Identification of key brittleness factors and multi-scenario analysis of the water-energy-food-ecology nexus vulnerability based on NRS-BN
- 1College of Economics and Management, Nanjing Forestry University, Nanjing, China
- 2Academy of Chinese Ecological Progress and Forestry Development Studies, Nanjing Forestry University, Nanjing, China
Water, energy and food are the basic resources on which human beings depend for survival. With the intensification of human activities, the demand for resources represented by water, energy and food continues to increase, resulting in increasing pressure on the ecological environment, and the vulnerability of water, energy, food and ecosystem becomes increasingly prominent. Identifying the critical vulnerability factors of the water-energy-food-ecology nexus and formulating targeted management measures have become the key to achieving sustainable development. This paper innovatively proposes to study the water-energy-food-ecology nexus from the perspective of vulnerability for the first time, in which the vulnerability evaluation index system of the water-energy-food-ecology nexus is firstly constructed based on the VSD framework, and the attribute reduction is carried out using neighborhood rough sets. Then, a Bayesian network model is built and parameter learning is performed by combining machine learning and expert experience. Finally, different scenarios are set up to identify the key factors that hinder the vulnerability reduction of the water-energy-food-ecology nexus and obtain the vulnerability probability of the nexus under different scenarios using forward and backward inference and sensitivity analysis of Bayesian networks, overcoming the drawback that many prediction models cannot achieve diagnostic inference. The results show that: 1) from 2008 to 2019, the overall vulnerability of the water-energy-food-ecology nexus in the Yangtze River Economic Belt is low. 2) The key factors at the indicator level that hinder the vulnerability reduction of the water-energy-food-ecology nexus mainly include the storage capacity of water conservancy projects, wastewater discharge per 10,000-yuan GDP, and water consumption per 10,000-yuan GDP, and the subsystem level is water, food, energy and ecology system, in that order. 3) The reduction in vulnerability within an individual subsystem can have a beneficial impact on reducing vulnerability within the water-energy-food-ecology nexus. However, this reduction may also lead to an increase in vulnerability within other subsystems. Therefore, in the process of developing water, energy, food, and ecology system, high priority should be given to the coordinated development of all four.
1 Introduction
Water, energy, and food are indispensable resources for human survival and development, as well as the key to national economic development, and their stable supply is essential for the smooth operation of society (Zhang et al., 2022). The concept of the “water-energy-food” nexus was first introduced in Bonn, Germany, in 2011, which clarified the complex relationship between water, energy and food systems (Wang et al., 2021). At present, scholars at home and abroad have explored and analyzed the impact of resources on regional economic development (Xu et al., 2021) and the sustainable development paths of resources (Luxon et al., 2018) at the national (White et al., 2018), regional (Markantonis et al., 2019) and urban (Li et al., 2016) levels, focusing on the social and natural properties of the water-energy-food nexus. Water, energy and food are relatively independent and inextricably coupled feeder systems, and developing one system often requires the consumption of resources of the other two systems. For example, the transportation of water resources and the processing of food require large amounts of energy. Also, the extraction of energy and the growth of food cannot be achieved without the support of water resources (Ma et al., 2021). However, these processes are often also closely related to the ecology system. The United Nations Economic Commission for Europe (UNECE) states that the resources and services provided by ecosystems are an essential guarantee for the security of the water-energy-food nexus and a necessary basis for achieving sustainable regional development (Wang et al., 2022). Currently, ecosystems have become an important indicator for measuring sustainability (Reyers and Selig, 2020), adaptability (Zhi et al., 2020), security (Ravar et al., 2020; Cansino-Loeza et al., 2022), and coupling coordination (Luo et al., 2022) of water-energy-food nexus (Ji et al., 2023). Urbanization and industrialization, as driving factors, not only increase the continuous consumption of resources, but also put tremendous pressure on the ecological environment, reducing the self-regulation and self-repair capacity of the ecosystem, which in turn hinders water, energy, food, and ecosystem security (Wang et al., 2021), making water, energy, food and ecology inextricably intertwined. As a result, a complex and dynamic water-energy-food-ecosystem is formed. Therefore, based on the importance of ecology to the water-energy-food system, the ecology system should be included in the water-energy-food nexus to understand the linkages among water, energy, food, and ecosystems from a more comprehensive perspective, and the concept of water-energy-food-ecology nexus is proposed on this basis. At this stage, studies on the water-energy-food-ecology nexus have mainly focused on developing countries with unstable social development, scarce natural resources, weak concepts of sustainable development, and poor ecological environments (Shi et al., 2020a). They have mainly focused on exploring resource sector management strategies (Howells et al., 2013) and addressing the resilience of social-ecological systems to external shocks (Schlör et al., 2018), and other frontier issues such as the value of services in the water-energy-food nexus (Sun and Xie, 2020). The research methods include life cycle assessment (Armengot et al., 2021), computable general equilibrium models (Chen et al., 2020), Bayesian network models (Shi et al., 2020b). For example, Sun and Xie (2020) established the “water-energy-food” service value accounting system based on the concept of the value of ecosystem services, and calculated the service value of “water-energy-food” on social economy and natural ecology and its linkage relationship in Guizhou Province during 2013–2017. The water-energy-food-ecology nexus has become a theory and tool to alleviate regional water, energy, food, and ecological tensions, as well as an effective method to achieve more efficient resource use (Vanham, 2016).
