- School of Economics and Management, China University of Petroleum, Beijing, China
Based on the panel data of 30 provinces in China from 2010 to 2019, this study empirically analyzes the relationship between fiscal decentralization and carbon productivity using a spatial econometric model and calculates the direct effect, spatial spillover effect, and total effect of fiscal revenue decentralization and fiscal expenditure decentralization on carbon productivity through effect decomposition. The empirical results show that 1) the spatial agglomeration effect of China’s provincial carbon productivity is obvious, which shows an upward trend. The heterogeneity of carbon productivity among different provinces is obvious. The overall performance is as follows: Eastern provinces > Central provinces > Western provinces. 2) Fiscal revenue decentralization and fiscal expenditure decentralization can significantly promote the improvement of carbon productivity. Fiscal expenditure decentralization plays a greater role in promoting carbon productivity than fiscal revenue decentralization. 3) Fiscal revenue decentralization and fiscal expenditure decentralization have significant positive direct effects and negative spatial spillover effects on the improvement of carbon productivity. Increasing fiscal decentralization is conducive to improving the carbon productivity of the province, but it will inhibit the carbon productivity of neighboring provinces. Finally, it puts forward policy suggestions to promote the improvement of carbon productivity from the perspective of fiscal decentralization.
1 Introduction
In 2003, Britain proposed “low-carbon economy.” Low-carbon development has become an inevitable choice to solve the prominent problems of resource and environmental constraints and achieve sustainable development (Bauer et al., 2013; Shen, 2017; Lin and Li, 2022). Low-carbon economy is guided by the comprehensive green transformation of economic and social development to realize the high-quality development of industrial structure, production mode, and lifestyle. Its most remarkable characteristics are low energy consumption, low pollution, and low emission (Liu and Feng, 2011; Zhang et al., 2015; Robertson, 2016; Sengupta et al., 2020; Wu et al., 2021; Rabia et al., 2022). According to the International Energy Agency (IEA), China’s total carbon dioxide emissions had nearly doubled from 5.407 billion tons in 2005 to 9.894 billion tons in 2020. According to the statistics of the World Bank, China overtook the United States to become the world’s largest carbon emitter in 2005. In terms of the proportion, China’s carbon emission has continuously increased in the total global carbon emission, reaching 31% at present. In September 2020, China proposed that carbon emissions should peak by 2030 and strive to achieve “carbon neutrality” by 2060. China is in the key stage of high-quality economic development. Therefore, how to coordinate the relationship between low-carbon development and high-quality economic development has become the focus from all walks of life. As a positive indicator to measure the economic benefits of CO2 emissions, carbon productivity has built a bridge between high-quality economic development and energy conservation and emission reduction. Therefore, improving the level of carbon productivity has become the key to the development of a low-carbon economy in China (Liu and Zhang, 2021a; Chen et al., 2022).
China’s carbon emission problem is caused by extensive economic development, which originates from the government behaviors under “China’s decentralization.” Because China’s economic development has typical characteristics of “government leading,” the behavior of local governments is bound to have a great impact on the economy and environment. The behavior of local governments under the fiscal decentralization system plays a vital role in carbon dioxide emissions (Kuai et al., 2019; Wang et al., 2022). In terms of revenue, it mainly promotes low-carbon development through green taxes and green government bonds; in terms of expenditure, it mainly promotes low-carbon development through government green procurement and green transfer payment. So what effect will fiscal decentralization have on carbon productivity? Does this effect have spatial spillover effect? Will there be heterogeneity in the impact of fiscal revenue decentralization and fiscal expenditure decentralization on carbon productivity? On the one hand, answering these questions can further enrich the relevant theories of fiscal decentralization system reform, innovation-driven development, and environmental governance with Chinese characteristics. On the other hand, it can provide relevant policy suggestions on how to maintain high-quality economic development and win the battle of carbon emission reduction under the new normal.
2 Literature Review
Carbon productivity is one of the important bridges used to measure economic growth and carbon emissions. The improvement of carbon productivity plays an important role in achieving the goal of carbon neutrality in the world. The research on carbon productivity mainly focuses on three aspects. 1) The first kind of literature focuses on the dynamic change trend of carbon productivity from the national, provincial, and industrial levels. The research shows that with the high-quality development of economy, carbon productivity shows an upward trend and heterogeneity (Zhou et al., 2010; Yu et al., 2017a; Qiu et al., 2017; Li et al., 2018; Bai et al., 2019; Yang et al., 2020a; Du et al., 2022). 2) The second kind of literature studies the influencing factors of carbon productivity. Most studies believe that technological progress, industrial scale, industrial structure, energy efficiency, and environmental regulation are important factors affecting carbon productivity (Lu et al., 2014; Wang et al., 2016; Yu et al., 2017b; Du and Li, 2019; Hu and Wang, 2020; Liu and Zhang, 2021b; Zhou and Tang, 2021; Lin and Jia, 2022). 3) The third kind of literature focuses on the convergence and divergence of carbon productivity, but there is no consensus on the convergence and divergence of carbon productivity. Shen et al. (2021) measured China’s carbon productivity by combining the directional distance function and meta-constrained production function based on the extended relaxation measure (SBM) model. The results show that China’s carbon productivity has spatial condition β convergence.
