- 1School of Management, Wuxi Institute of Technology, Wuxi, China
- 2School of Management, Ocean University of China, Qingdao, China
The improvement of carbon emission efficiency and the realization of the goal of “carbon peaking and carbon neutrality” are the key issues that China needs to solve urgently at this stage. The green and low-carbon transformation of the economy requires sufficient financial support. Whether green finance is an opportunity to improve China’s carbon emission efficiency is worth studying. For the aim, based on the macro-panel data of 30 provinces in China from 2010 to 2019, this paper uses fixed effect model and spatial Durbin model to study the impact of green finance on regional carbon emission efficiency. The results show that: First, the development of green finance can improve the carbon emission efficiency; Second, in addition to the “local effect”, the influence of green finance on carbon emission efficiency has a “neighborhood effect”, that is, it has a spatial spillover effect on carbon emission efficiency in neighboring areas, and this effect only exists in a short time; Third, the impact of green finance on carbon emission efficiency is heterogeneous in different regions with different environmental regulations. This paper has reference significance for green finance development and the implementation of the goal of “carbon peaking and carbon neutrality” in China.
1 Introduction
Climate warming caused by greenhouse gases, especially carbon dioxide emissions, has become one of the global environmental problems and has attracted much attention (Rezanezhad et al., 2020; Işık et al., 2021a, Işık et al., 2021b; Pan et al., 2022). Climate change has led to the frequent occurrence of extreme weather events around the world, further causing ecological imbalances, environmental damage (Işık et al., 2019), and affecting the normal production and living order (Wolff and Haase, 2019; Tuladhar et al., 2021). As the backbone of the global ecological civilization construction and green responsibility, China attaches great importance to climate governance (Meng et al., 2021). In September 2020, Chinese President Xi Jinping made it clear at the 75th United Nations General Assembly that China’s carbon dioxide emissions should peak by 2030 and achieve carbon neutrality by 2060 (Huang et al., 2022). Achieving the goal of “carbon peaking and carbon neutrality” is a broad and profound systemic change related to energy, environment, economy and society, and a historic turning point for China’s carbon emission reduction (Rehman et al., 2021; Dong et al., 2022; Pata et al., 2022; Ullah et al., 2022). As the world’s second largest economy and the largest developing country, China must focus on both economic development and environmental governance, and promote carbon emission reduction in the process of economic activities. Therefore, in addition to the rigid constraints of carbon emission quotas, China should pay more attention to carbon emission efficiency. Improving carbon emission efficiency is the key path to advance the “carbon peaking and carbon neutrality” goal, and it is an inherent requirement for the high-quality development of China’s economy (Sun and Huang, 2020). Furthermore, according to China’s Green Finance Development Report 2021, China needs to invest about 150–500 trillion yuan to realize the “carbon peaking and carbon neutrality” strategy, which cannot effectively meet such a huge capital demand only by fiscal strength. Therefore, financial support is an important part of improving carbon emission efficiency (Ali et al., 2022; Fareed et al., 2022; Rehman et al., 2022), and it is also an indispensable force for marketization to promote the realization of the “carbon peaking and carbon neutrality” goal (Zhang, 2011). In order to identify the flow of financial resources and ensure the accurate flow of supporting tools to the green development field, China actively explores green financial products and services and continuously improves the long-term development mechanism of green finance.
Green finance is a new financial development model that guides the transfer of financial resources to green and low-carbon industries and promotes the green transformation of industrial enterprises. Developing green finance to improve carbon emission efficiency is a requirement for China to achieve the carbon dioxide emission goal. In August 2016, seven ministries including the People’s Bank of China jointly issued the “Guiding Opinions on Building a Green Financial System”, which clearly proposed to establish a sound green financial system based on the overall situation of sustainable economic development. Since then, the top-level design of China’s green financial system has been gradually clarified, and the market environment and institutional arrangements to support the development of green finance have been gradually improved. For example, in 2021, the central bank issued a carbon emission reduction support tool. As of the end of 2021, the balance of China’s green loans is about 15 trillion yuan; as of the first half of 2021, the cumulative scale of green bond issuance in China and abroad has exceeded 1.73 trillion yuan. The scale of China’s green finance market is developing rapidly. Has it played a role in promoting the efficiency of carbon emissions? Will this effect be heterogeneous with different environmental regulations? Will green finance have an impact on carbon efficiency in other regions through externalities (spillover effects)? This paper selects the macro panel data of 30 provinces in China from 2010 to 2019 as the research object, and explores the “local effect” and “neighborhood effect” of green finance in affecting carbon emission efficiency.
The possible marginal contributions of this paper are as follows: First, few studies currently focus on the impact of green finance development on carbon emission efficiency. This paper incorporates green finance and carbon emission efficiency into the same analytical framework, which broadens the research scope of green finance and carbon emission efficiency respectively, and provides evidence support for the government to continue to support the development of green finance in the future. Second, green finance has strong spatial spillover effects (Li and Gan, 2021). However, few literatures incorporate spatial factors into the research on the impact of green finance development on carbon emission efficiency. Therefore, this paper attempts to use the spatial Durbin model to analyze whether the carbon emission efficiency improvement effect of green finance has spatial spillover. Third, from the perspective of environmental regulation, this paper provides policy inspiration for promoting the realization of the goal of “carbon peaking and carbon neutrality” and establishing a sound green financial system. The conclusion shows that, with different environmental regulations, the impact of green finance on carbon emission efficiency is heterogeneous.
