- 1Geography Department, Humboldt-Universität zu Berlin, Berlin, Germany
- 2Faculty of Sustainability, Institute of Ecology & Centre of Methods, Leuphana Universität Lüneburg, Lüneburg, Germany
- 3Movement Ecology Laboratory, Department of Ecology, Evolution and Behavior, Alexander Silberman Institute of Life Sciences, Hebrew University of Jerusalem, Jerusalem, Israel
- 4Vogelschutzwarte Storchenhof Loburg e.V., Loburg, Germany
- 5Dynamic Macroecology, Department Landscape Dynamics, Swiss Federal Research Institute WSL, Birmensdorf, Switzerland
- 6Plant Ecology and Nature Conservation, Institute of Biochemistry and Biology, Universität Potsdam, Potsdam, Germany
- 7Leibniz-Centre for Agricultural Landscape Research (ZALF) e.V., Müncheberg, Germany
- 8Department of Evolutionary and Environmental Biology, University of Haifa, Haifa, Israel
- 9Department of Migration and Immuno-Ecology, Max-Planck-Institute for Ornithology, Radolfzell, Germany
- 10Department of Biology, University of Konstanz, Konstanz, Germany
- 11Berlin-Brandenburg Institute of Advanced Biodiversity Research, Berlin, Germany
Biotelemetry is increasingly used to study animal movement at high spatial and temporal resolution and guide conservation and resource management. Yet, limited sample sizes and variation in space and habitat use across regions and life stages may compromise robustness of behavioral analyses and subsequent conservation plans. Here, we assessed variation in (i) home range sizes, (ii) home range selection, and (iii) fine-scale resource selection of white storks across breeding status and regions and test model transferability. Three study areas were chosen within the Central German breeding grounds ranging from agricultural to fluvial and marshland. We monitored GPS-locations of 62 adult white storks equipped with solar-charged GPS/3D-acceleration (ACC) transmitters in 2013–2014. Home range sizes were estimated using minimum convex polygons. Generalized linear mixed models were used to assess home range selection and fine-scale resource selection by relating the home ranges and foraging sites to Corine habitat variables and normalized difference vegetation index in a presence/pseudo-absence design. We found strong variation in home range sizes across breeding stages with significantly larger home ranges in non-breeding compared to breeding white storks, but no variation between regions. Home range selection models had high explanatory power and well predicted overall density of Central German white stork breeding pairs. Also, they showed good transferability across regions and breeding status although variable importance varied considerably. Fine-scale resource selection models showed low explanatory power. Resource preferences differed both across breeding status and across regions, and model transferability was poor. Our results indicate that habitat selection of wild animals may vary considerably within and between populations, and is highly scale dependent. Thereby, home range scale analyses show higher robustness whereas fine-scale resource selection is not easily predictable and not transferable across life stages and regions. Such variation may compromise management decisions when based on data of limited sample size or limited regional coverage. We thus recommend home range scale analyses and sampling designs that cover diverse regional landscapes and ensure robust estimates of habitat suitability to conserve wild animal populations.
Introduction
Land use change and increasing intensity of agriculture have decreased biodiversity worldwide (Sala et al., 2000; Green, 2005; Morán-Ordóñez et al., 2017), resulting in degraded habitats, displacement of species and population declines (Chamberlain and Fuller, 2000; Flynn et al., 2009; Ripple et al., 2014). Hence, sustainable management of cultural landscapes, restoration of landscapes and re-wilding are important topics in conservation (Navarro and Pereira, 2012; Fischer et al., 2014) as are conflicts between conservation and landscape planning, for example because of wind farming (Reid et al., 2015; Vasilakis et al., 2016). Often, management concepts focus on umbrella species such as the white stork that need large areas of natural habitat and whose protection will also benefit other less iconic species (Olsson and Rogers, 2009). In recent years, technological advances in telemetry and GPS bio-logging devices have facilitated studying habitat and resource use of wildlife populations at high spatial and temporal resolution (Kays et al., 2015), and results of these efforts can help to design informed management plans for various species (Kaczensky et al., 2008; Wilson et al., 2015; Margalida et al., 2016).
Habitat use by animals depends on intrinsic and extrinsic factors, and home ranges and resource selection are strongly driven by the quality and quantity of available resources (Börger et al., 2008; Buchmann et al., 2011). In cultural landscapes, these typically show patchy distributions (Kramer-Schadt et al., 2004; Niebuhr et al., 2015). Hence, home ranges may show marked between-individual differences within species specific characteristics and depending on resources (Bastille-Rousseau et al., 2015; Rotics et al., 2017), density effects (Chamberlain and Fuller, 1999; Kjellander et al., 2004; Blix et al., 2014) and the extent of landscape alterations by humans (Gurarie et al., 2011). Similarly, habitat or resource preferences may vary depending on these factors (Gadenne et al., 2014).
In addition to habitat use, the behavioral and social aspects of the animals may play an important role in shaping their home ranges. Animals may show different movement modes depending on the individual life cycle stage. For example, non-breeding birds (or floaters) have been reported to move larger distances or show more nomadic behavior than breeding birds while utilizing the same types of habitats (Penteriani et al., 2011; Tanferna et al., 2013; Margalida et al., 2016). This behavioral change could be interpreted as avoidance of competition with breeding adults that must provide sufficient resources to their young, thereby increasing reproductive success at the population-level and long-term population persistence (Penteriani et al., 2011). Clearly, a more composite understanding of such environmental, biotic and behavioral effects on home range sizes and resource use is required in order to guide conservation actions in the face of ever-increasing land use changes and other human-related environmental alterations.
Here, we analyze variation of home range sizes and habitat use in white storks across space as well as life stages. Knowledge about such variation and, thus, about the transferability of habitat selection models across populations is important for individual and population-based analyses that may serve as basis for meaningful conservation management plans. Specifically, we assessed variation in (i) home range sizes, in (ii) home range selection, and in (iii) within-home range resource selection depending on breeding status and across regions. To this end, we quantified home range sizes and resource selection in white storks (Ciconia ciconia) from GPS telemetry and analyzed these with respect to breeding status and for three different study areas along a land use gradient. Habitat selection was evaluated at multiple scales (McGarigal et al., 2016), and we assessed variation in habitat configuration both at the level of bird home range and within the home range by studying fine-scale resource use (Tanferna et al., 2013; Kaczensky et al., 2014). For the latter, we ensured that fine-scale movements are indicative of resource utilization and not confounded with other activities (Kie et al., 2010) by filtering the GPS data to feeding events using ACC-derived behavioral classification (Nathan et al., 2012; Resheff et al., 2014; Rotics et al., 2016).