The literature on the impact of fiscal decentralization on environmental governance can be divided into the following three types: 1) A higher degree of fiscal decentralization is more conducive to pollution control and carbon dioxide emission reduction. Fiscal decentralization can not only help local governments improve the efficiency of resource allocation but also improve regional environmental quality by promoting local governments to improve environmental standards (Millimet, 2003; Konisky, 2007; Rui, 2018; Yang et al., 2020b; Yacouba, 2022). 2) Many scholars believe that fiscal decentralization urges local governments to create more development space for economic development by weakening local environmental regulations, which leads to the increase of carbon dioxide emissions and is not conducive to the improvement of environmental quality (Konisky and Woods, 2015; Zhang et al., 2016; Zhang et al., 2017a; Zhang et al., 2018; Shan et al., 2021). 3) The impact of fiscal decentralization is different in different stages. Lopez et al. (2011) believed that increasing the proportion of social welfare and public goods expenditure in government expenditure will reduce pollutant emissions. However, without changing the expenditure structure, increasing the total amount of government expenditure will not reduce pollutant emissions.
By summarizing the literature on carbon productivity and fiscal decentralization, it is found that many scholars have carried out various studies on fiscal decentralization and carbon productivity from different angles, but few studies pay attention to the impact of fiscal decentralization on carbon productivity. The innovation of this study is to use the data of fiscal decentralization and carbon productivity of 30 provinces in China from 2010 to 2019 to make an empirical analysis on the impact of fiscal decentralization on carbon productivity by considering the horizontal dimension of space and the vertical dimension of time through spatial measurement methods, which broadens the perspective of fiscal decentralization and carbon productivity research. The marginal contributions of this study are as follows: first, most of the literature focuses on the impact of fiscal decentralization on carbon emissions or the environment, ignoring the impact of fiscal decentralization on carbon productivity, while this study explores the impact of fiscal decentralization on carbon productivity, which is helpful in clarifying the role path and internal mechanism of fiscal decentralization in reducing carbon emissions and promoting green economic growth. Second, corresponding to fiscal revenue and expenditure, revenue decentralization and expenditure decentralization are formed, respectively. Most literatures often choose one of them as the research object, and the analysis of the impact of the two decentralization methods on the environment is not comprehensive. Therefore, based on the dual perspective of revenue and expenditure, the two decentralization methods are introduced into the spatial econometric model at the same time, so as to better analyze the heterogeneity of the spatial effect of fiscal revenue decentralization and fiscal expenditure decentralization on carbon productivity, which has theoretical value and practical significance for realizing green development. Therefore, this study can provide more specific and targeted policy suggestions for reducing carbon emissions and realizing carbon neutralization.
The structure of the article is as follows: the second part is literature review. The third part is the model and data, which measures the impact of fiscal decentralization on carbon productivity through spatial econometric methods. The fourth part is the discussion of empirical results. The last part is policy suggestions, which puts forward policy suggestions for fiscal decentralization to improve carbon productivity.
3 Models and Data
3.1 Spatial Spillover Effect Test
This study uses the spatial correlation index of ESDA (Exploratory Spatial Data Analysis) to test the spatial spillover effect of carbon productivity among provinces, which is usually measured by Moran’s I and Geary’s C index:
where
This study constructs the following three kinds of spatial weight matrix.
The first is based on the Queen adjacency rule, and the geographical neighbor weight matrix is constructed by using map boundary vector data:
The second weight matrix constructed in this study is the economic distance weight matrix based on the reciprocal of the absolute value of economic gap.
Considering the limitations of the abovementioned single-geographical or economic weight matrix, the third kind of comprehensive weight matrix of geographical economy is constructed based on the ratio of GDP of each neighboring province in the total GDP of all neighboring provinces:
Based on the abovementioned three spatial weight matrices, the results of the global spatial correlation test of China’s province carbon productivity are shown in Table 1. It can be seen that the Moran’s I index of provincial carbon productivity is greater than 0 and the Geary’s C index is less than 1 under the geographical neighbor weight matrix and the comprehensive weight matrix of geographical economy, both of which are significant at the level of 1%, indicating that the distribution of provincial carbon productivity in China’s cities presents the spatial positive correlation distribution characteristics of “high–high” and “low–low” agglomeration. But at the same time, it can be found that under the weight matrix of pure economic distance, the Moran’s I and Geary’s C indices of provincial carbon productivity are not significant, indicating that the spatial correlation of provincial carbon productivity is mainly reflected in the geographical spatial correlation and the comprehensive spatial correlation characteristics of geography and economy but not in the economic development difference. Therefore, the subsequent spatial econometric analysis is only based on the geographical neighbor weight matrix and the comprehensive weight matrix of geographical economy for consideration.
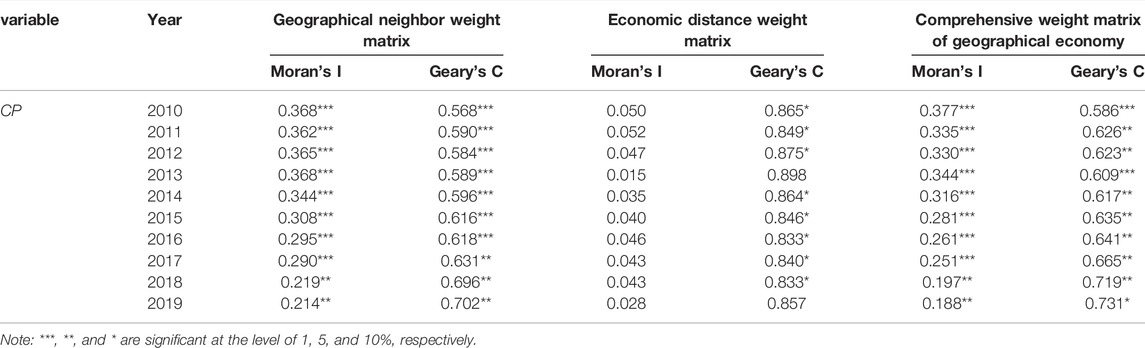
TABLE 1. Moran’s I and Geary’s C statistical indicators of China’s provincial carbon productivity under three weight matrices.