Water-energy-food-ecology nexus systems are enormous and complex. Vulnerability is the inherent attribute (Wang and Fu, 2019). In recent years, with the massive gathering of population and industries, the increasing shortage of water resources, fluctuations in food supply, dramatic increase in energy demand, and damage to ecological functions have become increasingly severe, and the water, energy, food, and ecological nexus systems have emerged with prominent vulnerability characteristics. Vulnerability is a comprehensive concept that includes risk, sensitivity, adaptability, resilience, resilience and other related elements, and there is no clear boundary between the components (Yang et al., 2019). The widely accepted elements of vulnerability are exposure, sensitivity, and adaptive capacity, as indicated in the IPCC report (IPCC, 2001). Based on the existing studies on the concept of vulnerability, this paper considers that the vulnerability of the water-energy-food-ecology nexus means that the structure, state and function of the system change toward the trend of dysfunction and subsequently present an unstable state under the influence of the disturbance of external environment such as human activities and natural conditions and the unbalanced development of the internal system. At this stage, most studies on the vulnerability of the water-energy-food-ecology nexus have explored the impact of changes in internal and external conditions on the vulnerability of individual subsystems such as water resources and ecology from the perspectives of climate, production and supply of resources (Yao et al., 2019), and optimal regulation of resources (Zhou et al., 2019). For example, Zhou et al. (2019) proposed a specific plan to control the vulnerability of groundwater resources under climate change through the assessment and scenario simulation of water resources vulnerability in Chongqing. Wang (2017) built a vulnerability assessment model of the food supply chain network and explored the vulnerable links in the food supply chain network. Song et al. (2021) analyzed the vulnerability of the energy system in the process of coping with climate change from different aspects, and put forward corresponding development suggestions for the development of China’s energy system in coping with climate change. These studies are conducive to the sustainable use of individual resources. However, to a certain extent, they ignore the interrelationships between the four, which is not conducive to the integrated management and regulation of the water-energy-food-ecology nexus, and only a few scholars have paid attention to this issue and evaluated the vulnerability of it from the perspective of complex systems (Chen et al., 2018). In addition, in the construction of the evaluation index system, due to the influence factors of the water-energy-food-ecology nexus exist not only within the nexus, but also in the external systems such as social and economic, the evaluation index system involves a vast range and content. Therefore, building an objective and comprehensive evaluation index system is challenging, and it is easy to ignore the dynamic linkage and degree of mutual influence between the indicators, which to a certain extent will increase the complexity of the prediction model. Forecasting research is still mainly focused on exploring the consumption of resources such as water, energy and food, as well as the impact on ecology and social environment. Fewer studies have been conducted to forecast vulnerability in the form of probability from the perspective of scenario analysis.
Therefore, this paper firstly constructs a vulnerability evaluation index system of the water-energy-food-ecology nexus based on the VSD framework which decomposes system vulnerability into three elements of system exposure, sensitivity and adaptive capacity, and uses neighborhood rough sets for attribute reduction to eliminate redundant indicators. Then, a Bayesian network is constructed based on machine learning and expert experience training, and its parameters are learned. Finally, the key vulnerability factors are identified, and different scenarios are set to perform Bayesian network inference. This paper introduces the Bayesian network into the vulnerability study of the water-energy-food-ecology nexus in the Yangtze River Economic Belt from the perspective of vulnerability. By using Bayesian networks with the features of sensitivity analysis, forward inference and backward inference to carry out prediction research, the vulnerability probability of the nexus under different scenarios can be obtained, overcoming the drawback that many prediction models cannot achieve diagnostic inference, and providing a reference for relevant departments to formulate strategic development plans for water, energy, food and ecology systems.
2 Methods and materials
2.1 Overview of the study area
The Yangtze River Economic Belt covers 11 provinces and cities, as is shown in Figure 1, including Shanghai, Jiangsu, Zhejiang, Anhui, Jiangxi, Hubei, Hunan, Chongqing, Sichuan, Guizhou, and Yunnan, with an area of about 2.05 million km2 and a GDP share of more than 40% of the country, making it one of the regions with the strongest comprehensive strength in China (He et al., 2019). With the constant advancement of industrialization and urbanization, the social economy has developed rapidly and people’s living standards have been greatly improved, but at the same time, problems such as resource shortage and ecological environment degradation have emerged. In terms of water resources, the regional distribution of water resources is uneven, the local water supply contradictions are prominent, and there are water quality and resource water shortages (Kong et al., 2021); in terms of energy supply and consumption, the total energy consumption in the Yangtze River Economic Belt accounts for about 36.8% of the country, but the primary energy production is low, mainly relying on the transfer and import from outside the province. What’s more, there are problems in ensuring energy security and promoting green and low-carbon energy transformation. In food, it is more difficult to balance food supply and demand, structural contradictions are increasingly prominent, and food production capacity is lower than the national average (Hu et al., 2019); in ecology, ecological land is occupied, air pollution is obvious, and ecological and environmental risks are prominent. Overall, the water-energy-food-ecology nexus and its subsystem security problems in the Yangtze River Economic Belt are becoming increasingly prominent and have emerged with obvious vulnerability characteristics which restrict the sustainable development. Therefore, it is crucial to investigate the vulnerability of the water-energy-food-ecology nexus in the Yangtze River Economic Belt to promote sustainable development in the region.
2.2 Data resources
The data involved in this study are derived from the 2009–2020 China Statistical Yearbook, China Energy Statistical Yearbook, China Environmental Statistical Yearbook, China Soil and Water Conservation Bulletin, and provincial and municipal statistical yearbooks, and some missing data are obtained by interpolation of adjacent years.
2.3 Research methods
The research in this paper is broadly divided into the following steps: the first step is to construct a vulnerability evaluation index system of the water-energy-food-ecology nexus based on the VSD framework and use the neighborhood rough set theory to approximate the attributes of the original index system; the second step is to train the Bayesian network structure and perform parameter learning based on machine learning and expert experience, and use Netica 5.18 to visualize the Bayesian network; In the third step, the key influencing factors are identified using sensitivity analysis and Bayesian network inference is performed. The specific process is shown in Figure 2.
2.3.1 Construction of vulnerability evaluation index system of the water-energy-food-ecology nexus
The VSD framework decomposes system vulnerability into three dimensions, which are exposure, sensitivity and adaptive capacity (Wang and Zhang, 2021). Among them, exposure refers to the extent to which the system is affected by uneven internal development and disturbed by external conditions such as human activities (Chen et al., 2018); sensitivity refers to the extent to which the structure, state and function of the system are affected; and adaptive capacity refers to the system’s ability to recover itself. On this basis, this paper combined the current situation of water, energy, food and ecology resources in the Yangtze River Economic Belt and the acquisition of data and information to construct a vulnerability evaluation index system for the water-energy-food-ecology nexus, which is shown in Table 1. Among them, the larger the value of the positive index and the smaller the value of the negative index, the more vulnerable the water-energy-food-ecology nexus is.
2.3.2 Neighborhood rough set
Rough set theory was initially proposed by Z. Pawlakab (1997), which is centered on knowledge simplification and can effectively eliminate unimportant and redundant information in evaluation object indicators, and has been widely used in water resources, ecology and other fields in recent years. However, the classical rough set is limited to discrete data, which may lead to inaccurate final results. In response, Hu et al. (2008) introduced the neighborhood model into rough sets based on the existing research. Neighborhood rough sets can handle discrete and continuous data and attribute reduction for symbolic data and their mixed data. The following are some basic definitions of neighborhood rough sets:
Definition 1. For any set
In the formula,
Definition 2.