2 Literature review
At present, many scholars have conducted research on carbon emission, including the measurement and evaluation (Fang et al., 2022), dynamic change (Cheng et al., 2018b), influencing factors and effects analysis (Xu et al., 2021; Zhang H et al., 2021) of carbon emission efficiency. Scholars think that policy and supervision (Calvo et al., 2021; Yu and Zhang, 2021), technological innovation (Gouveia et al., 2021; He et al., 2021; Wu H et al., 2021; Wyse et al., 2021; Xie et al., 2021), human capital (Song et al., 2020), industrial structure (Cheng et al., 2018a; Wu L et al., 2021) and urbanization (Sun and Huang, 2020; Wang F et al., 2021) will affect carbon emission efficiency. Fang et al. (2022) and Meng et al. (2016) used DEA model to measure the carbon emission efficiency of 42 thermal power plants and 30 provinces in China from the micro and macro levels respectively, and found that there was regional imbalance in China’s carbon emission efficiency. Cheng et al. (2018b) used NDDF function to calculate the total factor carbon emission efficiency (TCEI) of industrial sectors in various provinces in China. The results showed that TCEI showed an increasing trend from 2005 to 2015, but the growth rate decreased. Yu and Zhang (2021) evaluated the effect of the low-carbon city pilot policy, and found that the carbon emission efficiency of the pilot group (pilot city) increased by 1.7%, and the carbon emission was reduced by more than 8 million tons. He et al. (2021) constructed the Renewable Energy Technology Innovation Index (RETI) and Total Factor Carbon Emission Performance Index (TCPI). The research found that RETI can promote the growth of TCPI, but the relationship between them is affected by the market environment. The research of Xie et al. (2021) once again proves the importance of technological progress to carbon emission reduction.
Green finance has attracted more and more attention as a bright spot to support low-carbon transition and high-quality development (Tsoukala and Tsiotas, 2021; Yang et al., 2021). Green finance increases the social responsibility of financial institutions (Kurt and Peng, 2021), encourages banks to provide funds for enterprises’ development (Liu et al., 2021), and then promotes technological innovation (Wang M et al., 2021). The impact of green finance can be divided into three levels: enterprise, industry and region. For the enterprise level, Zheng et al. (2021) uses data from Bangladesh to confirm that the development of green finance business has a positive impact on the medium and long-term development of financial enterprises themselves. But there are also different research conclusions. Umar et al. (2021) believe that some financial enterprises have increased external costs and decreased profits because they have assumed social and environmental responsibilities. Zhang et al. (2021) used the data of 945 A-share companies to find that green financial incentives and punishment effects coexist, affecting the investment and financing behavior of enterprises, which in turn affects the quality of the environment. For the industry level, Wang and Wang (2021) used the grey correlation method and the GMM model as tools to test and found that green finance has a greater role in promoting the tertiary industry. For the regional level, Lee and Lee (2022) constructed a multi-dimensional index of green finance and incorporated the index into the framework of the theoretical model of green productivity. The study found that green finance is one of the basic paths for improving green productivity. In addition, Wang et al. (2021), Wang et al. (2022) and Yin and Xu. (2022) examine the impact of green finance on China’s high-quality development, ecological efficiency, and economic growth, respectively.
To sum up, some scholars have studied the relationship between finance and ecology (Işik et al., 2017; Su et al., 2021), environment and energy (Haas et al., 2021), there are still areas that need to be improved. Although the impact of green finance and the influencing factors of carbon emission efficiency have been discussed, few studies have put green finance and carbon emission efficiency into the same research framework. Second, for related research at the macro level, the existing literature mostly focuses on the impact of green finance on the development of the region, while ignoring the spatial spillover effects or externalities. Based on this, this paper integrates green finance and carbon emission efficiency into the same analytical framework, and explores the local effect and spatial spillover effect of the former on the latter, in order to provide guidance for financial model innovation, the realization of the “dual carbon” goal and sustainable development.
3 Empirical models and variables
3.1 Empirical model
3.1.1 Baseline model
To test the impact of green finance on regional carbon emission efficiency, this paper constructs a two-way fixed effect model of time and individual as follows:
Among them, CEE stands for carbon emission efficiency; i refers to the province; t represents the year;
3.1.2 Spatial durbin model
The impact of green financial development on carbon emission efficiency may not only be limited to this region, but also have a certain impact on neighboring regions. Ignoring spatial correlation may lead to the bias of estimation results. Therefore, this paper uses spatial Durbin model to test the spatial impact of green finance on carbon emission efficiency. The spatial Durbin model can simultaneously examine the influence of green financial development and carbon emission efficiency in neighboring regions on the carbon emission efficiency in this region. In this paper, the spatial Durbin model is established as follows:
Where ρ is the spatial autocorrelation coefficient of the dependent variable; As the spatial weight matrix, according to the particularity of the research variables, this paper considers using economic weight matrix to set the spatial weight. In this paper, the economic weight matrix is constructed according to the average GDP of each region in the sample period.
3.2 Variable measurement
3.2.1 Carbon emission efficiency
CEE is the dependent variable of this paper. SBM model and NDDF model are constructed to calculate GEE of each province. The CEE calculated by the SBM model and the NDDF model are represented by CEE_SBM and CEE, respectively. The inputs indicators include the capital stock of each province (city, autonomous region), the total number of employees in urban units, and energy consumption. The expected output indicators are GDP and carbon emissions, and the unexpected output involves coal, coke, crude oil, gasoline, kerosene, diesel, fuel oil, and natural gas. Based on this, CEE of 30 provinces (municipalities and autonomous regions) in China is calculated. Among them, the perpetual inventory method is adopted to calculate the capital stock of each province.
