- 1Program in Public Health, University of California, Irvine, Irvine, CA, United States
- 2Department of Biological Sciences, University of North Carolina, Charlotte, NC, United States
Landscape genetics aims to quantify the effect of landscape on gene flow. Broadly, the approach involves measuring genetic variation, quantifying landscape heterogeneity, and statistically testing the link between both genetic variation and landscape heterogeneity. This approach has been widely used by conservation biologists, for example to identify barriers restricting movement in threatened populations. More recently, landscape genetics has been used to study the epidemiology of infectious diseases, such as chronic wasting disease, raccoon rabies, and malaria. This method can be useful in identifying potential hotspot areas of disease movement for targeted public health interventions and containment of disease and drug resistance. However, vector-borne disease epidemiology is particularly complex, as it is affected by the movement of both the vector and human or vertebrate host. This feature could potentially inhibit the ability to detect the effect of landscape on gene flow, since the ecology of vectors and hosts are likely different and potentially conflicting. Here, we provide a summary of the latest innovations in the field of landscape genetics with a focus on those that could help increase the power to detect landscape effects in vector-borne human disease studies. We also provide a recommended framework for studying vector-borne diseases using a landscape genetics approach. Landscape genetics has the potential to be a powerful tool for the field of vector-borne disease epidemiology but has so far been underutilized. The provided synthesis of tools and considerations for conducting a landscape genetics study of a vector-borne disease aim to bridge the gap between the two disciplines.
Introduction
Broadly, landscape genetics aims to quantify the effects of landscape heterogeneity on microevolutionary processes, such as gene flow, genetic drift, and/or natural selection (Balkenhol et al., 2015). Landscape genetics has primarily and traditionally been used by conservation biologists, such as to identify vulnerable populations and identify areas where corridors are needed to promote gene flow (Storfer et al., 2010). More recently, landscape genetics has been used to study various infectious diseases, such as chronic wasting disease (Blanchong et al., 2008; Robinson et al., 2013), rabies in domestic dogs (Brunker et al., 2012), raccoon rabies (Rees et al., 2008, 2009; Cullingham et al., 2009; Côté et al., 2012), hantavirus (Guivier et al., 2011; Dubois et al., 2017), H5N1 avian influenza (Carrel et al., 2011), and malaria (Carrel et al., 2015; Lo et al., 2017a,b). This method can be useful to identifying potential hotspot areas of disease movement for targeted public health interventions and containment of disease and drug resistance. However, vector-borne disease epidemiology is particularly complex, as it is affected by the movement of both the vector and human or vertebrate host. This feature could potentially inhibit the ability to detect the effect of landscape on gene flow, since the ecology of vectors and hosts are likely different and potentially conflicting. The goal of this review is to provide a synopsis of the latest innovations in landscape genetics research with an emphasis on new tools that may be particularly useful to studying vector-borne human diseases.
There are already a number of excellent review papers on landscape genetics generally that provide an overall scope as well as technical details in conducting such studies (Holderegger and Wagner, 2006; Storfer et al., 2007, 2010; Manel and Holderegger, 2013; Hall and Beissinger, 2014). We suggest referencing these more general review papers to gain additional background in the field of landscape genetics if desired. In addition, conceptual considerations of using landscape genetics to study infectious diseases are discussed elsewhere (Biek and Real, 2010). We will therefore focus this review on practical landscape genetics resources that have high potential for improving understanding of vector-borne human disease transmission. We will highlight successes and synthesize lessons learned from these infectious disease landscape genetic studies. In addition, we will discuss tools from other fields that had yet to be used in the aforementioned studies.
Landscape genetic analysis begins by developing hypotheses of how landscape factors resist gene flow (Shirk et al., 2017). To test isolation-by-resistance hypotheses, landscape genetics integrates analytical tools across multiple disciplines, including landscape ecology, population genetics, and spatial statistics. It requires the use of molecular markers to measure genetic variation and to infer gene flow. Other tools needed are geographic information systems, remote sensing, population genetics, and statistical and mathematical modeling techniques (Manel and Holderegger, 2013). Moreover, landscape genetics analysis of vector-borne diseases is particularly complex and several factors must be considered when designing a study, such as those related to the environment, vector mobility, and human and pathogen mobility (Figure 1). Further, additional considerations for such a study must also be taken into account when measuring spatial and genetic distances to ensure sufficient sensitivity, which will be featured throughout the paper (Figure 1). Landscape genetics can be an extremely useful approach to improve our understanding of how vector-borne diseases spread (Biek and Real, 2010). Careful planning will be critical to the success of a landscape genetics study. With the advancement of molecular population genetics, remote sensing and modeling tools, it is possible to parse out the importance of key environmental variables and human factors influencing dispersal and potential spread of vector-borne diseases.
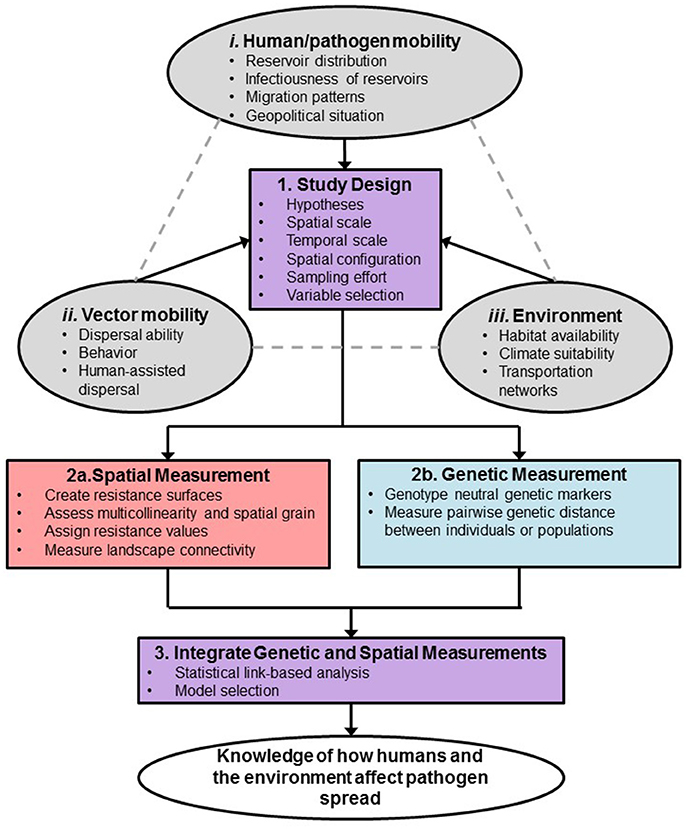
Figure 1. Framework for design and analysis of vector-borne disease landscape genetics study. Study system considerations that drive the sampling design are described in the orange ovals. Outcome of the study is in the yellow oval.
Study Design
Careful consideration for a sampling design is a critical first step in conducting any landscape genetics study. For a vector-borne disease study, it is especially critical due to the need for ultra-sensitive methods to detect landscape effects in a complex system. Important factors to consider include spatial and temporal scale, sampling regime, population or individual-based approach, sampling effort, and variables to test for isolation-by-resistance.
Spatial Scale
Propensity for dispersal is an important characteristic to consider in determining the appropriate spatial scale for a given study (Wright, 1943; Slatkin, 1987). For example, studies of organisms with longer dispersal distances may require data collection of larger spatial scales (Hall and Beissinger, 2014). Choosing an appropriate spatial scale for vector transmitted diseases is complicated in that one must consider the dispersal ranges of both the vectors and the hosts. Therefore, it may be useful to collect data at different transect widths, based on the dispersal behaviors of vectors and hosts, and assess the effect of spatial-scale variation (Murphy et al., 2010; Emaresi et al., 2011). In addition, a multiscale approach can help to reveal scale-dependent processes (Meentemeyer et al., 2012).