Definition 3. A non-empty finite set
Definition 4. The neighborhood decision table is mainly described by the basic attributes of objects and their attribute values. A neighborhood decision table can be expressed as:
Definition 5. In the neighborhood decision table, let
Definition 6. The dependence of decision attribute
Definition 7. Given the neighborhood decision table
When
2.3.3 Bayesian network
2.3.3.1 The basic principle of Bayesian network
Bayesian network is a probabilistic network model based on Bayesian causal inference, first proposed by American scholar Pearl. It can effectively combine priori knowledge and sample data, synthesize the practical expertise of domain experts, and organically unify subjectivity and objectivity, facilitating qualitative and quantitative analysis and avoiding the overfitting of data. Bayesian network is a directed acyclic graph model, the nodes represent the probability distribution of variables, the directed edges between nodes represent the “causality” represented by the conditional probability table, and the nodes are connected into a network by Bayesian conditional probability formula. The conditional probability distribution of network nodes is obtained by prior knowledge and observation data. When adjusting the probability distribution of some nodes, combined with Bayesian formula, Bayesian network can calculate the posterior conditional probability distribution of other nodes to achieve prediction or diagnostic analysis. Therefore, this paper introduces Bayesian network into the vulnerability study of water-energy-food-ecology system in the Yangtze River Economic Belt, carries out prediction research in the form of probability, and uses the characteristics of Bayesian network such as sensitivity analysis, forward reasoning and backward reasoning to screen key impact factors and explore the potential relationship between key impact factors and target nodes, which overcomes the drawback that many prediction models cannot achieve diagnostic inference.
2.3.3.2 Construction of Bayesian network model and parameter learning
Bayesian network structure learning mainly includes structure learning methods based on independence tests, scoring, and search. Among them, the structure learning method based on the independence test is more intuitive and has the advantages of small computational effort and fast convergence, but the accuracy of the learned network structure is lower. The scoring and search-based structure learning method constructs the network according to specific search strategies and scoring criteria in the structure space of all nodes (Zhang et al., 2014), and its learning goal is to search for network structures with high scores, so the accuracy is better than the independence-based structure learning method. The method mainly consists of selecting the network structure scoring function and the network structure learning algorithm. This paper proposes to construct a Bayesian network using the BIC scoring criterion and Hillclimb algorithm to process the sample data better. BIC (Bayes information criterion) approximates the marginal likelihood function, which is easy to use and helps avoid overfitting, and is the most commonly used scoring function in practice. Hillclimb algorithm starts the search from the initial model, and during the search process, the current model is first modified locally with the search operator to obtain a series of candidate models; then the score of each candidate model is calculated and the best candidate model is compared with the current model. If the best candidate model scores higher, it is used as the next current model, and the search continues; otherwise, stop searching and return to the current model (Li et al., 2019).
Parameter learning of Bayesian network models mainly refers to analyzing sample data to find the conditional probability distribution among the nodes with known network structure. Parameter learning in Bayesian networks can be divided into two cases: data-complete and data-incomplete, when data-complete is usually learned by Bayesian estimation and maximum likelihood method, and when data-incomplete can be learned by EM algorithm (Guan and He, 2016). Compared to the maximum likelihood method, Bayesian estimation treats the parameters to be estimated as random variables that conform to some prior probability distribution. The process of observation of the sample is to transform the previous probability density into the posterior probability density, which corrects the initial estimates of the parameters using the information from the sample and applies to the case of a small sample size. Therefore, this paper proposes to use Bayesian estimation for parameter learning and is implemented by the R language bnlearn program package.
2.3.3.3 Bayesian network model sensitivity analysis
Sensitivity analysis has been widely used in various fields of society, such as medical diagnosis and machine fault diagnosis. In Bayesian networks, Sensitivity Analysis (SA) is mainly used to quantify the impact of parameter changes on the target nodes by changing the parameter values of the input nodes. In Netica 5.18, sensitivity analysis is often performed using Mutual Information (MI) based on entropy reduction and Variance of Belief (VB) based on variance reduction (Shi et al., 2022) with the following equations:
Where,
2.3.4 Attribute reduction of evaluation indexes and data pre-processing
From the index system constructed above, it can be seen that the water-energy-food-ecology nexus is vulnerable to the joint influence of many internal and external factors, and the possible duplication and redundancy of information among the indexes will have an impact on the research results of the vulnerability of the water-energy-food-ecology nexus. In this paper, without changing the structure and classification ability of the indexes, we apply the neighborhood rough set theory to simplify the attributes of the original index system, which reduces information duplication, improves the accuracy of the evaluation work, and at the same time reduces the complexity of the prediction work. Both conditional and decision attributes are required when using neighborhood rough sets to approximate the evaluation index system. Among them, the decision attributes also need to be discretized data. Therefore, in this paper, the water-energy-food-ecology nexus vulnerability values are calculated using the TOPSIS model (Yue et al., 2019), and the discretization results are used as decision attributes using K-means clustering for discretization.
3 Results and analysis
3.1 Attribute reduction of the indexes
According to the results of discretization, the minimum approximate set is obtained after several times of parameter debugging. Among them, the approximate set of water subsystem is
3.2 Construction of Bayesian network
3.2.1 Structure of Bayesian network and parameter learning
First, the initial Bayesian network was constructed and parameters were learned using the R studio bnlearn package to obtain the initial conditional probabilities (Table 3). Then, the trained Bayesian network was visualized in Netica 5.18 (Figure 3), and it can be seen that the vulnerability of the water-energy-food-ecology nexus in the Yangtze River Economic Belt is at a low level overall and has a good development trend from 2008 to 2019. However, the probability of a vulnerability being at a high level is still 41.1%, which means there is still more room for improvement in future water, energy, food and ecological resource management. From the subsystem level, the probability that the vulnerability of the water resources subsystem is in a low state is 51.9% at this stage, which is slightly higher than 50%, indicating that the development situation of the water resources subsystem has been more complicated in recent years and the vulnerability status is not optimistic. The probability that the vulnerability of the energy subsystem is in a high state is 54.2%, which is about 10% higher than the low state of vulnerability, and the overall development situation is not good. The food and ecology subsystems perform better, with probabilities of low vulnerability of 80.4% and 74.0%, respectively, indicating that these two systems have better adaptive capacity and higher system security, and are less vulnerable to uneven internal development and external environmental changes. In addition, the complex relationship between the indicators interacting and constraining each other can be seen in Figure 3, and the subsystems are not entirely independent. The indicators can influence the vulnerability state of the subsystem and, thus, the overall vulnerability of the water-energy-food-ecology nexus.
