- School of Economics and Management, Wuhan University, Wuhan, China
With prominent environmental pollution and depleted resources, how to coordinate economic growth and eco-environmental protection to guide green development represented by green total factor productivity (GTFP) is an urgent issue. This study aims to empirically evaluate the direct effect, indirect effect, spatial spillover effect and non-linear effect of the digital economy on green development using the data of 284 prefecture-level cities in China. The empirical results indicate that: (1) the digital economy significantly improves GTFP, which is still valid after testing for robustness, including introducing instrumental variables, taking the “broadband China” pilot policy as a quasi-natural experiment, changing core explanatory variables and dependent variables, and changing the sample size; (2) the influence of the digital economy on GTFP is characterized by significant heterogeneity among resource dependence, geographical location, financial development level and openness level; (3) the mechanism analysis shows that the digital economy promotes GTFP by green technological innovation, industrial structure upgrading and energy conservation; (4) the spatial econometric models indicate that the digital economy significantly enhances GTFP of neighboring cities; (5) there is a non-linear relationship between the digital economy and GTFP using the threshold model. The findings could provide references for policymakers to promote urban green development.
1 Introduction
Under the background of the increasingly prominent environmental pollution and resource shortages, how to coordinate economic growth and eco-environmental protection is an urgent issue (Wang and Feng, 2021). Cities are the area where the deterioration of ecological environment is relatively serious, which is mainly due to the concentration of human energy consumption and carbon dioxide emissions. According to the statistics of UN Habitat, cities consume 78% of the world’s primary energy and account for more than 50% of the global total greenhouse gas emissions. Especially, as the largest emerging country and carbon emitter in the world, China bears the pressure of energy consumption and ecological destruction during its rapid urbanization. China’s urban energy consumption accounts for 85% of China’s carbon emissions, far exceeding the world average of 67%. To combat global warming caused by carbon emissions, the Chinese government proposed to achieve a carbon peak by 2030 and carbon neutrality by 2060 at the 75th United Nations General Assembly in 2020. The 20th National Congress of the Communist Party of China once again proposed to promote green development and harmonious coexistence between human beings and nature, which emphasize the coordinated development of socio-economy and eco-environment. Cities are the main battleground for carbon emissions reduction and national economic growth to achieve the “dual carbon” target. At this stage, the key to achieving this target lies in guiding green development in cities by promoting the green transformation of traditional industries and the development of low-carbon intelligent industries (Yang et al., 2019).
The digital economy may be a feasible option for China to achieve green development by promoting green transformation of traditional industries and facilitate forming sustainable green productivity (Tao et al., 2023). The digital economy refers to a serious of economic activities that take data as the key production factor, information network as the main carrier, and digital technology application as the driving force to improve the level of digitalization, networking and intelligence of the economy and society (G20, 2016; Zhang et al., 2022). China’s digital economy ranked second in the world in 2021, with a share of 39.8% of China’s GDP1. With the characteristics of permeability and sharing, the digital economy is conducive to the transformation of production and governance modes, which obviously provides the opportunities for the green development. On the one hand, the digital economy deeply integrates digital technology such as artificial intelligence, big data, the Internet of Things, cloud computing and blockchain with the real economy, favorable to achieve the green transformation of traditional industries (Li and Wang, 2022; Yi et al., 2022). On the other hand, the digital economy can extend the scale of environmentally friendly and intelligent digital industry. What cannot be ignored is that the digital economy may exacerbate pollution emissions through scale expansion owing to the energy rebound effect. Therefore, whether the digital economy can be a desirable way to achieve green development remains to be further explored.
Many scholars have studied the economic effect of the digital economy at macro, meso and micro levels and confirmed that the digital economy significantly improves production efficiency hence accelerating economic growth. At the macro level, Tranos et al. (2021) proved that the digital economy exerts a positive and lasting impact on subsequent regional productivity. Kim et al. (2021) empirically analyzed that information and communication technology (ICT), the basis of the digital economy, can both directly contribute to output growth and generates economic spillover effects to other industries using the country-level data from 15 European countries. Yabo and Jie (2022) demonstrated that the digital economy significantly improves the quality of exports. At the meso-regional level, Pan et al. (2022) proposed that the digital economy is an innovation driver for the development of provincial total factor productivity. At the micro level, the studies found that the digital economy is beneficial to firm productivity and performance (He and Liu, 2019; Li and Wang, 2021). The core of digital economy, digital technologies contributes to competition advantages of enterprises (Teece, 2018). It seems that there is a consensus that the digital economy has positive economic effects.
There is no agreed conclusion about the environmental effect of the digital economy with similar duality of ICT in both developed and developing countries. Extensive research has confirmed that the digital economy can mitigate climate change and exerts a positive impact on sustainable environment (Balogun et al., 2020). Schulte et al. (2016) showed that ICT significantly decreases energy demand using the panel data from OECD countries. Emerging countries have greatly reduced carbon emissions by increasing Internet access (Ozcan and Apergis, 2018). Danish (2019) proposed that ICT reduces the carbon dioxide emissions of countries along the Belt and Road. Wang et al. (2021) based on the data of OECD countries found that digital technology reduces the domestic carbon emission intensity since the carbon emissions reduction through cross-industry technology spillover exceeds the emission increased by technology innovation in the information industry. Yi et al. (2022) and Zhang et al. (2022) respectively demonstrated that the digital economy is conducive to the reduction of carbon emissions at the provincial and urban levels in China. On the contrary, some scholars believe that the digital economy exerts a negative impact on the environment due to the energy rebound effect (Lange et al., 2020). The application of digital technologies requires energy and resources inputs, such as the manufacture, processing, operation and distribution of electronic equipment, which greatly increases energy consumption and damages to the environment for European Union (EU) countries (Park et al., 2018). Specifically, the use of large global data centers and mobile data traffic may generate manufacturing-related electronic waste (Lennerfors et al., 2015). The studies on OECD countries and emerging economies found that the application of ICT significantly increases the electricity consumption in both the short and long term (Sadorsky, 2012; Salahuddin and Alam, 2016). Ren et al., 2021 proved that Internet development significantly improves the energy consumption scale by boosting economic growth using the provincial panel data of China. Xue et al. (2022) pointed out that digital economy development increases the energy consumption based on the urban panel data of China. Furthermore, Higón et al. (2017) claimed that there is an inverted U-shaped relationship between ICT and carbon emissions using the data from 142 countries, and many developed countries have already passed the turning point and gained the environmental dividend brought by ICT, while the developing countries have not yet reached the turning point. Li and Wang (2022) proved the inverted U-shaped relationship between digital economy and carbon emissions based on the city-level data of China. Stefanie and David (2021) discussed that whether digitalization can become part of the “solution” for environmental sustainability depends on the comprehensive effect of various mechanisms, like political and economic system. These controversial findings on the environmental effects of the digital economy provides room for further study on the impact of the digital economy on green development.
Research on the digital economy and green development is in its infancy, requiring more abundant data and empirical proofs (Hao et al., 2022; Hu and Guo, 2022; Ma and Zhu, 2022). Green total factor productivity (GTFP), which comprehensively seeks the coordination of economic growth and eco-environmental protection, is widely employed to measure green development (Qiu et al., 2021; Wang et al., 2021; Hao et al., 2022; Ma and Zhu, 2022). This indicator incorporates environmental factors into the economic efficiency analysis framework and covers both expected output and undesired output like industrial wastewater emissions, which can effectively reflect the sustainable growth of economy (Emrouznejad and Yang, 2018; Liu and Xin, 2019; Zhang and Vigne, 2021; Li et al., 2022). Hao et al. (2022) demonstrated that the digital economy improves the manufacturing GTFP of China. Hu and Guo (2022) confirmed that the digital economy positively impacts the GTFP of the Yangtze River Economic Belt in China. Ma and Zhu (2022) confirmed that the digital economy drives the high-quality green development of emerging economies by enhancing industrial restructuring and green technological innovation. However, the theoretical explanations and mechanisms of the digital economy’s impact on green development are inadequately studied. The spatial spillover effects of the digital economy and the regional heterogeneity of the impact of the digital economy on green development in China need further validation analysis. Whether the digital economy has played a critical role in promoting green development urgently need further empirical verification, which has vital theoretical and policy implication for emerging countries to achieve green development.
The aims of this paper are as follows. 1) Figure out whether the digital economy can promote green development represented by green total factor productivity and decompose the GTFP into green technology progress index (GTP) and green technology efficiency index (GTE) to evaluate its specific impact path. 2) Analyze the intrinsic mechanism of digital economy to impact the green total factor productivity. 3) Explore the spatial spillover effect of the digital economy on green total factor productivity using the spatial econometric models. 4) Ascertain whether there is a non-linear relationship between the digital economy and green total factor productivity by adopting the threshold model. 5) Further investigate the heterogenous effect of digital economy on green total factor productivity in terms of cities characteristics. This study uses the data of 284 prefecture-level cities in China from 2011 to 2019 to examine the effect of the digital economy on green development and its transmission mechanism, which is momentous to verify whether digital economy can become an efficient channel for emerging countries to achieve green development. The super-efficient SBM-DDF with pollution emission as undesirable output is employed to estimate the urban GTFP and the composite indicator of digital economy is constructed using principal component analysis at the city level (Huang et al., 2019; Zhao et al., 2020).