Temporal Scale
Temporal scale is an important factor to consider for a study in a changing landscape. For example, when historically high connectivity has recently declined, recent migration rates are over-estimated, which may lead to inaccurate conclusions (Samarasin et al., 2017). Since the ecology of vector-borne diseases are likely tightly linked to human-related factors, genetic connectivity is potentially changing with a high frequency. In this case, data collection at multiple time periods can help account for responses of genetic variation to a landscape change. This rate of response change differs among organisms. For organisms with short dispersal distances, such as mosquitoes, signals of a historic barrier can be maintained for greater than 100 generations. Thus, historic landscape traits may have a larger impact on genetic variation than the contemporary landscape (Landguth et al., 2010).
Spatial Sampling Regime
The chosen field sampling design can greatly impact the ability to detect effects of landscape pattern on gene flow. Since sensitive methods are needed to detect an effect of landscape on potentially complex disease patterns, choosing an appropriate sampling design is critical. Ideally, all populations should be sampled (van Strien, 2017). However, if not all populations are sampled, tests should be performed to assess inference sensitivity to missing populations (van Strien, 2017). Other possible sampling designs include: random, linear, systematic, and cluster. Random, linear, and systematic sampling designs have been shown to outperform a cluster design (Oyler-McCance et al., 2013). An alternative to the aforementioned sampling designs may be to optimize a sampling design based on a landscape feature that is hypothesized to influence gene flow. This optimization function is available as “opt.landgen” in the R package PopGenReport (Adamack and Gruber, 2014). The function evaluates the ability of hundreds of sampling designs to detect an effect of landscape for a given landscape resistance layer. It then provides the sampling designs that have the greatest power to detect a landscape effect if the landscape effect exists. This method may be particularly useful when the ecology of vectors and hosts are more well understood and the researcher is testing a specific hypothesis or a small set of specific hypotheses.
Individual-Based Analysis
An alternative to the traditional population-based analysis is to use an individual-based approach. This sampling method can be especially useful when population boundaries are not clearly defined or known (Richardson et al., 2016). This situation could be common in infectious disease studies. In addition, individual-based analysis can be more powerful in detecting fine-scale genetic patterns (Prunier et al., 2013; Luximon et al., 2014). For instance, this method has been used to study the variables that drive H5N1 avian influenza molecular change in Vietnam (Carrel et al., 2012). Carrel et al. (2012) found significant correlations between genetic differentiation of H5N1 and several population-environmental variables using individual-based analysis. Such associations at a fine-level may not be easily achieved with the population-based analysis.
Sampling Effort
The statistical power of analyses increases with an increasing number of samples. Simulations can help to estimate how power is affected by sample size. Such simulations can be implemented in programs, such as CDPOP (Landguth and Cushman, 2010). Based on population genetic simulations, it was found that in an individual-based landscape genetic approach, it may be more efficient to increase the number of loci rather than to increase sample size (Landguth et al., 2012).
Selecting Landscape Variables
Selecting appropriate landscape variables to test will determine whether a meaningful landscape effect will be able to be detected. It is important to carefully consider the biology of the study system when choosing variables. For example, unsuitable habitat may restrict dispersal, but this may not necessarily be the case. Moreover, since pathogen movement is affected by both the movement of vectors and hosts, it is essential to consider landscape variables that reflect the distribution of both vectors and hosts across space (Biek and Real, 2010).
Human Mobility Variables
Vector-borne disease landscape models can be improved by including measurements of human mobility. Human movement can affect disease outbreaks by introducing pathogens to a new population or by increasing the contact between infected and healthy individuals (Wesolowski et al., 2016). Monitoring human mobility and infectious disease dynamics is essential for predicting areas with high risks of outbreak. Roads and air travel patterns (at a larger scale) may be used as a method of estimating population movement. However, these features may not reveal the complexity of human migration patterns. For example, during the 1950 and 1960's, malaria eradication campaigns failed to take into account population patterns, which allowed for the resurgence of malaria in places that had already been eradicated (Martens and Hall, 2000). Therefore, it is important to take into account factors that contribute to mobility and disease transmission, such as increase in travel, population redistributions in developing worlds, natural disasters, and conflicts that account for the displacement of large amounts of people (Martens and Hall, 2000). In addition, seasonal migration, such as for agricultural work, may significantly influence pathogen spread. For instance, in Ethiopia, men who travel away from home were more likely to transmit malaria to high altitude villages due to traveling for seasonal jobs (Alemu et al., 2014). Therefore, new methods of measuring human mobility can be used to more accurately define migration patterns, as opposed to transportation features, such as roads. In this section, we discuss several forms of technology that can be utilized to capture spatial and temporal fluctuations in human mobility that affect disease dynamics such as anthropogenic light, mobile phone data, social media networks, and Global positioning Systems. We also address the advantages and limitations of using these forms of technology.
Anthropogenic light
Seasonal fluctuations of human populations can be measured by using remote sensing data for anthropogenic light (Bharti et al., 2011). For example, Bharti et al. (2011) found fluctuations in brightness and measle transmission to be strongly positively correlated in Niger. An important consideration for using anthropogenic light to assess human mobility is that nighttime light is associated with economic activity (Doll et al., 2006), and so important populations of people may be underestimated or missed in this measure. Nighttime light satellite imagery is available from the Defense Meteorological Satellite Program (DMSP) Operational Linescan System (OLS).
Mobile phone data
Mobile Phone Data can be used to predict the spread of infectious disease. A study conducted during the cholera epidemic in Haiti utilized phone data, created gravity models of population mobility, and compared the results to the reported cases of cholera (Bengtsson et al., 2015). It was assumed that the movement of mobile phone signals was proportional to the movement of individuals infected. Using this information, the infectious pressure was then calculated. Estimates showed a relationship with the risk of an area having an outbreak within 7 days (Bengtsson et al., 2015). The study found that the amount of local outbreaks could be predicted at early stages of the cholera epidemic (Bengtsson et al., 2015). A minor bias was found due to socio-economic group differences in mobile phone ownership.
Another study also suggested that mobile phone data could be used to study human mobility across spatial and temporal scales in many countries and pathogens to understand infectious epidemiology (Wesolowski et al., 2016). The study analyzed the dynamics of malaria and rubella in Kenya, where the mobile phone data was combined with the pathogen's life cycle, transmission and epidemiological inoculation rate to predict future outbreaks of disease (Wesolowski et al., 2016). By utilizing this information, it helped pinpoint high risk locations and helped to understand spatial transmission of disease. Some limitations in this study were that some information, such as seasonal fluctuation, was not available and cases were underreported (Wesolowski et al., 2016). The study also possessed a bias since children were underrepresented. However, these limitations did not significantly affect mobility predictions (Wesolowski et al., 2016). Since mobile phone data has been found to have predictive power of disease spread and outbreaks, it could therefore be useful to include mobile phone mobility data as a predictive variable in a landscape genetics study.
Social media
Social Media can also be used as a method of tracking human mobility. For instance, Tizzoni et al. (2015) used twitter geotags to scale human contact and epidemic processes in Metapopulations Networks. Two maps were created: one used the geotags to create census maps of areas with major transportation hubs; the other separated geotags into metropolitan areas located in the U.S and across European countries (Tizzoni et al., 2015). Using this data, it was evident that online telecommunication social networks can be used to map physical contact networks, and can indirectly be used as a tool to show real social ties for contagion processes (Tizzoni et al., 2015). They found that contact rates promoted epidemic spreading and increased based on the distribution of mobility flow between subpopulations (Tizzoni et al., 2015).
