Application of X-ray computed tomography in soil and plant -a review
- 1ICAR- Indian Agricultural Research Institute, New Delhi, India
- 2School of Life and Environmental Sciences, University of Sydney, Camperdown, NSW, Australia
- 3Department of Agriculture and Fisheries, Queensland Government, Toowoomba, QLD, Australia
X-ray computed tomography (X-ray CT) is a non-destructive method of soil analysis which can provide three-dimensional (3D) view, quantitative information of the internal organization of the soil. In this paper, we discuss the potential application of X-ray CT in characterization of soil properties like porosity and pore size distribution (PSD), root architecture, soil phase classification, water and solute transport in soil, and highlight the research during last 10–15 years. Here, we review the recent development of X-ray CT in soil science, use of artificial intelligence and machine learning in image analysis, point out the major challenges associated with its use, discuss few improvements to overcome these difficulties and elaborate the possible future technological developments for non-invasive/destructive soil characterization by integrating X-ray CT with recently available complementary techniques.
1 Introduction
The organization of soil elements is vital to understand the processes that regulate soil functions and ecosystem services (Helliwell et al., 2013). The varied and dynamic nature of soil mineral constituents, organic material, pore network, and biodiversity across scales (micro to field scale) make soil research challenging, and therefore, the soil is frequently designated as ‘the most complex biomaterial on the planet’ (Young and Crawford, 2004). The study of the huge variability of soil attributes with an acceptable spatial resolution is costly and time-consuming (Fathizad et al., 2020; Kamamia et al., 2021). However, the measurements of some soil parameters such as texture, soil aggregate stability, hydraulic conductivity (HC) and field capacity moisture content (SWCFC), soil bulk density (BD), porosity and pore size distribution (PSD), and organic carbon (OC) involves cumbersome processes like the collection of disturbed soil samples from the field, drying, grinding, and sieving before time-taking laboratory analysis. The textural analysis involves more than 4–8 h of sedimentation study of sand, silt, and clay by hydrometer or pipette (Gee and Bauder, 1986). At the same time, the disturbed samples do not retain the in-situ properties of soil. Though a laser diffraction particle size analyzer can provide soil textural information, it is expensive and involves sampling errors, especially with finer elements (Di Stefano et al., 2010; Fisher et al., 2017).
Soil BD can be measured by direct and indirect methods. The direct method includes core, clod and excavation techniques, whereas, the indirect method is radiation and regression techniques. The core method has maximum use throughout the world but it is time taking, problematic to collect samples from several depths, also the size of the core auger used, operator knowledge, soil depth, and in-situ soil moisture percentage meaningfully affect its precision (Al-Shammary et al., 2018). The clod method is suitable for heavy textured soil but its accuracy depends on equipment calibration, drying time, and operator skill (Page-Dumroese et al., 1999). The excavation technique is useful for forest soil and is greatly dependent on soil texture and the types of analysis used (Al-Shammary et al., 2018). Indirect methods are more accurate but involve more expertise like the accuracy of gamma radiation depends on soil depths whereas the regression approach is economical but it gives an indirect measurement of BD. The accuracy of indirect methods depends on high-quality soil texture and OC data and location and climatic characteristics (Pramanik and Aggarwal, 2013). Ex-situ measurement of OC involves conventional field collection of soil samples and subsequent chemical degradation by wet and dry combustion approach which is time-taking and naturally destructive (Bagherifam et al., 2021), and sampling and analysis errors may change the results. In-situ measurement of OC includes infrared reflectance spectroscopy, laser-induced breakdown spectroscopy, inelastic neutron scattering, and remote sensing which are considered more effective particularly for large-scale study, because speed and high accuracy may reduce the cost involved in traditional sample collection and analysis (Bagherifam et al., 2021). Though an automated carbon analyzer is used as the usual method for the ex-situ OC determination, the partial alteration of carbonate to CO2 under the ignition conditions might occur in extremely calcareous soils which is challenging (Bagherifam et al., 2021).
The most commonly used traditional approaches for soil moisture measurements are the gravimetric method, neutron moisture meter method, time-domain reflectometry, tensiometer, and gypsum block method. The gravimetric method is a widely used method of soil moisture measurements and it is quite accurate and simple but time-consuming and labour-intensive. Neutron probe moisture meters (Holmes, 1956) detect soil moisture using radioactive elements where the source (Am) emits fast neutrons and the detector (Br) detects the neutrons that come back after a collision with a hydrogen atom, the number of the thermal neutron is directly proportional to soil moisture. This method is fast and reliable but expensive, and equipped with radioactive elements so requires special care to handle however, it is semi-quantitative and requires calibration to understand the local water potential and also requires a borehole to be installed in the soil for measurement (Sharma et al., 2018). The time-domain reflectometry (Topp and Davis, 1985) system estimates the travel time of the electronic pulse passed in the waveguides (two or more probes), which are bounded by the soil. The soil water content is determined by considering the travel time which is influenced by the dielectric constant. This method gives fast and accurate measurements but is expensive (Sulthoni and Rizqulloh, 2019). Gypsum block (Bouyoucos and Mick, 1940) consists of calcium sulfate dehydrates. It is capable of absorbing water and its ability to come into equilibrium with the soil/medium makes it a suitable soil moisture sensor (Mukhlisin et al., 2021). The electric resistance between the electrodes is the measure of soil moisture of the soil. It is inexpensive and reliable, but it is less responsive (Singh et al., 2019). Conventionally, the soil–water retention curve (Niu et al., 2020) and mercury intrusion methods (Sun and Cui, 2020) are used extensively throughout the world for the study of the macroporosity and pore size distribution (PSD) of soils. Yet, these methods do not generate information on the distribution of pores greater than 300 μm in diameter and also these methods are limited to the applied matric potentials. Classical micromorphology can produce 2D true-scale images of the porosity of undisturbed soils but a recurrent dearth of synchronization of both sampling and analyses amongst the numerous experts working on the same site at different scales and conflicting results may be difficult to resolve and less progress has been made in image analysis techniques (Sadiq et al., 2020). Different methods are used to measure soil hydraulic conductivity (HC) (K-value) in the field and laboratory. The most commonly used approaches in the laboratory are constant head and falling head methods. In the field, HC is mostly determined by the infiltration method. Field methods are generally more costly than laboratory methods and the accuracy of field methods depends on precise information on aquifer geometry and hydraulic boundaries (Musa and Gupa, 2019; Ghosh et al., 2020b). The correlation method is also used for measuring HC where a predetermined relationship between soil properties (e.g., grain size distribution, texture, etc.) and K-value are used. The correlation method is faster than the direct method but there is a chance of random errors (Musa and Gupa, 2019).
Despite a number of improvements, the direct-measurements of soil structural stability, hydraulic properties and field capacity, OC, PSD, and BD have only marginally advanced over the last decades and also, most of the direct methods are difficult to use, labor-intensive, and time-consuming (Bhattacharya et al., 2021). Hence, there is a requirement to develop a fast, sophisticated and accurate technology for estimating soil parameters.
The radon transform mathematical theory is where the idea for X-ray computed tomography (CT) originated, at least as far back as 1917 (Radon, 1917). William H. Oldendorf received a U.S. patent in 1963 for a “radiant energy apparatus for investigating selected areas of interior objects obscured by dense material” (Oldendorf, 1978). Initially, X-ray CT was used as a diagnostic tool in medical science to detect soft tissue and bone visualization (Hounsfield, 1973), because of its ability to generate non-destructive and high-contrast cross-sectional images within a short time. In 1971, Sir Godfrey Hounsfield invented a scanner and successfully used it in the first clinical CT scan (Richmond, 2004). After that, various disciplines began to use it within a decade of its introduction; one of them is soil science. Petrovic et al. (1982) was the pioneer in to the use of X-ray CT in geological study particularly to evaluate the relationship between soil BD and X-ray attenuation coefficient, assuming consistent soil mineralogy, where they found the linear relation between BD and the X-ray attenuation coefficient which showed the technological potential for exploring the soil physical environment. This study catalyzed the interest among scientists to carry out the study of different soil physical, chemical, or biological properties with the help of X-ray CT. Hainsworth and Aylmore, (1983) investigated the root-related hydrology and water absorption attributes at a spatial resolution of 2 × 2 × 5 mm. Crestana et al. (1985) showed the linear relationship between X-ray attenuation and soil water content, allowing them to obtain reproducible and quantitative data on 3D space and time of water in the soil. These pioneering works demanded imaging across multiple scales and undoubtedly confirmed the applicability of X-ray CT for undisturbed soil systems studies. The instrument uses the X-ray region of the electromagnetic spectrum of wavelength 0.01–10 nm (Figure 1). The short wavelength of the X-ray allows it to penetrate the target sample, allowing physical characterization through attenuation measurements.
The main advantages over conventional techniques are that it provides a three-dimensional (3D) view, quantitative information about the sample’s physical environment, and it is non-destructive. So, the recent advancement in X-ray CT imaging techniques is becoming popular among the researchers for detailed study of pore-scale dynamic processes in soil. With X-ray CT with appropriate scanning parameters, it is possible to visualise and quantify the spatial distribution of particulate organic matter (POM) fractions., organization of soil aggregate, development of preferential flow paths, soil-root interaction, root or organic matter decomposition (by detecting temporal variation in the volume of decomposing root or organic matter). The correlative imaging combing X-ray CT and microbiological imaging techniques can be powerful to understand the relationship between soil habitat structure and microbial function (Charlotte et al., 2022). Correlative imaging like combing hyperspectral/FTIR/Raman Spectroscopy/XPS/XRF/XRD with CT images can be very useful to understand the microbial niche, organic matter stabilisation sites, relationship with mineralogical, and chemical composition, etc. Pore-scale modeling of hydraulic properties, diffusion of nutrients in the soil (both 2D and 3D modeling), and microbial growth are some other applications for CT images. The use of CT for 3D phenotyping of plant shoot/growth patterns is becoming much more common also investigating xylem cavitation, panicle/seed internal structure, and other issues. This review attempted to concentrate on studies that used X-ray CT to visualise and quantify soil physical features, root phenology, habitat architecture, and so on. We have also discussed the limitation in each case and how to overcome the challenges. We have explored further technological advancement which will significantly improve the spatio-temporal studies of pore-scale- processes in soils.
2 Theory of X-Ray computed tomography
2.1 X-ray tube
X-ray generation occurs in x-ray in a vacuum tube when accelerated electrons from a cathode filament through the vacuum bombard an anode target material (e.g., tungsten). The applied voltage of the X-ray tube determines the kinetic energy of electrons through the vacuum. The X-ray radiation or photons are generated by two processes (Taina et al., 2008). When the incident electron strikes with the atomic electron clouds of the target, it results in the deflection and deacceleration of incident electrons and subsequent conversion of kinetic energy to electromagnetic radiation. This process generates a continuous spectrum; the highest energy of the photon will be achieved when the total kinetic energy of an electron is converted (Jia et al., 2023). In the second process, when the electron strikes the target, the atoms become excited because of the movement of orbital electrons to the higher energy level. When the excited atom comes back to the ground state, distinctive X-ray spectra are released where the total energy is equal to the difference between the energy of the excited and ground state. The distinctive photon-energy amount is subject to the atomic configuration of the target material (Jia et al., 2023). The produced X-ray spectrum is then passed through a metal filter (e.g., Al, Cu) to remove the low-energy X-ray that is not important for imaging. The typical configuration of an X-ray tube is depicted in Figure 2.
The typical X-ray CT consists of generally 3 parts, namely-the X-ray source, sample manipulation stage and detector (Withers et al., 2021). There are mainly 2 types of X-ray instruments, one is for industrial use and the other one is for medical use. The principle behind the two types of system is the same but the basic difference is that in the case of the industrial CT system, the sample is rotated in front of the X-ray source (Figure 3) whereas, in the case of the medical CT system, both the source and detector are rotated around a static object (Helliwell et al., 2013).
2.2 X-ray attenuation
When the X-ray photon passes through the sample, it gets attenuated, or the intensity of the X-ray beam gets reduced. The decrease in radiation intensity is exponentially related to the distance traveled by the radiation. The attenuation of a monochromatic X-ray radiation beam is expressed by Beer–Lambert’s law (Withers et al., 2021). When an x-ray beam passes through a sample of the fixed thickness (x), the transmitted beam intensity (I) and the incident beam intensity (I0) assuming a constant density of the attenuating medium are related as follows (Eq. (1)):
Where ρ is the density of material; μ is the attenuation coefficient per unit mass. The distribution of the attenuation coefficients at discrete points within a material can be determined. Attenuation coefficients are dependent on the incident X-ray energy, thickness, and electron density of the absorbing materials.
2.3 Reconstruction of tomograms
X-ray CT can be applied for the visualization of the internal makeup of an object in 2-D and 3-D views, depending upon the attenuation of the X-ray. In a typical CT scan, a series of radiograph images are captured from the sample, at incremental angular sites, typically over 360o (Helliwell et al., 2013). The linear X-ray attenuation coefficient values obtained from the array of radiographic images is integrated and it forms the basis of tomographic reconstruction. Normally, mathematical filtered back-projection algorithms are used. (Figure 4).
