- 1Faculty of Economics and Management of Sfax, University of Sfax, Sfax, Tunisia
- 2Higher Institute of Business Administration, University of Sfax, Tunisia. BESTMOD Laboratory, University of Tunis, Tunis, Tunisia
- 3EDC Paris Business School, OCRE Laboratory, Paris, France
Climate change, pollution, drought, and rising seas impede the achievement of the seventh sustainable development goal SDG#7 “affordable and clean energies”. To counter these threats, the use of Renewable Energy (RE) as an alternative to conventional energy plays an important role in sustainable development. In this context, the purpose of our paper is to investigate the effect of Renewable Energy deployment on environmental protection in China, The United States of America (USA), and Germany: the top three ranked countries in terms of REs production, according to RENEWABLE 2021 GLOBAL STATUSREPORT. To achieve this objective, the paper adopts a Panel fully modified OLS (FMOLS) method. Results declare that renewable energy significantly reduce pollution indicators; furthermore, we find that Research and development fully moderate this relationship. The findings of this study emphasize the importance of increasing spending on Research and development activities in the RE sector. In addition, the countries studied and countries around the world should pay greater attention to investment in research and development to support the long-term plan for advancing sustainable energy sources for feasible energy and economic development.
1 Introduction
Climate change is an extreme threat to the survival of the planet and humanity in the 21st century. To deal with it, the use of renewable energies is an essential element. RE are the fastest-growing energy source, approximately, 11.2% of the world’s energy consumed for heating, electricity and transportation came from modern renewable (biomass, geothermal, solar, hydro, wind and biofuels) in 2019, compared to 8.7% in 2009 (Center for Climate and Energy Solutions, 2020).
Certain past events, and even crises, provide a favorable environment for turning to renewable energy. For example, high volatility of oil prices, dependence on these foreign resources, power generation mix1, environmental changes and significant pressure to reduce polluting gas emissions are all major concerns that have reinforced the need to convert non-renewable resources to renewable ones (Ullah et al., 2021). Without going back much in time, we take the case of the COVID-19 crisis, thus, in the midst of the COVID-19 pandemic, renewable energy production increased by about 3% (World Nuclear Industry Status Report, 20202). Renewable energies are resisting the crisis. Thus, even though the crisis has stopped renewable energy projects under construction or in development, it has little effect on existing capacities. COVID-19 crisis has led to substantial reductions in global electricity demand due to movement limits, lockdowns, and the economic downturn. For example, compared to April 2019, electricity consumption decreased by 5% in the United States in April 2020 and by 12% in Germany (Mahian et al., 2021). However, this pandemic indirectly contributed to the achievement of the UN Sustainable Development Goals for 2030 (namely “SUSTAINABLE CITIES AND COMMUNITIES”, “SUSTAINABLE CONSUMPTION AND PRODUCTION”, “MEASURES TO COMBAT CLIMATE CHANGE”, and “LAND LIFE”) by enhancing the overall health and safety of cities through reducing greenhouse gas (GHG) emissions, outdoor air pollution, land pressure, and wildlife.
In 2019, China played a leading role in investment in renewable sources (83.4 billion USD), the USA a second-tier role (55.4 billion USD), (Frankfurt School-UNEP Centre, Bloomberg NEF, 2020)3. The increase of the share of renewable in the electricity mix (due to ambitious renewable energy policies) and the continued decline in the costs of solar and wind technologies have contributed to a strong increase in renewable energy production, especially since 2010 in Europe (such countries as the United Kingdom (+33 pts. to 41%) and Germany (+24 pts. to 41%)), China (+10 pts. to 29%), and the USA (+10 pts. to 21%) (Enerdata, 2021). Despite their high share of renewable energy in the energy mix, these countries are not really so clean. It is for all these reasons that our paper focuses on these countries.
Energy consumption and environmental quality are among the most important determinants of human development (Hung, 2021). The existing literature on the impact of RE use in the reduction of pollution indicators mostly focused on the direct impact (Magazzino et al. (2021); Destek and Aslan, (2020); Ma et al. (2021)…). While the implementation of Research and development will further promote the reduction of pollution indicators. This paper applies a Panel Fully Modified Least Squares (FMOLS) Method to explore the pollution indicators reduction caused by the use of renewable energies, and further, studies whether Renewable energies achieve the effect of reducing pollution indicators through Research and development expenditures. Similarly, as an extension of the research, this paper exploits robustness analysis, thus, we further, tested the direct and indirect effects through other measures of renewable energies. Given the extant findings, our results show a negative relationship between REs and pollution, thus, the use of renewable energies have been reduced the effect of pollution indicators. In addition, we find that Research and development fully moderate this relationship.
Our paper makes contributions to the existing literature: 1) it belongs to the literature of the generation mix, which has the merit of being very useful both on a purely scientific level and on a practical and institutional level. 2) This is the first attempt to extend the previous literature by sampling the top three renewable energy leaders (China, USA and Germany). This choice gives robustness and applicability to real contexts to the results obtained. 3) Our paper treats each pollution indicator individually, moving from the general to the specific framework. 4). And importantly, since previous studies have ignored the moderating effect of research and development in the relationship between the use of renewable energies and the reduction of pollution indicators, our paper aimed to bridge this gap in the literature. Thus, integrating research and development work into the process of the energy transition, will achieve the various long-term energy and climate objectives.
Our paper can be useful for both academics and policymakers. For academics, it can provide an empirical basis for further research into the support of RE and its role in reducing or even eliminating pollution through Research and development in order to achieve a low-carbon world. For policymakers, an overview of the policies made by these leading countries may help inform their policymaking.
