10.19581/j.cnki.ciejournal.2017.09.009
Xin, C., Hao, X., and Cheng, L. (2022). Do environmental administrative penalties affect audit fees? Results from multiple econometric models. Sustainability 14 (7), 4268. [J]. doi:10.3390/su14074268
Xu, L., Lin, S. Y., and Qian, S. F. (2021). Slow-release effect of environmental information disclosure and green technology innovation on financing constraints. Secur. Mark. Her. 9, 23–33. [J].
Xu, Y. K., Qi, Y., and Song, P. F. (2020). Environmental punishment, corporate performance and emission reduction incentive——empirical evidence from China’s industrial listed companies. J. China Univ. Geosciences Soc. Sci. Ed. 20 (4), 72–89. [J].
Yan, Z. J., Zhang, B. B., and Hu, L. L. (2022). Can environmental information disclosure improve total factor energy efficiency? A quasi-natural experiment from the pollution information transparency index. China Popul. Resour. Environ. 32 (06), 67–75. [J].
Yang, G. Z., and Xi, Y. T. (2019). Empirical study on financing constraints of green technology innovation activities of enterprises. J. Industrial Technol. Econ. 38 (11), 70–76. [J].
Yang, Y., Liu, D., Zhang, L., and Yin, Y. (2021). Social trust and green technology innovation: Evidence from listed firms in China. Sustainability 13, 4828. doi:10.3390/su13094828
Ye, Q., Zeng, G., Dai, S. Q., and Wang, F. L. (2018). Research on the effects of different policy tools on China's emissions reduction innovation: Based on the panel data of 285 prefectural-level municipalities. China Popul. Resour. Environ. 28 (02), 115–122. [J].
Yin, Z. P., and Zhang, Z. G. (2016). Manager tenure, internal control and strategic differences. China Soft Sci. 12, 132–143. [J].
Yu, C. H., Wu, X. Q., Zhang, D. Y., Chen, S., and Zhao, J. S. (2021). Demand for green finance: Resolving financing constraints on green innovation in China. Energy Policy 153, 112255. doi:10.1016/j.enpol.2021.112255
Zeng, J. H., Yu, C. Y., Li, J. W., and Huang, X. R. (2020). Peer effect of high-tech enterprises' R&D investment ——adjustment of environmental uncertainty and intellectual property protection. Sci. Technol. Prog. Policy 37 (02), 98–105. [J].
Zhang, C., and Xu, C. (2022). Nature of property rights, capital structure and enterprise innovation [j]. Econ. Theory Bus. Manag. 42 (3), 38–53.
Zhang, G. S., and Du, P. F. (2022). The impact of digital transformation on technological innovation of Chinese enterprises: Quantity increase or quality improvement? Bus. Manag. J. 44 (06), 82–96. [J]. doi:10.19616/j.cnki.bmj.2022.06.005
Zhang, H. Z., and Xing, M. R. (2022). Global climate governance in the post-paris era: New challenges, new ideas and China’s solutions. Int. Rev. 2, 99–127. [J].
Zhang, J., and Zheng, W. P. (2018). Has catch-up strategy of innovation inhibited the quality of China's patents? Econ. Res. J. 53 (5), 28–41. [J].
Zheng, L. (2015). Neighborhood effects and peer effects in education: Methods, evidences, and policy implications. J. Educ. Stud. 11 (5), 99–110. [J]. doi:10.14082/j.cnki.1673-1298.2015.05.013
Keywords: environmental administrative penalty, green technology innovation, peer effect, heterogeneity, moderating effect
Citation: Chen X and Zhan M (2022) Does environmental administrative penalty promote the quantity and quality of green technology innovation in China? Analysis based on the peer effect. Front. Environ. Sci. 10:1070614. doi: 10.3389/fenvs.2022.1070614
Received: 15 October 2022; Accepted: 16 November 2022;
Published: 29 November 2022.
Edited by:
Ghassan H. Mardini, Qatar University, QatarReviewed by:
Chengyu Li, Zhengzhou University of Light Industry, ChinaOtilia Manta, Romanian Academy, Romania
Shahid Ali, Nanjing University of Information Science and Technology, China
Copyright © 2022 Chen and Zhan. This is an open-access article distributed under the terms of the Creative Commons Attribution License (CC BY). The use, distribution or reproduction in other forums is permitted, provided the original author(s) and the copyright owner(s) are credited and that the original publication in this journal is cited, in accordance with accepted academic practice. No use, distribution or reproduction is permitted which does not comply with these terms.
*Correspondence: Meng Zhan, emFtX2hlcmVAMTYzLmNvbQ==