White storks are large, iconic birds of general conservation concern in Europe that often serve as umbrella species for restoring wetlands. They are long-distance soaring migrants that usually breed in Central and East Europe and spend the winter in Africa. During the nestling-rearing phase in May-July, they exhibit typical central-place foraging within ca. 5 km radius from the nest (Zurell et al., 2015). White storks are opportunistic foragers feeding on insects, earthworms, amphibians, and small rodents (Alonso et al., 1991; Antczak et al., 2002). Although they preferably feed in wet open landscapes with low vegetation, they increasingly rely on agricultural landscapes (Tryjanowski et al., 2009) such as agricultural meadows (Johst et al., 2001) where monocultures and herbicide use have a negative effect on food resources (Kosicki, 2010). We chose three different sites along a land use gradient ranging from agriculturally dominated to fluvial and marshland landscapes with increasing amounts of pastures. We hypothesize variation in white stork space and resource use depending (i) on breeding status as has been shown in black kites (Tanferna et al., 2013) and (ii) on region because of regional differences in resource availability and distribution, for example due to different proportions of vertebrate and invertebrate prey.
Materials and Methods
Study Area and Data
The study sites are situated in the Central German breeding grounds in the vicinity of Loburg (52.118° N, 12.087° E), Beuster (52.939° N, 11.787° E), and the Drömling Nature Park (52.489° N, 11.022° E; Supplementary Figure S1). While all sites show a large amount of arable land (47–56%), they exhibit a land use gradient from low amounts of pastures in Loburg (6%) to high amounts in the fluvial area of Beuster (25%) and in the marshland landscape of Dromling (31%). At the same time, the amount of forested area decreases from Loburg (27%) to Beuster (15%) and Dromling (7%; Supplementary Figure S2).
From 2011 to 2014, we fitted solar-charged GPS/ACC transmitters (E-Obs GmbH; Munich, Germany) to 62 adult white storks in Germany. Transmitter plus mounting harness weight was about 2% of the average stork weight (Rotics et al., 2016), which is below the recommended threshold in wildlife tracking (Kenward, 2000). The transmitters recorded GPS locations (50% confidence intervals for accuracy are ±3.6 m) and ground speed between 2:00 and 20:00 GMT and 3-dimensional body acceleration (ACC) between 3:00 and 19:00 GMT. GPS location and speed were recorded every 5 min when solar recharge was high (ca. 95% of the time) and every 20 min otherwise. Body acceleration was recorded every 5 min. In our analyses, we restricted the tracking data to the early nestling-rearing phase of the first 30 days after hatching if hatching date was known or to the month of June when all nestlings are known to hatch. Usually, white storks care for their brood for 70 days (Van den Bossche et al., 2002; Tryjanowski et al., 2006). If the brood is lost, the pair typically stays together or even attempts another brood if early enough in the season (personal observation, M. Kaatz). In later stages of nestling-rearing, the adults leave the nests for longer time periods and distances. We thus chose to concentrate on the early nestling-rearing phase in order to capture distinct behavioral differences between breeding and non-breeding adults. We further restricted the tracking data to the 2013 and 2014 breeding seasons because many birds were tagged late in 2011–2012 and data were not available for the entire breeding season for these years. Overall, we analyzed tracking data for 31 individuals in 2013 and 34 individuals in 2014, of which 24 individuals were tracked in both years (Table 1).
For the resource selection analyses, we filtered the GPS data to foraging occasions using ACC-based behavioral classifiers in order to exclude potential biases that may arise from including GPS fixes corresponding to flight or rest. We used a supervised machine-learning algorithm (radial-basis-function kernel support vector machine) to classify the ACC records to behavioral modes (Nathan et al., 2012; Resheff et al., 2014). The model was trained on 3,815 ground-truthed ACC records of known behaviors and classified seven behavioral modes: active flight (flapping), passive flight (soaring or gliding), walking, pecking, standing, sitting, and preening, with an overall accuracy of 92% (Rotics et al., 2016). These behavioral classes were used to limit the analyzed GPS locations to foraging activities only (walking and pecking).
Habitat information was extracted from the Corine Land Cover 2006 raster map at a resolution of 100 m (https://www.eea.europa.eu/ds_resolveuid/ba3deb09ce0e4151a02a33dcd585aa04). Relevant classes occurring in the study area are urban areas (class 2), non-irrigated arable land (12), pastures (18) and mosaics (open semi-natural landscapes; 21), broad-leaved (23), coniferous (24) and mixed forests (25), woodland-shrub associations (29), and water bodies (40) (Figure 1). Additionally, we obtained MOD13Q1 Normalized Difference Vegetation Index (NDVI) data at 16 day and 250 m resolution for the considered early-nestling periods 2013 and 2014 (Didan, 2015). Overall, we obtained six NDVI tiles for 2013 and seven NDVI tiles for 2014 with useful to good reliability in 84–100% of the pixels (mean ± sd 98 ± 4%). NDVI ranged 0–0.9 in the study region (Supplementary Figure S1). For home range selection and the resource selection analyses, Corine and NDVI data were aggregated at the home range scale or used as point location, which we explain below. Furthermore, in the home range selection analyses, NDVI was averaged over all 16-day layers that matched the considered time span for each stork (first 30 days after hatching). In the resource selection analyses, each GPS location was matched to the respective 16-day NDVI layer. At both scales (home range scale and point location), bivariate Spearman correlations between Corine and NDVI data were |r| < 0.7, which is generally regarded as threshold under which collinearity is unproblematic (Dormann et al., 2013).
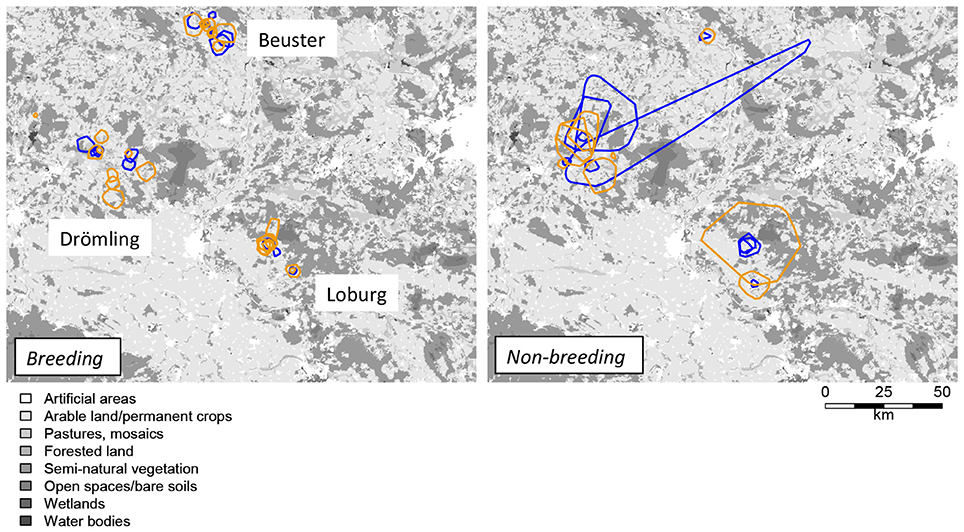
Figure 1. Home ranges of breeding and non-breeding (including brood loss) white storks in years 2013 (blue) and 2014 (orange). Presented are the 95% minimum convex polygons. Gray shading indicates Corine land cover types from 2006; for a colored version of land cover map See Supplementary Figure S1.