3.2.2 Green finance
GF is the independent variable of this paper. GF is the independent variable of this paper. GF consists of four indicators: green credit, green investment, green insurance and government support. The specific indicators are as follows:
3.2.3 Other variables
1) Control variables. Eco is the logarithm of real GDP per capita, which is used to indicate the economic growth level of provinces (autonomous regions or municipalities). Economic growth has driven the change of energy consumption structure and changed people’s awareness of environmental protection. Thus, economic growth directly or indirectly affects carbon emission efficiency (Salahuddin et al., 2016; Wu et al., 2021). Ind is the ratio of the added value of the secondary industry to GDP, which is used to indicate the industrial structure of each region. The continuous increase of the proportion of tertiary industry and the continuous decrease of the proportion of secondary industry are the main reasons for the decrease of carbon emission intensity (Zhang et al., 2020). Hum means regional human capital. Human capital is an important support for the continuous optimization of energy structure and the continuous decline of carbon emission intensity (Cheng and Yao, 2021). Urb is the level of urbanization, represented by the ratio of urban population to total population in each region, and the unit is 1. Urbanization mainly affects carbon emission efficiency through population structure, economic structure and energy consumption structure (Wang et al., 2021). 2) Heterogeneous analysis variables. ER is a case of environmental punishment in various regions (unit: 10,000 pieces), indicating the level of environmental regulation. When there are many cases of environmental punishment in a region, we think that the level of environmental regulation in that region is higher. The impact of ER on energy conservation and emission reduction efficiency has been confirmed by scholars (Wu et al., 2020). Table 1 is descriptive statistical analysis.
3.3 Data
Green finance data comes from China Statistical Yearbook, Provincial Statistical Yearbook and China Insurance Yearbook; carbon emission efficiency data comes from EPS (Express Professional Superior) database and the National Bureau of Statistics. The environmental regulation data comes from Chinalawinfo Pkulaw Database. Data for other control variables were obtained from the EPS database.
4 Empirical results and discussion
4.1 Baseline result
In Table 2, columns (1–2) and columns (3–4) are the analysis results using the OLS model and the FE (fixed effects) model, respectively. Among them, columns 1) and 3) do not add control variables, and columns 2) and 4) consider the influence of control variables. The fitting results show that no matter what model is used, and whether control variables are added or not, the coefficient of GF is always significantly positive at the level of 1%, which indicates that the development of green finance can promote the regional carbon emission efficiency and contribute to the realization of the “double carbon” goal. The overall regression results are in line with expectations. The reasons are as follows: First, green finance injects strong power into green technology innovation (Yu et al., 2021). Specifically, under the background of the development of green finance, the funds of financial institutions are inclined to green and high-tech industries, forcing the “two high and one surplus” industries to carry out green technological innovation under the constraint of funds (Wang et al., 2022). At the same time, the green finance policy can not only incite the resources of financial institutions, but also incite social capital to gather in green technology innovation of enterprises, and mobilize the vitality of green technology innovation and research and development of enterprises (Fang and Shao, 2022). Second, green finance contributes to the ecological transformation of industrial structure (Hu et al., 2020). Green finance can exert financing pressure on high-pollution industries, increase their capital cost, and force polluting industries to carry out ecological transformation (Cheng et al., 2022). To sum up, we believe that green finance can improve carbon emission efficiency through green technology innovation and green transformation of industrial structure.
4.2 Spatial estimation result
In the market economy, regional economic activities do not occur independently, but are integrated and influence each other (Griffith, 2021; Feng et al., 2022). Regional linkage development has become the trend of geo-economy (Hao et al., 2021). Studies have also shown that there are obvious spatial correlations between GF (Huang and Chen, 2022) and CEE (Liu and Song, 2020) in different regions. Therefore, this paper uses spatial Durbin model to empirically test the “neighborhood effect” of GF on CEE. Firstly, Moran’s I index is used to test the spatial autocorrelation of carbon emission efficiency. From the test results of Moran’s I index in Table 3, the Moran index of CEE from 2010 to 2019 is significantly positive. In addition, the local scatter plots of 2010, 2013, 2016 and 2019 in Figure 1 once again prove the positive correlation between them. It shows that China’s CEE presents a positive spatial agglomeration.