The contributions of this study can be reflected in the following aspects. First, it enriches the research on the impact and transmission mechanisms of the digital economy on green development taking the coordinated development of economy and environment into account at the urban level in emerging countries. The current literature mainly concentrates on the economic and environmental effect of the digital economy separately and draws controversial conclusions about environmental effects. Different from the most literature that utilizes the development level of ICT to represent the digital economy, which is not completely scientific and may lead to biased empirical results, a comprehensive indicator is constructed to measure the digital economy. This paper proves the impact and intrinsic mechanisms of the digital economy on GTFP from the aspects of green technological innovation, industrial structure upgrading and energy conservation at the urban level. Second, this paper investigates the heterogeneous effects of the digital economy on GTFP in terms of resource dependence, geographical location, financial development level and openness for cities. Finally, the appropriate spatial econometric method is adopted to analyze the spatial spillover effect of the digital economy on GTFP. The research conclusion has considerable application value for promoting urban green development.
2 Theoretical mechanism and research hypothesis
2.1 The direct impact of the digital economy on GTFP
With digital technologies as the driving force, the digital economy provides opportunities for achieving green development that emphasizes the coordination of economic growth and environmental protection. The digital economy breaks the bottleneck of factor supply for the development of emerging industries and innovates the business models, which in turn guarantee green production, green lifestyle and environmental governance (Xu et al., 2019). First, the deep integration of digital technologies with the real economy is conducive to the green transformation of production mode. The digital technology optimizes production processes and operation modes, replaces clean energy and promotes waste management processes, so as to improve energy efficiency and energy conservation. Simultaneously, the digital economy builds a digital platform for information sharing and exchange between the supply and the demand sides, thereby saving business costs (Hao et al., 2022). In terms of green lifestyle, the digital economy drives the green transformation of residents’ consumption pattern in digital application scenarios. The digital technology gives birth to digital consumption platforms such as online shopping, online meetings, remote learning, non-inductive payment and paperless office system, which reduce travel and cultivate a green lifestyle (Martin et al., 2018). The application of digital technologies in vehicles like shared-bikes can also contribute to the reduction of energy consumption and carbon emission by increasing vehicle usage and sharing rates. In terms of social governance, the digital technology optimizes the environmental governance mode and broaden the governance channels to achieve energy conservation and pollution emission reduction (Roscia et al., 2013). For one thing, the application of digital technologies in government departments could improve the efficiency of governmental affairs by enhancing the ability of pollution emission prediction, supervision, management, and emergency response. Digital government could obtain and track data from regional energy suppliers and consumers to better monitor and address corporate environmental pollution behavior (Li et al., 2022). It can also prevent corruption such as data forgery and collusion between government and enterprises through enhancing information transparence (Zhang et al., 2022). For another, digital economy encourages the public participation in supervising environmental governance by creating diversified communication platforms. The residents can perceive environmental changes and then give timely feedbacks through the digital media platform like TikTok, WeChat, Weibo and governmental websites. Moreover, the dramatic advancement of ICT has stimulated a free culture providing its consumer with utility and happiness, in which case, the consumers gradually tends to pursue social, cultural and emotional values rather than just economic value of the products (Watanabe et al., 2015). The current GDP statistics fails to capture the excess over the economic value owing to the digital contents’ characteristics of freebies, mass standardization and easy replication (Watanabe et al., 2018). Therefore, this paper proposes:
Hypothesis 1:. The digital economy promotes urban green total factor productivity.
2.2 The indirect impact mechanism of the digital economy on GTFP
2.2.1 The digital economy affects GTFP by advancing green technological innovation
With data as the key production factor and digital technologies as the driving force, the digital economy has realized the transformation from factor-driven to innovation-driven forms. The digital economy stimulates green technological innovation that innovates products and processes just as energy saving, pollution prevention and control, waste recycling and green product design (Luo et al., 2022), thus improving GTFP.
With the characteristics of fairness and real-time interactivity, the digital technology breaks the boundaries of time and space, reducing information asymmetry and transaction costs (Chung, 2018). In addition, digital technology triggers a learning and imitation effect by facilitating knowledge spillover and innovation resources exchange (Proeger and Runst, 2020). These make it possible to match human-centered information on innovation factors with individual skills in a timely manner, which greatly stimulate the green technological innovation. From the production side, green technological innovation optimizes the production process and augments the input of renewable resources (Danish and Ulucak, 2021), thus controlling pollution emissions at the source and in the production process. On the governance side, green technological innovation is conducive to updating the terminal treatment equipment or process to reduce pollution emissions at the end of production (Cai and Li, 2018). These could greatly reduce unnecessary production and operation costs (Wang et al., 2021), pollution emissions (Yi et al., 2020; Obobisa et al., 2022), and improving energy efficiency (Miao et al., 2017), thus improving urban GTFP. Therefore, the digital economy contributes to the GTFP by boosting green technological innovation.
2.2.2 The digital economy affects GTFP by promoting industrial structure upgrading
Relying on the digital technology, the digital economy eliminates time and space barriers to the flow of production factors between industries, hence accelerating emerging industries and upgrading traditional industries. For one thing, the digital economy fuels new business models and new business forms of industries by means of digital technology services and digital platform construction. It establishes a green and intelligence industrial chain for promoting the development of emerging industries with high added value and low pollution. For another thing, the digital economy guides the digital transformation of traditional industries such as agriculture, industry and tertiary industry to green and intelligent directions. With strong permeability and coordination of new production factors, the digital economy realizes the efficient matching of data elements and traditional production factors. For instance, digital technologies like artificial intelligence, big data, the Internet of Things and cloud computing enhances the technological innovation capability and production efficiency of manufacturing firms, and correspondingly increase the added value of manufacturing industries (Cardona et al., 2013; Li et al., 2014). Therefore, the digital economy drives the upgrade of industrial structure by promoting the development of emerging industries and increasing the added value of traditional industries, thus enhancing GTFP.
2.2.3 The digital economy affects GTFP by boosting energy conservation
The digital economy decreases energy consumption via changing production efficiency and energy efficiency, thus improving GTFP. Digital technologies optimize energy utilization technologies and production technologies, significantly reducing energy consumption in the production process of companies (Ramirez et al., 2019). On the one hand, the deep combination of digital technologies and green manufacturing technologies has given birth to green intelligent manufacturing platforms, including product design systems, process planning systems and manufacturing decision systems. These optimize power systems and realize intelligent production and industry chain reorganization, which improves the energy utilization efficiency of enterprises and directly reduces the energy consumption (Li and Du, 2021). On the other hand, enterprises rely on digital platforms to build their own energy management systems to achieve self-control and optimization of energy systems (Ren et al., 2021). The digital command platform can realize the interconnection and orderly coordination among numerous energy systems to facilitate the allocation of energy resources and improve the overall efficiency of energy systems (Iqbal et al., 2018; Pan and Dong, 2022).
Based on the above analysis, this paper proposes:
Hypothesis 2:. The digital economy enhances urban green total factor productivity by promoting green technological innovation, upgrading industrial structure and energy conservation.
2.3 The spatial spillover effects of the digital economy on GTFP
Relying on its networking features and digital technologies, the digital economy breaks through the restrictions of time, space and industrial boundaries, promoting information flow and interdepartmental connections between cities and regions, hence generating spatial spillover effect. For one thing, the digital technology accelerates the diffusion of information and various resource elements and guide the cross-regional labor division and cooperation. Accordingly, it enhances the economic ties between cities and achieves the coordinated progress of related areas. For another, digital platforms effectively match users and producers and improve resources utilization efficiency by reducing information asymmetry between supply and demand sides. Moreover, developed cities form regional growth poles through the “polarization effect” due to the output of advanced technologies and management methods. This process stimulates the diffusion of information technology to the surrounding developing cities and form a learning and imitation effect, thus improving the green total factor productivity in the neighboring areas. Therefore, this paper proposes:
Hypothesis 3:. The digital economy exerts spatial spillover effect on urban green total factor productivity of neighboring cities.
2.4 The non-linear effects of the digital economy on GTFP
On the basis of the network effect, the value of the network depends upon the size of its other users. This means that if the product or service provided by a firm can gain a certain number of users or suppliers faster, then it forms a network value advantage over the firm’s competitors, thus generating a positive feedback mechanism and vice versa a negative feedback mechanism (Li, 2019). According to the Metcalfe’s law, the value of the network is equal to the square of the number of network nodes and the value of the network explodes when the size of users exceeds a critical point (Pei et al., 2018). Digital economy possesses the attributes of network since its core is digital technological innovation and expeditious network (Ma and Zhu, 2022). As the broadband usage, digital infrastructure construction and Internet access continuously increase until the scale of connections reaches a critical value, the digital economy will generate incremental scale effects and positive network externalities. Therefore, digital economy development may exert a non-linear impact on urban green total factor productivity. In view of the above analysis, this paper proposes:
Hypothesis 4:. The digital economy has a non-linear impact on urban green total factor productivity.