Similarly, in another study conducted in Australia, six million geotagged tweets were analyzed and provided evidence that twitter is useful for tracking and predicting human movement (Jurdak et al., 2015). In addition, they compared the Twitter data to data collected with mobile phone records. Although the results were similar, the Twitter data had higher resolution and was able to map different movements in intra-site, metropolitan, and inter-city movement (Jurdak et al., 2015). The Twitter data captured mobility patterns, and found that people who traveled long distances spend most of their time in metropolitan areas in comparison to those who only traveled intermediately (Jurdak et al., 2015). The study also identified two types of Twitter users, one had highly predictable tweeting locations while the other was less predictable (Jurdak et al., 2015). Those who tweeted from home were more likely to return in comparison to long distance movers who were less likely to return (Jurdak et al., 2015). The study concluded that geotagged tweets is a useful tool for identifying population-level patterns, however individual patterns were more sensitive to contextual factors (Jurdak et al., 2015). Twitter-based mobility-patterns can be a useful proxy for characterizing the risk of disease by creating movement profiles across a landscape. With that said, Twitter data may not be as effective at measuring human mobility in low-income settings.
GPS systems
Global Positioning Systems (GPS) were used to track the mobility patterns of 582 participants from two neighborhoods in Iquitos, Peru (Vazquez-Prokopec et al., 2013). The study aimed to consider social interactions in environments that are resource-poor and have rapid growth when studying the dynamics of infectious disease spread (Vazquez-Prokopec et al., 2013). Vazquez-Prokopec et al. (2013) measured age-specific mobility and changes in colocation networks in all the participants. It was found that geographic space greatly influenced human mobility (Vazquez-Prokopec et al., 2013). Approximately 80% of movements were 1 km away from the person's home (Vazquez-Prokopec et al., 2013). About 38% of participants had a predictable routine showing contrasting results to more developed countries (Vazquez-Prokopec et al., 2013). As a result, the researchers conducted a case study to determine how unstructured routines would impact the transmission of infectious diseases. It was determined that unstructured routines increase disease outbreak in comparison to more structured routines (Vazquez-Prokopec et al., 2013). This result displayed the complexity in analyzing human mobility and its effects on infectious diseases in developing countries, revealing that further studies need to be developed to create a more reliable infectious disease model (Vazquez-Prokopec et al., 2013). In another study, Paz-Soldan et al. (2010) utilized GPS systems to study dengue virus transmission and possible barriers in Peru. They determined that using GPS to track human movement has many benefits. For instance, they are affordable and help determine rates of exposure in a population and the dynamics of vector-borne pathogens (Paz-Soldan et al., 2010). However, it is important to address participant concerns when using GPS in order to avoid participant associated errors (Ikanovic and Mollgaard, 2017).
Vector Mobility Variables
Landscape variables related to vector movement may vary greatly among study systems. A starting point for selecting variables of interest may be to reference habitat suitability models or previous field observations for important variables associated with vector habitats. For example, the population structure of Aedes mcintoshi, the primary vector for Rift Valley fever virus in Kenya, was found to correlate with mean precipitation values, a variable selected based on previous field observations (Campbell and Alexander, 2017). Notably, this approach assumes that unsuitable habitat impedes movement, which may not necessarily be the case. It is therefore critical to carefully consider the biology of your study system. Some variables of interest may include elevation, land cover type, temperature, and/or precipitation. Data for these variables are often publicly available by remotely-sensed data. For instance, global elevation data is available by NASA's Shuttle Radar Topographic Mission (SRTM). Global land cover data along with several other land products are available by NASA's Moderate Resolution Imaging Spectroradiometer (MODIS). Temperature and precipitation data is available by WorldClim (Hijmans et al., 2005).
In addition, humans can facilitate vector spread, such as an aircraft carrying insect vectors (Tatem et al., 2006). Therefore, some of the previously mentioned variables related to human mobility, such as roads and airfreight, may also apply to vector movement. For example, human-aided dispersal (highways) was found to reflect gene flow at a broad spatial scale for the Asian tiger mosquito, Aedes albopictus, whereas natural dispersal (forests) was more dominant at smaller spatial scales (Medley et al., 2015). Moreover, vector distribution may be tightly correlated with host distribution due to their dependence on host blood meals (Leo et al., 2016). Thus, factors related to vector mobility may closely reflect those of human mobility especially for study systems in which alternative hosts are absent or negligible, for instance in areas where the highly anthropophilic vector An. gambiae s.s. is the primary vector of the malaria parasite Plasmodium falciparum.
Spatial Measurement
Creating Landscape Resistance Surfaces
A resistance surface is a spatial layer where each grid cell is represented by the extent to which conditions at that cell constrain movement or gene flow (Balkenhol et al., 2015). Some knowledge of GIS software is necessary to create resistance surfaces, such as ArcMap or DIVA-GIS, a free computer program, though many other softwares exist.
Multicollinearity
It is important to avoid introducing multicollinearity when evaluating landscape variables. Multicollinearity, a general problem in modeling, is when there is a high degree of linear correlation among predictor variables, such as elevation and temperature. This issue can be addressed by checking a matrix of pairwise correlations among predictor variables. Generally, correlations above 0.7 are considered problematic (Dormann et al., 2013). If multicollinearity is present among two or more variables, then it is suggested to remove one or more of those variables.
Spatial Grain
When creating landscape resistance surfaces, choosing an appropriate spatial grain is critical because landscape genetic analysis is highly sensitive to grain size (Turner, 1989; Wu, 2004). Generally, the scale of how the landscape is connected should correspond to the scale of how organisms move and disperse (Galpern and Manseau, 2013). Anderson et al. (2010) recommends using a spatial grain size smaller than that of the organism's average dispersal range. However, too small of a grain can lead to unnecessary noise that may mask coarser patterns influencing connectivity (Anderson et al., 2010). In vector-borne disease studies, the parasite's dispersal range is complicated by having multiple dispersal mechanisms at potentially divergent spatial scales. Therefore, a method described by Galpern and Manseau (2013) using multi-scaled analysis to match the analysis grain to the functional grain may be especially useful for such studies and can help to improve the accuracy of identifying the contribution of landscape features to connectivity.
Assigning Resistance Values
After having decided on landscape variables and creating landscape resistance surfaces, it is necessary to assign features a landscape resistance to gene flow value. For continuous variables, such as elevation, it is possible to simply assign the raw elevation values as the landscape resistance value. However, this approach assumes a linear and positive relationship between elevation and landscape resistance to gene flow. For discrete variables, such as land cover type, it is necessary to assign each land cover type a landscape resistance value. Assigning appropriate landscape resistance values is essential to being able to detect landscape effects. Moreover, in vector-borne disease studies, parasite movement is particularly complex, and so precise landscape resistance values are especially important to have sufficient sensitivity toward to detect landscape effects.
Expert Opinion
Expert Opinion is the most common method to assign landscape resistance values (Shirk et al., 2010; Zeller et al., 2012). However, a major pitfall of expert opinion is that it is subjective (Zeller et al., 2012; Trainor et al., 2013; Dudaniec et al., 2016). In addition, expert-derived landscape resistance values have been found to perform worse than a null model (Charney, 2012). While expert opinion may be a useful starting point, it is suggested that if used, to further parameterize expert-derived values as discussed below in the optimization section (Shirk et al., 2010).
Habitat Suitability Models
Habitat Suitability Models can be used in assigning resistance values by directly converting the suitability value for a species to a resistance value. For instance, if a suitability scale is 0–100, the resistance value can be transformed by subtracting the habitat suitability value from 100 (Spear et al., 2010). Wang et al. (2008) found significant correlations between genetic distance and a resistance surface based on habitat suitability for the spiny rat, Niviventer coninga. Another study on wolves (Canis lupus) compared habitat suitability models to expert opinion and found the habitat suitability based models to have higher coefficient values than those based on expert opinion (Milanesi et al., 2017). However, it is important to note that habitat suitability models are based on presence and absence data and do not incorporate information on movement behavior (Spear et al., 2010). In addition, using habitat suitability models to assign resistance values would likely not be readily accessible for resistance surfaces based on human mobility.