Using a set of projections, the computational framework reconstructs the spatial distribution of the attenuation coefficient slice by slice to create a greyscale image of the object. Since similar quality images can be reconstructed at a lower dosage, image reconstructions that enhance image quality can cause decreased radiation exposure. The Radon transform provides a mathematical explanation of the link between the slices and projections. The transform is the foundation for two major groups of reconstruction algorithms, namely analytical and iterative reconstruction (IR). In commercial CT scanners, filtered back projection (FBP) is the most extensively used analytical reconstruction technique, which employs a 1D filter on the projection data before back projecting (2D/3D) the data onto the image space (Yu et al., 2016). The computational effectiveness and numerical stability of FBP-type methods are the fundamental reasons for the wide acceptance of CT scanners. Unlike analytical reconstruction techniques, image reconstruction using IR is accomplished by iteratively optimising an objective function, which characteristically comprises an edge-preserving regularisation term and a data fidelity term (Thibault et al., 2007). Iterations of forward and back-projection between image space and projection space are used in the optimization process in IR. Images via IR could look different from those utilising FBP reconstruction due to the inherent differences in data handling between FBP and iterative reconstruction (such as noise texture). More crucially, because of the non-linear regularisation term and other factors during the optimization process, the spatial resolution in a local region of IR-reconstructed images is highly reliant on the contrast and noise of the surrounding structures (Fessler and Rogers, 1996). Recently, several advanced algorithms are being used for X-ray CT image reconstruction like sparse-view statistical image reconstruction (Mahmoudi et al., 2019), deep learning (Wang et al., 2020), CNN-based projected gradient descent (Gupta et al., 2018), super-resolution reconstruction techniques (Tan et al., 2021), etc. The mathematical algorithms help to generate the cross-sectional 2D image slices from the radiographic projections based on the X-ray attenuation values. Each non-invasive tomographic image stack (i.e., image volume) consists of discrete voxels (the 3D equivalent of a pixel), which represent the resolution of the image stack.
2.4 Simple guidelines for using X-ray microtomography for soil-plant research
X-ray CT is an influential technique to visualise and quantify the architecture of soil particles, pores, root systems, or any other object of interest given that the object can sufficiently attenuate X-rays. This technique is increasingly adopted in soil, plant, and ecological sciences. The greatest opportunity it holds is the non-invasive visualization of the internal organisation of an opaque substance like soil. Looking through the soil also provides an opportunity to visualise the porous structure through which root grows, fluid flows and microbes thrive. Applications of X-ray microtomography are discussed in the following sections. Here some of the practical aspects of using X-ray microtomography is discussed to consider before designing research on quantitative analysis of the soil-plant system. Application of X-ray CT in different areas of soil science is summarised in Table 1.
o First and foremost is to consider whether the object of interest is visible under X-ray. Obviously, soil particles, pores, root systems, any other special features like disturbance caused by soil fauna, undecomposed or partially decomposed organic matter, and biochar can effectively be analysed using X-ray microtomography. The spatial and temporal distribution of water in a soil or glass beads can also be visualised using suitable X-ray parameters, contrast agent, and experimental setups.
o Appropriate X-ray parameters are essential for acquiring high quality images. The most important parameters are energy (KeV), current (µA) and resolution of scanning. Both X-ray energy and current determine the quality and contrast of the acquired images depending on the nature of the sample. Images with good contrast between different objects in the sample and the detector background are easier to segment into the objects of interest. Choosing appropriate voxel resolution determines the scale of observation, higher the voxel resolution, closer the sample to the X-ray tube and thus smaller the field of view. For instance, if the object of interest is 50 µm soil pores then the voxel resolution should be at least 25 µm if the spatial resolution is 2 times of the voxel resolution. Depending on the X-ray microtomography system spatial resolution can vary between 2 and 5 times of the voxel resolution. The voxel resolution is dependent on the distances between the X-ray source and the sample and the size of the detector and the sample. For example, if the diameter of the sample is 50 mm and the detector is 1,000 × 1,000 pixels, the highest voxel resolution can be attained is ∼60 µm. The number of projections, image averaging to minimise the noise, scan time and use of filters need to be considered before setting up the X-ray parameters.
o When image acquisition is done the images are reconstructed into 3D volume or image stacks using proprietary or bespoke reconstruction software. The region of reconstruction, beam hardening correction, geometric alignments, ring artefact correction, filtering and volume stitching need to be performed during image reconstruction. It is important to mention here, any little movement (other than 360° rotation) will create blurry 3D image volume, so care should be taken during image acquisition.
o Image analysis is the next step to extract quantitative information from the 3D image stack. The 3D stacks are usually TIFF, BMP or Net CDF image slices depending on X-ray tomography system. The goal is to segment image into one or more features. In a soil-plant system depending on the research objectives, the images are segmented into roots, pores, and solids. The simplest way to view and analyse the images is to upload the image stack in open access software ImageJ or Fiji (an ImageJ distribution for biologists) (Schindelin et al., 2012). ImageJ provides image processing tools including a wide array of plug-ins. ImageJ is also very useful for the development of macros and plug-ins to execute a specific task or series of image processing operations in batch mode. There is other image analysis software like Matlab®, Python® and Python Image analysis libraries, Avizo®, VG Studio Max®, Simpleware ScanIP®, PerGeos®, Dragonfly® that can also be used.
o Several steps are meticulously followed for quantitative image analysis. The steps generally are i) equalise image histogram across the image slices of a stack, ii) apply filters (median, mean, Gaussian etc.), iii) thresholding of features of interest, iv) quantitative analysis, v) 3D image rendering. However, the steps can be varied depending on the nature and quality of the 3D stacks. The algorithms available for image segmentation range from manual thresholding to machine learning and the choice of specific thresholding technique obviously depends on the research questions.
o The pore geometry of a soil-plant system can be quantitatively analysed by determining porosity, pore size distribution, pore fractal dimension, pore connectivity, pore throat diameter, pore diameter, anisotropy, spatial statistics, pore network tortuosity and hydraulic permeability. ImageJ, ImageJ plug-in (SCAMP (Rabbi et al., 2015), BoneJ (Domander et al., 2021), MorphoLibJ (Legland et al., 2016), Quantim4 (https://www.ufz.de/index.php?en=39198) and Dragonfly® are some useful applications for quantitative analysis of soil pore geometry. Total root length, number and length of lateral roots, root angle, average diameter and convex hull are important traits to describe the 3D architecture of the plant root system. The ImageJ plug-in Root1 (Flavel et al., 2017) and Rootine (Gao et al., 2019) can be used to extract and analyse 3D root architecture. The pore network modelling is a very powerful way to quantify the fluid flow properties of the extracted pores. Some open access tools like OpenPNM (Gostick et al., 2016) and OpenLB (Krause et al., 2021) are useful for pore network modelling.
3 Characterization of soil physical properties
3.1 Porosity and pore size distribution
The pore structure and distribution regulate the distribution of micro-organisms, moisture, and air in the soil (Helliwell et al., 2013; Ghosh et al., 2020a) and thus have a potential role in controlling soil organic matter decomposition (Rabbi et al., 2016). The quantification of the internal soil structure is an important key for understanding different physico-chemical and biological processes occurring in the soil. Recently X-ray CT gives a novel way to study soil pore structure (Pires et al., 2020; Liu et al., 2021). The characterization of soil structure is significantly influenced by the representation of X-ray CT data as grayscale images and the appropriate choice of segmentation techniques to binarize it (Wang et al., 2011; Torre et al., 2020). The application of X-ray CT for the evaluation of soil porosity has become a research tool for the investigation of soil porosity and pore size distribution (Rab et al., 2014; Ghosh et al., 2022). Yang et al. (2018) used different soil amendments like straw mulch, superabsorbent polymer (SAP), and organic fertilizers for improving the soil structure and porosity. They used X-ray CT to determine the number, size, location, and morphology of soil pores. They reported that the combined application of amendments improved the soil pore structure more effectively as compared to individual applications. They also found that the application of both straw mulch and organic manure improved the soil porosity and soil structure more effectively as compared to other combinations of amendments. Pires et al. (2019) employed the X-ray CT to evaluate the effect of three different tillage systems (ZT-zero tillage; RT-reduced tillage and CT-conventional tillage) in the soil porous system of an Oxisol. They used the undisturbed core of 0–10 cm soil depth for scanning through X-ray CT. The results showed that the soil under ZT had the smallest porosity when compared with the other management practices. The largest porosity and the most connected pores were seen in conventionally tilled soil. Young & Ritz, (2000) stated in their review that macropore continuity is meaningfully improved in the soil profile of the ZT system. X-ray CT images of macropore network (>1 mm) of soil cores (10 cm diameter) collected from (a) ZT and (b) CT plots. Macropores continuity is significantly improved in the soil of the ZT plot.
It has long been understood how significant soil macropores are as preferred channels for the flow of chemicals, air, and water in various soils. However, it is difficult to quantify complicated macropore structures and how they relate to different soil types and land uses. Luo et al. (2010) quantified 3-D macropore networks in unbroken soil core under contrasting soil types and land uses by X-ray CT. For every soil typeand land use grouping, intact soil columns of 102 mm diameter and about 350 mm length were scanned at energy levels of 130 kV and 100 mA and voxel resolution was 0.234 mm × 0.234 mm × 2.000 mm. After image reconstruction, the features of the macropore networks, such as the incessant change in macroporosity along the depth, the distribution of macropore sizes, the density of the network, the length density, the surface area, the length distribution, the mean hydraulic radius, the tortuosity, the inclination angle, and connectivity, were quantified. This study offers an enhanced quantitative assessment of several soil macropore characteristics that have substantial consequences for field soil modeling of chemical transport and non-equilibrium flow prediction. A study was done by Beraldo et al. (2014) for the quantification of soil porosity using X-ray CT under CT, ZT, and native forest. In this study, they acquired the images by a system of X-ray CT (Model 1,172 of SkyScan), consisting of a microfocus X-rays tube with a 100 kV high voltage source, the detector made of a CCD camera of 10 MP connected to the computer for image acquisition. The images had a resolution of 34.81 µm and it enables the detection of microporosity having pore diameter >0.05 mm) and a narrow range of micropores with diameter <0.05 mm. As a result, they reported that there was a change in soil porosity under different management practices, and the difference was more significant in conventional and no-tillage compared to the area under forest. Hamamoto et al. (2016) used X-ray computed tomography for obtaining information regarding pore networks under different compaction levels for the column of sand and glass beads which represents different shapes and sizes of particles. Luo et al. (2008) quantified soil structure and preferential flow in intact soil of 10 cm diameter and 30 cm height using X-ray CT (OMNI-X model, BioImaging Research, Lincolnshire, IL). The CT system had a 225 kV microfocus x-ray generator and a 225-mm-diameter image intensifier. The maximum resolution of ∼5 μm was attained by the microfocus system and the sample was rotated 360° to obtain the polychromatic x-ray beam. Image analysis was done by AMIRA software (TGS Inc., San Diego, CA), and the data obtained in this experiment was analyzed using ImageJ (Rasband, 2002). Their results showed that different types of macropores having dissimilar morphologies (like earthworm burrows and root channels, and interaggregate macropores) and their diverse roles in the solute transport process were detected, which changed in different soil depths and horizons. They observed that few biogenetic macropores which are tremendously continuous are responsible for solute transport in the deeper soil layers. Luo et al. (2008) reported that data from real-time solute transport can generate voxel-based breakthrough curves which are useful in getting solute dispersion parameters of small areas, estimating water movement and solute transport in varied soils and it is helpful to test existing dual-permeability models.
3.2 Soil strength
The soil strength in terms of compaction is a serious threat to agriculture, especially in conventional agriculture. Traditionally soil strength can be determined by soil penetrometer or as indirect determination from bulk density but they are unable to recognise a spatial pattern of soil strength. X-ray CT is an effective tool to characterised soil compaction. Kim et al. (2010) used intact soil core to show how 3D macropore geometry is affected by soil compaction. By using X-ray CT, they reported that the total numbers of macro- and mesopore increased when the surface compaction reduced by 69% and 64%, respectively.
X-ray CT images showed a consistently decreasing continuity of macropores as soil BD increased. The average Euler number increased to 0.999 in 1.8 Mg m-3 soil BD from 0.677 in 1.3 Mg m-3 soil BD (Feng et al., 2020). They also reported a negative correlation between macropore throat number and thickness, which indicated the loss of pore connectivity with the increase in the soil compaction. Previously, X-ray CT was used for soil BD measurements where gray level values were calibrated by measuring the attenuation of penetrating X-ray CT (Pires et al., 2019). Crestana et al. (1985) developed a soil mini-scanner to determine X-ray attenuation coefficients for estimating soil BD in the field. Previous studies had mainly used medical X-ray CT when laboratory-based fine-resolution X-ray CT was not widely available. There are numerous studies, investigating soil BD using laboratory-based X-ray CT, while they have been emphasising measuring X-ray attenuation as a function of soil density (Anderson et al., 1988). This technology has a unique ability to measure local BD along the soil core, and also visual assessment of density variation is possible, which adds a novel dimension to measuring BD (Le Roux et al., 2019). Le Roux et al. (2019) acquired 2000 to 3,000 images while the sample was rotated at 360° to get a highly magnified image. These images were reconstructed by 3D model, using Graphics VG Studio Max 3.0 (Du Plessis et al., 2016) software, which provides total volume and internal features of the sample. To determine the soil BD, the instrument specification used for the field-moist and wax-coated oven-dry clay soil clods were scanned at 200 kV and 140 μΑ at 100, 50 and 25 μm resolution whereas the sandy soil core was scanned at 150 kV and 100 μΑ with 50 μm resolution. Elias et al. (2021) used X-ray CT in the determination of the BD of cracking soils, they scanned intact clods at 120kv energy level and the pixel densities determined ranged from −1,500 to 3,000 Hounsfield materials, the soil clods were scanned horizontally having 1 mm thickness and a slice distance of 2 mm. The BD was measured by the relation given by Rogasik et al. (1999).
3.3 Soil phase classification
Destructive methods and 2D images, together with thin sections and electron microscopy, are used conventionally to understand soil structure but they are unable to study the spatial arrangements of undisturbed soil (Young et al., 2001). X-ray CT has a great potential to detect spatial arrangement of different constituents of the soil sample. With technological advancement, it is now used to interpret the finer soil fraction than sand with a voxel size of <15 microns (Feeney et al., 2006; Wang et al., 2012). Further improvement in image resolution enables the detection of clay fraction, nutrient liberation, and stage of weathering, etc (Helliwell et al., 2013). More X-ray attenuation at smaller energy levels helps to discriminate different minerals of comparable mass (Taina et al., 2008). But it is a challenge to isolate or quantify the soil particles, water volume, and air-filled porosity. Rogasik et al. (1999) used a medical X-ray CT (Siemens Somatom Plus-CT scanner, Siemens, Erlangen, Germany) with 80 kV (190 mA; 760 mAs) and 120 kV (85 mA; 340 mAs) energy levels to compute water and air content, and phase composition of two silt loam soils of chernozem (FAO classification), coherent subsoil Ck horizons. The resolution of the reconstruction matrix was 512 by 512 pixels. The results suggested that in low resolution, the gravimetric soil water content was inversely related to soil BD and no definite relation was found between water content and BD in high resolution. However, voxels encompassing solid and air portion occur mostly in the broader dry BD values.
