- Department of Civil and Resource Engineering, Faculty of Engineering, Dalhousie University, Halifax, NS, Canada
In the event of a marine oil spill and its subsequent response operations, different types of oily wastes are generated in large quantities, and their management is a significant challenge that oil spill responders face. The goal of this study is to develop a comprehensive pattern recognition modeling framework for deriving and grouping a set of unique clusters that separate different types of oily wastes from each other. The main idea is to group oily wastes based on their unique characteristics, such as the percentage of oil, percentage of water, percentage of mineral matter, and percentage of organic matter. Each cluster has a relatively homogeneous pattern of pollution characteristics. Prior to implementing the cluster analysis technique, it is important to evaluate and transform the raw oily waste data using well-defined criteria. An advanced machine learning technique, fuzzy C-means clustering algorithm, is employed to classify the oily wastes. The Kolmogorov–Smirnov tests are employed to examine the statistical significance of clustered data. Results show a heterogeneous diversity in seven identified clusters in relation to different types of oily wastes. The cluster-based analysis method presented in this article is an integral part of an integrated optimization-based model which will provide valuable inputs for adjustment of the existing management practices, enhancement of short-term pollution control strategies, and development of long-term oily waste management policies. The output of this study would provide a better tool to waste characterization and sorting steps that are required to immediately separate recovered waste to support downstream response efforts. This result of this study also supports the overall goal of minimizing impact on the environment by ensuring the maximum amount of recovered waste can be recycled or disposed in an environmentally friendly fashion. Moreover, properly classified, sorted, and labeled waste will greatly help with downstream steps of packaging, transportation, and tracking of waste, and as a result, it will minimize total waste management time and costs, under the constraints involving waste storage and transport capacities, waste pre-treatment and treatment facility capacities, and environmental regulatory compliance, as well as other operational and logistic constraints.
Introduction
When an oil spill occurs, the first action is to state the detected oil spill incident to the coast guard. In addition, spilled oil type and amount, spill location, weather conditions including wind and wave, currents and tides, air and water temperature, and the presence of ice, rain, or snow need to be recorded and observed. Subsequently, the coast guard stimulates the response team and activates response resources. Next, the coast guard takes the lead and works with the polluter, spill response organizations, and contractors to put together a suitable response system. The end of coast guard involvement in the response system is when spilled oil clean-up is accomplished. In the last step, a debriefing report will be prepared to summarize process specifics, reimbursement claims, lessons learned, and monitoring of the impacted areas.
After an oil spill event and through the clean-up process, a great amount of oily waste can be generated (REMPEC, 2010; REMPEC, 2011). Various oil spills could be produced including oil, weathered and/or emulsified oil, oiled material, oiled sediment, and oiled equipment (National Research Council, 2003). Several documents and strategies have been carried out by governments and other organizations to offer the responders policies for oily waste management (CEDRE, 1978; REMPEC, 2012; RRT/NWAC, 2020; European Maritime Safety Agency, 2018; CEDRE, 2016; ITOPF, 2014; Owens et al., 2009). These regulations and guidelines offer for the setting up of waste treatment systems in ports or offshore places; however, there are still complications that avoid the effective on-shore delivery of this waste (Lin et al., 2007; Silva et al., 2012; International Maritime Organization 2018).
The key struggle in planning oil spill waste management is to frame a waste management plan for an unforeseen nature and volume of oil spill waste, enchanting into account the possible classes of waste, the current services, and the treatment measures required. Reference must also be made to legal, financial, environmental, operational, and logistics issues (Aamo et al., 1997). Type of oil spill waste, type of treatment possible, facilities, and treatment plant need to be taken into account when considering waste management. Overall, waste management is a complex problem as relevant environmental, economic, and social features and factors have to be measured broadly in one framework (Beegle-Krause, 2001; Jordão da Silva, 2012; Ben Fieldhouse, 2018).
The process stages for the oily waste management mostly contain waste collection, waste segregation or sorting, temporary storage, transportation, intermediate storage, treatment, and disposal (REMPEC, 2010; IPIECA, 2014). Clearance of oil and oily waste is an important issue, mostly coastline clean-up when there is probable to be a great quantity of associated debris. Henceforth, contingency planning should reflect and design waste disposal strategies (Atlas and Hazen 2011). Even though different procedures have been recognized and hired to deal with oil and oily wastes, however, many of them have restricted applications and capacities. Consequently, in the incidence of a main spill how the approaches will be utilized needs to be considered and laid out.
