Effect of Science and Technology Finance Policy on Urban Green Development in China
- 1China Economics and Management Academy, Central University of Finance and Economics, Beijing, China
- 2Accounting School, Harbin University of Commerce, Harbin, China
- 3School of Economics, Beijing Technology and Business University, Beijing, China
Green sustainable development is a major challenge faced by countries worldwide. Against the strategic background of innovation-driven development, studying the impact of science and technology finance policy (STFP) on urban green development is of great practical significance. Based on urban panel data from 2003 to 2019, this study systematically examines whether and how STFP affects urban green development in China using the difference-in-differences (DID) method. The empirical results show that STFP has significantly stimulated the urban green development level, and the effect of policy implementation has increased first and then decreased over time. The findings remain robust when using propensity score matching DID to avoid selection bias and other factors that may interfere with the estimation results. Additionally, technological innovation and green innovation are essential channels for STFP to improve urban green development. The impact of STFP is found to vary by region and by the level of urban innovation. Specifically, the policy effect is more pronounced in midwestern and high-innovation cities but less obvious in eastern and low-innovation cities. In conclusion, this study provides city-level empirical evidence from China for an in-depth understanding of the green economy effect of STFP. It also provides theoretical guidance and policy references for accelerating the green transition in the context of sustainable development.
1 Introduction
In recent years, China’s economy has maintained rapid growth, rising to become the second largest globally. China has also contributed to the history of world development. However, as the economy transitions from high-speed growth to high-quality development, China must overcome important hurdles of pollution control and ecological restoration (Zhang, 2007; Sheng and Tang, 2016; Shao et al., 2018). In the traditional industrial era, production activities were driven by the input of factors that produced large amounts of pollutant emissions. This is not conducive to long-term economic development (Reid et al., 2007). Balsa-Barreiro et al. (2019) analyzed the global shift of centers of gravity related to gross domestic product (GDP), carbon dioxide (CO2) emissions, total population, and urban population during 1960–2016. They pointed out that China now shows evidence of a certain economic maturity, indicating more efficiency in the consumption of resources and energy. China is in a more advanced stage of industrialization than India, with a slight decoupling trend between economic growth and emissions. Yet, many regions and industries in China continue to pursue economic development through a model of high investment and high pollution, resulting in increasingly prominent environmental problems (Yue et al., 2018; Wang and Feng, 2021). China’s Environmental Performance Index score and ranking have remained relatively low over recent years (Pan et al., 2021). In January 2022, China’s urban air quality report showed an average of 59.5% of days with excellent air quality in 168 cities, a 5.3 percentage point decrease year on year. However, environmental quality varies greatly between Chinese cities.
The novel green development model represents a shift from the traditional development model. It incorporates environmental protection into the goal of sustainable development while remaining constrained by environmental and resource-carrying capacity constraints (Dai et al., 2016; Bagheri et al., 2018). The 14th Five-Year Plan points out that harnessing the urban and rural living environment and maintaining the stability of the ecosystem is vital to promoting high-quality economic development and improving people’s quality of life. As an important part of the new development concept, green development needs the participation and support of enterprises and the public. To this end, the government needs to elevate the concept to the national strategic level (Li et al., 2020). In 2011, the Ministry of Science and Technology and four other ministries established pilot cities to “promote the combination of technology and finance”—the country’s first policy measure to foster technological innovation at financial level. In general, the government is committed to increasing research and development (R&D) investment to pursue technological progress, aiming to improve production efficiency and energy efficiency (Liu and Lin, 2019). Continuous financial development makes high-tech industries and advanced technologies more attractive to domestic and foreign markets, thus helping to transform the current economic development model into green development (Qian et al., 2021). Previous studies have mainly examined the unilateral impact of technological innovation or financial development on environmental protection, with only a few scholars combining the two. Therefore, researching how science and technology finance policy (STFP) impacts urban green development has essential reference value and practical significance for China’s efforts toward establishing a new development pattern and promoting high-quality development.
Studies have shown that combining technology and finance can promote regional economic growth. However, few studies report the impact of STFP implementation on green development, and the effect mechanism has not been thoroughly examined. We address this gap in the literature as follows. First, we construct 18 secondary index evaluation systems based on ecology, economy, and society, then use the entropy weight method to measure the indicators of urban green development. Second, we utilize the difference-in-differences (DID) method to evaluate the effect of STFP implementation. In addition, we apply propensity score matching (PSM)–DID to eliminate selection bias. Third, we use dynamic tests to study the time-varying impact of STFP on urban green development. Fourth, we introduce technological innovation and green innovation as two variables for mechanism analysis, thus deepening our understanding of the relationship between STFP and green development. Finally, because economic development and innovation levels vary significantly across regions, we divide the sample into eastern and midwestern cities and into high-innovation and low-innovation cities to investigate the heterogeneity of the influence of STFP on urban green development.
The study makes four main contributions. First, by analyzing the impact of STFP on urban green development, we enrich theory on the green economy, expand the literature on evaluating STFP’s economic impact, and provide valuable insights for the widespread promotion of STFP. Second, we address the lack of empirical evidence on the impact of STFP on urban green development. Third, in terms of evaluation methods, our study considers the implementation of STFP as an exogenous impact on each city and uses the DID method to effectively analyze how STFP affects the green economy. Compared with previous research, our approach avoids interference from other relevant policies on the measurement results, producing more accurate findings. Fourth, our results show that continuing to deepen STFP can not only improve technological innovation but also help promote green innovation.
The remainder of this study is organized as follows: Section 2 describes the literature review and research hypothesis. Section 3 describes the methodology, which mainly introduces variables and model settings. Section 4 reports the results of the empirical analysis. Section 5 describes the discussion. Section 6 includes the conclusion and policy recommendations of this study.
2 Literature Review and Research Hypothesis
2.1 Literature Review
With the rapid development of big data, artificial intelligence, and cloud computing, the combination of technology and finance stems from the profit-seeking nature of financial capital and the high returns of technological innovation. The effective combination can promote joint technological and financial development (Perez, 2007). Now endorsed by many scholars, Schumpeter (1912) pointed out that entrepreneurs’ innovation can lead to the accumulation of financial capital. Through continuous innovation, enterprises can establish a competitive advantage in the market. Continued investments in tangible and intangible assets foster innovation (Porter, 1992). In addition, Bernier and Plouffe (2019) point out that financial innovation combines two essential drivers of economic growth. Technological innovation can boost financial competitiveness; in turn, financial deepening will nurture enterprises’ innovation vitality, thereby promoting sustainable economic development (Samila and Sorenson, 2011).
However, most studies fail to mention the concept of “science and technology finance,” and relevant literature mainly explores the relationship between scientific and technological innovation and financial development. Zhao et al. (2009) advanced relevant research by providing a relatively comprehensive definition of science and technology finance. Fang (2015) suggests that technology finance is a new economic paradigm that promotes the effective integration of innovative elements such as technology, capital innovation, and entrepreneurship. Its function is to help enterprises achieve high added value and improve market competitiveness. The literature has also empirically examined the effects of science and technology finance on the economy (Shen et al., 2022), innovation (Sheng et al., 2021), R&D (Brown et al., 2017), and industrial agglomeration (Zhang and Zhang, 2018). However, there has been little empirical research on whether and how it affects green development.