With the aim of achieving the purpose of this research, the remainder of this paper proceeds as follows: Section 2 presents a discussion of practices implemented by the studied countries and gives an overview of the previous literature. Then, the study design is introduced in Section 3. The impact of RE use on air pollutants emissions and the moderating role of R&D expenditure are outlined in Section 4. Finally, the conclusion highlights the contribution of this paper, policy implications and possible future research.
2 Approaches and strategies to enhancing energy security
In this study, the research framework was developed based on the “Energy security theory”. This theory shows that changes in the energy sector could profoundly alter the future energy outlook, and that the shift to renewable energy has positive climate and environmental effects. Energy security is a component of environmental security and community sustainability (Hossain et al., 2016). It is primarily concerned with whether governments have control over their energy production and supply resources. Energy security is also equated with the access of the poorest to energy services, or the mechanism that limits the dominance of a single energy system (Kuik et al., 2011). Adopting the concept of energy security is intended to emphasize the role of government and local institutions in protecting the interests of consumers, whether they are private or public entities. Energy security could be described as the state of the economy that allows for the current and future supply of both fuels and energy in a technically and economically justified manner while meeting environmental protection requirements.
2.1 Country strategies in renewable energy
China: The Chinese government has established a series of top-down policy initiatives related to environmental protection. Therefore, in 2013, the Chinese State Council established the National Action Plan for Air Pollution Control and Reduction (China State Council, 2013). In addition, in a restructuring process of the central government, the Ministry of Ecology and Environment was established and tasked with supporting better pollution control. China contributed the most to the growth of the renewable energy sector (1.0 EJ), followed by the United States (0.4 EJ) (BP statistical reviews of world energy, 2021)4. During the 2015 United Nations climate conference, China committed to reducing carbon intensity in 2030 by 60–65% compared to 2005 levels. Figures 1, 2 illustrate the global evolution of wind and solar capacity over the period 2010–2020. Global wind capacity has increased by 110 GW in 2020, while solar capacity is 128 GW during the same year, despite the massive disruptions associated with the global COVID-19 pandemic. Both figures prove that China accounts for nearly half of this global increase. Hence, China is the main driver of wind and solar capacity with 71 GW and 50 GW respectively in 2020.
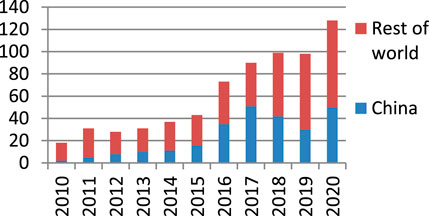
FIGURE 2. Solar capacity (Annual change, GW). Source: BP statistical reviews of world energy (2021).
United States of America (USA): Renewable energy represents the fastest-increasing source of energy globally and particularly in the United States, with a 90% increase between 2000 and 2020. They accounted for nearly 20% of U.S. electricity generation in 2020 on an industrial scale (Center for Climate and Energy Solutions, 2020). The United States signed the American Recovery and Reinvestment Act (ARRA) in2009 after the financial market collapse that led to the great recession. The ARRA was an unprecedented $900 billion economic stimulus package that included a series of measures for investment opportunities in renewable energy and energy efficiency (Mullen and Dong, 2022). Similarly, the proposed $1.2 trillion infrastructure bill for 2021 includes $500 million for renewable energy projects.
Several states have adopted renewable portfolio standards (RPS), with various goals and target dates. Figure 3 shows the details of state RPS programs. Thus, as of September 2020, 38 states and the District of Columbia had established an RPS or renewable energy goal, and in 12 of those states (and the District of Columbia), the requirements are for 100% clean electricity by 2050 or earlier. In addition to renewable energy standards, this map clearly shows that some states have programs in place that provide additional credits for solar or customer-installed renewable; various types of renewable space and water heating; fuel cells; energy efficiency measures and advanced fossil fuel technologies (Washington, Oregon, Michigan, Colorado, Texas, Arizona, and Utah). Other states have clean energy targets or goals, defining terms such as “carbon-free,” “carbon-neutral” or “clean energy”. As an example, 5 states have a clean energy standard and another 5 states have clean energy goals.
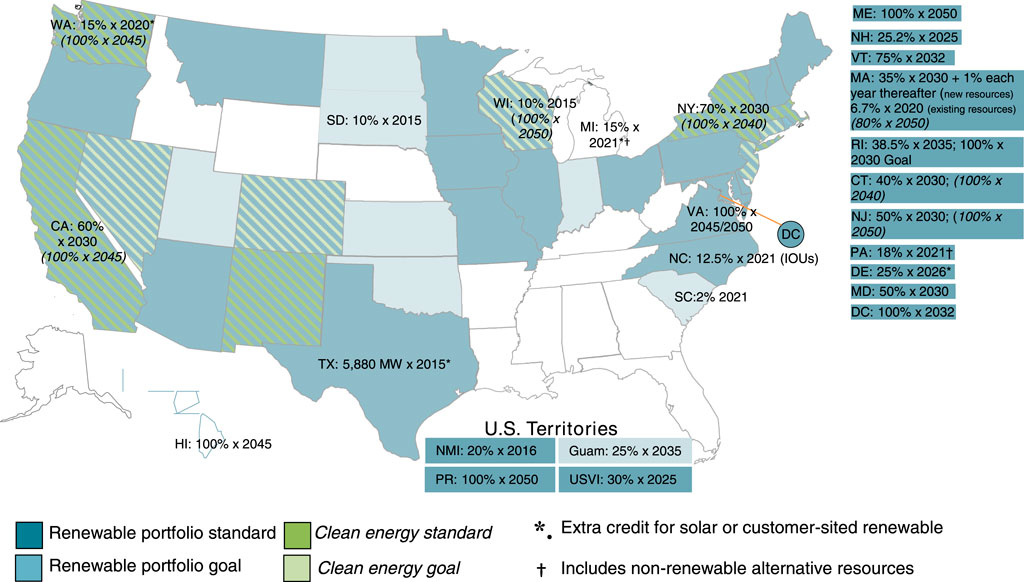
FIGURE 3. Figure 3: Summary map of Renewable and Clean Energy Standards in the USA. Source: Database of State Incentives for Renewable & Efficiency (www.dsireusa.org/ September 2020)
Germany: Together with France, Germany played a leading role in the Paris Agreement within and outside the European Union. They have set up various collaborative research programs, one of which is “Make Our Planet Great Again”, which invites researchers from around the world to fight global warming and climate change through various projects, both large and small. Figure 4 shows the production of renewable energies in Germany for the year 2020 in terawatt hours by energy type. Wind energy has the largest share with 23.4% representing 134.5 TWh, followed by geothermal energy; Biomass; Hydropower and finally Household waste with 1.0% (5.9 TWh).