3.4 Water content and solute transport
Earlier fluid flow studies in the porous soil used to be done by mathematically solving Darcy’s law using the fundamental Navier-Stokes equation. X-ray CT has great potential to detect water content and its movement within the soil matrix (Schlüter et al., 2020). The ability of X-ray CT to reproduce 3D images allows for the identification of interconnected void spaces (Perret et al., 2000; Mooney, 2002; Wildenschild et al., 2005). With recent advancements in X-ray CT and robust modeling techniques, pore-scale fluid flow can now be easily modeled using the Navier-Stokes equation (Fourie et al., 2007). The model provides data on the soil’s isotropy, tortuosity, and dispersivity in all directions. Rezanezhad et al. (2009) carried out a study to examine how the flow of water through peat soil was affected as the size and shape of the pores changed. They used X-ray CT at 45 µm resolution to detect pore size and configuration under changing water regimes. The study suggested that HC of peat soils was greatly regulated by a pore shape coefficient which was generally supposed to contain pore characteristics like PSD, path tortuosity, roundness, and sphericity of pores. A pore channel model developed with different pore segments showed that flow in water varied with the pore shape in 2D images whereas tortuosity is prominent in 3D images (Choi et al., 2020). For the characterization of all the factors required for pore geometry, X-ray micro-CT equipment was used with a voxel size ranging from 3 to 10 μm and commercial Avizo software was used to visualize, and analyse the stacked image. Stokes and Brinkman equations were used to capture the relation of flow pattern with apparent and indistinct pore geometry. There was a good agreement between the predicted permeability gained from the pore channel model and experimentally observed permeability. They reported that the pore channel model made the complex pore geometry study simpler and very much suitable for fluid flow study in water at the pore level. Grayling et al. (2018) used the Phoenix Nanotom® X-ray μCT scanner (GE Measurement and Control Solutions, Wunstorf, Germany) for tracing the movement of a particle in the soil. They used decabromodiphenyl ether (DBDE) as a proxy solute material of 1–2 μm sized particles. Scanning of discrete regions of interest (ROI) was done at 120 kV and 90 μA using a 0.1 mm copper filter. After reconstruction, Volume Graphics (VG) StudioMAX® 2.0 software was used to analyse the image (10 mm × 23 mm diameter). DBDE concentration in the leachate accumulated at the bottom of each soil column was determined after every scan using gas chromatography (GC) and a chemical breakthrough curve was made. The findings showed that at the beginning, the movement of the tracer material was very fast in the upper soil layer and a robust relationship between DBDE concentration and X-ray attenuation (R2 = 0.98) was obtained which confirmed that with an increase in concentration, the colour intensity of the tracer material in each tube became lighter. Clausnitzer and Hopmans (2000) and Anderson et al. (2003) used iodide (NaI or KI) or bromide (CaBr2) tracers for the study of solute transport in the soil materials due to their high contrasting X-ray attenuation. They proposed that the porosity can be estimated from CT-derived solute breakthrough curve. The CT-derived porosity showed a good agreement with laboratory-measured porosity by the differences varied from −4.5% to 4.2%. Low-resolution X-ray CT can quantify soil moisture content in the root rhizosphere and bulk soil; however, a linear calibration between X-ray attenuation and water content is required. (Hamza et al., 2001). As a single wavelength of X-ray CT will not be able to readily make a differentiation between changes in BD and water content, a uniform BD could be assumed (Moradi et al., 2011). Daly et al. (2015) utilized X-ray CT to visualize the soil water in-situ in 3D at successive increments in matric suction in bulk and rhizosphere soil, the study provides a complete picture of unsaturated HC and hydraulic properties with the help of numerical modeling. Similarly, Tracy et al. (2015) used high-contrast X-ray CT images to compute the water distribution in soil systems under sequential reductive drying in contrasting soil textures.
Combined application of the Lattice Boltzmann (LB) model and X-ray CT has been reported to simulate pore-scale water flow and chemical transport in a porous medium (Zhang et al., 2016). Pores can be quantified from X-ray CT data that are larger than the resolution of the data collected. Due to this limitation, X-ray CT obtained solid phase may be a grey phase in realism and contain considerably associated pores responsible for fluids and solute transport in soil. Single-relaxation time approach is used in the LB model and a modified partial bounce-back scheme is used to assess the resistance imposed by the permeable solid phase to chemical transport (Wang and Zhu, 2018). Zhang et al. (2016) applied the LB model to X-ray CT acquired images having 30 μm resolution to study how solute transport through the solid phase affects the average solute transport behaviour. They showed that the pore geometry has a pivotal role in water flow and solute transport in vadose zones of the soil and the pore geometry is extremely inconstant in cultivated lands where different management practices such as crop rotation, tillage and trafficking, and soil modifications, have been done. Filipović et al. (2020) combinedly applied laboratory and numerical methods with dye staining and X-ray CT to evaluate the porous system and preferential flow paths using an intact soil core of a vineyard. They analysed 600 images with Image J software and found that the largest pore diameter ranged from 5.2 to 10.6 mm, indicating that the pore source is earthworm burrows and plant roots known as biopores contributing to the preferential flow. Müller et al. (2018) characterized the hydraulic characteristics of macropores from the X-ray CT images captured from three horizons of an Andosol and a Gleysol. For the Andosol, images of soil cores were taken using a 160 kV X-ray source at 150 μA and 1,250 angular projections through isotropic voxel size of 0.0734 mm in each scan. Results indicated that imaged-limited microporosity could best predict Kunsat and a less total macroporosity along with high macropore density, showed the richness of smaller macropores, important to homogeneous matrix flux.
3.5 Organic components in soil
The characterization of organic constituents like decomposing crop residue and plant root identification is a more challenging task than characterisation of soil void space. As a result, little research has been conducted in this area, with the majority of studies focusing on characterising plant roots. (Mooney et al., 2006; Tracy et al., 2012b). Quinton et al. (2009) used X-ray CT (GE Medical CT, model MS8X-130) in highly organic soil like peat and found that a single large pore represented more than 99 percent of the active porosity, whereas 2D analysis revealed a relatively steady conversion of small pores to large ones. They faced a challenge to discriminate organic matter and soil water because being more porous, organic matter produced a similar kind of attenuation of X-ray when the soil was saturated with water. To avoid this problem, negligible values of the volumetric linear attenuation coefficient (LAC) of organic matter was taken for nearly saturated soil samples (0 to −40 cm). Lately, Elyeznasni et al. (2012) was able to detect coarse-sized organic matter (>40 µm) content from X-ray CT technique, and the grey level values of the scanned image, also found that 3D segmented images are well associated well measured porosity. Chenu and Plante (2006) used transmission electron microscopy (TEM), and Kinyangi et al. (2006), used synchrotron-based scanning transmission X-ray microscopy (SEM), reported that small organic particles are materially obstructed in micropores, emphasizing the role of microscale soil structures. Peth et al. (2014) used a synchrotron-based -µCT scanning with a voxel resolution of 9.77 µm and osmium as the staining agent to detect the presence of SOM soil aggregates under in-situ conditions. They showed that the relationship between osmium signal intensity and organic matter concentration is strong, but the relationship is not so variable among the samples and only the relative concentration of SOM may be measured. Their findings can be useful in geometry-based mechanistic modeling approaches to systematically study PSD on carbon decomposition (Peth et al., 2014) as such models need explicitly spatial explicit inputs stating from pore architecture geometry, and the spatial microbial distribution and SOM as decomposable substrates. A study of soil microbial functioning through X-ray CT analysis would be a commanding tool in understanding the role of soil pore geometry on SOM degradation as the tomographic technique is not harmful to the microbial community (Bouckaert et al., 2013). However, many researchers (De Gryze et al., 2006; Kaestner et al., 2006) reported that phase separation/segmentation became very difficult as the X-ray attenuation coefficients of SOM become in-between air/water-filled pores and mineral matrix. Consequently, in-situ measurement of low concentrations of SOM in cultivable mineral soils (i.e., 5 % wt. basis) is restricted due to the weak X-ray attenuation coefficient of SOM as compared to the other complexes and SOM has a very near association with the surfaces of clay mineral.
3.6 Rhizospheric process
The rhizosphere is the region/volume of soil which is influenced by a plant root (Rabbi et al., 2016) and very vital for plant growth and nutrient uptake. Compared to bulk soil, rhizosphere soil has different physical and chemical characteristics. Chemical alterations have not, however, been thoroughly measured in-situ in pore-scale level aside from root-induced physical changes. The different hydraulic properties of soil are highly influenced by soil pore geometry and its modification (i.e., alteration in porosity and diameters of the pore) root-microbe interactions and rhizodeposition (i.e., mucilage, exudates) at root rhizosphere (Mendes et al., 2013). Most commonly used methods to characterise the soil biotic properties like plant roots, movement of water to the roots and soil micro or macro fauna and flora, are destructive in nature, but X-ray CT has remarkably capable to characterize these biological characteristics in opaque and composite medium (Gregory et al., 2003; Young and Crawford, 2004). Van Veelen et al. (2020) wanted to understand the chemical changes brought about by plant roots, so they combine structural data formerly gained using synchrotron X-ray CT with synchrotron X-ray fluorescence microscopy (SR-XRF) and X-ray absorption near-edge structure (XANES). To directly identify different elements in biological and geological samples, XANES in conjunction with SR-XRF mapping has proven to be an effective non-destructive technique. This combined approach can be distinguished into 3 zones: the close rhizosphere with improved porosity, the compaction zone, and bulk soil. The primary processes, like reduction, microbial alteration, and surface-moderated reactions occurs in the first two zones. This zone also has substantially greater rates of species interactions and transformations. Helliwell et al. (2017) used high-resolution X-ray CT to investigate the influence of root growth on soil structural alteration at scales pertinent to discrete micropores and aggregates. Using a Phoenix Nanotom 180NF X-ray micro-CT scanner (GE Sensing and Inspection Technologies, Wunstorf, Germany), all samples were scanned, with X-ray energy of 115 kV, 110 µA current, and 750 µs exposure time resultant imaged voxel size of 12 µm. After tomographic reconstruction, all images were cropped 3 mm from the column border to prevent any possible edge effects and then processed using the VG StudioMax® 2.1 software. They reported that soil porosity around the roots in the rhizosphere initially rose in contrasting soils, nevertheless reduced in sandy loam soil while improved in clay loam soil. With the help of X-ray CT, it was possible to determine the precise changes in the soil structure caused by roots both close to and at some distances from the root-soil boundary. Helliwell et al. (2019) spatially quantified the variations in soil texture and compaction caused by three commonly occurring but different plant species (like pea (Cicer arietinum L), tomato (Solanum lycopersicum), and wheat (Triticum aestivum)). Initial scans of all samples were performed using a Phoenix Nanotom 180NF X-ray micro-CT scanner (GE Sensing and Inspection Technologies, Wunstorf, Germany). The source had a 3 m focused point, and since the sample’s centre was 5.4 cm from the X-ray source, the imaged voxel size was 12 μm. Utilizing the program VG StudioMax® 2.2, images were processed, and to reduce noise and preserve the structural boundaries, a median filter with a radius of 3 pixels was used before segmenting the soil, root, and pore phases. Plant species significantly impacted the zone of impact of the root (i.e., the spatial grade of any variation in porosity away from the bulk soil) in the order of wheat (Triticum aestivum) > pea (Cicer arietinum L) > tomato (Solanum lycopersicum) (average of 694.7,483.9, and 21.2 mm3, respectively). The use of X-ray CT also provides the means to immediately apply numerical models describing the diffusion of nutrients to the observed geometries, in addition, to direct in-situ visualization of plant-soil interaction (Daly et al., 2015; Tracy et al., 2015). Daly et al. (2016) used X-ray CT to understand how root hairs contribute to nutrient uptake at various soil water contents, to construct an image-based modeling approach that applied to root hairs surrounded by a vast or infinite amount of soil, and to calculate the impacts of root-hair growth on nutrient uptake. G. Wang et al. (2020) analysed the effects of water and salinity stresses on maize (both separately and in combination) (Zea mays L.). To collect the aggregates clinging to the roots, they removed the roots and used X-ray CT to scan them. All aggregates’ porosity and PSD were determined from pore-scale simulations of water and solute movement in the void area, while their permeability and tortuosity were measured from segmented pictures. Aggregates from the unstressed control were used for comparisons. With an electron acceleration energy of 85 keV and 100 A current, the samples were scanned with a spatial resolution of 4 μm, requiring about 30 min for each sample. Chapman et al. (2020) investigated how auxin affects root growth in Arabidopsis thaliana and Medicago truncatula using X-ray CT in conjunction with minirhizotrons and molecular biology techniques. It was discovered that the RSA, auxin levels, and rootward auxin transport are all under the control of these two-plant species’ soil-grown receptor mutants. It is claimed that the CEP signaling and CEP receptor downstream targets can be altered to disturb RSA. To define the dynamics of nutrient cycling and exudate release, Van Veelen et al. (2020) developed data-intensive modeling of the rhizospheric process. In their research, X-ray CT was used to evaluate the soil pore geometry. while MRI was used to determine the water distribution and plant exudates. Finally, it is noteworthy that Ganther et al. (2020) evaluated the rhizosphere’s reaction to X-ray exposure to determine the effect that X-ray CT analysis itself has on biological systems. According to these authors, the rhizosphere’s bacterial microbiome and microbial growth characteristics were not affected by the X-ray CT study, and a 24-h delay was enough to undo any effects on the system that had been noticed. Hamza et al. (2001) observed that transpiration rates change with the variations in root diameters. They also reported that the high-resolution X-ray CT image can acquire spatial information on the microstructure of the root rhizospheres and which would be helpful for modeling water movement in association with root axial expansion. Aravena et al. (2011) reported that with a increase in soil compaction in the root rhizosphere, the contact between the soil and plant roots increased, which leads to more unsaturated hydraulic conductivity between aggregates, and finally it causes a net positive effect on the accessibility and transportation of water to the root. A new method was developed by Schindelin et al. (2012) for the quantification of root-to-soil contact using X-ray CT images, they segmented the plant root and soil separately and were able to obtain detailed information on plant roots and soils under water stress in loamy sand and vermiculite compound. Hu et al. (2019) used X-ray CT to quantify the soil macropores and root architecture, and revealed the larger degree of macroporosity under shrubs was due to the greater root network density.
