The foundations of big data sharing: A CGIAR international research organization perspective
- 1International Centre for Tropical Agriculture, Centro Internacional de Agricultura Tropical (CIAT), Cali, Colombia
- 2African Institute for Mathematical Sciences, Cape Town, South Africa
- 3Mathematical Biosciences Hub, Department of Mathematical Sciences, Stellenbosch University, Matieland, South Africa
- 4International Centre for Tropical Agriculture, CIAT, Hanoi, Vietnam
- 5Bioversity International, Montpellier, France
The potential of big data capabilities to transform and understand global agricultural and biological systems often relies on data from different sources that must be considered together or aggregated to provide insights. The value of data is however not only in its collection and storage, but largely in its re-use. Big data storage repositories are not enough when we consider a world brimming with escalating volumes of data, here we need to consider innovative systems and tools which address data harmonization and standardization and importantly, ones that can bridge the gap between science and end users. In this paper, we will demonstrate how CGIAR (including the Alliance of Bioversity International and CIAT) develops a culture of co-operation and collaboration among custodians of agrobiodiversity data, as well as new directions for big data. CGIAR first launched the Platform for Big Data in Agriculture to enhance the development and maintenance of its data. This helped establish workflows of cross-platform synthesis, annotate and apply the lessons learnt. The Platform then built GARDIAN (Global Agricultural Research Data Innovation and Acceleration Network)—a digital tool that harvests from ∼40 separate open data and publication repositories that 15 CGIAR centres have used for data synthesis. While there have been significant advances in big data management and storage, we also identify the gaps to improve use, and the re-use of data in order to reveal its added value in decision making.
1 Introduction
The concept of “big data” has been gradually gaining popularity since its first emergence in the mid-1990s, and has seen a surge in research publications since 2008 (Li et al., 2016). Big data has been suggested as a predominant source of innovation and has caused a paradigm shift to data-driven research. The rapid growth of big data originating from expanding social systems in addition to traditional measurement and observation systems offers great potential to revolutionize our approaches to research. We are getting better at discovering knowledge from data and acquiring intelligence from information. Organisations involved in big data research are better harnessing the associated opportunities and more effectively addressing the corresponding challenges (Wang, 2016).
While big data is still not a clearly defined term, it often refers to a wide range of larger datasets that are difficult to store, manage, and process using traditional processing tools, due to their size, but also their complexity (Liu, 2015). The development of open-source frameworks has been essential for the growth of big data because these frameworks make data easier to access and work with. Platforms such as Google Earth Engine (GEE) have been in the remote sensing big data spotlight. GEE is a cloud-based platform that enables parallelized processing of geospatial data on a global scale, using Google’s cloud (Tamiminia et al., 2020). Remote-sensing systems have been collecting large volumes of data for decades, but managing and analysing these data are not practical using common desktop computing resources (Amani et al., 2020). This platform addressed a major problem for scientists, which was how to best access increasing amounts of satellite data while enabling an easy place for researchers to start searching, processing and analysing relevant data (Shelestov et al., 2017).
Big data and big data platforms to support research are necessary for agile and hyper-local responses to current challenges (Himesh et al., 2018; Rao, 2018). Considering the rising risks from changing climate and the increasing focus on food security and biodiversity, we need to target productive and sustainable agriculture (Mbow et al., 2019). The Global Biodiversity Facility (GBIF) makes biodiversity data accessible and open access (Robertson et al., 2022), and platforms such as GEE as well as Copernicus, USGS Earth Explorer, NASA open data portal, Natural Earth and OpenStreetMap provide access to extensive volumes of geospatial data. Tools have also been initiated such as the Earth System Data Lab (ESDL) which aim to address issues such as data standardization and harmonization. ESDL combine data from the atmosphere, terrestrial biosphere, hydrosphere, pedosphere and oceans into an easy to use analysis-ready format. ESDL produces products that are able to overcome various obstacles such as formatting inconsistencies, incompatible spatiotemporal resolutions and access restrictions. Research institutes and academics alike have access to these large stores of data and so too are they continuing to add to their own data sources through primary research, however the overlap of biodiversity data platforms with agroecology and agrobiodiversity to target issues such as food security is still limited (Arnaud et al., 2016).