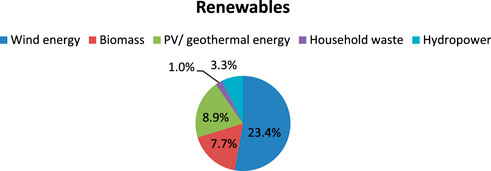
FIGURE 4. German gross renewable production in the year 2020 in (TWh). Source: Working Group on Energy Balances, (2020)5
The share of renewable in gross electricity consumption in Germany has increased significantly in recent years from 36.0% in 2017 to 45.7% in 2020, far exceeding the target of 35% set for that year (BMWi, 2021). Germany has also adopted a comprehensive package of measures for funding climate-related research and innovation under this program. One of the key elements of this 2030 climate action program is the introduction of a new carbon pricing system for the transport and heat sectors starting in 2021. On 14 August 2020, Germany also introduced a coal phase-out law, aiming to reduce or even stop coal-fired power generation by 2038. Figure 5 shows the stages of production reduction. Thus, coal- and lignite-fired generation is to be reduced from about 41 gigawatts (GW) in 2019 to 15 GW each in 2022 and eight to 9 GW in 2030. The phase-out is to be achieved no later than 2038. Germany’s Climate Action Plan 2050 serves as a framework for German climate policy and the achievement of the long-term goal of greenhouse gas neutrality by 2050.
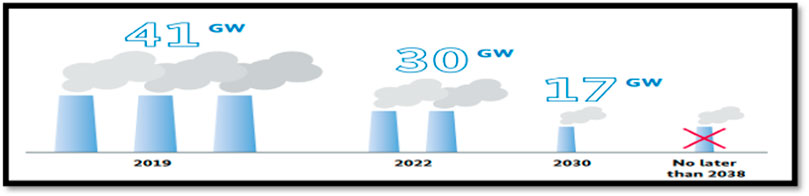
FIGURE 5. Schedule for coal phase-out in Germany. Source: BMWi (2021)
Table 1 shows the renewable energy production by type of energy in the surveyed countries (2019–2020). China is the most productive country with 742.0 terawatt-hours in 2019 and 863.1 terawatt-hours in 2020, followed by the USA and Germany. The total growth rate for the year 2020, adjusted for leap years is 16.0%; 13.8% and 4.1% in China, USA and Germany respectively. To these three world leaders in renewable energy production are added India (151.2 terawatt-hours); United Kingdom (127.8 terawatt-hours); Japan (125.6 terawatt-hours) and then, Brazil (120.3 terawatt-hours) (BP Statistical Review of World Energy 2021).
2.2 Prior literature and hypotheses development
There is vast literature on innovations in the energy sector and the use of renewable energies as alternatives to traditional energies. Through an empirical study, Destek and Aslan, (2020) reported that increasing biomass energy consumption reduced carbon emissions in Germany and the United States. Likewise, Biomass energy consumption decreases long-term Carbon dioxide (CO2) emissions in the U.S. for the monthly period 1984–2015, claimed Bilgili et al. (2016) using wavelet coherence analysis. Destek and Aslan, (2020) explored the multivariate relationship between the consumption of disaggregated renewable energy, economic growth and environmental pollution for the G-7 countries from 1991 to 2014. In this study, both a parametric and a causal approach are used, taking into account cross-sectional dependence and country-specific heterogeneity. The results showed that hydropower consumption appears to be the most effective renewable energy source for reducing carbon emissions in the G-7 countries. Ma et al. (2021) provided evidence regarding the positive effect of renewable energy in reducing carbon emissions in Germany and France, during the time when non-renewable energy consumption adds to carbon emissions. The analysis relied on several recently developed robust methods: cross-sectional correlation, panel unit root tests, panel co-integration tests, panel FMOLS and DOLS methods, panel vector error correction model, and causality testing. Based on the above description, the first hypothesis is specified as follows:
Hypothesis H1: The use of RE has a negative impact on pollution indicators.