3.2.2 Validation of Bayesian network
After the structure and parameters of the Bayesian network are learned, the validity of the model needs to be tested (Zhang and Sheng, 2019; Wang et al., 2021). In this paper, we use “water-energy-food-ecology nexus vulnerability” as the target variable and use ten-fold cross-validation to verify the validity of the model and obtain the confusion matrix shown in Table 4. It can be seen that the overall accuracy of the Bayesian network constructed in this paper is 77.2% in predicting the vulnerability of the water-energy-food-ecology nexus, which indicates its high accuracy and reliability.
3.3 Bayesian network inference
3.3.1 Sensitivity analysis
In Bayesian networks, the essence of sensitivity analysis is also probabilistic inference, which can infer the relationship between one variable and other variables, whereby the degree of influence of certain probabilistic parameters on the outcome is analyzed, and the cause that is most sensitive to the outcome is identified. In this paper, it is mainly used to explore the factors that have a greater influence on the vulnerability of the water-energy-food-ecology nexus at other linkage nodes to target the problems in the development of the water-energy-food-ecology nexus. To reflect the influence of each variable on the target variable, the target node “water-energy-food-ecology nexus vulnerability” was used as the analysis variable, and the sensitivity analysis of other variables was conducted using Netica software. As seen from Figure 4, the sensitivity analysis results based on mutual information and belief variance show the same trend, the larger the mutual information and belief variance, the higher the sensitivity of the target variables to them. From the subsystem level, the water resources subsystem is the key factor affecting the vulnerability of the water-energy-food-ecology nexus, which is also consistent with the existing findings (Scott et al., 2015; Shi et al., 2022), followed by the food and energy subsystems with mutual information of 0.15995 and 0.01981 and belief variances of 0.05161 and 0.00675, respectively, which means that the food and energy subsystems are the second and third influencing factor of the water-energy-food-ecology nexus, respectively. The ecology subsystem has the least influence, whose mutual information is 0.00423 and belief variance is 0.00141. From the indicator level, the storage capacity of water conservancy project, wastewater discharge per 10,000-yuan GDP and water consumption per 10,000-yuan GDP have a greater impact on the vulnerability of the water-energy-food-ecology nexus.
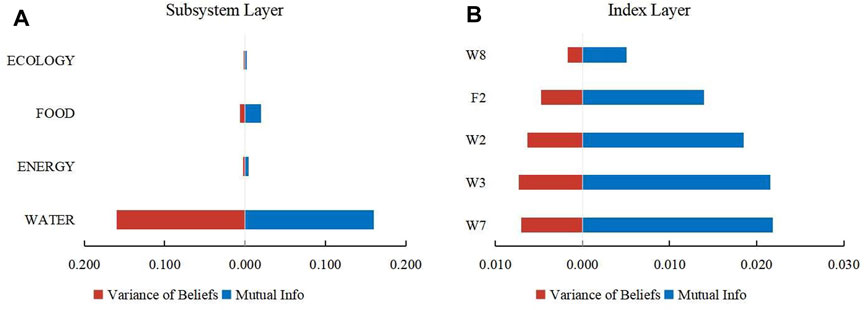
FIGURE 4. Sensitivity Analysis (A) Sensitivity analysis of subsystem layer (B) Sensitivity analysis of index layer.
3.3.2 Forward reasoning analysis
The forward reasoning is mainly used to infer the probability values of the water-energy-food-ecology nexus in different vulnerability states for situations already existing, such as large industrial wastewater effluent discharge and increased water consumption. Based on the results of sensitivity analysis, this paper selects the top three indicators from the subsystem level and the indicator level, sets different scenarios, and uses forward reasoning to examine the degree of impact on the vulnerability of the water-energy-food-ecology nexus when some indicators are optimized. Scenario 1 sets the probability that the water resources subsystem is in a low vulnerability state to 100%; Scenario 2 sets the probability that the food subsystem is in a low vulnerability state to 100%; Scenario 3 sets the probability that the energy subsystem is in a low vulnerability state to 100%; Scenario 4 sets the probability that the storage capacity of water conservancy project is in a low vulnerability state to 100%; Scenario 5 is set as the probability that Wastewater discharge per 10,000-yuan GDP is in a lower vulnerable state is 100%; Scenario 6 is set as the probability that the water consumption per 10,000-yuan GDP is in a lower vulnerable state is 100%. When setting the relevant scenarios, the socio-economic development level of the Yangtze River Economic Belt, scientific and technological innovation capacity, and the target planning of policy documents such as the “14th Five-Year Plan” and “14th Five-Year Plan” for water security were all taken into consideration.
The changes of the probability values of the water-energy-food-ecology nexus system being in different vulnerable states under these six different scenarios are shown in Table 5. As can be seen from the table, when the probability of the water resources subsystem being in a lower vulnerability state is 100%, the probability of the water-energy-food-ecology nexus system being in a lower vulnerability state has the largest rate of change, increasing by 21.9%. Therefore, the stable development of the water resources subsystem will rapidly decrease the vulnerability of the nexus and, vice versa, constrain the coordinated development of water, energy, food, and ecosystems. The decrease in the vulnerability of food and energy subsystems leads to a 4.1 percentage point increase in the probability that the water-energy-food-ecology nexus is in a lower vulnerability state, indicating that the decrease in the vulnerability of the food and energy subsystems also has a role in the decrease in the vulnerability of the water-energy-food-ecology nexus. In addition, increasing the storage capacity of water conservancy projects increases the probability of reducing the vulnerability of the water-energy-food-ecology nexus by 16%, indicating the importance of promoting water construction and optimizing water allocation. Moreover, reducing wastewater discharge per 10,000-yuan GDP and water consumption per 10,000-yuan GDP can increase the probability of vulnerability reduction of the water-energy-food-ecology nexus system by 5.9% and 4.5%, respectively. It shows that strict water resources regulation, increased treatment of urban sewage and industrial wastewater, and resource utilization of wastewater can improve water resources utilization efficiency and reduce water resources consumption, which can effectively promote the stable development of the water-energy-food-ecology nexus.