Decisions on waste treatments are best made at the beginning of the incident on the basis of realistic expectations of the classes and the amount of waste likely to be twisted. The probable waste recovery or clearance paths should comprise local waste regulation and proper authorities. The convenience of temporary storage should be pre-identified in areas of high spill risk. If the disposal paths exist for various waste streams, waste should be separated from the point of collection. The opportunity of refining operational oil should be examined before disposal. Measures that lead to the destruction of the oil are required to landfill, though they are likely to be more costly.
Through an emergency response, the quick operation has to overcome the hurdle of preparing operational computer time. Due to these restrictions, operational centers regularly implement and preserve nested estimates in areas of anticipated activity and high risk of potential oil spill accidents, which provide the first evidence through an emergency.
As part of the initial action to respond to an oil spill accident, the responders should deliver some elementary information comprising oil type to the coast guard. This study presents a comprehensive pattern recognition modeling framework for identifying the different types of oily wastes from each other using limited information. The cluster-based method can help responders to identify the type of oily waste using certain criteria. When the type of oil is not clear or responders need to confirm it, the responders can take a sample of oily waste and use an instant kit to examine the percentage of oil, percentage of water, percentage of mineral matter, and percentage of organic matter. Once this information is collected, the responders can enter them into the proposed model and identify the type of waste. The present model is able to identify the following waste types: liquids, semi-solids, and solids (sand), polluted pebbles and stones, polluted sorbent, polluted seaweed, polluted solid waste, and polluted fauna.
The cluster-based analysis method presented in this article is part of an integrated optimization-based model to address complexities and support decision-making for an oily waste management system. It is expected that using homogenous clusters will decrease uncertainties in the optimization of waste management costs. The overall objective of the optimization model is to minimize total waste management costs, under the constraints involving waste storage and transport capacities, waste pre-treatment and treatment facility capacities, environmental regulatory compliance, as well as other operational and logistic constraints.
The remainder of the article is structured as follows: first, we present a background to marine oil spill incidents and operations. Then, we discuss the data used and data transformation necessary for pattern recognition, followed by the presentation of the pattern recognition methods and a discussion of model results. The article concludes by providing a summary of contributions and future research directions.
Literature Review
One of the most critical issues in the world-wide economy iscrude obtainability and petroleum refining. Numerous types of wastes are generated in the sequence of oil invention, processing, transportation and further use of oil products is a subject to required disposal (Board 2014). Consequently, it is important to have a classification framework that include relevant information, such as type of waste, quantity, treatment, and disposal of waste (Chen et al., 2012) in an environment-friendly way and environmental monitoring in keeping with legal necessities (Clear Seas Centre for Responsible Marine Shipping, 2022).
Marine Oil Spill Incidents in Canada
Canada puts the responsibility for cleaning up a spill which is originated from a ship to the contaminator. Every ship that is incoming or passing through Canadian waters is obligated to have a Shipboard Oil Pollution Emergency Plan (SOPEP). The SOPEP provides adequate information to the ship’s crew about the activities and communication requirements during the event of an accidental spill. To operate in Canadian waters, all tankers of 150 gross tonnes, all vessels of 400 gross tonnes or greater, and oil handling facilities, must have an arrangement with an oil spill response organization. If the spill exceeds the ship’s clean-up capacity, the polluter can request assistance from the pre-arranged response organization to support the clean-up activities (Clear Seas Centre for Responsible Marine Shipping, 2022).
The Canadian Coast Guard (CCG) is an operating agency that takes the lead in the oil spill response activities throughout clean-up processes. The CCG also works with the polluter and other relevant response organizations to guarantee a proper response system (Canadian Coast Guard, 2019).
Marine Oil Spill Response Operations
Canada has four response organizations, and each organization has its own area-specific response plans so that they can respond at a short notice for all of Canada’s shipping waterways. Every few years, Transport Canada (TC) conducts existing capacity and process audits and re-certifies each response association based on their technical and operational arrangements (Government of Canada, 2010; Government of Canada, 2018; Clear Seas Centre for Responsible Marine Shipping, 2022).
Federal government agencies play a vital role in the spill response system in Canada. For instance, the framework of CCG’s roles, scope, and responsibilities are outlined by the Department of Fisheries and Oceans (DFO) Canada. Another federal agency is Environment and Climate Change Canada (ECCC), which provides environmental and scientific advice during an accidental oil spill response process, including the movement of the spilled oil, weather conditions, and species at risk. The other federal authority is TC which manages and supervises the National Oil Spill Preparedness and Response Regime, within which TC measures marine incidents risks, screens the waterways, and certifies response organizations have the capacity to respond to oil spills.
Provincial and territorial governments are another response organization. This group communicates with all oil spill response associates during clean-up and oil recovery processes to oversee the land. Finally, local government resources are also made available to support response processes by providing local information, employees, or clean-up equipment.
