Climatic Controls on C4 Grassland Distributions During the Neogene: A Model-Data Comparison
- 1Department of Earth Sciences, University of Minnesota, Minneapolis, MN, United States
- 2Department of Geography, Florida State University, Tallahassee, FL, United States
- 3Department of Animal and Plant Sciences, University of Sheffield, Sheffield, United Kingdom
- 4Department of Biology, University of Washington, Seattle, WA, United States
- 5Bristol Research Initiative for the Dynamic Global Environment, School of Geographical Sciences, University of Bristol, Bristol, United Kingdom
- 6Department of Forest Ecosystems and Society, Oregon State University, Corvallis, OR, United States
Grasslands dominated by taxa using the C4 photosynthetic pathway first developed on several continents during the Neogene and Quaternary, long after C4 photosynthesis first evolved among grasses. The histories of these ecosystems are relatively well-documented in the geological record from stable carbon isotope measurements (of fossil vertebrate herbivores and paleosols) and the plant microfossil record (pollen and/or phytolith assemblages). The distinct biogeography and ecophysiology of modern C3 and C4 grasses have led to hypotheses explaining the origins of C4 grasslands in terms of long-term changes in the Earth system, such as increased aridity and decreasing atmospheric pCO2. However, quantitative proxies for key abiotic drivers of these hypotheses (e.g., temperature, precipitation, pCO2) are still in development, not yet widely applied at the continental or global scale or throughout the late Cenozoic, and/or remain contentious. Testing these hypotheses globally therefore remains difficult. To understand better the potential links between changes in the Earth system and the origin of C4 grasslands, we undertook a global-scale comparison between observational records of C4 plant abundances in Miocene and Pliocene localities compiled from the literature and three increasingly complex models of C4 physiology, dominance, and abundance. The literature compilation comprises >2,600 δ13C-values each of fossil terrestrial vertebrates and of paleosol carbonates, which we interpret as primarily proxies for the abundance of C4 grasses, based on the modern contribution of C4 grasses to terrestrial net primary productivity. We forced the vegetation models with simulated monthly climates from the HadCM3 family of coupled ocean-atmosphere general circulation models (OAGCMs) over a range of pCO2-values for each epoch to model C4 dominance or abundance in grid cells as: (1) months per year exceeding the temperature at which net carbon assimilation is greater for C4 than C3 photosynthesis (crossover temperature model); (2) the number of months per year exceeding the crossover temperature and having sufficient precipitation for growth (≥25 mm/month; Collatz model); and (3) the Sheffield Dynamic Global Vegetation Model (SDGVM), which models multiple plant functional types (PFTs) (C3 and C4 grasses, evergreen, and deciduous trees). Model-data agreement is generally weak, although statistically significant for many comparisons, suggesting that regional to local ecological interactions, continent-specific plant evolutionary histories, and/or regional to local climatic conditions not represented in global scale OAGCMs may have been equally strong or stronger in driving the evolution of C4 grasslands as global changes in the Earth system such as decreases in atmospheric pCO2 and late Cenozoic global cooling and/or aridification.
Introduction
Plants using the C4 photosynthetic pathway comprise only a small fraction of the species diversity of the modern global flora, and about half of these extant C4 species are grasses. Despite the limited number of C4 species, tropical, and sub-tropical ecosystems dominated by C4 grasses account for almost one-quarter of modern terrestrial net primary productivity (Still et al., 2003; Sage, 2004; Sage et al., 2011). Molecular phylogenies indicate that the C4 syndrome evolved multiple times independently in grasses (Kellogg, 1999; GPWGII—Grass Phylogeny Working Group II, 2012), and the calibration of these phylogenies to time using the fossil record suggests that the earliest C4 grasses had evolved by the Oligocene epoch (33–25 Ma), with successive independent origins of C4 anatomy and biochemistry continuing throughout the Neogene Period (25–2.6 Ma; Christin et al., 2008, 2014; Spriggs et al., 2014).
The increasingly comprehensive species sampling of grass phylogenies has dramatically improved our ability to generate testable hypotheses about the timing of trait evolution and the diversification of C4 lineages, but cannot reveal the temporal pattern of increasing biomass and the ecological importance of C4 grasses in local and regional ecosystems. Many grasslands are dominated locally by only a few species in terms of frequency, abundance, and biomass (Smith and Knapp, 2003; Edwards et al., 2010; Griffith et al., 2015), such that species diversification over time does not necessarily predict the ecological success of C4 grasses generally. However, the geological record provides evidence about both when grasslands emerged and when they became dominated by C4 species.
The plant fossil record, particularly phytoliths (microscopic intra- and extracellular silica bodies diagnostic to specific clades of grasses), indicates that grass-dominated habitats first appeared on some continents by the late Oligocene to early Miocene epochs. Complementary evidence based on carbon isotope records from fossil teeth of mammalian herbivores and calcareous paleosols (fossilized soils that preserve soil-derived carbonate) shows that C4-dominated grasslands first appeared on various continents during the late Miocene to early Pleistocene (Jacobs et al., 1999; Tipple and Pagani, 2007; Edwards et al., 2010; Strömberg, 2011; Fox et al., 2012a). However, various terrestrial isotopic records (e.g., Kleinert and Strecker, 2001), as well as marine records of terrigenous materials (e.g., Feakins et al., 2013; Hoetzel et al., 2013), indicate that the temporal pattern of the emergence of C4-dominated grasslands was not a simple, monotonic increase in C4 biomass in some regions. Instead, some records (e.g., the terrigenous signal in marine sediments of northeast African vegetation during the Neogene; Feakins et al., 2013) indicate fluctuations through time in the C3:C4 ratio at regional scales prior to the emergence of modern, C4-dominated grasslands. Thus, the ecological dominance of C4 grasses in many grassland biomes today post-dates the origins of most C4 grass clades by many millions of years and reflects a complex history of ecosystem assembly. Despite the attention of a diverse community of systematists, ecologists, paleontologists, and geochemists over many years, the specific combinations of environmental and climatic changes responsible for the ecological success of C4 grasses over the Neogene and Quaternary both globally and in specific regions have remained elusive and debated (see discussions in, e.g., Edwards et al., 2010; Scheiter et al., 2012; Bond, 2015).
C4 photosynthesis describes a suite of anatomical and biochemical characteristics that together act as a CO2 pump to reduce photorespiration, thereby increasing the quantum yield, a measure of how efficiently plants fix CO2 relative to the amount of absorbed photosynthetically active radiation (APAR) received during light-limited photosynthesis (Ehleringer and Björkman, 1977; Collatz et al., 1998). Photorespiration rates are higher at warmer temperatures for a given atmospheric pCO2, and at lower pCO2 for a given temperature (Ehleringer et al., 1997; Sage, 2004). The dominance of modern C4 grasses in warm to hot and mesic to arid regions (e.g., Teeri and Stowe, 1976; Hattersley, 1983; von Fischer et al., 2008) has led to a long-held assumption that C4 photosynthesis evolved as an adaptation to warm and dry climates. However, ancestral state reconstructions of the climatic origins of C4 lineages have called this assumption into question, by showing that most C4 grass lineages derive from C3 ancestors that already inhabited warm climates (Edwards and Still, 2008; Edwards and Smith, 2010). From these origins in warm climates, C4 photosynthesis enabled diversification into cooler and drier climates (Osborne and Freckleton, 2008; Watcharamongkol et al., 2018).
Some paleoclimate records associated with estimates of C4 biomass, such as compound-specific isotope analyses of terrestrial leaf waxes in Pliocene marine sediments off of West Africa (Kuechler et al., 2018), are consistent with a relationship between aridity and C4 biomass during a time with atmospheric pCO2 ≥300 ppmV (i.e., above pre-industrial values; Foster et al., 2017). However, similar records from Pleistocene marine sediments off of North Africa (e.g., Kuechler et al., 2013) indicate an inverse relationship between regional aridity in northern Africa and C4 abundance against a background of low, but fluctuating glacial-interglacial pCO2 (see also Urban et al., 2015). These contrasting records suggest that the role of aridity in controlling the abundance of C4 plants in regional ecosystems may be strongly modulated by factors other than CO2. These observations are broadly consistent with our understanding of C4 grass biogeography today. For example, independently evolved C4 grass lineages show contrasting relationships with rainfall (Visser et al., 2012, 2014). In addition, very arid areas are rarely dominated by C4 species, whereas particular C4 grass clades may dominate humid regions if the dry season is sufficient to allow frequent fires (Bond, 2015).
Decreasing atmospheric pCO2 during the Cenozoic was initially proposed as an explanation for the appearance of C4-dominated grasslands beginning in the late Miocene (e.g., Cerling et al., 1997; Ehleringer et al., 1997). Subsequent work challenged this assertion, demonstrating that atmospheric pCO2 first decreased to levels that could favor C4 over C3 photosynthesis given prevailing climatic conditions during the Oligocene (Pagani et al., 1999; Beerling and Royer, 2011; Foster et al., 2017), not the late Miocene (although note that some CO2-proxy records indicates a rise and subsequent fall in pCO2 during the middle-late Miocene; e.g., Kürschner et al., 2008; Bolton and Stoll, 2013). The broad coincidence in the timing of declining atmospheric pCO2 and the proposed, earliest evolution of C4 photosynthesis in grasses has therefore pointed to a role for low pCO2 in the evolution of C4 photosynthesis (e.g., Christin et al., 2008; Spriggs et al., 2014). In contrast, the asynchronous appearance of C4-dominated grasslands in different continents from the late Miocene to the early Pleistocene (Fox and Koch, 2003; Edwards et al., 2010) suggests that additional environmental factors, in combinations specific to each region, were of equal or larger importance for increasing the dominance of C4 grasses in many ecosystems. These factors could include both biotic and abiotic influences such as disturbance by herbivores, changes in fire frequency and intensity, differential patterns of recovery by C3 trees and grasses and C4 grasses linked to traits other than photosynthetic pathway (i.e., evolutionary history of different lineages), soil type and characteristics, and region-specific patterns of climate change during the Neogene and Quaternary (e.g., Osborne, 2008; Edwards et al., 2010; Bond, 2015; Griffith et al., 2015; Charles-Dominique et al., 2016). The importance of declining CO2 as a single factor in promoting C4 dominance has also been called into question by the results of a 20 year free-air CO2 enrichment experiment that showed an eventual enhancement in C4 biomass relative to C3 biomass growing under experimentally increased CO2 (Reich et al., 2018). Water cycle feedbacks induced by enhanced CO2 have also been shown experimentally to cause C4 grasses to outperform C3 grasses, with greater water-use efficiency by C4 grasses driven by interacting effects of higher CO2 and warming on both soil moisture and plant photosynthetic responses as the proposed mechanism (Morgan et al., 2011).
Fire in particular is recognized as a critical factor in the abundance of C4 grasses in many modern ecosystems (Bond, 2015), and fire has been proposed as playing an important role in the emergence of C4-dominated grasslands during the late Neogene (Keeley and Rundel, 2005; Scheiter et al., 2012). The importance of fire for C4-dominated grasslands has support from micro-charcoal counts in both Pliocene (Hoetzel et al., 2013) and Holocene (Dupont and Schefuss, 2018) marine sediments from cores off of West Africa, but the terrestrial record of charcoal is still too incomplete (e.g., Scott, 2000) to allow for direct comparisons of charcoal occurrences with either paleobotanical or vertebrate and paleosol carbon isotope proxies documenting the emergence of C4-dominated ecosystems.
A wide range of continental paleoclimate proxies derived from paleontological and geological materials can provide critical information on the abiotic conditions associated with the increase of C4 biomass in local and regional ecosystems. Some of these, such as the oxygen isotope composition of fossil teeth of mammalian herbivores (e.g., Passey et al., 2002) or paleosol carbonates (e.g., Fox et al., 2012b) are controlled by both temperature and precipitation, so cannot generally be deconstructed to provide unambiguous quantitative estimates of paleoclimatic conditions. Other proxies, such as the relative tooth crown heights of mammalian herbivores (Eronen et al., 2010) or weathering indices based on elemental ratios of paleosols (Sheldon and Tabor, 2009; Stinchcomb et al., 2016), can provide quantitative estimates of mean annual temperature and precipitation. However, such proxies, as well as newer methods such as carbonate clumped isotope paleothermometry (Ghosh et al., 2006; Eiler, 2011), either rely on assumptions about faunal trait evolution that have been called into question (e.g., MacFadden et al., 1999; Strömberg, 2006), or have not yet been applied widely enough to allow for compilations at the continental or global scale with sufficient coverage to allow for meaningful analyses of empirical paleoclimate data in relation to records of the Neogene-to-Quaternary rise in global C4 biomass. In contrast, global scale, ocean-atmosphere general circulation models (OAGCMs) coupled with vegetation modeling provide a means to assess the record of C4 biomass on all continents in relation to possible past climatic conditions that ultimately can be tested with paleoclimate proxy data (e.g., see Lunt et al., 2007 for a comparison of late Oligocene and pre-industrial boundary conditions).