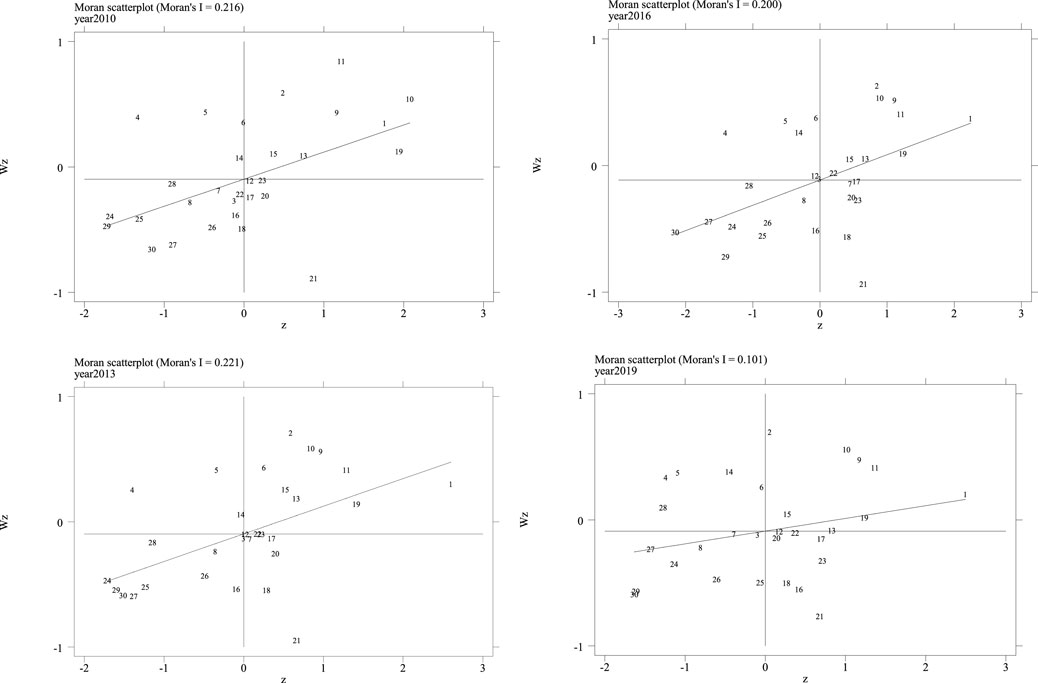
FIGURE 1. Local Moran’I scatter diagram of carbon emission efficiency of China’s provinces in 2010, 2013, 2016, and 2019.
In order to accurately measure the dynamic change of spatial spillover effect in the model, increase the explanatory power of the model and reduce the errors of the model, this paper uses the dynamic spatial model to further test. The direct and indirect effects of the dynamic Durbin two-way fixed effect model include short-term and long-term effects. It can be seen from the estimated results in Table 4: First, the lag term of the dependent variable is obviously not zero, indicating that the CEE of the previous period will promote the development of the current CEE, and it has inertia. Secondly, the estimated coefficients of GF and W*GF in columns (1–2) are all positive, indicating that GF has a positive direct impact on CEE and spatial spillover effect. Third, compared with the short-term spatial effect, the long-term spatial effect is not significant. This shows that in the long run, the influence of green finance on CC in “neighboring” areas is not clear. To a certain extent, this shows that the road of China’s green financial construction still needs to be explored. While pursuing short-term ecological benefits, we should also pay attention to the improvement of long-term green financial mechanism.
4.3 Robustness test
4.3.1 IV estimation result
There are missing variables, measurement errors and two-way causality in the empirical test, which will lead to endogenous problems, so that the regression results can’t converge to the real overall parameters. In order to make the research conclusion credible, this paper uses instrumental variable method to deal with endogeneity. In this paper, the lag period of independent variable is IV, and IV-2SLS regression is carried out. Generally, the selection of instrumental variables needs to meet two conditions: first, correlation: namely, instrumental variables are related to endogenous independent variables; Second, it is exogenous: that is, tool variables are not related to disturbance terms. The IV selected in this paper satisfies these two conditions. And the F value of weak tool variables test is far more than 10. According to the regression results in Table 5, there is a strong correlation between instrumental variables and independent variables, which is significant at 1%. The regression results of the second stage show that the main conclusions of this paper are still valid after dealing with endogenous problems.
4.3.2 Replace the measurement method of dependent variable
In order to ensure the reliability of the research conclusion, this part replaces dependent variables for robustness test. Specifically, the benchmark regression part is measured by NDDF model and replaced by SBM model here. Table 6 shows the results of stepwise regression estimation. With the gradual addition of control variables, the positive effect of GF on CEE still exists, and it is statistically significant. The positive effect of GF on CEE is steady.
5 Heterogeneity analysis of environmental regulation
Regional heterogeneity exists in regions with different economic development and carbon emission efficiency (Ahmad et al., 2021). Green finance expands more financing channels for green enterprises, on the contrary, it restricts the financing of polluting enterprises (Zhang and Wang, 2021). Therefore, it essentially belongs to the market incentive environmental regulation policy. Compared with the “soft constraint” of green finance, the mandatory environmental regulation belongs to the “hard constraint”. Has China’s green financial sector and environmental regulatory authorities achieved synergy, that is, in areas with strong environmental regulation, does GF play a greater role in promoting CEE? In the other case, there is substitution effect between “soft constraint” and “hard constraint”. Therefore, this paper takes the case of regional environmental punishment as the proxy variable of mandatory environmental regulation, and investigates what heterogeneous influence GF has on CEE. See Table 7 for the results: columns (1–2) and (3–4) are OLS and FE estimation results, and columns 1), 3) and 2) and 4) are the results of weak and strong environmental regulation. We found that, no matter the estimation results of OLS or FE, when the environmental regulation is strong, the promotion effect of GF on CEE in this area is weak. It shows that the linkage and coordination mechanism between China’s financial and environmental supervision departments is not perfect, and the two departments should establish a joint law enforcement mechanism to jointly contribute to the “double carbon” goal.
6 Conclusions and policy implications
Promoting the improvement of carbon emission efficiency is the basic requirement to achieve the “double carbon” goal, and it is also the proper path of China’s realization of high-quality and sustainable economic development. Based on the data of 30 provinces in China, this paper makes an empirical analysis, and finds that green finance can significantly promote the carbon emission efficiency in this region; This promotion utility has spatial spillover effect, but this effect is significant in the short term and not significant in the long term; The influence of green finance on carbon emission efficiency is obviously heterogeneous based on environmental regulation.