The concept of green development was proposed at the Fifth Plenary Session of the 18th Central Committee of the Communist Party of China and has since received growing research attention (Wang et al., 2018). Green development is an economic and social development strategy that aims for efficiency, sustainability, and harmony. It advocates that all countries should prioritize the future while stimulating economic growth, and join together to create a beautiful picture of “clear waters and green mountains as valuable as mountains of gold and silver.” Studies of green development have mainly focused on two aspects. First, scholars have used various methods—most commonly, data envelopment analysis—to calculate a green development efficiency index (Zhu et al., 2020; Li C. et al., 2021). Second, many studies have investigated the influencing factors of green development, including political, economic, technological, and natural factors. Political factors mainly include government support (Guo and Liu, 2022) and environmental regulations (Zou and Zhang, 2022); economic factors mainly include financial development (Yuan et al., 2019), level of openness (Huang and Liu, 2021), and industrial structure (Xie and Li, 2021); the main technological factor is innovation (Xu et al., 2021a); and natural factors primarily include resource structure (Fong et al., 2022) and energy consumption (Moutinho et al., 2017).
The ecological environment directly affects and is a critical subsystem supporting regional green development. It contributes to the harmonious coexistence of man and nature (Sun et al., 2018). Since China entered the World Trade Organization in 2001, its carbon dioxide emissions have shown a strong upward trend. Severe environmental problems have directly threatened China’s sustainable economic development and public health (Shahbaz et al., 2020). Many studies have shown that technological innovation and financial development impact the regional ecological environment (Abid et al., 2021; Kihombo et al., 2021).
Many scholars have used IPAT or STIRPAT methods to analyze the impact of technological innovation on the ecological environment (Dietz and Rosa, 1994; York et al., 2003). Technological innovation promotes energy efficiency through technology spillover effects. Nathaniel et al. (2021) contend that technological innovation could directly improve environmental quality by developing technologies related to environmental protection. However, some scholars argue that technological innovation has a negative or no impact on energy efficiency (Hübler and Keller, 2010; Adom and Amuakwa-Mensah, 2016). Relatedly, Usman and Hammar (2021) contend that technological innovation is not conducive to improving environmental quality. Specifically, they point out that technological innovation expands a region’s ecological footprint, making the ecological environment more vulnerable.
Financial development can improve environmental quality by encouraging investment in green technologies. For example, the development and use of energy-efficient appliances and electric vehicles can improve energy efficiency and thus help reduce carbon emissions (Shobande and Ogbeifun, 2022). Financial development also attracts foreign direct investment, which can promote the exchange of green technology, thereby positively affecting environmental quality (Ahmad et al., 2021). However, some scholars have shown that financial development is not conducive to improving environmental quality. Using data from 59 countries along the Belt and Road routes, Baloch et al. (2019) found that financial development worsened environmental quality by increasing the ecological footprint. In Yasin et al.’s (2020) study of 110 developed countries and developing countries, financial development was found to reduce the ecological footprint of developing countries but not improve their environmental quality. Tahir et al. (2021) revealed that financial development significantly promoted carbon emissions and reduced environmental quality in South Asian economies.
Market failure may cause environmental degradation and ecological damage in a market economy system, necessitating regulatory and public policy intervention by the government (Zhang et al., 2019). The Chinese government set up a pilot policy of “combining technology and finance” in 41 cities in 2011. Most research on STFP uses the DID method to examine its impact on the innovation level (Zheng S. M. et al., 2020), industrial structure development (Hu and Liu, 2021), and regional economic development (Xu et al., 2021b). The policy, in particular, has been found to ease corporate financing constraints by increasing financial investment in science and technology, driving regional financial development, and promoting the further improvement of innovation levels in pilot regions. The advancement of regional capabilities in scientific and technological innovation has accelerated the transformation of new and old kinetic energy and the optimization and upgrading of the industrial structure, thereby raising their potential economic return. However, the STFP implementation effect is highly variable. Ma and Li (2019) contend that STFP plays a positive role in promoting technological innovation in both high-level cities and low-level cities but with more obvious effects in the latter. According to Hu and Liu (2021), the impact of STFP on the transformation and upgrading of industrial structure is positive in the eastern region, negative in the central region, and non-existent in the western region. Xu et al. (2021a) found that the pilot policy has the greatest positive impact on economic development in the eastern region, followed by the central region, but has no such impact in the western region. Many other researchers have analyzed the impact of digital finance on green innovation (Cao et al., 2021; Liu J. et al., 2022). It is widely accepted that digital finance positively impacts green innovation, although the extent of this effect varies across regions and industries. Analyzing provincial panel data, Wang and Gu (2021) found that technology finance can propel the high-quality development of China’s economy. They also stated that future research should start with the impact of science and technology finance on the ecological environment. Thus, our research can fill the gap in the current research.
2.2 Research Hypothesis
As the two most active factors in economic and social development, technology and finance have together transformed the development mode from factor driven to innovation driven. Science and technology are the primary forces of production, and technological progress requires financial support. Continuously improving the combination of technology and finance is critical for promoting independent innovation and increasing total factor productivity. The primary goal of STFP is to leverage public funds to guide market financial investment, encourage enterprises’ technological innovation, optimize the industrial structure, and achieve high-quality economic development. Therefore, STFP can achieve regional green development through financing and innovation effects.
On the one hand, the government plays a guiding and driving role, using the market mechanism to encourage financial institutions to actively participate in technological innovation. The government thereby helps high-tech enterprises overcome financing barriers and provides financial support for the realization of regional green development. On the other hand, pilot cities are more likely than other cities to gather innovative talents, technologies, products, and markets, all of which can provide technical support for green development. Accordingly, we hypothesize:
Hypothesis 1 (H1). STFP promotes urban green development.
Technological innovation promotes the transformation of production modes to promote ecosystem restoration, which is crucial for resolving resource and environmental problems (Destek and Manga, 2021). It can also indirectly stimulate the development of a regional green economy by promoting the transformation of old and new kinetic energy and optimizing the industrial structure. In situations where local government competition aggravates deterioration of the urban ecological environment, financial marketization can promote the green development of the industry by improving urban innovation capabilities. Capital inflow enables the reallocation of financial resources from high-polluting, high-emission industries to clean-energy-based industries. After obtaining technology funds, some enterprises can develop technological innovation and upgrades, thereby improving their production efficiency and reducing pollution output. Green development differs from the traditional economic development of the past, with a fundamental change in the mode of production, as the driving force of development. Innovation not only distorts the market but also causes transformation. By adjusting the industrial structure, the level of green economic development can be driven up.
Hypothesis 2 (H2). STFP improves urban green development by promoting technological innovation.
Compared with ordinary projects, green technology innovation projects are characterized by a long R&D cycle and income uncertainty, resulting in more serious financial constraints (Zhang and Chiu, 2020). With the increasing prominence of environmental problems, the government and financial institutions will force and encourage enterprises to assume responsibility for sustainable development. The implementation of STFP has continuously improved investment and financing system of green intellectual property. Promoting the realization of science and technology innovation achievements will stimulate enterprises’ green innovation vitality. Correspondingly, enterprises that pursue green innovation can obtain more resources and benefits, such as a higher social reputation, good relationship with the government, and greater market share (Burns et al., 2016). Green technology innovation can effectively improve the use efficiency and market scope of eco-environmental elements to reflect the intrinsic value. Moreover, green innovation can continuously enhance the competitiveness of the green industry, cultivating new advantages that help it strongly support green development.
Hypothesis 3 (H3): STFP improves urban green development by promoting green innovation.
3 Methodology
3.1 Spatial Distribution
This study examines the impact of STFP on urban green development using the DID method and a quasi-natural experiment of STFP combined with panel data from 256 cities in China. To assess the effect of the pilot policy, this study uses the Stata16 external command “Spmap” to draw the spatial distribution comparison diagram of green development before and after implementing the STFP, as shown in Figure 1. The pilot cities for STFP are mainly distributed in the eastern and central regions. Through comparison, it is found that the green development level prior to the implementation of the STFP is relatively low. Most pilot cities had green development levels ranging from 0.0783 to 0.1474, with only a few cities falling between 0.1474 and 0.2165. After the policy’s implementation, the green development level of almost all pilot cities has increased to 0.1474. Moreover, the green development level of pilot cities in the eastern region is generally higher than that of pilot cities in the midwestern region, indicating that economic development is an essential reference point for the government when establishing pilot cities. It is necessary to investigate the impact of STFP on urban green development from the perspective of heterogeneity in this study.