In this paper, we undertake a global-scale comparison between a literature compilation of δ13C values of mineralized tissues of fossil herbivorous vertebrates (mammal teeth, ratite eggshell) and of paleosol carbonates from Miocene and Pliocene localities as proxies for the abundance of C4 grasses, and three increasingly complex models of C4 dominance and abundance driven by output from a global OAGCM. Specifically, we use climate output from the HadCM3LBL-M2.1 and HadCM3BL-M1 OAGCMs (Valdes et al., 2017) over a range of pCO2 values for the Miocene (Bradshaw et al., 2012) and Pliocene (Bragg et al., 2012; Conn, 2012) to model C4 dominance or abundance in grid cells as: (1) months per year exceeding the temperature at which net assimilation is greater for C4 than C3 photosynthesis (crossover temperature model; Ehleringer et al., 1997); (2) the number of months per year exceeding the crossover temperature and having sufficient precipitation for grass growth (≥25 mm/month in months that meets the crossover temperature criterion; Collatz model; Collatz et al., 1998), and (3) predictions of various measures of C4 biomass from the Sheffield Dynamic Global Vegetation Model (SDGVM; Woodward and Lomas, 2004), output from which includes a range of vegetation parameters of multiple plant functional types (PFTs), including C3 and C4 grasses. To account for the uncertainty in estimates and temporal variation in CO2 levels suggested by proxy data for this time interval (see Beerling and Royer, 2011), we used a range of pCO2 values in each analysis. Statistical comparisons of the isotopic databases with the paleoclimate and vegetation model outputs allow us to assess data-model agreement. We propose that close similarity between proxy data and a particular model will provide support for the explanatory factors inherent in the model playing an important role in C4 dominance globally (or regionally). A mismatch, on the other hand, will suggest that the factors accounted for in the model are not sufficient for explaining the patterns seen in the data, although alternative reasons are possible. Specifically, we discuss the limitations and opportunities with current data and model resolution for inferring the role of abiotic factors in the evolution of the modern C4 grasslands over the Neogene and Quaternary.
Carbon Isotope Proxies for C4 Biomass
The basis for using the stable carbon isotope composition (δ13C) of carbonate-bearing minerals of vertebrate herbivores and authigenic soil carbonates as proxies for C4 biomass is the difference in isotope discrimination by C3 and C4 plants during fixation of CO2. Due to physiological differences, C3 plants discriminate more strongly against 13CO2 than do C4 plants (O'Leary, 1981), and, consequently, tissues of C3 plants have much lower (more negative) δ13C values than do C4 plants. Here, we treat the δ13C values of modern plants reported by Passey et al. (2002) as end-members, whereby modern C3 plants have mean δ13C (±1 s.d.) of −27.4 ± 1.6% and modern C4 monocots have mean δ13C of −12.7 ± 1.1%. Passey et al. (2002) assumed an average δ13C value for atmospheric CO2 for these plant data of −8.0%, which allowed estimation of apparent fractionation factors during fixation of CO2 by C3 and C4 photosynthesis of −19.6 and −4.7%, respectively.
Authigenic carbonate precipitates in soils in isotopic equilibrium with the CO2 that is dissolved in soil water (Cerling, 1984). For soils with moderate to high respiration rates and at modern atmospheric pCO2, all CO2 below about 30 cm in the profile is derived from the standing plant biomass or respiration of plant-derived components in the soil, and has the carbon isotopic signature of the standing biomass (Cerling et al., 1991). Relative to atmospheric CO2, pedogenic carbonate is enriched in 13C by ca. 14–17% due to faster diffusion of 12CO2 from the soil to the atmosphere and the temperature-dependent fractionation of carbonate precipitation over the range of typical soil temperatures (Cerling et al., 1991). Paleosol carbonates form on timescales of 102-105 years, depending on sedimentation rates and landscape position (Gile et al., 1966; Birkeland, 1999); however, they sample the landscape on very localized spatial scales assuming minimal horizontal advection of CO2 in the subsurface (i.e., by soil water flow). Importantly, vegetation, soil type and properties, and presence or preservation of carbonate can all vary on small spatial scales with landscape position (Zamanian et al., 2016). Thus, the δ13C of paleosol carbonates preserves a time-integrated signature of plant biomass that can be used to estimate the C3:C4 ratio of the local ecosystem on relatively long timescales but small spatial scales.
The δ13C values of vertebrate herbivore tissues record the δ13C of diet with tissue-specific fractionation factors or apparent enrichment factors (in the case of tissues such as enamel for which the biosynthetic reactions are not reversible, DeNiro and Epstein, 1978; Cerling and Harris, 1999; Passey et al., 2005). For structurally bound carbonate in the hydroxyapatite of tooth enamel of large-bodied mammalian herbivores, the apparent enrichment factor relative to diet is +14.1 ± 0.5% (Cerling and Harris, 1999). The isotopic composition of mammalian tooth enamel is generally considered to be resistant to alteration during fossilization (Wang and Cerling, 1994; Koch et al., 1997; Zazzo et al., 2004), so that fossil teeth of herbivorous mammals faithfully record the dietary proportions of C3 and C4 plants during the interval of tooth formation (Kohn and Cerling, 2002). Additionally, Griffith et al. (2017) showed that the δ13C-values of grazer tissue (bison, mammoth) faithfully records the C3:C4 ratio of grasslands across larger spatial scales. The carbonate of ratite eggshell also records the δ13C of the diet of the hen while the egg is mineralizing (Schirnding et al., 1982), and eggshell δ13C values also appear to be resistant to alteration during fossilization (Stern et al., 1994; Miller et al., 2005). Fossil deposits are subject to time averaging, such that data for fossils from individual localities can represent a wide range of ages (Behrensmeyer et al., 2000). However, individual teeth and eggshells only represent a few months to years of mineralization; thus, the temporal resolution of teeth is higher than that for paleosols. On the other hand, the animals did not necessarily live where the fossils accumulated (Behrensmeyer et al., 2000), and mammalian home range generally scales positively with body size (Harestad and Bunnel, 1979). For example, a large-bodied mammal like a moose has a home range of ca. 1,500 ha, but a smaller bodied species such as a peccary has a home range of only ca. 135 ha (data from Harestad and Bunnel, 1979). Teeth of large mammalian herbivores, which have been most commonly used for paleovegetation reconstruction, therefore integrate the landscape C3:C4 ratio over a much larger area (up to 100s of km2) over a shorter timescale than do paleosol carbonates.
Clades other than Poaceae (grasses) also include lineages that have independently evolved C4 photosynthesis and may be ecologically dominant, notably Cyperaceae (sedges) (Sage et al., 2011), and some marine and lacustrine isotopic records of C4 biomass likely reflect local abundance of C4 plants other than grasses (e.g., Schefuß et al., 2011; Ivory and Russell, 2016). However, the herbaceous component of the spatially extensive modern tropical and temperate grasslands and savannas are overwhelmingly dominated by C4 grasses (Still et al., 2003; Lehmann et al., 2011). Relatively few studies have compared carbon isotopic data and plant microfossil assemblages from the same terrestrial sites, but those that have (e.g., McInerney et al., 2011; Strömberg and McInerney, 2011; Cotton et al., 2012; Chen et al., 2015; Smiley et al., 2016) suggest that C4 isotopic signatures in terrestrial sediments are dominated by grasses and not by other clades, such as sedges, that also evolved C4 photosynthesis. Based on this reasoning, we assume that the terrestrial isotopic records analyzed herein primarily reflect the abundance of C4 grasses in Neogene ecosystems.
Carbon Isotope Data Compilations
The dataset of paleosol carbonate δ13C-values was compiled from publications and datasets in DLF's personal collection and from extensive (but potentially not exhaustive) bibliographic searches using Google Scholar and the GeoRef bibliographic database maintained by the American Geosciences Institute accessed via the University of Minnesota Libraries during 2011–2013. Search terms included combinations of paleosol, pedogenic, carbon*, isotop*, soil, Neogene, Quaternary, Miocene, Pliocene, and Pleistocene, where * is a wildcard operator appropriate for each database. To be included, published datasets had to include specific geographic information for measured sections or individual samples and radiometric ages and/or precise chronostratigraphic assignments in the Miocene (25.0–4.9 Ma) and/or Pliocene (4.9–2.6 Ma) epochs. Most papers included tables of individual δ13C values, but for papers in which data were only presented in figures, Data Thief (https://datathief.org/) was used to capture digitally individual data points from figures. Tests of Data Thief on figures with accompanying data tables verified the accuracy of data capture. The paleosol compilation includes data from 44 publications published from 1986 to 2012 (Data Sheet 1), including 1,280 values from 189 Miocene sections and 1,379 values from 101 Pliocene sections in Africa, Eurasia (including southeast Europe), North America, and South America (Figures 1A, 2).
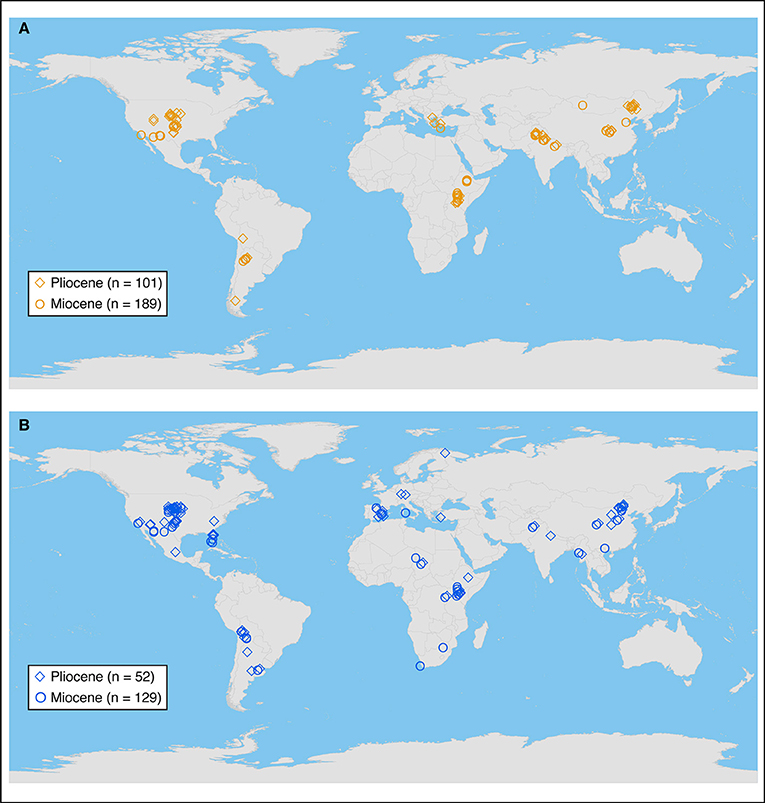
Figure 1. Locations of sample localities for Miocene and Pliocene paleosol carbonates (A) and biominerals (B).
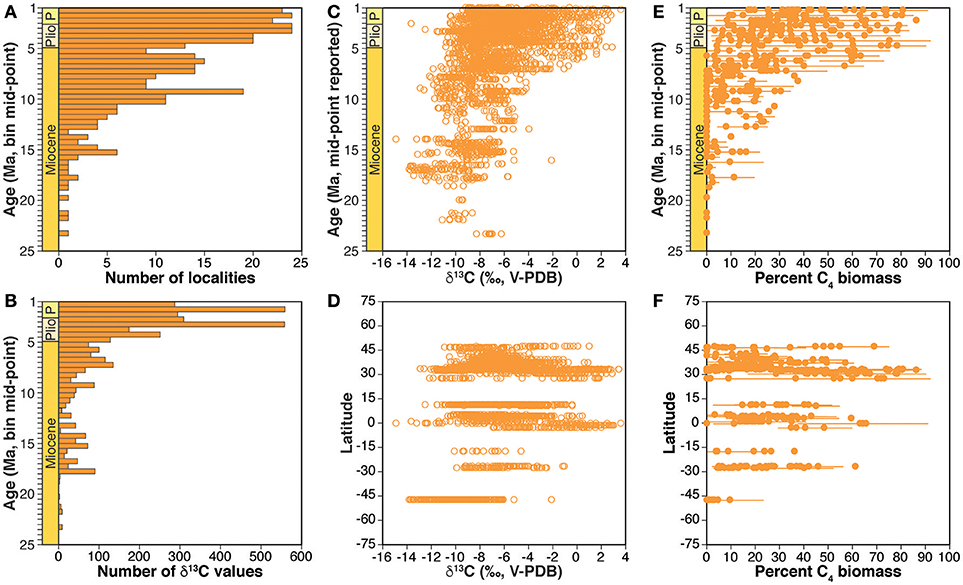
Figure 2. Summary of the compilation of paleosol carbonate δ13C values. Number of localities (A) and δ13C values (B) in each 0.5 Myr bin. All δ13C values by mid-point of 0.5 Myr age bin (C) and by latitude of sample site (D). Mean and standard deviation of estimated percent C4 biomass for each locality by midpoint of 0.5 Myr age bin (E) and by latitude of site (F).