3 Methodology
3.1 Model
The following fixed effects model are constructed to verify the above proposed theoretical hypothesis of the impact of the digital economy on urban green development:
where i and t represent cities and years, respectively.
3.2 Description of variables and data
3.2.1 Relevant indicators for GTFP measurement
This paper applies the super-efficient slack-based measure (SBM) directional distance function (DDF) and Global Malmquist - Luenberger (GML) index to measure GTFP at the urban level (Fukuyama and Weber, 2009; Yang et al., 2019). The GML index and its corresponding decomposition of the green technical efficiency index (GTE) and the green technical progress index (GTP) of cities are calculated by using the MaxDEA software. The green technical efficiency (GTE) index measures the changes of technical efficiency due to resource allocation efficiency and management system while the green technical progress (GTP) index reflects the changes of the technical progress likes improvement of production technological capability and manufacturing process. The GML index reflects the growth rate of urban GTFP, where GML = GTE × GTP. Drawing on the methods of Qiu et al. (2008) and Yuan and Xie (2015), the growth rate of GTFP is converted into the corresponding absolute values, and the GTFP of 2011 is set as the base period with the corresponding value of 1. The GML indices of GTFP, GTE, and GTP are cumulatively multiplied year by year in turn to obtain the corresponding levels of GTFP, GTE, and GTP of each city from 2011 to 2019 respectively. The specific indicators of GTFP are set as in Table 1.
3.2.1.1 Input index
The employees of the whole society represent labor input, the sum of employees in urban units, private employees and individual employees. Capital inputs is expressed by capital stock of each city at constant prices after estimating a base year based on the perpetual inventory method referring to Zhang et al. (2004). Given the available data, the energy input is measured by the total social electricity consumption (million kilowatt-hours) of each city in that year, with a uniform caliber for the whole city.
3.2.1.2 Output index
The expected output is represented by the urban actual GDP at constant prices in 2011. The undesired output consists of industrial wastewater discharge, sulfur dioxide discharge and soot discharge which are called “three waste emissions”. In the robustness test, this paper adds CO2 emissions as the undesired output on the basis of “three waste emissions” and construct a new index using CO2 emissions alone as the undesired output. Drawing on the calculation method of Wu and Guo (2016) and the report of the United Nations Intergovernmental Panel on Climate Change (IPCC), the carbon emissions from gas, electrical heat and LPG consumption are summed to obtain the CO2 emissions, with the data base of the primary energy consumption of coal, crude oil and natural gas.
3.2.2 The digital economy index
Due to the limited methodology and statistical data, there is no commonly accepted index to directly measure the digital economy (DE). This paper constructs the digital economy development index in terms of both digital finance and Internet development level (Huang et al., 2019; Zhao et al., 2020). The former is represented by the overall digital inclusive finance index jointly compiled by the Digital Finance Research Center of Peking University and Ant Finance Group. The latter is evaluated from four aspects: telecommunication business revenue, the number of employees in computer services and software industry, the number of mobile phone users and the number of Internet broadband access subscribers at the end of the year. The specific calculation methods of each index are per capita telecommunication business volume, the proportion of employees in computer and software industry to the total number of employees in the city, the number of mobile phone users per 100 people and the number of Internet access subscribers per 100 people, respectively. The comprehensive index of the digital economy at the urban level is calculated using principal component analysis (PCA) using the software stata15.0 based on the above five dimensions.
3.2.3 Control variables
Drawing on existing studies, the control variables in this paper are as follows: (1) Actual utilization of foreign capital (Fdi) is defined as the ratio of actual utilization foreign capital of each city to GDP in that year. (2) Human capital (Hum) promotes the growth of GTFP (Hu and Guo, 2022), indicated by the proportion of the number of students enrolled in general colleges and universities to the total population at the end of the year (Wu et al., 2021; Hao et al., 2022; Hu et al., 2022; Ren et al., 2022). This may be because the enhancement of human capital through education improves energy efficiency and renewable energy consumption through skilled labor, knowledge sharing and technological progress to reduce energy consumption and pollution emissions (Bano et al., 2018). Besides, it brings additional benefits such as compliance with government rules, reduction of unfairness and crime rates, which are conducive to economic growth (Mehrara et al., 2015). (3) Fiscal intervention (Gover) is proxied by the proportion of fiscal expenditure to GDP. (4) Industrial structure (Indu) is given by the ratio of the added value of the secondary industry to GDP. (5) Environmental regulation (Envir) is expressed as the number of employees in the water, environment and public facilities management industry that reflects the governmental environmental protection. (6) Economic development (lnPgdp) is represented by the logarithm of GDP per capita and its squared term (Pgdp2) to reflect the change of economic scale and its non-linear impact on GTFP. The environmental Kuznets curve hypothesis (EKC) suggests that the impact of economic growth on the environment rises and then declines (Dinda, 2004). It is assumed that the relationship between regional output per capita and GTFP may be U-shaped.
3.2.4 Data source and descriptive statistics
In view of the missing data and administrative area adjustment, a total of 284 Chinese prefecture-level cities from 2011–2019 are used as the research sample. The data is mainly derived from: (1) Digital Inclusive Finance Index jointly published by Digital Finance Research Center of Peking University and Ant Financial Services Group; (2) relevant statistical yearbooks including China Statistical Yearbook, China Regional Economic Statistical Yearbook, China City Statistical Yearbook, statistical yearbooks of provinces and cities and statistical bulletins of prefecture-level cities; (3) China Research Data Service Platform (CNRDS) Innovation Patent Research Database. Table 2 summarizes the descriptive statistics of the main variables in this paper.
4 Empirical analysis
4.1 Benchmark regression results
Table 3 presents the estimated results of the impact of digital economy on GTFP. The coefficient of DE is always significantly positive regardless of gradually adding fixed effects and control variables. The finding in column (6) suggests that the coefficient of DE is 0.0681 at the significant level of 1%, revealing that the digital economy has significantly promoted GTFP. Hypothesis H1 has been well verified. As a key driver of innovation and technology diffusion, the digital economy stimulates to innovate products and processes just as energy saving, pollution prevention and control, waste recycling and green product design (Luo et al., 2022), thus contributing to GTFP of Chinese cities. Next, the coefficient of the control variable basically conforms to the expectation.
To be specific, we distinguish the positive impact path on GTFP. The GTFP can be decomposed into green technology progress index (GTP) and green technology efficiency index (GTE) (Jiang et al., 2021). The specific regression results are reported in Table 4. The findings reveal that DE significantly promotes GTP, but not significantly affects GTE. This means that the digital economy mainly promotes GTFP through motivating technological progress in production technology capabilities, manufacturing process progress, etc. Compared with the growth of green efficiency, the growth of China’s GTFP mainly depends on the progress of green technology. Green technology efficiency reflects the ability to release the potential of existing technology to a greater extent, create economic output and reduce environmental pollution emissions by coordinating production factors under the condition that technology remains unchanged. Strong coordination and efficient integration of resources are the primary conditions for the development of the data element market. However, the cultivation of data element market is still in the exploration stage and there are barriers to data resource sharing. The coordination between production factors is not enough, which leads to the decline of economic output capacity and utilization efficiency of production factors.
4.2 Endogeneity analysis
4.2.1 Instrument variable approach
Considering that the above benchmark regression model may have endogeneity, which may affect the estimation results. First, the higher level of regional green development, the better external resources may be, which will inevitably accelerate the development of digital economy, causing the problem of reverse causality. Second, there are many other unobservable factors that affect the GTFP, which may cause the problem of missing control variables. Therefore, this paper uses instrument variables (IV) to solve above endogenous problems.
On the one hand, historical variables have good exogeneity, many scholars tend to use historical information to solve endogenous problems (Au and Henderson, 2006). This paper adopts the number of telephones, post offices, and total postal services owned by per 10,000 people in 1984 as instrument variables (Huang et al., 2019). The early stage of China’s Internet development is mainly started with analogue telephones lines. Hence the Internet development level is high in regions with more fixed telephone subscribers, meeting the relevance conditions. The post office was a main traditional tool for information transmission and exchange in the past, which was the executive department of laying fixed line. Its regional distribution affects the distribution of fixed telephone to a certain extent, thus affecting the early access of the Internet (Huang et al., 2019). Therefore, it can be expected that the Internet development level is relatively high in regions with more post offices and postal services, meeting the relevance conditions. At the same time, the number of fixed telephones, post offices and postal services in history can hardly affect the green development, hence meeting the exogenous conditions.