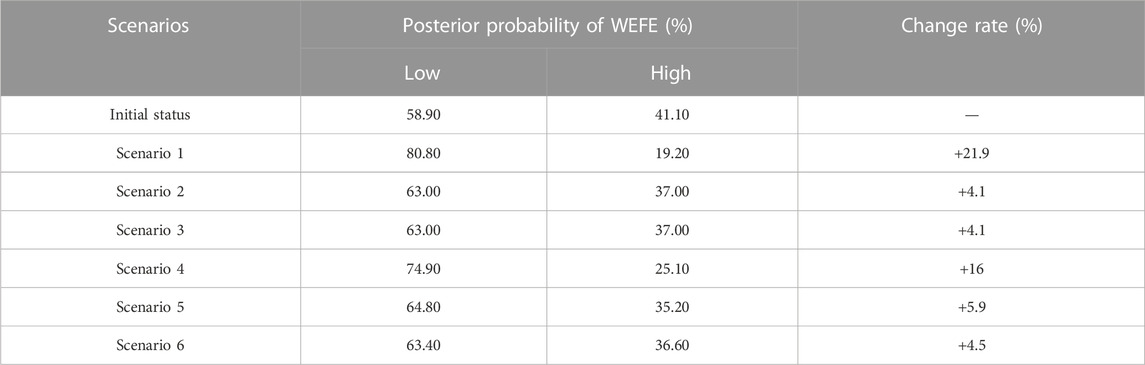
TABLE 5. Changes in the probability values of vulnerability of the water-energy-food-ecology nexus under different scenarios.
In addition, as shown in Table 6, the probability of a subsystem being in a lower vulnerability state changes with the probability of other subsystems. For example, when scenario 1 occurs, the probability of the energy, food, and ecosystem subsystem vulnerability being in a lower vulnerability state change to 46.5%, 75.6%, and 70.7%. The energy subsystem improves by 0.7%, while the food and ecology subsystem decrease by 4.8% and 3.3%, respectively. This also occurs in scenario 3. The probability of the food and ecology subsystems being in a lower state of vulnerability also increase to varying degrees when scenario 2 occurred. This suggests that although the decrease in the vulnerability of individual subsystems can contribute positively to the decrease in the vulnerability of the water-energy-food-ecology nexus, promoting the sustainability of the system, it may also cause an increase in the vulnerability of other subsystems. Therefore, it is necessary to pay attention to the coordinated development of the four. For example, while optimizing water resource allocation, improving water resource utilization efficiency, adjusting energy structure, and reducing energy consumption intensity, we also need to pay attention to the use of pesticides and chemical fertilizers and strengthen ecological protection.

TABLE 6. Probability of water, energy, food, and ecosystem being in a less vulnerable state under different scenarios and their changes (%).
3.3.3 Backward reasoning analysis
In 2020, President Xi pointed out at the symposium on comprehensively promoting the development of the Yangtze River Economic Belt that promoting the development of the Yangtze River Economic Belt requires the integrated consideration of the organic linkage of various aspects such as water ecology and water culture, and it is necessary to optimize the industrial layout, strengthen the protection and restoration work of the ecological and environmental systems, and pay great attention to the issue of food security at the same time. In this regard, this paper takes the vulnerability of the water-energy-food-ecology nexus as the target node and sets scenario 7, that is, the probability that the water-energy-food-ecology nexus is in a lower vulnerability state is 100%, so as to obtain the probability of each node, as shown in Figure 5.
As can be seen from Figure 5, the probabilities of vulnerability states of water resources, energy, food, and ecological subsystems all change accordingly, and the probabilities of being in lower vulnerability states are 71.1%, 49%, 85.9, and 75.4%, respectively. When the target node is in the ideal state, that is, the probability of the water-energy-food-ecology nexus being in a lower vulnerability state is 100%, the water resources subsystem changes the most, the food and energy subsystems follow, and the ecology subsystem changes the least, with change rates of 19.2%, 5.5%, 3.2%, and 1.4%, respectively. In other words, the water resources subsystem is the most prominent factor affecting the vulnerability of the water-energy-food-ecology nexus at the subsystem level. Therefore, to reduce the vulnerability of the water-energy-food-ecology nexus in the Yangtze River Economic Belt, the primary focus must be on how to reduce the vulnerability of the water resources subsystem. Since the food, energy and ecology subsystems also have an impact on the whole vulnerability, the role of these three cannot be ignored either. In addition, changes in the vulnerability state of the water-energy-food-ecology nexus have somewhat different effects on different indicators. Compared with the initial state, the most obvious change is the wastewater discharge per 10,000-yuan GDP, followed by the storage capacity of water conservancy projects, water consumption of 10,000 Yuan GDP, and then the per capita grain sown area. The probability of being in a lower vulnerability state increases by 6.8%, 5.9%, 5.7% and 4.5%, respectively. From this, the vulnerability of the water-energy-food-ecology nexus is mainly influenced by the increase in water consumption, large discharge of wastewater, and decrease in per capita grain sown area during the economic development process. It is worth noting that although the changes in the other initial nodes are small, they can trigger larger changes in the intermediate nodes, which in turn trigger changes in the vulnerability of the water-energy-food-ecology nexus. Therefore, good coordination is needed for future development.
3.4 Discussion
Compared with the existing research on the water-energy-food-ecology nexus in the world, this paper focuses more on the vulnerability of the nexus. At present, the research on water-energy-food-ecology nexus is still mainly focus on subsystem research, and more attention is paid to water resources and ecology. For example, In terms of water resources, Kong et al. (2023) analyzed the adaptability between water pollution and advanced industrial structure. Yuan et al. (2023) improved the method of calculating the value of water resources and assessed the efficiency and quality of water use in Hubei Province. In terms of ecosystems, Yuan et al. (2022) analyzed the coordination degree of the industrial-ecological economy in the Yangtze River Economic Belt based on Lotka-Volterra model. However, none of these studies paid attention to the vulnerability of water-energy-food-ecology nexus. Therefore, this paper proposes to study water-energy-food-ecology nexus from the perspective of vulnerability, and constructs the overall framework of water-energy-food-ecology nexus vulnerability research, which widens the existing research perspective of water-energy-food-ecology nexus to some extent. Additionally, compared to previous research findings, this paper’s conclusions are more beneficial in reducing fragmented comprehension of each element of the water-energy-food-ecology nexus in various departments, which offers a fresh concept for regional governance.
In addition, in terms of research methods, the Bayesian network in this paper also has certain advantages. Compared with existing methods such as system dynamics (Ji et al., 2023), coupling coordination degree model (Wu et al., 2023) and data enveloping analysis (Liu et al., 2023), the Bayesian network adopted in this paper can model the complex causal relationship in the system better, and the form of probability distribution can effectively express the uncertainty of variables. What’s more, it can screen out the key factors affecting the vulnerability of water-energy-food-ecology through sensitivity analysis, and obtain the vulnerability state and corresponding probability of key nodes through forward and backward reasoning, which solves the defect that many existing methods cannot carry out diagnostic reasoning, and makes up for the deficiency of existing research.