The dataset of fossil mammal tooth and ratite eggshell δ13C values was initially compiled by Ben Passey (now at University of Michigan, Ann Arbor, MI, U.S.A.), and was completed with the addition of data from publications and datasets in DLF's personal collection and from extensive bibliographic searches using Google Scholar and GeoRef. Search terms included combinations of mammal*, fossil, tooth, enamel, apatite, bioapatite, carbon*, isotop*, Neogene, Quaternary, Miocene, Pliocene, and Pleistocene. Inclusion of papers and data acquisition followed the criteria for paleosol carbonates. The biomineral compilation includes data from 57 papers published from 1994 to 2013 (Data Sheet 2), including 1,853 values from 173 Miocene faunal sites and 792 values from 52 Pliocene faunal sites in Africa, Eurasia, North America, and South America (Figures 1B, 3). Paleosol carbonate and biomineral data were also compiled for Pleistocene localities (not exhaustively), and these are summarized in Figures 2, 3 but not analyzed here.
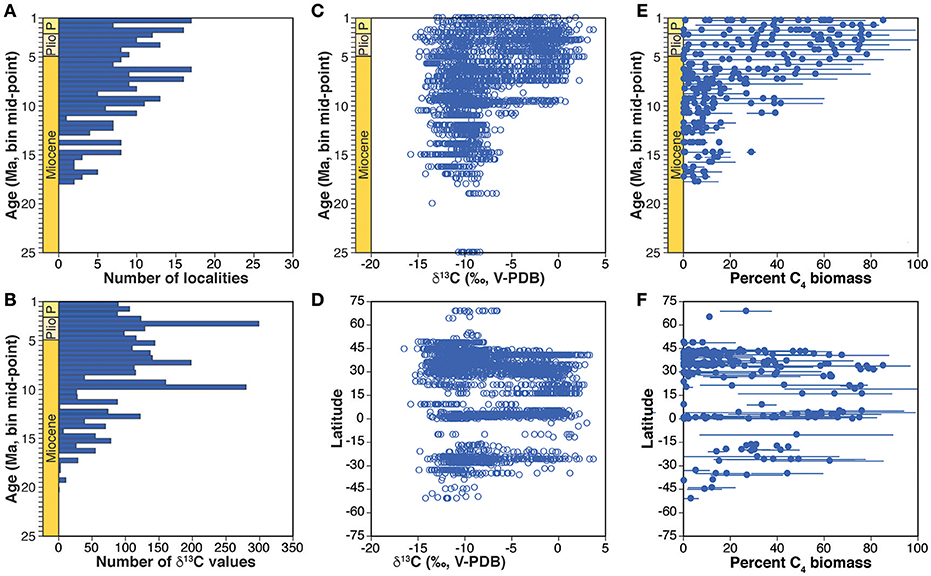
Figure 3. Summary of the compilation of biomineral δ13C values. Number of localities (A) and δ13C values (B) in each 0.5 Myr bin. All δ13C values by mid-point of 0.5 Myr age bin (C) and by latitude of sample site (D). Mean and standard deviation of estimated percent C4 biomass for each locality by midpoint of 0.5 Myr age bin (E) and by latitude of site (F).
To compare published δ13C values to the vegetation model results, we first had to correct the δ13C values for secular change in the δ13C of atmospheric CO2 over the Miocene and Pliocene (Passey et al., 2009; Tipple et al., 2010), which causes the end-member C3- and C4-values to vary through time. To do this, we binned the data in both compilations into 0.5 Myr intervals (approximating the Miocene-Pliocene boundary as 5.0 Ma and the end of the Pliocene as 2.5 Ma) and used the estimates of the δ13C of atmospheric CO2 (based on the δ13C of benthic foraminifera) in those 0.5 Myr bins to correct the measured δ13C values to an equivalent value assuming a “modern” δ13C value of −8.0% (following Passey et al., 2002, 2009). The modern mean values for C3 and C4 plants, the apparent enrichment factors for each photosynthetic pathway, and the uncertainties for the mean values and enrichment factors served as inputs to the linear mixing model IsoError 1.04 (Phillips and Gregg, 2001), which were used to calculate the mean percentage C3 and C4 biomass for each corrected δ13C value, as well as the standard deviation for each mean value and the 95% confidence interval for each estimate. The enrichment in 13C by C3 photosynthesis during fixation of CO2 decreases with increasing irradiance and water deficit (Cernusak et al., 2013), reducing the spacing between C3 and C4 end-members, but given the temporal and geographic scope of the analyses here and for the sake of simplicity, we did not consider the potential influence of light availability and water deficits on δ13C value of C3 plants in our interpretations of the carbon isotopic data.
Paleoclimate Simulations
We forced the vegetation models with simulated monthly climates from the HadCM3 family of coupled OAGCMs (Valdes et al., 2017) for Miocene (HADCM3BL-M2.1; Bradshaw et al., 2012) and Pliocene (HADCM3B-M1; Bragg et al., 2012; Conn, 2012, unpublished Master's thesis) paleogeography. Details of these models are given in Valdes et al. (2017). Grid cells are 2.5° latitude by 3.75° longitude and outputs taken from the OAGCM for each grid cell include monthly temperature, precipitation, and humidity. We used two Miocene simulations: a low pCO2 case (280 ppmV), which is close to the best fit for proxy data during the late Miocene (Foster et al., 2017) and a high pCO2 case (401 ppmV), which could represent conditions just after the Miocene climatic optimum (ca. 16.5–15 Ma) (e.g., Kürschner et al., 2008). We used three Pliocene simulations: a low pCO2 case (280 ppmV), a moderate pCO2 case (405 ppmV, considered here equivalent to the Miocene high pCO2 case), and a high pCO2 case (560 ppmV) that represents high pCO2 forcing of the Mid Pliocene Warm Period (ca. 3.3–3.0 Ma) (e.g., Haywood et al., 2016). The SDGVM averages the climates for neighboring grid cells and restricts humidity to the range 30–95%, and for consistency these averaged climates were used for all three approaches.
Vegetation Models
We used the simulated climates to predict dominance or abundance of C4 grasses in each grid cell employing three increasingly complex vegetation models. The simplest model is the crossover temperature model. A hallmark of C4 plants is their dominance in high-light and high-temperature environments such as grasslands and savannas (Long, 1999; Sage et al., 1999). The dominant process-based explanation for environmental controls on C3 and C4 grass distributions is the crossover temperature hypothesis, which is based on the different quantum yields of grass species using each pathway (Ehleringer, 1978; Ehleringer et al., 1997). The quantum yield describes how much CO2 is absorbed by a leaf compared to the amount of APAR it receives during light limited photosynthesis (Ehleringer and Björkman, 1977; Ehleringer, 1978; Collatz et al., 1998). The quantum yield in C3 plants decreases with increasing leaf temperature, and increases with CO2 at a given leaf temperature, essentially reflecting the influence of these factors on photorespiration (Ehleringer and Björkman, 1977; Pearcy and Ehleringer, 1983; Collatz et al., 1998; Sage, 2004). By comparison, the quantum yield of C4 plants is relatively constant across a range of temperatures and CO2 levels due to the C4 carbon-concentrating mechanism. The point at which the quantum yield of C3 grasses equals the quantum yield of C4 grasses was defined as the “crossover temperature” (Ehleringer et al., 1997; Collatz et al., 1998). At temperatures below this cutoff, C3 grasses should have a higher capacity to fix carbon, while C4 grasses should have higher capacities at temperatures above this cutoff. The crossover temperature model simplifies physiological differences between C3 and C4 plants but has nevertheless been fairly successful in predicting large-scale distributions (Collatz et al., 1998; Still et al., 2003; Griffith et al., 2015).
For each of the five paleoclimate simulations, we estimated crossover temperatures based on the assumed values of atmospheric pCO2. Representative photosynthetic fluxes were predicted using the coupled C3 and C4 leaf photosynthesis and stomatal conductance models of Collatz et al. (1991, 1992). Parameter values, such as maximum carboxylation rates (Vmax) and temperature response functions, were taken from Sellers et al. (1996). Vmax for C3 grasses was assumed to be 90 μmol m−2 s−1 at 298 K, and 30 μmol m−2 s−1 for C4 grasses at 298 K. These models, which are described in detail elsewhere, estimate gross photosynthetic rates as a function of temperature, relative humidity, insolation, and the partial pressures of atmospheric CO2 and O2. Crossover temperature values were estimated for light-limited conditions and represent the leaf temperature where C3 and C4 photosynthetic rates were equal. Crossover temperatures calculated for the 280, 401/405, and 560 ppmV cases are 14, 20, and 24°C, respectively.
For each grid cell in each paleoclimate simulation, the crossover temperature model as used here is simply the number of months for which the temperature is equal to or greater than the calculated crossover temperature (based on the assumed pCO2 in each OAGCM simulation), which is an estimate of the number of months that production of C4 grasses should be favored, all else being equal. Our use of the crossover temperature concept is intentionally simplistic as a first step in estimating the dominance or abundance of C4 grasses.
Our second vegetation model, the Collatz model (Collatz et al., 1998), is slightly more complex, and includes a more reasonable modern climate threshold for C4 grass dominance that accounts for both crossover temperature (22°C for contemporaneous pCO2) and the moisture necessary for plant growth (≥25 mm of precipitation in a month that meets the crossover temperature criterion). Thus, for each grid cell in each paleoclimate simulation, the Collatz model determines the number of months for which the modeled temperature is equal to or greater than the calculated crossover temperature and for which precipitation is also equal to or >25 mm. The inclusion of a precipitation screen effectively limits the months considered to the growing season.
Finally, we used the Sheffield Dynamic Global Vegetation Model (SDGVM; Woodward and Lomas, 2004) to estimate the proportion of C4 biomass in each OAGCM grid cell. Inputs for the SDGVM are monthly temperature, precipitation, and humidity (taken from each OAGCM simulation) and soil texture and dynamics. The SDGVM, which has been used extensively to model both modern and past vegetation (e.g., Beerling and Woodward, 2001; Bond et al., 2005; Scheiter et al., 2012), simulates photosynthesis, respiration, and transpiration for six natural PFTs, and soil carbon and nitrogen dynamics. PFTs include broadleaf deciduous trees, needleleaf deciduous trees, broadleaf evergreen trees, needleleaf evergreen trees, C3 grasses, and C4 grasses. Annual C3 and C4 grasses are the primary successional PFTs. If the climate permits, the four woody PFTs may acquire land cover at the expense of grasses over decadal and longer timescales, but trees are killed by several processes, including fire. At the beginning of each model year, the probability of fire determines the fraction of land which will be available for grass encroachment at any gridpoint. The SDGVM then assigns cover to C3 and C4 grasses based on their relative NPP, without explicit reference to a crossover temperature (Nemani and Running, 1996). With regard to this study, limitations of the SDGVM include the lack of savanna-specific PFTs and a detailed treatment of fire and herbivory. Although NPP and leaf area index are calculated daily and outputted monthly in the model, biomass is updated only at the end of the year, restricting our ability to examine seasonal root and stem growth. Here, we use average annual leaf area index for each PFT, which scales with biomass, to estimate C4 grass biomass as a percentage of total simulated plant biomass in each grid cell. This third vegetation model represents a considerable increase in complexity relative to the crossover temperature and Collatz models as used here.