On the other hand, geographic location variable can also act as exogenous instrument variables (Bai and Zhang, 2021). This paper selects the distance to coastal port, and the distance between each city and Hangzhou as IV, respectively (Bai and Yu, 2021). Geographic location is a fixed fait accompli which is not affected by external factors, thus meeting the exogenous conditions. As the core resource and open platform of a region, coastal port is a basic pivotal facility and an important support for economic development. It can bring linkage effect and radiation effect to the regional digital economy. In addition, the development of digital finance represented by Alipay originates in Hangzhou. In summary, the distance to coastal port, the distance to Hangzhou is related to the development level of regional digital economy. The closer the city is to the coastal port and Hangzhou, the higher the level of digital development will be.
In order to make the instrument variables meet the time variability of panel data, this paper uses the interactive term of the logarithm of the above variables and the logarithm of the number of broadband Internet access ports as the instrument variables of the digital economy (Nunn and Qian, 2014). Table 5 reports the estimated results. The first stage regression results of 2SLS indicate that the regression coefficients of Telephone-1984, Post office-1984 and Total postal services-1984 are respectively 0.1213, 0.04796, and 0.09297, passing the statistical test at 1%. At the same time, the estimated coefficients of Distance to coastal port and Distance to Hangzhou are significantly negative at 5% and 1%, respectively. The above first stage results indicate that DE is significantly related to the instrumental variables. The F statistics of the first stage are 149.22, 146.96, 156.39, 171.75 and 172.65, respectively, which are far greater than the critical value of the rule of thumb 10, proving that there is no problem of weak IV. The second stage estimated results of 2SLS show that no matter what instrumental variables are selected, DE is still significant positive at the level of 5% or more, indicating that the digital economy has significantly promoted urban GTFP. These results indicate that the relationship between the digital economy and green development is still stable and effective after overcoming the endogenous issues.
4.2.2 Exogenous policy impact test
The quasi-natural experiment is applied to further eliminate the endogenous problems, demonstrating robustness of results. To address the slow network speed, low broadband coverage together with uneven regional development of information infrastructure construction, the State Council of China released the implementation plan of the “Broadband China” strategy in 2013. This plan deployed the broadband development goals and paths in the next 8 years and selected 120 cities (clusters) in three batches in 2014, 2015 and 2016 as the “Broadband China” pilot cities. The “Broadband China” strategy drives the implementation of new infrastructure construction such as 5G and gigabit broadband, accelerating the popularization and development of urban Internet. The digital economy can’t develop without the support of network infrastructure. In other words, the more perfect the urban network infrastructure is, the higher the level of urban digital economy development. As an exogenous impact, the “Broadband China” demonstration policy can effectively represent the development of the digital economy (Zhao et al., 2020). Consequently, the demonstration policy of “Broadband China” is used as a quasi-natural experiment to test the causal influence of the digital economy on GTFP. Considering that this strategy is a policy experiment from pilot to popularization in batches, the multi-period difference in difference (DID) method proposed by Beck et al. (2010) is adopted in this paper. The empirical model is set in Formula (2):
Table 6 reports the regression results. As shown in column (1), the coefficient of Policy is 0.0784 and passes the 1% significance level, which indicates that the demonstration policy of Broadband China has improved the urban GTFP. After replacing GTFP with GTP and GTE respectively, the results show that the pilot policy significantly promotes GTP, but not significantly affects GEC. Hypothesis H1 is confirmed again. The parallel trend results are shown in Table 7. The estimated coefficients of Pre1, Pre2, Pre3 and Pre4 are not significant, and the results show that there is no systematic difference between the change trends of demonstration cities and non-demonstration cities before the implementation of policies, meeting the parallel trend test. In terms of dynamic effects, from the second year to the fifth year of the demonstration cities, Post2 passes the 10% significance level, Post3 passes the 5% significance level, and Post4 and Post5 pass the 1% significance test. The positive promotion effect shows an increasing trend year by year, and the parallel trend hypothesis is satisfied.
4.3 Robustness tests
4.3.1 Replacing independent variable and dependent variable
To mitigate the error caused by the index construction method, this paper constructs a new index to replace the core explanatory variable and dependent variable. On the one hand, a new measure index of the digital economy is constructed by entropy method in this paper. Column (1) of Table 8 reports the regression result. The regression coefficient of DE is still significantly positive, passing the 1% confidence interval. It indicates that the empirical results of the positive impact of the digital economy on urban GTFP remain robust. On the other hand, this paper changes the calculation method of GTFP. Considering that the unexpected output used to measure the GTFP index is mainly Industrial soot, SO2 and industrial wastewater, which may lead to estimation errors. This paper recalculates the urban GTFP by adding carbon dioxide emissions data as the unexpected output on the basis of the original unexpected output and using carbon dioxide emissions data as the unexpected output alone. As shown in column (2) and column (3) of Table 8, the regression coefficient of DE is still significantly positive, proving the robustness of the benchmark estimation results again.
4.3.2 Excluding samples of key cities
With a large population and relatively concentrated social resources, municipalities directly under the Central Government play an important role in China’s economic development. In order to eliminate the impact of special samples on the results, this paper change the sample size to exclude the samples of these big cities, namely Beijing, Tianjin, Shanghai and Chongqing. It can be seen from column (4) of Table 8, the regression coefficient of DE is 0.0654, passing the 1% confidence interval, which has no substantial change compared with the baseline regression results.
4.4 Heterogeneity analysis
4.4.1 Heterogeneity based on urban resource dependence
Cities are the most concentrated and prominent regions of global ecological environment problems. With the strong resource orientation, the resource-based cities are generally facing serious ecological and environmental problems. In recent years, scholars have also been exploring the green development path of resource-based cities. To investigate whether the digital economy promotes the green development of cities with different resource dependence, the 284 cities are divided into resource-based and non-resource-based cities according to the National Sustainable Development Plan for Resource-based Cities (2013–2020).
Table 9 reports the regression results. The estimated results in columns (1) and (4) show that DE exerts a positive impact on GTFP at a significance level of 1% in non-resource-based cities, while not significant in resource-based cities. As the digital economy has obvious characteristics of time stages, it is necessary to compare the stage changes generated by the development of the digital economy in different resource-dependent cities. Since the “13th Five-Year Plan Proposal” in 2015 first proposed to expand the network economic space and implement the national big data strategy, the scale of the digital economy has achieved leapfrog development and relevant policies have been implemented. Therefore, this paper divides the sample into two time periods: 2011–2014 and 2015–2019. The regression results are shown in columns (2)–(3) and (5)–(6). The estimated coefficient of DE is significantly positive at the 1% level before and after 2015 in non-resource-based cities, and the direct effect increases from 0.0423 to 0.0429. As for resource-based cities, the coefficient of DE is not significant before 2015, but significantly after 2015. The result indicates that the digital economy significantly promotes GTFP in resource-based cities after 2015. The reason for this may be that early resource-based cities are rich in resources and their economic development is mainly based on energy-intensive industries such as coal, metallurgy and coking. These cities have a single industrial structure and weak development of successive industries, which are more likely to produce “black” industrial path dependence and more prominent environmental problems, resulting in the fact that the influence of the digital economy on GTFP in resource-based cities is not significant. After 2015, the strong economic vitality and industrial resilience of the digital economy bring about an efficient matching of technological resources and traditional factor resources, which makes resource-based cities have a stronger marginal effect in improving energy efficiency with digital empowerment. Therefore, the digital economy can change the economic development mode of resource-based cities with low added value and break the “resource curse” to low-carbon, green and intelligent and promote the green transformation of cities. The above results suggest that the impact of the digital economy on urban GTFP is heterogeneous in resource dependence.
4.4.2 Heterogeneity analysis of geographical location
The unbalanced and insufficient economic development in different regions of China may lead to a “digital divide” between regions (Ren et al., 2021). Based on this, we first divided the 284 cities into eastern coastal cities and non-eastern coastal cities according to the classification standard of China Ocean Statistical Yearbook. Additionally, we further divided the 284 cities into the eastern, central and western regions according to the economic development level. Table 10 reports the location heterogeneity regression results.
Our results in columns (1)–(2) present that the coefficient of DE is 0.0638 at the 1% significance level in eastern coastal cities, and 0.0512 at the 5% significance level in non-eastern coastal cities. These findings indicate that the digital economy plays a positive role in promoting GTFP in both eastern and non-eastern coastal cities. The significance and contribution of the digital economy to GTFP in eastern coastal cities are better than those in non-eastern coastal cities. The reason is that the eastern coastal areas have policy advantages and the geographical location advantages close to the ports, which are beneficial to attract production factors such as talents, technology and information and generate a strong level of digital technology application and innovation, thus promoting green development. Our results present that the coefficients of DE in east and central regions are 0.0562 and 0.0893 at the 1% significant level respectively, but not significantly in the western region (columns 3–5). Specifically, the positive impact of the digital economy on GTFP shows a trend of central > eastern > western. There are several possible reasons. First, the development level of the digital economy in the eastern and central regions is relatively high, which helps to promote GTFP. However, the construction and investment of digital infrastructure in the western regions is not perfect, resulting that the development level of digital economy in the western region is too low, which is not conducive to the green growth effect of cities. The above conclusions are consistent with the analysis results of the threshold effect of the digital economy below. Second, the level of green development and digital economy in the eastern region is higher than that in the central region. Due to the law of diminishing marginal effect, the marginal green emission reduction effect of the digital economy is lower than that in the central region. The above findings show that there is geographical location heterogeneity on the impact of the digital economy to GTFP.