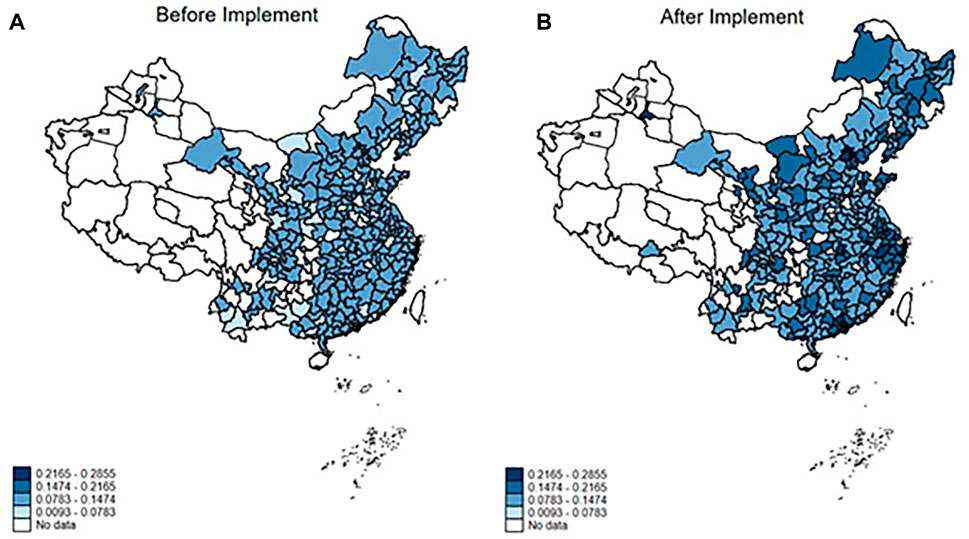
FIGURE 1. Spatial distribution of green development before and after the implementation of STFP. (A) Green development level before the implementation of STFP; (B) Green development level after the implementation of STFP.
3.2 Model Setting
The DID method is commonly used to study the effects of policy evaluations related to technological innovation. It effectively avoids the bias of estimation results caused by trends and random factors (Acemoglu and Johnson, 2007). The Ministry of Science and Technology issued the STFP in 2011, and the pilot policy covers 16 regions in China, including 41 cities. The sample duration selected in this study is from 2003 to 2019. The policy immediately affected the cities that participated in the pilot work in 2016. Therefore, this study uses the data from the first batch of pilot cities as the experimental group for investigation, and the data from the second batch of pilot cities to test the robustness of the benchmark results. Although Ningbo was included in the second batch of pilot cities, the Ningbo High-Tech Zone policy pilot began in 2012. Considering that the high-tech urban zone is the focus of urban innovation, this study includes Ningbo in the first batch of research pilot cities. Based on this, this study selects 215 other cities as the control group in addition to the 41 pilot cities, providing a good quasi-natural experiment for analyzing the implementation effect of STFP. This study uses the DID method to test the impact of STFP on urban green development. The model is constructed as follows:
where
3.3 Variable Description
3.3.1 Dependent Variable: Indicators for Urban Green Development
Starting with the definition of green development and based on the United Nations Sustainable Development Goals and the “society-economy-nature” composite ecosystem theory (Commission on Sustainable Development, 2000), this study constructs three first-level indicators, including ecological development (
3.3.2 Explanatory Variable: Science and Technology Finance Policy
This study uses cross terms (
3.3.3 Other Variables: Control Variable and Mediator Variable
In order to prevent endogenous problems caused by missing important variables, this study selects the following control variables based on the relevant literature on the influencing factors of green economy development (Zheng Y. et al., 2020; Liu B. et al., 2021; Wang and Yi, 2021): 1) Financial development (
3.4 Parallel Trend Test
The DID method’s premise is to ensure that the regression results are unbiased. The condition is that the treatment and control groups must maintain parallel time trends before implementing the policy. If the pre-event time trends are inconsistent between the treatment group and the control group, other exogenous shocks are considered to have caused the explained variable to change. Figure 2 shows the time trend of the green development level of pilot cities and non-pilot cities for STFP and judges whether the two curves have an ex ante parallel trend based on the practice of Yu and Zhang (2017). It can be seen that the green development level of the treatment and control groups has always maintained the same trend from 2003 to 2011, with no difference. From 2011 to 2019, the two sets of curves show obvious differences. The growth rate of the green economy in pilot cities is significantly higher than that in non-pilot cities, especially in 2014. The treatment group grew further apart from the control group. Therefore, this study adopts the DID model to examine the implementation effect of the STFP, which satisfies the parallel trend assumption.
4 Empirical Results
4.1 Benchmark Regression Test Results
In order to analyze the impact of STFP on urban green development, the DID method is used for estimation in this study, and the regression results are shown in Table 4. Column (1) shows the estimated results after adding control variables and without controlling time and region fixed effects. Column (2) reports the estimated results after adding control variables while controlling time and region fixed effects. From the results in the first two columns, it is found that the coefficients of the
At the same time, we use DID method to further study the impact of STFP on the three sub-variables of urban green development. These findings are reported in columns (3) to (5) of Table 4. The results show that the STFP has strongly impeded urban economic and social development. The findings imply that the implementation of the pilot policy can drive local government funds to enter the technology industry, thereby effectively solving the financing constraints of technology companies. Once a region helps companies solve financing constraints, it will attract more high-tech companies to settle in. The entry of new enterprises increases the entrepreneurial and employment opportunities of residents and promotes the development of the local economy. However, an unexpected result is that the STFP had a non-significant inhibitory effect on urban ecological development. One possible explanation is that while the pilot policy has driven the city’s overall development, enterprises will produce a large amount of waste in rapid economic development, resulting in ecosystem destruction. Will this negative effect ameliorate over time? This study will examine the dynamic effects of pilot policies on ecological development in the following sections.
4.2 Dynamic Effect Test Results
In order to reflect the impact of STFP on the changes in the green development of pilot cities since 2011, this study uses a dynamic effect test. Table 5 shows the dynamic regression results for the impact of STFP on green development and its three sub-variables. It can be found that urban green development, economic development, and social development have been continuously improved for some time after the implementation of the STFP. Until 2013, economic development had reached its peak, while green development and social development had reached their peak in 2014. Subsequently, the effect of the pilot policy showed a downward trend, but the coefficient remained significantly positive. It means that the implementation effect of STFP on urban green development, economic development, and social development has increased first and then decreased over time. From the regression results of ecological development, it can be seen that although the implementation of the STFP initially has an inhibitory effect on urban ecological development, its inhibitory effect gradually decreases over time. It reflects that the negative impact of STFP on the ecological environment has been weakening. However, by 2015, the inhibitory effect had become a facilitative effect.
4.3 Robustness Test Results
4.3.1 PSM-DID Results
This study aims to examine the impact of STFP on urban green development. The pilot and non-pilot cities could be analyzed separately to compare changes in green development from before to after the pilot policy’s implementation, and thereby infer the policy effect. However, given the non-random nature of STFP, this simple direct comparison would lead to selective errors. The PSM method can solve this problem (Rosenbaum and Rubin, 1983). Specifically, the probability of each city being included in the pilot is estimated based on city characteristics, then cities in the treatment group, and matched with cities in the control group that have the most similar probability of pilot inclusion. After the samples are matched, the DID method is used to estimate the impact of STFP on urban green development.