Movement Studies
Movement studies, such as mark-recapture studies, radio telemetry, GPS, or track or fecal surveys, may also be used to assign resistance values (Spear et al., 2010). Resistance values may be similarly assigned as in the case of suitability models, where if for example, the highest traveled corridor had an average of 10 crossings per day, the resistance value for every corridor would be calculated by subtracting the average number of crossings per day from 10. However, movement data can be difficult and time-intensive to obtain. Collecting this type of data may also be somewhat paradoxical since a major motivation for conducting landscape genetic studies is because this data is difficult to obtain (Spear et al., 2010).
Optimization
Optimization may help to improve the accuracy of resistance values derived from expert opinion. For example, Shirk et al. (2010) refined a model optimization approach introduced by Cushman et al. (2006) and demonstrated its utility on a population of mountain goats in the Cascade Range. The approach began with an expert opinion-derived parametrization of landscape features, such as distance to escape terrain, roads, land cover type, and elevation. Each variable was related to landscape resistance with a simple mathematical function. Then, expert opinion parameter values were systematically varied for each variable to find the model with the highest correlation to genetic distance. The resulted optimized parameter values differed from expert opinion values for all variables and the elevation and land cover models were found to have a modest correlation with gene flow (Shirk et al., 2010). Moreover, Gharehaghaji et al. (2017) also implemented this optimization approach and found significant associations between elevation and gene flow of valley oaks. Thus, this optimization approach may help to improve the ability to detect landscape effects over expert opinion only models.
An alternative optimization approach devoid of expert opinion is to optimize landscape resistance surfaces based on a genetic algorithm, which has been used to study salamander species (Peterman et al., 2014, 2015). This method can be implemented using the R package ResistanceGA (Peterman, 2014). ResistanceGA uses a genetic algorithm to optimize landscape resistance surfaces based on pairwise genetic distances and resistance distances and can optimize both categorical and continuous resistance surfaces. This approach may provide an unbiased optimization of resistance surfaces.
Landscape Connectivity
It is difficult to directly correlate a landscape resistance surface to pairwise genetic data. Therefore, it is necessary to convert the data in a landscape resistance surface to a pairwise number of “landscape distance” between populations or individuals. There are two common methods outlined below for making this translation to pairwise measures.
Least Cost Path
Least Cost Path has been the most popular approach in landscape genetics (Storfer et al., 2007). This approach simply involves drawing a line between two populations or individuals that minimizes the cumulative movement cost across the landscape resistance surface. For the pairwise distance measure, the researcher can either use the Euclidean length of the least-cost path (Spear et al., 2005) or calculate the weighted resistance cost across the path (Cushman et al., 2006; Shafer et al., 2012). However, both methods of calculating “landscape distance” assume that the organism possesses enough knowledge to follow the optimal path between points in the landscape. In addition, the weighted resistance cost path is particularly sensitive to relative costs, and so it may not be ideal for situations in which the resistance parameterization is uncertain (Balkenhol et al., 2015). Least cost paths can be calculated using the R package gdistance (van Etten, 2017).
Circuit Theory
Circuit Theory can be used to model dispersal through a resistance surface between populations. In this approach, landscapes are represented as grids of regularly-spaced nodes that are connected by resistors. The provided landscape resistance surface determines the level of resistance among the adjacent nodes. This method incorporates all possible pathways between populations and provides summary pairwise “landscape distance” values. This approach may be especially beneficial in landscapes where there are multiple paths between populations or individuals of similar total cost, so that a least-cost path would only represent a fraction of realized connectivity. This approach can be implemented using Circuitscape software (McRae and Beier, 2007).
Genetic Measurement
Genotyping
Molecular Markers
The majority of molecular markers used in landscape genetic studies include microsatellites, single nucleotide polymorphisms (SNPs), allozymes, and amplified fragment length polymorphisms (AFLPs). Statistical power increases with the number of loci, as well as the number of alleles per loci. Power related to the number of loci evaluated can be estimated prior to the study using simulations in CDPOP (Landguth and Cushman, 2010; Landguth et al., 2012). While microsatellites are the most commonly used marker in landscape genetic studies, high coverage SNP markers may provide a better ability to detect population differences than traditional microsatellites. For example, Campos et al. (2017) found that by using a ddRADseq-derived SNP dataset, they were able to differentiate Anopheles darlingi populations that were missed when using a nine microsatellite loci dataset.
Measure Pairwise Genetic Distance
Using genotype data, a metric of genetic distance among all pairs of populations or individuals is calculated. Population-level metrics, such as F-statistics, chord, and Nei's standard distance assume mutation-drift equilibrium (Hall and Beissinger, 2014). Therefore, in study systems where there has been a recent habitat disturbance, which may be common in human disease systems, these statistics may not be ideal. Rather, alternative statistics such as those described below may be better-suited for such a study.
Principle coordinate analysis (PCA)-based
In a comparison of individual-based genetic distance metrics, simulations demonstrated that PCA-based metrics performed best under the most challenging conditions, when sample sizes are limited and dispersal is high (Shirk et al., 2017). Therefore, using PCA-based metrics may maximize model selection accuracy and improve the reliability of landscape genetic analysis.
Identity by descent
FST has been shown to be less reliable at descriminating population structure at small spatial scales (Miotto et al., 2013). Identity by descent (IBD), which relates ancestry to variability due to recombination can provide insight into more recent demographic events than FST, since recombination works on shorter time scales than mutation and genetic drift (Thompson, 2013). In a malaria parasite study where samples were collected from four clinics with an area spanning approximately 120 km, there was no relationship between clinic distance and FST genetic distance. However, a significant decline in IBD-based relatedness was found with increasing inter-clinic distance (Taylor et al., 2017). Thus, at small spatial scales, using IBD as the genetic distance metric can increase sensitivity in detecting population differentiation.
Spatially explicit summary statistics
Spatially explicit summary statistics (SSS), such as Euclidean distances in spatial-PCA space or Monmonier's identification of genetic breaks, can improve the ability to discriminate between complex evolutionary histories (Alvarado-Serrano and Hickerson, 2016). These methods can capture spatial distribution patterns underlying the genetic samples, and so SSS can be useful for investigating demographic histories across a landscape.
Integrate Genetic and Spatial Measurements
We will outline link-based analysis statistical methods to integrate genetic and spatial measurement data. The link-based approach relates neutral variation between sites to between-site landscape factors to test hypotheses related to isolation-by-environment, which we anticipate to be the most common scenario in a vector-borne disease study. For information on link-, neighborhood-, and boundary-based methods, we suggest referencing Hall and Beissinger (2014). For link-based analysis, there are several statistical analysis options that may be employed to test the correlation of spatial and genetic distance. Here, we briefly discuss common statistical tests.
Link-Based Statistical Analyses
Partial Mantel Tests
Partial Mantel tests are the most common analytical method in landscape genetics. Mantel tests examine the correlation between a matrix of pairwise genetic distances and a matrix of spatial distances. While common, Mantel tests have been heavily criticized for having an inflated type I error rate and low statistical power caused by the non-independence in response and predictor variables (Balkenhol et al., 2009; Cushman and Landguth, 2010; Graves et al., 2013). It is therefore advised to avoid the use of Mantel tests in landscape genetic studies (Manel and Holderegger, 2013). Multiple regression on distance matrices are similar to Mantel tests and should likewise be avoided (Manel and Holderegger, 2013).
Multiple Matrix Regression With Randomizations (MMRR)
Multiple matrix regression with randomizations (MMRR) is a regression on the pairwise distance matrices that assesses the significance of regression parameters by special randomizations (Wang, 2013). This method accounts for the non-independence of variables and provides a regression coefficient and significance test for each cost matrix. This test can be done using the function “lgrMMRR” in the R package PopGenReport (Adamack and Gruber, 2014).
Linear Mixed Effects Model With a Maximum Likelihood Population Effects Parameterization (MLPE)
Linear mixed effects model with a maximum likelihood population effects parameterization (MLPE) is another method that accounts for non-independence among pairwise data. This method does so by including a random or population effect based on covariate structure (Clarke et al., 2002; van Strien et al., 2012). This test can be done using the “lmer” function in the R package lme4 (Bates et al., 2015).