3.7 Plant root study
Plant roots play a vital role in the growth and development of plants as roots can acquire and deliver water and nutrients to the above-ground plant (Perret et al., 2007). Previously, the commonly used destructive methods of root analysis such as root washing could not deliver thorough information on root architecture, as well as branching features and extension rate, which are integrally connected to environments within the soil profile (Tracy et al., 2010). Rhizotrons are one of the oldest non-destructive techniques to study root growth inside the soil. Rhizotrons covered underground walkways with clear windows on one or both sides are used to study the roots and their rhizosphere organisms detected at the soil-wall boundary. Rhizotrons typically are costly to build and work on (Taylor and Brar, 1991). With the advancement of technology, the X-ray CT allows us to envisage root system architecture (RSA) under the soil, non-destructively and in a 3D arrangement. Though, CT imaging, reconstruction procedures, and root separation from X-ray CT volumes, consume substantial time. Mooney et al. (2006) used X-ray CT for the first time to study lodging roots in the soil and they reported that the root lodging formed a substantial rise in porosity and size of the pore at the macroscale. Perret et al. (2007) & Hamza et al. (2007) have done remarkable contributions to root length imaging using X-ray CT. Perret et al. (2007) also developed a protocol for the quantification of chickpea (Cicer arietinum L) roots after 21 days of growth and they also determined some important parameters of roots like the number of lateral roots, their volume, length, wall area, tortuosity and orientation. They reported that X-ray CT analytically undervalued root length compared to the destructive method. Hamza et al. (2007) tried to investigate the effect of salt stress on root growth of lupin (Lupinus angustifolius L) and radish (Raphanus sativus L.) in −2.0 MPa to −0.10 MPa range by using X-ray CT. Han et al. (2009) studied the effect of scan-inducing bacterium density on potato root growth. Daly et al. (2015) did an image-based modeling study using X-ray CT to understand and visualize the spatial distribution of water around the roots, and also calculated the water flux into the root. They quantified the effect of root architecture and its geometry on water uptake by doing a comparison between image-based models and spatially average models. They revealed that the spatially averaged models did better when compared to the image-based models and the observed difference in uptake was <2% Different plant root studies using X-ray CT are listed in Table 2.
X-ray CT performs a leading role as a modern research tool to understand root architecture, interaction with soil, root development, etc. Using X-ray CT, it is possible to study the same distinct root growth over time and to observe root development procedures in pot study, also it offers the chance to discover the unchanged conformation of the 3D root architecture intermingling with a real field soil matrix. X-ray CT-based phenotyping techniques i.e., the characterization of realistic root structures of plant establishments in the field (Araus and Cairns, 2014) allow the spatio-temporal quantification of roots, aids to recognize the novel adaptive root architectural changes to the abiotic and biotic stresses (Mairhofer et al., 2017). Hu et al. (2019) reported more root development in porous soil and revealed a strong positive relationship between root volume, length, surface area and the solid surface/solid volume ratio. Gao et al. (2019) showed that X-ray CT could give a higher root length as compared to the root length gained by root washing along with 2D light scanning. Almost all land plants depend on their root systems to survive, and agricultural species must improve their ability to withstand abiotic stress, accumulate nutrients, and produce more, which shows the importance of root phenotyping. The classical destructive method of root phenotyping is where roots are removed from soil, washed, scanned then analysed using either commercially available (such as WinRHIZO, Regent Instruments Inc., Sainte-Foy, Quebec, Canada) or specially designed software (such as the so-called “shovelomics” approaches (Trachsel et al., 2011; Colombi et al., 2015). In contrast, X-ray CT is a more powerful tool for root phenotyping having several advantages, like, in pot studies, the development and growth of a single root can be tracked over time. Furthermore, the 3D root system architecture (RSA), in its unaltered configuration, can be investigated as it interacts with a real field soil matrix (Pfeifer et al., 2015). Using the variations in attenuation brought on by X-rays between different materials, X-ray CT can see roots in the soil. For X-ray CT imaging, densitometric slice pictures (reconstructions) are computed using signal data from multi-angle projections. These reconstructions are then stacked to create 3-D volumes (Heeraman et al., 1997). However, the techniques involved in X-ray CT scanning, reconstruction, and root separation from the voluminous images are time-consuming. Teramoto et al. (2020) have created a high-throughput process flow for the three-dimensional imagining of rice (Oryza sativa) RSA, which consists of radicle and crown roots. The procedure involves using calcined clay with even particle size to lessen the likelihood of seeing non-root sections, using a greater tube voltage and current in the X-ray CT scanning to boost root-to-soil distinction, and a 3-D median filter and edge detection algorithm were used to isolate root parts. To accomplish this procedure, 10 min of scanning and reconstruction, and 2 min of image processing were required, they named the technique for RSA visualisation as “RSAvis3D” and were assured enough that it would provide a possibly effective use for 3-D RSA phenotyping of different plant species. Herrero-Huerta et al. (2021) created a spatial-temporal root architecture modeling technique based on 4D information from X-ray CT. This innovative method takes into account the precision and robustness of the results as well as the cost-effective time required to process the data for high-throughput phenotyping. They used X-ray CT with an energy level of 200 kV and 3.5 mA with a pixel pitch of 90 μm, after the root reconstruction skeletonization was done for temporal analysis, and the root system architecture model was used for spatial analysis. Therefore, significant root architectural traits are mined from the model relying on the digital twin, which includes root elongation rate, number, length, growth angle, height, diameter, branching map, axial volume and lateral roots. Rogers et al. (2016) used an X-ray CT system to measure how rice’s RSA altered due to the changes in physical characteristics of the growth media, they discovered that the interactions between a plant’s genotype and its environment are what cause the RSA that is seen in that particular plant. The contrast among the roots, soil, and SOM is typically low, although that X-ray CT has been employed regularly to examine roots. Keyes et al. (2017) proposed using iodinated contrast media (ICM) to enhance phase contrast in root system studies, particularly for root vascular structures and rhizobial nodules.
3.8 Habitat architecture
Presently available X-ray CT are unable to detect microorganisms in the soil as X-ray has a high attenuating nature in the soil in contrast to minimum attenuation from microbes (O’Donnell et al., 2007). Despite the inability to envisage microbial communities directly, X-ray CT offers a chance to perceive their habitat and the structural dynamics within the soil. Different studies on soil microorganisms using X-ray CT are listed in Table 3. X-ray CT used combined with additional methods like thin sectioning and molecular biology methods offer very valuable information regarding habitat structure, resource flow, microbial populations (size and structure) and spatial distributions (Crawford et al., 2012). X-ray CT is a very sophisticated tool for qualitative and quantitative characterisation of invertebrate burrows in soil and also helps to visualize with time, ultimately providing useful information for soil physical properties. Capowiez et al. (2011) employed X-ray CT for the discrimination of burrows between anecic and endozoic species. They reported that the endozoic burrows were narrower and in more numbersand branched and less perpendicular. Once the earthworm density folded twice, the volume and length of the endozoic burrow system improved while no increase was detected for anecic earthworms. Ma et al. (2021) used X-ray CT to evaluate the influence of anecic earthworm Metaphire guillelmi on the properties of macropores and water content of the soil. They also reported that earthworms enhanced the soil macropores and microporosity, and the soil water content (SWC) linearly declined with growing earthworm density. Balseiro-Romero et al. (2020) employed X-ray CT to assess the earthworm behaviour in heterogeneously multi-polluted soils and they noticed that the earthworms sidestepped the polluted regions differently. Booth et al. (2020) applied X-ray CT to characterise and quantify wireworms, their tunnel arrangements and the rooting characteristics of two distinct crop types (barley-Hordeum vulgare L and maize-Zea mays L) in a pot experiment, samples were imaged at different times gap. They reported that the burrow systems were set up rapidly and persisted at a comparable volume. A significant difference was there in the burrow systems volume formed by wireworms amongst the two crop types which suggested the variations in wireworm behaviour caused by different crop types. Bailey et al. (2015) tried to determine the effect of mole cricket (Scapteriscus spp) tunnel on soil hydraulic conductivity, they revealed that the biopore formed by mole crickets led to the preferential flow hence, enhanced the soil water movements. Figure 5 illustrates some examples of X-ray CT images under different applications.
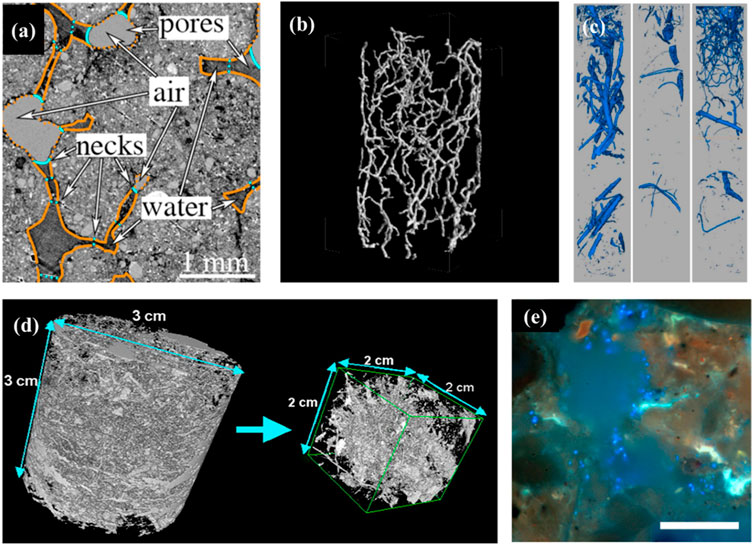
FIGURE 5. (A) Section from the x-ray computed tomography (X-ray CT) of an unsaturated porous soil aggregate, saturated and unsaturated pores and neck area (Xu and Louge, 2015); (B) Micro-computed tomography scanned reconstructed section of soil with roots (Flavel et al., 2012); (C) Three-dimensional visualization of root networks in the soil cores (Hu et al., 2019); (D) Characterization of soil aggregate using Synchrotron based x-ray CT (Wang et al., 2021); (D) DAPI-stained Pseudomonas fluorescens cells (bright blue colour) in thin sections of soil microcosms packed at 1.3 g cm−3 bulk density (Juyal et al., 2021).
4 Recent advancements in X-ray CT application
X-ray computed tomography is a well-established technique to characterize different aspects of soil and plant in 3D and spatially. Ferreira et al. (2022) intended to look at the impacts of soil surface liming on the intraaggregate pore structure of soil aggregates using synchrotron-based computed microtomography. The IMX Beamline of the Brazilian Synchrotron Light Source Facility (LNLS - CNPEM) was used for the scanning, with a pink beam, 550 μm Silicon filter, and 1,024 projections over an angle range of 180°, with a voxel size of 1.64 × 1.64×1.64. In the Rhenish lignite mining region, Lucas et al. (2019) examined the evolution of soil structure over a “space-for-time” chronosequence. X-ray CT images were used to characterize variables like connectedness (Euler number), while PSD was used to describe changes in the soil pore system. PSD and connectivity were computed on binary images. To calculate PSD, Fiji (version 1.4.6) used the maximum inscribed sphere method and the local thickness method. Diel et al. (2019) investigated the impact of desiccation fracture dynamics on pore space characteristics and soil structure turnover using X-ray CT during repetitive wetting-drying. X-ray tomographs were obtained using a Nikon Metrology X-TEk XCT 225 at 110 kV energy, 100 A beam current, 2,300 projections 2 projections per second. With a resolution of 8 μm and an 8-bit grayscale depth, the projections were converted into a 3D tomogram using a filtered back-projection procedure developed in the 3D CT-Pro software (Nikon Metrology). Soto-Gomez et al. (2020) shed light on the significance of soil macropore network characteristics for solute and colloidal transport as well as soil filtration. In intact columns taken from top soils with various tillage treatments, they used percolation theory and network analysis to the pore network recovered using X-ray CT (image-derived porosity). Additionally, using the free and open-source imaging programme ImageJ, they developed a method to extract the backbone, the portion of the percolation band that governs the straight flow amongst two borders under nearly saturated environments. Significant relationships were discovered between the volume of the backbone and the average stained surface, as well as between the dispersive transport of solute and colloidal tracers and the backbone parameters. Pires et al. (2020) used high resolution X-ray during the process of evaluating the water retention curve, and computed micro-tomography was used to assess changes in the soil pore architecture during the wetting-drying cycles. To increase our knowledge of in-situ root-soil interactions, non-invasive methods are crucial. High-resolution studies of these interactions have been successfully carried out using techniques like neutron computed tomography (NCT) (Mawodza et al., 2020). Their findings reveal that the NCT can detect the inherent variability of root architecture of wheat grown in aggregated sandy loam soil with significant levels of SOM. This is a change from the NCT’s predominant usage of sandy soils, however, it also presents new difficulties. Computed tomography (CT) images have been employed more frequently during the past 10 years to develop a thorough understanding of the microscale variables that govern soil dynamics. Pore network extraction using non-invasive X-ray microtomography (XMT) to characterize the PSD of aggregates and their possible alterations with continuing immersion in water. For the first time, Menon et al. (2020) showed utilizing imaging methods that stable aggregates from grassland and forest do not significantly modify their pore structure with extended immersion in water. To achieve the highest resolution for a specific aggregate size for this scanner, they used the Skyscan 1172 XMT scanner, which has effective pixel sizes of 10 m for L-size aggregates and 5 m for S- and M-size aggregates. In undisturbed soil columns, Koestel et al. (2020) evaluated representative elementary volumes (REVs) for the X-ray-derived porosity and pore-connectivity. Pore space characteristics are inextricably related to soil hydraulics and water retention. Average porosity was found to be scale-independent over a variety of observation scales, ranging from 15 to 65 mm. However, the existence of porosity REVs was still ambiguous because of a lack of statistical uniformity. For the pore-network connectivity, the scientists were unable to identify any potential REVs. Future investigations utilizing larger soil samples are advised, as well as a revaluation of the REV criteria needed in the context of continuous modeling. Koestel and Schlüter (2019) studied soil structural dynamics by time-lapse imaging of an in-growth core that was frequently reburied in garden soil under natural conditions. Using this concept, they have enlightened the sequential variations of pore space made by biotic and abiotic phenomena viz, soil settling, root growth, and/or faunal activity. An industrial X-ray scanner called the GE Phoenix v|tome|x 240 was used to capture X-ray pictures. For getting every 3-D image, 2000 projections with an isotropic resolution of 65 μm were obtained. They concentrated on tracking the changes in soil morphologic parameters known to be crucial for soil air and water flow additionally, they measured the soil matrix’s deformation. Piccoli et al. (2019) investigated the impact of scale effects on the relationship between soil structure and gas transport parameters. They demonstrated that larger, continuous biopores with more effective gas transport became more prevalent the larger the volume of intact samples. The pore network in the smallest sample size taken into consideration (4.7 cm3) was dominated by narrow, isolated air-filled holes that prevent gas diffusion at field capacity (−100 hPa). In the explored range of sample sizes and image resolutions, several microstructural features demonstrated signs of scale invariance, while emergent system behaviour in terms of gas movement does not. Therefore, while comparing gas transport parameters between studies, the sample size must be taken into consideration. So, new opportunities in soil and plant research are made possible by recent advancements in imaging hardware and modalities, image reconstruction, and analysis, improved resolution and contrast, mathematical modeling, and data sharing. Despite the significant reductions in X-ray CT acquisition time, the bulk and fixed design of the majority of X-ray CT scanners still needs to transport the target samples to X-ray laboratory facilities for imaging.