Oily Wastes Generated From Operations
Waste is defined as any quantifiable or object that the holder discards, aims to discard, or is vital to discard (Carson et al., 2004; Barenboim et al., 2013). The seven main categories of oil spill waste are as follows, fauna, seaweed, and vegetation, seawater, solid waste (plastic, textile, metal, etc.), cleaning products, sorbent material, and PPE, and sediments (mud, sand, and pebbles). The sustainable management of wastes framework contains four ideas and arrangements: reduction, recovery, reuse, and disposal (Bayat et al., 2005).
Oily Waste Management Practices: Treatment and Disposal
An oil spill clean-up effort involves activities including, recovering the oil from the water, and shoreline cleaning if it is affected by the spill. The first phase is to contain the spill so that responders can control the damage and recover as much oil as possible. Usually, containment booms (floating barricades) are deployed as the first line of protection to stop the slick from spreading and reaching environmentally sensitive areas and shorelines. The next phase is to separate the oil from the water. Followed by oil containment, responders evaluate various response techniques to reduce the damage. Typically, the chosen technique has to use a Net Environmental Benefit Analysis (NEBA) method to demonstrate that it will minimize impacts on individuals and the environment (Clear Seas Centre for Responsible Marine Shipping, 2022). The third phase is to clean the shoreline. In the event, that oil spreads onto the shore, response teams are prepared for shoreline clean-up (Chandrasekar, Sorial, Weaver 2006). Usually, a shoreline clean-up approach contains equipment, including, sorbents, berms, shoreline flushing, and vacuums.
The Role of Waste Classifications in the Oily Waste Management
Usually, different oily wastes are kept separately as the treatment and disposal process depend on waste type and level of contamination. In the situation where oil is recovered quickly and is not contaminated by water and debris, it can be directly recycled and reprocessed. Oily waste that is heavily contaminated is usually taken to a disposal site. Disposal techniques depend on the: oily waste type, amount of recovered oil, spill location, and volume to make further decisions to store and transport the recovered oil and oily waste, arrangement of the storage facility, treatment and disposal facilities, and costs involved.
Assessment of the oil spill waste category based on the type of oil spilled is a tough task since it comprises several restrictions (Government of Canada, European Maritime Safety Agency, OPRC, 1995). As acknowledged from the prior studies and a conducted survey, the waste classification needs to be conducted with more rigor basis for better waste management (Bostrom et al., 2015). Therefore, this study develops a comprehensive pattern recognition modeling framework for deriving and grouping a set of unique clusters that separate different types of oily wastes from each other. The main idea is to group oily wastes based on their unique characteristics, such as percentage of oil, percentage of water, percentage of mineral matter, and percentage of organic matter. The proposed approach can be widely used on sites where oil spill incidents happened to identify the type of oily waste. After responders collected a sample of waste and identified various required materials percentages, they can use the proposed model to identify the waste type.
One of many potential applications of the proposed methods is to use in an integrated optimization-based model to address complexities and support decision-making for an oily waste management system. The overall objective of the optimization model is to minimize total waste management costs under the constraints involving waste storage and transport capacities, waste pre-treatment and treatment facility capacities, environmental regulatory compliance, as well as other operational and logistic constraints.
Hypothetical Case Study
Through an oil spill, oil spill modeling predictions are needed for planning the response activities over the next few days and longer for the deployment of greater resources into position. Smaller-scale procedures, such as sea breeze and topographical steering, are vital considerations in the timing and position of oil accomplishment on the shoreline, however, are not commonly determined in operational meteorological models, so user forecasters are used to estimating the potential for incidence. The management between the trajectory specialist and the field viewers is vital for ground-truthing and updating the spill forecasts. The model outcomes are stimulated by schemes and maps for use by responders and decision-makers to recognize the condition at hand and the possible development of the spill. Such maps can classify key risk areas and assist responders rank response activities in order to minimize environmental and socioeconomic harm (Berninger, Williams, Brooks, 2011).
As part of the first action to respond to an oil spill accident, the responders should deliver some basic information including oil type to the coast guard. This study presents a comprehensive pattern recognition modeling framework for identifying the different types of oily wastes from each other using limited information. As a requirement for the use of the proposed cluster-based technique, responders should have different waste properties including the percentage of oil, percentage of water, percentage of mineral matter, and the percentage of organic matter. The present model is able to identify the following waste types: liquids, semi-solids, and solids (sand … ), polluted pebbles and stones, polluted sorbent, polluted seaweed, polluted solid waste, and polluted fauna.