4.4.3 Heterogeneity of openness and financial development perspectives
The impact of the digital economy on GTFP may be affected by a number of local characteristics. We focus on two potential moderators, namely, openness and financial development.
Many countries have formulated various incentive measures and policies to improve the degree of openness. Openness can drive regional economic growth by absorbing technology spillovers generated by foreign investment. The degree of openness of a city is measured by the proportion of the total amount of foreign capital actually utilized to GDP in that year. According to the median of the openness in the sample, the sample is divided into two groups. As shown in columns (1)–(2) of Table 11, the coefficient of DE is positive and has passed the significance level of 1% in cities with high level of openness, while the coefficient is not significant in areas with low level of openness. These results suggest that openness plays a positive moderating role. For one thing, the increase in foreign direct investment inflows has improved the ability to absorb, adapt and innovate technologies and strengthens industrial specialization, so as to promote the development of ICT (Arvin et al., 2021). For another, regions with a high degree of economic openness usually realize economies of scale by using foreign markets and improve their technological level accordingly, while the application of international advanced technology plays an important role in reducing energy consumption and pollution emissions (Adom and Amuakwa-Mensah, 2016).
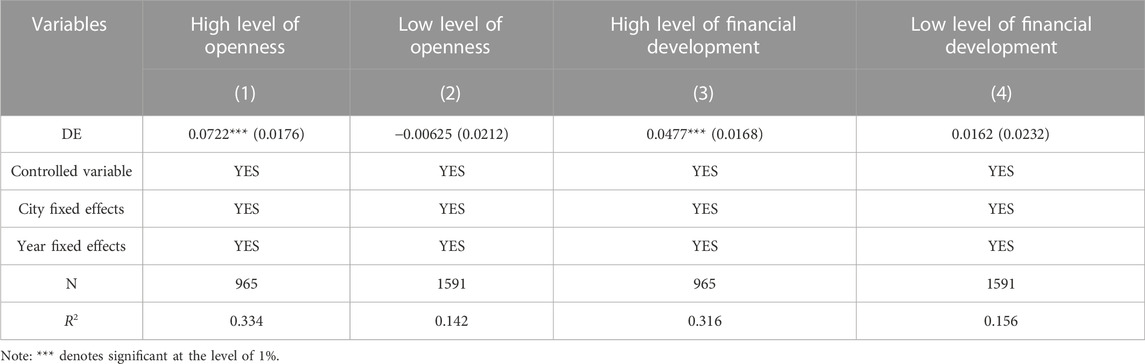
TABLE 11. Heterogeneity test regression results based on External Opening and Financial Development.
As an important part of the country’s economic development, financial development can stimulate enterprises to engage in technological innovation. We use the proportion of RMB deposits and loans of banking financial institutions to the regional GDP to measure the financial development level in each city. We divide the sample into two groups according to the intermediate level of financial development, and columns (3)–(4) of Table 11 present the regression results. The coefficient of DE is positive at 1% significance level in cities with high level of financial development, while the coefficient is not significant in areas with low level of financial development. The possible reason is that data plays a key role in the core function of finance to enhance resource allocation efficiency. A sound financial service system can ease the financing constraints of various innovative entities and provide digital payment services and financing services for the development of the digital economy.
The above results indicate that the influence of the digital economy on GTFP is heterogeneous, which is manifested in the difference between the level of urban openness and the level of financial development, both of which have played a positive regulatory role.
5 Influencing mechanism analysis
The results of the previous study indicate that the development of the digital economy can significantly promote the growth of urban GTFP. Then what is its transmission mechanism? We explore the possible mechanisms based on the mediating effect model (Baron and Kenny, 1986), and establish the following regression equation:
where Mechanic is the mechanism variable in this paper, three mediators are used, namely green technological innovation, industrial structure upgrading and energy conservation. The other variables are the same as in Equation 1, with individual fixed effects
5.1 Green technological innovation effect
The digital economy encourages green technological innovation, thus promoting urban GTFP. To verify this mechanism, this paper uses the number of green invention patents per 10,000 people to measure urban green technological innovation. As shown in column (1) of Table 12, the coefficient of DE on green technological innovation is 0.101 at the 1% significance level. The result implies that the digital economy promotes urban green technological innovation. The coefficient of green technological innovation on urban GTFP is positive at the 1% significance level (column 2). In a word, green technological innovation is an effective mediating pathway whereby the digital economy can promote GTFP.
5.2 Industrial structure upgrading effect
Theoretically, the digital economy can change the original service mode and realize the green development through the optimization and upgrading of industrial structure. According to the study of Zhao et al. (2021), this paper uses the ratio of the value added of tertiary industry to secondary industry to measure industrial structure upgrading. In column (3) of Table 12, the coefficient of DE is significantly positive, indicating that the digital economy has significantly promoted the upgrading of industrial structure. Column (4) shows that the coefficient of industrial structure upgrading is also positive at the 1% significance level. In a word, industrial structure upgrading is an effective mediating pathway whereby the digital economy can promote GTFP.
5.3 Energy conservation effect
Theoretically, the digital economy can promote the efficiency of energy utilization thus promoting the green development of cities. To prove this influence mechanism, this paper uses the city’s total social electricity consumption per unit of GDP to measure energy consumption. As shown in column (5) of Table 12, the coefficient of DE is significantly negative at 5% confidence level. The result implies that the development of the digital economy significantly reduces energy consumption. In column (6), the energy consumption has a significant negative effect on the urban GTFP at 1% confidence level. In a word, energy conservation effect is an effective mediating pathway whereby the digital economy can promote GTFP. The digital economy can reduce energy consumption by optimizing production processes, reducing and replacing economic activities, introducing the use of complementary products and improving waste management processes (Cecere et al., 2014), thus promoting the urban GTFP.
To sum up, the results in this section show that the digital economy can improve the growth of GTFP by green technological innovation, industrial structure upgrading and energy conservation. Hypothesis 2 is verified.
6 Analysis of spatial spillover effects
In the previous analysis, we constructed a multiple linear regression and exogenous policy impact test to verify the influence of the digital economy on GTFP. However, due to the scale effect and networking characteristics of the digital economy, the flow of it among cities is not independent of each other. Based on this, this part invokes the spatial interaction term in Equation 1 using the spatial Durbin model (SDM) to examine the spatial spillover effect of the digital economy on GTFP. The model is constructed as follows:
W represents the n × n dimensional spatial weight matrix;
It can be seen from Table 13 that after transforming the spatial weight matrices, the regression coefficients of DE are 0.0659, 0.0712, 0.0527, and 0.0506, all of which are positive at 1% significance level. Since the SDM explains the spatial economic correlations among cities, the estimated results of its spatial econometric model including spatial lags cannot directly report the real impact of the spatial spillover effects of the explanatory variables on the explained variables. The decomposition results through spatial effects show that all effects are significantly positive regardless of the spatial weights matrices chosen, and the indirect effect accounts for more than 50% of the total effect. The digital technology accelerates the diffusion of information and various resource elements and guide the cross-regional labor division and cooperation. It triggers a learning and imitation effect by facilitating knowledge spillover and innovation resources exchange (Proeger and Runst, 2020), thus driving the improvement of green total factor productivity in the neighboring areas. The results prove that the digital economy significantly enhances green GTFP of neighboring cities and hypothesis 3 is verified.
7 Threshold effect
Considering the network effect of the Internet and Metcalfe’s Law, there may have a non-linear relationship of the digital economy on GTFP. Therefore, we use the dynamic panel threshold regression model proposed by Hansen (1999) to test the threshold effect, as shown in the formula:
Where, qit is the threshold variable of digital economy, I (·) is the indicator function, and Y is the threshold parameter. Eq. 6 considers the case of a single threshold, and the multi threshold model can be extended from Eq. 6. Whether the single threshold model or the multi threshold model is used needs further testing.
Considering the “network effect” of the digital economy, there is a non-linear relationship (hypothesis 4). First of all, based on the Bootstrap method proposed by Hansen (1999), we repeatedly sampled 1000 times to verify whether there is a threshold effect with DE as the threshold variable. As shown in Table 14 and Table 15, DE has passed the single threshold test at the 1% significance level, and the threshold value is -0.7448, but the double threshold test has not passed the significance test.