Throughout the world, research and development efforts must focus on renewable energies. Thus, in order to make such large-scale deployments possible, continued research and development efforts are required, with the primary goal of ensuring economic feasibility. Larger devices and power plants hold the promise of economies of scale, especially lower energy costs (Blaabjerg and Ionel, 2015). Research and development activities in the renewable energy sector include new devices and system installations for power plants. Adedoyin et al. (2020) used data from 1997 to 2015 for 16 EU countries to demonstrate how research and development spending boosts growth in the presence of renewable and non-renewable energy consumption. Blaabjerg and Ionel, (2015) present research and development opportunities for each type of renewable energy, for example for Wind -On-shore (Wind farm interconnection technologies, Wind energy converters based on new solid state devices), Wind-Offshore (Interconnection of onshore and offshore wind farms), Hydrogen (new methods of hydrogen storage and transport, Thermal Management) and Wave Energy (going further offshore, to greater depths and with higher waves, synergistic research with the offshore wind industry with shared infrastructures). Zhu et al. (2020) explored, for 31 Chinese provinces during 2011/2017, the relationship between renewable energy technology innovation (RETI) and air pollution through Moran’s Index and spatial panel econometric models. Results indicated that investments in renewable energy technologies contribute to lower concentrations of nitrogen oxides (NOx) and respirable suspended particulate matter (PM10), whereas they are not significantly associated with sulfur dioxide (SO2). With an innovative complex ML model called “Quantum”, Magazzino et al. (2021) confirmed the powerful role of biomass energy in carbon dioxide emission reductions in Germany using a linear Harrow-Hassidim-Lloyd (HHL) method, and those, considering the data set as a set of non-linear equations. The authors showed that the effect of renewable energy technology is far greater than biomass energy in reducing emissions. Lin and Zhu, (2019) investigated the link between Renewable Energy Technology Innovation (RETI) and CO2 emissions. The results of linear regression analysis show a clear effect of RETI on CO2 reduction based on the energy structure, similarly, observations on threshold test results confirm that the energy consumption structure dominated by coal will hinder RETI’s CO2 reduction effect. Through a case study of the United Arab Emirates, Kolsi and Al-Hiyari (2022) highlighted the CSR disclosure practices of Masdar Co. (a leading renewable energy and sustainability group in the United Arab Emirates and the Middle East region) against the Global Reporting Initiative GRI 2016 standards. The results of the study show that Masdar’s Co. has boosted its brand by considering valuable CSR practices. It uses key dimensions such as ethics, law and philanthropy to manage and coordinate effective interactions with society. Masdar Co. was also found to be more successful in complying with the GRI 2016 standards in GRI 100 general disclosures, GRI 200 economic disclosures, GRI 300 environmental disclosures, and GRI 400 social disclosures. Horbach and Rammer, (2018) discussed the specific role of the regional environment of German firms in the ability to adopt energy technologies using renewable sources, using two-stage mixed effects models and the Probit model with clustered standard errors. They found that geographic proximity to renewable energy-based power generation and a region’s orientation toward “green issues” are both correlated with these innovations.
However, the impact of the use of renewable energies on the protection of the environment from polluting air cannot be done in isolation; it requires heavy investment in research and development, digitization of the energy transition, conscious and intentional government policies, as well as increased opportunities for foreign investment. Research and development activities occupy the most important place. Thus, the second hypothesis to be tested in this study is as follows:
Hypothesis H2: The implementation of R&D moderates the relationship between RE and Pollution indicators.
3 Research design
To evaluate the effect of Renewable energies on pollution indicators, we gather data on the RE use, pollution indicators such as Total greenhouse gas emissions,CO2 emissions, Other GHG emissions, (HFC, PFC and SF6) and other variables of interest. This section presents the data, the variables and the methodology.
3.1 Data and variables
The model was implemented by collecting panel data for the period 1990–2020 depending on data availability for China, the USA and Germany. The choice of these countries is explained by the fact that they rank in the top three in terms of renewable energy production, according to the RENEWABLE 2021 GLOBAL STATUSREPORT. China remains the top bioenergy producer, followed by the United States and Germany. So, they are lead markets in renewable energy.
Table 2 provides the variables’ definitions and measures, as well as data sources.
In order to identify the effect of renewable energy use on pollution indicators for China, the USA and Germany, we estimate econometric models where the dependent variable is pollution expressed by three indicators: Total greenhouse gas emissions; CO2 emissions and other greenhouse gas emissions, (HFC, PFC and SF6). Each variable is defined in Table 3:
The model equation is as follows:
Since our first dependent variable includes all greenhouse gas emissions, we perform a second evaluation that treats CO2 and fluorinated gas emissions separately to find out which pollution indicator will be impacted by the use of renewable energy. This leads to the following equations.
To test the moderating role of Research and development in this relationship, the panel regression models took the following form:
i = 1, 2…….N: the number of years.
t = 1, 2 …… T, T corresponds to the number of countries.
3.2 Methodology
3.2.1 Panel unit root tests
Before building the panel regression models, we test the stability of all variables. A variety of tests exist for unit roots or stationarity in panel data sets. These include the tests of Harris-Tzavalis (1999); Breitung (2001); Fisher (2001); Levin et al. (2002) and Im et al. (2003). All of these tests have the null hypothesis that the panels have a unit root, only the Lagrangian multiplier (LM) test of Hadri (2000) has the null hypothesis that all panels are stationary (trend). Since our data set is an unbalanced panel, we adopted the Im-Pesaran-Shin and Fisher tests to check the stationarity of our variables.
3.2.2 Panel co-integration tests
We apply a co-integration test to check whether there is a long-run relationship between dependent and independent variables. We follow the residual Kao co-integration test proposed by Kao (1999). Kao’s null hypothesis assumes that there is no co-integration between the variables. The panel co-integration test proposed by Kao (1999) is as:
In order to verify the robustness of our results, we use another cointegration test, namely the test of Pedroni (2004) which model form will be as:
3.2.3 Panel fully modified least squares (FMOLS)
Since the results of the Kao and Pedroni tests confirmed the cointegration between the variables, and because our model contains a small sample, we use the FMOLS method to test our research hypotheses. Kao and Chiang, (2001) have proved that FMOLS outperform for a small sample.