First, the government is required to establish and improve the green financial development system, and innovate and enrich green financial products. The conclusion of this article shows that green finance can improve the efficiency of carbon emission, so the Chinese government should speed up the innovation of green financial instruments and promote the diversified and sustainable development of green financial market. Specific measures include encouraging banking institutions to develop financing products and services based on the pledge of ecological resources rights and interests, and piloting other innovative green bond products such as green collective bonds, water-saving loans and climate bonds for small and medium-sized enterprises. In addition, China should continue to expand the scope of the green financial reform and innovation pilot zone and promote the business model of the pilot zone.
Second, the government is required to encourage green finance, environmental protection supervision departments, enterprises and other forces to link up, coordinate and extensively carry out cooperation in the field of green finance. Doing a good job in green finance is not something that one department or one region can accomplish alone, and it needs to gather all forces. Specific measures include: increasing financial incentives to guide carbon reduction, increasing the support of financial departments and institutions, and improving the carbon supervision and statistical system. In addition, market-driven environmental regulation policies (such as green finance) and mandatory environmental regulation tools (such as environmental protection law) should coordinate with each other, establish various environmental regulation systems, and work together to promote the realization of China’s “3,060″ dual-carbon goal officially promised to the international community.
Third, the government is required to build a cross-regional cooperation platform for green finance and carry out regional cooperation projects to realize communication and cooperation with surrounding areas. In view of the conclusion that green finance has a positive impact on the carbon emission efficiency of surrounding areas, we think that government departments should encourage green financial institutions to innovate financial products, disclose environmental information and exchange and cooperate with professional and technical personnel, so as to avoid resource waste caused by information asymmetry, redundant construction and disorderly competition, and promote the efficiency of financial resource allocation and use.
This article empirically analyzes the impact of green finance on carbon emission efficiency from the perspectives of “local effect” and “neighboring effect”. Although this article has expanded the research scope of green finance and carbon emission efficiency, there are still some shortcomings, which can also be considered as the future research prospect: First, due to the limitation of data volume, this article only selects provincial data as the research sample, but the data of prefecture-level cities or microcosmic data may be more representative and research-oriented. Second, this article does not deeply study the dynamic impact of green finance on carbon emission efficiency. With the sustainable development of China’s green finance, we will pay attention to it dynamically and strive to make the research conclusion more accurate and perfect.
Data availability statement
The original contributions presented in the study are included in the article/supplementary material, further inquiries can be directed to the corresponding authors.
Author contributions
All authors listed have made a substantial, direct, and intellectual contribution to the work and approved it for publication.
Funding
This work was supported by the General project of philosophy and social science research in Colleges and universities in Jiangsu Province in 2022 “Research on the strategy of green finance adjusting industrial structure under the background of financial risk” (2022SJYB1042) and the Academic subjects of Wuxi Institute of Technology “Research on the mechanism and practice of green finance promoting the construction of modern industrial system” (BS202201).
Conflict of interest
The authors declare that the research was conducted in the absence of any commercial or financial relationships that could be construed as a potential conflict of interest.
Publisher’s note
All claims expressed in this article are solely those of the authors and do not necessarily represent those of their affiliated organizations, or those of the publisher, the editors and the reviewers. Any product that may be evaluated in this article, or claim that may be made by its manufacturer, is not guaranteed or endorsed by the publisher.
References
Ahmad, M., Işık, C., Jabeen, G., Ali, T., Ozturk, I., Atchike, D. W., et al. (2021). Heterogeneous links among urban concentration, non-renewable energy use intensity, economic development, and environmental emissions across regional development levels. Sci. Total Environ. 765, 144527. doi:10.1016/j.scitotenv.2020.144527
Ali, R., Rehman, M. A., Rehman, R. U., and Ntim, C. G. (2022). Sustainable environment, energy and finance in China: Evidence from dynamic modelling using carbon emissions and ecological footprints. Environ. Sci. Pollut. Res. Int. 29, 1–16. doi:10.1007/s11356-022-21337-0
