Drivers of the European Union’s Environmental Performance
- 1Department of Agrifood and Environmental Economics, The Faculty of Agrifood and Environmental Economics, The Bucharest University of Economic Studies, Bucharest, Romania
- 2Department of Finance, The Faculty of Finance and Banking, The Bucharest University of Economic Studies, Bucharest, Romania
The interactions between nature and humankind produce a series of ecological, social, economic, institutional, technological, and other types of restrictions, which can alter the development of the future society and Earth. Exploring the influencing factors of environmental performance can create better solutions and it can emphasize the gaps from past and current policies. Also, they can contribute to achieve sustainable development. In this regard, more indices are developing for assessing a specific theme that targets sustainability, such as carbon footprint, Environmental Performance Index, or sustainable development index. Thus, this study aims to analyze the influencing factors of Environmental Performance Index (EPI) at an EU level by collecting data between 2010 and 2020, once in 2 years and by using a panel data model. The results were estimated using the Period SUR effect within the generalized least square method and using the White period matrix within the correlation matrix. The results indicate positive relationships between EPI and area under organic farming, circular material use rate, eco-innovation index, energy productivity, ratio of female-to-male labor force participation rate, forest area, Human Development Index, Internet users, livestock production index, new business density, patent applications-residents, tertiary school enrollment, the share of renewable energy consumption in gross final energy consumption, and the proportion of seats held by women in national parliaments. In addition, higher inequality between individuals, natural resources rents, trade volume index, and environmental taxes in total tax revenues seem to constrain the EU’s environmental performance. The results are important both for academicians and policymakers.
Introduction
The twenty-first century came with the settlement of more ambitious political and policy goals for improving the quality of the environment and the welfare of the biodiversity, while increasing the state of the socioeconomic dimension, by promoting sustainable development. Thus, all economic models aimed at the transition to sustainability. Overall, these models aim to prevent, control, and, where possible, resolve planetary problems, such as, energy crises, highly generated and wrongly disposed waste, resource depletion, questionable human and animal welfare, pollution, bad diets, and so on.
Recently, more and more organizations are developing assessment and monitoring macro indices on issues such as environmental performance, eco-innovation, human welfare, and circular economy (SEDAC 2020; UNDP United Nations Development Programme 2020; Eurostat 2022). These are trying to capture several dimensions and themes of sustainability through a numerical value, which gives an overview of a certain space during a certain historical time. In addition, some of them indicate the achievement of objectives and targets established by international organizations in tackling the problems mentioned earlier or simply in informing the society about the state of an issue. For example, the Environmental Performance Index (SEDAC 2020; Wendling et al., 2020) tries to capture the ecosystem vitality and the environmental health at the country level for hierarchy purposes.
Furthermore, the correlations between macro indicators and indices on different subjects (Jorgenson and Clark, 2011; Liu, 2014; Narula and Reddy, 2015; Zaharia, 2017) are slowly starting to be of interest for emphasizing the drivers, which might influence more or less the transition toward sustainable development of a country through environmental, social, and economic considerations. However, only some studies (Samimi et al., 2011; Shahabadi et al., 2017; Zaharia 2017- see literature review) focus on understanding the drivers of Environmental Performance Index (EPI), from perspectives and periods other than those discussed in this article. For example, Samimi et al. (2011) analyzed the relationship between EPI and Human Development Index (HDI) for 114 countries during 2006 and 2010 and concluded that there is a significant and positive relationship between Human Development Index and Environmental Performance Index for all countries included into the analysis.
The aim of this article is to assess and to discuss the drivers of Environmental Performance Index (EPI) at the EU level in order to detect which factors better affect its evolution and which have a negative effect. We investigated the impact of HDI, GINI index, eco-innovation index, circular material use rate, forest area, area under organic farming, heating days, environmental taxes, natural resources rents, renewable energy consumption, energy productivity, new business density, Internet users, patent applications, trade volume index, women impact, and tertiary education on EPI. Although, the impact of HDI on EPI was tested on different time frames, we also considered it into this analysis for better indicating the influence of human welfare on the environment at the EU level. The article is structured in several parts. First, we discussed the specific scientific information on the uses of Environmental Performance Index or other indices in explaining macro relationships and their implications on society and nature by using econometric techniques. Second, we explained the methodological framework for this research, which is based on panel data modeling. Third, we emphasized the results of the factors influencing the environmental performance of EU’s countries, and we discussed the implication on policies for sustainable development of society. In the end, we concluded with highlighting the main key outcomes of our research, pointing out further research and presenting the main implications for both practitioners and academic community.
Literature Review
The role of examining the state and the evolution of the constraints within the nature–society system on one or more of its components, using indices and indicators that are related to different geographical areas, is to provide options for improving current public policies for the future (Meadows et al., 1972). For instance, Rehman Khan S. A. R. et al. (2021) acknowledge the importance of technology and incentives in developing circular economy at the firm level. Furthermore, Haider et al. (2021) emphasize the impact of economic growth on intensifying environmental degradation, but sustainable resource consumption could be achieved by good governance and political stability. Also, adopting renewable energy in strategical measures for transportation and production sectors could enhance resource efficiency (Yu et al., 2022). So, the challenges posed by the interactions between the economy, social structure, and environment, such as pollution, lack of energy, food and water, degradation of natural ecosystems, and depletion of energy resources intensify ultimately affect the development of society and, perhaps, in the long run, the survival of humankind. Thus, significantly increased publications focus on understanding the relationship between nature and society by analyzing indicators at the macro and micro levels. Looking at microeconomic analyses, it seems that progress was obtained in environmental performance through digital technology and institutional regulations for the environment (Khan H. et al., 2021). On other hand, macroeconomic analyses suggest that a healthy ecosystem could generate good environmental services for society (Lai and Chen, 2020), government effectiveness positively impact energy consumption (Haider et al., 2021), the use of renewable energy improves sustainability by controlling oil consumption (Yu et al., 2022), and foreign direct investments should be used in sustainable businesses only for enhancing sustainability (Khan et al., 2022). However, there is still a gap in regarding the link between indices and indicators that look at different environmental, social, or economic features.
Several studies report different global hierarchies according to these indices, based on more or less subjective methodologies created by international organizations or researchers targeting different fields.
The United Nations Development Program (UNDP United Nations Development Programme 2019; UNDP United Nations Development Programme 2020) proposes the Human Development Index (HDI) to assess social welfare by including the index variables related to education, standard of living, and public health of a country. The hierarchy created allows the comparison of social welfare at the country level. The data on HDI reveal that EU member states were all ranked with a very high HDI in 2019 compared to 2010, when only Bulgaria registered a lower score which is high HDI. The highest ranked countries were Germany, Netherlands, and Denmark in 2010 and Ireland, Germany, and Sweden in 2019. Simultaneously, the lowest ranked countries were Bulgaria, Romania, and Croatia in both 2010 and 2019. Also, in average, the EU registered 3.46% increase of HDI in 2019 compared to 2010, while Lithuania, Ireland, and Latvia had the highest growth of HDI in this period of time.
Another important index is the environmental performance index (EPI) that assesses the vitality of ecosystems and environmental health once in 2 years, with EU member states having scores over 57 in 2020 and over 56 in 2010 (SEDAC 2020; Wendling et al., 2020). According to the data, the highest ranked countries were Sweden, France, and Austria in 2010 and Denmark, Luxemburg, and France in 2020. Simultaneously, the lowest ranked countries were Cyprus, Belgium, and Greece in 2010 and Bulgaria, Poland, and Latvia in 2020. In average, the EU registered 2.23% increase of EPI in 2020 compared to 2010, while Belgium, Luxemburg, and Denmark had the highest growth of EPI, over 15% in this period of time. In contrast with HDI, 13 member states had also decreases of EPI in time, and the changes were considerable, with percentages of over 15% for seven countries and over 7% for nine states.
In addition, the Eco-innovation Observatory (2022) developed the eco-innovation index, which aims to compare the EU’s member states in terms of eco-innovation inputs, activities, resource efficiency, and socioeconomic outcomes. The data revealed that the highest ranked countries were Denmark (149), Sweden (143), and Finland (139) in 2010 and Luxemburg (165), Denmark (146), and Finland (145) in 2019. Simultaneously, the lowest ranked countries were Bulgaria (31), Poland (40), Slovakia (43), and Greece (43) in 2010 and Bulgaria (34), Hungary (54), and Cyprus (56) in 2019. Also, 11 countries surpassed the 100 score in 2010, while, in 2019, only ten member states did so. However, we observe an overall improvement overtime in the eco-innovation index, as its value was higher for all countries in 2019 compared to 2010. The average score of EU27 states increased slowly in the past years, reaching the value of 94.