Home Range Size and Selection
Home range sizes were estimated using minimum convex polygons (MCP). We used different quantiles for estimating home ranges: 50% representing core areas, 95% as the most commonly applied conservative estimate excluding extreme outliers, and 99.9% including (almost) all points. MCPs constitute very simple home range estimators. However, we judge these as purposeful for subsequent analyses and, also, although previous comparisons showed significant differences between home range estimators, all tested estimators based on GPS data showed excellent performance in terms of Area under the ROC curve (AUC) with values above 0.9 in all cases (Walter et al., 2015). We evaluated differences in home range size depending on breeding status, location, sex, and year using ANOVA.
Home range selection models were used to assess whether the habitat composition within the 95% MCPs were different from random expectation. For this, we compared observed home ranges against habitat compositions of pseudo home ranges of the same shape to control for home range size. The data thus follow a presence-absence design (occupied home range vs. pseudo home range). For each observed home range, one pseudo home range was simulated by randomly shifting the center coordinates of each home range within the study area extent. Then, we calculated the percentage cover of each land cover class as well as the mean NDVI within all home ranges and pseudo home ranges to characterize home range composition. We used generalized linear mixed models to relate home range selection to habitat composition with occupied home range vs. pseudo home range as response variable, proportional land cover, and mean NDVI as predictor variables, and year and animal as random factors. However, as the random effects did not improve models in terms of AIC over simpler models, these were discarded and we subsequently used simple generalized linear models (Zuur et al., 2009). Our aim was to compare whether home range selection varied across regions and across life stages, and we thus estimated separate GLMs for each region and breeding status. However, this limits the available sample sizes substantially (Table 1). We thus adapted a recently proposed framework based on ensembles of small models (Lomba et al., 2010; Breiner et al., 2015). This involved fitting a larger number of bivariate models with only two predictors at a time, and averaging them in an ensemble prediction using weights based on models performances. We thus estimated all combinations of bivariate generalized linear models (GLMs) with binomial error structure with occupied home range vs. pseudo home range as response variable, and proportional land cover and mean NDVI as predictor variables. For NDVI, we included both the linear and quadratic term. The ensembles of bivariate models were averaged based on Akaike information criterion. We tested prediction accuracy of the models by estimating the area under the ROC curve (AUC), which ranges between 0 and 1, with 1 indicating perfect discrimination, 0.5 indicating predictions no better than chance, and values above 0.7 indicating useful predictions (Hosmer and Lemeshow, 2000). Model performance was evaluated internally by predicting to the training data set and cross-prediction accuracy was evaluated by predicting to a different data set (or subset). We were mainly interested how models from different regions and life stages were able to predict suitability for establishing breeding home ranges. We thus cross-predicted models calibrated on breeding stork home ranges from different regions (incl. the entire study area) to all other regions. Also, we cross-predicted models calibrated on non-breeding stork home ranges from different regions to breeding stork home ranges of different regions.
Within-Home Range Resource Selection
Resource selection was evaluated for foraging occasions only, and GPS data were filtered using ACC-based behavioral classification. To reduce temporal autocorrelation, we only included data points that were recorded at least 30 min apart. In breeding white storks, foraging trips last up to 2 h (Johst et al., 2001) such that our temporal resolution corresponds to a maximum of 4 data points per foraging trip. We tested three different buffer radii (1, 2.5, and 5 km) for deriving pseudo-absences. Then, for each presence point we randomly sampled 3 pseudo-absences from within the buffer. With gliding velocities of 0.5–1 km/min and additional time costs for circling in thermals and orientation (Johst et al., 2001), 5 km approximately correspond to the maximum (return) flight distance within 30 min while most storks exhibit much smaller flight distances during the nestling-rearing phase (Zurell et al., 2015). Then, for each presence and pseudo-absence point, we extracted the land cover and NDVI value at this point location and time. We used GLMMs with binomial error structure to infer resource selection from use-availability data with presence vs. pseudo-absence as response variable, animal, year, and region as random factors, and the different land cover classes and NDVI as predictor variables. For NDVI, we included both the linear and quadratic term. We also estimated separate GLMMs for each region with the difference that only animal and year were considered as random factors. Inspection of model residuals by Moran's I correlograms showed no significant spatial autocorrelation; thus, we did not investigate this further. Analogously to the home range selection analyses, we tested cross-prediction accuracy of the models in terms of AUC.
All analyses were carried out with R version 3.2.3 (R Core Team, 2015) using the packages adehabitatHR (Calenge, 2006), MuMIn (Barton, 2016), lme4 (Bates et al., 2015), and ncf (Bjornstad, 2016).
Results
Home Range Sizes
Home range sizes differed considerably between individuals with mean (± SD) size of 78.3 ± 219.9 km2 (MCP 95%; Table 2). We could not find any significant differences in home range size between different locations, sexes or years, whereas we found significant differences in home range size depending on breeding status (Supplementary Table S1). Effects of non-breeding white storks and stork having lost their brood were not consistent between different home range quantiles and we subsequently pooled these groups into one group of non-breeding storks. Overall, the home ranges of non-breeding white storks were significantly larger than those of breeding storks (Table 2, Figure 1).
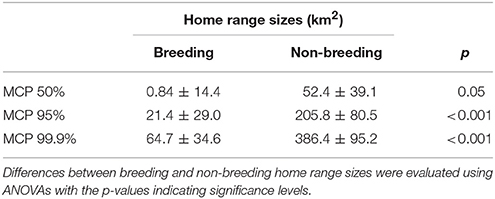
Table 2. Mean home range sizes (± standard error) estimated using minimum convex polygons (MCP) depending on breeding status.