However, the parallel trend assumption must be satisfied before using PSM. The kernel density distribution is used to test the matching effect of the propensity score (Liu X. et al., 2022), as shown in Figure 3. Before matching, the treatment and control groups’ propensity scores were quite different; after matching, the two groups had very close probability density function values, indicating that matched samples can somewhat reduce the selection bias. In addition, a common support hypothesis test was conducted to determine whether the mean value of covariates in the two sample groups significantly differed after matching compared to before matching, as shown in Figure 4. We found that most of the samples after matching were in the common support area, with no apparent difference. This shows that the quality of the matched samples is relatively good and that the PSM-DID method is appropriate for robustness testing. The PSM-DID regression results are reported in column (1) of Table 6. They show that the implementation of STFP significantly propelled urban green development, which is consistent with the benchmark regression estimation results, indicating their robustness.
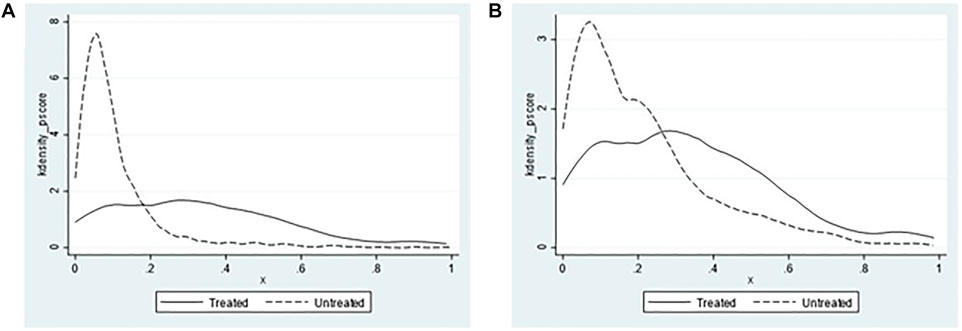
FIGURE 3. Nuclear density distribution. (A) Prior to matching, the treatment and control group’s propensity scores are quite different; (B) After matching, the distribution probability density function values of the two groups are very close.
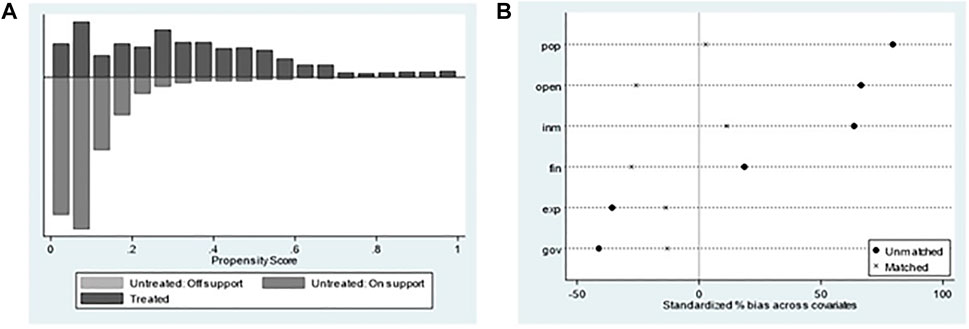
FIGURE 4. Common support hypothesis testing. (A) Common value range of propensity score matching; (B) The standardization of control variables before and after matching.
4.3.2 Other Robustness Test Results
In addition to using the PSM-DID method to solve the possible impact of the selection bias on regression results, we also apply the following three methods to test the robustness:
First, we eliminate the extreme values of this study. To eliminate any extreme outliers for each variable, which may distort results on the STFP implementation effect, we performed 1% abbreviated processing for all samples. The regression results after eliminating extreme values are reported in column (2) of Table 6. The regression coefficient of STFP was significantly positive, indicating that STFP is conducive to urban green development. This is consistent with the benchmark regression results, indicating that they are robust.
Second, we exclude any other policy effects. When estimating the impact of STFP on urban green development, the concurrent influence of other policies may cause the actual effect of STFP to be overestimated or underestimated. To avoid such interference, we identified other policy events that followed the implementation of STFP. Smart city construction began in 2013, marking a new era in urban development strategy, supported by emerging information technology. Therefore, we incorporate the smart city construction policy of 2013 into the benchmark regression model as another quasi-natural experiment. As reported in column (3) of Table 6, the results show that smart city construction has played a significant role in promoting urban green development. Although the coefficient of STFP remained significantly positive, its coefficient value decreased compared with the benchmark regression results. This demonstrates that the benchmark results overestimate the role of STFP on urban green development but are nonetheless relatively robust.
Third, we introduce the second batch of pilot cities in 2016. After introducing the second batch of pilot cities for STFP, if the sign of the policy coefficient remains significantly positive, it is confirmed that the role of STFP on urban green development is effective. Because the two batches of cities are selected as pilot projects at different times, this study used the staggered DID method to re-estimate the benchmark model. The results are shown in column (4) of Table 6. The significance and coefficient of policy variables are consistent with the benchmark regression results after introducing the second batch of pilot studies, demonstrating that the results of this study are robust.
4.4 Mediating Effect Test Results
Using the intermediary-effect model proposed by Baron and Kenny (1986), we constructed the following recursive model to verify whether technological innovation and green innovation are the mechanisms by which STFP affects urban green development. Based on Eq. 1, the following equations are constructed:
where
Columns (1) and (3) of Table 7 show the estimation results of Eq. 2, that is, the impact of the implementation of STFP on technological innovation and green innovation, respectively. It can be seen that the estimated coefficients of STFP are significantly positive, indicating that STFP can significantly boost urban independent innovation ability and accelerate green-innovation-level improvement. Columns (2) and (4) of Table 7 report the estimation results of Eq. 3, which tests whether technological innovation and green innovation are the channels through which STFP affects urban green development. According to the results in column (2), the estimated coefficient of technological innovation is negative, which indicates that technological innovation as a mediator masks the effect of an independent variable on a dependent variable. It is a generalized mediation effect (Wen and Ye, 2014). The result in column (4) shows that the estimated coefficients of green innovation are significantly positive, which preliminarily indicates that green innovation is an important channel for STFP to promote urban green development.
4.5 Heterogeneity Analysis
4.5.1 Regional Heterogeneity
The above results show that STFP can significantly promote urban green development but is subject to regional variation, depending on the development situation. According to their regions, we divide the sample into eastern and midwestern cities. As reported in columns (1) and (2) of Table 8, the regression results show that the implementation of STFP has a significantly positive influence on green development in both eastern and midwestern cities. However, comparing the coefficient of the explanatory variable reveals that the effect is greater in midwestern cities.
4.5.2 Innovative-Level Heterogeneity
According to the 2017 China Urban and Industrial Innovation Report, China’s innovation index accounts for 16–26% of the economy. The eastern region is home to 13 of the top 20 cities in the innovation index. The report also identified a considerable imbalance in the regional distribution of the urban innovation index, with prominent agglomeration in the eastern region. It can thus be inferred that, against the background of different innovation levels, the impact of STFP implementation on urban green development is heterogeneous. Following the methodology of Ma and Li (2019), we divided the sample cities into two groups (high innovation and low innovation) according to the mean value of innovation level from 2003 to 2019. As reported in columns (3) and (4) of Table 8, the results show that the implementation of STFP played a significant role in promoting green development in both high-innovation and low-innovation cities. However, the policy effect is greater in high-innovation cities.