Calvo, R., Amigo, C., Billi, M., Fleischmann, M., Urquiza, A., Álamos, N., et al. (2021). Territorial energy vulnerability assessment to enhance just energy transition of cities. Front. Sustain. Cities 3, 635976. doi:10.3389/frsc.2021.635976
Cheng, Q., Lai, X., Liu, Y., Yang, Z., and Liu, J. (2022). The influence of green credit on China’s industrial structure upgrade: Evidence from industrial sector panel data exploration. Environ. Sci. Pollut. Res. 29 (15), 22439–22453. doi:10.1007/s11356-021-17399-1
Cheng, Y., and Yao, X. (2021). Carbon intensity reduction assessment of renewable energy technology innovation in China: A panel data model with cross-section dependence and slope heterogeneity. Renew. Sustain. Energy Rev. 135, 110157. doi:10.1016/j.rser.2020.110157
Cheng, Z., Li, L., and Liu, J. (2018a). Industrial structure, technical progress and carbon intensity in China's provinces. Renew. Sustain. Energy Rev. 81, 2935–2946. doi:10.1016/j.rser.2017.06.103
Cheng, Z., Li, L., Liu, J., and Zhang, H. (2018b). Total-factor carbon emission efficiency of China's provincial industrial sector and its dynamic evolution. Renew. Sustain. Energy Rev. 94, 330–339. doi:10.1016/j.rser.2018.06.015
Dong, F., Li, Y., Gao, Y., Zhu, J., Qin, C., Zhang, X., et al. (2022). Energy transition and carbon neutrality: Exploring the non-linear impact of renewable energy development on carbon emission efficiency in developed countries. Resour. Conservation Recycl. 177, 106002. doi:10.1016/j.resconrec.2021.106002
Fang, T., Fang, D., and Yu, B. (2022). Carbon emission efficiency of thermal power generation in China: Empirical evidence from the micro-perspective of power plants. Energy Policy 165, 112955. doi:10.1016/j.enpol.2022.112955
Fang, Y., and Shao, Z. (2022). Whether green finance can effectively moderate the green technology innovation effect of heterogeneous environmental regulation. Int. J. Environ. Res. Public Health 19 (6), 3646. doi:10.3390/ijerph19063646
Fareed, Z., Rehman, M. A., Adebayo, T. S., Wang, Y., Ahmad, M., Shahzad, F., et al. (2022). Financial inclusion and the environmental deterioration in eurozone: The moderating role of innovation activity. Technol. Soc. 69, 101961. doi:10.1016/j.techsoc.2022.101961
Feng, Y., Zou, L., Yuan, H., and Dai, L. (2022). The spatial spillover effects and impact paths of financial agglomeration on green development: Evidence from 285 prefecture-level cities in China. J. Clean. Prod. 340, 130816. doi:10.1016/j.jclepro.2022.130816
Gouveia, J. P., Seixas, J., Palma, P., Duarte, H., Luz, H., Cavadini, G. B., et al. (2021). Positive energy district: A model for historic districts to address energy poverty. Front. Sustain. Cities 3, 648473. doi:10.3389/frsc.2021.648473
Griffith, D. A. (2021). Urban economics: Geography and spatial dependence matter to the sustainability of cities. Front. Sustain. Cities 74, 723561. doi:10.3389/frsc.2021.723561
Haas, R., Ajanovic, A., Ramsebner, J., Perger, T., Knápek, J., and Bleyl, J. W. (2021). Financing the future infrastructure of sustainable energy systems. Green Finance 3, 90–118. doi:10.3934/gf.2021006
Hao, Y., Gai, Z., Yan, G., Wu, H., and Irfan, M. (2021). The spatial spillover effect and nonlinear relationship analysis between environmental decentralization, government corruption and air pollution: Evidence from China. Sci. Total Environ. 763, 144183. doi:10.1016/j.scitotenv.2020.144183
He, A., Xue, Q., Zhao, R., and Wang, D. (2021). Renewable energy technological innovation, market forces, and carbon emission efficiency. Sci. Total Environ. 796, 148908. doi:10.1016/j.scitotenv.2021.148908
Hu, Y., Jiang, H., and Zhong, Z. (2020). Impact of green credit on industrial structure in China: Theoretical mechanism and empirical analysis. Environ. Sci. Pollut. Res. 27 (10), 10506–10519. doi:10.1007/s11356-020-07717-4
Huang, R., Zhang, S., and Wang, P. (2022). Key areas and pathways for carbon emissions reduction in Beijing for the “Dual Carbon” targets. Energy Policy 164, 112873. doi:10.1016/j.enpol.2022.112873
Huang, Y., and Chen, C. (2022). The spatial spillover and threshold effect of green finance on environmental quality: Evidence from China. Environ. Sci. Pollut. Res. 29 (12), 17487–17498. doi:10.1007/s11356-021-16892-x
Işık, C., Ahmad, M., Ongan, S., Ozdemir, D., Irfan, M., Alvarado, R., et al. (2021a). Convergence analysis of the ecological footprint: Theory and empirical evidence from the USMCA countries. Environ. Sci. Pollut. Res. 28 (25), 32648–32659. doi:10.1007/s11356-021-12993-9
Işik, C., Kasımatı, E., and Ongan, S. (2017). Analyzing the causalities between economic growth, financial development, international trade, tourism expenditure and/on the CO2 emissions in Greece. Energy Sources, Part B Econ. Plan. Policy 12 (7), 665–673. doi:10.1080/15567249.2016.1263251
Isik, C., Ongan, S., Ozdemir, D., Ahmad, M., Irfan, M., Alvarado, R., et al. (2021b). The increases and decreases of the environment Kuznets curve (EKC) for 8 OECD countries. Environ. Sci. Pollut. Res. 28 (22), 28535–28543. doi:10.1007/s11356-021-12637-y
Isik, C., Ongan, S., and Özdemir, D. (2019). The economic growth/development and environmental degradation: Evidence from the US state-level EKC hypothesis. Environ. Sci. Pollut. Res. 26 (30), 30772–30781. doi:10.1007/s11356-019-06276-7