BEDASSLE
BEDASSLE is a package available in R that implements a bayesian approach and Markov chain Monte Carlo algorithm (Bradburd et al., 2013). This method is different from Mantel tests, MMRR, and linear mixed effect models with MLPE in that BEDASSLE models the covariance in allele frequencies between populations as a decreasing function of ecological and geographic distance (Wang and Bradburd, 2014). The coefficients are then estimated for the pairwise distance measures and compared. The resulted method is able to quantify the relative contributions of geographic and ecological distance to genetic differentiation.
Machine Learning
Machine learning has been used in the analysis of landscape genetic studies, which investigated the landscape factors associated with resistance to gene flow in amphibian populations (Murphy et al., 2010; Hether and Hoffman, 2012). Random forest (RF), the machine learning algorithm used, is based on regression tree analysis and can be useful in landscape genetic analysis because it can be used for data sets with many redundant or irrelevant predictors (Hether and Hoffman, 2012). The method involves first combining genetic and landscape data, running the RF model using the R package “RandomForest” for all predictors, and then converting the result into model improvement ratios (MIR) (Hether and Hoffman, 2012). Using this method, RF was able to identify habitats associated with genetic differentiation (Hether and Hoffman, 2012).
Model Selection
Finally, it is important to note that a priori selection of a single landscape resistance model will likely lead to a high but false correlation (Cushman and Landguth, 2010; Cushman et al., 2013). Thus, it is important to test a set of realistic models involving several landscape variables, as well as multiple functional responses to each variable, such as by using methods previously mentioned in the optimization sub-section (Shirk et al., 2010; Peterman, 2014). Alternatively, an approach that does not rely on iterative optimization algorithms may be used by simply assessing landscape variables at varying relative costs, i.e., creating and testing many hypothetical resistance surfaces and selecting the highest ranked models according to values such as the Akaike information criterion (AIC) (Cushman et al., 2006; Wang et al., 2009). Moreover, multivariate models may also be similarly evaluated, potentially by creating several candidate combinations of top univariate models or simply testing all possible combinations of variables.
Landscape Genomics
The previous sections have assumed that the objective of a given landscape genetics study is to understand how gene flows between populations. To understand how landscape and environmental factors influence selection and local adaptation, a landscape genomics approach may be used. Landscape genomics offers the potential for researchers to fine-tune molecular variation underlying the changing environments and landscapes within a region. In landscape genomics, we seek to identify which genes influence a trait or confer an environmental preference, which alleles in those genes are most desirable for the conditions observed, and how much each gene contributes to the adaptation to those conditions (Schwartz et al., 2010). Different from landscape genetics that evaluates genetic relatedness among individuals/populations using multi-locus markers such as microsatellites and AFLPs, the first step in landscape genomics is to characterize the genome of the organisms of interest. This includes the identification of genetic variants, their putative function, and their genic or allelic diversity. The advent of next generation sequencing technology has made this process increasingly feasible and affordable, both technically and financially. Bioinformatics and correlation analyses (such as categorical tests, logistic regressions, matrix correlations, general linear models and mixed effects models) of next generation genome data will allow the identification of key genes or variants under selection from environmental factors (Rellstab et al., 2015). The next step is to characterize the spatial distribution of allelic diversity across the landscape. Landscape genomics approach is useful when sampled populations have weak or unknown structure (Jones et al., 2013) because correlated allele frequencies between populations have been shown to substantially increase type I errors in certain landscape genomics approaches (Bradburd et al., 2013; De Mita et al., 2013). By identifying the associations between genotypes and environmental traits/phenotypes, the genetic architecture of selection can be investigated at the individual, population, or species levels while incorporating the effect of spatial heterogeneity of the landscape on patterns of allelic variation (Schoville et al., 2012). The results of these analyses may be used to predict or model which populations are best adapted to the studied areas.
Conclusions
Despite that landscape genetics can provide useful insights into variables influencing pathogen spread, the approach has been underutilized in studying vector-borne disease epidemiology. As countries approach disease elimination and drug resistance increases in prevalence, knowledge of how pathogens spread becomes even more critical to informing targeted interventions. Careful planning of a study design is essential to being able to detect landscape effects, especially in a system as complex as a vector-borne disease. We have provided a summary of important landscape genetics resources and considerations for designing and carrying out an effective vector-borne disease study (see Supplementary Table for a summary of resources discussed in the text). With the provided recommendations and resources, we aim to close the gap between the two disciplines to better understand the underlying factors influencing pathogen spread.
Author Contributions
EH-S and EL conceived and designed the review; EH-S, EL, CS, and SP contributed to the literature review; EH-S, EL, CS, SP, and GY contributed to the writing.
Funding
This project was funded by National Institutes of Health grants R01 A1050243, D43 TW01505, and U19 AI129326.
Conflict of Interest Statement
The authors declare that the research was conducted in the absence of any commercial or financial relationships that could be construed as a potential conflict of interest.
Supplementary Material
The Supplementary Material for this article can be found online at: https://www.frontiersin.org/articles/10.3389/fevo.2018.00021/full#supplementary-material
References
Adamack, A. T., and Gruber, B. (2014). PopGenReport: simplifying basic population genetic analyses in R. Methods Ecol. Evolut. 5, 384–387. doi: 10.1111/2041-210X.12158
Alemu, K., Worku, A., Berhane, Y., and Kumie, A. (2014). Men traveling away from home are more likely to bring malaria into high altitude villages, northwest Ethiopia. PLoS ONE 9:e95341. doi: 10.1371/journal.pone.0095341
Alvarado-Serrano, D. F., and Hickerson, M. J. (2016). Spatially explicit summary statistics for historical population genetic inference. Methods Ecol. Evol. 7, 418–427. doi: 10.1111/2041-210X.12489
Anderson, C. D., Epperson, B. K., Fortin, M. J., Holderegger, R., James, P., Rosenberg, M. S., et al. (2010). Considering spatial and temporal scale in landscape-genetic studies of gene flow. Mol. Ecol. 19, 3565–3575. doi: 10.1111/j.1365-294X.2010.04757.x
Balkenhol, N., Cushman, S., Storfer, A., and Waits, L. (2015). Landscape Genetics: Concepts, Methods, Applications. Chichester, UK: John Wiley & Sons.