Finding value in big data is an entire process, and while managing big data has evolved significantly, its value is only now being realised. How do we continue to handle and add value to the escalating volumes of data? CGIAR (including the Alliance of Bioversity International and CIAT) is a consortium of Research Centres that works with partners in six major global regions to jointly address challenges to food, land, and water systems (FLWs) (see http://www.cgiar.org for more details). To assess the status of these FLWs and associated trends requires a vast amount of relevant information across varying spatial and temporal scales. The increasing volume and varying format of big data presents challenges for successful storage, management, analysis and sharing of high quality data for both science and end users. Consequently, large research institutions like CGIAR need to establish multidisciplinary collaboration and operational flows of big data.
2 CGIAR and big data
2.1 CGIAR’s data assets
CGIAR is a consortium that unites an array of international organizations aiming to reduce rural poverty, improve human health and nutrition, introduce sustainable management of natural resources and strengthen food security, primarily in developing countries. In close collaboration with national research institutions, almost 10,000 CGIAR scientists, researchers and technicians are collecting, analysing and synthesizing data on agricultural and biological systems across Asia, Africa, Latin America and the Pacific. With its 15 international research centres, 11 genebanks (that safeguard a unique global resource of crop and tree diversity and respond to thousands of requests for samples per year in more than 100 countries worldwide), 12 Global Research Programs as well as various research platforms, the CGIAR has collected and generated data through a variety of avenues and these data include but are not limited to: Long-term trials, baseline data collections, genomic data, value-added secondary datasets, spatial data and data collected in public-private partnerships. A key objective of CGIAR is to integrate the work of the centres and their partners, avoiding fragmentation and duplication of effort, specifically around these data resources.
In addition to data and databases, there are also other types of information products such as reports, books and book chapters, data analysis and collection tools, video, audio and images and the metadata associated with the information products listed above. In the CGIAR, these information products are stored and preserved in a joint repository called CGSpace (https://cgspace.cgiar.org). CGSpace hosts research outputs and knowledge products for several of CGIAR centres and research programs (Figure 1). For storing and publishing data and databases, other centres also created a range of data portals and also disseminate data through repositories such as the Harvard Dataverse. Even though these portals and repositories have stimulated open science and made research more transparent, the increase in number and size of these tools has signalled a coordination issue between individual CGIAR centres.
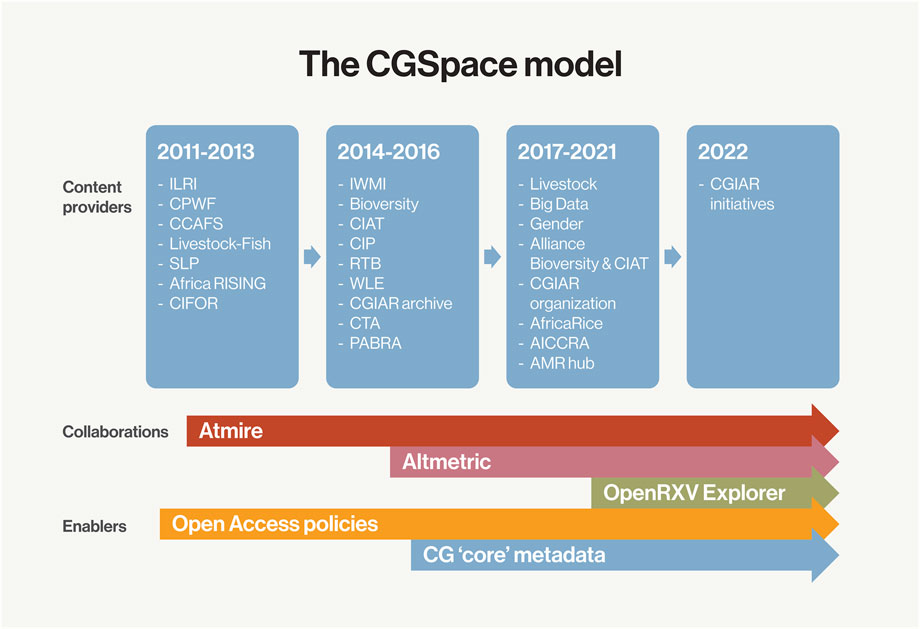
FIGURE1. CGSpace model (Ballantyne et al., 2022).