4 Results
This paper studies the negative relationship between RE use and pollution indicators emissions. In order to better analyze the relationship between the two, descriptive analysis, correlation analysis, unit root tests, co-integration tests, fully modified least squares (FMOLS)method, moderating effect analysis, and robustness test were carried out.
4.1 Results of tests
The statistical descriptions of all variables used in our study are presented in Table 4. These statistics (mean, median, minimum value, maximum value, standard deviation) give us an idea of the evolution of the data over time.
Similarly, we checked the independence of the variables to ensure that there were no problems of multicollinearity that might affect our results. The correlations between the variables identified in the model are presented in Table 5. Overall, the results show no problems with collinearity between the independent variables, as multicollinearity can be a problem when the correlation is >0.80 (Kennedy, 2008). In this regard, the estimation is valid and robust.
The results of the panel unit root tests mentioned in Table 6 reveal that the p-value of all variables in 1st difference is lower than the significance level alpha = 0.01, we have to reject the null hypothesis H0, and retain the alternative hypothesis H1 of stationarity of the series. Thus, the first difference of all the variables is stationary. Only the variables “OGHG”, “GDP” and “CH” are stationary in level.
Table 7 reports the results of co-integration tests. The null hypothesis of no cointegration is rejected for all models. All five tests used support the co-integration hypothesis, rejecting the null hypothesis at the 1% level. Table 7 is providing evidence that all panels of the data are cointegrated, which confirms a robust long-term association between the variables.
4.2 Results of FMOLS
Results from Models 1, 2 and 3 from Table 8 reveal that FMOLS yield nearly similar results in terms of sign, magnitude, and statistical significance. Combustible renewable and waste “CRW” have a significant negative effect on TGHG, OGGE and CO2E, this result clearly shows that the use of solid biomass, liquid biomass, biogas, industrial waste and municipal waste reduces emissions of air pollutants. This result is consistent with the most recent literature on the catalytic role of biomass energy in reducing air pollution. Indeed, Magazzino et al. (2021) confirmed that biomass energy consumption significantly reduces CO2 emissions in Germany. Similarly, Bilgili et al. (2016); Bilgili et al. (2017) argued that biomass energy mitigates the level of emissions in the USA.
Alternative and nuclear energy “ANE” has a negative impact on “OGHG” and “CO2E”, but an unexpected significant positive effect on “LNTGHG”, this surprising result can be explained to our knowledge by the fact that some renewable energy emits greenhouse gases during the production of energy devices. These emissions are much lower than those of fossil fuels.
For the variable “GDP”, it has a significant positive effect on the emission of industrial gases (OGHG), an increase in gross domestic product in turn increases the emissions of fluorinated gases: (HFCs; PFCs and SF6). To the best of our knowledge, these results are clearly explained by the economic structure of the mentioned countries, especially China, which is generally based on secondary industry, contributing significantly to air pollutant emissions, China consumed nearly 70% (69.77%) of the secondary industry of its total energy consumption (Lin and Zhu, 2019), with the main energy consumption in China is still fossil fuels (Zhu et al., 2020). Similarly, Germany still relies heavily on fossil fuels (especially coal). This finding is reinforced by the variable Industry (including construction) which has a significant positive effect on the “OGHG”. Our result is consistent with the work of Dong et al. (2019), Lin and Zhu (2019) and Zhu et al. (2020).
Our empirical findings support the “Energy security theory”. This theory states that rapid technological changes in the energy sector could radically alter future energy prospects, while the shift to renewable energy has positive effects on the climate and the environment.
4.3 Moderating effect
In addition to the direct effect, this study discusses the moderating effect. In accordance with the mentioned assumptions, this paper tests the Research and development activities as a moderating variable; the results are presented in Table 9.
It can be seen from Table 9 that the Research and development activities have played a significant positive moderating effect between RE and Pollution indicators reduction. This shows that after the use of RE, pollution indicators can be decreased; this effect accelerates even further after the integration of research and development. In order to enable such large-scale deployments, continued research and development efforts are required, with an emphasis on economic sustainability (Blaabjerg and Ionel, 2015). Thus, supporting research, development and innovation is one of the key elements of the energy transition to accompany the corresponding sectors towards maturity, competitiveness and the long-term targets of environmental protection.
4.4 Robustness test
To check the robustness of our results, we exploited a robustness analysis. We re-estimate all models using the variable “REC” (Renewable Energy Consumption) as a new measure of RE.
To check the robustness of our results, we exploited a robustness analysis. We re-estimate all models using the variable “REC” (Renewable Energy Consumption) as a new measure of RE. Table 10 presents the results found following the integration of new variables, Renewable energy consumption “REC” has a significant negative effect on air pollutants, which indicates that the increase in “REC” is beneficial for reducing pollution indicators. Our results are similar to those obtained by Ma et al. (2021) who demonstrated a significant reduction in carbon emissions from renewable energy in Germany and France.
Table 11 presents the results found after introducing the moderating effect of the R&D variable. The results using a new measure appear very similar to the original results of the basic model. Thus, the use of renewable energies is perceived as a slowing down of the pollution indicators.
The results of the robustness test prove that there is a significantly negative relationship between the use of renewable energy and the reduction of air pollution, with investment in research and development activities strengthening this negative relationship.
Results from the alternative measure “REC” confirm the robustness of our results.