According to Popescu et al. (2017), aggregate indices explain a complex subject by a single value, which was previously evaluated by several indicators, in order to observe historical performance to simulate future developments. However, there are certain limitations of these indices, which are related to the definition, perception, and complexity of sustainable concepts, as well as more technical aspects of their computation regarding consistency, standardization, different evaluation results, and uncertainty of data used or generated (Čuček et al., 2012; Liu 2014; Narula and Reddy 2015). However, the indices are becoming increasingly relevant in comparing environmental issues in different countries and have methodological improvements in order to highlight national best practices (Popescu et al., 2017). With the development and continuous improvement of indices in different fields, research aimed at analyzing them in relation to other indicators or indices that simplistically report the state at a given time and on certain area issues. At such, the study of Jorgenson and Clark (2011) propose the investigation of the determinants of the ecological footprint based on 5-year intervals panel data between 1960 and 2003 and concluded that political economic factors together with ecological and structural associations between countries can influence the environmental and ecological conditions.
Even though many studies focus on economic performance and economic sustainability, the literature regarding the influence on Environmental Performance Index is still limited. Some studies have been conducted in different regions and different periods of time, considering different factors that could affect it. The main relationship on which previous research focused was the relationship between Human Development Index and Environmental Performance Index.
Samimi et al. (2011) demonstrate the positive impact of HDI on EPI in 28 developed countries by using a panel data regression during 2006 and 2010. In the same time, a negative influence of HDI on EPI was found in the analyzed 86 developing countries.
Also, Maccari (2014) demonstrates a U-shaped impact of HDI on EPI for 129 countries in 2012 using a model based on quadratic equation. However, Shahabadi et al. (2017) indicate inconclusive results on the influence of HDI on EPI in OPEC countries during 2000 and 2012 using a panel model as the way of research. In the meantime, Arfanuzzaman (2016) indicates a negative impact of per capita income on EPI, but a positive impact of CO2 emissions and HDI on EPI for Bangladesh during 2000 and 2013.
Ghodrati et al. (2018) made an investigation of the relationships between EPI and democracy in different regions, according to the dimension of their HDI: very high, high, medium, and low. They (Ghodrati et al., 2018) found that per capita income has an N-shape relationship with EPI, suggesting mixed results depending on the HDI level of the group of countries considered.
Jain and Nagpal (2019) indicate a positive influence of HDI on EPI in five South Asian countries during 2002 and 2016 proving that higher HDI leads to smaller damage to the environment and to a better environmental performance.
The study of Lai and Chen (2020) is extremely important as they avoid the problem of weighted components of HDI and EPI. More precisely, they emphasize the cross relationship among individual dimensions of EPI and HDI, proving that the education is the main factor that affect environmental management and that promote environmental sustainability.
Not only the relationship between HDI and EPI but also the link between EPI and other indicators was analyzed, as it can be observed in the following section.
Hsu et al. (2013) show the correlations between HDI without gross domestic product, GDP, corruption index, public accountability index, and EPI in 2010 globally, highlighting the tendency of rich countries to perform poorer in energy policy-climate change.
Using a panel econometric model approach, Chakraborty and Mukherjee (2013) demonstrate the negative influence of financial development, merchandise export, and democracy indices on EPI, as well as the positive impact of economic growth, the hybrid HDI, services export, and the corruption index on EPI in 114 countries between 2000 and 2010.
Another important research is the one realized by Gallego-Alvarez et al. (2014) on 149 countries who looked at the influence of socioeconomic factors, such as economic wealth, education, and the control of corruption variable on EPI, which prove to be determinant factors of environmental performance. On the other hand, the internal characteristics of a country or the political factors have no statistically significant effect on environmental performance.
Liu et al. (2017) analyze the influences of different environmental and human development performance indices with socioeconomic indicators at the level of 100 countries grouped by regions, including Europe, and at three points in time, namely, the years 2000, 2004, and 2008. This study (Liu et al., 2017) indicates high values for previously created EPI named EmSi, HDI, and the environmental footprint in countries with high GDP, and the existence of a high HDI in countries under good environmental conditions. These conditions were assessed by environmental health, an indicator that is part of EPI. Furthermore, another research (Chowdhury and Islam, 2017) based on Pearson correlation test indicates a negative impact of GDP growth rate on EPI for BRICS countries during 2008–2016, once in 2 years.
The study of Shahabadi et al. (2017) showed that governance, fuel exports in total merchandize exports seen as resource abundance index, and Internet users positively impact EPI, while trade openness and CO2 emissions per GDP negatively influence EPI in OPEC countries.
The study of Fakher and Abebi (2017) look at the impact of environmental quality, measured through the Environmental Performance Index, the direct foreign investment, and trade openness on economic growth, using data collected from International Financial Statistic Yearbook involving the period 1983–2013. The result reveals a positive and significant impact of EPI on economic growth.
Moreover, some studies (Popescu et al., 2017) address the causality between different indices by using non-parametric techniques to confirm the correlations between them in order to compare states, demonstrating the existence of bidirectional influences between EPI and other indices and indicators.
Among recent studies, we can mention the ones conducted by Pimonenko et al. (2018), Halkos and Zisiadou (2018), Ghodrati et al., 2018, Pourali et al. (2019), Jain and Nagpal (2019), Raza et al. (2021), Li et al. (2021), Mance et al. (2021), and Nogueira and Madaleno (2021).
Pimonenko et al. (2018) proved that countries that are high ranked on environmental performance index have a high sustainable development goal index and a high social progress index, while countries with huge level of CO2 emissions should improve their environmental policy.
Another research was conducted by Halkos and Zisiadou (2018) where the EPI is analyzed in relationship with different cultural and socioeconomic variables. The authors (Halkos and Zisiadou, 2018) observed an inverted U-shape form between the EPI and the economic growth for Globe and Middle East and Africa and an N-shape for Europe, while the results were not statistically significant for Asia and Oceania. The population density is in a negative relationship with EPI. On the other hand, masculinity and power distance are statistically insignificant with individualism and uncertainty avoidance, affecting positively Asia and Oceania and negatively Middle East, Africa, and Europe. Long-term orientation affects negatively Asia and Oceania while indulgence influences negatively Asia, Oceania, and the Globe.
In another study, it seems that both the democracy level and stock level positively impact EPI in countries with very high, high, and medium HDI, while the level of democracy had a negative influence on EPI in nations with low HDI during 2002 and 2012 (Ghodrati et al., 2018).
In their study, Jain and Nagpal (2019) suggest that urbanization, energy use, and population density negatively impacts EPI in five South Asian nations during 2002 and 2016.
Pourali et al. (2019) demonstrated a positive influence of GDP per capita on EPI for 101 countries during 2005–2015, same relationship being reported between trade openness, political–civil liberties, good governance, government effectiveness, rule of law, and EPI. Their study found similar results for the governance impact on EPI, like the one conducted by Shahabadi et al. (2017), but contrary ones for trade openness, which impacts EPI positively in the case of all 101 analyzed countries.
The research conducted by Raza et al. (2021) aims to examine the link between environmental performance in South and East Asian countries and variables that are associated with trade and other economic indicators. The analysis was conducted for 15 years, from 2002 to 2016, using the panel regression technique. The results reveal a negative relationship between trade liberalization and EPI, which points out that countries with higher international trade are more inclined to have bad environmental quality.
Another important study is the research conducted by Li et al. (2021) that proved the factors which drive the environmental performance index in Asian countries. The analysis is conducted on 34 Asian countries, with data from 2006 to 2017. Among independent variables are GDP per capita, fertilizer consumption, renewable energy use, forest area, and food production index. The results reveal positive significance between forest area and EPI and renewable energy use and EPI, and negative significance both between the food production index and fertilizer use and dependent variable EPI.
In addition to these studies, it is interesting to consult the research of Mance et al. (2021), who investigates the relationship of HDI on two indices related to EPI, namely, the protected species index and the terrestrial protected areas index. Also, the research of Nogueira and Madaleno (2021) include EPI as an influencing factor of GDP per capita and of GINI index. Thus, it seems that EPI positively affects GDP per capita, but, although with positive coefficient, it has no statistical significance on GINI index (Nogueira and Madaleno 2021).
Table 1 summarizes the variables, methodological approaches, results, and the time on which the analysis was conducted, as well as the region and the source of the most relevant publications considered in this review that have the environmental performance index as a dependent variable, considering that the aim of the research is to reveal the factors that affect the EPI both in term of indexes and indicators.
Table 1 synthesizes research on the variables impacting the Environmental Performance Index using econometric techniques worldwide.
Therefore, it is observed that causal studies between EPI and other indices and indicators are expanding, as testing this relationship may be a support not only for the creators of sustainable public policies in any field, but also for representatives of companies and civil society. Moreover, there is a need to retest certain relationships between macro indices and indicators for the current period as some reveal opposite results. Furthermore, we can observe a lack of providing evidence of several drivers that affect the Environmental Performance Index and we aim to provide proper guidelines for its improvement (improvement of environmental performance). Hence, we aim to observe the influence of composed indices and macroeconomic and social indicators. The analysis is conducted on European members, having countries as cross-sectional observation and time as a proxy for time observations.