In this section, an overall explanation of the data used, and data processing steps used in this study is presented. This study uses a waste classification guide, from the CEDRE, 2016. Table 1 provides a categorization of the waste in seven groups depending on their nature and relative content and matching to discrete waste management streams. Note that the percentages are given in weight and basically deliver an indication of the relative values.
Source: Centre de documentation de recherche et d’expérimentations sur les pollutions accidentelles des eaux” (CEDRE).
Data Processing
Prior to employing pattern recognition methods, it was important to convert the waste classification data. The data transformation process is done using defined ranges for each category mentioned in Table 1. In this study, a total of 1,000 data records are randomly generated. The random generation process involved creating sample data from each of seven waste classification types. For instance, a new liquid sample data was created using the oil category greater than 10%, the water (free) category between 0 and 90%, the mineral matter category less than 10%, and the organic matter category less than 10%. Table 2 provides a snapshot illustrating sample data produced from Table 1 in this study. The waste classifications were split into 100 intervals. Each of the 100 cells was coded with one of the four major categories as defined in Table 1 (% Oil, % Water, % Mineral matter, % Organic matter). Consequently, the preliminary transformed matrix dimensions size is 1,000 × 100 × 4. The final step in the data transformation process was to transform waste data into the binary format. Each of the four major waste categories was transformed to a “1” or “0” binary code such that if the waste elaborated in the particular waste type a code of “1” was recorded, and otherwise “0”.
Methods
This section emphasizes the development of an inclusive pattern recognition modeling framework for deriving and grouping a set of unique clusters that separate different types of oily wastes from each other. The core idea is to cluster oily wastes based on their unique features, such as oily sand sedimentation, containment booms, sorbent booms, debris, oily vegetation, dead animals, and contaminated personal protective equipment.
Each cluster has a relatively homogeneous pattern of pollution features. Prior to employing the cluster analysis method, it is essential to assess and convert the oily waste data using well-defined criteria. Examples of raw data and defined criteria contain the physical data, such as the types and quantities of waste to be treated and processed, the location of waste generation, processing/treatment, and disposal facilities; the economic data, such as the costs related to the transportation and freight, processing/treatment and disposal as well as the value of the recovered oil; and other information such as, expert opinion, stakeholder perspective, and governmental regulations.
The pattern recognition modeling framework comprises the development of two algorithm components to implement the cluster analysis including a subtractive clustering algorithm (SCA) and Fuzzy C-Means (FCM) algorithm. Initially, we use the SCA for initializing the total cluster number and cluster centroids. Earlier studies (Pena, Lozano and Larranaga 1999; Erisoglu, Calis and Sakallioglu 2011) recommended initializing these two values before employing any clustering algorithm such as, K-means or C-means, in order to increase the performance of the main clustering algorithm (Hafezi et al., 2017; Hafezi et al., 2021). We used Dunn’s index to quantity cluster validity.
The SCA looks for cluster centers based on the density of neighboring data points. In general, the SCA contains five stages.
The parameter
The subsequent step in the SCA is to compute density for all data points:
Following, the algorithm re-estimates the density of all data points using the difference between the highest selected density in the last step and the new computed density:
The process of finding a new cluster center is continued if
The cluster number and cluster centroids recognized through the subtractive clustering algorithm are used as inputs for the fuzzy C-means (FCM) algorithm in the next step of the pattern recognition modeling framework. The FCM algorithm determines the final memberships in each cluster through a fuzzy process. The density (
Suppose
We employed the Kolmogorov–Smirnov (KS) test on the waste classification distributions between pairs of clusters, to test for significant differences. The Kolmogorov-Smirnov statistical test can determine whether or not two non-parametric datasets are drawn from similar distributions (Sheskin 2003). In this study, we utilized the Kolmogorov-Smirnov statistical test in order to understand the differences between waste classification distributions associated with all aggregated data for each cluster. The Kolmogorov–Smirnov test is defined by
where
Discussion of Results
We applied the proposed pattern recognition modeling framework to data associated with 1,000 random records drawn from the CEDRE Waste classification. The FCM clustering method bundled individual activity patterns into seven discrete clusters. The Dunn’s index showed seven to be the best number of clusters. In the following section, a discussion for each of the seven clusters and their waste categories is presented.
Cluster #1: liquids, comprised a group of wastes whose percentage of oil is greater than 10%. The water proportion of wastes in this group is between 0 and 90%. On the other hand, mineral matter and organic matter quantities for wastes in this group are less than 10%.
Cluster #2: semi-solids and solids (sand … ), consisted of a group of wastes that included more than 10% oil. The proportion of water is limited to 10% in this waste group. Unlike the liquids group, the semi-solids and solids wastes have more than 10% mineral matter. Furthermore, the proportion of organic matter in this waste group is less than 10%.