5 Discussion
The benchmark regression results indicate that science and technology finance policy (STFP) can significantly promote urban green development. The government’s policy support for high-quality economic development is still in the continuous improvement stage, and STFP is an important influencing factor. This is consistent with the findings of King and Levine (1993), Yan and Wu (2020), and Wang and Gu (2021). Compared with developed countries, China’s financial market is still maturing, raising the urgent need for a policy-based financial system with complete structure and functions to support technological innovation (Zheng S. M. et al., 2020). In this regard, the government’s guiding role is crucial, and STFP can ease the financing constraints of technology-based enterprises and thereby stimulate innovation vitality. In recent years, government policies have encouraged technological innovation and green development in China, while scientific research institutions and scholars have become increasingly interested in green and sustainable development. Continuous improvement of the policy environment is also significant in enhancing urban green development. Therefore, our findings support H1.
We also discovered that STFP is an important driver of urban economic development and social development. Contrary to traditional theories, however, STFP has no significant effect on ecological development. This could indicate that the pilot policy has not done enough to promote, or perhaps has not even considered, ecological protection. Importantly, the initial plan for implementing STFP aimed to provide high-quality financial services to support the development of technological innovation (Yuan et al., 2018). The government must attach importance to the upgrading of STFP from product systems to ecosystems, thereby promoting the achievement of carbon peak and, ultimately, carbon neutrality nationwide. In addition, the dynamic effect test used in this study suggests that the impact of STFP will change over time. When the government formulates a policy, it should account for time variability in the policy effect to maximize its effectiveness.
In the intermediary-effect model, we investigated the situation of technological innovation and green innovation as mediating variables simultaneously. STFP exhibits a positive correlation with urban technological innovation, confirming the finding of Ma and Li (2019). Several scholars have demonstrated that technological innovation promotes economic development (Pradhan et al., 2016; Kogan et al., 2017). However, our results indicate that technological innovation inhibits urban green development. Hence, technological innovation appears to mask the relationship between STFP and green development. When the masking effect exists, the research perspective should change from how the independent variable affects the dependent variable to how the independent variable does not affect the dependent variable. Specifically, technological innovation constrains the impact of STFP on urban green development. One explanation is that the implementation of STFP encourages the agglomeration of resources, talents, and enterprises to engage in innovative activities, leading to a negative effect on urban green development through elevated energy demand, resource shortages, and pollutant discharges. Another explanation is that under the sound policy guarantee and government investment, STFP can indeed attract market capital injection and activate technological progress. However, due to China’s lack of supporting policies, the capital flows into high-polluting industries. Hence, technological progress can produce energy rebounds (Hu et al., 2019).
STFP and green innovation have a significant positive correlation, indicating that the implementation of STFP will lead to improvement in the green innovation level, which is consistent with the findings of Gu and Chai (2021). We also draw a similar conclusion to Ahmed et al. (2022) that green innovation contributes to green economic growth. In this regard, STFP can guide market funds to flow into fundamental innovation fields with high risk and low return and promote economic structural adjustment to enhance energy utilization, thereby promoting urban green development (Liu Y. et al., 2021). In addition, the implementation of STFP provides greater opportunities for innovative enterprises and talents. Enterprises adopt green production mode and incubate green technology products, which is significant in promoting urban green development further. STFP can also promote technological innovation to improve energy efficiency and shift the energy consumption structure, thereby pushing urban development in a resource-saving direction. Exploring the mechanism through which STFP influences urban green development provides a rational basis for assessing the effect of STFP implementation.
In the regional heterogeneity test, STFP was found to promote green development more effectively in midwestern cities than in eastern cities. This is consistent with the results of Zhang et al. (2021). Possible explanations are as follows. Compared to midwestern cities, eastern cities have a higher level of economic development and better infrastructure. The government of eastern cities can formulate other relevant policies to promote green development, making the precise role of STFP unclear. Conversely, compared with the eastern region, the midwestern region has much greater room to improve through scientific and technological achievements and the construction of a financial service system. Therefore, the marginal utility of the pilot policy is greater in midwestern cities. With regards to urban green development, the implementation of STFP has provided timely help in midwestern cities but merely put the “icing on the cake” in eastern cities.
In the innovation-level heterogeneity test, the policy was found to have a greater positive effect in high-innovation cities, which have better technological innovation industries than low-innovation cities. The implementation of STFP can greatly alleviate financing constraints on green science and technology industries, while further driving other supporting industries for environmental protection. Despite significant policy support for developing a green economy in low-innovation cities, they still lack advanced technology and a sound financial system (Ma and Li, 2019).
6 Conclusion and Recommendations
We use DID model to deeply explore the impact of STFP on urban green development. This study considers STFP implementation as a quasi-natural experiment and analyzes panel data of 256 cities in China from 2003 to 2019. The conclusions are as follows. First, there is a significant positive correlation between STFP and urban green development, indicating that STFP has supported the acceleration of green city construction. The results are robust to using PSM-DID to avoid selection bias and overcome the potential interference of other determinants of green development. Second, regarding the three sub-variables of urban green development, we find that STFP significantly promoted economic and social development—consistent with the green development results—but appears to inhibit ecological development. However, the latter relationship is not statistically significant and gradually diminishes over time. Third, technological innovation and green innovation are the paths through which STFP facilitated urban green development. STFP promotes urban green development by improving green innovation, whereas technological innovation exerts a “masking effect” on the effects of STFP in pilot cities. Forth, heterogeneity analysis shows that STFP can significantly promote green development in any area, thereby confirming the stability of our main results. However, the effect of this policy shows regional heterogeneity and innovation heterogeneity. Specifically, STFP has had a stronger positive impact on green development in midwestern and high-innovation cities than in eastern and low-innovation cities.
Based on our research conclusions, we propose the following policy recommendations. First, local governments should pay attention to the positive externality of STFP on urban green development. Meanwhile, local governments should vigorously support investment in science and technology finance based on regional characteristics, aiming to ease the financing constraints of technological innovation enterprises (Hu et al., 2021). In addition, it is considered to include cities with a good innovation environment and rich financial resources in the scope of the policy pilot. When formulating policies to promote the green development of cities, policymakers should guide various social capitals toward actively supporting the green economy. Attention must be paid to coordination and linkages between cities so as to meet the requirements of harmonious regional development.
Second, considering that STFP has a time-varying influence on urban green development, local governments should formulate technological finance policies from a developmental and forward-looking perspective. In all stages of the policy implementation process, local governments should play a prominent guiding role by scientifically evaluating and flexibly using the policy’s advantages to maximize its contribution to urban sustainable green development (Li G. et al., 2021). In addition, the promulgation of policies should not be too hasty. Recognizing the time lag from implementation to maximizing utility, policymakers should avoid promulgating too many policies of the same nature in a short time.
Third, local governments should pay more attention to investing more in technology research and green innovation. By improving the level of talent training and enhancing the technological innovation service system, local governments can direct the flow of funds to core high-tech industries and push forward high-quality economic development. On the one hand, local governments should improve traditional industries’ quality, capacity, and efficiency through emerging technologies such as financial big data, blockchain, cloud computing, and artificial intelligence. The advantages brought by financial technology innovation can better serve urban green development. On the other hand, given the importance of green innovation to sustainable economic development, the government should encourage enterprises to shift the focus of innovation activities from traditional technology to green technology.
Finally, given the regional imbalances in economic development and innovation, differentiated policies should be formulated for combining technology and finance. The empirical results show that STFP plays a more prominent role in the green development of midwestern cities and high-innovation cities, which is partly explained by marginal utility. As each city has unique resource endowments and industrial characteristics, undifferentiated technological and financial policies waste resources and exacerbate the problem of unbalanced development.