Kurt, H., and Peng, X.School of Accounting, Xijing University, China (2021). Does corporate social performance lead to better financial performance? Evidence from Turkey. Green Finance 3 (4), 464–482. doi:10.3934/gf.2021021
Lee, C. C., and Lee, C. C. (2022). How does green finance affect green total factor productivity? Evidence from China. Energy Econ. 107, 105863. doi:10.1016/j.eneco.2022.105863
Li, C., and Gan, Y. (2021). The spatial spillover effects of green finance on ecological environment—Empirical research based on spatial econometric model. Environ. Sci. Pollut. Res. 28 (5), 5651–5665. doi:10.1007/s11356-020-10961-3
Liu, H., and Song, Y. (2020). Financial development and carbon emissions in China since the recent world financial crisis: Evidence from a spatial-temporal analysis and a spatial Durbin model. Sci. Total Environ. 715, 136771. doi:10.1016/j.scitotenv.2020.136771
Liu, S., Shen, X., Jiang, T., and Failler, P.Bank of Communications Pacific Credit Card Center, Shanghai, ChinaEconomics and Finance Subject Group, Portsmouth Business School, University of Portsmouth, Portsmouth, United Kingdom (2021). Impacts of the financialization of manufacturing enterprises on total factor productivity: Empirical examination from China's listed companies. Green Finance 3 (1), 59–89. doi:10.3934/gf.2021005
Meng, F., Guo, J., Guo, Z., Lee, J. C., Liu, G., Wang, N., et al. (2021). Urban ecological transition: The practice of ecological civilization construction in China. Sci. Total Environ. 755, 142633. doi:10.1016/j.scitotenv.2020.142633
Meng, F., Su, B., Thomson, E., Zhou, D., and Zhou, P. (2016). Measuring China’s regional energy and carbon emission efficiency with DEA models: A survey. Appl. Energy 183, 1–21. doi:10.1016/j.apenergy.2016.08.158
Pan, W., Gulzar, M. A., Wang, Z., and Guo, C. (2022). Spatial distribution and regional difference of environmental efficiency based on carbon reduction goals: Evidence from China. Front. Environ. Sci. 647, 816071. doi:10.3389/fenvs.2021.816071
Pata, U. K., Shahzad, F., Fareed, Z., and Rehman, M. A. (2022). Revisiting the ekc hypothesis with export diversification and ecological footprint pressure index for India: A RALS-fourier cointegration test. Front. Environ. Sci. 10, 886515. doi:10.3389/fenvs.2022.886515
Rehman, M. A., Fareed, Z., Salem, S., Kanwal, A., and Pata, U. K. (2021). Do diversified export, agriculture, and cleaner energy consumption induce atmospheric pollution in asia? Application of method of moments quantile regression. Front. Environ. Sci. 497, 781097. doi:10.3389/fenvs.2021.781097
Rehman, M. A., Fareed, Z., and Shahzad, F. (2022). When would the dark clouds of financial inclusion be over, and the environment becomes clean? The role of national governance. Environ. Sci. Pollut. Res. 29 (19), 27651–27663. doi:10.1007/s11356-021-17683-0
Rezanezhad, F., McCarter, C. P., and Lennartz, B. (2020). Editorial: Wetland biogeochemistry: Response to environmental change. Front. Environ. Sci. 8, 55. doi:10.3389/fenvs.2020.00055
Salahuddin, M., Alam, K., and Ozturk, I. (2016). The effects of internet usage and economic growth on CO2 emissions in OECD countries: A panel investigation. Renew. Sustain. Energy Rev. 62, 1226–1235. doi:10.1016/j.rser.2016.04.018
Song, Y., Zhang, J., Song, Y., Fan, X., Zhu, Y., Zhang, C., et al. (2020). Can industry-university-research collaborative innovation efficiency reduce carbon emissions? Technol. Forecast. Soc. Change 157, 120094. doi:10.1016/j.techfore.2020.120094
Su, Y., Li, Z., and Yang, C. (2021). Spatial interaction spillover effects between digital financial technology and urban ecological efficiency in China: An empirical study based on spatial simultaneous equations. Int. J. Environ. Res. Public Health 18 (16), 8535. doi:10.3390/ijerph18168535
Sun, W., and Huang, C. (2020). How does urbanization affect carbon emission efficiency? Evidence from China. J. Clean. Prod. 272, 122828. doi:10.1016/j.jclepro.2020.122828
Tsoukala, A. K., and Tsiotas, G.Department of Economics, University of Crete, Panepistimioupolis, Rethymnon 74100, Greece (2021). Assessing green bond risk: An empirical investigation. Green Finance 3 (2), 222–252. doi:10.3934/gf.2021012
Tuladhar, A., Manandhar, P., and Shrestha, K. L. (2021). Assessment of health impact of PM2. 5 exposure by using WRF-chem model in kathmandu valley, Nepal. Front. Sustain. Cities 42, 672428. doi:10.3389/frsc.2021.672428
Ullah, I., Rehman, A., Svobodova, L., Akbar, A., Shah, M. H., Zeeshan, M., et al. (2022). Investigating relationships between tourism, economic growth, and CO2 emissions in Brazil: An application of the nonlinear ARDL approach. Front. Environ. Sci. 10, 843906. doi:10.3389/fenvs.2022.843906
Umar, M., Ji, X., Mirza, N., and Naqvi, B. (2021). Carbon neutrality, bank lending, and credit risk: Evidence from the eurozone. J. Environ. Manag. 296, 113156. doi:10.1016/j.jenvman.2021.113156
Wang, F., Wang, R., and He, Z. (2021). The impact of environmental pollution and green finance on the high-quality development of energy based on spatial Dubin model. Resour. Policy 74, 102451. doi:10.1016/j.resourpol.2021.102451
Wang, M., Gu, R., Wang, M., Zhang, J., Press, B. C. S., and Branch, B. O. C. S. (2021). Research on the impact of finance on promoting technological innovation based on the state-space model. Green Finance 3 (2), 119–137. doi:10.3934/gf.2021007
Wang, Q. J., Wang, H. J., and Chang, C. P. (2022). Environmental performance, green finance and green innovation: What's the long-run relationships among variables? Energy Econ. 110, 106004. doi:10.1016/j.eneco.2022.106004