Cluster #3: polluted pebbles and stones, was a group of wastes in which mineral matter had the highest quantity compared to others. The percentage of oil is greater than 10%, whereas, the percentage of organic matter is less than 10%.
Cluster #4: polluted sorbent, involved a group of wastes that had very less proportion of organic matter (less than 5%). In this group, the quantity of water and mineral matter is less than 10%. The highest proportion of waste in this group included oil.
Cluster #5: polluted seaweed, comprised a group of wastes that had very a high quantity of organic matter (more than 80%). The proportion of water and mineral matter in this waste group is less than 20%. The proportion of oil in this group is greater than 5%.
Cluster #6: polluted solid waste, involved a group of wastes that included less than 10% water and mineral matter. The proportion of oil in this group is greater than 5%. The quantity of organic matter in this waste group is variable. This group of waste includes various wastes such as, gloves, boots, overalls, plastics, wood, etc.
Cluster #7: polluted fauna, comprised a group of wastes which more than 70% included organic matter. The proportion of oil in this group is more than 5%. The mineral water quantity is less than 10%. Furthermore, the proportion of water in this waste group is less than 15%.
The result of KS tests for seven different clusters and all waste categories are shown in Table 3. The KS test is built as a statistical hypothesis test. The null hypothesis (
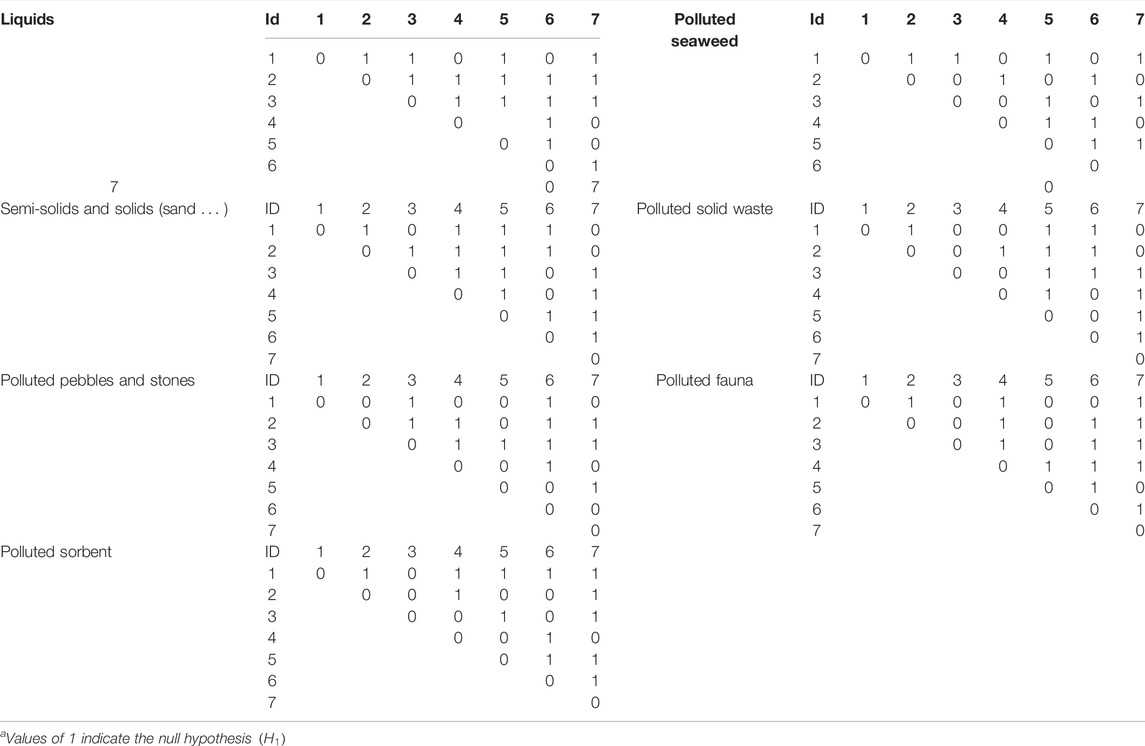
TABLE 3. Summary of Kolmogorov–Smirnov test waste type distribution by different groups (5% significance level)a.