4 Conclusion
In this paper, firstly, a vulnerability evaluation index system of water-energy-food-ecology nexus was constructed based on the VSD framework, and the attribute approximation was performed using neighborhood rough sets. Then, a Bayesian network model was built by structure learning and parameter learning. Finally, sensitivity analysis, forward reasoning, and backward reasoning were performed using Bayesian networks to derive the key influencing factors that hinder the vulnerability reduction of the water-energy-food-ecology nexus, and targeted suggestions were made. The main conclusions of this paper are as follows:
From 2008 to 2019, the development of the water-energy-food-ecology nexus in the Yangtze River Economic Belt is relatively good, and the vulnerability is at a low level overall, especially for the food and ecological subsystems, which have better adaptive capacity and higher security. However, the vulnerability status of the water and energy subsystems is not optimistic, and the probability of the vulnerability of the water-energy-food-ecology nexus being at a high level is still 41.1%, indicating that there is still much room for improvement in the management of water, energy, food and ecosystems in the future. From the indicator level, the key factors that hinder the vulnerability reduction of the water-energy-food-ecology nexus at this stage mainly include the storage capacity of water conservancy projects, wastewater discharge per 10,000-yuan GDP, water consumption per 10,000-yuan GDP, and per capita grain sown area. At the subsystem level, the water resources subsystem is the main system affecting the vulnerability of the water-energy-food-ecology nexus, followed by the food and energy subsystems, and the ecological subsystem is the weakest. Although the decrease in the vulnerability of individual subsystems can contribute positively to the decrease in the vulnerability of the water-energy-food-ecology nexus and promote the sustainability of the nexus, it may also cause an increase in the vulnerability of other subsystems. These findings are significant to reduce the fragmented comprehension of each element of the water-energy-food-ecology nexus in various departments and remind managers that in the development process of water, energy, food, and ecosystems, the coordinated development of the four should be prioritized.
This paper has certain innovations and features. Considering the correlation between ecology and water, energy and food systems, the study of the water-energy-food-ecology nexus from the perspective of vulnerability is carried out for the first time and the whole framework of water-energy-food-ecology vulnerability research is constructed. To some extent, this will help broaden the existing research perspective of water-energy-food-ecology nexus and provide new ideas for regional governance. In addition, this paper uses neighborhood rough sets to simplify the attributes of indicators, which reduces the repetition of indicator information and makes the evaluation index system more reasonable. What’s more, the key influencing factors are screened by sensitivity analysis, and the potential relationship between key influencing factors and target nodes is explored by using forward and backward reasoning of Bayesian networks, which has certain scientific merit. At the same time, using Bayesian network to carry out water-energy-food-ecology nexus vulnerability prediction research in the form of probability can solve the defect that many existing prediction methods cannot achieve diagnostic inference, so as to open up a new path for water-energy-food-ecology nexus vulnerability prediction research.
There are certain limitations in this paper. For example, a static Bayesian network model is constructed in this paper, and in the future, we will consider adding the time factor to carry out a more profound study at the city level using dynamic Bayesian networks.
Data availability statement
The original contributions presented in the study are included in the article/Supplementary Material, further inquiries can be directed to the corresponding author.
Author contributions
YC: Writing–original draft, Writing–review and editing. YP: Writing–original draft. MG: Writing–review and editing.
Funding
The author(s) declare financial support was received for the research, authorship, and/or publication of this article. This study was supported by National Social Science Fund of China (Grant No. 21BGL181) to YC.
Conflict of interest
The authors declare that the research was conducted in the absence of any commercial or financial relationships that could be construed as a potential conflict of interest.
Publisher’s note
All claims expressed in this article are solely those of the authors and do not necessarily represent those of their affiliated organizations, or those of the publisher, the editors and the reviewers. Any product that may be evaluated in this article, or claim that may be made by its manufacturer, is not guaranteed or endorsed by the publisher.
References
Armengot, L., Beltrán, M. J., Schneider, M., Simón, X., and Pérez-Neira, D. (2021). Food-energy-water nexus of different cacao production systems from a LCA approach. J. Clean. Prod. 304, 126941. doi:10.1016/J.JCLEPRO.2021.126941
Cansino-Loeza, B., Munguia-Lopez, A. D., and Ponce-Ortega, J. M. (2022). A water-energy-food security nexus framework based on optimal resource allocation. Environ. Sci. Policy 133, 1–16. doi:10.1016/J.ENVSCI.2022.03.006
Chen, C. F., Feng, K. L., and Ma, H. (2020). Uncover the interdependent environmental impacts associated with the water-energy-food nexus under resource management strategies. Resour. Conservation Recycl. 160, 104909. doi:10.1016/j.resconrec.2020.104909
Chen, F., Li, Z. H., Dong, S. C., Ren, Y., Li, J. N., and Pavel, V. R. (2018). Evaluation of ecological vulnerability in gully - hilly region of Loess Plateau based on VSD Model-A case of Lintao county. J. Arid Land Resour. Environ. 32 (11), 74–80. doi:10.13448/j.cnki.jalre.2018.336
Chen, J., Yu, X., Qiu, L., Deng, M., and Dong, R. (2018). Study on vulnerability and coordination of Water-Energy-Food system in Northwest China. Sustainability 10, 3712. doi:10.3390/su10103712
Chen, W. Z., Chen, Y., and Feng, Y. Z. (2021). Assessment and prediction of water resources vulnerability based on a NRS-RF model: a case study of the song-liao river basin, China. Entropy 23 (7), 882. doi:10.3390/E23070882
Guan, X. D., and He, J. H. (2016). Prime farmland protection zoning based on bayesian network. J. Nat. Resour. 31 (06), 1061–1072. doi:10.11849/zrzyxb.20150639
He, G., Xia, Y. L., Qin, Y., Zhu, Y. N., and Wang, W. W. (2019). Evaluation of spatial-temporal dynamic change of water resources carrying capacity in the Yangtze River Economic Belt. Res. Soil Water Conservation 26 (01), 287–292+300. doi:10.13869/j.cnki.rswc.2019.01.042
Howells, M., Hermann, S., Welsch, M., Bazilian, M., Segerström, R., Alfstad, T., et al. (2013). Integrated analysis of climate change, land-use, energy and water strategies. Nat. Clim. Change 3, 621–626. doi:10.1038/nclimate1789
Hu, H. Z., Wang, J. L., Wang, Y., and Long, X. Y. (2019). Spatial-temporal pattern and influencing factors of grain production and food security at county level in the Yangtze River basin from 1990 to 2015. Resour. Environ. Yangtze Basin 28 (02), 359–367. doi:10.11870/cjlyzyyhj201902012
Hu, Q. H., Yu, D. R., and Xie, Z. X. (2008). Numerical attribute reduction based on neighborhood granulation and rough approximation. J. Softw. 3, 640–649. doi:10.3724/sp.j.1001.2008.00640
IPCC (2001). Climate change: impacts, adaptation and vulnerability. Cambridge, UK: Cambridge University Press.