Balkenhol, N., Waits, L. P., and Dezzani, R. J. (2009). Statistical approaches in landscape genetics: an evaluation of methods for linking landscape and genetic data. Ecography 32, 818–830. doi: 10.1111/j.1600-0587.2009.05807.x
Bates, D., Mächler, M., Bolker, B., and Walker, S. (2015). Fitting linear mixed-effects models using lme4. J. Stat. Soft. 67, 1–48. doi: 10.18637/jss.v067.i01
Bengtsson, L., Gaudart, J., Lu, X., Moore, S., Wetter, E., Sallah, K., et al. (2015). Using mobile phone data to predict the spatial spread of cholera. Sci. Rep. 5:8923. doi: 10.1038/srep08923
Bharti, N., Tatem, A. J., Ferrari, M. J., Grais, R. F., Djibo, A., and Grenfell, B. T. (2011). Explaining seasonal fluctuations of measles in Niger using nighttime lights imagery. Science 334, 1424–1427. doi: 10.1126/science.1210554
Biek, R., and Real, L. A. (2010). The landscape genetics of infectious disease emergence and spread. Mol. Ecol. 19, 3515–3531. doi: 10.1111/j.1365-294X.2010.04679.x
Blanchong, J. A., Samuel, M. D., Scribner, K. T., Weckworth, B. V., Langenberg, J. A., and Filcek, K. B. (2008). Landscape genetics and the spatial distribution of chronic wasting disease. Biol. Lett. 4, 130–133. doi: 10.1098/rsbl.2007.0523
Bradburd, G. S., Ralph, P. L., and Coop, G. M. (2013). Disentangling the effects of geographic and ecological isolation on genetic differentiation. Evolution 67, 3258–3273. doi: 10.1111/evo.12193
Brunker, K., Hampson, K., Horton, D. L., Biek, R., and Randolph, S. E. (2012). Integrating the landscape epidemiology and genetics of RNA viruses: rabies in domestic dogs as a model. Parasitology 139, 1899–1913. doi: 10.1017/S003118201200090X
Campbell, L. P., and Alexander, A. M. (2017). Landscape Genetics of Aedes mcintoshi (Diptera: Culicidae), an important vector of rift valley fever virus in Northeastern Kenya. J. Med. Entomology. 18:tjx072. doi: 10.1093/jme/tjx072
Campos, M., Conn, J. E., Alonso, D. P., Vinetz, J. M., Emerson, K. J., and Ribolla, P. E. (2017). Microgeographical structure in the major Neotropical malaria vector Anopheles darlingi using microsatellites and SNP markers. Parasit. Vectors 10, 76. doi: 10.1186/s13071-017-2014-y
Carrel, M. A., Emch, M., Nguyen, T., Todd Jobe, R., and Wan, X. F. (2012). Population-environment drivers of H5N1 avian influenza molecular change in Vietnam. Health Place. 18, 1122–1131. doi: 10.1016/j.healthplace.2012.04.009
Carrel, M., Patel, J., Taylor, S. M., Janko, M., Mwandagalirwa, M. K., Tshefu, A. K., et al. (2015). The geography of malaria genetics in the Democratic Republic of Congo: a complex and fragmented landscape. Soc. Sci. Med. 133, 233–241. doi: 10.1016/j.socscimed.2014.10.037
Carrel, M., Wan, X. F., Nguyen, T., and Emch, M. (2011). Genetic Variation of highly pathogenic H5N1 avian influenza viruses in vietnam shows both species-specific and spatiotemporal associations. Avian Dis. 55, 659–666. doi: 10.1637/9785-051811-Reg.1
Charney, N. D. (2012). Evaluating expert opinion and spatial scale in an amphibian model. Ecol. Modell. 242, 37–45. doi: 10.1016/j.ecolmodel.2012.05.026
Clarke, R. T., Rothery, P., and Raybould, A. F. (2002). Confidence limits for regression relationships between distance matrices: estimating gene flow with distance. J. Agric. Biol. Environ. Stat. 7, 361. doi: 10.1198/108571102320
Côté, H., Garant, D., Robert, K., Mainguy, J., and Pelletier, F. (2012). Genetic structure and rabies spread potential in raccoons: the role of landscape barriers and sex-biased dispersal. Evol. Appl. 5, 393–404. doi: 10.1111/j.1752-4571.2012.00238.x
Cullingham, C. I., Kyle, C. J., Pond, B. A., Rees, E. E., and White, B. N. (2009). Differential permeability of rivers to raccoon gene flow corresponds to rabies incidence in Ontario, Canada. Mol. Ecol. 18, 43–53. doi: 10.1111/j.1365-294X.2008.03989.x
Cushman, S. A., and Landguth, E. L. (2010). Spurious correlations and inference in landscape genetics. Mol. Ecol. 19, 3592–3602. doi: 10.1111/j.1365-294X.2010.04656.x
Cushman, S. A., McKelvey, K. S., Hayden, J., and Schwartz, M. K. (2006). Gene flow in complex landscapes: testing multiple hypotheses with causal modeling. Am. Nat. 168, 486–499. doi: 10.1086/506976
Cushman, S. A., Shirk, A. J., and Landguth, E. L. (2013). Landscape genetics and limiting factors. Conserv. Genet. 14, 263–274. doi: 10.1007/s10592-012-0396-0
Doll, C. N., Muller, J. P., and Morley, J. G. (2006). Mapping regional economic activity from night-time light satellite imagery. Ecol. Econ. 57, 75–92. doi: 10.1016/j.ecolecon.2005.03.007
Dormann, C. F., Elith, J., Bacher, S., Buchmann, C., Carl, G., Carré, G., et al. (2013). Collinearity: a review of methods to deal with it and a simulation study evaluating their performance. Ecography 36, 27–46. doi: 10.1111/j.1600-0587.2012.07348.x
Dubois, A., Galan, M., Cosson, J. F., Gauffre, B., Henttonen, H., Niemimaa, J., et al. (2017). Microevolution of bank voles (Myodes glareolus) at neutral and immune-related genes during multiannual dynamic cycles: consequences for Puumala hantavirus epidemiology. Infect. Genet. Evol. 49, 318–329. doi: 10.1016/j.meegid.2016.12.007
Dudaniec, R. Y., Worthington Wilmer, J., Hanson, J. O., Warren, M., Bell, S., and Rhodes, J. R. (2016). Dealing with uncertainty in landscape genetic resistance models: a case of three co-occurring marsupials. Mol. Ecol. 25, 470–486. doi: 10.1111/mec.13482
Emaresi, G., Pellet, J., Dubey, S., Hirzel, A. H., and Fumagalli, L. (2011). Landscape genetics of the Alpine newt (Mesotriton alpestris) inferred from a strip-based approach. Conserv. Genet. 12, 41–50. doi: 10.1007/s10592-009-9985-y
Galpern, P., and Manseau, M. (2013). Finding the functional grain: comparing methods for scaling resistance surfaces. Landsc. Ecol. 28, 1269–1281. doi: 10.1007/s10980-013-9873-1
Gharehaghaji, M., Minor, E. S., Ashley, M. V., Abraham, S. T., and Koenig, W. D. (2017). Effects of landscape features on gene flow of valley oaks (Quercus lobata). Plant Ecol. 218, 487–499. doi: 10.1007/s11258-017-0705-2
Graves, T. A., Beier, P., and Royle, J. A. (2013). Current approaches using genetic distances produce poor estimates of landscape resistance to interindividual dispersal. Mol. Ecol. 22, 3888–3903. doi: 10.1111/mec.12348
Guivier, E., Galan, M., Chaval, Y., Xuéreb, A., Ribas Salvador, A., et al. (2011). Landscape genetics highlights the role of bank vole metapopulation dynamics in the epidemiology of Puumala hantavirus. Mol. Ecol. 20, 3569–3583. doi: 10.1111/j.1365-294X.2011.05199.x
Hall, L. A., and Beissinger, S. R. (2014). A practical toolbox for design and analysis of landscape genetics studies. Landsc. Ecol. 29, 1487–1504. doi: 10.1007/s10980-014-0082-3
Hether, T. D., and Hoffman, E. A. (2012). Machine learning identifies specific habitats associated with genetic connectivity in Hyla squirella. J. Evol. Biol. 25, 1039–1052. doi: 10.1111/j.1420-9101.2012.02497.x