5 Machine learning and deep learning in X-ray CT image processing
CT imaging of soil samples (after reconstruction) produces grayscale images with a series of grey values corresponding to soil components with varying densities. The majority of current techniques for quantifying the soil pore system rely on binary images, with target objects or foreground pixels (e.g., pores) labeled as 1 and background pixels (e.g., soil) labeled as 0 (Ferreira et al., 2022). This means that before further quantification, the grayscale images must be segmented into binary images. However, due to the partial volume effect and noise in the images, the histogram of the grayscale image does not always show clear, distinct peaks, making image segmentation a difficult task. Many filtering techniques have been developed to smooth image noise and improve the contrast between the target and background, which, when used correctly, can greatly aid image segmentation. A plethora of image segmentation techniques has also been developed to address specific issues in a variety of disciplines. Machine learning (ML) and deep learning (DL) have recently been used to segment soil CT images, and it has shown great promise in extracting not only soil pore space but also other soil components. Deep learning (Goodfellow et al., 2016) is now used as an alternative to traditional purely handcrafted tools in almost all domains of image analysis around the world. In this context, soil and plant science (Pound et al., 2017; Kamilaris and Prenafeta-Boldú, 2018) is a promising area for the application of deep learning for a variety of reasons. For starters, it involves a wide range of biological variables, including growth, response to biotic and abiotic stress, and physiology. Second, plants exhibit enormous variability (e.g., size, shape, colour), and taking into account all of these variables exceed the human capacity for software development and responding to the needs of soil and plant scientists. Deep learning is part of a larger family of machine learning methods based on artificial neural networks (ANNs) with representation learning, which can be supervised, semi-supervised, or unsupervised. Deep neural networks have shown promising results in computer vision, medical image processing, and root segmentation in soil science. Deep learning algorithms for segmenting roots in soil using X-ray CT images have been developed with great success of the ANNs. The segmentation of roots and soil in X-ray tomography is one of the most difficult computer vision problems in plant sciences (Xu et al., 2018). Douarre et al. (2018) addressed the soil-root segmentation problem in X-ray tomography using transfer learning, a variant of supervised deep learning-based classification where the learning stage is based on simulated data. The transfer learning approach was used to train the convolutional deep network to select a feature space, which was then used to train a support vector machine (SVM) for the segmentation task. Wieland et al. (2021) used a deep learning classification technique that can deal with low phase contrast X-ray CT data due to the neural network’s robustness to solve the root segmentation challenge. Despite being trained with only synthetic soil columns, the deep network was able to successfully segment roots from the soil. Soltaninejad et al. (2020) used an encoder-decoder network-based deep learning approach to segment root system architecture from the soil. By explicitly obtaining local high-resolution segmentation and wider low-resolution segmentation, multiple resolutions were obtained. As a result, the network can extract fine root information from large X-ray CT volumes (1,670× 1,667×2,541 pixels). The multi-resolution method was shown to improve segmentation accuracy in both small and large receptive fields. Deep learning approaches have limitations such as unscalable models, massive datasets for training, the need for benchmarked datasets, misunderstanding the context of data, and opacity. The segmentation of image data is now possible quickly and accurately thanks to recent developments in machine learning, particularly the use of convolutional neural networks for image analysis. However, there are still difficulties when using convolutional neural networks to analyse images that are pertinent to agriculture and the environment (Raja et al., 2021).
6 Conclusion
Plant growth is improved by understanding the complex and heterogeneous structure of soils and their interactions with roots, but is hard to study using conventional approaches. The X-ray CT has a great advantage over the traditional method as it is non-destructive with high resolution 3D images. Combining X-ray CT with complementary approaches provides a powerful tool for studying soil pore architecture (which influences water and solute movement), root system architecture, the interaction between soils and root growth, the interactions between soils and roots within the rhizosphere, as well as the interactions between soil structure and microbial community functions and behaviour. The article focuses on soil and plant applications, advantages, and limitations of X-ray CT, including the possibility of using synchrotron-based X-ray CT to overcome some of the current limitation. We hope that the information compiled in this article will facilitate other researchers to use X-ray CT for examining a range of problems in soil–plant systems.
Author contributions
TG, PM, and TD: Conceptualization, writing and editing; SR and RB: Writing and Editing. All authors contributed to the article and approved the submitted version.
Conflict of interest
The authors declare that the research was conducted in the absence of any commercial or financial relationships that could be construed as a potential conflict of interest.
Publisher’s note
All claims expressed in this article are solely those of the authors and do not necessarily represent those of their affiliated organizations, or those of the publisher, the editors and the reviewers. Any product that may be evaluated in this article, or claim that may be made by its manufacturer, is not guaranteed or endorsed by the publisher.
References
Al-Shammary, A. A. G., Kouzani, A. Z., Kaynak, A., Khoo, S. Y., Norton, M., and Gates, W. (2018). Soil bulk density estimation methods: a review. Pedosphere 28, 581–596. doi:10.1016/s1002-0160(18)60034-7
Anderson, S. H., Gantzer, C. J., Boone, J. M., and Tully, R. J. (1988). Rapid nondestructive bulk density and soil-water content determination by computed tomography. Soil Sci. Soc. Am. J. 52, 35–40. doi:10.2136/sssaj1988.03615995005200010006x
Anderson, S. H., Wang, H., Peyton, R. L., and Gantzer, C. J. (2003). Estimation of porosity and hydraulic conductivity from X-ray CT-measured solute breakthrough. Geol. Soc. Lond. Spec. Publ. 215, 135–149. doi:10.1144/gsl.sp.2003.215.01.13
Araus, J. L., and Cairns, J. E. (2014). Field high-throughput phenotyping: the new crop breeding frontier. Trends Plant Sci. 19, 52–61. doi:10.1016/j.tplants.2013.09.008
Aravena, J. E., Berli, M., Ghezzehei, T. A., and Tyler, S. W. (2011). Effects of root-induced compaction on rhizosphere hydraulic properties-X-ray microtomography imaging and numerical simulations. Environ. Sci. Technol. 45, 425–431. doi:10.1021/es102566j
Atkinson, J. A., Hawkesford, M. J., Whalley, W. R., Zhou, H., and Mooney, S. J. (2020). Soil strength influences wheat root interactions with soil macropores. Plant. Cell Environ. 43, 235–245. doi:10.1111/pce.13659
Bagherifam, S., Delavar, M. A., Keshavarz, P., and Karimi, A. (2021). A review of soil carbon measurement methods: experimental considerations, advantages and disadvantages. J. Soil Manag. Sustain. Prod. 10, 1–30. doi:10.22069/EJSMS.2021.18039.1955
Bailey, D. L., Held, D. W., Kalra, A., Twarakavi, N., and Arriaga, F. (2015). Biopores from mole crickets (Scapteriscus spp.) increase soil hydraulic conductivity and infiltration rates. Appl. Soil Ecol. 94, 7–14. doi:10.1016/j.apsoil.2015.04.011
Balseiro-Romero, M., Mazurier, A., Monoshyn, D., Baveye, P. C., and Clause, J. (2020). Using X-ray microtomography to characterize the burrowing behaviour of earthworms in heterogeneously polluted soils. Pedobiol. (Jena). 83, 150671. doi:10.1016/j.pedobi.2020.150671
Beraldo, J. M. G., Scannavino Junior, F. de A., and Cruvinel, P. E. (2014). Application of x-ray computed tomography in the evaluation of soil porosity in soil management systems. Eng. Agrícola. 34, 1162–1174. doi:10.1590/s0100-69162014000600012
Bhattacharya, P., Maity, P. P., Ray, M., and Mridha, N. (2021). Prediction of mean weight diameter of soil using machine learning approaches. Agron. J. 113, 1303–1316. doi:10.1002/agj2.20469
Booth, S., Kurtz, B., de Heer, M. I., Mooney, S. J., and Sturrock, C. J. (2020). Tracking wireworm burrowing behaviour in soil over time using 3D X-ray computed tomography. Pest Manag. Sci. 76, 2653–2662. doi:10.1002/ps.5808
Borges, J. A. R., Pires, L. F., Cássaro, F. A. M., Auler, A. C., Rosa, J. A., Heck, R. J., et al. (2019). X-ray computed tomography for assessing the effect of tillage systems on topsoil morphological attributes. Soil Tillage Res. 189, 25–35. doi:10.1016/j.still.2018.12.019
Bouckaert, L., Sleutel, S., Van Loo, D., Brabant, L., Cnudde, V., Van Hoorebeke, L., et al. (2013). Carbon mineralisation and pore size classes in undisturbed soil cores. Soil Res. 51, 14–22. doi:10.1071/sr12116
Bouyoucos, G. J., and Mick, A. H. (1940). An electrical resistance method for the continuous measurement of soil moisture under field conditions. Tech. Bull. Mich. Agric. Exp. Stn. 172.
Capowiez, Y., Sammartino, S., and Michel, E. (2011). Using X-ray tomography to quantify earthworm bioturbation non-destructively in repacked soil cores. Geoderma 162, 124–131. doi:10.1016/j.geoderma.2011.01.011
Chapman, K., Ivanovici, A., Taleski, M., Sturrock, C. J., Ng, J. L. P., Mohd-Radzman, N. A., et al. (2020). CEP receptor signalling controls root system architecture in Arabidopsis and Medicago. new Phytol. 226, 1809–1821. doi:10.1111/nph.16483
Charlotte, V., Laure, V. G., Naoise, N., and Claire, C. (2022). Opportunities and limits in imaging microorganisms and their activities in soil microhabitats. Soil Biol. biochem. 174, 108807. doi:10.1016/j.soilbio.2022.108807
Chenu, C., and Plante, A. F. (2006). Clay-sized organo-mineral complexes in a cultivation chronosequence: revisiting the concept of the ‘primary organo-mineral complex. Eur. J. Soil Sci. 57, 596–607. doi:10.1111/j.1365-2389.2006.00834.x
Choi, C.-S., Lee, Y.-K., and Song, J.-J. (2020). Equivalent pore channel model for fluid flow in rock based on microscale X-ray CT imaging. Mater. (Basel) 13, 2619. doi:10.3390/ma13112619
Clausnitzer, V., and Hopmans, J. W. (2000). Pore-scale measurements of solute breakthrough using microfocus X-ray computed tomography. Water Resour. Res. 36, 2067–2079. doi:10.1029/2000wr900076
Colombi, T., Kirchgessner, N., Le Marié, C. A., York, L. M., Lynch, J. P., and Hund, A. (2015). Next generation shovelomics: set up a tent and REST. Plant Soil 388, 1–20. doi:10.1007/s11104-015-2379-7
Couradeau, E., Felde, V. J., Parkinson, D., Uteau, D., Rochet, A., Cuellar, C., et al. (2018). In situ X-ray tomography imaging of soil water and cyanobacteria from biological soil crusts undergoing desiccation. Front. Environ. Sci. 6, 65. doi:10.3389/fenvs.2018.00065
Crawford, J. W., Deacon, L., Grinev, D., Harris, J. A., Ritz, K., Singh, B. K., et al. (2012). Microbial diversity affects self-organization of the soil–microbe system with consequences for function. J. R. Soc. Interface 9, 1302–1310. doi:10.1098/rsif.2011.0679
Crestana, S., Mascarenhas, S., and Pozzi-Mucelli, R. S. (1985). Static and dynamic three-dimensional studies of water in soil using computed tomographic scanning1. Soil Sci. 140, 326–332. doi:10.1097/00010694-198511000-00002
Cuneo, I. F., Barrios-Masias, F., Knipfer, T., Uretsky, J., Reyes, C., Lenain, P., et al. (2021). Differences in grapevine rootstock sensitivity and recovery from drought are linked to fine root cortical lacunae and root tip function. New Phytol. 229, 272–283. doi:10.1111/nph.16542
Daly, K. R., Keyes, S. D., Masum, S., and Roose, T. (2016). Image-based modelling of nutrient movement in and around the rhizosphere. J. Exp. Bot. 67, 1059–1070. doi:10.1093/jxb/erv544
Daly, K. R., Mooney, S. J., Bennett, M. J., Crout, N. M. J., Roose, T., and Tracy, S. R. (2015). Assessing the influence of the rhizosphere on soil hydraulic properties using X-ray computed tomography and numerical modelling. J. Exp. Bot. 66, 2305–2314. doi:10.1093/jxb/eru509
De Gryze, S., Jassogne, L., Six, J., Bossuyt, H., Wevers, M., and Merckx, R. (2006). Pore structure changes during decomposition of fresh residue: X-ray tomography analyses. Geoderma 134, 82–96. doi:10.1016/j.geoderma.2005.09.002
de Oliveira, J. A. T., Cássaro, F. A. M., and Pires, L. F. (2021). Estimating soil porosity and pore size distribution changes due to wetting-drying cycles by morphometric image analysis. Soil Tillage Res. 205, 104814. doi:10.1016/j.still.2020.104814
Di Stefano, C., Ferro, V., and Mirabile, S. (2010). Comparison between grain-size analyses using laser diffraction and sedimentation methods. Biosyst. Eng. 106, 205–215. doi:10.1016/j.biosystemseng.2010.03.013
Diel, J., Vogel, H.-J., and Schlüter, S. (2019). Impact of wetting and drying cycles on soil structure dynamics. Geoderma 345, 63–71. doi:10.1016/j.geoderma.2019.03.018
Domander, R., Felder, A. A., and Doube, M. (2021). BoneJ2-refactoring established research software. Wellcome Open Res. 6, 37. doi:10.12688/wellcomeopenres.16619.2
Douarre, C., Schielein, R., Frindel, C., Gerth, S., and Rousseau, D. (2018). Transfer learning from synthetic data applied to soil–root segmentation in x-ray tomography images. J. Imaging 4, 65. doi:10.3390/jimaging4050065
Du Plessis, A., Olawuyi, B. J., Boshoff, W. P., and Le Roux, S. G. (2016). Simple and fast porosity analysis of concrete using X-ray computed tomography. Mat. Struct. 49, 553–562. doi:10.1617/s11527-014-0519-9
Duddek, P., Carminati, A., Koebernick, N., Ohmann, L., Lovric, G., Delzon, S., et al. (2022). The impact of drought-induced root and root hair shrinkage on root–soil contact. Plant Physiol. 189, 1232–1236. doi:10.1093/plphys/kiac144
Elias, E. A., Onasch, I. O. I., and Alaily, F. (2021). Dry bulk density of Gezira Vertisol as determined by X-ray computed tomography. Gezira J. Agric. Sci. 1, 121–127.