Home Range Selection Analyses
Home range selection in white storks was significantly different from random, and the presence of occupied home ranges was well explained by proportional land cover and NDVI with explained variances of 41–68% (Table 3). Thereby, the home range selection model calibrated on non-breeding storks in Beuster needs to be judged with caution as the low sample size (only three non-breeding storks) led to inflated parameter estimates. According to our models, breeding white storks select home ranges that contain a mix of semi-natural habitats with significant preference for non-irrigated arable land, pastures and semi-natural open landscape mosaics, while forests tend to be avoided (Table 3). Variable importance differed between breeding and non-breeding storks and across regions (Figures 2A,B). In breeding storks, pastures were most important in all regions, while the importance of other variables varied more. Pastures also showed up as important variable in non-breeding storks, but variable importance across regions was much more variable, and avoidance of forests was overall more important than in breeding storks. Despite differences in variable coefficients and importance, models of breeding white storks cross-predicted well to other regions with AUC scores above 0.7 (Figure 2C). By contrast, models calibrated on non-breeding storks were less successful at predicting breeding home range selection. Especially, models trained in the Loburg region were performing worse than random (Figure 2D). Using the model-averaged GLMs calibrated on breeding home ranges from the entire study area, we predicted home range suitability (the probability of establishing a home range based on the habitat compositions in a given patch or landscape) across the study region and compared this against observed white stork breeding pair density per municipal district. We found a significant Pearson correlation of 0.62 indicating that our home range selection model well explained observed white stork density in Central Germany (t = 4.12, p < 0.001) (Supplementary Table S2, Supplementary Figure S3).
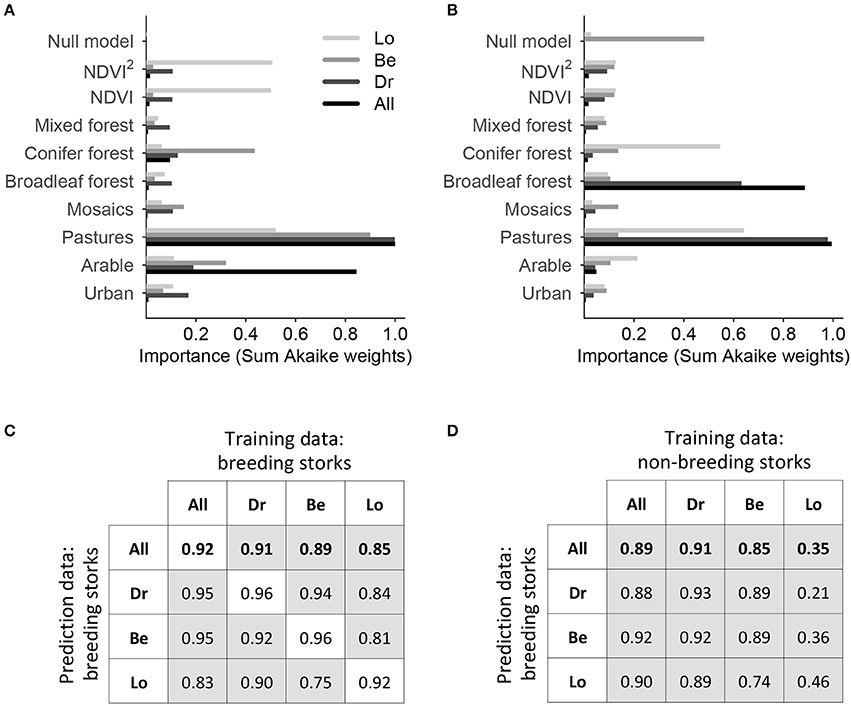
Figure 2. Variable importance (A,B) and cross-prediction results (C,D) of home range selection models for breeding (A,C) and non-breeding white storks (B,D). To reduce bias from small sample sizes, we used ensembles of small bivariate generalized linear models fitted with binomial error structure (1 for occupied home range, 0 for random home range). Models were fitted for the entire study area (All = entire region) and for single regions (Dr, Drömling; Be, Beuster; Lo, Loburg). (A,B) Variable importance for each region and breeding status is given by the sum of Akaike weights over all bivariate models including the respective variable. (C,D) We assessed the ability of the ensembles to predict breeding home ranges in the entire area or single regions by calculating the area under ROC curve (AUC), which ranges between 0 and 1, with 1 indicating perfect discrimination and 0.5 predictions no better than chance. AUC scores for predicting all breeding storks are highlighted in bold. White boxes indicate internal predictions, meaning that training and prediction data were identical.
Resource Selection Analyses
The results of the fine-scale resource selection model based only on foraging events were largely consistent with the coarse-scale home range configuration analyses. Tracked white storks chose foraging sites that were significantly different from random, although the explained variance was comparably low, ranging from 3 to 14% (Table 4, Supplementary Table S3). We found important differences in resource selection depending on breeding status, and to a lesser extent depending on region. While breeding white storks showed general preference for non-forested landscapes (urban, arable, pasture, and semi-natural mosaic landscapes), non-breeding storks showed no preference for arable land and tended to avoid mosaic landscapes. Preference and avoidance of forests further differed between regions. While breeding storks of Drömling and Loburg generally avoided forests, storks of Beuster also foraged in (or near) broad-leaved and mixed forests. These differences in model coefficients led to differences in predicted resource suitability (Figure 3). Models calibrated on breeding stork data from Dromling and Loburg tended to underpredict resource suitability (relative to the model calibrated on all available breeding stork data), while the model calibrated on Beuster breeding stork data overpredicted resource suitability (Figure 3B). Models calibrated on non-breeding storks generally underpredicted resource suitability (relative to the model calibrated on all available breeding stork data; Figure 3C). However, discrimination ability of the fine-scale resource selection models was generally very poor. For models calibrated on breeding stork data, AUC ranged from 0.62 to 0.67 for internal validation and from 0.56 to 0.66 for cross-predictions between regional data sets (Figure 3D). When cross-predicting from non-breeding to breeding storks, predictive performance was even lower and not much better than random (Figure 3E).
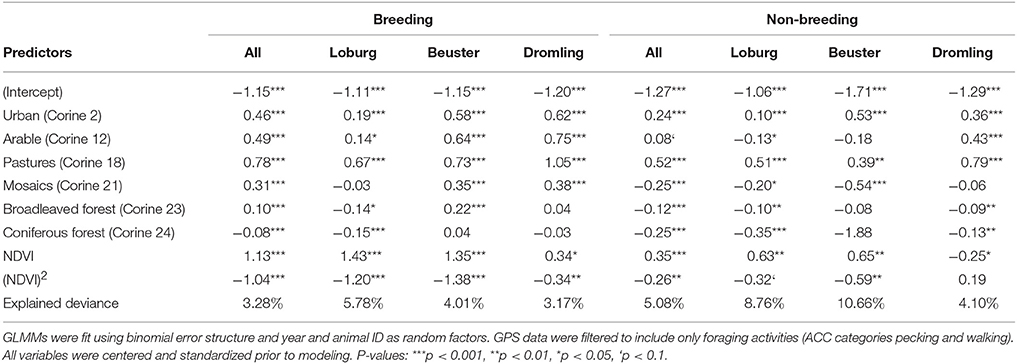
Table 4. Estimates of generalized linear mixed models (GLMMs) quantifying fine-scale resource selection of breeding and non-breeding white storks within 1 km radii for different regions.