Conclusion
Several types of wastes are generated in the course of oil production, processing, transportation, and additional use of oil products and are subjected required disposal. When an oil spill occurrs, the first phase is to state the perceived oil spill incident to the coast guard. The responders should deliberate variables, such as the category of oil, the quantity of oil, the position of the spill, wind and wave action, currents and tides, temperature of the air and water, and presence of ice, rain, or snow. As part of the first action to respond to an oil spill accident, the responders should provide some basic information including oil type to the coast guard. This study presents a comprehensive pattern recognition modeling framework for identifying the different types of oily wastes from each other using limited information. The proposed cluster-based method can help responders to identify oily waste types using different waste properties including a percentage of oil, percentage of water, percentage of mineral matter, and, percentage of organic matter. The current model is able to classify the following waste types: liquids, semi-solids, and solids (sand … ), polluted pebbles and stones, polluted sorbent, polluted seaweed, polluted solid waste, and polluted fauna. The proposed cluster-based method can be applied to different waste properties. In this study, we used seven waste classifications outlined in Table 1 as a target to identify representative clusters for oily waste types. Different waste properties can be added to the target and as a result, the proposed cluster-based method will have new clustering predictions.
The main idea is to cluster oily wastes based on their unique features, such as oily sand sedimentation, containment booms, sorbent booms, debris, oily vegetation, dead animals, and contaminated personal protective equipment. Each cluster has a relatively homogeneous pattern of pollution characteristics. Prior to implementing the cluster analysis technique, it is important to evaluate and transform the raw oily waste data using well-defined criteria.
Examples of raw data and defined criteria consist of the physical data, such as the types and amounts of waste to be treated and processed, the location of waste generation, processing/treatment, and disposal facilities; the economic data, such as the costs associated with the transportation and freight, processing/treatment and disposal as well as the value of the recovered oil; and other information, such as expert opinion, stakeholder perspective, and governmental regulations. We applied the proposed model to 1,000 randomly generated records associated with the CEDRE Waste classification with various waste categories. The clustering algorithm rapidly converged and resulted in seven clusters of waste types, each with homogeneous activity patterns. These clusters are liquids, semi-solids and solids (sand … ), polluted pebbles and stones, polluted sorbent, polluted seaweed, polluted solid waste, and, polluted fauna. It should be noted that other categorizations could be used, such as pure oil, oil and sediment, oil and organic debris, oil and water, and, oil and PPE/equipment. Further study can include extending the current modeling framework to include other waste classifications. Another potential future study would be to fine-tuning the proposed model to better estimate the number of clusters and cluster memberships.
One of the innovations in this study is that w incorporated two stages in identifying unique clusters: a subtractive clustering algorithm (SCA) and fuzzy C-Means (FCM) algorithm. First, we use a subtractive clustering algorithm for initializing the total cluster number and cluster centroids. We used Dunn’s index to measure cluster validity. Then, FCM was employed to identify cluster memberships.
The cluster-based analysis method presented in this article is expected to be incorporated into an integrated optimization-based model to address complexities and support decision-making for an oily waste management system. It is expected that using homogenous clusters will decrease uncertainties in the optimization of waste management costs. The overall objective of the optimization model is to minimize total waste management costs, under the constraints involving waste storage and transport capacities, waste pre-treatment and treatment facility capacities, environmental regulatory compliance, as well as other operational and logistic constraints.
Data Availability Statement
The raw data supporting the conclusions of this article will be made available by the authors, without undue reservation.
Author Contributions
The authors confirm contribution to the article as follows: study conception and design, data collection, and analysis and interpretation of results: MH and ND; draft manuscript preparation: MH, ND, and LL. All authors reviewed the results and approved the final version of the manuscript.
Conflict of Interest
The authors declare that the research was conducted in the absence of any commercial or financial relationships that could be construed as a potential conflict of interest.
Publisher’s Note
All claims expressed in this article are solely those of the authors and do not necessarily represent those of their affiliated organizations, or those of the publisher, the editors, and the reviewers. Any product that may be evaluated in this article, or claim that may be made by its manufacturer, is not guaranteed or endorsed by the publisher.
Acknowledgments
The authors thank the DFO-MPRI for their contribution in supporting the research.