Methodology
The purpose of this research is to provide evidence about the link between Environmental Performance Index and several variables related with macro socioeconomic indicators and indices of EU member states that can affect the sustainable development of these countries. Furthermore, we propose some guidelines on environmental policy, considering the results found. The methodology on which the relationship between them was determined is based on panel data analysis.
The multiple regressions allow the observation of the influence generated by the relations between the exogenous variables and the endogenous ones (Greene 2012: 52). In this context, the analysis proposes several models of multiple regressions with the data panel technique that allows estimating the fixed and dynamic effects of the investigated phenomenon and that takes into account the way the heteroskedasticity and autocorrelation is avoided. The analysis is conducted in EViews. The following stages were taken:
1) Providing information about the descriptive statistic of the variables included into the analysis.
2) Looking at the stationarity as panel data analysis includes time series. The stationarity can be conducted using the five tests available in EViews: Levin et al. (2002), Im et al. (2003), Dickey and Fuller’s (1981) improved the test called ADF (Phillips and Perron, 1988 and Breitung, 2000).
For further information on the advantages and disadvantages of these tests, see the publication of Mátyás and Sevestre (2008), and for guidance on the null hypotheses of each test and their interpretation, see Greene (2012: 982-1012) and Wooldridge (2002).
However, the data on which the analysis is conducted is considered to be a micro panel. In general, micro panels have data with a large number of cross-sectional observations (27 in our case) and have small time period (six periods in our case).
Baltagi (2008) reveals that unit root tests have small power of reliability when time observation is small and if a linear trend is included; the risk to conclude that all panel data series are stationary exists when only some series are stationary and it cannot be concluded which test provides a higher unbiased performance.
Based on the assumptions mentioned and due to low number of time observations, we assume that our panel data do not deal with stationarity problems. However, in order to be more specific, we conducted the individual stationarity of each series, but we avoided to put it into the article as the results are contradictory (some test provide information about the stationary of a series in level, while others reveal stationarity only at first difference) and are in line with Baltagi (2008). Moreover, as data are random selected-considering each country’s regulation, we expect to encounter small or lack of cross sectional dependences. On the other hand, it has to be mentioned that the presence of cross-sectional dependence (correlation of errors) in panel data that encounters economic information is rather a rule than an exception (Chudik and Pesaran 2013; Pesaran 2013).
When analyzing the results, we look at the cross-dependence test of the error. Even though more statistical tests are provided, the Monte Carlo simulation revealed that Pearson test provide better results than other cross-dependence tests, when the number of cross section observation is larger and the number of periods is low. However, the assumption regarding the large number of cross-sectional data can be challenged. On the other hand, we also provide information about cross-sectional dependence test Pearson scaled LM as it sometimes provides contradictory results than the cross-sectional dependence test of Pearson. These results are based on the dimension of the used database.
3) Testing the correlation between variables by making correlation matrices for avoiding multicollinearity effects as they create bias estimation results. In order to have reliable results, we took the same degrees of integration, as stated by Pao and Tsai (2011).
4) Estimating the multiple regressions to observe the main determinants and their action on EPI during 2010 and 2020, once in 2 years.
In order to conduct the analysis, the data available for the European countries were selected from 2010 to 2020, taking into consideration the most recent information and trying to avoid the period when financial crisis occur, even that it could affect the reliability of the results. On the other hand, from 2010, some countries started to recover to their macroeconomic stability, so the data are similar in terms of the information included. As some variables are reported 2 years apart, the time period includes data for 2010, 2012, 2014, 2016, 2018, and 2020, thus having in fact only series with six time period information. We took all European countries into consideration from 2022, so the number of cross-sectional observations was 27. Moreover, there are some unreported elements, thus the data is unbalanced.
The macroeconomic indices and indicators considered for this research represent secondary data, which was retrieved from Yale Center for Environmental Law and Policy of Yale University and Center for International Earth Science Information Network of Columbia University (SEDAC 2020; Wendling et al., 2020), United Nations Development Programme (UNDP United Nations Development Programme, 2019; UNDP United Nations Development Programme 2020), World Bank (2022) and Eurostat (2022), the database of the European Commission. The variables considered in the models are:
• Environmental Performance Index (EPI) was retrieved from SEDAC (2020) once in 2 years from 2010 to 2020. EPI assesses two main themes, that is, the vitality of ecosystems and the health of the natural environment, by considering from 25 indicators in 2010 to 32 indicators in 2020 when creating the index (SEDAC 2020; Wendling et al., 2020). The policy categories considered in 2010 were based on pollution–climate change, agriculture, fisheries, forestry, biodiversity and habitat, water resources and quality, water access and sanitation, and environmental burden of disease, while in 2020 the policy categories considered were based on water resources, agriculture, fisheries, pollution–climate change, ecosystem services, biodiversity and habitat, waste management heavy metals, sanitation and drinking water, and air quality (SEDAC 2020; Wendling et al., 2020).
• The Human Development Index (HDI) was retrieved from UNDP United Nations Development Programme (2020), UNDP United Nations Development Programme (2019), HDRO calculations based on data from UNDESA (2019)) once in 2 years from 2009 to 2019, as the data for 2020 were not available. This index is trying to emphasize a more complete consideration of the social welfare than GDP does. So, it takes into consideration education, life expectancy, and gross national income (UNDP United Nations Development Programme, 2019; UNDP United Nations Development Programme 2020).
• The variables retrieved from Eurostat (2022) are eco-innovation index (EII, index EU = 100), circular material use rate (CMUR, %), share of environmental taxes in total tax revenues (XENVT, %), area under organic farming as percentage of utilized agricultural area (AOF, %), heating degree days (number), energy productivity (EP, euro per kilogram of oil equivalent-kgoe), the share of renewable energy consumption in gross final energy consumption (XREC, %), and trade volume index (export/import ratio). The eco-innovation index gathers 16 indicators in one value related to eco-innovation inputs, activities, outputs, resource efficiency, and socioeconomic outcomes (Eurostat 2022). The circular material use rate (CMUR), used as proxy for circular economy in this research, represents “the share of material recovered and fed back into the economy in overall material use” (Eurostat, 2022). The energy productivity represents the ratio between the economic output produced in a country and the available energy produced to satisfy all demand (Eurostat 2022).
• The variables retrieved from World Bank (2022) are new business density (NBD, new registrations per 1,000 people ages 15–64, patent applications from residents (PAR, number), livestock production index (LPI, 2014–2016 = 100), forest area (FA, % of land area), total natural resources rents as percentage of GDP (NRR, %), Gini index, tertiary school enrollment (TSE, % gross), ratio of female-to-male labor force participation rate (XFMLF, %), proportion of seats held by women in national parliaments (XWNP, %), and individuals using the Internet (IUI, % of population).
The model on which the analysis was conducted is the panel model and has the form proposed in Eq. 1
In Eq. 1, εit represents the error generated by the fact that there are other elements of the nature–society complex that influence the analyzed variables (Greene, 2012: 53), and i and t indicate the country and year related to the considered variables, respectively, because the data panel assumes inclusion of cross-sectional analysis, that is, member states, with time.
The dependent variable is EPI, Environmental Performance Index. Regarding the independent variables included into the analysis, they are grouped in several categories:
In conducting the panel analysis, some methodological features were tested among them being the fixed effects (both cross-sectional and period), random effects, and omitted effects. The fixed effects were tested with the redundant fixed effect test, which null hypothesis is that fixed effects are all equal to each other, while the alternative reveals that there are significant differences between observation in cross-sectional analysis or time analysis. The selection of fixed or random effects was conducted after the Hausman (1978) test was performed. The Hausman test considers in the null hypothesis that both random and fixed effects are consistent estimators, and the random effect model is efficient, while the alternative provides evidence that only fixed effect model is consistent. Moreover, the omitted random effects test has the null hypothesis that there is no need for random effects as the pool model is a better estimator. The alternative is that the random effect should be used. It has to be mentioned that in a random effect model the time invariant variables can be controlled, but the results can be biased as the omitted variables are assumed to be statistically independent from all other variables. In practice, this assumption is unreliable.