Next, we estimate the parameters of the threshold model through empirical analysis. Table 16 reports the regression results of panel threshold model, indicating that there is a single threshold effect on the impact of the digital economy on GTFP. When DE is less than the threshold value of -0.7448, the impact is not significant. When DE crosses -0.7448, the regression coefficient increases to 0.132 at the 1% significance level. On the basis of the network effect, the value of the network depends upon the size of its other users. When the development level of the digital economy is low, its network effect is too small to form a network value advantage and economies of scale relative to competitors. Enterprises have no incentive to achieve energy conservation and emission reduction to obtain high profits, which is not conducive to the play of urban green growth effect. Therefore, when DE is lower than the threshold value of -0.7448, the digital economy has no significant impact on green development. As the broadband usage, digital infrastructure construction and Internet access continuously increase until the network effect exceeds a critical value, the enterprise’s products or services can quickly obtain a sufficient number of users or suppliers, which is conducive to the exertion of the network effect and initiation of the positive influence mechanism, thus promoting urban GTFP.
8 Conclusions and discussions
8.1 Conclusions
The limited resources and environmental degradation make it necessary to achieve green development. This paper empirically proves the impact, transmission mechanism, spatial spillover effect and non-linear effect of the digital economy on green development using the data of 284 prefecture-level cities in China. Different from the research result of Lange et al. (2020), this paper believes that the hope of digitalization to promote sustainable development can be realized. The overall research conclusion has strong application value in the current era of digital transformation, providing a reference for emerging countries to achieving green development through the digital economy. The main research conclusions present as follows:
(1) The digital economy can significantly improve urban GTFP. The growth of China’s GTFP is mainly attributed to green technological progress (GTP). (2) The boosting effect of the digital economy on GTFP has significant heterogeneity on city resource dependence, geographical location, openness and financial development level. First, the digital economy significantly boosts GTFP of resource-based cities after 2015 and the boosting effect is greater than that of non-resource-based cities. Next, the boosting effect of the digital economy on GTFP shows that the eastern coastal cities are greater than the non-eastern coastal cities, and the central-east regions are greater than the west regions. Finally, cities with higher level of financial development and openness, the stronger its promotion. This paper analyzes heterogeneity from a new perspective, enriching the existing heterogeneity analysis. It provides an empirical evidence for the government to improve the opening up, the level of financial development and accelerate the digital transformation of resource-based cities. (3) Green technological innovation, industrial structure upgrade and energy conservation are the important mediating mechanisms for the digital economy on GTFP. The more comprehensive mechanism test in this paper deepens the existing literature. (4) The spatial Durbin econometric model analysis reveals that the digital economy has a significant spatial spillover impact on GTFP, and it can promote GTFP on the surrounding areas. (5) Using the threshold model, it is found that the effect of the digital economy on urban GTFP growth is non-linear and has a single threshold. The low development level of the digital economy is not conducive to the green growth effect.
8.2 Discussions
8.2.1 Policy implications
On the basis of the above empirical results, the following important policy suggestions are put forward:
1) Accelerate the development of the digital economy to promote the green development of cities. First of all, it is necessary to actively improve the deep integration of digital technology and the real economy. Enterprises can increase the application of digital technologies such as industrial Internet, big data and 5G, giving full play to the green enabling role of digital technology from the energy supply side and the industrial demand side to energy saving and waste reduction in the production process. Secondly, intelligence or information technology should be introduced into urban management. For example, in the field of smart transportation, reduce carbon emissions through new energy technologies and intelligent networking. Finally, in terms of government governance, it is necessary to actively participate in the construction of digital society and digital government, realizing intelligent interconnection and data sharing. In addition, it is also necessary to promote the research, development and application of green energy-related patents, and promote the intelligent and green development of traditional industries by optimizing and upgrading the traditional industrial structure.
2) Implement targeted and precise regional policies to address the imbalance and regional differences brought about by the development of the digital economy. On the one hand, the government should base itself on the low-cost advantages of the central and western regions, increase the construction and investment of infrastructure related to the digital economy, and promote the penetration of the digital economy in these regions. On the other hand, local governments should speed up the digital transformation of resource-based cities for considering resource endowment conditions and local industrial chain ecology. Finally, it is worthwhile to improve the level of urban openness and financial development, enhancing the positive impact of the digital economy on urban GTFP. At the same time, considering that cities with developed digital economy have radiation and spillover effects, it is necessary to strengthen exchanges and cooperation in technology, talents, data and other resources between cities. It conduces to formation of complementary advantages and coordinated development between regions, so as to drive the green development of surrounding cities.
8.2.2 Future research directions and limitations
There are some limitations in this study. First of all, this paper combined urban panel data with certain sample limitations. Subsequent studies can further demonstrate the relationship between digital economy and green development from a micro perspective such as enterprise level or county level data. Secondly, this paper does not establish a theoretical model, and subsequent research can build models to enrich relevant theoretical mechanisms. Finally, there is no uniform standard and normative guidance for the selection and measurement of indicators of the digital economy. In future research, scholars can formulate unified urban digital economy measurement indicators to accurately measure comparable digital economy development scale. Subsequent studies can also further confirm the impact of various indicators of the digital economy segmentation on green development, such as industrial digitization and digital industrialization.
Data availability statement
The raw data supporting the conclusions of this article will be made available by the authors, without undue reservation.
Author contributions
Conceptualization, YX and SW; methodology, YX; software, YX; validation, YX; formal analysis, YX, SW, and HL; investigation, YX; data curation, YX and HL; writing—original draft preparation, YX and SW; writing—review and editing, YX and SW; visualization, YX and SW; supervision, ZL; All authors have read and agreed to the published version of the manuscript.
Funding
This research was funded by National Social Science Foundation of China (grant number20STA029).
Conflict of interest
The authors declare that the research was conducted in the absence of any commercial or financial relationships that could be construed as a potential conflict of interest.
Publisher’s note
All claims expressed in this article are solely those of the authors and do not necessarily represent those of their affiliated organizations, or those of the publisher, the editors and the reviewers. Any product that may be evaluated in this article, or claim that may be made by its manufacturer, is not guaranteed or endorsed by the publisher.
Footnotes
1The data are obtained from the “Development of China’s Digital Economy” white paper (2022).
References
Adom, P. K., and Amuakwa-Mensah, F. (2016). What drives the energy saving role of FDI and industrialization in East Africa? Renew. Sustain. Energy Rev. 65, 925–942. doi:10.1016/j.rser.2016.07.039
Arvin, M. B., Pradhan, R. P., and Nair, M. (2021). Uncovering interlinks among ICT connectivity and penetration, trade openness, foreign direct investment, and economic growth: The case of the G-20 countries. Telematics Inf. 60, 101567. doi:10.1016/j.tele.2021.101567
Au, C. C., and Henderson, J. V. (2006). Are Chinese cities too small? Rev. Econ. Stud. 73, 549–576. doi:10.1111/j.1467-937X.2006.00387.x
Bai, P. W., and Yu, L. (2021). Digital economy development and firms’ markup: Theoretical mechanisms and empirical facts. China Ind. Econ. 11, 59–77. doi:10.19581/j.cnki.ciejournal.2021.11.003
Bai, P. W., and Zhang, Y. (2021). The digital economy, the declining demographic dividend and the rights of low- and middle-skilled workers. Econ. Res. 5, 91–108.