Some study limitations must be considered when interpreting our findings. First, we could not comprehensively investigate the policy effect in cities in remote areas for which data of sufficient authenticity and integrity were unavailable. Second, cities included in the second batch of pilot projects beginning in 2016 were excluded from the main effect test, as they have only been affected by the policy for a short time. Therefore, the effectiveness of STFP in these cities was not evaluated, raising the need for further investigation. Future research could examine the impact of STFP on green development from a micro perspective, for example, by considering county-level or enterprise-level data. Moreover, it is important to explore multiple channels for the impact of STFP on green development.
Data Availability Statement
The original contributions presented in the study are included in the article/Supplementary Material; further inquiries can be directed to the corresponding author.
Author Contributions
CG: conceptualization, writing—original draft, and formal analysis; PS: data curation, methodology, and software; YW: visualization and investigation; and DY: writing—review and editing and supervision.
Funding
This study was funded by the Projects of National Social Science Fund of China (20FJLB010).
Conflict of Interest
The authors declare that the research was conducted in the absence of any commercial or financial relationships that could be construed as a potential conflict of interest.
Publisher’s Note
All claims expressed in this article are solely those of the authors and do not necessarily represent those of their affiliated organizations, or those of the publisher, the editors, and the reviewers. Any product that may be evaluated in this article, or claim that may be made by its manufacturer, is not guaranteed or endorsed by the publisher.
References
Abid, A., Mehmood, U., Tariq, S., and Haq, Z. U. (2021). The Effect of Technological Innovation, FDI, and Financial Development on CO2 Emission: Evidence from the G8 Countries. Environ. Sci. Pollut. Res. 29 (8), 11654–11662. doi:10.1007/s11356-021-15993-x
Acemoglu, D., and Johnson, S. (2007). Disease and Development: The Effect of Life Expectancy on Economic Growth. J. Political Econ. 115, 925–985. doi:10.1086/529000
Adom, P. K., and Amuakwa-Mensah, F. (2016). What Drives the Energy Saving Role of FDI and Industrialization in East Africa? Renew. Sustain. Energy Rev. 65, 925–942. doi:10.1016/j.rser.2016.07.039
Ahmad, M., Khan, Z., Rahman, Z. U., Khattak, S. I., and Khan, Z. U. (2021). Can Innovation Shocks Determine CO2 Emissions (CO2e) in the OECD Economies? A New Perspective. Econ. Innovation New Technol. 30 (1), 89–109. doi:10.1080/10438599.2019.1684643
Ahmed, F., Kousar, S., Pervaiz, A., Trinidad-Segovia, J. E., del Pilar Casado-Belmonte, M., and Ahmed, W. (2022). del Pilar Casado-Belmonte, M., and Ahmed, WRole of Green Innovation, Trade and Energy to Promote Green Economic Growth: A Case of South Asian Nations. Environ. Sci. Pollut. Res. 29 (5), 6871–6885. doi:10.1007/s11356-021-15881-4
Bagheri, M., Guevara, Z., Alikarami, M., Kennedy, C. A., and Doluweera, G. (2018). Green Growth Planning: A Multi-Factor Energy Input-Output Analysis of the Canadian Economy. Energy Econ. 74, 708–720. doi:10.1016/j.eneco.2018.07.015
Baloch, M. A., Zhang, J., Iqbal, K., and Iqbal, Z. (2019). The Effect of Financial Development on Ecological Footprint in BRI Countries: Evidence from Panel Data Estimation. Environ. Sci. Pollut. Res. 26 (6), 6199–6208. doi:10.1007/s11356-018-3992-9
Balsa-Barreiro, J., Li, Y., Morales, A., and Pentland, A. S. (2019). Globalization and the Shifting Centers of Gravity of World's Human Dynamics: Implications for Sustainability. J. Clean. Prod. 239, 117923. doi:10.1016/j.jclepro.2019.117923
Baron, R. M., and Kenny, D. A. (1986). The Moderator-Mediator Variable Distinction in Social Psychological Research: Conceptual, Strategic, and Statistical Considerations. J. Personality Soc. Psychol. 51, 1173–1182. doi:10.1037/0022-3514.51.6.1173
Bernier, M., and Plouffe, M. (2019). Financial Innovation, Economic Growth, and the Consequences of Macroprudential Policies. Res. Econ. 73, 162–173. doi:10.1016/j.rie.2019.04.003
Brown, J. R., Martinsson, G., and Petersen, B. C. (2017). What Promotes R&D? Comparative Evidence from Around the World. Res. Policy 46, 447–462. doi:10.1016/j.respol.2016.11.010
Burns, B. L., Barney, J. B., Angus, R. W., and Herrick, H. N. (2016). Enrolling Stakeholders under Conditions of Risk and Uncertainty. Strategic Entrepreneursh. J. 10 (1), 97–106. doi:10.1002/sej.1209
Cao, S., Nie, L., Sun, H., Sun, W., and Taghizadeh-Hesary, F. (2021). Digital Finance, Green Technological Innovation and Energy-Environmental Performance: Evidence from China's Regional Economies. J. Clean. Prod. 327, 129458. doi:10.1016/j.jclepro.2021.129458
Commission on Sustainable Development (2001). Report on the Ninth Session. New York: United Nations Economic and Social Council.
Dai, H., Xie, X., Xie, Y., Liu, J., and Masui, T. (2016). Green Growth: The Economic Impacts of Large-Scale Renewable Energy Development in China. Appl. Energy 162, 435–449. doi:10.1016/j.apenergy.2015.10.049
Destek, M. A., and Manga, M. (2021). Technological Innovation, Financialization, and Ecological Footprint: Evidence from BEM Economies. Environ. Sci. Pollut. Res. 28, 21991–22001. doi:10.1007/s11356-020-11845-2
Dietz, T., and Rosa, E. A. (1994). Rethinking the Environmental Impacts of Population, Affluence and Technology. Hum. Ecol. Rev. 1 (2), 277–300.
Fang, H. T. (2015). Essence of Sci-Tech Finance. Forum Sci. Technol. China 5, 5–10. doi:10.13580/j.cnki.fstc.2015.05.002
Fong, W., Sun, Y., and Chen, Y. (2022). Examining the Relationship between Energy Consumption and Unfavorable CO2 Emissions on Sustainable Development by Going through Various Violated Factors and Stochastic Disturbance-Based on a Three-Stage SBM-DEA Model. Energies 15, 569. doi:10.3390/en15020569
Gu, J. H., and Chai, H. Q. (2021). Has the Science and Technology Finance Promoted Green Innovation: Evidence from the Pilot Policy of Combining Science and Technology with Finance. Sci. Technol. Progr. Policy 11, 1–8.