Moreover, we took into consideration that the number of countries is at least twice of the number of periods. Considering this, the method of estimation, when the pool scenario was accepted, was the Period SUR (Parks estimators) effect within the generalized least square method linked White period in the correlation matrix. The Period SUR method considers that there is a general correlation between residuals considering period of time for specific cross section clustering. It corrects for heteroskedasticity and general correlation of observations within a cross section as they could appear due to similar regulations within that country. Regarding the correlation matrix, the White period method of estimation emphasis that a cross-sectional correlation exists between the series errors and tries the cross-sectional grouping. The idea of this methodology is to correct the arbitrary heteroskedasticity that can be encountered by cross-sectional series. The innovation of the methodology is linked both with the Period SUR effect (or the use of Parks’ estimators) within the generalized least square method and with the White period within the correlation matrix when the influencing variables of Environmental Performance Index are analyzed. When analyzing the White period correlation matrix (Wooldrige 2002: 276), the robustness of the results is ensured in the presence of any kind of heteroscedasticity or any kind of serial correlation when time (T) is relatively small to the number of cross-sectional data (N). The generalized least squared method with the Period SUR effects is based on the assumption that the mean of the error term is zero and that the unconditional variance of errors is both positive and non-singular.
Results and Discussions
Descriptive Statistics and Correlation Matrix
The purpose of this analysis is to provide evidence of the influencing factors of environmental performance index using a panel data analysis considering values without lag, took for the same period of time. The results will be useful both for academics and for policymakers as some environmental policy guidelines are going to be provided.
For conducting the analysis, first the descriptive statistics is presented in Table 2.
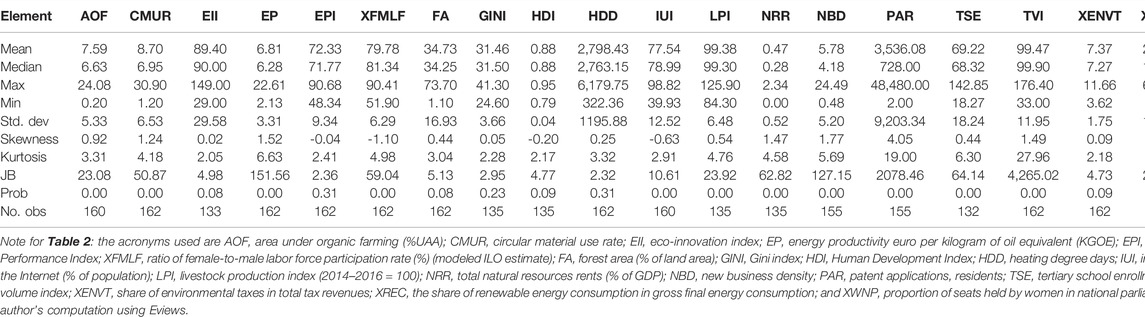
TABLE 2. Descriptive statistics for the dependent and independent variables considering the same period of time.
From Table 2, we can observe the descriptive statistic of the variables included into the analysis. For example, the median value of EPI (environmental performance index) was 72.33 for the European countries. The maximum value was 90.68 for Finland in 2016, while its minimum value was 48.34 for Romania in 2012. For eco-innovation index the average value was 89.4, its maximum value was 149 for Denmark in 2010, while its minimum value was registered in Bulgaria in 2016. Regarding the Gini Index, the average value was 31.46, as the maximum value was registered in Bulgaria in 2018, (value 41.3) which reveals higher inequality of income or consumption among individuals or households within Bulgarian economy, while its minimum value was in Slovenia in 2018 (value 24.6), which reveals higher equality on consumption or investment between individuals. The smallest value for HDI was also in Bulgaria in 2010, HDI being 0.788 in 2010, while the highest value was registered in Ireland in 2018, the value being 0.951.
Looking at statistical data, it can be observed that some of them do not have a normal distribution, the exempt being EPI, Gini Index, and heating degree days. On the other hand, we consider that it is more important to have normality in final results, so we did not standardize the variables, even though they have different unit measures. After the descriptive statistics, we looked at the correlation matrix, in order to find which variables are supposed to incur multicollinearity. The results are presented in Table 3.
From the correlation matrix, it can be observed that there are some variables that are highly correlated. In order to avoid the multicollinearity, it is recommended that the correlation coefficient found between variables to be under 0.3 (around 0.3 - for example, we admit that correlation of 0.3001 is not a high correlation, so variables can be simultaneously included into analysis) in absolute values. Including high correlated variables into analysis creates biased results that are doubtful and provide unrealistic conclusions.
Panel Data Results and Policy Implications
The purpose of this analysis is to provide evidence of the influence of variables on Environmental Performance Index, including indexes, environmental variables, and social variables. Based on the results, some policy guidelines are presented.
Additional elements have to be mentioned regarding the methodology used. We observed that there are significant period effects when conducting the redundant fixed effect tests, the fixed effect model seems to be more performant than the random one, while the omitted random effect reveal that the random could be better in some cases than pool. On the other hand, when estimated with period fixed effect, we registered very high values of R squared (higher than 70%) and the problem could not be solved. Thus, when conducting Period SUR estimation with the White period covariance matrix, the results seemed to be more reliable. The errors had a normal distribution, the value of R squared halved, and the variables became statistically significant. Thus, the results of the Period SUR effect within the generalized least square method associated with White period within the correlation matrix are presented in Table 4.
From Table 4, we can observe that among all variables included into the analysis only heating degree days variable was not statistically significant. Regarding the other variables, we encountered both positive and negative relationship as follows:
• Positive relationship between EPI and area under organic farming (%UAA) circular material use rate, eco-innovation index, energy productivity euro per kilogram of oil equivalent (kgoe), ratio of female-to-male labor force participation rate (%), forest area (% of land area, Human Development Index, and individuals using the Internet (% of population, livestock production index (2014–2016 = 100), new business density, patent applications, residents, tertiary school enrollment, the share of renewable energy consumption in gross final energy consumption, and proportion of seats held by women in national parliaments.
• Negative relationship between EPI and Gini index (that has a positive economic meaning), total natural resources rents (% of GDP), trade volume index, and share of environmental taxes in total tax revenues.
From Table 4, several conclusions can be observed. An increase of the area under organic farming (%UAA) with 1% creates an increase of Environmental Performance Index between 0.25 and 0.40. The relationship provides evidence that an increase in organic farming has positive relationship with Environmental Performance Index affecting both the ecosystem vitality and the environmental health. We consider that an increase of area under organic farming will affect the climate and energy component, the air pollution component and the water resources component of ecosystem vitally by reducing them and thus, by improving the Environmental Performance Index. Moreover, the increase of area under organic farming will create less exposure to heavy metals and improve air quality, which are the components of environmental health feature of EPI. The results sustain the idea pointed out by IFOAM Organics Europe (2022) as organic farming reduces the environmental impact compared to the one provided by conventional farming. Thus, increasing land under organic farming mitigates climate change, improves diversity and soil health, and contributes to the strategy of EU to achieve the target of 25% organic agricultural land by 2030. Even though the yield of organic farming seems to be 20% lower than that of conventional farming (IFOAM Organics Europe 2022), the diversification and the improvement of agricultural management at the country level can mitigate this impact (Ponisio et al., 2015). As a consequence, countries should encourage organic farming for mitigating the impact on environment (green gas emission, soil pollution, small biodiversity, water resources, and air quality), while searching for better management agricultural ideas that mitigate the yield gap between organic farming and conventional one.
When circular material use rate is analyzed, we observed a positive relationship link with Environmental Performance Index. The results suggest that an increase with 1% in the share of material recycled and fed back into the economy will increase the index with 0.18. We consider that the impact on EPI is related with improving more the air quality, the biodiversity and habitat component, both with the air pollution, water resources, and climate and energy component. On the practical level, the circularity rate or circulation rate, as it is named, is closely related with recycling and it is one of the features that EU considered for setting waste management targets (Zisopoulos et al., 2022). The authors (Zisopoulos et al., 2022) sustain that neither European country achieved total robustness in the circulatory rate, nor in the material flow networks, which suggest that waste management have to become more robust to shocks. At national and local levels, the improvement of circulatory rate will create better waste management, will encourage the achievement of a circular economy, and will affect positively the environmental performance. The policymakers should focus on creating strategies for stimulating all actors to improve both waste reusing and recycling for achieving the so-desired circular economy.
Eco-innovation Index has a positive relationship with Environmental Performance Index. Thus, eco-innovation improvements related with innovation activities (inputs, outputs, and results) together with other social variables increase the overall Environmental Performance Index. An increase with one unit of it determines an increase with 0.11 in the Environmental Performance Index value. From the practical point of view, the eco-innovation features affect positively the green growth, as all form of eco-innovation significantly reduces environmental impacts and mitigates the environmental pressure (Jang et al., 2015). Recent research conducted by Sobczak et al. (2022) reveals that eco-innovation is linked with methods of using natural resources, the concepts of eco-efficiency and green industry. Moreover, Europe needs to take advantage of eco-innovation solutions, to design and to implement new eco innovative features in order to mitigate the negative impact of economic activity on the environment. The results of the research are in line with the recommendation of Sobczak et al. (2022), as an improvement of eco-innovation activities, deals with an improvement of environmental performance. The policymakers should encourage eco-innovation in terms of eco technological improvements, support new markets developments, and input regulation that increase eco-innovation activities. Furthermore, they should encourage the environmentally friendly projects that could increase the competition in the European Union and that could create better environmental sustainability.