Elyeznasni, N., Sellami, F., Pot, V., Benoit, P., Vieublé-Gonod, L., Young, I., et al. (2012). Exploration of soil micromorphology to identify coarse-sized OM assemblages in X-ray CT images of undisturbed cultivated soil cores. Geoderma 179, 38–45. doi:10.1016/j.geoderma.2012.02.023
Fathizad, H., Ardakani, M. A. H., Sodaiezadeh, H., Kerry, R., and Taghizadeh-Mehrjardi, R. (2020). Investigation of the spatial and temporal variation of soil salinity using random forests in the central desert of Iran. Geoderma 365, 114233. doi:10.1016/j.geoderma.2020.114233
Feeney, D. S., Crawford, J. W., Daniell, T., Hallett, P. D., Nunan, N., Ritz, K., et al. (2006). Three-dimensional microorganization of the soil–root–microbe system. Microb. Ecol. 52, 151–158. doi:10.1007/s00248-006-9062-8
Feng, Y., Wang, J., Bai, Z., Reading, L., and Jing, Z. (2020). Three-dimensional quantification of macropore networks of different compacted soils from opencast coal mine area using X-ray computed tomography. Soil Tillage Res. 198, 104567. doi:10.1016/j.still.2019.104567
Ferreira, S. J., Senning, M., Sonnewald, S., Keßling, P.-M., Goldstein, R., and Sonnewald, U. (2010). Comparative transcriptome analysis coupled to X-ray CT reveals sucrose supply and growth velocity as major determinants of potato tuber starch biosynthesis. BMC Genomics 11, 93–17. doi:10.1186/1471-2164-11-93
Ferreira, T. R., Cássaro, F. A. M., Zhou, H., and Pires, L. F. (2022). “X-Ray computed tomography image processing and segmentation: a case study applying machine learning and deep learning-based strategies,” in X-ray imaging of the soil porous architecture (Cham: Springer), 57–80.
Fessler, J. A., and Rogers, W. L. (1996). Spatial resolution properties of penalized-likelihood image reconstruction: space-invariant tomographs. IEEE Trans. Image Process. 5, 1346–1358. doi:10.1109/83.535846
Filipović, V., Defterdarović, J., Šimůnek, J., Filipović, L., Ondrašek, G., Romić, D., et al. (2020). Estimation of vineyard soil structure and preferential flow using dye tracer, X-ray tomography, and numerical simulations. Geoderma 380, 114699. doi:10.1016/j.geoderma.2020.114699
Fisher, P., Aumann, C., Chia, K., O’Halloran, N., and Chandra, S. (2017). Adequacy of laser diffraction for soil particle size analysis. PLoS One 12, e0176510. doi:10.1371/journal.pone.0176510
Flavel, R. J., Guppy, C. N., Rabbi, S. M. R., and Young, I. M. (2017). An image processing and analysis tool for identifying and analysing complex plant root systems in 3D soil using non-destructive analysis: root1. PLoS One 12, e0176433. doi:10.1371/journal.pone.0176433
Flavel, R. J., Guppy, C. N., Tighe, M., Watt, M., McNeill, A., and Young, I. M. (2012). Non-destructive quantification of cereal roots in soil using high-resolution X-ray tomography. J. Exp. Bot. 63, 2503–2511. doi:10.1093/jxb/err421
Fourie, W., Said, R., Young, P., and Barnes, D. L. (2007). “The simulation of pore scale fluid flow with real world geometries obtained from X-ray computed tomography,” in Proceedings of the Boston COMSOL Conference.
Ganther, M., Yim, B., Ibrahim, Z., Bienert, M. D., Lippold, E., Maccario, L., et al. (2020). Compatibility of X-ray computed tomography with plant gene expression, rhizosphere bacterial communities and enzyme activities. J. Exp. Bot. 71, 5603–5614. doi:10.1093/jxb/eraa262
Gao, W., Schlüter, S., Blaser, S. R. G. A., Shen, J., and Vetterlein, D. (2019). A shape-based method for automatic and rapid segmentation of roots in soil from X-ray computed tomography images: Rootine. Plant Soil 441, 643–655. doi:10.1007/s11104-019-04053-6
Gee, G. W., and Bauder, J. (1986). Particle size analysis. Methods soil Anal. Part 1 1986, 383–411. doi:10.2136/sssabookser5.1.2ed.c15
Ghosh, T., Maity, P. P., Das, T. K., Chakraborty, D., Bhatia, A., Ray, M., et al. (2022). Characterization of soil pores through X-ray computed microtomography and carbon mineralization under contrasting tillage and land configurations in the indo-gangetic plains of India. Front. Environ. Sci. 551. doi:10.3389/fenvs.2022.898249
Ghosh, T., Maity, P. P., Das, T. K., Krishnan, P., Bhatia, A., Bhattacharya, P., et al. (2020a). Variation of porosity, pore size distribution and soil physical properties under conservation agriculture. Indian J. Agric. Sci. 90, 2051–2058. doi:10.56093/ijas.v90i11.108558
Ghosh, T., Maity, P. P., Das, T. K., Krishnan, P., Bhatia, A., Roy, M., et al. (2020). Evaluation of different infiltration models under long term conservation agricultural practices. Indian J. Agric. Sci. 90, 2379–2384. doi:10.56093/ijas.v90i12.110345
Gostick, J., Aghighi, M., Hinebaugh, J., Tranter, T., Hoeh, M. A., Day, H., et al. (2016). OpenPNM: a pore network modeling package. Comput. Sci. Eng. 18, 60–74. doi:10.1109/mcse.2016.49
Grayling, K. M., Young, S. D., Roberts, C. J., de Heer, M. I., Shirley, I. M., Sturrock, C. J., et al. (2018). The application of X-ray micro computed tomography imaging for tracing particle movement in soil. Geoderma 321, 8–14. doi:10.1016/j.geoderma.2018.01.038
Gregory, P. J., Hutchison, D. J., Read, D. B., Jenneson, P. M., Gilboy, W. B., and Morton, E. J. (2003). Non-invasive imaging of roots with high resolution X-ray micro-tomography. Plant Soil 255, 351–359. Roots: The Dynamic Interface between Plants and the Earth. Springer. doi:10.1023/A:1026179919689
Griffiths, M., Mellor, N., Sturrock, C. J., Atkinson, B. S., Johnson, J., Mairhofer, S., et al. (2022). X-ray CT reveals 4D root system development and lateral root responses to nitrate in soil. Plant Phenome J. 5, e20036. doi:10.1002/ppj2.20036
Gupta, H., Jin, K. H., Nguyen, H. Q., McCann, M. T., and Unser, M. (2018). CNN-based projected gradient descent for consistent CT image reconstruction. IEEE Trans. Med. Imaging 37, 1440–1453. doi:10.1109/tmi.2018.2832656
Hainsworth, J. M., and Aylmore, L. A. G. (1983). The use of computer assisted tomography to determine spatial distribution of soil water content. Soil Res. 21, 435–443. doi:10.1071/sr9830435
Hamamoto, S., Moldrup, P., Kawamoto, K., Sakaki, T., Nishimura, T., and Komatsu, T. (2016). Pore network structure linked by X-ray CT to particle characteristics and transport parameters. Soils Found. 56, 676–690. doi:10.1016/j.sandf.2016.07.008
Hamza, M. A., Anderson, S. H., and Aylmore, L. A. G. (2007). Computed tomographic evaluation of osmotica on shrinkage and recovery of lupin (Lupinus angustifolius L.) and radish (Raphanus sativus L.) roots. Environ. Exp. Bot. 59, 334–339. doi:10.1016/j.envexpbot.2006.04.004
Hamza, M. A., Anderson, S. H., and Aylmore, L. A. G. (2001). Studies of soil water drawdowns by single radish roots at decreasing soil water content using computer-assisted tomography. Soil Res. 39, 1387–1396. doi:10.1071/sr98057
Han, L., Dutilleul, P., Prasher, S. O., Beaulieu, C., and Smith, D. L. (2009). Assessment of density effects of the common scab-inducing pathogen on the seed and peripheral organs of potato during growth using computed tomography scanning data. Trans. ASABE 52, 305–311. doi:10.13031/2013.25924
Heeraman, D. A., Hopmans, J. W., and Clausnitzer, V. (1997). Three dimensional imaging of plant roots in situ with X-ray computed tomography. Plant Soil 189, 167–179. doi:10.1023/b:plso.0000009694.64377.6f
Helliwell, J. R., Sturrock, C. J., Grayling, K. M., Tracy, S. R., Flavel, R. J., Young, I. M., et al. (2013). Applications of X-ray computed tomography for examining biophysical interactions and structural development in soil systems: a review. Eur. J. Soil Sci. 64, 279–297. doi:10.1111/ejss.12028
Helliwell, J. R., Sturrock, C. J., Mairhofer, S., Craigon, J., Ashton, R. W., Miller, A. J., et al. (2017). The emergent rhizosphere: imaging the development of the porous architecture at the root-soil interface. Sci. Rep. 7, 14875–14910. doi:10.1038/s41598-017-14904-w
Helliwell, J. R., Sturrock, C. J., Miller, A. J., Whalley, W. R., and Mooney, S. J. (2019). The role of plant species and soil condition in the structural development of the rhizosphere. Plant. Cell Environ. 42, 1974–1986. doi:10.1111/pce.13529
Herrero-Huerta, M., Meline, V., Iyer-Pascuzzi, A. S., Souza, A. M., Tuinstra, M. R., and Yang, Y. (2021). 4D Structural root architecture modeling from digital twins by X-Ray Computed Tomography. Plant Methods 17, 123–212. doi:10.1186/s13007-021-00819-1
Holmes, J. W. (1956). Measuring soil water content and evaporation by the neutron scattering method. Neth. J. Agric. Sci. 4, 30–34. doi:10.18174/njas.v4i1.17769
Hounsfield, G. N. (1973). Computerized transverse axial scanning (tomography): Part 1. Description of system. Br. J. Radiol. 46, 1016–1022. doi:10.1259/0007-1285-46-552-1016
Hu, X., Li, X.-Y., Guo, L.-L., Liu, Y., Wang, P., Zhao, Y.-D., et al. (2019). Influence of shrub roots on soil macropores using X-ray computed tomography in a shrub-encroached grassland in Northern China. J. Soils Sediments 19, 1970–1980. doi:10.1007/s11368-018-2218-6
Jenneson, P. M., Gilboy, W. B., Morton, E. J., Luggar, R. D., Gragory, P. J., and Hutchinson, D. (1999). “Optimisation of X-ray micro-tomography for the in situ study of the development of plant roots,” in 1999 IEEE Nuclear Science Symposium. Conference Record. 1999 Nuclear Science Symposium and Medical Imaging Conference (Cat. No. 99CH37019), Seattle, WA, USA, 24-30 October 1999, 429–432.