Ji, J., Chen, J. F., Deng, M. H., Gu, Y., and Yan, X. D. (2023). Simulation and optimization of water-energy-food-ecology system for green development in the Yangtze River Economic Belt. Soft Sci. 37 (05), 105–114. doi:10.13956/j.ss.1001-8409.2023.05.14
Kong, Y., He, W. J., Shen, J. Q., Yuan, L., Gao, X., Ramsey, T. S., et al. (2023). Adaptability analysis of water pollution and advanced industrial structure in Jiangsu Province, China. Ecol. Model. 481 (481), 110365. doi:10.1016/J.ECOLMODEL.2023.110365
Kong, Y., He, W. J., Yuan, L., Zhang, Z. F., Gao, X., Degefu, D. M., et al. (2021). Decoupling economic growth from water consumption in the Yangtze River Economic Belt, China. Ecol. Indic. 123, 107344. doi:10.1016/j.ecolind.2021.107344
Li, D. L., Xu, W., Wu, D., and Xing, G. X. (2019). On learning method of Bayesian network structure. J. Nav. Univ. Eng. 31 (06), 61–64. doi:10.7495/j.issn.1009-3486.2019.06.012
Li, G. J., Li, Y. L., Jia, X. J., Du, L., and Huang, D. H. (2016). Establishment and simulation study of system dynamic model on sustainable development of water-energy-food nexus in beijing. Manag. Rev. 28 (10), 11–26. doi:10.14120/j.cnki.cn11-5057/f.2016.10.002
Liu, L. M., Chen, J. F., and Wang, C. B. (2023). Spatial and temporal characteristics and influencing mechanisms of water-energy-food-ecology vulnerability in the Yangtze River Economic Belt. Resour. Environ. Yangtze Basin 32 (08), 1628–1640. doi:10.11870/cjlyzyyhj202308007
Luo, W., Yang, X. Z., Yang, Y. F., and Cheng, S. (2022). Co-evolution of water-energy-food nexus in the Yellow River Basin and forecast of future development. Resour. Sci. 44 (3), 608–619. doi:10.18402/resci.2022.03.14
Luxon, N., Bekithemba, N., Charles, N., Tafadzwanashe, M., Sylvester, M., and Greenwell, M. (2018). The water-energy-food nexus: climate risks and opportunities in southern africa. Water 10 (5), 567. doi:10.3390/w10050567
Ma, Y., Li, Y., Zhang, Y., and Huang, G. H. (2021). Mathematical modeling for planning water-food-ecology-energy nexus system under uncertainty: a case study of the aral sea basin. J. Clean. Prod. 308, 127368. doi:10.1016/J.JCLEPRO.2021.127368
Markantonis, V., Reynaud, A., Karabulut, A., El Hajj, R., Altinbilek, D., Awad, I. M., et al. (2019). Can the implementation of the water-energy-food nexus support economic growth in the Mediterranean region? The current status and the way forward. Front. Environ. Sci. 7, 84. doi:10.3389/fenvs.2019.00084
Pawlakab, Z. (1997). Rough set approach to knowledge-based decision support. Eur. J. Operational Res. 99 (1), 48–57. doi:10.1016/S0377-2217(96)00382-7
Ravar, Z., Zahraie, B., Sharifinejad, A., Gozini, H., and Jafari, S. (2020). System dynamics modeling for assessment of water-food-energy resources security and nexus in gavkhuni basin in Iran. Ecol. Indic. 108, 105682. doi:10.1016/j.ecolind.2019.105682
Reyers, B., and Selig, E. R. (2020). Global targets that reveal the social-ecological interdependencies of sustainable development. Nat. Ecol. Evol. 4 (8), 1011–1019. doi:10.1038/s41559-020-1230-6
Schlör, H., Venghaus, S., and Hake, J.-F. (2018). The FEW-Nexus City index—measuring urban resilience. Appl. Energy 210, 382–392. doi:10.1016/j.apenergy.2017.02.026
Scott, C. A., Kurian, M., and Wescoat, J. L. (2015). “The water-energy-food nexus: enhancing adaptive capacity to complex global challenges,” in Governing the nexus. Editors K. Mathew, and A. Reza (Cham: Springer), 15–38.