3.2 Measurement Model
Because the ordinary panel model cannot describe the spatial interaction relationship of economic variables among different regions, combined with the abovementioned analysis, the spatial econometric model is introduced when discussing the effect of fiscal decentralization on carbon productivity. Taking carbon productivity as the explained variable and fiscal revenue decentralization and fiscal expenditure decentralization as the explanatory variables, this study discusses the impact of fiscal decentralization on carbon productivity. Because different spatial models express different practical economic meanings, OLS (Ordinary Least Squares), SAR (Spatial Autoregressive Model), SEM (Spatial Error Model), and SAC (Spatial Autocorrelation Model) are used as specific model applications to estimate the specific effects of fiscal revenue decentralization and fiscal expenditure decentralization on carbon productivity. The specific models are as follows:
Equation 9 is the spatial autocorrelation model SAC, and Eqs 6–8 can be regarded as the OLS model, SAR model, and SEM model obtained after setting some constraints on the SAC model, respectively. When the SAC model does not consider the influence of the lag term of the explained variable on itself, that is, the coefficient of the spatial lag term of the model ρ = 0, the SEM model of spatial error can be obtained, as shown in formula (8). When the SAC model excludes the influence of the disturbance term, that is, the coefficient of spatial error term λ = 0, the SAR model is obtained, as shown in Eq. 7. As the OLS model is a nonspatial econometric model and does not consider the spatial correlation among different provinces, it can be regarded as the result of excluding the influence of spatial lag term and spatial error term in the SAC model, as shown in formula (6). In Eqs 6–9, cp is the explained variable, cp is carbon productivity, W is the geographic distance weight matrix or geographic economic comprehensive weight matrix, X is the control variable, μ and ε are the perturbation terms subject to independent identical distribution.
3.3 Index Selection and Statistical Description
From the perspective of data availability, this study selects 30 provinces in China (except Tibet, Hong Kong, Macao, Taiwan, etc.) from 2010 to 2019 as the research object. After combining a large number of previous literatures, it is found that scholars in various fields have conducted extensive and in-depth discussions on the influencing factors of carbon productivity. Referring to previous studies, this study selects carbon productivity as the explained variable, fiscal decentralization as the explanatory variable, and digital economy, urbanization rate, industrial structure, foreign direct investment level, and technological innovation as the control variables to study the spatial heterogeneity of the impact of provincial fiscal decentralization on carbon productivity. The statistical description of variables is shown in Tables 2–4.
Carbon Productivity
Carbon productivity refers to the level of GDP output per unit of carbon dioxide. First, carbon emissions are calculated, and the formula is as follows:
where
Development Level of Digital Economy
Defining the connotation of digital economy and combined with the availability of data, this study decomposes the digital economy index from three dimensions of information development, internet development, and digital transaction development and selects the following 13 measurement indicators to design the digital economy index measurement body as shown in Table 3. The basic data of the measurement indicators are all derived from China Statistical Yearbook from 2010 to 2020.
In this article, the linear weighed method is used to calculate the development level of digital economy:
where
Referring to the information level index (ILI) established by Zhang et al. (2017b) and the network preparation index (NBI) constructed by Harvard University and The World Economic Forum, in order to make the digital economic index comparable across the years, this study sets the measurement index based on 2015 and standardizes the measurement indicators. At the same time, in order to make the index of each province comparable between different statistical years, the formula in the article is as follows:
where
In order to test the value of the degree of multicollinearity between the observed values of independent variables, this study tests the collinearity of variables. The test results are shown in Table 5. The maximum variance expansion factors are 6.48, 7.42, and less than 10, and the average variance expansion factors are 3.79, 3.64, and less than 5. It shows that there is no multicollinearity between the selected variables, and the selection of variables is reasonable.