References
Aamo, O. M., Reed, M., and Lewis, A. (1997). “Regional Contingency Planning Using the OSCAR Oil Spill Contingency and Response Model,” in Arctic and Marine Oil Spill Program Technical Seminar (Canada: Ministry of Supply and Services), 289
Atlas, R. M., and Hazen, T. C. (2011). Oil Biodegradation and Bioremediation: A Tale of the Two Worst Spills in U.S. History. Environ. Sci. Technol. 45 (16), 6709–6715. doi:10.1021/es2013227
Barenboim, G., Borisov, V., Saveka, A., Avandeeva, O., Khristoforov, O., and Stepanovskaya, I. (2013). “Development of a System for the Early Detection and Monitoring of Oil Spills on Water Bodies with a Glance to its Use in the Arctic Zone,” in Proceedings of the 36th AMOP technical seminar on environmental contamination and response, 565
Bayat, A., Aghamiri, S. F., Moheb, A., and Vakili-Nezhaad, G. R. (2005). Oil Spill Cleanup from Sea Water by Sorbent Materials. Chem. Eng. Technol. 28, 1525–1528. doi:10.1002/ceat.200407083
Beegle-Krause, J. (2001). “General NOAA Oil Modeling Environment (GNOME): a New Spill Trajectory Model,” in International Oil Spill Conference (American Petroleum Institute), 865–871. doi:10.7901/2169-3358-2001-2-865
Ben Fieldhouse, A. A. (2018). “Assessing the Effect of Temperature on the Use of Oil Spill Treating Agents,” in Ottawa: Environment and Climate Change Canada (Retrieved from ECCC).
Berninger, J. P., Williams, E. S., and Brooks, B. W. (2011). An Initial Probabilistic Hazard Assessment of Oil Dispersants Approved by the United States National Contingency Plan. Environ. Toxicol. Chem. 30, 1704–1708. doi:10.1002/etc.532
Board, O. S. (2014). Reducing Coastal Risk on the East and Gulf Coasts. Washington, DC: National Academies Press.
Bostrom, A., Walker, A. H., Scott, T., Pavia, R., Leschine, T. M., and Starbird, K. (2015). Oil Spill Response Risk Judgments, Decisions, and Mental Models: Findings from Surveying U.S. Stakeholders and Coastal Residents. Hum. Ecol. Risk Assess. Int. J. 21, 581–604. doi:10.1080/10807039.2014.947865
Canadian Coast Guard (2019). Marine Spills Contingency Plan. Ottawa, ON: Canadian Coast Guard as an Assisting Agency Government of Canada.
Carson, R. T., Conaway, M. B, Hanemann, W. M., Krosnick, J. A., Mitchell, R. C., and Presser, S. (2004). Valuing Oil Spill Prevention: A Case Study of California’s Central Coast. Dordrecht: KluwerSpringer Science & Business Media
CEDRE (1978). Centre de documentation de Recherche et d’Expérimentations Sur les Pollutions Accidentelles des eaux” (CEDRE). Retrieved from: http://wwz.cedre.fr/en/Planning/An-integrated-approach.
CEDRE (2016). Oil Spill Waste Management Manual, Preparedness for Oil-Polluted Shoreline Cleanup and Oiled Wildlife Interventions (POSOW). Valletta, Malta. Available at: https://www.posow.org/documentation/manual/cleanup-manual-2/
Chandrasekar, S., Sorial, G. A., and Weaver, J. W. (2006). Dispersant Effectiveness on Oil Spills - Impact of Salinity. ICES J. Mar. Sci. 63, 1418–1430. doi:10.1016/j.icesjms.2006.04.019
Chen, B., Zhang, B., Cai, Q., Lin, W., and Liu, B. (2012). From Challenges to Opportunities: Towards Future Strategies and a Decision Support Framework for Oil Spill Preparedness and Response in Offshore Newfoundland and Labrador, Project Report. The Harris Centre. Available at: http://www.mun.ca/harriscentre/reports/arf/2011/11-12-ARF-Final-Chen.pdf
Clear Seas Centre for Responsible Marine Shipping. (2022). Responding to Oil Spills in Canadian Waters.Documents
da Silva, L. J., Alves, F. C., and de França, F. P. (2012). A Review of the Technological Solutions for the Treatment of Oily Sludges from Petroleum Refineries. Waste Manag. Res. 30 (10), 1016–1030. doi:10.1177/0734242x12448517
Erisoglu, M., Calis, N., and Sakallioglu, S. (2011). A New Algorithm for Initial Cluster Centers in K-Means Algorithm. Pattern Recognit. Lett. 32 (14), 1701–1705. doi:10.1016/j.patrec.2011.07.011
European Maritime Safety Agency (2018). Oil Spill Response Services. Retrieved from EMSA: http://www.emsa.europa.eu/oil-spill-response/oil-recovery-vessels.html.
Government of Canada (2010). Marine Acts and Regulations. Retrieved from Government of Canada: https://www.tc.gc.ca/eng/marinesafety/tp-tp14609-2-marine-acts-regulations-617.htm
Government of Canada (2018). Marine Spills Contingency Plan – National Chapter. Retrieved from Canadian Coast Gaurd: http://www.ccg-gcc.gc.ca/folios/01230/docs/MSCP2018-eng.pdf
Hafezi, M. H., Liu, L., and Millward, H. (2017). A Time-Use Activity-Pattern Recognition Model for Aactivity-Based Travel Demand Modeling. Transportation 46, 1–26. doi:10.1007/s11116-017-9840-9
Hafezi, M. H., Daisy, N. S., Millward, H., and Liu, L. (2021). Framework for Development of the Scheduler for Activities, Locations, and Travel (SALT) Model. Transp. A Transp. Sci. 0, 1. doi:10.1080/23249935.2021.1921879
International Maritime Organization (2018). MEPC.1-Circ.834-Rev.1.,44 Consolidated Guidance for Port Reception Facility Providers and Users §. London, UK.