5 Discussion
Our findings are consistent with previous literature that links renewable energy use with decreases in pollution indicators. Thus, several research studies have shown that the use of renewable energies is very effective in the mitigation of environmental pollution. Renewable energy is one of the solutions to climate change mitigation. The growth of renewable energy yields significant reductions of carbon emissions (Kelly et al., 2019) and overall ecological footprint reduction (Alola et al., 2019). Therefore, the achievement of climate change mitigation requires the promotion of renewable energy consumption. Thus, the continuous increase in energy demand is among the factors that have significantly influenced policy makers to encourage local communities and private sectors to play their role in sustainable energy production. This covers the saturation of human welfare, and the consequent impairment of human health due to environmental pollution (Hung, 2021). For the countries studied, the real problem does not lie in the production of these renewable energies, but really in their uses, taking for example the case of China, although it presents the first producer of these energies, it is currently the largest carbon emitter. This is explained by the low use of these energies. In this context, a better integration of renewable energies in the industrial sector of the countries studied is highly recommended.
While existing works on this topic only deal with the CO2 variable, we fill the gap in the literature and treat each pollution indicator individually, moving from the general framework (“Total Greenhouse Gas Emissions”) to the specific framework (“CO2 Emissions” and after “Other Greenhouse Gas Emissions” which includes only fluorinated gas emissions (HFCs, PFCs and SF6)). The consumption of renewable energy has very little negative impact on the environment because it does not produce waste or polluting gas emissions. This means that renewable energies offer a global solution because they do not inject polluting gas emissions into the environment. The results suggest the introduction of renewable energies and the support of research and development projects. The development of research and innovation in renewable energies is highly recommended in order to find profitable solutions and benefit from the use of inexhaustible sources such as the sun or the seabed for energy supply. For this reason, Governments around the world must support research and development activities. In addition, dedicated calls for research and innovation projects can also accelerate the development of renewable energies by providing specific support to project developers.
6 Conclusion
Given the growing importance of long-term sustainable goals over short-term goals, as well as the importance of this issue, and since we all know the firm belief that “there is no other Planet B to live on”, this paper aims to present the renewable energy practices for the panel of China; USA and Germany, and to determine whether these efforts have contributed to reducing environmental problems and enjoying a clean world. Panel fully modified OLS method showed that the deployment of renewable energies has significantly contributed to the decrease of polluting gas emissions; also, Research and development fully moderate this relationship. The results of the empirical analysis support the research hypotheses, and confirm on the one hand that the use of RE can reduce emissions, and on the other hand that R&D spending reinforces this relationship. For the studied countries, the real problem does not lie in the production of these renewable energies, but really in the right use, taking, for example, the case of China, although it presents the first producer of these energies, it is currently the largest carbon emitter. In this context, better investment in R&D activities is highly recommended.
To enhance energy security and reduce sensitivity to fluctuations in conventional energy prices, we recommend i) digitization of the energy transition so as to equip as many metering points as possible with smart meters and to maximize the use of secured gateways for the implementation of energy transition-related applications; ii) Investing in the most efficient sustainable energy source to reduce environmental pollution in each country, could be a rational policy; iii) Integrate research and development work into the process of energy transition and climate action, to achieve the various long-term energy and climate objectives. And finally, Place energy and climate policy efforts in an international context to achieve long-term goals and ensure the competitiveness of the global economy.
Due to the rise in the involvement of environmental organizations and climate activists, as well as the increasing interest in sustainability goals, corporate social responsibility (CSR), and ESG criteria by various stakeholders, more studies on the importance of innovation and sustainability in the energy sector should be conducted.
Future research could also be expanded for firms in other sensitive sectors for example tourism and transport. An analysis of the differences between socially responsible and non-responsible companies can also be conducted in order to draw further conclusions about the need to be committed to humanity, environment and society.
Data availability statement
The datasets presented in this study can be found in online repositories. The names of the repository/repositories and accession number(s) can be found below: https://databank.worldbank.org/reports.aspx?source=world-development-indicators.
Author contributions
All authors listed have made a substantial, direct, and intellectual contribution to the work and approved it for publication.
Conflict of interest
The authors declare that the research was conducted in the absence of any commercial or financial relationships that could be construed as a potential conflict of interest.
Publisher’s note
All claims expressed in this article are solely those of the authors and do not necessarily represent those of their affiliated organizations, or those of the publisher, the editors and the reviewers. Any product that may be evaluated in this article, or claim that may be made by its manufacturer, is not guaranteed or endorsed by the publisher.
Footnotes
1The European Union’s energy mix was composed mainly of five different sources: petroleum products (including crude oil) (35%), natural gas (24%) and renewable energy (17%). %), nuclear power (13%) and solid fossil fuels (12%).
2Retrieved from World Nuclear Industry Status Report 2020 (worldnuclearreport.org).
3(Frankfurt School-UNEP Centre, BloombergNEF, “GLOBAL TRENDS IN RENEWABLE ENERGY INVESTMENT 2020”).
4Retrieved from:https://www.bp.com/content/dam/bp/business-sites/en/global/corporate/pdfs/energy-economics/statistical-review/bp-stats-review-2021-full-report.pdf.
5https://mining-report.de/english/blog/tag/working-group-on-energy-balances-ageb/.