4 The Discussion of Empirical Results
4.1 Empirical Results
Through the Hausman test of panel data, the Hausman statistic is significantly positive, so this study selects the fixed-effect model. The spatial econometric model includes spatial fixed, temporal fixed, and spatiotemporal double-fixed effects. Therefore, the fixed-effect forms of the SAR, SEM, and SAC models are tested according to the likelihood ratio (LR) test and natural logarithm (Log-L) test, and the model is set in combination with the data structure. The regression results are shown in Tables 6 and 7. It can be seen from the results that under the SAR, SEM, and SAC models, the spatial term coefficients ρ and λ show a high significance, indicating that there is, indeed, a high spatial correlation among carbon productivity in various regions of China. Considering the spatial effect, the regression coefficients of fiscal revenue decentralization and fiscal expenditure decentralization in the model are significantly positive, and their impact on carbon productivity is significantly positive at the level of 5%, which indicates that fiscal decentralization has a positive correlation with carbon productivity, and appropriately improving fiscal decentralization is conducive to improving carbon productivity. In the spatial econometric model, the coefficient of fiscal revenue decentralization of 13.9627 is less than that of fiscal expenditure decentralization of 16.8367. The estimation results of the ordinary OLS model also have similar characteristics. This means that fiscal revenue decentralization and fiscal expenditure decentralization can not only improve carbon productivity but also have heterogeneity in their respective marginal effects. Fiscal expenditure decentralization plays a greater role in improving carbon productivity than fiscal revenue decentralization. From the estimation results of the model, compared with the SAR, SEM, and ordinary panel data OLS models, the SAC model has the most significant characteristics of spatial effect, and the SAC model includes two spatial transmission mechanism assumptions of autocorrelation and random disturbance, which cannot be ignored for analyzing the spatial effect of fiscal revenue decentralization and fiscal expenditure decentralization on carbon productivity. In order to further verify the rationality of the SAC model, the LR test is carried out on the SAC model, and the results show that the statistic of χ2 of p value is 0, that is, the original assumption that the spatial SAR and SEM models replace the SAC model is rejected. Therefore, this study selects the SAC model for empirical research. From the analysis of other variables, the improvement of the development level of digital economy and technological innovation level is conducive to the improvement of carbon productivity. This is because digital economy can promote technological progress, optimize resource allocation, and improve energy efficiency through technological effect. The industrial structure inhibits the improvement of carbon productivity because the secondary industry is mostly high-carbon industries. With the increasing proportion of the secondary industry, carbon emissions will keep rising, resulting in the decline of carbon productivity. The impact of foreign direct investment and urbanization rate on carbon productivity is not significant, which may be because they do not provide sufficient driving force for low-carbon development and do not produce significant pollution reduction or increase effects.
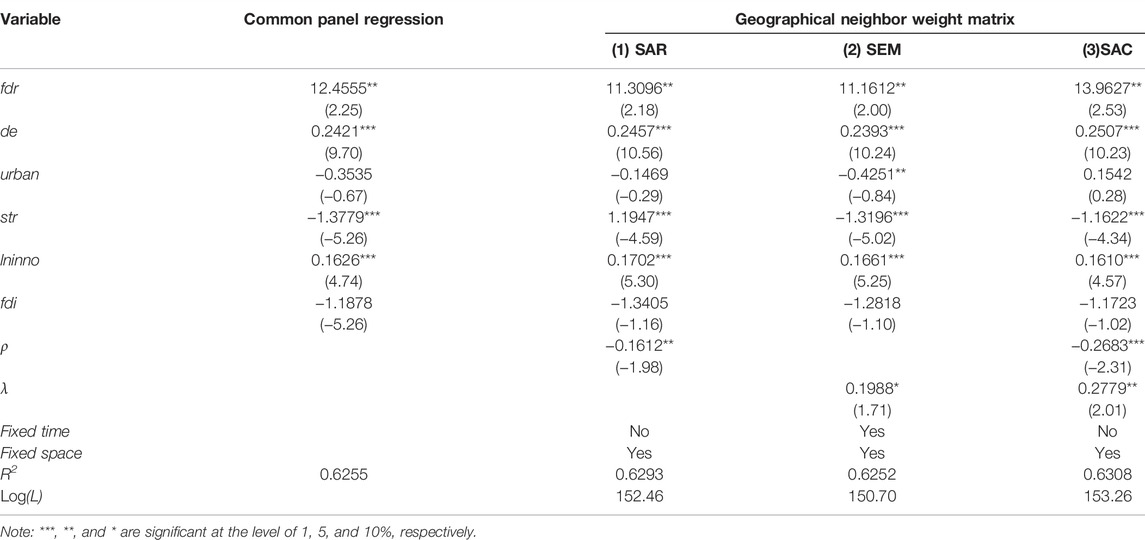
TABLE 6. Regression results of fiscal revenue decentralization spatial panel data under geographical neighbor weight matrix.
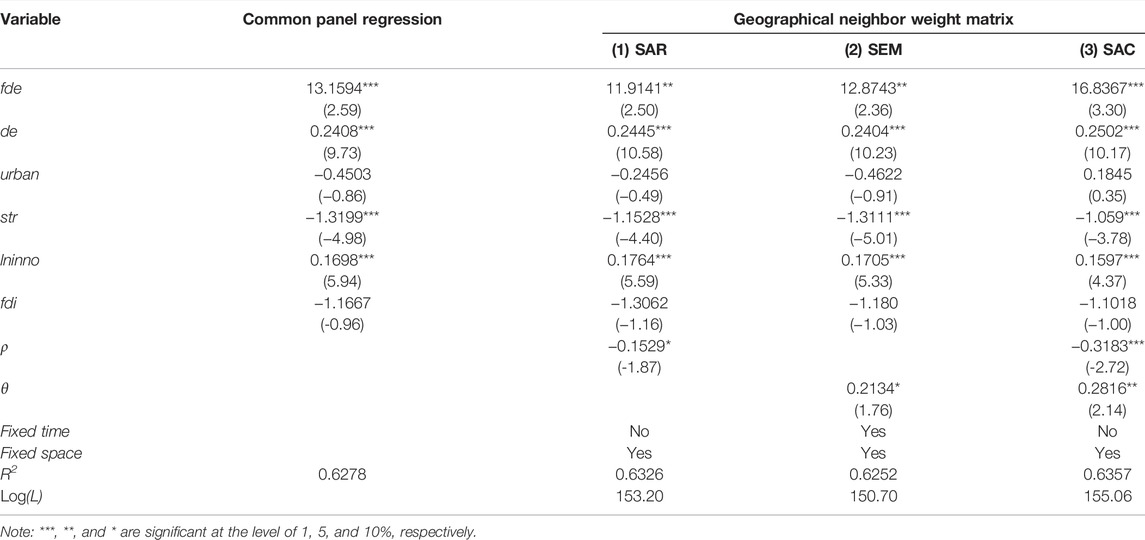
TABLE 7. Regression results of fiscal expenditure decentralization spatial panel data under geographical neighbor weight matrix.