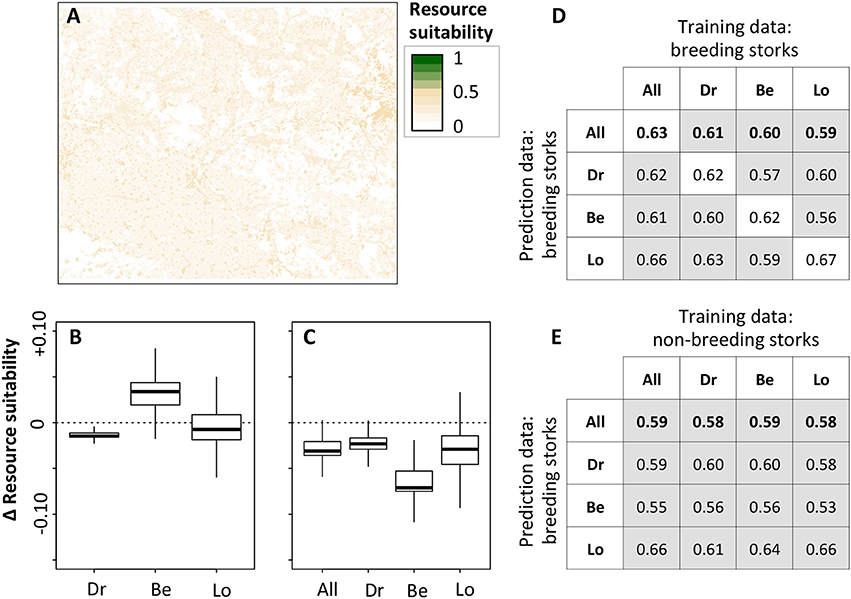
Figure 3. Predicted resource suitability and cross-prediction results from fine-scale resource selection analysis. GLMMs were fitted using binomial error structure with presences and pseudo-absences (within 1 km buffers around presence points) as response variable, year, and animal as random factors, and Corine land cover variables and NDVI as predictor variables. GPS data were filtered to include only foraging activities (ACC categories pecking and walking). (A) shows the predicted resource suitability from a GLMM calibrated on breeding stork data from entire study region. (B,C) show overprediction (positive) and underprediction (negative) of predicted resource suitability (relative to a) when models were calibrated on data from (B) breeding white storks and (C) non-breeding white storks in different regions (All = entire region, Dr, Drömling; Be, Beuster; Lo, Loburg). (D,E) show the cross-prediction results in terms of AUC. AUC scores for predicting all breeding storks are highlighted in bold. White boxes indicate internal predictions, meaning that training and prediction data were identical.
Discussion
Building on a large and highly detailed set of spatially and temporally resolved movement and behavioral data of white storks in the Central German breeding grounds, we investigated variation in home range sizes and multi-scale habitat selection between and within (sub-) populations. Our results corroborated earlier findings that breeding status considerably affects space use and habitat selection in birds (Tanferna et al., 2013). Additionally, we were able to show that white storks may exhibit regional differences in home range and resource selection. These results call for caution when deriving large-scale management strategies from limited sample sizes or from a limited number of (sub-)populations or regions because these may not capture the full spectrum of habitat selection behaviors.
We assessed within and between-population variation in three main features of space and habitat use in birds, namely home range size, home range selection, and fine-scale resource selection. First, we expected home range size to differ with breeding status and across regions. In our study region, breeding and non-breeding individuals (floaters) co-occur, and non-breeding individuals can be expected to be of similar age as breeding individuals as white storks typically return to the breeding grounds only after reaching maturity. Floaters are thus individuals that did not attempt to breed, for example because of territory competition, late arrival, no arrival of the partner, or individuals that have lost their brood during the breeding season, for example because of adverse weather or predation. Confirming previous findings from other bird species, home range sizes were significantly larger for non-breeding compared to breeding individuals (Tanferna et al., 2013; Reid et al., 2015; Margalida et al., 2016). These differences were consistent irrespective of whether the individuals did not attempt to breed at all or lost their eggs or brood during the breeding season. By contrast, we did not find any significant differences in home range sizes between regions. Hence, white storks seem to be very conservative in their overall space use, which is probably related to energetic and behavioral constraints. When caring for a brood, white storks return to their nest to feed (and shelter) at least every 2 h, which ultimately limits their activity radius (Johst et al., 2001; Zurell et al., 2015). There seem to be differences in home range sizes of non-breeding storks between regions (Figure 1), but we could not evaluate this statistically because of low sample size.
Second, we assessed habitat selection at the home range scale and compared home range selection across breeding status and across regions. Overall, our home range selection models showed high predictive performance and well predicted overall density of white stork breeding pairs in the study area. The models indicated differences in variable importance for breeding and non-breeding individuals, with non-breeding storks showing less preference for arable land and a stronger avoidance of forested areas. Most models cross-predicted well, meaning that region-specific models and also models calibrated on non-breeding storks were well able to predict home range selection of breeding storks over the entire study area. The exception was the home range selection model calibrated on non-breeding storks in the Loburg region that showed very poor cross-prediction performance. These results partially corroborate our hypotheses that home range selection varies across regions and across life stages. Because of the opportunistic foraging behavior of white storks, we expected regional differences in white stork home range selection. From previous analyses on black kites (Tanferna et al., 2013), we expected home range selection to differ between non-breeding and breeding birds because floaters typically show a more nomadic behavior where normal home range activities of foraging, mating and caring for young are (partially) replaced by more explorative and sporadic activities (Burt, 1943; Kie et al., 2010). Overall, our results indicate that at the home range scale, breeding white storks are largely conservative in their habitat selection. Yet, home range selection of non-breeding storks may differ more strongly from that of breeding storks and also between regions. From the current results, we cannot infer the reasons why especially the non-breeding storks of Loburg behaved differently. Although wild storks are not fed at or near the Loburg white stork sanctuary, we cannot completely rule out any anthropogenic effects. Nevertheless, our results indicate that home range selection is sensitive to life stage and regional differences.