To compare the δ13C data to the model estimates of C4 dominance or abundance, we used statistical summaries of all Miocene and Pliocene δ13C values for fossil biominerals and for paleosol carbonates separately in each grid cell. Given the global distribution of Miocene and Pliocene continental rock units and associated terrestrial vertebrate faunas, most continental grid cells in the model domains did not have carbon isotope records from Miocene or Pliocene sites, so by necessity only a subset of model grid cells are analyzed in each time interval. When aggregated at the geographic scale of grid cells, the mean and median δ13C values are virtually indistinguishable and yield similar results, so below we present only results for mean values. Because the observed and modeled results are not necessarily normally distributed, we used the non-parametric Kendall rank correlation coefficient (Kendall's tau, τ) to test for statistically significant correlations between % C4 biomass based on mean δ13C values in grid cells and the modeled estimates of C4 dominance (number of months that C4 is favored) and biomass (SDGVM output). Kendall's τ ranges from +1 for perfect positive correlation of rank orders of the data to −1 for perfect inverse correlation of ranks, and a τ of 0 indicates complete lack of correlation of ranks. Kendall's τ was calculated using the Stats package in R (R Development Core Team, 2013) and in JMP Pro 13.
Results
Comparison of the percentage of C4 biomass estimated from paleosol carbonate δ13C values with the number of months favoring C4 photosynthesis based on the crossover temperature model is presented in Figure 4. Kendall's rank correlation coefficients (τ) for the two Miocene models are positive and low, but the correlation for the 401 ppmV case is statistically significant (p = 0.016). Kendall's τ for the Pliocene models are all statistically significant, and the correlations are stronger than for the Miocene models, but they are all negative, indicating that grid cells with more months above the modeled crossover temperature have generally lower δ13C values for paleosol carbonates, suggesting less C4 biomass. Comparison of the results for the biomineral data with the crossover temperature model results are presented in Figure 5. Kendall's τ values are positive for all models, and most are statistically significant. The rank correlations are generally stronger than for the paleosol carbonate results, with τ values up to 0.395.
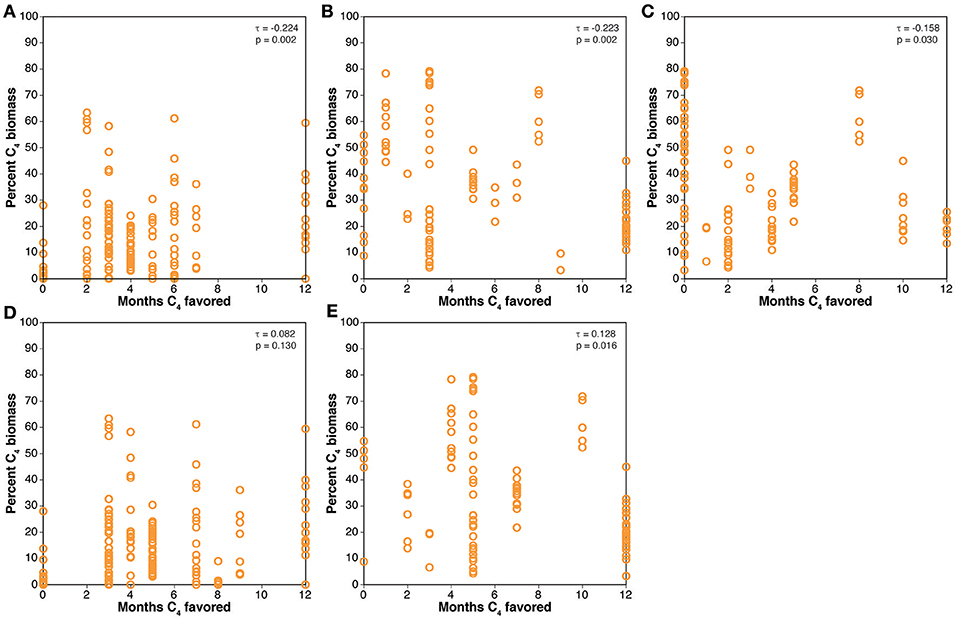
Figure 4. Comparisons of results of the crossover temperature model to mean estimated percent C4 biomass from the paleosol carbonate compilation. (A) Pliocene, 280 ppmV CO2. (B) Pliocene, 405 ppvM CO2. (C) Pliocene, 560 ppmV CO2. (D) Miocene, 280 ppmv CO2. (E) Miocene, 401 ppmV CO2.
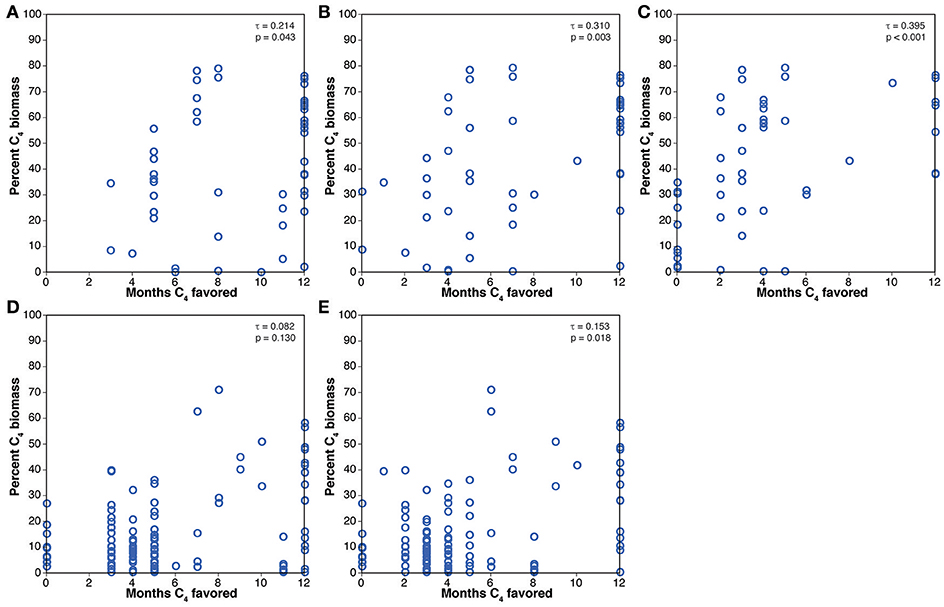
Figure 5. Comparisons of results of the crossover temperature model to mean estimated percent C4 biomass from the biomineral compilation. (A) Pliocene, 280 ppmV CO2. (B) Pliocene, 405 ppvM CO2. (C) Pliocene, 560 ppmV CO2. (D) Miocene, 280 ppmv CO2. (E) Miocene, 401 ppmV CO2.
Comparison of the paleosol carbonate data with the Collatz model are presented in Figure 6. The results are similar to those for the crossover temperature model. The Miocene cases have positive τ values that are statistically significant, but the Pliocene cases have statistically significant, negative correlations between percentage of C4 biomass estimated from the paleosol carbonate δ13C values and the number of months that favor C4 photosynthesis. Comparison of the results for the biomineral data with the Collatz model results are presented in Figure 7 and are generally similar to the comparison of the biomineral data with the crossover temperature model (Figure 5): all τ values are positive and several are statistically significant, but the rank correlations are generally weak with most having τ values of 0.062 to 0.200.
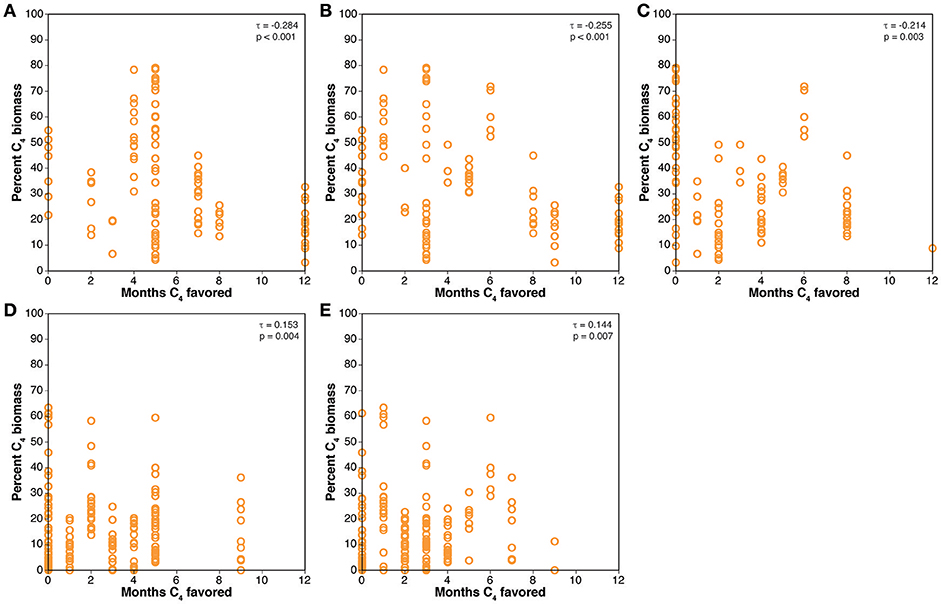
Figure 6. Comparisons of results of the Collatz model to mean estimated percent C4 biomass from the paleosol carbonate compilation. (A) Pliocene, 280 ppmV CO2. (B) Pliocene, 405 ppvM CO2. (C) Pliocene, 560 ppmV CO2. (D) Miocene, 280 ppmv CO2. (E) Miocene, 401 ppmV CO2.
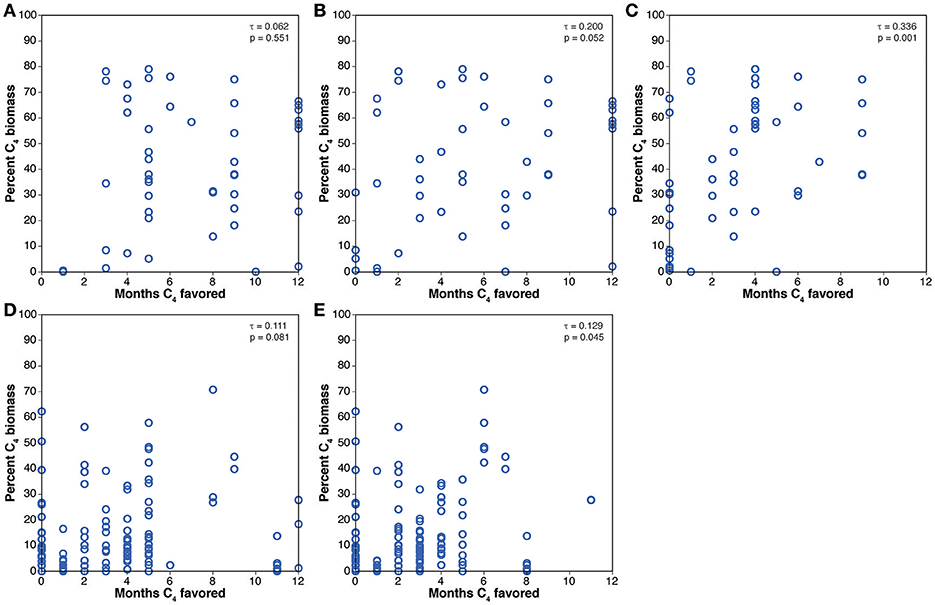
Figure 7. Comparisons of results of the Collatz model to mean estimated percent C4 biomass from the biomineral compilation. (A) Pliocene, 280 ppmV CO2. (B) Pliocene, 405 ppvM CO2. (C) Pliocene, 560 ppmV CO2. (D) Miocene, 280 ppmv CO2. (E) Miocene, 401 ppmV CO2.
Comparisons of the paleosol carbonate data with the SDGVM output for the two Miocene and three Pliocene cases are presented in Tables 1, 2, respectively. For both Miocene cases, all correlation coefficients are positive and many are statistically significant, although generally the correlations are weak (τ ranges from 0.052 to 0.322 for the 280 ppmV case and from 0.053 to 0.264 for the 401 ppmV case). For both sets of results, the strongest correlations are for the percentage of total annual biomass that is C4 grasses (highest τ for both models) and percent C4 cover. For the Pliocene cases, many correlations are statistically significant, but negative, and the correlations are generally weak.