References
Adedoyin, F. F., Bekun, F. V., and Alola, A. A. (2020). Growth impact of transition from non-renewable to renewable energy in the EU: The role of research and development expenditure. Renewable Energy. néerlando-britannique: Elsevier. doi:10.1016/j.renene.2020.06.015
Alola, A. A., Yalçiner, K., Alola, U. V., and Saint Akadiri, S. (2019). The role of renewable energy, immigration and real income in environmental sustainability target. Evidence from Europe largest states. Sci. Total Environ. 674, 307–315. doi:10.1016/j.scitotenv.2019.04.163
Apergis, N., and Payne, J. E. (2012). Renewable and non-renewable energy consumption growth nexus: Evidence from a panel error correction model. Energy Econ. 34 (3), 733–738. doi:10.1016/j.eneco.2011.04.007
Bilgili, F., Koçak, E., Bulut, Ü., and Kus¸kaya, S. (2017). Can biomass energy be an efficient policy tool for sustainable development? Renew. Sustain. Energy Rev. 71, 830–845. doi:10.1016/j.rser.2016.12.109
Bilgili, F., Öztürk, İ., Koçak, E., Bulut, Ü., Pamuk, Y., Muğaloğlu, E., et al. (2016). The influence of biomass energy consumption on CO2 emissions: A wavelet coherence approach. Environ. Sci. Pollut. Res. 23 (19), 19043–19061. doi:10.1007/s11356-016-7094-2
Blaabjerg, F., and Ionel, D. M. (2015). Renewable energy devices and systems–state-of-the-art technology, research and development, challenges and future trends. Electr. Power Components Syst. 43 (12), 1319–1328. doi:10.1080/15325008.2015.1062819
Bmwi, (2021). Federal Ministry for economic affairs and energy. Retrieved from Available at: https://www.cleanenergywire.org/experts/bmwi-federal-ministry-economic-affairs-and-energy.
Breitung, J. (2001). “The local power of some unit root tests for panel data,” in Nonstationary panels, panel cointegration, and dynamic panels (Emerald Group Publishing Limited). 978-0-76230-688-6.
British Petroleum Company, (2021). BP statistical review of world energy, reviewing world energy data for 70 years. 70th edition. LondonPrint: British Petroleum Co, 1–72. Retrieved from: Available at: https://www.bp.com/content/dam/bp/business-sites/en/global/corporate/pdfs/energy-economics/statistical-review/bp-stats-review-2021-full-report.pdf.
Center for Climate and Energy Solutions (2020). Renewable energy. Available at: https://www.c2es.org/content/renewable-energy.
China State Council, (2013). Daqi WuranFangzhiXingdongJihua [national action plan on air pollution]. Beijing).
Choi, I. (2001). Unit root tests for panel data. J. Int. money Finance 20 (2), 249–272. doi:10.1016/S0261-5606(00)00048-6
Destek, M. A., and Aslan, A. (2020). Disaggregated renewable energy consumption and environmental pollution nexus in G-7 countries. Renew. Energy 151, 1298–1306. doi:10.1016/j.renene.2019.11.138
Dogan, E., and Aslan, A. (2017). Exploring the relationship among CO 2 emissions, real GDP, energy consumption and tourism in the EU and candidate countries: Evidence from panel models robust to heterogeneity and cross-sectional dependence. Renew. Sustain. Energy Rev. 77, 239–245. doi:10.1016/j.rser.2017.03.111
Dong, K., Hochman, G., Kong, X., Sun, R., and Wang, Z. (2019). Spatial econometric analysis of China’s PM10 pollution and its influential factors: Evidence from the provincial level. Ecol. Indic. 96, 317–328. doi:10.1016/j.ecolind.2018.09.014
GSR (2021). Renewables 2021 global status report (2021). A comprehensive annual overview of the state of renewable energy. Retrieved from: Available at: https://www.ren21.net/wp-content/uploads/2019/05/GSR2021_Full_Report.pdf.
Harris, R. D., and Tzavalis, E. (1999). Inference for unit roots in dynamic panels where the time dimension is fixed. J. Econ. 91 (2), 201–226. doi:10.1016/S0304-4076(98)00076-1
Horbach, J., and Rammer, C. (2018). Energy transition in Germany and regional spill-overs: The diffusion of renewable energy in firms. Energy Policy 121, 404–414. doi:10.1016/j.enpol.2018.06.042
Hossain, Y., Loring, P. A., and Marsik, T. (2016). Defining energy security in the rural north—historical and contemporary perspectives from Alaska. Energy Res. Soc. Sci. 16, 89–97. doi:10.1016/j.erss.2016.03.014
Hung, N. T. (2022). Biomass energy consumption and economic growth: Insights from BRICS and developed countries. Environ. Sci. Pollut. Res. 29 (20), 30055–30072. doi:10.1007/s11356-021-17721-x
Hung, N. T. (2022). Effect of economic indicators, biomass energy on human development in China. Energy & Environ. 33 (5), 829–852. doi:10.1177/0958305X211022040
Hung, N. T. (2021). Quantile dependence between green bonds, stocks, bitcoin, commodities and clean energy. Econ. Comput. Econ. Cybern. Stud. Res. 55 (3), 71–86. doi:10.24818/18423264/55.3.21.05
Im, K. S., Pesaran, M. H., and Shin, Y. (2003). Testing for unit roots in heterogeneous panels. J. Econ. 115 (1), 53–74. doi:10.1016/S0304-4076(03)00092-7
Kao, C., and Chiang, M. H. (2001). “On the estimation and inference of a cointegrated regression in panel data,” in Nonstationary panels, panel cointegration, and dynamic panels (Emerald Group Publishing Limited).