2.2 CGIAR’s data as international public goods
A fundamental step towards open science is to offer open access data. The CGIAR therefore developed a clear mandate to recognize data as important information assets and project deliverables. In 2012, the CGIAR approved its Intellectual Assets Principles (CGIAR IA Principles) (CGIAR—IEA, 2018). A year later, in 2013, the CGIAR approved its Open Access and Data Management Policy (OA/DM Policy), which expanded on CGIAR IA Principles. OA/DM Policy officially recognized CGIAR’s information products as international public goods. This stated that all research data, generated as a result of research funded by CGIAR programmes, must, subject to confidentiality of respondents, be deposited in a suitable repository and made publicly available following a pre-determined timeline. OA/DM Policy also acted as a guiding framework for data producers and publishers to implement FAIR principles (Findability, Accessibility, Interoperability, and Reusability) (Wilkinson et al., 2016). The FAIR data principles have rapidly become a standard for assessing responsible and reproducible research (Bezuidenhout and Shanahan., 2022).
CGIAR, being a global network of agricultural research centres recognized the importance of standardisation and data interchange in building a global network of data and service providers. In 2017, the CGIAR launched the Platform for Big Data in Agriculture as a coordinating mechanism to deliver CGIAR’s strategy and to align CGIAR’s Research Programs and data management processes (CGIAR Advisory Services Shared Secretariat, 2021). Prior to the Platform for Big data in Agriculture, CGIAR had some 40 separate open data and publication repositories. For the first time, these intellectual assets became discoverable in one place via GARDIAN (https://gardian.bigdata.cgiar.org). GARDIAN (Global Agricultural Research Data Innovation and Acceleration Network) is the first pan-CGIAR search engine for agricultural data. This platform harvests from separate open data and publication repositories that 15 CGIAR centres have used for data synthesis. GARDIAN also incorporated metadata standards and quality control measures to ensure the accuracy and reliability of the data. Metadata sharing is a critical way to ensure that data is discoverable (Contaxis et al., 2022).
There are however also many other actors in the big data realm which makes this space highly fragmented. Private companies, government institutions, and university research institutes have also been generating immense amounts of data. Access to these data might be restricted due to protection of the privacy of human subjects, compliance with policies and regulations, and following intellectual property rights. The CGIAR has partly addressed this by updating and refining an open Guideline for Responsible Data (https://bigdata.cgiar.org/responsible-data-guidelines). However, to further tackle the issue, there is a strong need to cultivate strategic partnerships and to build appropriate policies and business models (King et al., 2021). Furthermore, CGIAR needs to enable interoperability and shared infrastructure that allows data to flow seamlessly beyond CGIAR systems, and allows partnerships with other organisations to promote the overlap of biodiversity data platforms with agroecology and agrobiodiversity.
2.3 The gap—Data paralysis
Enabling data discoverability is not quite enough. In addition to ensuring that data is discoverable, it is essential to evaluate the impact and value of data on end users as well as the ability of available data to influence scientific research and development. An evaluation by the CGIAR Advisory Services Shared Secretariat (2021), an independent panel of experts tasked with evaluating CGIAR’s programs, pointed out the lack of analysis on the Platform as well as the scarcity of data downloads. The focus had been on data uploads and not a lot on data re-use and the scientific application of the data. While data uploads are a necessary first step in the platform the lack of subsequent data re-use was considered a missed opportunity to help bridge the gap between science and potential data end-users.
A wealth of information may mean a lack of attention on the part of its recipients, since attention should be allocated wisely, given the overabundance of information stimuli (Kambatla et al., 2014; Madison et al., 2022). The rise of data brings with it two problems. Firstly, data users are overwhelmed with information as well as sources of information and have a shortage of attention for a full screening of all available data. Secondly, with the exponential growth of the data being produced daily, the volumes of data available for exploitation in this Big Data era do not just offer answers to a specific set of questions, they also proffer yet-to-be-asked questions, which means data exploration and not just discovery is also important.