Jia, L., Gu, Y., Zhang, Q., Zhang, J., Yan, X., Zhang, Y., et al. (2023). The accuracy evaluation method of baseline estimation algorithms in energy dispersive X-ray fluorescence spectrum analysis. X-Ray Spectrom. 52, 22–27. doi:10.1002/xrs.3180
Juyal, A., Eickhorst, T., Falconer, R., Baveye, P. C., Spiers, A., and Otten, W. (2018). Control of pore geometry in soil microcosms and its effect on the growth and spread of Pseudomonas and Bacillus sp. Front. Environ. Sci. 6, 73. doi:10.3389/fenvs.2018.00073
Juyal, A., Otten, W., Baveye, P. C., and Eickhorst, T. (2021). Influence of soil structure on the spread of Pseudomonas fluorescens in soil at microscale. Eur. J. Soil Sci. 72, 141–153. doi:10.1111/ejss.12975
Juyal, A., Otten, W., Falconer, R., Hapca, S., Schmidt, H., Baveye, P. C., et al. (2019). Combination of techniques to quantify the distribution of bacteria in their soil microhabitats at different spatial scales. Geoderma 334, 165–174. doi:10.1016/j.geoderma.2018.07.031
Kaestner, A., Schneebeli, M., and Graf, F. (2006). Visualizing three-dimensional root networks using computed tomography. Geoderma 136, 459–469. doi:10.1016/j.geoderma.2006.04.009
Kamamia, A. W., Vogel, C., Mwangi, H. M., Feger, K.-H., Sang, J., and Julich, S. (2021). Mapping soil aggregate stability using digital soil mapping: a case study of Ruiru reservoir catchment, Kenya. Geoderma Reg. 24, e00355. doi:10.1016/j.geodrs.2020.e00355
Kamilaris, A., and Prenafeta-Boldú, F. X. (2018). Deep learning in agriculture: a survey. Comput. Electron. Agric. 147, 70–90. doi:10.1016/j.compag.2018.02.016
Keyes, S. D., Gostling, N. J., Cheung, J. H., Roose, T., Sinclair, I., and Marchant, A. (2017). The application of contrast media for in vivo feature enhancement in X-ray computed tomography of soil-grown plant roots. Microsc. Microanal. 23, 538–552. doi:10.1017/s1431927617000319
Keyes, S., Van Veelen, A., McKay Fletcher, D., Scotson, C., Koebernick, N., Petroselli, C., et al. (2022). Multimodal correlative imaging and modelling of phosphorus uptake from soil by hyphae of mycorrhizal fungi. New Phytol. 234, 688–703. doi:10.1111/nph.17980
Kim, H., Anderson, S. H., Motavalli, P. P., and Gantzer, C. J. (2010). Compaction effects on soil macropore geometry and related parameters for an arable field. Geoderma 160, 244–251. doi:10.1016/j.geoderma.2010.09.030
Kinyangi, J., Solomon, D., Liang, B., Lerotic, M., Wirick, S., and Lehmann, J. (2006). Nanoscale biogeocomplexity of the organomineral assemblage in soil: application of STXM microscopy and C 1s-NEXAFS spectroscopy. Soil Sci. Soc. Am. J. 70, 1708–1718. doi:10.2136/sssaj2005.0351
Koestel, J., Larsbo, M., and Jarvis, N. (2020). Scale and REV analyses for porosity and pore connectivity measures in undisturbed soil. Geoderma 366, 114206. doi:10.1016/j.geoderma.2020.114206
Koestel, J., and Schlüter, S. (2019). Quantification of the structure evolution in a garden soil over the course of two years. Geoderma 338, 597–609. doi:10.1016/j.geoderma.2018.12.030
Krause, M. J., Kummerländer, A., Avis, S. J., Kusumaatmaja, H., Dapelo, D., Klemens, F., et al. (2021). OpenLB—open source lattice Boltzmann code. Comput. Math. Appl. 81, 258–288. doi:10.1016/j.camwa.2020.04.033
Le Roux, S. G., Du Plessis, A., and Clarke, C. E. (2019). MicroCT-based bulk density measurement method for soils. J. South Afr. Inst. Civ. Eng. 61, 2–9. doi:10.17159/2309-8775/2019/v61n1a1
Legland, D., Arganda-Carreras, I., and Andrey, P. (2016). MorphoLibJ: integrated library and plugins for mathematical morphology with ImageJ. Bioinformatics 32, 3532–3534. doi:10.1093/bioinformatics/btw413
Li, P., and Shao, S. (2020). Can X-ray computed tomography (CT) be used to determine the pore-size distribution of intact loess? Environ. Earth Sci. 79, 29. doi:10.1007/s12665-019-8777-z
Liu, B., Ma, R., and Fan, H. (2021). Evaluation of the impact of freeze-thaw cycles on pore structure characteristics of black soil using X-ray computed tomography. Soil Tillage Res. 206, 104810. doi:10.1016/j.still.2020.104810
Lontoc-Roy, M., Dutilleul, P., Prasher, S. O., Han, L., Brouillet, T., and Smith, D. L. (2006). Advances in the acquisition and analysis of CT scan data to isolate a crop root system from the soil medium and quantify root system complexity in 3-D space. Geoderma 137, 231–241. doi:10.1016/j.geoderma.2006.08.025
Lu, S., Yu, X., and Zong, Y. (2019). Nano-microscale porosity and pore size distribution in aggregates of paddy soil as affected by long-term mineral and organic fertilization under rice-wheat cropping system. Soil Tillage Res. 186, 191–199. doi:10.1016/j.still.2018.10.008
Lucas, M., Schlüter, S., Vogel, H.-J., and Vetterlein, D. (2019). Soil structure formation along an agricultural chronosequence. Geoderma 350, 61–72. doi:10.1016/j.geoderma.2019.04.041
Luo, L., Lin, H., and Halleck, P. (2008). Quantifying soil structure and preferential flow in intact soil using X-ray computed tomography. Soil Sci. Soc. Am. J. 72, 1058–1069. doi:10.2136/sssaj2007.0179
Luo, L., Lin, H., and Li, S. (2010). Quantification of 3-D soil macropore networks in different soil types and land uses using computed tomography. J. Hydrol. 393, 53–64. doi:10.1016/j.jhydrol.2010.03.031
Ma, L., Shao, M., Fan, J., Wang, J., and Li, Y. (2021). Effects of earthworm (Metaphire guillelmi) density on soil macropore and soil water content in typical Anthrosol soil. Agric. Ecosyst. Environ. 311, 107338. doi:10.1016/j.agee.2021.107338
Mahmoudi, G., Fouladi, M. R., Ay, M. R., Rahmim, A., and Ghadiri, H. (2019). Sparse-view statistical image reconstruction with improved total variation regularization for X-ray micro-CT imaging. J. Instrum. 14, P08023. doi:10.1088/1748-0221/14/08/p08023
Mairhofer, S., Zappala, S., Tracy, S. R., Sturrock, C., Bennett, M., Mooney, S. J., et al. (2012). RooTrak: automated recovery of three-dimensional plant root architecture in soil from X-ray microcomputed tomography images using visual tracking. Plant Physiol. 158, 561–569. doi:10.1104/pp.111.186221
Mairhofer, S., Pridmore, T., Johnson, J., Wells, D. M., Bennett, M. J., Mooney, S. J., et al. (2017). X-ray computed tomography of crop plant root systems grown in soil. Curr. Protoc. Plant Biol. 2, 270–286. doi:10.1002/cppb.20049
Mawodza, T., Burca, G., Casson, S., and Menon, M. (2020). Wheat root system architecture and soil moisture distribution in an aggregated soil using neutron computed tomography. Geoderma 359, 113988. doi:10.1016/j.geoderma.2019.113988
Mendes, R., Garbeva, P., and Raaijmakers, J. M. (2013). The rhizosphere microbiome: significance of plant beneficial, plant pathogenic, and human pathogenic microorganisms. FEMS Microbiol. Rev. 37, 634–663. doi:10.1111/1574-6976.12028
Menon, M., Mawodza, T., Rabbani, A., Blaud, A., Lair, G. J., Babaei, M., et al. (2020). Pore system characteristics of soil aggregates and their relevance to aggregate stability. Geoderma 366, 114259.
Mooney, S. J., Morris, C., and Berry, P. M. (2006). Visualization and quantification of the effects of cereal root lodging on three-dimensional soil macrostructure using X-ray computed tomography. Soil Sci. 171, 706–718. doi:10.1097/01.ss.0000228041.03142.d3
Mooney, S. J. (2002). Three-dimensional visualization and quantification of soil macroporosity and water flow patterns using computed tomography. Soil Use Manag. 18, 142–151. doi:10.1111/j.1475-2743.2002.tb00232.x
Moradi, A. B., Carminati, A., Vetterlein, D., Vontobel, P., Lehmann, E., Weller, U., et al. (2011). Three-dimensional visualization and quantification of water content in the rhizosphere. New Phytol. 192, 653–663. doi:10.1111/j.1469-8137.2011.03826.x
Mukhlisin, M., Astuti, H. W., Wardihani, E. D., and Matlan, S. J. (2021). Techniques for ground-based soil moisture measurement: a detailed overview. Arab. J. Geosci. 14, 2032–2034. doi:10.1007/s12517-021-08263-0
Müller, K., Katuwal, S., Young, I., McLeod, M., Moldrup, P., de Jonge, L. W., et al. (2018). Characterising and linking X-ray CT derived macroporosity parameters to infiltration in soils with contrasting structures. Geoderma 313, 82–91. doi:10.1016/j.geoderma.2017.10.020
Musa, J. J., and Gupa, Y. U. (2019). An overview of methods used in the determination of soil hydraulic conductivity. AJPAS.
Niu, G., Shao, L., Sun, D., and Guo, X. (2020). A simplified directly determination of soil-water retention curve from pore size distribution. Geomech. Eng. 20, 411–420. doi:10.12989/gae.2020.23.5.431
O’Donnell, A. G., Young, I. M., Rushton, S. P., Shirley, M. D., and Crawford, J. W. (2007). Visualization, modelling and prediction in soil microbiology. Nat. Rev. Microbiol. 5, 689–699. doi:10.1038/nrmicro1714
Oldendorf, W. H. (1978). The quest for an image of brain: a brief historical and technical review of brain imaging techniques. Neurology 28, 517. doi:10.1212/wnl.28.6.517
Page-Dumroese, D. S., Brown, R. E., Jurgensen, M. F., and Mroz, G. D. (1999). Comparison of methods for determining bulk densities of rocky forest soils. Soil Sci. Soc. Am. J. 63, 379–383. doi:10.2136/sssaj1999.03615995006300020016x
Perret, J., Prasher, S. O., Kantzas, A., and Langford, C. (2000). A two-domain approach using CAT scanning to model solute transport in soil. New York: Wiley Online Library.
Perret, J. S., Al-Belushi, M. E., and Deadman, M. (2007). Non-destructive visualization and quantification of roots using computed tomography. Soil Biol. biochem. 39, 391–399. doi:10.1016/j.soilbio.2006.07.018
Peth, S., Chenu, C., Leblond, N., Mordhorst, A., Garnier, P., Nunan, N., et al. (2014). Localization of soil organic matter in soil aggregates using synchrotron-based X-ray microtomography. Soil Biol. biochem. 78, 189–194. doi:10.1016/j.soilbio.2014.07.024
Petrovic, A. M., Siebert, J. E., and Rieke, P. E. (1982). Soil bulk density analysis in three dimensions by computed tomographic scanning. Soil Sci. Soc. Am. J. 46, 445–450. doi:10.2136/sssaj1982.03615995004600030001x
Pfeifer, J., Kirchgessner, N., Colombi, T., and Walter, A. (2015). Rapid phenotyping of crop root systems in undisturbed field soils using X-ray computed tomography. Plant Methods 11, 41–48. doi:10.1186/s13007-015-0084-4
Piccoli, I., Schjønning, P., Lamandé, M., Zanini, F., and Morari, F. (2019). Coupling gas transport measurements and X-ray tomography scans for multiscale analysis in silty soils. Geoderma 338, 576–584. doi:10.1016/j.geoderma.2018.09.029
Pires, L. F., Auler, A. C., Roque, W. L., and Mooney, S. J. (2020). X-ray microtomography analysis of soil pore structure dynamics under wetting and drying cycles. Geoderma 362, 114103. doi:10.1016/j.geoderma.2019.114103
Pires, L. F., Roque, W. L., Rosa, J. A., and Mooney, S. J. (2019). 3D analysis of the soil porous architecture under long term contrasting management systems by X-ray computed tomography. Soil Tillage Res. 191, 197–206. doi:10.1016/j.still.2019.02.018
Pound, M. P., Atkinson, J. A., Townsend, A. J., Wilson, M. H., Griffiths, M., Jackson, A. S., et al. (2017). Deep machine learning provides state-of-the-art performance in image-based plant phenotyping. Gigascience 6, 1–10. doi:10.1093/gigascience/gix083
Pramanik, P., and Aggarwal, P. (2013). Comparison of thermal properties of three texturally different soils under two compaction levels. Afr. J. Agric. Res. 8, 3679–3687. doi:10.5897/ajar2013.7345
Quinton, W. L., Elliot, T., Price, J. S., Rezanezhad, F., and Heck, R. (2009). Measuring physical and hydraulic properties of peat from X-ray tomography. Geoderma 153, 269–277. doi:10.1016/j.geoderma.2009.08.010
Rab, M. A., Haling, R. E., Aarons, S. R., Hannah, M., Young, I. M., and Gibson, D. (2014). Evaluation of X-ray computed tomography for quantifying macroporosity of loamy pasture soils. Geoderma 213, 460–470. doi:10.1016/j.geoderma.2013.08.037
Rabbi, S. M. F., Daniel, H., Lockwood, P. V., Macdonald, C., Pereg, L., Tighe, M., et al. (2016). Physical soil architectural traits are functionally linked to carbon decomposition and bacterial diversity. Sci. Rep. 6, 33012–33019. doi:10.1038/srep33012
Rabbi, S. M. F., Wilson, B. R., Lockwood, P. V., Daniel, H., and Young, I. M. (2015). Aggregate hierarchy and carbon mineralization in two oxisols of new south wales, Australia. Soil Tillage Res. 146, 193–203. doi:10.1016/j.still.2014.10.008
Radon, J. (1917). On the determination of functions from their integrals along certain manifolds. Ber. Verh. Sachs Akad. Wiss. 69, 262–277.
Raja, P., Olenskyj, A., Kamangir, H., and Earles, M. (2021). Simultaneously predicting multiple plant traits from multiple sensors via deformable CNN regression. arXiv Prepr. arXiv2112.03205. Available at: https://doi.org/10.48550/arXiv.2112.03205.
Rasband, W. (2002). ImageJ: image processing and analysis in java. Astrophysics Source Code Library.