Guo, Y., and Liu, Y. (2022). Sustainable Poverty Alleviation and Green Development in China's Underdeveloped Areas. J. Geogr. Sci. 32, 23–43. doi:10.1007/s11442-021-1932-y
Hu, D. L., Shen, H., and Liu, Z. M. (2019). Study on the Spatial-Temporal Evolution and Formation Mechanism of Energy Eebound Effect in Chinese Cities. Chin. Soft Sci. 11, 96–108. doi:10.3390/rs11242933
Hu, H., and Liu, C. (2021). Whether Science and Technology Finance Policy Promote the Transformation and Upgrading of Industrial Structure? Stud. Inter. Fin. 5, 24–33. doi:10.16475/j.cnki.1006-1029.2021.05.003
Hu, S., Zeng, G., Cao, X., Yuan, H., and Chen, B. (2021). Does Technological Innovation Promote Green Development? A Case Study of the Yangtze River Economic Belt in China. Int. J. Environ. Res. Public Health 18, 6111. doi:10.3390/ijerph18116111
Huang, Q., and Liu, M. (2021). Trade Openness and Green Total Factor Productivity: Testing the Role of Environment Regulation Based on Dynamic Panel Threshold Model. Environ. Dev. Sustain 24, 9304–9329. doi:10.1007/s10668-021-01825-y
Hübler, M., and Keller, A. (2010). Energy Savings via FDI? Empirical Evidence from Developing Countries. Envir. Dev. Econ. 15 (1), 59–80. doi:10.1017/S1355770X09990088
Kihombo, S., Ahmed, Z., Chen, S., Adebayo, T. S., and Kirikkaleli, D. (2021). Linking Financial Development, Economic Growth, and Ecological Footprint: What Is the Role of Technological Innovation? Environ. Sci. Pollut. Res. 28 (43), 61235–61245. doi:10.1007/s11356-021-14993-110.1007/s11356-021-14993-1
King, R. G., and Levine, R. (1993). Finance, Entrepreneurship and Growth. J. Monetary Econ. 32 (3), 513–542. doi:10.1016/0304-3932(93)90028-E
Kogan, L., Papanikolaou, D., Seru, A., and Stoffman, N. (2017). Technological Innovation, Resource Allocation, and Growth*. Q. J. Econ. 132 (2), 665–712. doi:10.1093/qje/qjw040
Li, C., Wan, J., Xu, Z., and Lin, T. (2021). Impacts of Green Innovation, Institutional Constraints and Their Interactions on High-Quality Economic Development across China. Sustainability 13 (9), 5277. doi:10.3390/su13095277
Li, Y., Chen, Y., and Li, Q. (2020). Assessment Analysis of Green Development Level Based on S-type Cloud Model of Beijing-Tianjin-Hebei, China. Renew. Sustain. Energy Rev. 133, 110245. doi:10.1016/j.rser.2020.110245
Li, G., Zhou, Y., Liu, F., and Wang, T. (2021). Regional Differences of Manufacturing Green Development Efficiency Considering Undesirable Outputs in the Yangtze River Economic Belt Based on Super-SBM and WSR System Methodology. Front. Environ. Sci. 8, 631911. doi:10.3389/fenvs.2020.631911
Liu, B., Zuo, Q., and Diao, Y. (2021). The Value and Pathways of Green Technology Innovation for Ecological Conservation and High-Quality Development of the Yellow River Basin. Resour. Sci. 43 (02), 423–432. doi:10.18402/resci.2021.02.19
Liu, J., Jiang, Y., Gan, S., He, L., and Zhang, Q. (2022). Can Digital Finance Promote Corporate Green Innovation? Environ. Sci. Pollut. Res. 29, 35828–35840. doi:10.1007/s11356-022-18667-4
Liu, K., and Lin, B. (2019). Research on Influencing Factors of Environmental Pollution in China: A Spatial Econometric Analysis. J. Clean. Prod. 206, 356–364. doi:10.1016/j.jclepro.2018.09.194
Liu, X., Li, Y., Chen, X., and Liu, J. (2022). Evaluation of Low Carbon City Pilot Policy Effect on Carbon Abatement in China: An Empirical Evidence Based on Time-Varying DID Model. Cities 123, 103582. doi:10.1016/j.cities.2022.103582
Liu, Y., Liu, M., Wang, G., Zhao, L., and An, P. (2021). Effect of Environmental Regulation on High-Quality Economic Development in China-An Empirical Analysis Based on Dynamic Spatial Durbin Model. Environ. Sci. Pollut. Res. 28, 54661–54678. doi:10.1007/s11356-021-13780-2
Ma, L. Y., and Li, X. M. (2019). Does Science and Technology Finance Policies Promote Regional Innovation? Quasi-Natural Experiment Based on the Pilot Policy of Combining Science and Technology with Finance. Chin. Soft Sci. 33, 30–42.
Moutinho, V., Madaleno, M., and Robaina, M. (2017). The Economic and Environmental Efficiency Assessment in EU Cross-Country: Evidence from DEA and Quantile Regression Approach. Ecol. Indic. 78, 85–97. doi:10.1016/j.ecolind.2017.02.042
Nathaniel, S. P., Murshed, M., and Bassim, M. (2021). The Nexus between Economic Growth, Energy Use, International Trade and Ecological Footprints: The Role of Environmental Regulations in N11 Countries. Energy Ecol. Environ. 6 (6), 496–512. doi:10.1007/s40974-020-00205-y
Pan, X., Cheng, W., Gao, Y., Balezentis, T., and Shen, Z. (2021). Is Environmental Regulation Effective in Promoting the Quantity and Quality of Green Innovation? Environ. Sci. Pollut. Res. 28, 6232–6241. doi:10.1007/s11356-020-10984-w
Porter, M. E. (1992). Capital Disadvantage: America's Failing Capital Investment System. Harv Bus. Rev. 70 (5), 65–82. doi:10.1108/eb022838
Pradhan, R. P., Arvin, M. B., Hall, J. H., and Nair, M. (2016). Innovation, Financial Development and Economic Growth in Eurozone Countries. Appl. Econ. Lett. 23 (16), 1141–1144. doi:10.1080/13504851.2016.1139668
Qian, Y., Liu, J., and Forrest, J. Y.-L. (2021). Impact of Financial Agglomeration on Regional Green Economic Growth: Evidence from China. J. Environ. Plan. Manag. 65, 1611–1636. doi:10.1080/09640568.2021.1941811
Reid, H., Simms, A., and Johnson, V. (2007). “Up in Smoke? Asia and the Pacific,” in Fifth Report of the Working Group on Climate Change and Development (London: New Economics Foundation).
Rosenbaum, P. R., and Rubin, D. B. (1983). The Central Role of the Propensity Score in Observational Studies for Causal Effects. Biometrika 70, 41–55. doi:10.1093/biomet/70.1.41
Samila, S., and Sorenson, O. (2011). Venture Capital, Entrepreneurship, and Economic Growth. Rev. Econ. Statistics 93, 338–349. doi:10.1162/rest_a_00066
Schumpeter, J. A. (1912). The Theory of Economy Development. Cambridge M A: Harvard University Press.