4.2 Sub Effect Test
In order to further reveal the extent of the spatial spillover effect of explanatory variables on the explained variables, the direct effect, indirect effect, and the total effect of fiscal revenue decentralization and fiscal expenditure decentralization on carbon productivity are calculated through effect decomposition. The direct effect is used to describe the average impact of fiscal decentralization on the carbon productivity of the region. The indirect effect, that is, spatial spillover effect reveals the average impact of fiscal decentralization on the carbon productivity of other provinces. The total effect reflects the average impact of the explanatory variable fiscal decentralization on all regions. As the empirical results shown in Table 8, the results show that the two decentralization models have a significant direct effect on carbon productivity, but they have a significant inhibitory effect on carbon productivity indirectly through spatial spillover effect. Judging by p value, the direct effect, indirect effect, and total effect are highly significant. From the perspective of fiscal revenue decentralization: the direct effect of fiscal revenue decentralization on its own carbon productivity is significantly positive, and the direct effect coefficient is 14.4292, indicating that improving the degree of fiscal revenue decentralization of local governments is conducive to the improvement of carbon productivity in all provinces and plays a positive role in the reduction of carbon emissions and the improvement of carbon intensity. The possible reason is that under China’s decentralization system, on the one hand, with the increasing proportion of fiscal revenue, the government pays more and more attention to the development of local low-carbon economy and promotes the formation of environment-friendly behaviors and industries through good tax design and inductive tax burden tilt mechanism, thus inhibiting the emergence of high-carbon industries. On the other hand, the improvement of fiscal revenue decentralization expands the autonomy of local fiscal revenue. Local governments can formulate industrial policies with comparative advantages and focus on realizing green economic development so as to increase the intensity of environmental governance, improve regional environmental standards, and reduce pollution emissions. Fiscal revenue decentralization has a significant negative indirect spatial spillover effect on carbon productivity, with an impact degree of −3.1500, indicating that the improvement of fiscal revenue decentralization of the local government has an inhibitory effect on the improvement of carbon productivity in other provinces. The possible reason is that various regions have produced the phenomenon of “free riding” under the incentives and constraints of new development concepts and ecological protection policies.
From the perspective of fiscal expenditure decentralization: fiscal expenditure decentralization in this region has a significant positive direct effect on local carbon productivity, with an impact degree of 17.4471, indicating that the improvement of fiscal expenditure decentralization in each province has promoted carbon productivity in this province. The main reason may be that the central government continuously improves the weight of indicators such as ecological and environmental protection in the assessment mechanism, which makes local governments continuously increase the expenditure level of environmental protection and governance. Therefore, the government continuously improves environmental standards and increases governance investment and directly adjusts the distribution relationship of economic benefits to promote the development of green industry and green science and technology. The spatial spillover effect of fiscal expenditure decentralization is significantly negative, and the impact degree is −4.3798, indicating that the decentralization of fiscal expenditure in this province reduces the carbon productivity of neighboring provinces. The possible reason is that the improvement of fiscal expenditure decentralization has raised local environmental standards and increased the cost of polluting enterprises in the province, which makes local polluting enterprises migrate to neighboring provinces or other regions. However, from the perspective of the overall effect, fiscal expenditure decentralization has a positive correlation with carbon productivity, indicating that the improvement of fiscal expenditure decentralization is generally conducive to solving the prominent problems of ecological environment caused by carbon emissions and realizing green development.
4.3 Robustness Test
Robustness test under different matrix selection: based on the spatial and geographical distance, the spatial weight matrix is constructed in the research, and mainly, maximum likelihood estimation is carried out. In order to further verify the robustness of the estimation results of the model, a comprehensive weight matrix of geographical economy is constructed in each urban geographical neighboring province based on the ratio of the GDP of each neighboring province to the total GDP of all neighboring provinces, and the SAC model is estimated again to check whether the results are stable. The test results are reported in Table 9. It can be seen that after selecting the new spatial weight matrix for model estimation, the regression results show that the regression coefficient of the SAC model is still significant. The spatial term coefficients ρ and λ show a high significance, which show that it is appropriate to use the SAC model to empirically test the spatial effect of fiscal revenue decentralization and fiscal expenditure decentralization on carbon productivity. Furthermore, through the results of spatial effect decomposition, it can be seen that the estimation results of direct effect, indirect effect, and total effect of fiscal revenue decentralization and fiscal expenditure decentralization on carbon productivity are significant, and the directions of the three effects are still consistent with those of the previous conclusions. Therefore, the research results are robust and reliable.