IPIECA (2014). Oil Spill Waste Minimization and Management. London, UK: IPIECA-OGP Good Practice Guide.
Jordão da Silva, L. (2012). A Review of the Technological Solutions for the Treatment of Oily Sludges from Petroleum Refineries. Waste Manag. Res. 30, 106. doi:10.1177/0734242X12448517
Lin, B., and Lin, C.-Y. (2007). Investigation of Strategies to Improve the Recycling Effectiveness of Waste Oil from Fishing Vessels. Mar. Policy 31 (4), 415–420. doi:10.1016/j.marpol.2007.01.004
National Research Council (2003). Oil in the Sea III: Inputs, Fates, and Effects. Washington, DC: National Academies Press
Ngo, L. T., and Pham, B. H. (2012). “A Type-2 Fuzzy Subtractive Clustering Algorithm,” in Mechanical Engineering and Technology: Selected and Revised Results of the 2011 International Conference on Mechanical Engineering and Technology. Editor T. Zhang (London, UKBerlin, Heidelberg: Springer Berlin Heidelberg), 395–402. doi:10.1007/978-3-642-27329-2_54
OPRC (1995). Article 6 of the International Convention on Oil Pollution Preparedness, Response and Co-operation (OPRC). Available at: https://treaties.un.org/doc/publication/unts/volume%201891/volume-1891-i-32194-english.pdf.
Owens, E. H., Taylor, E., O’Connell, K., and Smith, C. (2009). “Waste Management Guidelines for Remote (Arctic) Regions,” in Proceedings 32nd Arctic and Marine Oilspill Programme (AMOP) Technical Seminar (Ottawa, ON. Available at: https://oaarchive.arctic-council.org/bitstream/handle/11374/108/Guidelines%20and%20Strategies%20for%20Oil%20Spill%20Waste%20Management%20in%20Arctic%20Regions%20Final%20report.pdf?sequence=1&isAllowed=y.
Peña, J. M., Lozano, J. A., and Larrañaga, P. (1999). An Empirical Comparison of Four Initialization Methods for the K-Means Algorithm. Pattern Recognit. Lett. 20 (10), 1027–1040. doi:10.1016/S0167-8655(99)00069-0
REMPEC (2010). “Guidelines on Oil Spill Waste Management,” in Mediterranean Technical Working Group (MTWG) Programme. Editors F. Poncet, and L. Page-Jones. Available at: http://www.imo.org/en/OurWork/Environment/PollutionResponse/Documents/Oil%20spill%20waste%20management%20decision%20support%20tool.pdf.
REMPEC (2012). Documents, Section 12, Mediterranean Oil Spill Waste Management Guidelines. Sa Maison Hill, Floriana: International Maritime Organization.
REMPEC (2011). Regional Information Syste. Part D Operational Guidelines and Technical. Sa Maison Hill, Floriana: International Maritime Organization.
RRT/NWAC (2020). Disposal and Waste Management Guidance for the Northwest Area, Northwest Area Contingency Plan (NWACP). Seattle, WA. Available at: https://rrt10nwac.com/Files/NWACP/2020/Section%209405%20v21.pdf
Keywords: waste, machine learning, oil spill, cluster, patterns
Citation: Hafezi MH, Daisy NS and Liu L (2022) A Cluster-Based Technique for Identifying and Grouping Oily Waste Types Generated From Marine Oil Spill Response Operations. Front. Environ. Sci. 10:910214. doi: 10.3389/fenvs.2022.910214
Received: 01 April 2022; Accepted: 19 April 2022;
Published: 08 June 2022.
Edited by:
Oladele Ogunseitan, University of California, Irvine, United StatesCopyright © 2022 Hafezi, Daisy and Liu. This is an open-access article distributed under the terms of the Creative Commons Attribution License (CC BY). The use, distribution or reproduction in other forums is permitted, provided the original author(s) and the copyright owner(s) are credited and that the original publication in this journal is cited, in accordance with accepted academic practice. No use, distribution or reproduction is permitted which does not comply with these terms.
*Correspondence: Lei Liu, bGVpLmxpdUBkYWwuY2E=