The energy productivity, expressed in euro per kilogram of oil equivalent (KGOE), is also positive and statistically significant with Environmental Performance Index. This variable is related with increasing energy efficiency and savings at the European level. Thus, an increase of energy productivity determines an increase between 0.60 and 0.88 of EPI. In this case, policymakers should act more on the drivers of energy productivity change for further growth of environmental performance. Among these drivers could be technological development, lower energy consumption and lowered costs of energy.
Regarding the ratio of female-to-male labor force participation rate (%), the relationship with EPI is also positive. The results reveal that an increase with 1% of the ratio of female-to-male labor force participation rate creates an increase between 0.19 and 0.26 of Environmental Performance Index. The explanation could be related that once there are more females in the labor force, there is also a higher probability for them to be in the management board. According to gender socialization theory (Chodorow 1978), women tend to be more compassionate for others, more sensitive to environmental problems and are more concerned about the risks related with the climate change. We consider that climate and energy component of ecosystem vitality of EPI will be the most affected one, by increasing the number of women in the labor force. According to Altunbas et al. (2022), who conducted an analysis on 1,951 companies from 24 countries between 2009 and 2019, an increase of female managers to total managers leads to CO2 decreasing even when controlling for institutional differences caused by culture and religion. Moreover, gender diversity has effects that are more powerful when women are represented in different organization. The recommendation is to encourage gender diversity through national regulation.
The increase of the forest area (% of land area) with 1% will create an increase in Environmental Performance Index with 0.1. If we look at the elements that form the Environmental Performance Index we observe that forest is an important component. Increasing the forest area implies decreasing the area of tree cuts lost, increasing the quality of air and the biodiversity of the regions. The results are in line with the results found by Li et al. (2021), who also detected a positive correlation between the increase of the forest area and the Environmental Performance Index. In terms of policy, we recommend stronger regulation in terms of forest cuts, stronger support for improving the forest area and subsidies for those that protect the existence forest area.
The Gini Index has a negative relationship with the Environmental Performance Index. The explanation is related with the fact that Gini Index refers to the extent to which the distribution of income or consumption among individuals or households within an economy is revealing high inequality when the value is close to one and high equality when the index is close to zero. The results provide evidence that an increase in the inequality between individuals creates smaller environmental performance, while an increase in equality between individuals affects positively the environmental performance. The results are in line with the results of Morse (2018), who suggests that better environmental performance is linked to greater equality, thus decreasing Gini coefficient creates higher environmental performance. Another important research is the one conducted by Soares et al. (2018) who proposed a modified application of Gini Index to measure the CO2 emissions concentration. The study is conducted on 84.95% of world GDP and 71.87% of CO2 emissions. The results reveal that developed countries have better environmental performance (Soares et al., 2018).
Regarding the Human Development Index, the results provide evidence of a strong correlation between this variable and the Environmental Performance Index. The results reveal that an increase with 0.01 of Human Development index emphasis an increase between 0.82 and 0.90 of EPI. The results are in line with the results of Arfanuzzaman (2016), Jain and Nagpal (2019) and others, proving that an increase of Human Development Index creates better environmental performance. The policymakers should focus on all components of HDI, namely, the education, the standard of living and the public health of a country through national programs, which can decrease the gap between individuals in terms of education access, public health access and their standard of living (policies where minimum wage is implemented, facilities are related with social condition of the individual).
Increasing the share of individuals using the Internet (% of population) is linked with higher environmental performance of EPI. The increase with 1% creates an increase between 0.25 and 0.27 of Environmental Performance Index. We consider that the explanation resides on the fact that higher the share of people using the Internet is, higher their degree of conscience is related with environmental problems, and thus, their attitude will improve the environmental performance. The results are in line with the results found by Ozcan and Apergis (2018) who suggest that increasing Internet access decreases the level of air pollution, thus, improving the environmental performance. Similar results are presented by Wang and Xu (2021) who pointed out that Internet usage and human capital are essential drivers of low-carbon economy development. Furthermore, the study of Roblek et al. (2020) emphasizes the link between Internet and sustainable development by pointing out the correlation between development, social features, environmental elements and performance. So, in this case, policymakers should focus on improving the Internet infrastructure and the quality of the information, which could enhance the general knowledge of the population about sustainable practices. As a practical example, government representatives could create awareness campaigns about sustainability by using Internet celebrities, such as influencers, social media experts, and sport figures.
Interestingly, the Livestock Production Index (2014–2016 = 100) increases EPI with 0.1–0.2 points, which means that the growth of the livestock products stimulates EPI rises. An explanation could be the fact that higher production does not necessarily mean higher number of livestock, but the animal breed, production processes, the type of animal husbandry and the type of animals preponderantly grown in a region. The result is interesting as livestock influences negatively the emissions, thus putting a higher pressure on the environment. Specifically, the emissions from ruminants are significant in slowing down sustainable development (Zervas and Tsiplakou 2012; Bellarby et al., 2013), but studies show that, with certain diets these emissions could be lowered (Haque 2018). So, policymakers should focus on stimulating more sustainable livestock production.
As expected, EPI will decrease by 5.63 points at an increase of 1% of the total natural resources’ rents (% of GDP), which means that the EU member states should invest in other type of capital than that of natural resources, especially the fossil fuels, as their impact on EPI is a negative one, thus lowering the environmental performance. The results can be linked with those of Zuo et al. (2022), who show that natural resource rents drastically damage the quality of the environment between 1991 and 2018 in BRI countries. Similar results are the ones conducted by He et al. (2022) as they reveal that natural resources hinders environmental sustainability because of its positive effects on greenhouse gas emissions. In this case, policymakers could focus on attracting investments in renewable resources instead of fossil fuels ones, as these are the best options among resources known currently to improve sustainability.
New business density seems to be positively statistically significant correlated with the EPI indicator, even though in the other models it seems to have a negative relationship with EPI. The results suggest that an increase with one unit of new business density (new registration per 1,000 people age 15–64) increase the Environmental Performance Index with 0.16. We consider that the explanation is related with the fact that more business will aim to achieve environmental performance and thus, will affect positively the climate change, air pollution, biodiversity, and water use. In order to understand the results, we can consult the report provided by LinkedIn Economic Graph (2022), who has around 800 million members around the world. Based on their analysis, the job postings requiring green skills grew at 8% annually over last years, between 2016 and 2021, while the share of green talent grew at more than 6% annually in the same period. Overall, considering the new climate policies and commitments in the next decade, an increase in the green jobs is expected to be observed. For example, for the period considered, in the United States, the number of renewables and environment jobs increased by 237%, in contrast to the 19% increase for oil and gas jobs. The share of green talent increased from 9.6% in 2015 to 13.3% in 2021, having a growth rate of 38.5%. The study actually emphasizes the need of new green businesses and skills, which is going to be observed in the variable new business density as green is mandatory for complying with climate change and environmental needs. In this context, policymakers could provide incentives and financing opportunities for businesses, which are more sustainable than the conventional ones. Also, they could develop social policies for stimulating the workforce to be employed in sustainable businesses.
Regarding the patent applications made by residents, the results show that an increase with 1% of the number of patent applicants will create an increase between 0.7 and 1.16 of the Environmental Performance Index. This result is somewhat in line with the finding of Carrión-Flores and Innes (2010), who demonstrates that increases of patents generate reductions of emissions, thus, increased environmental performance. So, policymakers should focus on stimulating innovations by ensuring better financing for research and better access to education to all.
Tertiary school enrollment is also important for Environmental Performance Index. Higher value of enrollment in tertiary school emphasis a higher value of EPI. An increase with 1% of tertiary school in total school attendance is increasing the value of EPI with 0.03 units. The results are in line with those of Lai and Chen (2020) and Gallego-Alvarez et al. (2014). Also, these can be correlated with the European University Association report (Stöber et al., 2021), where 392 higher education institutions from the European Higher Education Area reveal, though a survey, their greening activities and initiatives measures. About 64% of them have greening activities in place across the institution (Stöber et al., 2021). Greening is a topic of high interest in the area of learning and teaching for almost all university taken into analysis. Among the main drivers of higher education institutions to engage in greening are local and regional contexts, European funding, collaboration with the industry, European policies/guidelines, leadership and staff and student engagement. Moreover, due to the Covid crisis, there seem to be more awareness of environmental issues, thus, higher education has a positive influence on environmental performance. In this context, policymakers could stimulate universities to adopt sustainable practices and sustainable curricula for increasing students’ knowledge and awareness about environmental issues and sustainability. Moreover, governmental representatives could ensure better financing for universities for adopting such actions.