At the later development phase of the Platform, GARDIAN introduced a mapping and analytics tool where users can better visualise, explore, and understand the data (https://gardian.bigdata.cgiar.org/#/tools; https://gardian.bigdata.cgiar.org/#/maps). A lack of analysis on the platform may however indicate how these exploration tools should have been co-developed with current and potential users, especially researchers and policymakers, to allow generating clear added value of the datasets. Perhaps however this is also an indication of the overabundance of data availability leading users to data paralysis. Indeed, there are numerous other high-level interfaces for data retrieval such as DataOne and Globus, and if CGIAR aims to be part of these global data providers it is crucial that CGIAR ensures effective data interchange in this community of repositories and big data services. Infrastructure, tools, and approaches to make CGIAR data more visible, interoperable and reusable continue to be further refined.
Lastly, in the current era of research in order to support better diagnosis of problems or monitoring of interventions such as those related to agroecology, conservation ecology and climate change, biodiversity informatics must embrace real time, near real time or high frequency data streams. Real time high frequency data streams are data sets that are collected in near-instance intervals. These datasets are typically collected though sensors or other automated processes and are used in many projects in CGIAR to gain insights into the complex systems that shape our world. As the Platform for Big Data has now come to the end of its cycle, CGIAR must consider new ways to reuse data to generate and answer common research questions and integrate research across domains, which is fundamental for making progress on solutions. We also need to consider ways to combine real time data within the classical collection of data stores we have available.
2.4 Enter the era of the dashboard (dashboard 2.0)
The concept of interoperability was popularized by peer-to-peer systems such as Napster, BitTorrent and Gnutella, who emphasized the “data as a resource” concept with aggregation, resource sharing and cost reduction (Kambatla et al., 2014). However, while the integration across research domains increases the use and accessibility of data, it does not necessarily reveal its value (Hai et al., 2016). Metrics such as number of open access items may measure openness and accessibility of data and information products, but they do not reflect the impact of data on research and development. Big data needs decision support. From this perspective, there are several tools and techniques that have been applied to big data for decision making (Casado and Younas, 2015). Enter the era of the dashboard. Dashboards or platforms utilize one or several components of optimization methods, statistics, data mining, machine learning and numerous visualization approaches. In terms of data interchange, dashboards can be designed to allow users to easily export and share data in a variety of formats. Although these may be far from perfect, they allow data discovery and integrated data exploration as a coherent toolkit (Casado and Younas, 2015). More importantly, they enable the data to be used for its fundamental purpose—enabling decision making. It has been shown that reduced cognitive load is vital for information processing, and ease-of-use is a crucial factor influencing human interaction with technology (Lah et al., 2020; Castro-Alonso et al., 2021).
The utilization of dashboards also presents a significant benefit in that they can be easily designed to conform to data standards and APIs, which ensures that data is interoperable and can be shared across different systems or platforms. This is particularly important when working with global initiatives and programs. Dashboards will also allow users from academia to the private sector and government bodies to understand what notable information from different sources is available, democratizing data discovery and exploration. Furthermore, these systems derive information from the data, leading to knowledge and then achieve wisdom from knowledge, leading to intelligent decision making (Hai et al., 2016). Lastly, while GARDIAN was designed to handle historic and processed data, with the new stream of “real time”, data dashboards seem better equipped to address such needs. Real time FLWs data such as sensor data, satellite data, remote sensing data and weather/climate data can be easily integrated into dashboards by connecting the data stream to a cloud based storage platform for users to more easily access real time data produced by CGIAR and integrate data in agroecology and agrobiodiversity to target issues such as food security and other global challenges.
3 Discussion
The current volume of data and information collected, stored, shared and used is almost unlimited. This data possesses a high value, and calls for smart logistic and cognitive management of the complex data that is available in order to exploit the data while serving a multiplicity of interests from stakeholders and policymakers to diverse research groups. CGIAR is a major producer of agricultural data and information and has paved the way for open access and FAIR data through its principles, policies, frameworks and platforms. While CGIAR has learnt many lessons from these processes, it must apply these lessons in its future workings.