Lastly, we assessed variation in fine-scale resource selection during foraging within and between (sub-)populations. We found support for both our hypotheses that resource (or foraging site) selection differs according to breeding status and across regions. Yet, similar to the analyses of home range selection, differences between breeding and non-breeding storks were slightly more pronounced than differences between regions. Generally, non-breeding storks tended to be more selective for a few habitat types. This could mirror the high pressure of breeding white storks to gather food within limited time and movement distance (Johst et al., 2001) while non-breeding white storks are relieved from such constraints. Overall, however, all resource-selection models showed poor predictive performance and poor cross-prediction. Thus, fine-scale resource selection seems rather variable and much less conservative than habitat selection at the home range scale. Foraging site selection may depend on several other factors, for example individual behavior and individual experience, weather conditions, biotic factors such as the presence of competitors or predators, anthropogenic disturbances. Beside these factors, foraging site selection also seems to be random to some extent, corroborating the opportunistic foraging behavior of white storks.
Our results show that white storks are highly conservative in their overall space use and, to some extent, also in their home range selection behavior. By contrast, fine-scale resource selection is hardly predictable and also not transferable across breeding status and regions. Thus, habitat selection may vary within and between populations, and the magnitude of this variation is highly scale dependent, which needs to be taken into account when using habitat selection studies to inform landscape planning and conservation management (Reid et al., 2015; Margalida et al., 2016; Vasilakis et al., 2016). Highly resolved movement data are becoming increasingly available through advances in GPS technology and development of increasingly smaller data loggers (Kranstauber et al., 2011; Kays et al., 2015). These technological advances offer great opportunities to study different aspects of animals' life history and space use (Flack et al., 2016; Rotics et al., 2017) and can substantially aid conservation and management of wild populations (Wilson et al., 2015; McGowan et al., 2017). However, studies based on biotelemetry tags are often limited to relatively low sample size, for example because deployment of tags is costly and individuals may be difficult to capture. Here, we show that space use and multi-scale habitat selection of wild animals may considerably differ with breeding status and between regions. This calls for caution when informing conservation actions and management decisions on data of limited sample size or regional coverage. We thus recommend diverse sampling designs that cover both within and between population variations, which will facilitate more robust estimates of habitat suitability for wild animal populations.
Data Availability Statement
The data will be archived in Movebank and will be available upon reasonable request.
Ethics Statement
This research was carried out with approvals from (a) the National Administrative Office of Sachsen-Anhalt, Germany, Division of Nature Conservation, 407.3.3/255.13-2248/2, (b) the State Office for Environment, Health and Consumer Protection of Brandenburg, Germany, V3-2347-8-2012.
Author Contributions
DZ, HvW, and FJ designed the study with the help of SR, MW, and RN. SR, MK, and ST collected the data. SR, HG, LS, and MS contributed to data preparation and analyses. DZ ran the analyses, and drafted the manuscript. All authors significantly contributed to editing and revising the manuscript.
Funding
We acknowledge the generous funding of DIP grants (DFG) NA 846/1-1 and WI 3576/1-1 to RN, FJ, and MW. FJ further acknowledges support by Deutsche Forschungsgemeinschaft in the framework of the BioMove Research Training Group (DFG-GRK 2118/1). We acknowledge support by the German Research Foundation (DFG) and the Open Access Publication Fund of Humboldt-Universität zu Berlin.
Conflict of Interest Statement
The authors declare that the research was conducted in the absence of any commercial or financial relationships that could be construed as a potential conflict of interest.
Acknowledgments
The MOD13Q1 data product was retrieved from the online Data Pool, courtesy of the NASA Land Processes Distributed Active Archive Center (LP DAAC), USGS/Earth Resources Observation and Science (EROS) Center, Sioux Falls, South Dakota, https://lpdaac.usgs.gov/data_access/data_pool.
Supplementary Material
The Supplementary Material for this article can be found online at: https://www.frontiersin.org/articles/10.3389/fevo.2018.00079/full#supplementary-material
References
Alonso, J. C., Alonso, J. A., and Carrascal, L. M. (1991). Habitat selection by foraging White Storks, Ciconia ciconia, during the breeding season. Can. J. Zool. 69, 1957–1962. doi: 10.1139/z91-270
Antczak, M., Konwerski, S., Grobelny, S., and Tryjanowski, P. (2002). The food composition of immature and non-breeding white storks in Poland. Waterbirds 25, 424–428. doi: 10.1675/1524-4695(2002)025[0424:TFCOIA]2.0.CO;2
Barton, K. (2016). MuMIn: Multi-Model Inference. R Package Version 1.15.6. Available online at: https://CRAN.R-project.org/package=MuMIn
Bastille-Rousseau, G., Potts, J. R., Schaefer, J. A., Lewis, M. A., Ellington, E. H., Rayl, N. D., et al. (2015). Unveiling trade-offs in resource selection of migratory caribou using a mechanistic movement model of availability. Ecography 38, 1049–1059. doi: 10.1111/ecog.01305
Bates, D., Maechler, M., Bolker, B., and Walker, S. (2015). Fitting linear mixed-effects models using lme4. J. Stat. Softw. 67, 1–48. doi: 10.18637/jss.v067.i01
Bjornstad, O. N. (2016). ncf: Spatial Nonparametric Covariance Functions. Available online at: https://CRAN.R-project.org/package=ncf
Blix, A. W., Mysterud, A., Loe, L. E., and Austrheim, G. (2014). Temporal scales of density-dependent habitat selection in a large grazing herbivore. Oikos 123, 933–942. doi: 10.1111/oik.01069