Rezanezhad, F., Quinton, W. L., Price, J. S., Elrick, D., Elliot, T. R., and Heck, R. J. (2009). Examining the effect of pore size distribution and shape on flow through unsaturated peat using computed tomography. Hydrol. Earth Syst. Sci. 13, 1993–2002. doi:10.5194/hess-13-1993-2009
Rippner, D. A., Raja, P. V., Earles, J. M., Momayyezi, M., Buchko, A., Duong, F. V., et al. (2022). A workflow for segmenting soil and plant X-ray computed tomography images with deep learning in Google’s Colaboratory. Front. Plant Sci. 13, 893140. doi:10.3389/fpls.2022.893140
Rogasik, H., Crawford, J. W., Wendroth, O., Young, I. M., Joschko, M., and Ritz, K. (1999). Discrimination of soil phases by dual energy x-ray tomography. Soil Sci. Soc. Am. J. 63, 741–751. doi:10.2136/sssaj1999.634741x
Rogers, E. D., Monaenkova, D., Mijar, M., Nori, A., Goldman, D. I., and Benfey, P. N. (2016). X-ray computed tomography reveals the response of root system architecture to soil texture. Plant Physiol. 171, 2028–2040. doi:10.1104/pp.16.00397
Sadiq, S. M., Kubba, F. A., and Ahmed, F. K. I. (2020). 2-D steady seepage flow model to simulate the contaminates transportation through homogeneous earth dam using Geo–Studio software. IOP Conf. Ser. Mat. Sci. Eng. 870, 012028. doi:10.1088/1757-899X/870/1/012028
Schindelin, J., Arganda-Carreras, I., Frise, E., Kaynig, V., Longair, M., Pietzsch, T., et al. (2012). Fiji: an open-source platform for biological-image analysis. Nat. Methods 9, 676–682. doi:10.1038/nmeth.2019
Schlüter, S., Albrecht, L., Schwärzel, K., and Kreiselmeier, J. (2020). Long-term effects of conventional tillage and no-tillage on saturated and near-saturated hydraulic conductivity–Can their prediction be improved by pore metrics obtained with X-ray CT? Geoderma 361, 114082. doi:10.1016/j.geoderma.2019.114082
Schlüter, S., Leuther, F., Albrecht, L., Hoeschen, C., Kilian, R., Surey, R., et al. (2022). Microscale carbon distribution around pores and particulate organic matter varies with soil moisture regime. Nat. Commun. 13, 2098. doi:10.1038/s41467-022-29605-w
Schmidt, H., Vetterlein, D., Köhne, J. M., and Eickhorst, T. (2015). Negligible effect of X-ray μ-CT scanning on archaea and bacteria in an agricultural soil. Soil Biol. biochem. 84, 21–27. doi:10.1016/j.soilbio.2015.02.010
Schmidt, S., Bengough, A. G., Gregory, P. J., Grinev, D. V., and Otten, W. (2012). Estimating root–soil contact from 3D X-ray microtomographs. Eur. J. Soil Sci. 63, 776–786. doi:10.1111/j.1365-2389.2012.01487.x
Schnepf, A., Carminati, A., Ahmed, M. A., Ani, M., Benard, P., Bentz, J., et al. (2022). Linking rhizosphere processes across scales: opinion. Plant Soil 478, 5–42. doi:10.1007/s11104-022-05306-7
Sharma, P. K., Kumar, D., Srivastava, H. S., and Patel, P. (2018). Assessment of different methods for soil moisture estimation: a review. J. Remote Sens. GIS 9, 57–73.
Singh, A. K., Bhardwaj, A. K., Verma, C. L., Mishra, V. K., Singh, A. K., Arora, S., et al. (2019). Soil moisture sensing techniques for scheduling irrigation. J. Soil Salin. Water Qual. 11, 68–76.
Soltaninejad, M., Sturrock, C. J., Griffiths, M., Pridmore, T. P., and Pound, M. P. (2020). Three dimensional root CT segmentation using multi-resolution encoder-decoder networks. IEEE Trans. Image Process 29, 6667–6679. doi:10.1109/tip.2020.2992893
Soto-Gomez, D., Perez-Rodriguez, P., Juiz, L. V., Paradelo, M., and Lopez-Periago, J. E. (2020). 3D multifractal characterization of computed tomography images of soils under different tillage management: linking multifractal parameters to physical properties. Geoderma 363, 114129. doi:10.1016/j.geoderma.2019.114129
Sulthoni, M. A., and Rizqulloh, M. A. (2019). “A low cost microcontroller-based time domain reflectometer for soil moisture measurement,” in 2019 International Conference on Electrical Engineering and Informatics (ICEEI), Bandung, Indonesia, 09-10 July 2019, 197–200.
Sun, W.-J., and Cui, Y.-J. (2020). Determining the soil-water retention curve using mercury intrusion porosimetry test in consideration of soil volume change. J. Rock Mech. Geotech. Eng. 12, 1070–1079. doi:10.1016/j.jrmge.2019.12.022
Taina, I. A., Heck, R. J., and Elliot, T. R. (2008). Application of X-ray computed tomography to soil science: a literature review. Can. J. Soil Sci. 88, 1–19. doi:10.4141/cjss06027
Tan, W., Liu, P., Li, X., Liu, Y., Zhou, Q., Chen, C., et al. (2021). Classification of COVID-19 pneumonia from chest CT images based on reconstructed super-resolution images and VGG neural network. Heal. Inf. Sci. Syst. 9, 10–12. doi:10.1007/s13755-021-00140-0
Taylor, H., and Brar, G. S. (1991). Effect of soil compaction on root development. Soil Tillage Res. 19, 111–119. doi:10.1016/0167-1987(91)90080-h
Teramoto, S., Takayasu, S., Kitomi, Y., Arai-Sanoh, Y., Tanabata, T., and Uga, Y. (2020). High-throughput three-dimensional visualization of root system architecture of rice using X-ray computed tomography. Plant Methods 16, 66–14. doi:10.1186/s13007-020-00612-6
Thibault, J., Sauer, K. D., Bouman, C. A., and Hsieh, J. (2007). A three-dimensional statistical approach to improved image quality for multislice helical CT. Med. Phys. 34, 4526–4544. doi:10.1118/1.2789499
Thieme, J., Schneider, G., and Knöchel, C. (2003). X-ray tomography of a microhabitat of bacteria and other soil colloids with sub-100 nm resolution. Micron 34, 339–344. doi:10.1016/s0968-4328(03)00061-1
Tollner, E. W., Ramseur, E. L., and Murphy, C. (1994). Techniques and approaches for documenting plant root development with x-ray computed tomography. Tomogr. Soil-Water-Root Process. 36, 115–133. doi:10.2136/sssaspecpub36.c10
Topp, G. C., and Davis, J. L. (1985). Measurement of soil water content using time-domain reflectrometry (TDR): a field evaluation. Soil Sci. Soc. Am. J. 49, 19–24. doi:10.2136/sssaj1985.03615995004900010003x
Torre, I. G., Martín-Sotoca, J. J., Losada, J. C., López, P., and Tarquis, A. M. (2020). Scaling properties of binary and greyscale images in the context of X-ray soil tomography. Geoderma 365, 114205. doi:10.1016/j.geoderma.2020.114205
Trachsel, S., Kaeppler, S. M., Brown, K. M., and Lynch, J. P. (2011). Shovelomics: high throughput phenotyping of maize (Zea mays L.) root architecture in the field. Plant Soil 341, 75–87. doi:10.1007/s11104-010-0623-8
Tracy, S. R., Black, C. R., Roberts, J. A., McNeill, A., Davidson, R., Tester, M., et al. (2012a). Quantifying the effect of soil compaction on three varieties of wheat (Triticum aestivum L.) using X-ray Micro Computed Tomography (CT). Plant Soil 353, 195–208. doi:10.1007/s11104-011-1022-5
Tracy, S. R., Black, C. R., Roberts, J. A., Sturrock, C., Mairhofer, S., Craigon, J., et al. (2012b). Quantifying the impact of soil compaction on root system architecture in tomato (Solanum lycopersicum) by X-ray micro-computed tomography. Ann. Bot. 110, 511–519. doi:10.1093/aob/mcs031
Tracy, S. R., Daly, K. R., Sturrock, C. J., Crout, N. M. J., Mooney, S. J., and Roose, T. (2015). Three-dimensional quantification of soil hydraulic properties using X-ray computed tomography and image-based modeling. Water Resour. Res. 51, 1006–1022. doi:10.1002/2014wr016020
Tracy, S. R., Roberts, J. A., Black, C. R., McNeill, A., Davidson, R., and Mooney, S. J. (2010). The X-factor: visualizing undisturbed root architecture in soils using X-ray computed tomography. J. Exp. Bot. 61, 311–313. doi:10.1093/jxb/erp386
Van den Bulcke, J., Boone, M., Van Acker, J., and Van Hoorebeke, L. (2009). Three-dimensional X-ray imaging and analysis of fungi on and in wood. Microsc. Microanal. 15, 395–402. doi:10.1017/s1431927609990419
Van Veelen, A., Koebernick, N., Scotson, C. S., McKay-Fletcher, D., Huthwelker, T., Borca, C. N., et al. (2020). Root-induced soil deformation influences Fe, S and P: rhizosphere chemistry investigated using synchrotron XRF and XANES. New Phytol. 225, 1476–1490. doi:10.1111/nph.16242
Wang, G., Ye, J. C., and De Man, B. (2020). Deep learning for tomographic image reconstruction. Nat. Mach. Intell. 2, 737–748. doi:10.1038/s42256-020-00273-z
Wang, M., and Zhu, W. (2018). Pore-scale study of heterogeneous chemical reaction for ablation of carbon fibers using the lattice Boltzmann method. Int. J. Heat. Mass Transf. 126, 1222–1239. doi:10.1016/j.ijheatmasstransfer.2018.05.133
Wang, W., Kravchenko, A. N., Smucker, A. J. M., Liang, W., and Rivers, M. L. (2012). Intra-aggregate pore characteristics: X-ray computed microtomography analysis. Soil Sci. Soc. Am. J. 76, 1159–1171. doi:10.2136/sssaj2011.0281
Wang, W., Kravchenko, A. N., Smucker, A. J. M., and Rivers, M. L. (2011). Comparison of image segmentation methods in simulated 2D and 3D microtomographic images of soil aggregates. Geoderma 162, 231–241. doi:10.1016/j.geoderma.2011.01.006
Wang, W., Zhang, Y., and Li, H. (2021). Quantification of soil structure via synchrotron X-ray tomography after 22 years of fertilization. Eur. J. Soil Sci. 72, 2115–2127. doi:10.1111/ejss.13108
Wieland, R., Ukawa, C., Joschko, M., Krolczyk, A., Fritsch, G., Hildebrandt, T. B., et al. (2021). Use of deep learning for structural analysis of computer tomography images of soil samples. R. Soc. Open Sci. 8, 201275. doi:10.1098/rsos.201275
Wildenschild, D., Hopmans, J. W., Rivers, M. L., and Kent, A. J. R. (2005). Quantitative analysis of flow processes in a sand using synchrotron-based X-ray microtomography. Vadose Zo. J. 4, 112–126. doi:10.2113/4.1.112
Withers, P. J., Bouman, C., Carmignato, S., Cnudde, V., Grimaldi, D., Hagen, C. K., et al. (2021). X-ray computed tomography. Nat. Rev. Methods Prim. 1, 18. doi:10.1038/s43586-021-00015-4
Xu, J., and Louge, M. Y. (2015). Statistical mechanics of unsaturated porous media. Phys. Rev. E 92, 062405. doi:10.1103/physreve.92.062405
Xu, Z., Valdes, C., and Clarke, J. (2018). Existing and potential statistical and computational approaches for the analysis of 3D CT images of plant roots. Agronomy 8, 71. doi:10.3390/agronomy8050071
Yang, Y., Wu, J., Zhao, S., Han, Q., Pan, X., He, F., et al. (2018). Assessment of the responses of soil pore properties to combined soil structure amendments using X-ray computed tomography. Sci. Rep. 8, 695–710. doi:10.1038/s41598-017-18997-1
Young, I. M., and Crawford, J. W. (2004). Interactions and self-organization in the soil-microbe complex. Sci. (80) 304, 1634–1637. doi:10.1126/science.1097394
Young, I. M., Crawford, J. W., and Rappoldt, C. (2001). New methods and models for characterising structural heterogeneity of soil. Soil Tillage Res. 61, 33–45. doi:10.1016/s0167-1987(01)00188-x
Young, I. M., and Ritz, K. (2000). Tillage, habitat space and function of soil microbes. Soil Tillage Res. 53, 201–213. doi:10.1016/s0167-1987(99)00106-3
Yu, Z., Leng, S., Li, Z., and McCollough, C. H. (2016). Spectral prior image constrained compressed sensing (spectral PICCS) for photon-counting computed tomography. Phys. Med. Biol. 61, 6707–6732. doi:10.1088/0031-9155/61/18/6707
Keywords: bulk density, machine learning, organic carbon, pore size distribution, root morphology, synchrotron
Citation: Ghosh T, Maity PP, Rabbi SMF, Das TK and Bhattacharyya R (2023) Application of X-ray computed tomography in soil and plant -a review. Front. Environ. Sci. 11:1216630. doi: 10.3389/fenvs.2023.1216630
Received: 04 May 2023; Accepted: 03 October 2023;
Published: 17 October 2023.
Edited by:
Gilles Colinet, University of Liège, BelgiumReviewed by:
Marko Černe, Institute of Agriculture and Tourism, CroatiaSidhu Murmu, Bidhan Chandra Krishi Viswavidyalaya, India
Copyright © 2023 Ghosh, Maity, Rabbi, Das and Bhattacharyya. This is an open-access article distributed under the terms of the Creative Commons Attribution License (CC BY). The use, distribution or reproduction in other forums is permitted, provided the original author(s) and the copyright owner(s) are credited and that the original publication in this journal is cited, in accordance with accepted academic practice. No use, distribution or reproduction is permitted which does not comply with these terms.
*Correspondence: Pragati Pramanik Maity, pragati.iari@gmail.com