Shahbaz, M., Shafiullah, M., Khalid, U., and Song, M. (2020). A Nonparametric Analysis of Energy Environmental Kuznets Curve in Chinese Provinces. Energy Econ. 89, 104814. doi:10.1016/j.eneco.2020.104814
Shao, S., Tian, Z., and Fan, M. (2018). Do the Rich Have Stronger Willingness to Pay for Environmental Protection? New Evidence from a Survey in China. World Dev. 105, 83–94. doi:10.1016/j.worlddev.2017.12.033
Shen, L., He, G., and Yan, H. (2022). Research on the Impact of Technological Finance on Financial Stability: Based on the Perspective of High-Quality Economic Growth. Complexity 2022, 15. doi:10.1155/2022/2552520
Sheng, N., and Tang, U. W. (2016). The First Official City Ranking by Air Quality in China - A Review and Analysis. Cities 51, 139–149. doi:10.1016/j.cities.2015.08.012
Sheng, X., Lu, B., and Yue, Q. (2021). Impact of Sci-Tech Finance on the Innovation Efficiency of China's Marine Industry. Mar. Policy 133, 104708. doi:10.1016/j.marpol.2021.104708
Shobande, O. A., and Ogbeifun, L. (2022). The Criticality of Financial Development and Energy Consumption for Environmental Sustainability in OECD Countries: Evidence from Dynamic Panel Analysis. Int. J. Sustain. Dev. World Ecol. 29 (2), 153–163. doi:10.1080/13504509.2021.1934179
Sun, C., Tong, Y., and Zou, W. (2018). The Evolution and a Temporal-Spatial Difference Analysis of Green Development in China. Sustain. Cities Soc. 41, 52–61. doi:10.1016/j.scs.2018.05.006
Tahir, T., Luni, T., Majeed, M. T., and Zafar, A. (2021). The Impact of Financial Development and Globalization on Environmental Quality: Evidence from South Asian Economies. Environ. Sci. Pollut. Res. 28 (7), 8088–8101. doi:10.1007/s11356-020-11198-w
Usman, M., and Hammar, N. (2021). Dynamic Relationship between Technological Innovations, Financial Development, Renewable Energy, and Ecological Footprint: Fresh Insights Based on the STIRPAT Model for Asia Pacific Economic Cooperation Countries. Environ. Sci. Pollut. Res. 28 (12), 15519–15536. doi:10.1007/s11356-020-11640-z
Wang, M.-X., Zhao, H.-H., Cui, J.-X., Fan, D., Lv, B., Wang, G., et al. (2018). Evaluating Green Development Level of Nine Cities within the Pearl River Delta, China. J. Clean. Prod. 174, 315–323. doi:10.1016/j.jclepro.2017.10.328
Wang, M., and Feng, C. (2021). The Win-Win Ability of Environmental Protection and Economic Development during China's Transition. Technol. Forecast. Soc. Change 166, 120617. doi:10.1016/j.techfore.2021.120617
Wang, Q., and Yi, H. (2021). New Energy Demonstration Program and China's Urban Green Economic Growth: Do Regional Characteristics Make a Difference? Energy Policy 151, 112161. doi:10.1016/j.enpol.2021.112161
Wang, S., and Gu, S. (2021). Research on the Impact of S&T Finance on the High-Quality Development of Chinese Economy—Theoretical Analysis and Empirical Test. Economist 2, 81–91. doi:10.16158/j.cnki.51-1312/f.2021.02.009
Wen, Z., and Ye, B. (2014). Analyses of Mediating Effects: The Development of Methods and Models. Adv. Psychol. Sci. 22 (5), 731. doi:10.3724/SP.J.1042.2014.00731
Xie, W., and Li, X. (2021). Can Industrial Agglomeration Facilitate Green Development? Evidence from China. Front. Environ. Sci. 9, 745465. doi:10.3389/fenvs.2021.745465
Xu, Y., Dong, B., and Chen, Z. (2021a). Can Foreign Trade and Technological Innovation Affect Green Development: Evidence from Countries along the Belt and Road. Econ. Change Restruct. 55, 1063–1090. doi:10.1007/s10644-021-09337-5
Xu, Y., Li, T., and Lu, L. (2021b). Research on the Effect of the Pilot Policy of Combining Technology and Finance on Regional Economic Growth: Based on the Perspective of Technological Innovation and Industrial Structure Rationalization. J. Chongqing Univ. 27, 1–15. doi:10.11835/j.issn.1008-5831.jg.2021.03.004
Yan, Y., and Wu, Z. (2020). Regional Innovation Distribution and its Dynamic Evolution: Policy Impact and Spillover Effect-Based on the Perspective of Innovation Motivation. PLoS One 15 (7), e0235828. doi:10.1371/journal.pone.0235828
Yasin, I., Ahmad, N., and Chaudhary, M. A. (2020). Catechizing the Environmental-Impression of Urbanization, Financial Development, and Political Institutions: A Circumstance of Ecological Footprints in 110 Developed and Less-Developed Countries. Soc. Indic. Res. 147 (2), 621–649. doi:10.1007/s11205-019-02163-3
York, R., Rosa, E. A., and Dietz, T. (2003). STIRPAT, IPAT and ImPACT: Analytic Tools for Unpacking the Driving Forces of Environmental Impacts. Ecol. Econ. 46 (3), 351–365. doi:10.1016/S0921-8009(03)00188-5
Yu, Y. Z., and Zhang, S. H. (2017). Urban Housing Prices, Purchase Restriction Policy and Technological Innovation. Chin. Ind. Econ. 6, 98–116. doi:10.19581/j.cnki.ciejournal.2017.06.020
Yuan, H., Zhang, T., Feng, Y., Liu, Y., and Ye, X. (2019). Does Financial Agglomeration Promote the Green Development in China? A Spatial Spillover Perspective. J. Clean. Prod. 237, 117808. doi:10.1016/j.jclepro.2019.117808
Yuan, Z. M., Li, T., and Jia, Y. H. (2018). Research on Technological Finance Policy Implementation: Factors and Model Building—Questionnaire and Case Study. Res. Econ. Manag. 39 (4), 55–66. doi:10.13502/j.cnki.issn1000-7636.2018.04.005
Yue, S., Lu, R., Chen, H., and Yuan, J. (2018). Does Financial Development Promote the Win-Win Balance between Environmental Protection and Economic Growth? Environ. Sci. Pollut. Res. 25, 36438–36448. doi:10.1007/s11356-018-3549-y
Zhang, D., Mohsin, M., Rasheed, A. K., Chang, Y., and Taghizadeh-Hesary, F. (2021). Public Spending and Green Economic Growth in BRI Region: Mediating Role of Green Finance. Energy Policy 153, 112256. doi:10.1016/j.enpol.2021.112256
Zhang, G., Deng, N., Mou, H., Zhang, Z. G., and Chen, X. (2019). The Impact of the Policy and Behavior of Public Participation on Environmental Governance Performance: Empirical Analysis Based on Provincial Panel Data in China. Energy Policy 129, 1347–1354. doi:10.1016/j.enpol.2019.03.030
Zhang, W., and Chiu, Y.-B. (2020). Do Country Risks Influence Carbon Dioxide Emissions? A Non-Linear Perspective. Energy 206, 118048. doi:10.1016/j.energy.2020.118048
Zhang, Y. H., and Zhang, T. (2018). Research on Impact of Sci-Tech Finance on the Co-Agglomeration of Producer Service and Manufacturing Industry. Chin. Soft Sci. 3, 47–55.
Zhang, Z.-X. (2007). China Is Moving Away the Pattern of “Develop First and Then Treat the Pollution”. Energy Policy 35, 3547–3549. doi:10.1016/j.enpol.2007.02.002
Zheng, S. M., Wu, Y. J., and Zhou, K. (2020). Research on Policy Effect the Integration of Technology and Finance: Based on DID Model. Chin. Soft Sci. 1, 49–58.
Zheng, Y., Chen, S., Chen, S., and Wang, N. (2020). Does Financial Agglomeration Enhance Regional Green Economy Development? Evidence from China. Green Financ. 2, 173–196. doi:10.3934/GF.2020010
Zhu, B., Zhang, M., Huang, L., Wang, P., Su, B., and Wei, Y.-M. (2020). Exploring the Effect of Carbon Trading Mechanism on China's Green Development Efficiency: A Novel Integrated Approach. Energy Econ. 85, 104601. doi:10.1016/j.eneco.2019.104601
Keywords: science and technology finance policy, green development, technological innovation, green innovation, China, DID method
Citation: Gao C, Song P, Wen Y and Yang D (2022) Effect of Science and Technology Finance Policy on Urban Green Development in China. Front. Environ. Sci. 10:918422. doi: 10.3389/fenvs.2022.918422
Received: 12 April 2022; Accepted: 23 June 2022;
Published: 26 July 2022.
Edited by:
Jose Balsa, Massachusetts Institute of Technology, United StatesReviewed by:
Elchin Suleymanov, Baku Engineering University, AzerbaijanCüneyt Dumrul, Erciyes University, Turkey
Copyright © 2022 Gao, Song, Wen and Yang. This is an open-access article distributed under the terms of the Creative Commons Attribution License (CC BY). The use, distribution or reproduction in other forums is permitted, provided the original author(s) and the copyright owner(s) are credited and that the original publication in this journal is cited, in accordance with accepted academic practice. No use, distribution or reproduction is permitted which does not comply with these terms.
*Correspondence: Chen Gao, gaoc9206@163.com