Hijmans, R. J., Cameron, S. E., Parra, J. L., Jones, P. G., and Jarvis, A. (2005). Very high resolution interpolated climate surfaces for global land areas. Int. J. Climatol. 25, 1965–1978. doi: 10.1002/joc.1276
Holderegger, R., and Wagner, H. H. (2006). A brief guide to landscape genetics. Landsc. Ecol. 21, 793–796. doi: 10.1007/s10980-005-6058-6
Ikanovic, E. L., and Mollgaard, A. (2017). An alternative approach to the limits of predictability in human mobility. EPJ Data Sci. 6, 12. doi: 10.1140/epjds/s13688-017-0107-7
Jones, M. R., Forester, B. R., Teufel, A. I., Adams, R. V., Anstett, D. N., Goodrich, B. A., et al. (2013). Integrating landscape genomics and spatially explicit approaches to detect loci under selection in clinal populations. Evolution 67, 3455–3468. doi: 10.1111/evo.12237
Jurdak, R., Zhao, K., Liu, J., AbouJaoude, M., Cameron, M., and Newth, D. (2015). Understanding human mobility from Twitter. PLoS ONE 10:e0131469. doi: 10.1371/journal.pone.0131469
Landguth, E. L., and Cushman, S. A. (2010). CDPOP: a spatially explicit cost distance population genetics program. Mol. Ecol. Resour. 10, 156–161. doi: 10.1111/j.1755-0998.2009.02719.x
Landguth, E. L., Cushman, S. A., Schwartz, M. K., McKelvey, K. S., Murphy, M., and Luikart, G. (2010). Quantifying the lag time to detect barriers in landscape genetics. Mol. Ecol. 19, 4179–4191. doi: 10.1111/j.1365-294X.2010.04808.x
Landguth, E. L., Fedy, B. C., Oyler-McCance, S. A., Garey, A. L., Emel, S. L., Mumma, M., et al. (2012). Effects of sample size, number of markers, and allelic richness on the detection of spatial genetic pattern. Mol. Ecol. Resour. 12, 276–284. doi: 10.1111/j.1755-0998.2011.03077.x
Leo, S. S., Gonzalez, A., and Millien, V. (2016). Multi-taxa integrated landscape genetics for zoonotic infectious diseases: deciphering variables influencing disease emergence. Genome 59, 349–361. doi: 10.1139/gen-2016-0039
Lo, E., Hemming-Schroeder, E., Yewhalaw, D., Nguyen, J., Kebede, E., Zemene, E., et al. (2017a). Transmission dynamics of co-endemic Plasmodium vivax and P. falciparum in Ethiopia and prevalence of antimalarial resistant genotypes. PLoS Negl. Trop. Dis. 11:e0005806. doi: 10.1371/journal.pntd.0005806
Lo, E., Lam, N., Hemming-Schroeder, E., Nguyen, J., Zhou, G., Lee, M. C., et al. (2017b). Frequent spread of Plasmodium vivax malaria maintains high genetic diversity at the Myanmar-China Border, without distance and landscape barriers. J. Infect. Dis. 216, 1254–1263. doi: 10.1093/infdis/jix106
Luximon, N., Petit, E. J., and Broquet, T. (2014). Performance of individual vs. group sampling for inferring dispersal under isolation-by-distance. Mol. Ecol. Resour. 14, 745–752. doi: 10.1111/1755-0998.12224
Manel, S., and Holderegger, R. (2013). Ten years of landscape genetics. Trends Ecol. Evol. (Amst) 28, 614–621. doi: 10.1016/j.tree.2013.05.012
Martens, P., and Hall, L. (2000). Malaria on the move: human population movement and malaria transmission. Emerg. Infect. Dis. 6, 103. doi: 10.3201/eid0602.000202
McRae, B. H., and Beier, P. (2007). Circuit theory predicts gene flow in plant and animal populations. Proc. Natl. Acad. Sci. U.S.A. 104, 19885–19890. doi: 10.1073/pnas.0706568104
Medley, K. A., Jenkins, D. G., and Hoffman, E. A. (2015). Human-aided and natural dispersal drive gene flow across the range of an invasive mosquito. Mol. Ecol. 24, 284–295. doi: 10.1111/mec.12925
Meentemeyer, R. K., Haas, S. E., and Václavík, T. (2012). Landscape epidemiology of emerging infectious diseases in natural and human-altered ecosystems. Annu. Rev. Phytopathol. 50, 379–402. doi: 10.1146/annurev-phyto-081211-172938
Milanesi, P., Holderegger, R., Caniglia, R., Fabbri, E., Galaverni, M., and Randi, E. (2017). Expert-based versus habitat-suitability models to develop resistance surfaces in landscape genetics. Oecologia 183, 67–79. doi: 10.1007/s00442-016-3751-x
Miotto, O., Almagro-Garcia, J., Manske, M., MacInnis, B., Campino, S., Rockett, K. A., et al. (2013). Multiple populations of artemisinin-resistant Plasmodium falciparum in Cambodia. Nat. Genet. 45, 648. doi: 10.1038/ng.2624
Mita, S., Thuillet, A. C., Gay, L., Ahmadi, N., Manel, S., Ronfort, J., et al. (2013). Detecting selection along environmental gradients: analysis of eight methods and their effectiveness for outbreeding and selfing populations. Mol. Ecol. 22, 1383–1399. doi: 10.1111/mec.12182
Murphy, M. A., Evans, J. S., and Storfer, A. (2010). Quantifying Bufo boreas connectivity in Yellowstone National Park with landscape genetics. Ecology 91, 252–226. doi: 10.1890/08-0879.1
Oyler-McCance, S. J., Fedy, B. C., and Landguth, E. L. (2013). Sample design effects in landscape genetics. Conserv. Genet. 14, 275–285. doi: 10.1007/s10592-012-0415-1
Paz-Soldan, V. A., Stoddard, S. T., Vazquez-Prokopec, G., Morrison, A. C., Elder, J. P., et al. (2010). Assessing and maximizing the acceptability of global positioning system device use for studying the role of human movement in dengue virus transmission in Iquitos, Peru. Am. J. Trop. Med. Hyg. 82, 723–730. doi: 10.4269/ajtmh.2010.09-0496
Peterman, W. E. (2014). ResistanceGA: an R package for the optimization of resistance surfaces using genetic algorithms. bioRxiv 1:007575. doi: 10.1101/007575
Peterman, W. E., Anderson, T. L., Ousterhout, B. H., Drake, D. L., Semlitsch, R. D., and Eggert, L. S. (2015). Differential dispersal shapes population structure and patterns of genetic differentiation in two sympatric pond breeding salamanders. Conserv. Genet. 16, 59–69. doi: 10.1007/s10592-014-0640-x
Peterman, W. E., Connette, G. M., Semlitsch, R. D., and Eggert, L. S. (2014). Ecological resistance surfaces predict fine-scale genetic differentiation in a terrestrial woodland salamander. Mol. Ecol. 23, 2402–2413. doi: 10.1111/mec.12747
Prunier, J. G., Kaufmann, B., Fenet, S., Picard, D., Pompanon, F., Joly, P., et al. (2013). Optimizing the trade-off between spatial and genetic sampling efforts in patchy populations: towards a better assessment of functional connectivity using an individual-based sampling scheme. Mol. Ecol. 22, 5516–5530. doi: 10.1111/mec.12499
Rees, E. E., Pond, B. A., Cullingham, C. I., Tinline, R., Ball, D., Kyle, C. J., et al. (2008). Assessing a landscape barrier using genetic simulation modelling: implications for raccoon rabies management. Prev. Vet. Med. 86, 107–123. doi: 10.1016/j.prevetmed.2008.03.007
Rees, E. E., Pond, B. A., Cullingham, C. I., Tinline, R. R., Ball, D., Kyle, C. J., et al. (2009). Landscape modelling spatial bottlenecks: implications for raccoon rabies disease spread. Biol. Lett. 5, 387–390. doi: 10.1098/rsbl.2009.0094
Rellstab, C., Gugerli, F., Eckert, A. J., Hancock, A. M., and Holderegger, R. (2015). A practical guide to environmental association analysis in landscape genomics. Mol. Ecol. 24, 4348–4370. doi: 10.1111/mec.13322
Richardson, J. L., Brady, S. P., Wang, I. J., and Spear, S. F. (2016). Navigating the pitfalls and promise of landscape genetics. Mol. Ecol. 25, 849–863. doi: 10.1111/mec.13527