Kao, C. (1999). Spurious regression and residual-based tests for cointegration in panel data. J. Econ. 90 (1), 1–44. doi:10.1016/s0304-4076(98)00023-2
Kelly, C., Onat, N. C., and Tatari, O. (2019). Water and carbon footprint reduction potential of renewable energy in the United States: A policy analysis using system dynamics. J. Clean. Prod. 228, 910–926. doi:10.1016/j.jclepro.2019.04.268
Kolsi, M. C., and Al-Hiyari, A. (2022). “Masdar sustainable company and the global reporting initiative standards 2016: A case study from the united Arab Emirates,” in Contemporary research in accounting and finance (Singapore: Palgrave Macmillan), 177–194.
Kuik, O. J., Lima, M. B., and Gupta, J. (2011). Energy security in a developing world. Wiley Interdiscip. Rev. Clim. Change 2 (4), 627–634. doi:10.1002/wcc.118
Levin, A., Lin, C. F., and Chu, C. S. J. (2002). Unit root tests in panel data: Asymptotic and finite-sample properties. J. Econ. 108 (1), 1–24. doi:10.1016/S0304-4076(01)00098-7
Lin, B., and Zhu, J. (2017). Energy and carbon intensity in China during the urbanization and industrialization process: A panel var approach. J. Clean. Prod. 168, 780–790. doi:10.1016/j.jclepro.2017.09.013
Lin, B., and Zhu, J. (2019). The role of renewable energy technological innovation on climate change: Empirical evidence from China. Sci. Total Environ. 659, 1505–1512. doi:10.1016/j.scitotenv.2018.12.4
Ma, X., Ahmad, N., and Oei, P.-Y. (2021). Environmental Kuznets curve in France and Germany: Role of renewable and nonrenewable energy. Renew. Energy 172, 88–99. doi:10.1016/j.renene.2021.03.014
Ma, X., Wang, C., Dong, B., Gu, G., Chen, R., Li, Y., et al. (2019). Carbon emissions from energy consumption in China: Its measurement and driving factors. Sci. total Environ. 648, 1411–1420. doi:10.1016/j.scitotenv.2018.08.183
Magazzino, C., Mele, M., Schneider, N., and Shahbaz, M. (2021). Can biomass energy curtail environmental pollution? A quantum model approach to Germany. J. Environ. Manag. 287, 112293. doi:10.1016/j.jenvman.2021.112293
Mahian, O., Bellos, E., Markides, C. N., Taylor, R. A., Alagumalai, A., Yang, L., et al. (2021). Recent advances in using nanofluids in renewable energy systems and the environmental implications of their uptake. Nano Energy 86, 106069. doi:10.1016/j.nanoen.2021.106069
Meng, B., Wang, J., Andrew, R., Xiao, H., Xue, J., and Peters, G. P. (2017). Spatial spillover effects in determining China's regional CO2 emissions growth: 2007–2010. Energy Econ. 63, 161–173. doi:10.1016/j.eneco.2017.02.001
Mullen, J. D., and Dong, L. (2022). Effects of state and federal policy on renewable electricity generation capacity in the United States. Energy Econ. 105, 105764. doi:10.1016/j.eneco.2021.105764
Paramati, S. R., Shahbaz, M., and Alam, M. S. (2017). Does tourism degrade environmental quality? A comparative study of eastern and western European union. Transp. Res. Part D Transp. Environ. 50, 1–13. doi:10.1016/j.trd.2016.10.034
Pedroni, P. (2004). Panel cointegration: Asymptotic and finite sample properties of pooled time series tests with an application to the PPP hypothesis. Econ. theory 20 (3), 597–625. doi:10.1017/s0266466604203073
Pesaran, M. H. (2007). A simple panel unit root test in the presence of cross-section dependence. J. Appl. Econ. 22 (2), 265–312. doi:10.1002/jae.951
Ullah, A., Zhang, Q., Raza, S. A., and Ali, S. (2021). Renewable energy: Is it a global challenge or opportunity? Focusing on different income level countries through panel smooth transition regression model. Renew. Energy 177, 689–699. doi:10.1016/j.renene.2021.05.132
Xie, Q., Xu, X., and Liu, X. (2019). Is there an EKC between economic growth and smog pollution in China? New evidence from semi-parametric spatial autoregressive models. J. Clean. Prod. 220, 873–883. doi:10.1016/j.jclepro.2019.02.166
Zhao, D., Chen, H., Li, X., and Ma, X. (2018). Air pollution and its influential factors in China’s hot spots. J. Clean. Prod. 185, 619–627. doi:10.1016/j.jclepro.2018.02.181
Keywords: renewable energies, pollution indicators, China, Germany, USA, research and development (R & D)
Citation: Gharbi I, Kammoun A and Kefi MK (2023) To what extent does renewable energy deployment reduce pollution indicators? the moderating role of research and development expenditure: Evidence from the top three ranked countries. Front. Environ. Sci. 11:1096885. doi: 10.3389/fenvs.2023.1096885
Received: 12 November 2022; Accepted: 23 January 2023;
Published: 13 February 2023.
Edited by:
Mohamed Chakib Kolsi, Emirates College of Technology, United Arab EmiratesReviewed by:
Hafeez Ullah, Shanghai Jiao Tong University, ChinaNgo Thai Hung, University of Finance and Marketing, Vietnam
Copyright © 2023 Gharbi, Kammoun and Kefi. This is an open-access article distributed under the terms of the Creative Commons Attribution License (CC BY). The use, distribution or reproduction in other forums is permitted, provided the original author(s) and the copyright owner(s) are credited and that the original publication in this journal is cited, in accordance with accepted academic practice. No use, distribution or reproduction is permitted which does not comply with these terms.
*Correspondence: Inés Gharbi, Z2hhcmJpaW5lczAwOUBnbWFpbC5jb20=
†These authors have contributed equally to this work