Börger, L., Dalziel, B. D., and Fryxell, J. M. (2008). Are there general mechanisms of animal home range behaviour? A review and prospects for future research. Ecol. Lett. 11, 637–650. doi: 10.1111/j.1461-0248.2008.01182.x
Breiner, F. T., Guisan, A., Bergamini, A., and Nobis, M. P. (2015). Overcoming limitations of modelling rare species by using ensembles of small models. Methods Ecol. Evol. 6, 1210–1218. doi: 10.1111/2041-210X.12403
Buchmann, C. M., Schurr, F. M., Nathan, R., and Jeltsch, F. (2011). An allometric model of home range formation explains the structuring of animal communities exploiting heterogeneous resources. Oikos 120, 106–118. doi: 10.1111/j.1600-0706.2010.18556.x
Burt, W. H. (1943). Territoriality and home range concepts as applied to mammals. J. Mammal. 24, 346–352. doi: 10.2307/1374834
Calenge, C. (2006). The package adehabitat for the R software: tool for the analysis of space and habitat use by animals. Ecol. Modell. 197, 1035–1035. doi: 10.1016/j.ecolmodel.2006.03.017
Chamberlain, D. E., and Fuller, R. J. (1999). Density-dependent habitat distribution in birds: issues of scale, habitat definition and habitat availability. J. Avian Biol. 30, 427–436. doi: 10.2307/3677015
Chamberlain, D. E., and Fuller, R. J. (2000). Local extinctions and changes in species richness of lowland farmland birds in England and Wales in relation to recent changes in agricultural land-use. Agric. Ecosyst. Environ. 78, 1–17. doi: 10.1016/S0167-8809(99)00105-X
Didan, K. (2015). MOD13Q1 MODIS/Terra vegetation indices 16-day L3 global 250m SIN Grid V006 [Data set]. NASA EOSDIS LP DAAC. doi: 10.5067/MODIS/MOD13Q1.006
Dormann, C. F., Elith, J., Bacher, S., Buchmann, C. M., Carl, G., Carré, G., et al. (2013). Collinearity: a review of methods to deal with it and a simulation study evaluating their performance. Ecography 36, 27–46. doi: 10.1111/j.1600-0587.2012.07348.x
Fischer, J., Abson, D. J., Butsic, V., Chappell, M. J., Ekroos, J., Hanspach, J., et al. (2014). Land sparing versus land sharing: moving forward. Conserv. Lett. 7, 149–157. doi: 10.1111/conl.12084
Flack, A., Fiedler, W., Blas, J., Pokrovsky, I., Kaatz, M., Mitropolsky, M., et al. (2016). Costs of migratory decisions: a comparison across eight white stork populations. Sci. Adv. 2:e1500931. doi: 10.1126/sciadv.1500931
Flynn, D. F. B., Gogol-Prokurat, M., Nogeire, T., Molinari, N., Trautman Richers, B., Lin, B. B., et al. (2009). Loss of functional diversity under land use intensification across multiple taxa. Ecol. Lett. 12, 22–33. doi: 10.1111/j.1461-0248.2008.01255.x
Gadenne, H., Cornulier, T., Eraud, C., Barbraud, J.-C., and Barbraud, C. (2014). Evidence for density-dependent habitat occupancy at varying scales in an expanding bird population. Popul. Ecol. 56, 493–506. doi: 10.1007/s10144-014-0435-4
Green, R. E. (2005). Farming and the fate of wild nature. Science 307, 550–555. doi: 10.1126/science.1106049
Gurarie, E., Suutarinen, J., Kojola, I., and Ovaskainen, O. (2011). Summer movements, predation and habitat use of wolves in human modified boreal forests. Oecologia 165, 891–903. doi: 10.1007/s00442-010-1883-y
Hosmer, D. W., and Lemeshow, S. (2000). Applied Logistic Regression. New York, NY: John Wiley & Sons, Inc.
Johst, K., Brandl, R., and Pfeifer, R. (2001). Foraging in a patchy andlandscape landscape: human land use and the white stork. Ecol. Appl. 11, 60–69. doi: 10.1890/1051-0761(2001)011[0060:FIAPAD]2.0.CO;2
Kaczensky, P., Adiya, Y., von Wehrden, H., Mijiddorj, B., Walzer, C., Güthlin, D., et al. (2014). Space and habitat use by wild Bactrian camels in the Transaltai Gobi of southern Mongolia. Biol. Conserv. 169, 311–318. doi: 10.1016/j.biocon.2013.11.033
Kaczensky, P., Ganbaatar, O., Wehrden, H. V., and Walzer, C. (2008). Resource selection by sympatric wild equids in the Mongolian Gobi. J. Appl. Ecol. 45, 1762–1769. doi: 10.1111/j.1365-2664.2008.01565.x
Kays, R., Crofoot, M. C., Jetz, W., and Wikelski, M. (2015). Terrestrial animal tracking as an eye on life and planet. Science 348:aaa2478. doi: 10.1126/science.aaa2478
Kie, J. G., Matthiopoulos, J., Fieberg, J., Powell, R. A., Cagnacci, F., Mitchell, M. S., et al. (2010). The home-range concept: are traditional estimators still relevant with modern telemetry technology? Phil. Trans. R. Soc. B 365, 2221–2231. doi: 10.1098/rstb.2010.0093
Kjellander, P., Hewison, A. J. M., Liberg, O., Angibault, J. M., Bideau, E., and Cargnelutti, B. (2004). Experimental evidence for density-dependence of home-range size in roe deer (Capreolus capreolus L.): a comparison of two long-term studies. Oecologia 139, 478–485. doi: 10.1007/s00442-004-1529-z
Kosicki, J. Z. (2010). Reproductive success of the white stork Ciconia ciconia population in intensively cultivated farmlands in Western Poland. Ardeola 57, 243–255.
Kramer-Schadt, S., Revilla, E., Wiegand, T., and Breitenmosers, U. (2004). Fragmented landscapes, road mortality and patch connectivity: modelling influences on the dispersal of Eurasian lynx. J. Appl. Ecol. 41, 711–723. doi: 10.1111/j.0021-8901.2004.00933.x
Kranstauber, B., Cameron, A., Weinzerl, R., Fountain, T., Tilak, S., Wikelski, M., et al. (2011). The Movebank data model for animal tracking. Environ. Model. Softw. 26, 834–835. doi: 10.1016/j.envsoft.2010.12.005
Lomba, A., Pellissier, L., Randin, C., Vicente, J., Moreira, F., Honrado, J., et al. (2010). Overcoming the rare species modelling paradox: a novel hierarchical framework applied to an Iberian endemic plant. Biol. Conserv. 143, 2647–2657. doi: 10.1016/j.biocon.2010.07.007
Margalida, A., Perez-Garcia, J. M., Afonso, I., and Moreno-Opo, R. (2016). Spatial and temporal movements in Pyrenean bearded vultures (Gypaetus barbatus): integrating movement ecology into conservation practice. Sci. Rep. 6:35746. doi: 10.1038/srep35746
McGarigal, K., Wan, H. Y., Zeller, K. A., Timm, B. C., and Cushman, S. A. (2016). Multi-scale habitat selection modeling: a review and outlook. Landsc. Ecol. 31, 1161–1175. doi: 10.1007/s10980-016-0374-x
McGowan, J., Beger, M., Lewison, R. L., Harcourt, R., Campbell, H., Priest, M., et al. (2017). Integrating research using animal-borne telemetry with the needs of conservation management. J. Appl. Ecol. 54, 423–429. doi: 10.1111/1365-2664.12755