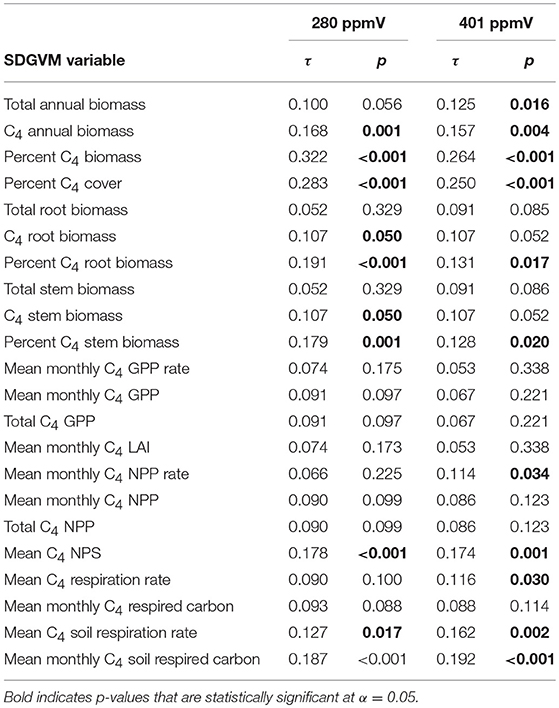
Table 1. Kendall's rank correlation coefficients (τ) and p-values for comparisons of estimates of mean percent C4 biomass from δ13C-values of Miocene paleosol carbonate with results of two cases of the SDGVM.
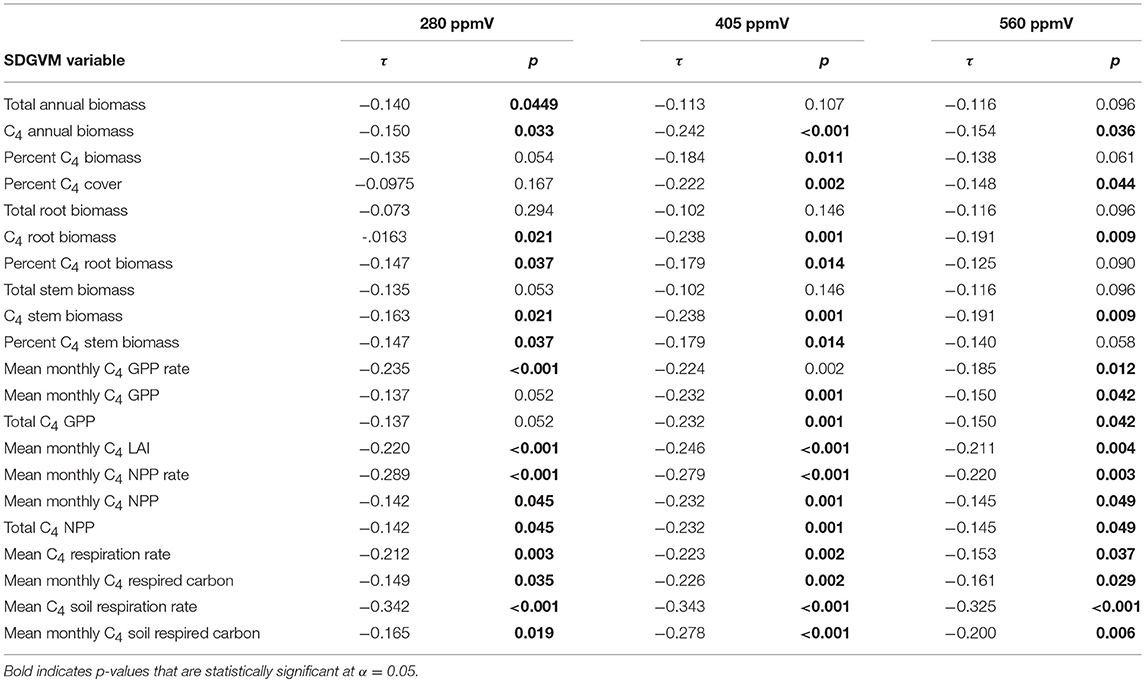
Table 2. Kendall's rank correlation coefficients (τ) and p-values for comparisons of estimates of mean percent C4 biomass from δ13C-values of Pliocene paleosol carbonate with results of three cases of the SDGVM.
Comparisons of the biomineral data with the SDGVM output for the two Miocene and three Pliocene cases are presented in Tables 3, 4, respectively. For both Miocene cases, all correlations are positive and many are statistically significant, particularly for the 401 ppmV case. The correlations are generally weak, as for the paleosol carbonate data, and the maximum correlation coefficients are slightly lower than for the Miocene paleosol carbonates (τ of ca. 0.22–0.27 for percentage C4 grass biomass and cover). The rank correlations of the biomineral estimates of C4 biomass for the three Pliocene cases are the strongest of any of our comparisons, and most are statistically significant. The correlations are negative for three of the SDGVM output parameters for the 280 ppmV case, and otherwise all correlations are positive. The strongest rank correlations are moderately strong (τ ca. 0.4 for all three cases for percentage C4 grass biomass and cover), particularly compared to most of the other results. It is also noteworthy that these significant, positive τ values are only for C4-specific model outputs, whereas summary values for all PFTs such as total annual biomass do not show significant positive correlations with proxy data.
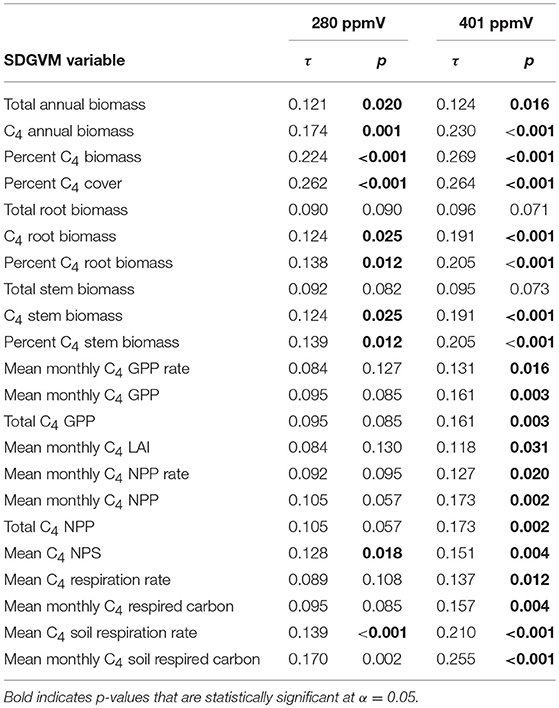
Table 3. Kendall's rank correlation coefficients (τ) and p-values for comparisons of estimates of mean percent C4 biomass from δ13C-values of Miocene biominerals with results of two cases of the SDGVM.
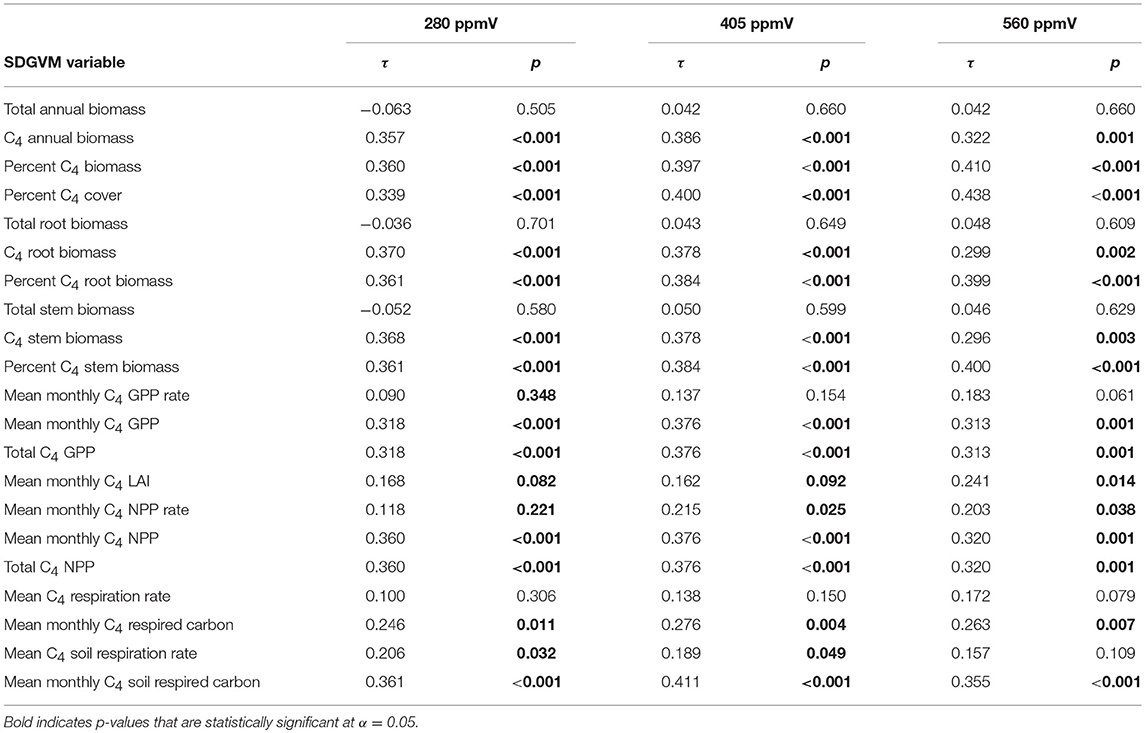
Table 4. Kendall's rank correlation coefficients (τ) and p-values for comparisons of estimates of mean percent C4 biomass from δ13C-values of Pliocene biominerals with results of three cases of the SDGVM.
Discussion
Our analyses indicate that none of the models have strong explanatory power for the paleo-proxy data at a global scale, with rank correlations reaching a maximum of ca. 0.4. Thus, the degree of process model complexity does not appear to matter substantially for better hindcasting of C4 grass dominance, at least not when the data from different regions are analyzed together. In other words, it is not clear which of temperature, length of growing season, and ecological interactions (as variously simulated in the three models) mattered most for Neogene C4 grass distribution and which other factors may have been equally or more important.
Nevertheless, some general conclusions can be drawn. First, many of the comparisons for which ranks are positively correlated are indeed statistically significant, and these results necessarily imply non-random associations between the measures of C4 dominance and abundance and the predictions based on the three models. In other words, they provide evidence that climate, as the driver of our vegetation models, played a role in the distribution and abundance of C4 plants (primarily grasses) during the Neogene. However, most of the positive correlations, including those that are statistically significant, are weak to moderate (all τ-values are <0.44 and most for the Miocene are <0.20). These results indicate that factors other than climate and atmospheric pCO2 were as or more important controls on the dominance and abundance of C4 plants during the Neogene, at least to the extent that our various models capture climate and atmospheric CO2 impacts on vegetation physiology and dynamics. That the controls on the distribution of C4 plants are multifaceted is well-understood. However, climate and atmospheric pCO2 are the two parameters for which we have the most reliable methods of quantitative estimation in the geological record. Other factors, such as fire frequency and intensity and herbivore disturbance, cannot yet be estimated quantitatively in meaningful ways for most terrestrial sites. Thus, our results highlight the current limits on our ability to make sense of the isotopic proxy record of C4 abundance in the past using our understanding of modern processes.
Recent studies in modern ecosystems have revealed a broad, but imperfect fit between modeled vegetation output and (proxy) vegetation data in terms of C4 biomass and dominance (e.g., Ehleringer et al., 1997; Collatz et al., 1998; Cramer et al., 2001; Sitch et al., 2003; Still et al., 2003; Lehmann et al., 2011; Ardö, 2015). Although climate undoubtedly plays a major role in driving the balance between (C4) grasses and trees in low- to mid-latitude ecosystems (e.g., Sankaran et al., 2005; Hirota et al., 2011; Staver et al., 2011), differential responses to climate in the distribution of savannas across continents point to the role of both disturbance (fire, herbivory) and ecosystem history (e.g., biogeography, soil type) in shaping vegetation dynamics (e.g., Bond, 2008; Lehmann et al., 2011). In light of these at least generally consistent results, the agreement between the carbon isotope data compilations and the vegetation model estimates of C4 dominance and abundance is poor despite the frequency of positive and statistically significant results. For many of the cases, all three models predict strong dominance by, or high abundance of, C4 grasses in many grid cells for which the isotopic data suggest only modest C4 biomass. Additionally, in many cases the models predict essentially no C4 biomass for many grid cells, for which estimates based on isotopic data range from 0% to almost 80% mean C4 biomass. We used the average end member δ13C values for C3 and C4 plants to estimate the percentage of C4 biomass, which could lead to overestimates of C4 biomass from sites which experienced high irradiance and/or water deficits. Those conditions lead to a net decrease in carbon isotope fractionation during fixation of CO2 by C3 plants (Cernusak et al., 2013), which decreases the isotopic offset between C3 and C4 plants. However, even if we adjusted some or all values to account for this possible effect, the decrease in the abundance of C4 biomass would be no >20% and many grid cells modeled as having no C4 grasses would still have mean observed δ13C values consistent with 50–60% C4 biomass.