Trade Volume Index is negatively correlated with Environmental Performance Index. The Trade Volume Index measures the amount of money flowing in and out of a security or the market. The relationship can be explained by the fact that as people are more concerned in producing capital they can ignore the performance of the environment. The results suggest than an increase 1 of TVI is linked with a decrease between 0.7 and 0.15 of EPI. The results are similar with the results found regarding the trade openness variable as they also reveal a negative correlation (Shahabadi et al. (2017), Fakher and Abebi (2017), Pourali et al. (2019).
The negative relationship between the share of environmental taxes in total tax revenues and Environmental Performance Index is related with the fact that the increase of the independent variable can be due to higher taxes or due to much more behavior that is harmful for the planet’s health. The environmental taxes are based on avoiding climate change and on combating air, soil or other natural pollution. Looking at the components of EPI we observe that it aims in improving the elements related with planet health, thus being in opposite direction than environmental taxes. The results reveal that an increase with 1% of environmental taxes in total taxes decreases the EPI with 0.87 units. The results sustain the idea that environmental taxes are key elements for reducing CO2 emission (Dogan et al., 2022). The results are also in line with Ganda and Garidzirai (2021), who reveal that aggregate environmental tax improves environmental sustainability in the European Union. Moreover, when environmental tax is disaggregated into energy tax and transport tax, they are more efficient in lessening emissions and improve environmental sustainability, especially in the case of transport tax. However, may be for faster results, policymakers should focus on stimulating, not restricting, consumers to adopt sustainable behaviors by using green incentives and non-monetary benefits.
The share of renewable energy consumption in gross final energy consumption is positively linked with the Environmental Performance Index. An increase with 1% will create an increase between 0.05 and 0.16 units in EPI. As many studies (Fotis and Polemis 2018; Khan H. et al., 2021; Musa et al., 2021; Feng et al., 2022) suggest that renewable energy stimulates the reduction of emissions, it means that further the environmental performance could be improved. The practical idea would be that governments should emphasize the need of increasing the share of renewable energy in total energy as it creates less pollution, more reliable solution that mitigates the negative impact on environmental and thus, increases the environmental performance. The results are in line with the results Li et al. (2021), who proved that there is a positive correlation between the share of renewable energy and the Environmental Performance Index.
The increase in the proportion of seats held by women in national parliaments with 1% will create an increase of Environmental Performance Index with 0.27 units. The explanation is linked with the more inclined environmental attitude toward environmental considering that several studies proved that gender diversity affects positively the environment features. The results are in line with the idea emphasized by the research conducted by European Central Bank Study (2022) where it is shown that an increase of female managers to total managers leads to CO2 decreasing even when controlling for institutional differences caused by culture and religion. Gender diversity seems to have effects that are more powerful when women are represented in different organization such as political institutions and civil society organizations.
Conclusion and Policy Recommendations
This research aimed to assess the drivers and barriers of environmental performance index (EPI is the dependent variable) at the EU level during 2010– 2020, with data collected once in 2 years, by considering the following independent variables: Human Development Index, GINI index, eco-innovation index, circular material use rate, forest area, area under organic farming, heating days, environmental taxes, natural resources rents, renewable energy consumption, energy productivity, new business density, Internet users, patent applications, trade volume index, women impact, and tertiary education.
The integration of the indices in the analysis together with the environmental performance and other macro factors allowed us to obtain new findings in the field. As a fact, the EU’s environmental performance, assessed in a more complex way by the index EPI, was positively influenced by eco-innovation index, circular material use rate, forest area, area under organic farming, renewable energy consumption, energy productivity, new business density, Internet users, patent applications, women impact and tertiary education between 2010 and 2020, once in 2 years. Another finding points out the negative influences of GINI index (higher inequality), total natural resources rents, trade volume index and environmental taxes on EPI. Although newly considered, the number of heating degree days should be retested as it indicated inconclusive result.
Based on the results obtained, we consider that policymakers should provide aid to community and regions in order to obtain higher environmental performance. The recommendations are presented in Table 5.
Furthermore, all variables should be reassessed in a longer time frame, as well as in other regions, in order to formulate better environmental policies, to observe if the results are similar in terms of effect, size, and dimension. The guidelines of policymakers should focus on achieving the environmental factors proposed to 2030, to encourage environmental close behavior and to discourage environmental deterioration. Also, it is similarly important to not forget about the diversity of national conditions in the environmental proposals and the measures that aimed improving the sustainability of different regions.
Given all the findings of the analysis, it is necessary to rethink and to reduce the objectives of economic development of each country based on continuous progress and to stimulate other components of social welfare to protect the natural environment and long-term survival of humankind. In this context, the equitable integrated approach of the components of the nature–society system becomes imperative. However, there are limitations to the research conducted regarding the tests allowed by EViews, the data panel technique used, the specific conditions of each EU country on energy consumption patterns, the data availability, the size of the database, the problems occurred in the estimation technique because of the small time period (6) included into the analysis even though we have 27 cross country observations and the combinations of variables introduced in the panel model. In reality, all the factors considered are interdependent and, therefore, in a future research, we aim to develop new mathematical models based on the science of complexity to consider all variables of the nature–society system as a whole when evaluating the influences of its components in order to increase the possibilities of predicting their evolution. Another way of improving the results is related with using more advanced techniques estimation. In addition, future research can test the causality between variables as recent research reveals bidirectional causality between them. The research can be improved by analyzing the drivers of environmental performance based on micro data, more precisely, at the firm level, by conducting a research based on a questionnaire at the firms’ level where the structural equation modeling with a graphical design could be applied. However, the research does not lose its relevance because it shows many historical trends and impacts of some components of the nature–society system, specifically the environmental performance drivers, which must be considered in future sustainable policies and in substantiating and setting targets in every sector of our society.
Data Availability Statement
The data is available upon reasonable request made to the corresponding author.
Author Contributions
All authors listed have made a substantial, direct, and intellectual contribution to the work and approved it for publication.
Conflict of Interest
The authors declare that the research was conducted in the absence of any commercial or financial relationships that could be construed as a potential conflict of interest.
Publisher’s Note
All claims expressed in this article are solely those of the authors and do not necessarily represent those of their affiliated organizations, or those of the publisher, the editors, and the reviewers. Any product that may be evaluated in this article, or claim that may be made by its manufacturer, is not guaranteed or endorsed by the publisher.
Acknowledgments
This research is part of a revised and updated section of the doctoral thesis “Politicile energetice și dezvoltarea durabilă” by AZ. The authors are grateful to the reviewers for their insightful comments and recommendations which improved considerably the value of our paper. As well, the authors thank the editor for his inputs and management of this paper during the review process.
References
Altunbas, Y., Gambacorta, L., Reghezza, A., and Velliscig, G. (2022). Does Gender Diversity in the Workplace Mitigate Climate Change? SSRN J. doi:10.2139/ssrn.4046329
Arfanuzzaman, M. D. (2016). Impact of CO2 Emission, Per Capita Income and HDI on Environmental Performance Index: Empirical Evidence from Bangladesh. Int. J. Green Econ. 10 (3-4), 213–225. doi:10.1504/ijge.2016.081900
Baltagi, B. H. (2008). Econometric Analysis of Panel Data. Fourth Edition. Chichester: John Wiley & Sons.
Bellarby, J., Tirado, R., Leip, A., Weiss, F., Lesschen, J. P., and Smith, P. (2013). Livestock Greenhouse Gas Emissions and Mitigation Potential in Europe. Glob. Change Biol. 191, 3–18. doi:10.1111/j.1365-2486.2012.02786.x
Breitung, J. (2000). The Local Power of Some Unit Root Tests for Panel Data. Adv. Econ. 15, 161–177. doi:10.1016/S0731-9053(00)15006-6
Carrión-Flores, C. E., Innes, R., and Robert, I. (2010). Environmental Innovation and Environmental Performance. J. Environ. Econ. Manag. 591, 27–42. doi:10.1016/j.jeem.2009.05.003
Chakraborty, D., and Mukherjee, S. (2013). How Do Trade and Investment Flows Affect Environmental Sustainability? Evidence from Panel Data. Environ. Dev. 6, 34–47. doi:10.1016/j.envdev.2013.02.005
Chodorow, N. (1978). “The Reproduction of Mothering,” in The Reproduction of Mothering. Psychoanalysis and the Sociology of Gender (Berkeley: University of California Press). doi:10.1525/9780520924086
Chowdhury, T., and Islam, S. (2017). Environmental Performance Index and GDP Growth Rate: Evidence from BRICS Countries. Environ. Econ. 84, 31–36. doi:10.21511/ee.08(4).2017.04
Chudik, A., and Pesaran, M. H. (2013). Large Panel Data Models with Cross-Sectional Dependence: a Survey. SSRN J. 4371, 1–54. doi:10.2139/ssrn.2319840
Čuček, L., Klemeš, J. J., and Kravanja, Z. (2012). A Review of Footprint Analysis Tools for Monitoring Impacts on Sustainability. J. Clean. Prod. 34, 9–20. doi:10.1016/j.jclepro.2012.02.036
Dickey, D. A., and Fuller., W. A. (1981). Likelihood Ratio Statistics for Autoregressive Time Series with a Unit Root. Econometrica 49 (4), 1057–1072. doi:10.2307/1912517
Dogan, E., Hodžić, S., and Šikić, T. F. (2022). A Way Forward in Reducing Carbon Emissions in Environmentally Friendly Countries: the Role of Green Growth and Environmental Taxes. Econ. Research-Ekonomska Istraživanja, 1–16. doi:10.1080/1331677X.2022.2039261
Eco-innovation Observatory (2022). European Commission. AvaliableAt: https://ec.europa.eu/environment/ecoap/indicators/index_en (Accessed March 14, 2022).