Balogun, A. L., Marks, D., Sharma, R., Shekhar, H., Balmes, C., Maheng, D., et al. (2020). Assessing the potentials of digitalization as a tool for climate change adaptation and sustainable development in urban centres. Sustain. Cities Soc. 53, 101888. doi:10.1016/j.scs.2019.101888
Bano, S., Zhao, Y. H., Ahmad, A., Wang, S., and Liu, Y. (2018). Identifying the impacts of human capital on carbon emissions in Pakistan. J. Clean. Prod. 183, 1082–1092. doi:10.1016/j.jclepro.2018.02.008
Baron, R. M., and Kenny, D. A. (1986). The moderator-mediator variable distinction in social psychological research: Conceptual, strategic, and statistical considerations. J. Personality Soc. Psychol. 51, 1173–1182. doi:10.1037//0022-3514.51.6.1173
Beck, T., Levine, R., and Levkov, A. (2010). Big bad banks? The winners and losers from bank deregulation in the United States. J. Finance 65, 1637–1667. doi:10.1111/j.1540-6261.2010.01589.x
Cai, W., and Li, G. (2018). The drivers of eco-innovation and its impact on performance: Evidence from China. J. Clean. Prod. 176, 110–118. doi:10.1016/j.jclepro.2017.12.109
Cardona, M., Kretschmer, T., and Strobel, T. (2013). ICT and productivity: Conclusions from the empirical literature. Inf. Econ. Policy 25, 109–125. doi:10.1016/j.infoecopol.2012.12.002
Cecere, G., Corrocher, N., Gossart, C., and Ozman, M. (2014). Technological pervasiveness and variety of innovators in green ICT: A patent-based analysis. Res. Policy 43, 1827–1839. doi:10.1016/j.respol.2014.06.004
Chung, H. (2018). ICT investment-specific technological change and productivity growth in Korea: Comparison of 1996–2005 and 2006–2015. Telecommun. Policy 42, 78–90. doi:10.1016/j.telpol.2017.08.005
Danish, K. (2019). Effects of information and communication technology and real income on CO2 emissions: The experience of countries along Belt and road. Telematics Inf. 45, 101300. doi:10.1016/j.tele.2019.101300
Danish, K., and Ulucak, R. (2021). Renewable energy, technological innovation and the environment: A novel dynamic auto-regressive distributive lag simulation. Renew. Sustain. Energy Rev. 150, 111433. doi:10.1016/j.rser.2021.111433
Dinda, S. (2004). Environmental Kuznets curve hypothesis: A survey. Ecol. Econ. 49, 431–455. doi:10.1016/j.ecolecon.2004.02.011
Emrouznejad, A., and Yang, G. L. (2018). A survey and analysis of the first 40 years of scholarly literature in DEA: 1978–2016. Socio-Economic Plan. Sci. 61, 4–8. doi:10.1016/j.seps.2017.01.008
Fukuyama, H., and Weber, W. L. (2009). A directional slacks-based measure of technical inefficiency. Socio-Economic Plan. Sci. 43, 274–287. doi:10.1016/j.seps.2008.12.001
G20 (2016). G20 digital economy development and cooperation initiative [EB/OL]. (2016-09-20) [2019-08-01] Available at: http://www.g20chn.org/hywj/dncgwj/201609/t20160920_3474.html (Accessed September 20, 2016).
Hansen, B. E. (1999). Threshold effects in non-dynamic panels: Estimation, testing, and inference. J. Econ. 93, 345–368. doi:10.1016/S0304-4076(99)00025-1
Hao, X. L., Wang, X. H., Wu, H. T., and Hao, Y. (2022). Path to sustainable development: Does digital economy matter in manufacturing green total factor productivity? Sustain. Dev. doi:10.1002/sd.2397
He, F., and Liu, H. X. (2019). Assessment of the performance enhancement effect of digital change in real enterprises from the perspective of digital economy. Reform 4, 137–148.
Higón, D. A., Gholami, R., and Shirazi, F. (2017). ICT and environmental sustainability: A global perspective. Telematics Inf. 34, 85–95. doi:10.1016/j.tele.2017.01.001
Hu, X. Y., and Guo, P. F. (2022). A spatial effect study on digital economy affecting the green total factor productivity in the Yangtze River Economic Belt. Environ. Sci. Pollut. Res. 29, 90868–90886. doi:10.1007/s11356-022-22168-9
Huang, Q. H., Yu, Y. Z., and Zhang, S. L. (2019). Internet development and manufacturing productivity improvement: Intrinsic mechanism and Chinese experience. China Ind. Econ. 8, 5–23. doi:10.19581/j.cnki.ciejournal.2019.08.001
Iqbal, J., Khan, M., Talha, M., Farman, H., Jan, B., Muhammad, A., et al. (2018). A generic internet of things architecture for controlling electrical energy consumption in smart homes. Sustain. Cities Soc. 43, 443–450. doi:10.1016/j.scs.2018.09.020
Jiang, H. L., Jiang, P. C., Wang, D., and Wu, J. H. (2021). Can smart city construction facilitate green total factor productivity? A quasi-natural experiment based on China’s pilot smart city. Sustain. Cities Soc. 69, 102809. doi:10.1016/j.scs.2021.102809
Kim, K., Bounfour, A., Nonnis, A., and Ozaygen, A. (2021). Measuring ICT externalities and their contribution to productivity: A bilateral trade based approach. Telecommun. Policy 55, 102085. doi:10.1016/j.telpol.2020.102085
Lange, S., Santarius, T., and Pohl, J. (2020). Digitalization and energy consumption: Does ICT reduce energy demand? Ecol. Econ. 176, 106760–106773. doi:10.1016/j.ecolecon.2020.106760
Lennerfors, T. T., Fors, P., and Rooijen, V. J. (2015). ICT and environmental sustainability in a changing society: The view of ecological World Systems Theory. Inf. Technol. People 28, 758–774. doi:10.1108/ITP-09-2014-0219
Li, H. S., Tian, Y. X., and Li, W. J. (2014). Internet thinking and traditional enterprise reengineering. China Ind. Econ. 10, 135–146. doi:10.19581/j.cnki.ciejournal.2014.10.011
Li, J. X., Zhou, K., and Cheng, Z. H. (2022). Does China's “Belt and Road” Initiative promote green total factor productivity growth in countries along the route? J. Clean. Prod. 93, 133004. doi:10.1016/j.jclepro.2022.133004
Li, M. J., and Du, W. J. (2021). Can Internet development improve the energy efficiency of firms: Empirical evidence from China. Energy 237, 121590. doi:10.1016/j.energy.2021.121590
Li, X. H. (2019). New features of the digital economy and the formation mechanism of new dynamics of the digital economy. Reform 11, 40–51.
Li, Z., G., and Wang, J. (2022). The dynamic impact of digital economy on carbon emission reduction: Evidence city-level empirical data in China. J. Clean. Prod. 351, 131570. doi:10.1016/j.jclepro.2022.131570
Li, Z. G., and Wang, J. (2021). Digital economy development, data factor allocation and manufacturing productivity improvement. Econ. 10, 41–50. doi:10.16158/j.cnki.51-1312/f.2021.10.005
Liu, Z., and Xin, L. (2019). Has China’s Belt and road initiative promoted its green total factor productivity? Evidence from primary provinces along the route. Energy Policy 129, 360–369. doi:10.1016/j.enpol.2019.02.045
Luo, S., Yimamu, N., Li, Y., Wu, H., Irfan, M., and Hao, Y. (2022). Digitalization and sustainable development: How could digital economy development improve green innovation in China? Business strategy and the environment, 1–25. doi:10.1002/bse.3223
Ma, D., and Zhu, Q. (2022). Innovation in emerging economies: Research on the digital economy driving high-quality green development. J. Bus. Res. 145, 801–813. doi:10.1016/j.jbusres.2022.03.041
Martin, C. J., Evans, J., and Karvonen, A. (2018). Smart and sustainable? Five tensions in the visions and practices of the smart-sustainable city in europe and north America. Technol. Forecast. Soc. Change 133, 269–278. doi:10.1016/j.techfore.2018.01.005
Mehrara, M., Rezaei, S., and Razi, D. H. (2015). Determinants of renewable energy consumption among ECO countries; based on Bayesian model averaging and weighted-average least square. Int. Lett. Soc. Humanist. Sci. 54, 96–109. doi:10.18052/www.scipress.com/ilshs.54.96
Miao, C. L., Fang, D. B., Sun, L. Y., and Luo, Q. L. (2017). Natural resources utilization efficiency un-der the influence of green technological innovation. Resour. Conservation Recycl. 126, 153–161. doi:10.1016/j.resconrec.2017.07.019
Nunn, N., and Qian, N. (2014). US food aid and civil conflict. Am. Econ. Rev. 104, 1630–1666. doi:10.1257/aer.104.6.1630
Obobisa, E. S., Chen, H. B., and Mensah, I. A. (2022). The impact of green technological innovation and institutional quality on CO2 emissions in African countries. Technol. Forecast. Soc. Change 180, 121670. doi:10.1016/j.techfore.2022.121670
Ozcan, B., and Apergis, N. (2018). The impact of internet use on air pollution: Evidence from emerging countries. Environ. Sci. Pollut. Res. 25, 4174–4189. doi:10.1007/s11356-017-0825-1
Pan, W. R., Xie, T., Wang, Z. W., and Ma, L. (2022). Digital economy: An innovation driver for total factor productivity. J. Bus. Res. 139, 303–311. doi:10.1016/j.jbusres.2021.09.061
Pan, Y. L., and Dong, F. (2022). Dynamic evolution and driving factors of new energy development: Fresh evidence from China. Technol. Forecast. Soc. Change 176, 121475. doi:10.1016/j.techfore.2022.121475
Park, Y., Meng, F., and Baloch, M. A. (2018). The effect of ICT, financial development, growth, and trade openness on CO2 emissions: An empirical analysis. Environ. Sci. Pollut. Res. 25, 30708–30719. doi:10.1007/s11356-018-3108-6
Pei, C. H., Ni, J. F., and Li, Y. (2018). Analysis of the political economy of the digital economy. Finance Trade Econ. 39, 5–22. doi:10.19795/j.cnki.cn11-1166/f.2018.09.001
Proeger, T., and Runst, P. (2020). Digitization and knowledge spillover effectiveness—evidence from the “German mittelstand”. J. Knowl. Econ. 11, 1509–1528. doi:10.1007/s13132-019-00622-3
Qiu, B., Yang, S. H., and Xin, P. J. (2008). A study on FDI technology spillover channel and manufacturing productivity growth in China: An analysis based on panel data. World Econ. 8, 20–31.