Wang, R., Zhao, X., and Zhang, L. (2022). Research on the impact of green finance and abundance of natural resources on China's regional eco-efficiency. Resour. Policy 76, 102579. doi:10.1016/j.resourpol.2022.102579
Wang, W. Z., Liu, L. C., Liao, H., and Wei, Y. M. (2021). Impacts of urbanization on carbon emissions: An empirical analysis from OECD countries. Energy Policy 151, 112171. doi:10.1016/j.enpol.2021.112171
Wang, X., and Wang, Q. (2021). Research on the impact of green finance on the upgrading of China's regional industrial structure from the perspective of sustainable development. Resour. Policy 74, 102436. doi:10.1016/j.resourpol.2021.102436
Wolff, M., and Haase, D. (2019). Mediating sustainability and liveability—Turning points of green space supply in European cities. Front. Environ. Sci. 7, 61. doi:10.3389/fenvs.2019.00061
Wu, H., Hao, Y., and Ren, S. (2020). How do environmental regulation and environmental decentralization affect green total factor energy efficiency: Evidence from China. Energy Econ. 91, 104880. doi:10.1016/j.eneco.2020.104880
Wu, H., Xue, Y., Hao, Y., and Ren, S. (2021). How does internet development affect energy-saving and emission reduction? Evidence from China. Energy Econ. 103, 105577. doi:10.1016/j.eneco.2021.105577
Wu, L., Sun, L., Qi, P., Ren, X., and Sun, X. (2021). Energy endowment, industrial structure upgrading, and CO2 emissions in China: Revisiting resource curse in the context of carbon emissions. Resour. Policy 74, 102329. doi:10.1016/j.resourpol.2021.102329
Wyse, S. M., Das, R. R., Hoicka, C. E., Zhao, Y., and McMaster, M. L. (2021). Investigating energy justice in demand-side low-carbon innovations in ontario. Front. Sustain. Cities 3, 633122. doi:10.3389/frsc.2021.633122
Xian, C., and Zhang, D. (2022). Does green finance improve environmental quality? Evidence from the Western region. Regional Financial Res. 596 (03), 24–30. (In Chinese).
Xie, Z., Wu, R., and Wang, S. (2021). How technological progress affects the carbon emission efficiency? Evidence from national panel quantile regression. J. Clean. Prod. 307, 127133. doi:10.1016/j.jclepro.2021.127133
Xu, L., Fan, M., Yang, L., and Shao, S. (2021). Heterogeneous green innovations and carbon emission performance: Evidence at China's city level. Energy Econ. 99, 105269. doi:10.1016/j.eneco.2021.105269
Yang, Y., Su, X., and Yao, S. (2021). Nexus between green finance, fintech, and high-quality economic development: Empirical evidence from China. Resour. Policy 74, 102445. doi:10.1016/j.resourpol.2021.102445
Yin, X., and Xu, Z. (2022). An empirical analysis of the coupling and coordinative development of China's green finance and economic growth. Resour. Policy 75, 102476. doi:10.1016/j.resourpol.2021.102476
Yu, C. H., Wu, X., Zhang, D., Chen, S., and Zhao, J. (2021). Demand for green finance: Resolving financing constraints on green innovation in China. Energy Policy 153, 112255. doi:10.1016/j.enpol.2021.112255
Yu, Y., and Zhang, N. (2021). Low-carbon city pilot and carbon emission efficiency: Quasi-experimental evidence from China. Energy Econ. 96, 105125. doi:10.1016/j.eneco.2021.105125
Zhang, B., and Wang, Y. (2021). The effect of green finance on energy sustainable development: A case study in China. Emerg. Mark. Finance Trade 57 (12), 3435–3454. doi:10.1080/1540496x.2019.1695595
Zhang, F., Deng, X., Phillips, F., Fang, C., and Wang, C. (2020). Impacts of industrial structure and technical progress on carbon emission intensity: Evidence from 281 cities in China. Technol. Forecast. Soc. Change 154, 119949. doi:10.1016/j.techfore.2020.119949
Zhang, H., Jin, G., and Zhang, Z. (2021). Coupling system of carbon emission and social economy: A review. Technol. Forecast. Soc. Change 167, 120730. doi:10.1016/j.techfore.2021.120730
Zhang, S., Wu, Z., Wang, Y., and Hao, Y. (2021). Fostering green development with green finance: An empirical study on the environmental effect of green credit policy in China. J. Environ. Manag. 296, 113159. doi:10.1016/j.jenvman.2021.113159
Zhang, Y. J. (2011). The impact of financial development on carbon emissions: An empirical analysis in China. Energy policy 39 (4), 2197–2203. doi:10.1016/j.enpol.2011.02.026
Keywords: green finance, carbon emission efficiency, China, environmental regulation, sustainable development
Citation: Liang J and Song X (2022) Can green finance improve carbon emission efficiency? Evidence from China. Front. Environ. Sci. 10:955403. doi: 10.3389/fenvs.2022.955403
Received: 28 May 2022; Accepted: 14 July 2022;
Published: 23 August 2022.
Edited by:
Cem Işık, Anadolu University, TurkeyReviewed by:
Mubeen Abdur Rehman, The University of Lahore, PakistanHaitao Wu, Beijing Institute of Technology, China
Copyright © 2022 Liang and Song. This is an open-access article distributed under the terms of the Creative Commons Attribution License (CC BY). The use, distribution or reproduction in other forums is permitted, provided the original author(s) and the copyright owner(s) are credited and that the original publication in this journal is cited, in accordance with accepted academic practice. No use, distribution or reproduction is permitted which does not comply with these terms.
*Correspondence: Jinhao Liang, bGlhbmdqaW5oYW9Ad3hpdC5lZHUuY24=; Xiaowei Song, c29uZ3hpYW93ZWlAc3R1Lm91Yy5lZHUuY24=