Robinson, S. J., Samuel, M. D., Rolley, R. E., and Shelton, P. (2013). Using landscape epidemiological models to understand the distribution of chronic wasting disease in the Midwestern, U. S. A. Landsc. Ecol. 28, 1923–1935. doi: 10.1007/s10980-013-9919-4
Samarasin, P., Shuter, B. J., Wright, S. I., and Rodd, F. H. (2017). The problem of estimating recent genetic connectivity in a changing world. Conserv. Biol. 31, 126–135. doi: 10.1111/cobi.12765
Schoville, S. D., Bonin, A., François, O., Lobreaux, S., Melodelima, C., and Manel, S. (2012). Adaptive genetic variation on the landscape: methods and cases. Annu. Rev. Ecol. Evol. Syst. 43, 23–43. doi: 10.1146/annurev-ecolsys-110411-160248
Schwartz, M. K., McKelvey, K. S., Cushman, S. A., and Luikart, G. (2010). Landscape Genomics: A Brief Perspective. In Spatial Complexity, Informatics, and Wildlife Conservation. Tokyo: Springer. 165–174
Shafer, A., Northrup, J. M., White, K. S., Boyce, M. S., Côté, S. D., and Coltman, D. W. (2012). Habitat selection predicts genetic relatedness in an alpine ungulate. Ecology 93, 1317–1329. doi: 10.1890/11-0815.1
Shirk, A. J., Landguth, E. L., and Cushman, S. A. (2017). A comparison of individual-based genetic distance metrics for landscape genetics. Mol. Ecol. Resour. 17, 1308–1317. doi: 10.1111/1755-0998.12684
Shirk, A. J., Wallin, D. O., Cushman, S. A., Rice, C. G., and Warheit, K. I. (2010). Inferring landscape effects on gene flow: a new model selection framework. Mol. Ecol. 19, 3603–3619. doi: 10.1111/j.1365-294X.2010.04745.x
Slatkin, M. (1987). Gene flow and the geographic structure of natural populations. Science 236, 787–792. doi: 10.1126/science.3576198
Spear, S. F., Balkenhol, N., Fortin, M. J., McRae, B. H., and Scribner, K. (2010). Use of resistance surfaces for landscape genetic studies: considerations for parameterization and analysis. Mol. Ecol. 19, 3576–3591. doi: 10.1111/j.1365-294X.2010.04657.x
Spear, S. F., Peterson, C. R., Matocq, M. D., and Storfer, A. (2005). Landscape genetics of the blotched tiger salamander (Ambystoma tigrinum melanostictum). Mol. Ecol. 14, 2553–2564. doi: 10.1111/j.1365-294X.2005.02573.x
Storfer, A., Murphy, M. A., Evans, J. S., Goldberg, C. S., Robinson, S., Spear, S. F., et al. (2007). Putting the 'landscape'in landscape genetics. Heredity 98, 128–142. doi: 10.1038/sj.hdy.6800917
Storfer, A., Murphy, M. A., Spear, S. F., Holderegger, R., and Waits, L. P. (2010). Landscape genetics: where are we now?. Mol. Ecol. 19, 3496–3514. doi: 10.1111/j.1365-294X.2010.04691.x
Tatem, A. J., Rogers, D. J., and Hay, S. I. (2006). Global transport networks and infectious disease spread. Adv. Parasitol. 62, 293–343. doi: 10.1016/S0065-308X(05)62009-X
Taylor, A. R., Schaffner, S. F., Cerqueira, G. C., Nkhoma, S. C., Anderson, T. J. C., Sriprawat, K., et al. (2017). Quantifying connectivity between local Plasmodium falciparum malaria parasite populations using identity by descent. PLoS Genet. 13:e1007065. doi: 10.1371/journal.pgen.1007065
Thompson, E. A. (2013). Identity by descent: variation in meiosis, across genomes, and in populations. Genetics 194, 301–326. doi: 10.1534/genetics.112.148825
Tizzoni, M., Sun, K., Benusiglio, D., Karsai, M., and Perra, N. (2015). The scaling of human contacts and epidemic processes in metapopulation networks. Sci. Rep. 5:15111. doi: 10.1038/srep15111
Trainor, A. M., Walters, J. R., Morris, W. F., Sexton, J., and Moody, A. (2013). Empirical estimation of dispersal resistance surfaces: a case study with red-cockaded woodpeckers. Landsc. Ecol. 28, 755–767. doi: 10.1007/s10980-013-9861-5
Turner, M. G. (1989). Landscape ecology: the effect of pattern on process. Annu. Rev. Ecol. Syst. 20, 171–197. doi: 10.1146/annurev.es.20.110189.001131
van Etten, J. (2017). R Package gdistance: distances and routes on geographical grids. J. Stat. Softw. 76, 1–21. doi: 10.18637/jss.v076.i13
van Strien, M. J. (2017). Consequences of population topology for studying gene flow using link-based landscape genetic methods. Ecol. Evol. 7, 5070–5081. doi: 10.1002/ece3.3075
van Strien, M. J., Keller, D., and Holderegger, R. (2012). A new analytical approach to landscape genetic modelling: least-cost transect analysis and linear mixed models. Mol. Ecol. 21, 4010–4023. doi: 10.1111/j.1365-294X.2012.05687.x
Vazquez-Prokopec, G. M., Bisanzio, D., Stoddard, S. T., Paz-Soldan, V., Morrison, A. C., Elder, J. P., et al. (2013). Using GPS technology to quantify human mobility, dynamic contacts and infectious disease dynamics in a resource-poor urban environment. PLoS ONE 8:e58802. doi: 10.1371/journal.pone.0058802
Wang, I. J. (2013). Examining the full effects of landscape heterogeneity on spatial genetic variation: a multiple matrix regression approach for quantifying geographic and ecological isolation. Evolution 67, 3403–3411. doi: 10.1111/evo.12134
Wang, I. J., and Bradburd, G. S. (2014). Isolation by environment. Mol. Ecol. 23, 5649–5662. doi: 10.1111/mec.12938
Wang, I. J., Savage, W. K., and Shaffer, H. B. (2009). Landscape genetics and least-cost path analysis reveal unexpected dispersal routes in the California tiger salamander (Ambystoma californiense). Mol. Ecol. 18, 1365–1374. doi: 10.1111/j.1365-294X.2009.04122.x
Wang, Y. H., Yang, K. C., Bridgman, C. L., and Lin, L. K. (2008). Habitat suitability modelling to correlate gene flow with landscape connectivity. Landsc. Ecol. 23, 989–1000. doi: 10.1007/s10980-008-9262-3
Wesolowski, A., Buckee, C. O., Engø-Monsen, K., and Metcalf, C. J. (2016). Connecting mobility to infectious diseases: the promise and limits of mobile phone data. J. Infect. Dis. 214, S414–S420. doi: 10.1093/infdis/jiw273
Wu, J. (2004). Effects of changing scale on landscape pattern analysis: scaling relations. Landsc. Ecol. 19, 125–138. doi: 10.1023/B:LAND.0000021711.40074.ae
Keywords: landscape genetics, vector-borne diseases, molecular epidemiology, population genetics, spatial ecology, infectious diseases
Citation: Hemming-Schroeder E, Lo E, Salazar C, Puente S and Yan G (2018) Landscape Genetics: A Toolbox for Studying Vector-Borne Diseases. Front. Ecol. Evol. 6:21. doi: 10.3389/fevo.2018.00021
Received: 31 October 2017; Accepted: 20 February 2018;
Published: 08 March 2018.
Edited by:
Ben L. Phillips, University of Melbourne, AustraliaReviewed by:
Mariano Ordano, Fundación Miguel Lillo and Unidad Ejecutora Lillo (UEL-FML-CONICET), ArgentinaRubén Bueno-Marí, Lokimica Laboratorios, Spain
Copyright © 2018 Hemming-Schroeder, Lo, Salazar, Puente and Yan. This is an open-access article distributed under the terms of the Creative Commons Attribution License (CC BY). The use, distribution or reproduction in other forums is permitted, provided the original author(s) and the copyright owner are credited and that the original publication in this journal is cited, in accordance with accepted academic practice. No use, distribution or reproduction is permitted which does not comply with these terms.
*Correspondence: Guiyun Yan, Z3VpeXVueUB1Y2kuZWR1