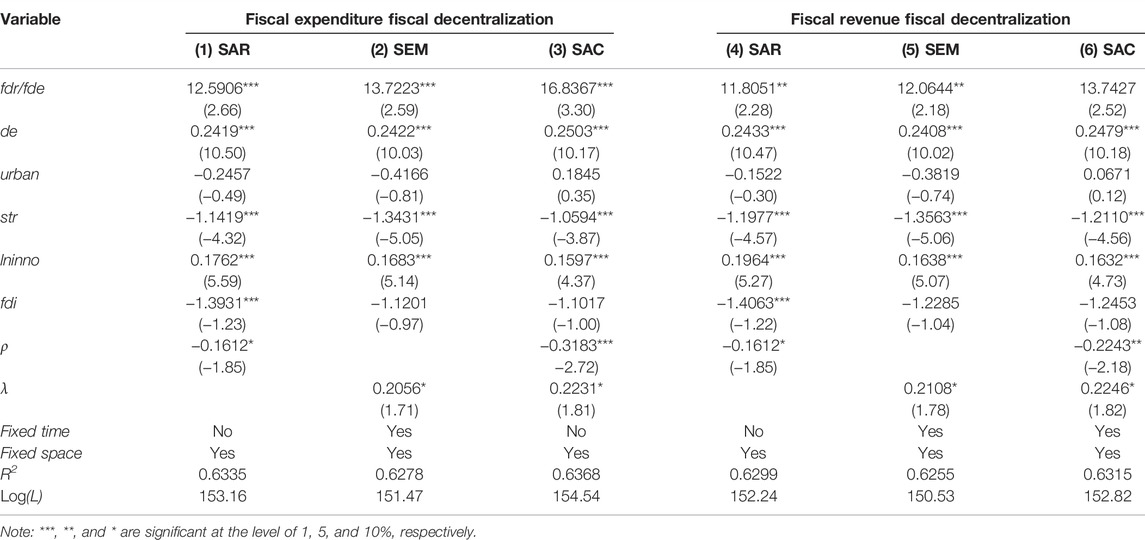
TABLE 9. Regression results of fiscal decentralization spatial panel data under comprehensive weight matrix of geographical economy.
5 Conclusions and Recommendations
Using the data of fiscal decentralization and carbon productivity of 30 provinces in China from 2010 to 2019, this study empirically tests the impact of fiscal decentralization and carbon productivity. The empirical results show that 1) the spatial agglomeration effect of China’s provincial carbon productivity is obvious, which shows an upward trend. The heterogeneity of carbon productivity among different provinces is obvious. The overall performance is as follows: Eastern provinces > Central provinces > Western provinces. 2) Whether based on the geographical proximity weight matrix or the geographical comprehensive weight matrix, the development of fiscal decentralization has significantly improved China’s carbon productivity, and its impact on carbon productivity is significantly positive below 10%. The promotion effect of fiscal expenditure decentralization on carbon productivity is greater than that of fiscal revenue decentralization. 3) Fiscal revenue decentralization and fiscal expenditure decentralization have significant positive direct effects and negative spatial spillover effects on the improvement of carbon productivity. Improving fiscal decentralization is conducive to the improvement of carbon productivity in this province, but it will inhibit the carbon productivity of adjacent provinces. 4) For other control variables, technological innovation and digital economy can significantly promote the improvement of carbon productivity, and the industrial structure significantly inhibits the improvement of carbon productivity.
Fiscal decentralization plays an irreplaceable role in improving carbon productivity and realizing low-carbon development. Based on the abovementioned conclusions, this study puts forward the following policy suggestions: first, expanding the autonomy of fiscal decentralization appropriately. Based on the current performance appraisal system, the central government should expand the financial autonomy of local governments and provide local governments more “financial power.” When the financial autonomy increases, local governments will actively increase green R&D investment while pursuing GDP growth under the pressure of the “dual carbon” goal so as to curb the generation of environmental adverse behaviors or industries and then drive the development of green technology and industry so as to promote the improvement of carbon productivity. Second: establishing the function mechanism of green fiscal revenue and expenditure. The main body of green fiscal revenue is green tax. Strengthening the scientific and feasible tax collection, promoting the formation of environment-friendly behavior and industry through good tax design and inductive tax burden tilt mechanism, and strengthening the cultivation of green environmental protection industry; the government’s purchasing expenditure has an important impact on green consumption and green production. The scale of government purchasing expenditure will promote the production of green products and the progress of green technology. Third: strengthening regional cooperation and mobilizing the enthusiasm for developing low-carbon economy. In order to control the negative impact of the spatial spillover effect of fiscal decentralization on carbon productivity in other regions, formulating the development strategy of low-carbon economy, each region should not only fully consider its own resource advantage endowment but also combine the endowment of adjacent provinces. Formulating targeted policies suitable for the region and promoting the development of low-carbon economy in adjacent regions, reasonably guiding the flow of resources among regions, and realizing the stability of low-carbon economic development in the region is a part of the process.
Data Availability Statement
The original contributions presented in the study are included in the article/Supplementary Material, further inquiries can be directed to the corresponding authors.
Author Contributions
GF calculated the main data of carbon productivity and built the model and was a major contributor in writing the manuscript. XS contributed to the conception of the study and revised the article. SR checked the data and was responsible for the suggestions of the article. All authors read and approved the final manuscript.
Conflict of Interest
The authors declare that the research was conducted in the absence of any commercial or financial relationships that could be construed as a potential conflict of interest.
Publisher’s Note
All claims expressed in this article are solely those of the authors and do not necessarily represent those of their affiliated organizations, or those of the publisher, the editors, and the reviewers. Any product that may be evaluated in this article, or claim that may be made by its manufacturer, is not guaranteed or endorsed by the publisher.