Morán-Ordóñez, A., Whitehead, A. L., Luck, G. W., Cook, G. D., Maggini, R., Fitzsimons, J. A., et al. (2017). Analysis of trade-offs between biodiversity, carbon farming and agricultural development in northern australia reveals the benefits of strategic planning. Conserv. Lett. 10, 94–104. doi: 10.1111/conl.12255
Nathan, R., Spiegel, O., Fortmann-Roe, S., Harel, R., Wikelski, M., and Getz, W. M. (2012). Using tri-axial acceleration data to identify behavioral modes of free-ranging animals: general concepts and tools illustrated for griffon vultures. J. Exp. Biol. 215, 986–996. doi: 10.1242/jeb.058602
Navarro, L. M., and Pereira, H. M. (2012). Rewilding abandoned landscapes in Europe. Ecosystems 15, 900–912. doi: 10.1007/s10021-012-9558-7
Niebuhr, B. B. S., Wosniack, M. E., Santos, M. C., Raposo, E. P., Viswanathan, G. M., da Luz, M. G. E., et al. (2015). Survival in patchy landscapes: the interplay between dispersal, habitat loss and fragmentation. Sci. Rep. 5:11898. doi: 10.1038/srep11898
Olsson, O., and Rogers, D. J. (2009). Predicting the distribution of a suitable habitat for the white stork in Southern Sweden: identifying priority areas for reintroduction and habitat restoration. Anim. Conserv. 12, 62–70. doi: 10.1111/j.1469-1795.2008.00225.x
Penteriani, V., Ferrer, M., and Delgado, M. M. (2011). Floater strategies and dynamics in birds, and their importance in conservation biology: towards an understanding of nonbreeders in avian populations. Anim. Conserv. 14, 233–241. doi: 10.1111/j.1469-1795.2010.00433.x
R Core Team (2015). R: A Language and Environment for Statistical Computing. Vienna: R Foundation for Statistical Computing. Available online at: https://www.R-project.org/
Reid, T., Krüger, S., Whitfield, D. P., and Amar, A. (2015). Using spatial analyses of bearded vulture movements in southern Africa to inform wind turbine placement. J. Appl. Ecol. 52, 881–892. doi: 10.1111/1365-2664.12468
Resheff, Y. S., Rotics, S., Harel, R., Spiegel, O., and Nathan, R. (2014). AcceleRater: a web application for supervised learning of behavioral modes from acceleration measurements. Movement Ecol. 2, 27–27. doi: 10.1186/s40462-014-0027-0
Ripple, W. J., Estes, J. A., Beschta, R. L., Wilmers, C. C., Ritchie, E. G., Hebblewhite, M., et al. (2014). Status and ecological effects of the world's largest carnivores. Science 343:1241484. doi: 10.1126/science.1241484
Rotics, S., Kaatz, M., Resheff, Y. S., Feldman, S., Zurell, D., Sapir, N., et al. (2016). The challenges of the first migration: movement and behavior of juvenile versus adult white storks with insights regarding juvenile mortality. J. Anim. Ecol. 85, 938–947. doi: 10.1111/1365-2656.12525
Rotics, S., Turjeman, S., Kaatz, M., Resheff, Y. S., Zurell, D., Sapir, N., et al. (2017). Wintering in Europe instead of Africa enhances juvenile survival in a long-distance migrant. Anim. Behav. 126, 79–88. doi: 10.1016/j.anbehav.2017.01.016
Sala, O. E., Chapin, F. S. III., Armesto, J. J., Berlow, E., Bloomfield, J., Dirzo, R., et al. (2000). Global biodiversity scenarios for the year 2100. Science 287, 1770–1774. doi: 10.1126/science.287.5459.1770
Tanferna, A., López-Jiménez, L., Blas, J., Hiraldo, F., and Sergio, F. (2013). Habitat selection by Black kite breeders and floaters: implications for conservation management of raptor floaters. Biol. Conserv. 160, 1–9. doi: 10.1016/j.biocon.2012.12.031
Tryjanowski, P., Sparks, T. H., and Profus, P. (2009). Severe flooding causes a crash in production of white stork (Ciconia ciconia) chicks across Central and Eastern Europe. Basic Appl. Ecol. 10, 387–392. doi: 10.1016/j.baae.2008.08.002
Tryjanowski, P., Sparks, T., and Jerzak, L. (2006). The White Stork in Poland: Studies in Biology, Ecology and Conservation. Poznań: Bogucki Wydawnictwo Naukowe.
Van den Bossche, W., Berthold, P., Kaatz, M., Nowak, E., and Querner, U. (2002). Eastern European White Stork Populations: Migration Studies and Elaboration of Conservation Measures. Bonn: Bundesamt für Naturschutz (BfN)/German Federal Agency for Nature Conservation.
Vasilakis, D. P., Whitfield, D. P., Schindler, S., Poirazidis, K. S., and Kati, V. (2016). Reconciling endangered species conservation with wind farm development: cinereous vultures (Aegypius monachus) in south-eastern Europe. Biol. Conserv. 196, 10–17. doi: 10.1016/j.biocon.2016.01.014
Walter, W. D., Onorato, D. P., and Fischer, J. W. (2015). Is there a single best estimator? Selection of home range estimators using area-under-the-curve. Movement Ecol. 3: 10. doi: 10.1186/s40462-015-0039-4
Wilson, A. D. M., Wikelski, M., Wilson, R. P., and Cooke, S. J. (2015). Utility of biological sensor tags in animal conservation. Conserv. Biol. 29, 1065–1075. doi: 10.1111/cobi.12486
Keywords: 3D-acceleration sensor, biotelemetry, Ciconia ciconia, home range selection, resource selection
Citation: Zurell D, von Wehrden H, Rotics S, Kaatz M, Groß H, Schlag L, Schäfer M, Sapir N, Turjeman S, Wikelski M, Nathan R and Jeltsch F (2018) Home Range Size and Resource Use of Breeding and Non-breeding White Storks Along a Land Use Gradient. Front. Ecol. Evol. 6:79. doi: 10.3389/fevo.2018.00079
Received: 03 April 2018; Accepted: 23 May 2018;
Published: 12 June 2018.
Edited by:
Pedro J. Leitão, Technische Universitat Braunschweig, GermanyReviewed by:
Marcelo Bertellotti, Centro Para el Estudio de Sistemas Marinos (CONICET), ArgentinaMirko Di Febbraro, University of Molise, Italy
Copyright © 2018 Zurell, von Wehrden, Rotics, Kaatz, Groß, Schlag, Schäfer, Sapir, Turjeman, Wikelski, Nathan and Jeltsch. This is an open-access article distributed under the terms of the Creative Commons Attribution License (CC BY). The use, distribution or reproduction in other forums is permitted, provided the original author(s) and the copyright owner are credited and that the original publication in this journal is cited, in accordance with accepted academic practice. No use, distribution or reproduction is permitted which does not comply with these terms.
*Correspondence: Damaris Zurell, damaris.zurell@hu-berlin.de