As data becomes more and more pervasive, users can become intimidated or overwhelmed by its sheer volume, even before any analysis is performed. If we apply the principle of “less is more”, the CGIAR is arguably its own worst enemy with regard to effective data sharing and consumption. Given the abundant data flow, the next challenge is not how to acquire more data, but how to re-use and translate it into something meaningful. User-friendly dashboards or tools that allow data discovery to flow into data exploration, analytics and data interchange offer the means for this. CGIAR has already implemented several dashboards to help facilitate research and collection (https://www.cgiar.org/dashboards/) and these need to continually be refined. While dashboards are an attractive way to bridge this gap we must also not overlook the human aspect of big data. Upstream processing for data curation and data governance is required and so sharing data learnings and pipelines such as those used and developed by CGIAR and the Platform is essential. Using this knowledge we can cultivate an open access ecosystem, and improve our knowledge on how to store, use, and re-use data more effectively.
Author contributions
AB took the lead in writing the manuscript with consultation of KN. Author AC helped shape the direction of the paper and AC and EA provided critical feedback and review.
Acknowledgments
The authors acknowledge the editorial input of the Bioversity-CIAT Alliance Science Writing Service, provided by Vincent Johnson. The authors also thank Leroy Mwanzia for his knowledge input.
Conflict of interest
The authors declare that the research was conducted in the absence of any commercial or financial relationships that could be construed as a potential conflict of interest.
Publisher’s note
All claims expressed in this article are solely those of the authors and do not necessarily represent those of their affiliated organizations, or those of the publisher, the editors and the reviewers. Any product that may be evaluated in this article, or claim that may be made by its manufacturer, is not guaranteed or endorsed by the publisher.
References
Amani, M., Ghorbanian, A., Ahmadi, S. A., Kakooei, M., Moghimi, A., Mirmazloumi, S. M., et al. (2020). Google Earth engine cloud computing platform for remote sensing big data applications: A comprehensive review. IEEE J. Sel. Top. Appl. Earth Observations Remote Sens. 13, 5326–5350. doi:10.1109/jstars.2020.3021052
Arnaud, E., Castañeda Álvarez, N. P., Ganglo Cossi, J., Endresen, D., Jahanshiri, E., et al. (2016). Final report of the Task Group on GBIF data fitness for use in agrobiodiversity.
Ballantyne, P. G., Yabowork, A., Victor, M., and Orth, A. (2022). CGSpace - an open access knowledge and information repository for CGIAR research. Figure. Nairobi: Kenya.
Bezuidenhout, L., and Shanahan, H. (2022). Rethinking the a in FAIR data: Issues of data access and accessibility in research. Front. Res. Metrics Anal. 42, 912456. doi:10.3389/frma.2022.912456
Casado, R., and Younas, M. (2015). Emerging trends and technologies in big data processing. Concurrency Comput. Pract. Exp. 27 (8), 2078–2091. doi:10.1002/cpe.3398
Castro-Alonso, J. C., de Koning, B. B., Fiorella, L., and Paas, F. (2021). Five strategies for optimizing instructional materials: Instructor-and learner-managed cognitive load. Educ. Psychol. Rev. 33 (4), 1379–1407. doi:10.1007/s10648-021-09606-9
CGIAR - IEA (2018). Review of CGIAR’s open access/open data policy and implementation support. Independent evaluation arrangement (IEA) of CGIAR. Rome: Italy. Available at: http://iea.cgiar.org/.
CGIAR Advisory Services Shared Secretariat. (2021). Evaluation of CGIAR platform for big data in agriculture. Report. Rome: CAS Secretariat Evaluation Function. Available at: https://cas.cgiar.org/.