References
Bai, C., Du, K., Yu, Y., and Feng, C. (2019). Understanding the Trend of Total Factor Carbon Productivity in the World: Insights from Convergence Analysis. Energ. Econ. 81 (6), 698–708. doi:10.1016/j.eneco.2019.05.004
Bauer, N., Mouratiadou, I., Luderer, G., and Baumstark, L. (2013). Global Fossil Energy Markets and Climate Change Mitigation—An Analysis with REMIND[J]. Climatic Change 136, 69–82. doi:10.1007/s10584-013-0901-6
Chen, Z., Zhou, C., Zhao, Y., and Zhong, Z. (2022). Does Political Resource Endowment Promote Carbon Productivity? A Case Study of Counties along the Long March Areas in China. Environ. Impact Assess. Rev. 93, 106739. doi:10.1016/j.eiar.2022.106739
Du, K., and Li, J. (2019). Towards a green World: How Do green Technology Innovations Affect Total-Factor Carbon Productivity[J]. Energy Policy 131, 240–250. doi:10.1016/j.enpol.2019.04.033
Du, M., He, L., Zhao, M., and Li, J. (2022). Examining the Relations of Income Inequality and Carbon Productivity: A Panel Data Analysis[J]. Sustainable Prod. Consumption 31, 249–262. doi:10.1016/j.spc.2022.01.027
Hu, W., and Wang, D. (2020). How Does Environmental Regulation Influence China's Carbon Productivity? an Empirical Analysis Based on the Spatial Spillover Effect[J]. J. Clean. Prod. 257, 120484. doi:10.1016/j.jclepro.2020.120484
Konisky, D. M. (2007). Regulatory Competition and Environmental Enforcement: Is There a Race to the Bottom?[J]. Am. J. Polit. Sci. 51 (4), 853–872. doi:10.1111/j.1540-5907.2007.00285.x
Konisky, D. M., and Woods, N. D. (2015). Environmental Free Riding in State Water Pollution Enforcement[J]. Social Sci. Electron. Publishing 12 (3), 227–251. doi:10.1177/1532440012438891
Kuai, P., Yang, S., Tao, A., Zhang, S. a., and Khan, Z. D. (2019). Environmental Effects of Chinese-style Fiscal Decentralization and the Sustainability Implications. J. Clean. Prod. 239, 118089. doi:10.1016/j.jclepro.2019.118089
Li, W., Wang, W., Wang, Y., and Ali, M. (2018). Historical Growth in Total Factor Carbon Productivity of the Chinese Industry - A Comprehensive Analysis. J. Clean. Prod. 170, 471–485. doi:10.1016/j.jclepro.2017.09.145
Lin, B., and Li, Z. (2022). Towards World's Low Carbon Development: The Role of Clean Energy. Appl. Energ. 307, 118160. doi:10.1016/j.apenergy.2021.118160
Lin, B. Q., and Jia, H. Y. (2022). Does the Development of China's High-Speed Rail Improve the Total-Factor Carbon Productivity of Cities? [J]. Transportation Res. D: Transport Environ. 105, 103230. doi:10.1016/j.trd.2022.103230
Liu, C., and Feng, Y. (2011). Low-carbon Economy: Theoretical Study and Development Path Choice in China. Energ. Proced. 5 (1), 487–493. doi:10.1016/j.egypro.2011.03.085
Liu, X. P., and Zhang, X. L. (2021). Industrial Agglomeration, Technological Innovation and Carbon Productivity: Evidence from China[J]. Resour. Conservation Recycling 166, 105330. doi:10.1016/j.resconrec.2020.105330
Liu, X., and Zhang, X. (2021). Industrial Agglomeration, Technological Innovation and Carbon Productivity: Evidence from China. Resour. Conservation Recycling 166, 105330. doi:10.1016/j.resconrec.2020.105330
Lopez, R., Galinato, G., and Islam, A. (2011). Fiscal Spending and the Environment: Theory and Empirics[J]. J. Environ. Econ. Manag. 62 (2), 180–198. doi:10.1016/j.jeem.2011.03.001
Lu, Z. G., Yang, Y., and Wang, J. (2014). Factor Decomposition of Carbon Productivity Change in China's Main Industries: Based on the Laspeyres Decomposition Method[J]. Energ. Proced. 61, 1893–1896. 10.10161.egypt.2014.12236
Millimet, D. L. (2003). Assessing the Empirical Impact of Environmental Federalism[J]. J. Reg. Sci. 43 (4), 711–733. doi:10.1111/j.0022-4146.2003.00317.x
Qiu, X., Fang, Y., Yang, X., and Zhu, F. (2017). Tourism Eco-Efficiency Measurement, Characteristics, and its Influence Factors in China. Sustainability 9 (9), 1634. doi:10.3390/su9091634
Rabia, A., Muhammad, U., Gan, X. L., and Fuzhong, C. (2022). Dynamic Linkages between Energy Efficiency, Renewable Energy along with Economic Growth and Carbon Emission. A Case of MINT Countries an Asymmetric Analysis [J]. Energ. Rep. 8, 2119–2130. doi:10.1016/j.egyr.2022.01.153
Robertson, S. (2016). A Longitudinal Quantitative–Qualitative Systems Approach to the Study of Transitions toward a Low Carbon Society[J]. J. Clean. Prod. 128, 221–233. doi:10.1016/j.jclepro.2015.04.074
Rui, M. (2018). Bounded Rationality in the Developmental Trajectory of Environmental Target Policy in China, 1972-2016[J]. Sustainability 10 (1), 199. doi:10.3390/su10010199
Sengupta, P., Choudhury, B. K., Mitra, S., and Agrawal, K. M. (2020). Low Carbon Economy for Sustainable Development. Encyclopedia Renew. Sust. Mater. 3, 551–560. doi:10.1016/b978-0-12-803581-8.11217-2