Another important observation from our study is that the results for the biomineral data (predominantly from fossil mammalian herbivore tooth enamel) are stronger than for the paleosol data in terms of both frequency of statistical significance, and the strength and direction of the rank correlations. For example, many of the results for all three vegetation models yield negative correlations with the estimates of C4 biomass from the paleosol carbon isotope data, and many of these cases are statistically significant, which is the opposite of expectations. Most striking is the uniformity of negative correlations for all of the comparisons with the Pliocene paleosol carbonate data, including for the most sophisticated of our vegetation models, the SDGVM. One explanation for the stronger correlations with biomineral isotopic data is the different biases inherent in the two types of proxy data we analyzed. The isotopic signal in vertebrate herbivores integrates vegetation growing across the landscape, whereas paleosols are essentially time-integrated point samples (Griffith et al., 2017). It may also be that consumption of aboveground biomass by herbivores better mirrors the variation in total C4 biomass than paleosol carbonates, the carbon for which derives mostly from soil- and root-respired CO2, thus more closely reflecting underground biomass, as in modern soils (Angelo and Pau, 2016). However, the fact that only the Pliocene data show contrasting correlations for the different types of isotopic data suggests that broad formational factors are not sufficient as an explanation.
Sources of Uncertainty in Model-Proxy Data Comparisons
Despite these weak and partly contradictory results, we see no reason to question the utility of carbon isotopic data for inferring the abundance of C4 plants in soil biomass and aspects of the diet of primary consumers (see discussion in Cerling and Quade, 1993; Kohn and Cerling, 2002; Cerling et al., 2010). However, interpreting either type of isotopic data in terms of landscape scale patterns of C4 abundance is perhaps not as straightforward as is often assumed. As discussed above, isotopic data from paleosol carbonates are likely to be much less spatially averaged (and more temporally averaged) than isotopic data from vertebrate teeth. Similarly, isotopic data from herbivore teeth suffer from a number of unique biases. For example, carbon isotope data for consumers for a single locality are subject to many filters that blur or obscure how the distribution of diets in terms of C4 consumption by individuals and averages for species relate to the abundance of C4 grasses locally or regionally. First, herbivores may not sample the vegetation evenly or completely, so that even if the fauna could be analyzed in its entirety, it would not provide an unbiased isotopic picture. Second, taphonomic factors can alter the taxonomic composition of faunas, both in terms of presence or absence of species in the fossil record and also relative abundance and evenness of species relative to the original living faunal community (Behrensmeyer et al., 2000). Sampling of fossil taxa rarely accounts for the relative abundance of species in the fossil assemblage, which could be an important consideration for interpreting distributions of δ13C values or species or faunal mean values in relation to the inferred nature of the ancient habitat. Third, in modern ecosystems in which essentially all grasses are C4 and all shrubs and trees are C3, such as much of low elevation east African savannas today, using δ13C values to classify consumers into distinct dietary categories (C4 grazer, mixed feeder, C3 browser, closed canopy C3 browser) and then to classify faunas into proportions of species in each category, as Cerling et al. (2015) did, is a possible way to convert consumer isotopic data into quantitative models of biome type. However, in regions that have ecologically meaningful abundance of C3 grasses, this approach may not work as well because some grazers will have a C3 signal. These filters and biases mean that, for application to fossil faunas, careful consideration of feeding ecology, taphonomy, and sampling are necessary. Nevertheless, the isotopic proxies for C4 are reliable in principle, if somewhat more nuanced than they are commonly treated, so we do not think that the isotopic data themselves are necessarily problematic.
Similarly, we do not question the basic concepts of the crossover temperature or the modern climatic threshold proposed by Collatz et al. (1998) as predictors of the physiological advantage of C4 plants under the right environmental conditions. The fact that neither our simple crossover temperature model nor the only slightly more complex Collatz model performs well suggests that pCO2, temperature, and moisture availability alone are not adequate to explain the history of C4 grasses, despite the fact that the Collatz model does reasonably well at predicting the modern distribution of C3, C4, and mixed grasslands today (Collatz et al., 1998). Other local to regional biotic and abiotic factors on each continent must also have been important—and continue to be important—as has been suggested before (Fox and Koch, 2003; Edwards et al., 2010; Lehmann et al., 2014). Even the SDGVM results are not particularly compelling, despite the attempt in this model to account for a broader range of environmental variables and a more nuanced set of plant life strategies, and a demonstrated ability to reasonably recreate modern vegetation patterns (Cramer et al., 2001; Woodward et al., 2001).
Instead, we argue that an important reason for the relatively weak correlations between model output and proxy-data could be the difference in temporal and spatial scale and resolution between the paleosol and consumer records on the one hand and the OAGCM grid cells on the other. Individual paleosol samples are essentially point samples and at the scale of the OAGCM grid cells even multiple stratigraphic series of samples from a field area are still essentially point samples. The few cases of extensive lateral sampling at the outcrop and field area scale have shown considerable heterogeneity in the abundance of C4 grasses on length scales of 10s to 1,000s of meters that reflect the influence of landscape processes, even in the context of regional transitions from C3 to C4 dominated ecosystems (e.g., Behrensmeyer et al., 2007 for the classic Siwaliks record of Pakistan). Landscape position, local topography, and edaphic factors such as grain size and clay content are critical controls on soil drainage and the potential for carbonate precipitation in addition to climate (Birkeland, 1999), so the paleosol record may not be representative of the average conditions across entire OAGCM grid cells. Vertebrate consumers collectively sample the landscape much more broadly than do soils, but only some of the largest species might have had individual home ranges that approach the scale of the model grid cells and we presume that relatively few species had long distance migration routes, as is the case for most modern mammals (Harris et al., 2009). In addition, as mentioned above, even these large herbivores are unlikely to have sampled the landscape in a perfectly representative way. Given that the consumer data reflect a mix of the feeding preferences of individuals and the typical behavior of populations and species, it is therefore possible that the biomineral dataset also does not completely reflect average conditions at the scale of every OAGCM cell (although our strongest results are for the Pliocene biomineral data in relation to the SDGVM output). Thus, the proxies may be sampling small parts of the area of a grid cell relatively accurately, but those may not be representative of the entire area of the grid cell, while the SDGVM results may also be relatively accurately predicting average conditions, but at the scale of the grid cell. Spatial averaging of non-linear physiological responses to temperature and CO2 (“Jensen's inequality”; Denny, 2017), would result in over- or under-estimation of C4 dominance and abundance in the large grid cells of the SDGVM.
The temporal scales of our comparisons may also not be commensurate. The isotopic datasets both span millions of years and are unevenly distributed in time and space. Moreover, in some areas (e.g., south Asia), the isotopic record in either the Miocene or the Pliocene or both has known patterns of temporal variability. The OAGCM outputs for the late Miocene and the Pliocene are single time slices that necessarily represent climate over millions of years for each interval and use geographic boundary conditions in the Miocene and Pliocene with their own uncertainties. Furthermore, global and, more importantly, regional climates were likely more dynamic during both periods in ways that could have been important for the evolution of C4 grasslands. For the Pliocene, the multiple models at different pCO2 may capture some aspects of the global scale dynamic of the transition into and out of the Mid Pliocene Warm Period (see Haywood et al., 2016). Also, given the relative brevity of the Pliocene compared to the Miocene, the geographic boundary conditions during the Pliocene may not have changed enough to matter. However, that is probably not the case for the Miocene given the histories of active tectonism in western North America, east Africa, and south Asia during this time, and the known influence of changes in orography on climate. These considerations highlight the fact that the paleoclimate simulations that underlie our vegetation models may be inaccurate in specific grid cells or even over regions in ways that contribute to the relatively low correlations between data and models that we observe.
Similarly, despite the comparative sophistication of the SDGVM, various factors may complicate its ability to model ancient ecosystems in a ways that limit the comparisons we have made here, particularly with regard to how grass-dominated ecosystems are modeled. The SDGVM does not have savanna-specific FTs such as fire-adapted, shade-intolerant trees, or a distinct shrub FT. The treatment of fire in this implementation of SDGVM is likely another important factor, given the prominence of fire as a control on modern grass-dominated ecosystems (e.g., Bond et al., 2005). Fire probability is calculated at the beginning of the year using climate envelopes and fuel loads depend on biomass, which is updated annually, so the model is relatively insensitive to vegetation dynamics; in addition, fire intensity is not modeled. The PFTs do not differ in flammability and have no fire-specific adaptations such as thick bark. The treatment of fire may be a reason in some cases for the SDGVM under-predicting C4 biomass in areas that the isotopic data indicate abundant C4 biomass. The model does not include herbivory, which like fire is a vital factor in maintaining grassy biomes, and has been implicated in the origins of savannas in Africa (Charles-Dominique et al., 2016). In the implementation we used, climate envelopes directly control tree distribution but only indirectly control grasses via their net primary production. The SDGVM does not have competition between the PFTs, except for crude competition for water resources, but without hydraulic redistribution. These points are not intended as criticisms of the SDGVM, since all models make simplifications. However, these factors may limit how well the SDGVM can model the types of biomes that were dominated by C4 grasses today and in the past. This is particularly true if a non-analog combination of factors (biotic and abiotic) promoted the spread of C4-dominated vegetation during the Neogene.
Finally, we note that all three vegetation models are underlain by the simulated climates, which have uncertainties that may be equally high or even higher than those of the SDGVM. Indeed, the climate simulated can be highly dependent on the geographical boundary conditions assumed (e.g., Zhang et al., 2012; Brierley and Fedorov, 2016; Ahlström et al., 2017). Validation of the climates simulated by the OAGCM are complicated by the same limitations of the paleoclimate proxy record that preclude quantitative analysis of the abiotic factors that controlled the history of C4 grasslands globally over the Neogene. However, numerous model-proxy data comparisons for the Cenozoic attest to the inability of OAGCMs to reproduce climatic events in the past (e.g., Herold et al., 2010; Lunt et al., 2012), with the Paleocene-Eocene Thermal Maximum as a particularly well-studied example (McInerney and Wing, 2011). These model-data mismatches indicate fundamental gaps in our understanding of Earth's climate system that have to be filled before more meaningful comparisons can be made between simulations of past climates and proxy data for past vegetation.
Conclusions
In the end, given all of the possible complications in our data-model comparisons, as well as the complexity of the regional and local processes involved in the evolution of C4 grasslands over the Neogene (and Quaternary), perhaps the statistically significant comparisons with rank correlation coefficients in the 0.2–0.4 range should actually be viewed as good agreement with the models, particularly the SDGVM. The models are intentional simplifications and abstractions of complex interaction properties and processes, and the historical patterns in the carbon isotopic (and other paleontological and geological) records are complicated in both space and time. Our results are consistent with prior findings that global changes in pCO2 and global to regional changes in temperature and precipitation alone are not sufficient to explain the histories of C4-dominated ecosystems since the beginning of the Neogene. Our results also highlight the need for means to estimate in the geological record other factors that are known from modern studies to be important controls on C4 abundance, such as fire intensity and herbivore disturbance. Fire and herbivory should lead to model under-predictions of C4 abundance, whereas relying only on climate and CO2 might lead to over-predictions of C4 abundance. Since abundance of C4 grasses depends on disturbance factors that limit tree growth, then model omission or over simplistic representation of herbivory and fire can lead to an underestimation of C4 grass dominance. Similarly, if C4 grass dominance is simulated solely on the basis of physiological differences with C3 plants (the crossover and Collatz models) without considering the ecological interactions between grasses and trees, then models will tend to overestimate C4 grass abundance. Those aspects of the SDGVM that may complicate its ability to predict grassy biomes suggest that additional model complexity may also be necessary, and regional scale models with higher spatial resolution for multiple time steps through the Neogene and Quaternary are needed to address the scale issues we identified here. However, increasing model complexity and resolution may not yield better model-data comparisons if local ecological interactions and evolutionary history have equally strong effects as abiotic conditions, so that the physiological responses to environment are only one factor among several. Either way, our results should add caution to the reliance on current models for predicting the future of C4 grasslands, and point to the importance of considering scale when comparing different sources of both ecological and paleoecological data.
Author Contributions
DF, SP, CS, CO, and CJS designed the project. DF compiled the isotopic database, performed the statistical analyses, and wrote the initial draft of the paper. CS calculated the crossover temperatures and Collatz model values. CB and SC performed the climate simulations. LT and DB performed the SDGVM simulations. DF, CS, SP, CO, LT, and CS edited and revised the paper.