Qiu, S. L., Wang, Z. L., and Liu, S. (2021). The policy outcomes of low-carbon city construction on urban green development: Evidence from a quasi-natural experiment conducted in China. Sustain. Cities Soc. 66, 102699. doi:10.1016/j.scs.2020.102699
Ramirez, L. L. J., Puerta Aponte, G., and Rodriguez Garcia, A. (2019). Internet of things applied in healthcare based on open hardware with low-energy consumption. Healthc. Inf. Res. 25, 230–235. doi:10.4258/hir.2019.25.3.230
Ren, S. M., Li, L. Q., Han, Y. Q., Hao, Y., and Wu, H. T. (2022). The emerging driving force of inclusive green growth: Does digital economy agglomeration work. Bus. Strategy Environ. 31, 1656–1678. doi:10.1002/bse.2975
Ren, S. Y., Hao, Y., Xu, L., Wu, H. T., and Ba, N. (2021). Digitalization and energy: How does internet development affect China's energy consumption? Energy Econ. 98, 105220. doi:10.1016/j.eneco.2021.105220
Roscia, M., Longo, M., and Lazaroiu, G. C. (2013). Smart City by multi-agent systems. Int. Conf. Renew. Energy Res. Appl. (ICRERA), 371–376. doi:10.1109/ICRERA.2013.6749783
Sadorsky, P. (2012). Information communication technology and electricity consumption in emerging economies. Energy Policy 48, 130–136. doi:10.1016/j.enpol.2012.04.064
Salahuddin, M., and Alam, K. (2016). Information and communication technology, electricity consumption and economic growth in OECD countries: A panel data analysis. Int. J. Electr. Power Energy Syst. 76, 185–193. doi:10.1016/j.ijepes.2015.11.005
Schulte, P., Welsch, H., and Rexhäuser, S. (2016). ICT and the demand for energy: Evidence from OECD countries. Environ. Resour. Econ. 63, 119–146. doi:10.1007/s10640-014-9844-2
Stefanie, K., and David, T. (2021). Digitalisation, sustainable industrialisation and digital rebound – asking the right questions for a strategic research agenda. Energy Res. Soc. Sci. 82, 102295. doi:10.1016/j.erss.2021.102295
Tao, C. Q., Yi, M. Y., and Wang, C. S. (2023). Coupling coordination analysis and Spatiotemporal heterogeneity between data elements and green development in China. Econ. Analysis Policy 77, 1–15. doi:10.1016/j.eap.2022.10.014
Teece, D. J. (2018). Profiting from innovation in the digital economy: Enabling technologies, standards, and licensing models in the wireless world. Res. Policy 47, 1367–1387. doi:10.1016/j.respol.2017.01.015
Tranos, E., Kitsos, T., and Ortega-Argilés, R. (2021). Digital economy in the UK: Regional productivity effects of early adoption. Reg. Stud. 55, 1924–1938. doi:10.1080/00343404.2020.1826420
Wang, H. R., Cui, H. R., and Zhao, Q. Z. (2021). Effect of green technology innovation on green total factor productivity in China: Evidence from spatial durbin model analysis. J. Clean. Prod. 288, 125624. doi:10.1016/j.jclepro.2020.125624
Wang, L., Chen, Y. Y., Ramsey, T. S., and Hewing, G. (2021). Will researching digital technology really empower green development. Technol. Soc. 66, 101638. doi:10.1016/j.techsoc.2021.101638
Wang, M., and Feng, C. (2021). Revealing the pattern and evolution of global green development between different income groups: A global meta-frontier by production technology approach. Environ. Impact Assess. Rev. 89, 106600. doi:10.1016/j.eiar.2021.106600
Watanabe, C., Naveed, K., Tou, Y., and Neittaanmäkia, P. (2018). Measuring GDP in the digital economy: Increasing dependence on uncaptured GDP. Technol. Forecast. Soc. Change 137, 226–240. doi:10.1016/j.techfore.2018.07.053
Watanabe, C., Naveed, K., and Zhao, W. (2015). New paradigm of ICT productivity – increasing role of un-captured GDP and growing anger of consumers. Technol. Soc. 41, 21–44. doi:10.1016/j.techsoc.2014.10.006
Wu, H. T., Xue, Y., Hao, Y., and Ren, S. Y. (2021). How does internet development affect energy-saving and emission reduction? Evidence from China. Energy Econ. 103, 105577. doi:10.1016/j.eneco.2021.105577
Wu, J. X., and Guo, Z. Y. (2016). Convergence analysis of carbon emissions in China based on the continuous dynamic distribution method. Stat. Res. 1, 54–60. doi:10.19343/j.cnki.11-1302/c.2016.01.008
Xu, X. C., Ren, X., and Chang, Z. H. (2019). Big data and green development. China Ind. Econ. 4, 5–22. doi:10.19581/j.cnki.ciejournal.2019.04.001
Xue, Y., Tang, C., Wu, H. T., Liu, J. M., and Hao, Y. (2022). The emerging driving force of energy consumption in China: Does digital economy development matter. Energy Policy 165, 112997. doi:10.1016/j.enpol.2022.112997
Yabo, L., and Jie, C. (2022). Research on the export quality effect of digital economy. World Econ. Stud. 17, 32–134. doi:10.13516/j.cnki.wes.2022.03.006
Yang, X., Li, X. P., and Zhong, Chunping. (2019). Study on the evolutionary trend of industrial biased technological progress and influencing factors in China. Res. Quantitative Econ. Technol. Econ. 4, 101–119. doi:10.13653/j.cnki.jqte.2019.04.006
Yi, M., Liu, Y. F., Sheng, M. S., and Wen, L. (2022). Effects of digital economy on carbon emission reduction: New evidence from China. Energy Policy 171, 113271. doi:10.1016/j.enpol.2022.113271
Yi, M., Wang, Y., Sheng, M., Sharp, B., and Zhang, Y. (2020). Effects of heterogeneous technological progress on haze pollution: Evidence from China. Ecol. Econ. 169, 106533. doi:10.1016/j.ecolecon.2019.106533
Yuan, Y. J., and Xie, R. H. (2015). FDI, environmental regulations and green total factor productivity growth in Chinese industry: An empirical study based on the luenberger index. Int. Trade Issues 8, 84–93. doi:10.13510/j.cnki.jit.2015.08.009
Zhang, D., and Vigne, S. (2021). How does innovation efficiency contribute to green productivity? A financial constraint perspective. J. Clean. Prod. 280, 124000. doi:10.1016/j.jclepro.2020.124000
Zhang, J. N., Lyu, Y. W., Li, Y. T., and Geng, Y. (2022). Digital economy: An innovation driving factor for low-carbon development. Environ. Impact Assess. Rev. 96, 106821. doi:10.1016/j.eiar.2022.106821
Zhang, J., Wu, G. Y., and Zhang, J. P. (2004). Interprovincial physical capital stock estimation in China: 1952-2000. Econ. Res. 10, 35–44.
Zhang, W., Liu, X. M., Wang, D., and Zhou, J. P. (2022). Digital economy and carbon emission performance: Evidence at China's city level. Energy Policy 165, 112927. doi:10.1016/j.enpol.2022.112927
Zhao, J., Shahbaz, M., Dong, X., and Dong, K. (2021). How does financial risk affect global CO2 emissions? The role of technological innovation. Technol. Forecast. Soc. Change 168, 120751. doi:10.1016/j.techfore.2021.120751
Keywords: digital economy, green total factor productivity, innovation, industrial structure, energy conservation
Citation: Xiao Y, Wu S, Liu ZQ and Lin HJ (2023) Digital economy and green development: Empirical evidence from China’s cities. Front. Environ. Sci. 11:1124680. doi: 10.3389/fenvs.2023.1124680
Received: 15 December 2022; Accepted: 30 January 2023;
Published: 09 February 2023.
Edited by:
Evgeny Kuzmin, Ural Branch of the Russian Academy of Sciences, RussiaReviewed by:
Luigi Aldieri, University of Salerno, ItalyNatalia Dneprovskaya, National Research University Higher School of Economics, Russia
Liudmila Kabir, Financial Research Institute of the Ministry of Finance of the Russian Federation, Russia
Pekka Neittaanmäki, University of Jyväskylä, Finland
Copyright © 2023 Xiao, Wu, Liu and Lin. This is an open-access article distributed under the terms of the Creative Commons Attribution License (CC BY). The use, distribution or reproduction in other forums is permitted, provided the original author(s) and the copyright owner(s) are credited and that the original publication in this journal is cited, in accordance with accepted academic practice. No use, distribution or reproduction is permitted which does not comply with these terms.
*Correspondence: Yue Xiao, eHlfZWNvbm9teUAxNjMuY29t; Zai Qi Liu, bHpxMTkxMUAxMjYuY29t