Eurostat (2022). European Commission. AvaliableAt: https://ec.europa.eu/eurostat/data/database (Accessed February 20, 2022).
Fakher, H-A., and Abedi, Z. (2017). Relationship between Environmental Quality and Economic Growth in Developing Countries (Based on Environmental Performance Index). Environ. Energy Econ. Res. 13, 299–310. doi:10.22097/EEER.2017.86464.1001
Feng, J., Sun, Q., and Sohail, S. (2022). Financial Inclusion and its Influence on Renewable Energy Consumption-Environmental Performance: the Role of ICTs in China. Environ. Sci. Pollut. Res., 1–8. doi:10.1007/s11356-022-19480-9
Fotis, P., and Polemis, M. (2018). Sustainable Development, Environmental Policy and Renewable Energy Use: A Dynamic Panel Data Approach. Sustain. Dev. 26, 726–740. doi:10.1002/sd.1742
Gallego-Alvarez, I., Vicente-Galindo, M., Galindo-Villardón, M., Rodríguez-Rosa, M., and Rodríguez-Rosa, M. (2014). Environmental Performance in Countries Worldwide: Determinant Factors and Multivariate Analysis. Sustainability 6, 7807–7832. doi:10.3390/su6117807
Ganda, F., and Garidzirai, R. (2021). “The Environmental Influence of Tax Regimes in Selected European Union Economies,” in Sabaan, Albert. 2021. Green Computing Technologies And Computing Industry in 2021 (UKLondon: IntechOpen). doi:10.5772/intechopen.94552
Ghodrati, S., Harati, J., and Nazari, A. (2018). The Democracy and Environment Quality in Selected Countries: An Application of Panel Data. Iran. Econ. Rev. 221, 21–49. doi:10.22059/IER.2018.65348
Halkos, G., and Zisiadou, A. (2018). Relating Environmental Performance with Socioeconomic and Cultural Factors. Environ. Econ. Policy Stud. 201, 69–88. doi:10.1007/s10018-017-0182-9
Haque, M. N. (2018). Dietary Manipulation: a Sustainable Way to Mitigate Methane Emissions from Ruminants. J. Anim. Sci. Technol. 60 (1), 1–10. doi:10.1186/s40781-018-0175-7
HDRO calculations based on data from UNDESA (2019). United Nations Statistics Division (2020b), World Bank (2020a), Barro and Lee (2018) and IMF. UNESCO Institute for Statistics. AvaliableAt: https://hdr.undp.org/en/indicators/137506.
He, Y., Li, X., Huang, P., and Wang, J. (2022). Exploring the Road toward Environmental Sustainability: Natural Resources, Renewable Energy Consumption, Economic Growth, and Greenhouse Gas Emissions. Sustainability 14, 1579. doi:10.3390/su14031579
Hsu, A., Lloyd, A., and Emerson, J. W. (2013). What Progress Have We Made since Rio? Results from the 2012 Environmental Performance Index (EPI) and Pilot Trend EPI. Environ. Sci. Policy 33, 171–185. doi:10.1016/j.envsci.2013.05.011
IFOAM Organics Europe (2022). Organic Agriculture and its Benefits for Climate and Biodiversity. AvaliableAt: https://www.organicseurope.bio/content/uploads/2022/04/IFOAMEU_advocacy_organic-benefits-for-climate-and-biodiversity_2022.pdf?dd (Accessed June 13, 2022).
Im, K. S., Pesaran, M. H., and Shin, Y. (2003). Testing for Unit Roots in Heterogeneous Panels. J. Econ. 1151, 53–74. doi:10.1016/S0304-4076(03)00092-7
Jain, M., and Nagpal, A. (2019). Relationship between Environmental Sustainability and Human Development Index: A Case of Selected South Asian Nations. Vision 232, 125–133. doi:10.1177/0972262919840202
Jang, E., Park, M., Park, T. W. R., Roh, T., and Han, K. (2015). Policy Instruments for Eco-Innovation in Asian Countries. Sustainability 7, 12586–12614. doi:10.3390/su70912586
Jorgenson, A. K., and Clark., B. (2011). Societies Consuming Nature: A Panel Study of the Ecological Footprints of Nations, 1960-2003. Soc. Sci. Res. 40, 226–244. doi:10.1016/j.ssresearch.2010.09.004
Khan, H., Weili, L., Khan, I., and Khamphengxay, S. (2021a). Renewable Energy Consumption, Trade Openness, and Environmental Degradation: a Panel Data Analysis of Developing and Developed Countries. Math. Problems Eng. 2021, 1–13. doi:10.1155/2021/6691046
Khan, S. A. R., Ponce, P., Thomas, G., Yu, Z., Al-Ahmadi, M. S., Tanveer, M., et al. (2021b). Digital Technologies, Circular Economy Practices and Environmental Policies in the Era of COVID-19. Sustainability 13, 12790. doi:10.3390/su132212790
Khan, S. A., Hassan, S., Khan, M. A., Khan, M. R., Godil, D. I., Tanveer, M., et al. (2022). Nexuses between Energy Efficiency, Renewable Energy Consumption, Foreign Direct Investment, Energy Consumption, Global Trade, Logistics and Manufacturing Industries of Emerging Economies: In the Era of COVID-19 Pandemic. Front. Environ. Sci. 10, 880200. doi:10.3389/fenvs.2022.880200
Lai, S. L., and Chen, D.-N. (2020). A Research on the Relationship between Environmental Sustainability Management and Human Development. Sustainability 12, 9001. doi:10.3390/su12219001
Levin, A., Lin, C.-F., and James Chu, C.-S. (2002). Unit Root Tests in Panel Data: Asymptotic and Finite-Sample Properties. J. Econ. 1081, 1–24. doi:10.1016/S0304-4076(01)00098-7
Li, H., Xu, X., Rafique, M. Z., Naqvi, S. A. A., and Nadeem, A. M. (2021). What Drives the Environmental Performance? Evidence from Asian Countries. Pol. J. Environ. Stud. 30 (4), 3685–3697. doi:10.15244/pjoes/131155
LinkedIn Economic Graph (2022). Global Green Skills Report 2022. AvaliableAt: https://economicgraph.linkedin.com/content/dam/me/economicgraph/en-us/global-green-skills-report/global-green-skills-report-pdf/li-green-economy-report-2022.pdf (Accessed June 16, 2022).
Liu, G., Brown, M., and Casazza, M. (2017). Enhancing the Sustainability Narrative through a Deeper Understanding of Sustainable Development Indicators. Sustainability 96, 1078. doi:10.3390/su9061078
Liu, G. (2014). Development of a General Sustainability Indicator for Renewable Energy Systems: A Review. Renew. Sustain. energy Rev. 31, 611–621. doi:10.1016/j.rser.2013.12.038
Maccari, N. (2014). Environmental Sustainability and Human Development: A Greening of Human Development Index. SSRN J. 2 (1), 29–34. doi:10.2139/ssrn.2426073
Mahmood, H., Tanveer, M., and Furqan, M. (2021). Rule of Law, Corruption Control, Governance, and Economic Growth in Managing Renewable and Nonrenewable Energy Consumption in South Asia. Ijerph 18, 10637. doi:10.3390/ijerph182010637
Mance, D., Krunić, K., and Mance, D. (2021). Protecting Species by Promoting Protected Areas and Human Development-A Panel Analysis. Sustainability 13, 11970. doi:10.3390/su132111970
Mátyás, L., and Sevestre, P. (2008). The Econometrics of Panel Data: Fundamentals and Recent Developments in Theory and Practice. Third edition (Germany: Springer).
Meadows, D. H., Meadows, D. l., Randers, J., and Behrens, W. W. (1972). The Limits to Growth: A Report for the Club of Rome's Project on the Predicament of Mankind. New York, USA: Universe Books. AvaliableAt: http://www.ask-force.org/web/Global-Warming/Meadows-Limits-to-Growth-Short-1972.pdf (Accessed June 12, 2016).