Funding
A grant from the National Evolutionary Synthesis Center (NESCent; funded by NSF EF0905606) to CO, CAES, and CJS funded the Origins of C4 grasslands: a new synthesis of phylogeny, ecology and paleobiology working group that led to this work.
Conflict of Interest Statement
The authors declare that the research was conducted in the absence of any commercial or financial relationships that could be construed as a potential conflict of interest.
Acknowledgments
We thank Ben Passey for sharing his initial work on the biomineral isotope data compilation. We also thank the other members of the NESCent C4 Grasslands Working Group for valuable discussions that helped shaped this project: T. Michael Anderson, William Bond, Erika Edwards, Elisabeth Forrestel, William Hoffmann, Ben Passey, Nicolas Salamin, and Melinda Smith.
Supplementary Material
The Supplementary Material for this article can be found online at: https://www.frontiersin.org/articles/10.3389/fevo.2018.00147/full#supplementary-material
Data Sheet 1. Palesol carbonate stable isotope data compilation.
Data Sheet 2. Biomineral stable isotope data compilation.
References
Ahlström, A., Schurgers, G., and Smith, B. (2017). The large influence of climate model bias on terrestrial carbon cycle simulations. Environ. Res. Lett. 12:014004. doi: 10.1088/1748-9326/12/1/014004
Angelo, C. L., and Pau, S. (2016). Root biomass and soil δ13C in C3 and C4 grasslands along a precipitation gradient. Plant Ecol. 216, 615–627. doi: 10.1007/s11258-015-0463-y
Ardö, J. (2015). Comparison between remote sensing and a dynamic vegetation model for estimating terrestrial primary production of Africa. Carbon Balance Manage. 10:8. doi: 10.1186/s13021-015-0018-5
Beerling, D. J., and Royer, D. L. (2011). Convergent cenozoic CO2 history. Nat. Geosci. 4, 418–420. doi: 10.1038/ngeo1186
Beerling, D. J., and Woodward, F. I. (2001). Vegetation and the Terrestrial Carbon Cycle: The First 400 Million Years. Cambridge, UK: Cambridge University Press.
Behrensmeyer, A. K., Kidwell, S. M., and Gastaldo, R. A. (2000). Taphonomy and paleobiology. Paleobiology 26(sp4), 103–147. doi: 10.1666/0094-8373(2000)26[103:TAP]2.0.CO;2
Behrensmeyer, A. K., Quade, J., Cerling, T. E., Kappelman, J., Khan, I. A., Copeland, P., et al. (2007). The structure and rate of late Miocene expansion of C4 plants: evidence from lateral variation in stable isotopes in paleosols of the Siwalik Group, northern Pakistan. GSA Bull. 119, 1486–1505. doi: 10.1130/B26064.1
Bolton, C. T., and Stoll, H. M. (2013). Late Miocene threshold response of marine algae to carbon dioxide limitation. Nature 500, 558–562. doi: 10.1038/nature12448
Bond, W. J. (2008). What limits trees in C4 grasslands and savannas? Annu. Rev. Ecol. Evol. System. 39, 641–659. doi: 10.1146/annurev.ecolsys.39.110707.173411
Bond, W. J. (2015). Fires in the Cenozoic: a late flowering of flammable ecosystems. Front. Plant Sci. 5:749. doi: 10.3389/fpls.2014.00749
Bond, W. J., Woodward, F. I., and Midgley, G. F. (2005). The global distribution of ecosystems in a world without fire. New Phytol. 165, 525–538. doi: 10.1111/j.1469-8137.2004.01252.x
Bradshaw, C., Lunt, D., Flecker, R., Salzmann, U., Pound, M., Haywood, A., et al. (2012). The relative roles of CO2 and palaeogeography in determining Late Miocene climate: results from a terrestrial model-data comparison. Clim. Past 8, 715–786. doi: 10.5194/cpd-8-715-2012
Bragg, F. J., Lunt, D. J., and Haywood, A. M. (2012). Mid-Pliocene climate modelled using the UK Hadley Centre Model: PlioMIP experiments 1 and 2. Geosci. Model Dev. 5, 1109–1125. doi: 10.5194/gmd-5-1109-2012
Brierley, C. M., and Fedorov, A. V. (2016). Comparing the impacts of Miocene–Pliocene changes in inter-ocean gateways on climate: Central American Seaway, Bering Strait, and Indonesia. Earth Planet. Sci. Lett. 444, 116–130. doi: 10.1016/j.epsl.2016.03.010
Cerling, T. E. (1984). The stable isotope composition of modern soil carbonate and its relationship to climate: Earth Planet. Sci. Lett. 71, 229–240. doi: 10.1016/0012-821X(84)90089-X
Cerling, T. E., Andanje, S. A., Blumenthal, S. A., Brown, F. H., Chritz, K. L., Harris, J. M., et al. (2015). Dietary changes of large herbivores in the Turkana Basin, Kenya from 4 to 1 Ma. Proc. Natl. Acad. Sci. U.S.A. 112, 11467–11472. doi: 10.1073/pnas.1513075112
Cerling, T. E., and Harris, J. M. (1999). Carbon isotope fractionation between diet and bioapatite in ungulate mammals and implications for ecological and paleoecological studies. Oecologia 120, 347–363. doi: 10.1007/s004420050868
Cerling, T. E., Harris, J. M., MacFadden, B. J., Leakey, M. G., Quade, J., Eisenmann, V., et al. (1997). Global vegetation change through the Miocene/Pliocene boundary. Nature 389, 153–158. doi: 10.1038/38229
Cerling, T. E., Levin, N. E., Quade, J., Wynn, J. G., Fox, D. L., Kingston, J. D., et al. (2010). Comment on the paleoenvironment of Ardipithecus ramidus. Science 328:1105. doi: 10.1126/science.1185274
Cerling, T. E., and Quade, J. (1993). “Stable carbon and oxygen isotopes in soil carbonate,” in Climate Change in Continental Isotopic Records, Geophysical Monograph 78, eds P. K. Swart, K. C. Lohmann, J. McKenzie, and S. Savin (Washington DC: American Geophysical Union), 217–231. doi: 10.1029/GM078p0217
Cerling, T. E., Solomon, D. K., Quade, J., and Bowman, J. R. (1991). On the isotopic composition of carbon in soil carbon dioxide. Geochim. Cosmochim. Acta 55, 3403–3405. doi: 10.1016/0016-7037(91)90498-T
Cernusak, L. A., Ubierna, N., Winter, K., Holtum, J. A. M., Marshall, J. D., and Farquhar, G. D. (2013). Environmental and physiological determinants of carbon isotope discrimination in terrestrial plants. New Phytol. 200, 950–965. doi: 10.1111/nph.12423
Charles-Dominique, T., Davies, T. J., Hempson, G. P., Bezeng, B. S., Daru, B. H., Kabongo, R. M., et al. (2016). Spiny plants, mammal browsers, and the origin of African savannas. Proc. Natl. Acad. Sci. U.S.A. 113, E5572–E5579. doi: 10.1073/pnas.1607493113
Chen, S. T., Smith, S. Y., Sheldon, N. D., and Strömberg, C. A. E. (2015). Regional-scale variability in the spread of grasslands in the late Miocene. Palaeogeogr. Palaeoclimatol. Palaeoecol. 437, 42–52. doi: 10.1016/j.palaeo.2015.07.020
Christin, P.-A., Spriggs, E., Osborne, C. P., Strömberg, C. A. E., Salanin, N., and Edwards, E. J. (2014). Molecular dating, evolutionary rates, and the age of the grasses. Syst. Biol. 63, 153–165. doi: 10.1093/sysbio/syt072
Christin, P. A., Besnard, G., Samaritani, E., Duvall, M. R., Hodkinson, T. R., Savolainen, V., et al. (2008). Oligocene CO2 decline promoted C4 photosynthesis in grasses. Curr. Biol. 18, 37–43. doi: 10.1016/j.cub.2007.11.058
Collatz, G. J., Ball, J. T., Grivet, C., and Berry, J. A. (1991). Physiological and environmental regulation of stomatal conductance, photosynthesis and transpiration: a model that includes a laminar boundary layer. Agric. For. Meteorol. 54, 107–136. doi: 10.1016/0168-1923(91)90002-8
Collatz, G. J., Berry, J. A., and Clark, J. S. (1998). Effects of climate and atmospheric CO2 partial pressure on the global distribution of C4 grasses: present, past, and future. Oecologia 114, 441–454. doi: 10.1007/s004420050468
Collatz, G. J., Ribas-Carbo, M., and Berry, J. A. (1992). Coupled photosynthesis-stomatal conductance model for leaves of C4 plants. Aust. J. Plant Physiol. 19, 519–538. doi: 10.1071/PP9920519
Conn, S. G. (2012). Quantifying CO2 Levels of the Mid-Pliocene: A Data-Model Comparison Approach. Master's thesis, University of Bristol.
Cotton, J. M., Sheldon, N. D., and Strömberg, C. A. E. (2012). High-resolution isotopic record of C4 photosynthesis in a Miocene grassland. Palaeogeogr. Palaeoclimatol. Palaeoecol. 337–338, 88–98. doi: 10.1016/j.palaeo.2012.03.035
Cramer, W., Bondeau, A., Woodward, F. I., Prentice, I. C., Betts, R. A., Brovkin, V., et al. (2001). Global response of terrestrial ecosystem structure and function to CO2 and climate change: results from six dynamic global vegetation models. Glob. Chang. Biol. 7, 357–373. doi: 10.1046/j.1365-2486.2001.00383.x
DeNiro, M. J., and Epstein, S. (1978). Influence of diet on the distribution of carbon isotopes in animals. Geochim. Cosmochim. Acta 42, 495–506. doi: 10.1016/0016-7037(78)90199-0
Denny, M. (2017). The fallacy of the average: on the ubiquity, utility and continuing novelty of Jensen's inequality. J. Exp. Biol. 220, 139–146. doi: 10.1242/jeb.140368
Dupont, L. M., and Schefuss, E. (2018). The roles of fire in Holocene ecosystem changes of West Africa. Earth Planet. Sci. Lett. 481, 255–263. doi: 10.1016/j.epsl.2017.10.049
Edwards, E. J., Osborne, C. P., Strömber, C. A. E., Smith, S. A., and The C4 Grasses Consortium (2010). The origins of C4 grasslands: integrating evolutionary and ecosystem science. Science 328, 587–591. doi: 10.1126/science.1177216
Edwards, E. J., and Smith, S. A. (2010). Phylogenetic analyses reveal the shady history of C4 grasses. Proc. Natl. Acad. Sci. U.S.A. 107, 2532–2537. doi: 10.1073/pnas.0909672107
Edwards, E. J., and Still, C. J. (2008). Climate, phylogeny and the ecological distribution of C4 grasses. Ecol. Lett. 11, 266–276. doi: 10.1111/j.1461-0248.2007.01144.x
Ehleringer, J., and Björkman, O. (1977). Quantum yields for CO2 uptake in C3 and C4 plants. Plant Physiol. 59, 86–90. doi: 10.1104/pp.59.1.86
Ehleringer, J. R. (1978). Implications of quantum yield differences on the distributions of C3 and C4 grasses. Oecologia 31, 255–267. doi: 10.1007/BF00346246
Ehleringer, J. R., Cerling, T. E., and Helliker, B. R. (1997). C4 photosynthesis, atmospheric CO2, and climate. Oecologia 112, 285–299. doi: 10.1007/s004420050311
Eiler, J. M. (2011). Paleoclimate reconstruction using carbonate clumped isotope thermometry. Quat. Sci. Rev. 30, 3575–3588. doi: 10.1016/j.quascirev.2011.09.001
Eronen, J. T., Puolamäki, L. K., Lintulaakso, K., Damuth, J., Janis, C., and Fortelius, M. (2010). Precipitation and large herbivorous mammals I: estimates from present-day communities. Evol. Ecol. Res. 12, 217–233.