Shi, H. Y., Luo, G. P., Zhen, H. W., Chen, C. B., Bai, J., and Liu, T. (2020a). Water ues analysis of Syr Darya river basin: based on “Water-Energy-Food-Ecology” nexus and Bayesian network. Acta Geogr. Sin. 75 (05), 1036–1052. doi:10.11821/dlxb202005011
Shi, H. Y., Luo, G. P., Zheng, H. W., Chen, C. B., Bai, J., Liu, T., et al. (2020b). Coupling the water energy food ecology nexus into a Bayesian network for water resources analysis and management in the Syr Darya River basin. J. Hydrology 581, 124387. doi:10.1016/j.jhydrol.2019.124387
Shi, Y. X., Liu, S. N., and Shi, H. Y. (2022). Analysis of the water-food-energy nexus and water competition based on a bayesian network. Water Resour. Manag. 36 (9), 3349–3366. doi:10.1007/S11269-022-03205-1
Song, M., Wang, H., and Wang, Y. (2021). Research on the vulnerability and adaptation measures of energy system to climate change. Energy China 201 43 (01), 26–32. doi:10.3969/j.issn.1003-2355.2021.01.005
Sun, J., and Xie, X. X. (2020). Estimation of water-energy-food (WEF) servicing values based on a case study on Guizhou province. Resources& Ind. 22 (05), 37–47. doi:10.13776/j.cnki.resourcesindustries.20200902.004
Vanham, D. (2016). Does the water footprint concept provide relevant information to address the water-food-energy-ecosystem nexus. Ecosyst. Serv. 17, 298–307. doi:10.1016/j.ecoser.2015.08.003
Wang, C. Y., and Zhang, H. (2021). Research on the vulnerability of the land system in yunnan province and its countermeasures. J. Anhui Agric. Sci. 49 (11), 80–84. doi:10.3969/j.issn.0517-6611.2021.11.021
Wang, D. Q. (2017). Research on Vulnerability assessment of food supply chain network. J. Shandong Coll. Agric. Eng. 34 (05), 5–8. doi:10.15948/j.cnki.37-1500/s.2017.05.002
Wang, H. R., Zhao, W. J., Deng, C. Y., and Yan, J. W. (2022). Analysis on issues of water-energy-food nexus. J. Nat. Resour. 37 (02), 307–319. doi:10.31497/zrzyxb.20220203
Wang, M., Zhu, Y. F., Gong, S. W., and Ni, C. Y. (2021). Spatiotemporal differences and spatial convergence of the water-energy-food-ecology nexus in northwest China. Front. Energy Res. 9, 665140. doi:10.3389/FENRG.2021.665140
Wang, R. X., and Fu, T. T. (2019). The evaluation of coupling system vulnerability of technology and finance based on the empirical research in Hubei province. Sci. Technol. Manag. Res. 39 (06), 35–40. doi:10.3969/j.issn.1000-7695.2019.06.006
Wang, Y., Zhao, Y., Wang, Y. Y., Ma, X. J., Bo, H., and Luo, J. (2021). Supply-demand risk assessment and multi-scenario simulation of regional water-energy-food nexus: a case study of the Beijing-Tianjin-Hebei region. Resour. Conservation Recycl. 174, 105799. doi:10.1016/J.RESCONREC.2021.105799
Wang, Y. J., Liu, Y. X., Song, S., and Fu, B. J. (2021). Research progress of the water-food-energy-ecosystem nexus. Adv. Earth Sci. 36 (7), 684. doi:10.11867/j.issn.1001-8166.2021.073
White, D. J., Hubacek, K., Feng, K. S., Sun, L. X., and Meng, B. (2018). The Water-Energy-Food Nexus in East Asia: a tele-connected value chain analysis using inter-regional input-output analysis. Appl. Energy 210, 550–567. doi:10.1016/j.apenergy.2017.05.159
Wu, Y. T., Guo, L. D., Jing, P. R., Huang, F., and Wang, H. X. (2023). Water-energy-food-ecology coupling and its spatiotemporal differentiation in five Central Asian countries. J. Res. arid areas 40 (4), 573–582. doi:10.13866/j.azr.2023.04.06
Xu, S. S., He, W. J., Yuan, L., Mulugeta, D. D., Yang, Y., and Li, H. (2021). The relationship between coordination degree of the water-energy-food system and regional economic development. Sustainability 13 (3), 1305. doi:10.3390/SU13031305
Yang, F., Ma, C., and Fang, H. J. (2019). Research progress on vulnerability: from theoretical research to comprehensive practice. Acta Ecol. Sin. 39 (02), 441–453. doi:10.5846/stxb201802020289
Yao, C. S., Yin, W., Huang, L., and Cui, H. F. (2019). Spatial-Temporal pattern and coupling coordination evolution of the vulnerability of food production and consumption ability in China. Econ. Geogr. 39 (12), 147–156. doi:10.15957/j.cnki.jjdl.2019.12.017
Yuan, L., Ding, L., He, W., Kong, Y., Ramsey, T. S., Degefu, D. M., et al. (2023). Compilation of water resource balance sheets under unified accounting of water quantity and quality, a case study of Hubei province. Water 15 (7), 1383. doi:10.3390/W15071383
Yuan, L., Li, R. Y., He, W. J., Wu, X., Kong, Y., Degefu, D. M., et al. (2022). Coordination of the industrial-ecological economy in the Yangtze River Economic Belt, China. Front. Environ. Sci. 10 (10). doi:10.3389/FENVS.2022.882221
Yue, J. T., Yan, X. Y., and Liu, Q. Y. (2019). Evaluation of water use efficiency in Jiangxi based on CRITIC-TOPSIS. Jiangxi Hydraulic Sci. Technol. 45 (04), 275–279. doi:10.3969/j.issn.1004-4701.2019.04-08
Zhang, N., and Sheng, W. (2019). Causes analysis of coal mine gas explosion accidents based on Bayesian network. Industry Mine Automation 45 (07), 53–58. doi:10.13272/j.issn.1671-251x.2019010049
Zhang, Z. H., Sun, S. M., and Gao, J. (2022). Evolution characteristic and influencing mechanism of water-energy-food stress in Yangtze River delta urban agglomeration. J. Nat. Resour. 37 (06), 1586–1597. doi:10.31497/zrzyxb.20220615
Zhang, Z. H., Wang, X. M., Dang, J. W., and Min, Y. Z. (2014). Bayesian network structure learning method based on expert knowledge fusion. Comput. Eng. Appl. 50 (02), 1–4+9. doi:10.3778/j.issn.1002-8331.1304-0254
Zhi, Y. L., Chen, J. F., Wang, H. M., Liu, G., and Zhu, W. M. (2020). Assessment of water-energy -food nexus fitness in China from the perspective of symbiosis. China Popul. Resour. Environ. 30 (1), 129–139. doi:10.12062/cpre.20190502
Keywords: water-energy-food-ecology nexus, vulnerability, neighborhood rough set, Bayesian network, Yangtze River Economic Belt
Citation: Chen Y, Pan Y and Geng M (2023) Identification of key brittleness factors and multi-scenario analysis of the water-energy-food-ecology nexus vulnerability based on NRS-BN. Front. Environ. Sci. 11:1273755. doi: 10.3389/fenvs.2023.1273755
Received: 07 August 2023; Accepted: 10 November 2023;
Published: 27 November 2023.
Edited by:
Salvador García-Ayllón Veintimilla, Polytechnic University of Cartagena, SpainReviewed by:
Liang Yuans, China Three Gorges University, ChinaAnik Bhaduri, Griffith University, Australia
Copyright © 2023 Chen, Pan and Geng. This is an open-access article distributed under the terms of the Creative Commons Attribution License (CC BY). The use, distribution or reproduction in other forums is permitted, provided the original author(s) and the copyright owner(s) are credited and that the original publication in this journal is cited, in accordance with accepted academic practice. No use, distribution or reproduction is permitted which does not comply with these terms.
*Correspondence: Yan Chen, sanchen007@njfu.edu.cn