Morse, S. (2018). Relating Environmental Performance of Nation States to Income and Income Inequality. Sust. Dev. 26 (1), 99–115. doi:10.1002/sd.1693
Musa, M. S., Jelilov, G., Iorember, P. T., and Usman, O. (2021). Effects of Tourism, Financial Development, and Renewable Energy on Environmental Performance in EU-28: Does Institutional Quality Matter? Environ. Sci. Pollut. Res. 2838, 53328–53339. doi:10.1007/s11356-021-14450-z
Narula, K., and Reddy, B. S. (2015). Three Blind Men and an Elephant: The Case of Energy Indices to Measure Energy Security and Energy Sustainability. Energy 80, 148–158. doi:10.1016/j.energy.2014.11.055
Nogueira, M. C., and Madaleno, M. (2021). Are International Indices Good Predictors of Economic Growth? Panel Data and Cluster Analysis for European Union Countries. Sustainability 1311, 6003. doi:10.3390/su13116003
Ozcan, B., and Apergis, N. (2018). The Impact of Internet Use on Air Pollution: Evidence from Emerging Countries. Environ. Sci. Pollut. Res. 25 (5), 4174–4189. doi:10.1007/s11356-017-0825-1
Pao, H.-T., and Tsai, C.-M. (2011). Multivariate Granger Causality between CO2 Emissions, Energy Consumption, FDI (Foreign Direct Investment) and GDP (Gross Domestic Product): Evidence from a Panel of BRIC (Brazil, Russian Federation, India, and China) Countries. Energy 361, 685–693. doi:10.1016/j.energy.2010.09.041
Pesaran, H. (2013). Large Panel Data Models with Cross-Sectional Dependence: a Survey. AvaliableAt: https://www.econ.cam.ac.uk/CSDPDM/course-files/Pesaran_LargePanels_Cambridge_26May2013.pdf (Accessed May 10, 2022).
Phillips, P. C. B., and Perron, P. (1988). Testing for a Unit Root in Time Series Regression. Biometrika 75, 335–346. doi:10.1093/biomet/75.2.335
Pimonenko, T., Lyulyov, O., Chygryn, O., and Palienko, M. (2018). Environmental Performance Index: Relation between Social and Economic Welfare of the Countries. Environ. Econ. 9, 1–11. doi:10.21511/ee.09(3).2018.01
Ponisio, L. C., M'Gonigle, L. K., Mace, K. C., Palomino, J., de Valpine, P., and Kremen, C. (2015). Diversification Practices Reduce Organic to Conventional Yield Gap. Proc. R. Soc. B 282, 20141396. doi:10.1098/rspb.2014.1396
Popescu, G., Boboc, D., Stoian, M., Zaharia, A., and Georgiana-Raluca Lădaru, G. R. (2017). A Cross-Sectional Study of Sustainability Assessment. Econ. Comput. Econ. Cybern. Stud. Res. 51 (1), 21–36.
Pourali, A., Ali Falahi, M., and Meidani, A. K. N. (2019). The Effects of Good Governance and Political-Civil Liberties Indices on Environmental Performance Index (EPI): An Analysis of 101 Countries Worldwide. J. Econ. Regional Dev. 26 (17), 63–94. doi:10.22067/ERD.V26I17.69596
Raza, A., Sui, H., Jermsittiparsert, K., Żukiewicz-Sobczak, W., and Sobczak, P. (2021). Trade Liberalization and Environmental Performance Index: Mediation Role of Climate Change Performance and Greenfield Investment. Sustainability 13, 9734. doi:10.3390/su13179734
Roblek, V., Meško, M., Bach, M. P., Thorpe, O., and Šprajc, P. (2020). Bach, Oshane Thorpe, and Polona ŠprajcThe Interaction between Internet, Sustainable Development, and Emergence of Society 5.0. Data 5, 80. doi:10.3390/data5030080
Samimi, A. J., Ahmad, J., Kashefi, A., Salatin, P., and Lashkarizadeh, M. (2011). Environmental Performance and HDI: Evidence from Countries Around the World. Middle-East J. Sci. Res. 10 (1), 294–301.
SEDAC (2020). Environmental Performance Index (EPI). Palisades, New York: NASA Socioeconomic Data and Applications Center SEDAC.
Shahabadi, A., Samari, H., and Nemati, M. (2017). The Factors Affecting Environmental Performance Index (EPI) in Selected OPEC Countries. Iran. Econ. Rev. 21 (3), 457–467. doi:10.22059/IER.2017.62925
Soares, T. C., Fernandes, E. A., and Toyoshima, S. H. (2018). The CO 2 Emission Gini Index and the Environmental Efficiency: An Analysis for 60 Leading World Economies. EconomiA 19, 266–277. doi:10.1016/j.econ.2017.06.001
Sobczak, E., Głuszczuk, D., and Raszkowski, A. (2022). Eco-Innovation and Innovation Level of the Economy as a Basis for the Typology of the EU Countries. Ijerph 194, 2005. doi:10.3390/ijerph19042005
Stöber, H., Gaebel, M., and Morrisroe, A. (2021). Greening in European Higher Education Institutions, EUA Survey Data, September 2021. Report. European University Association. AvaliableAt: https://eua.eu/downloads/publications/greening%20report.pdf (Accessed June 13, 2022).
UNDP-United Nations Development Programme (2019). Human Development Report. AvaliableAt: http://hdr.undp.org/en/composite/HDI.
UNDP-United Nations Development Programme (2020). Human Development Report 2020: The Next Frontier—Human Development and the Anthropocene. AvaliableAt: http://hdr.undp.org/en/2020-report.
Wang, J., and Xu, Y. (2021). Internet Usage, Human Capital and CO2 Emissions: A Global Perspective. Sustainability 1315, 8268. doi:10.3390/su13158268
Wendling, Z. A., Emerson, J. W., de Sherbinin, A., and Etsy, D. C. (2020). Environmental Performance Index 2020. AvaliableAt: https://epi.yale.edu/epi-results/2022/component/epi (Accessed January 10, 2022).doi:10.13140/RG.2.2.21182.51529
Wooldridge, J. M. (2002). Econometric Analysis of Cross Section and Panel Data. London, UK: MIT Press.
World Bank (2022). Data from Database: World Development Indicators. Washington, DC: World Bank. AvaliableAt: https://databank.worldbank.org/source/world-development-indicators (Accessed February 20, 2022).
Yu, Z., Zia-ul-haq, H. M., Irshad, A. u. R., Tanveer, M., Jameel, K., Janjua, L. R., et al. (2022). Nexuses between Crude Oil Imports, Renewable Energy, Transport Services, and Technological Innovation: A Fresh Insight from Germany. J. Pet. Explor Prod. Technol., 1–11. doi:10.1007/s13202-022-01487-0
Zaharia, A. (2017). Politicile Energetice Si Dezvoltarea Durabilă. PhD diss. Bucharest, Romania: The Bucharest University of Economic Studies.
Zervas, G., and Tsiplakou, E. (2012). An Assessment of GHG Emissions from Small Ruminants in Comparison with GHG Emissions from Large Ruminants and Monogastric Livestock. Atmos. Environ. 49, 13–23. doi:10.1016/j.atmosenv.2011.11.039
Zisopoulos, F. K., Schraven, D. F. J., and de Jong, M. (2022). How Robust Is the Circular Economy in Europe? an Ascendency Analysis with Eurostat Data between 2010 and 2018. Resour. Conservation Recycl. 178, 106032. doi:10.1016/j.resconrec.2021.106032
Keywords: policy guidelines, eco-innovation, EPI, HDI, panel data, patents, renewable energy, sustainability
Citation: Stoian M, Brad L and Zaharia A (2022) Drivers of the European Union’s Environmental Performance. Front. Environ. Sci. 10:954612. doi: 10.3389/fenvs.2022.954612
Received: 27 May 2022; Accepted: 22 June 2022;
Published: 03 August 2022.
Edited by:
Syed Abdul Rehman Khan, Xuzhou University of Technology, ChinaReviewed by:
Muhammad Tanveer, Prince Sultan University, Saudi ArabiaMuhammad Umer Quddoos Attari, Bahauddin Zakariya University, Pakistan
Copyright © 2022 Stoian, Brad and Zaharia. This is an open-access article distributed under the terms of the Creative Commons Attribution License (CC BY). The use, distribution or reproduction in other forums is permitted, provided the original author(s) and the copyright owner(s) are credited and that the original publication in this journal is cited, in accordance with accepted academic practice. No use, distribution or reproduction is permitted which does not comply with these terms.
*Correspondence: Alina Zaharia, alina.zaharia@eam.ase.ro