Shan, S., Munir, A., Tan, Z. X., and Adebayo, T. S. (2021). The Role of Energy Prices and Non-linear Fiscal Decentralization in Limiting Carbon Emissions: Tracking Environmental Sustainability[J]. Energy 234, 121243. doi:10.1016/j.energy.2021.121243
Shen, N., Peng, H., and Wang, Q. W. (2021). Spatial Dependence, Agglomeration Externalities and the Convergence of Carbon Productivity[J]. Socio-Economic Plann. Sci. 78, 101060. doi:10.1016/j.seps.2021.101060
Shen, W. (2017). Who Drives China's Renewable Energy Policies? Understanding the Role of Industrial Corporations. Environ. Dev. 21 (21), 87–97. doi:10.1016/j.envdev.2016.10.006
Wang, F., He, J., and Niu, Y. (2022). Role of Foreign Direct Investment and Fiscal Decentralization on Urban Haze Pollution in China. J. Environ. Manage. 305, 114287. doi:10.1016/j.jenvman.2021.114287
Wang, K., Xian, Y., Wei, Y., and Huang, Z. (2016). Sources of Carbon Productivity Change: A Decomposition and Disaggregation Analysis Based on Global Luenberger Productivity Indicator and Endogenous Directional Distance Function[J]. Ecol. Indicators 66, 545–555. doi:10.1016/j.ecolind.2016.02.034
Wu, W., Zhang, T., Xie, X., and Huang, Z. (2021). Regional Low Carbon Development Pathways for the Yangtze River Delta Region in China. Energy Policy 151, 112172. doi:10.1016/j.enpol.2021.112172
Yacouba, K. (2022). Fiscal Decentralization and Public Budgets for Energy RD&D: A Race to the bottom?[J]. Energy Policy 161, 112761. doi:10.1016/j.enpol.2021.112761
Yang, H., Lu, Z., Shi, X., and Mensah, I. (2020). Multi-region and Multi-Sector Comparisons and Analysis of Industrial Carbon Productivity in China[J]. J. Clean. Prod. 279, 123623. doi:10.1016/j.jclepro.2020.123623
Yang, Y., Tang, D., and Zhang, P. (2020). Effects of Fiscal Decentralization on Carbon Emissions in China[J]. Int. J. Energ. Sector Manag. 14 (10), 213–228. doi:10.1108/ijesm-03-2019-0001
Yu, Y. N., Choi, Y., Wei, X., and Chen, Z. (2017). Did China's Regional Transport Industry Enjoy Better Carbon Productivity under Regulations? [J]. J. Clean. Prod. 165, 777–787. doi:10.1016/j.jclepro.2017.07.105
Yu, Y., Qian, T., and Du, L. (2017). Carbon Productivity Growth, Technological Innovation, and Technology gap Change of Coal-Fired Power Plants in China. Energy Policy 109, 479–487. doi:10.1016/j.enpol.2017.05.040
Zhang, B., Chen, X., and Guo, H. (2018). Does central Supervision Enhance Local Environmental Enforcement? Quasi-Experimental Evidence from China[J]. J. Public Econ. 164, 70–90. doi:10.1016/j.jpubeco.2018.05.009
Zhang, B., Peng, Z. D., and Zhao, L. (2017). International Comparative Study on Information Development in China [J]. Inf. Syst. Eng. 6, 122–125.
Zhang, J., Zeng, W., Wang, J., and Yang, F. (2015). Regional Low-Carbon Economy Efficiency in China: Analysis Based on the Super-SBM Model with CO2 Emissions[J]. J. Clean. Prod. 163, 202–211. doi:10.1016/j.jclepro.2015.06.111
Zhang, K., Wang, D. F., and Zhou, H. Y. (2016). Regional Endogenetic Strategic Interaction of Environmental protection Investment and Emission[J]. China Ind. Econ. 2, 68–82.
Zhang, K., Zhang, Z. Y., and Liang, Q. M. (2017). An Empirical Analysis of the green Paradox in China: From the Perspective of Fiscal Decentralization[J]. Energy Policy 103, 203–211. doi:10.1016/j.enpol.2017.01.023
Zhou, L., and Tang, L. Z. (2021). Environmental Regulation and the Growth of the Total-Factor Carbon Productivity of China's Industries: Evidence from the Implementation of Action Plan of Air Pollution Prevention and Control[J]. J. Environ. Manage. 296, 113078. doi:10.1016/j.jenvman.2021.113078
Keywords: fiscal revenue decentralization, fiscal expenditure decentralization, carbon productivity, spatial effect, low-carbon development
Citation: Feng G, Shulian X and Renjin S (2022) Does Fiscal Decentralization Promote or Inhibit the Improvement of Carbon Productivity? Empirical Analysis Based on China’s Data. Front. Environ. Sci. 10:903434. doi: 10.3389/fenvs.2022.903434
Received: 24 March 2022; Accepted: 08 April 2022;
Published: 12 May 2022.
Edited by:
Fuzhong Chen, University of International Business and Economics, ChinaReviewed by:
Peng Gao, Southeast University, ChinaYayun Ren, Guizhou University of Finance and Economics, China
Copyright © 2022 Feng, Shulian and Renjin. This is an open-access article distributed under the terms of the Creative Commons Attribution License (CC BY). The use, distribution or reproduction in other forums is permitted, provided the original author(s) and the copyright owner(s) are credited and that the original publication in this journal is cited, in accordance with accepted academic practice. No use, distribution or reproduction is permitted which does not comply with these terms.
*Correspondence: Xue Shulian, ODYyNTc0MjFAMTYzLmNvbQ==