Contaxis, N., Clark, J., Dellureficio, A., Gonzales, S., Mannheimer, S., Oxley, P. R., et al. (2022). Ten simple rules for improving research data discovery. PLoS Comput. Biol. 18 (2), e1009768. doi:10.1371/journal.pcbi.1009768
Himesh, S., Rao, E. P., Gouda, K. C., Ramesh, K. V., Rakesh, V., Mohapatra, G. N., et al. (2018). Digital revolution and big data: A new revolution in agriculture. CABI Rev. 2018, 1–7. doi:10.1079/pavsnnr201813021Ajilesh
Kambatla, K., Kollias, G., Kumar, V., and Grama, A. (2014). Trends in big data analytics. J. parallel distributed Comput. 74 (7), 2561–2573. doi:10.1016/j.jpdc.2014.01.003
King, B., Devare, M., Overduin, M., Wong, K., Kropff, W., Perez, S., et al. (2021). Toward a Digital One CGIAR. Strategic research on digital transformation in food, land, and water systems in a climate crisis. Int. Cent. Trop. Agric. (CIAT), 112. Available at: https://cgspace.cgiar.org/handle/10568/113555
Lah, U., Lewis, J. R., and Šumak, B. (2020). Perceived usability and the modified technology acceptance model. Int. J. Human–Computer Interact. 36 (13), 1216–1230. doi:10.1080/10447318.2020.1727262
Li, S., Dragicevic, S., Castro, F. A., Sester, M., Winter, S., Coltekin, A., et al. (2016). Geospatial big data handling theory and methods: A review and research challenges. ISPRS J. Photogrammetry Remote Sens., 115, 119–133. doi:10.1016/j.isprsjprs.2015.10.012
Liu, P. (2015). A survey of remote-sensing big data. Front. Environ. Sci. 3, 45. doi:10.3389/fenvs.2015.00045
Madison, M. J., Frischmann, B. M., Sanfilippo, M. R., and Strandburg, K. (2022). Too much of a good thing? A governing knowledge commons review of abundance in context. Front. Res. Metrics Anal. 45, 959505. doi:10.3389/frma.2022.959505
Mbow, C., Rosenzweig, C., Barioni, L. G., Benton, T. G., Herrero, M., Krishnapillai, M., et al. (2019). Food security.
Rao, N. H. (2018). Big data and climate smart agriculture-status and implications for agricultural research and innovation in India. In Proc. Indian Natl. Sci. Acad. 84, 625–640.
Robertson, T., Wieczorek, J., and Raymond, M. (2022). Diversifying the GBIF data model. Biodivers. Inf. Sci. Stand. 6, e94420. doi:10.3897/biss.6.94420
Shelestov, A., Lavreniuk, M., Kussul, N., Novikov, A., and Skakun, S. (2017). Exploring Google Earth Engine platform for big data processing: Classification of multi-temporal satellite imagery for crop mapping. Front. Earth Sci. 5, 17. doi:10.3389/feart.2017.00017
Tamiminia, H., Salehi, B., Mahdianpari, M., Quackenbush, L., Adeli, S., and Brisco, B. (2020). Google earth engine for geo-big data applications: A meta-analysis and systematic review. ISPRS J. Photogrammetry Remote Sens. 164, 152–170. doi:10.1016/j.isprsjprs.2020.04.001
Wilkinson, M. D., Dumontier, M., Aalbersberg, I. J., Appleton, G., Axton, M., Baak, A., et al. (2016). The FAIR Guiding Principles for scientific data management and stewardship. Sci. data 3 (1), 160018–160019. doi:10.1038/sdata.2016.18
Keywords: big data, data management, data storage, agrobiodiversity, ecology, dashboards, open source
Citation: Basel AM, Nguyen KT, Arnaud E and Craparo ACW (2023) The foundations of big data sharing: A CGIAR international research organization perspective. Front. Environ. Sci. 11:1107393. doi: 10.3389/fenvs.2023.1107393
Received: 24 November 2022; Accepted: 22 February 2023;
Published: 07 March 2023.
Edited by:
Vernon Visser, University of Cape Town, South AfricaReviewed by:
Polina Lemenkova, Université libre de Bruxelles, BelgiumAndrii Shelestov, Kyiv Polytechnic Institute, Ukraine
Ebrahim Jahanshiri, Crops For the Future UK, United Kingdom
Copyright © 2023 Basel, Nguyen, Arnaud and Craparo. This is an open-access article distributed under the terms of the Creative Commons Attribution License (CC BY). The use, distribution or reproduction in other forums is permitted, provided the original author(s) and the copyright owner(s) are credited and that the original publication in this journal is cited, in accordance with accepted academic practice. No use, distribution or reproduction is permitted which does not comply with these terms.
*Correspondence: Ashleigh M. Basel, A.Basel@cgiar.org