Feakins, S. J., Levin, N. E., Liddy, H. M., Sieracki, A., Eglinton, T. I., and Bonnefille, R. (2013). Northeast African vegetation change over 12 m.y. Geology 41, 295–298. doi: 10.1130/G33845.1
Foster, G. L., Royer, D. L., and Lunt, D. L. (2017). Future climate forcing potentially without precedent in the last 420 million years. Nat. Commun. 8:14845. doi: 10.1038/ncomms14845
Fox, D. L., and Koch, P. L. (2003). Tertiary history of C4 biomass in the Great Plains, USA. Geology 31, 809–812. doi: 10.1130/G19580.1
Fox, D. L., Martin, R. A., Honey, J. G., and Pelaez-Campomanes, P. (2012a). Pedogenic carbonate stable isotope record of environmental change during the Neogene in the southern Great Plains, southwest Kansas, USA: carbon isotopes and the evolution of C4-dominated grasslands. GSA Bull. 124, 444–462. doi: 10.1130/B30401.1
Fox, D. L., Martin, R. A., Honey, J. G., and Pelaez-Campomanes, P. (2012b). Pedogenic carbonate stable isotope record of environmental change during the Neogene in the southern Great Plains, southwest Kansas, USA: oxygen isotopes and paleoclimate during the evolution of C4-dominated grasslands. GSA Bull. 124, 431–443. doi: 10.1130/B30402.1
Ghosh, P., Adkins, J., Affek, H., Balta, B., Guo, W., Schauble, E. A., et al. (2006). 13C-18O bonds in carbonate minerals: a new kind of paleothermometer. Geochim. Cosmochim. Acta 70, 1439–1456. doi: 10.1016/j.gca.2005.11.014
Gile, L. H., Peterson, F. F., and Grossman, R. B. (1966). Morphological and genetic sequences of carbonate accumulation in desert soils. Soil Sci. 101, 347–360.
GPWGII—Grass Phylogeny Working Group II (2012). New grass phylogeny resolves deep evolutionary relationships and discovers C4 origins. New Phytol. 193, 304–312. doi: 10.1111/j.1469-8137.2011.03972.x
Griffith, D. M., Anderson, M. T., Osborne, C. P., Strömberg, C. A. E., Forrestel, E. J., and Still, C. J. (2015). Biogeographically distinct controls on C3 and C4 grass distributions: merging community and physiological ecology. Global Ecol. Biogeogr. 24, 304–313. doi: 10.1111/geb.12265
Griffith, D. M., Cotton, J. M., Powell, R. L., Sheldon, N. D., and Still, C. J. (2017). Multi-century stasis in C3 and C4 grass distributions across the contiguous United States since the industrial revolution. J. Biogeogr. 44, 2564–2574. doi: 10.1111/jbi.13061
Harestad, A. S., and Bunnel, F. L. (1979). Home range and body weight-a reevaluation. Ecology 60, 389–402. doi: 10.2307/1937667
Harris, G., Thirgood, S., Hopcraft, J. G. C., Cromsigt, J. P. G. M., and Berger, J. (2009). Global decline in aggregated migrations of large terrestrial mammals. Endanger. Species Res. 7, 55–76. doi: 10.3354/esr00173
Hattersley, P. W. (1983). The distribution of C3 and C4 grasses in Australia in relation to climate. Oecologia 57, 113–128. doi: 10.1007/BF00379569
Haywood, A. M., Dowsett, H. J., and Dolan, A. M. (2016). Integrating geological archives and climate models for the mid-Pliocene warm period. Nat. Commun. 7:10646. doi: 10.1038/ncomms10646
Herold, N., Müller, R. D., and Seton, N. (2010). Comparing early to middle Miocene terrestrial climate simulations with geologic data. Geosphere 6, 952–961. doi: 10.1130/GES00544.1
Hirota, M., Holmgren, M., Van Nes, E. H., and Scheffer, M. (2011). Global resilience of tropical forest and savanna to critical transitions. Science 334, 232–235. doi: 10.1126/science.1210657
Hoetzel, S., Dupont, L., Schefuss, E., Rommerskirchen, F., and Wefer, G. (2013). The role of fire in Miocene to Pliocene C4 grassland and ecosystem evolution. Nat. Geosci. 6, 1027–1030. doi: 10.1038/ngeo1984
Ivory, S. J., and Russell, J. (2016). Climate, herbivory, and fire controls on tropical African forest for the last 60ka. Q. Sci. Rev. 148, 101–114. doi: 10.1016/j.quascirev.2016.07.015
Jacobs, B. F., Kingston, J. D., and Jacobs, L. L. (1999). The origin of grass-dominated ecosystems. Ann. Miss. Bot. Gard. 86, 590–643. doi: 10.2307/2666186
Keeley, J. E., and Rundel, P. W. (2005). Fire and the miocene expansion of C4 grasslands. Ecol. Lett. 8, 683–690. doi: 10.1111/j.1461-0248.2005.00767.x
Kellogg, E. A. (1999). “Phylogenetics aspects of the evolution of C4 photosynthesis,” in C4 Plant Biology, eds R. F. Sage and R. K. Monson (San Diego, CA: Academic Press), 411–444. doi: 10.1016/B978-012614440-6/50013-6
Kleinert, K., and Strecker, M. R. (2001). Climate change in response to orographic barrier uplift: paleosol and stable isotope evidence from the late Neogene Santa María basin, northwestern Argentina. GSA Bull. 113, 728–742. doi: 10.1130/0016-7606(2001)113<0728:CCIRTO>2.0.CO;2
Koch, P. L., Tuross, N., and Fogel, M. L. (1997). The effects of sample treatment and diagenesis on the isotopic integrity of carbonate in biogenic hydroxylapatite. J. Archaeol. Sci. 24, 417–429. doi: 10.1006/jasc.1996.0126
Kohn, M. J., and Cerling, T. E. (2002). “Stable isotope compositions of biological apatite,” in Phosphates: Geochemical, Geobiological, and Materials Importance, Reviews in Mineralogy and Geochemistry, Vol. 48, eds M. J. Kohn, J. Rakovan, and J. M. Hughes (Washington, DC: Mineralogical Society of America and The Geochemical Society), 455–488. doi: 10.2138/rmg.2002.48.12
Kuechler, R. R., Dupont, L. M., and Schefuss, E. (2018). Hybrid insolation forcing of Pliocene monsoon dynamics in West Africa. Clim. Past 14, 73–84. doi: 10.5194/cp-14-73-2018
Kuechler, R. R., Schefuss, E., Beckmann, B., Dupont, L., and Wefer, G. (2013). NW African hydrology and vegetation during the Last Glacial cycle reflected in plant-wax-specific hydrogen and carbon isoptes. Q. Sci. Rev. 82, 65–67. doi: 10.1016/j.quascirev.2013.10.013
Kürschner, W. M., Kvaček, A., and Dilcher, D. L. (2008). The impact of Miocene atmospheric carbon dioxide fluctuations on climate and the evolution of terrestrial ecosystems. Proc. Natl. Acad. Sci. U.S.A. 105, 449–453. doi: 10.1073/pnas.0708588105
Lehmann, C. E. R., Anderson, T. M., Sankaran, M., Higgins, S. I., Archibald, S., Hoffmann, W. A., et al. (2014). Savanna vegetation-fire-climate relationships differ among continents. Science 343, 548–552. doi: 10.1126/science.1247355
Lehmann, C. E. R., Archibald, S. A., Hoffman, W. A., and Bond, W. J. (2011). Deciphering the distribution of the savanna biome. New Phytol. 91, 197–209. doi: 10.1111/j.1469-8137.2011.03689.x
Long, S. P. (1999). “Environmental responses,” in C4 Plant Biology, eds R. F. Sage and R. K. Monson (San Diego, CA: Elsevier), 215–249. doi: 10.1016/B978-012614440-6/50008-2
Lunt, D. J., Dunkley Jones, T., Heinemann, M., Huber, M., LeGrande, A., Winguth, A., et al. (2012). A model-data comparison for a multi-model ensemble of early Eocene atmosphere-ocean simulations: EoMIP. Clim. Past 8, 1717–1736. doi: 10.5194/cp-8-1717-2012
Lunt, D. J., Ross, I., Hopley, P. J., and Valdes, P. J. (2007). Modelling late Oligocene C4 grasses and climate. Palaeogeogr. Palaeoclimatol. Palaeoecol. 251, 239–253. doi: 10.1016/j.palaeo.2007.04.004
MacFadden, B. J., Solounias, N., and Cerling, T. E. (1999). Ancient diets, ecology, and extinction of 5-million-year-old horses from Florida. Science 283, 824–827. doi: 10.1126/science.283.5403.824
McInerney, F. A., Strömberg, C. A. E., and White, J. W. C. (2011). The Neogene transition from C3 to C4 grasslands in North America: stable carbon isotope ratios of fossil phytoliths. Paleobiology 37, 23–49. doi: 10.1666/09068.1
McInerney, F. A., and Wing, S. L. (2011). The paleocene-eocene thermal maximum: a perturbation of carbon cycle, climate, and biosphere with implications for the future. Annu. Rev. Earth Planet. Sci. 39, 489–516. doi: 10.1146/annurev-earth-040610-133431
Miller, G. H., Fogel, M. L., Magee, J. W., Gagan, M. K., Clarke, S. J., and Johnson, B. J. (2005). Ecosystem collapse in Pleistocene Australia and a human role in megafaunal extinction. Science 309, 287–290. doi: 10.1126/science.1111288
Morgan, J. A., LeCain, D. R., Pendall, E., Blumenthal, D. M., Kimball, B. A., Carrillo, Y., et al. (2011). C4 grasses prosper as carbon dioxide eliminates desiccation in warmed semi-arid grassland. Nature 476, 202–205. doi: 10.1038/nature10274
Nemani, R., and Running, S. W. (1996). Implementation of a hierarchical global vegetation classification in ecosystem function models. J. Veget. Sci. 7, 337–346. doi: 10.2307/3236277
Osborne, C. P. (2008). Atmosphere, ecology and evolution: what drove the Miocene expansion of C4 grasslands? J. Ecol. 96, 35–45. doi: 10.1111/j.1365-2745.2007.01323.x
Osborne, C. P., and Freckleton, R. P. (2008). Ecological selection pressures for C4 photosynthesis in the grasses. Proc. R. Soc. B. 276, 1753–1760. doi: 10.1098/rspb.2008.1762
Pagani, M., Freeman, K. H., and Arthur, M. (1999). Late Miocene atmospheric CO2 concentrations and the expansion of C4 grasses. Science 285, 876–879. doi: 10.1126/science.285.5429.876
Passey, B. H., Ayliffe, L. K., Kaakinen, A., Zhang, Z., Eronen, J. T., Zhu, Y., et al. (2009). Strengthened East Asian summer monsoons during a period of high-latitude warmth? Isotopic evidence from Mio-Pliocene fossil mammals and soil carbonates from northern China. Earth Planet. Sci. Lett. 277, 443–452. doi: 10.1016/j.epsl.2008.11.008
Passey, B. H., Cerling, T. E., Perkins, M. E., Voorhies, M. R., Harris, J. M., and Tucker, S. T. (2002). Environmental change in the Great Plains: an isotopic record from fossil horses. J. Geol. 110, 123–140. doi: 10.1086/338280
Passey, B. H., Robinson, T. F., Ayliffe, L. K., Cerling, T. E., Sponheimer, M., Dearing, M. D., et al. (2005). Carbon isotope fractionation between diet, breath CO2, and bioapatite in different mammals. J. Archaeol. Sci. 32, 1459–1470. doi: 10.1016/j.jas.2005.03.015
Pearcy, R. W., and Ehleringer, J. R. (1983). Comparative ecophysiology of C3 and C4 plants. Plant Cell Environ. 7, 1–13. doi: 10.1111/j.1365-3040.1984.tb01194.x
Phillips, D. L., and Gregg, J. W. (2001). Uncertainty in source partitioning using stable isotopes. Oecologia 127, 171–179. doi: 10.1007/s.004420000578
R Development Core Team (2013). R: A Language and Environment for Statistical Computing. Vienna: R Development Core Team.
Reich, P. B., Hobbie, S. E., Lee, T. D., and Pastore, M. A. (2018). Unexpected reversal of C3 versus C4 grass response to elevated CO2 during a 20-year field experiment. Science 360, 317–320. doi: 10.1126/science.aas9313
Sage, R. F. (2004). The evolution of C4 photosynthesis. New Phytol. 161, 341–370. doi: 10.1111/j.1469-8137.2004.00974.x
Sage, R. F., Christin, P. A., and Edwards, E. J. (2011). The C-4 plant lineages of planet Earth. J. Exp. Bot. 62, 3155–3169. doi: 10.1093/jxb/err048
Sage, R. F., Wedin, D. A., and Li, M. (1999). “The biogeography of C4 photosynthesis: patterns and controlling factors,” in C4 Plant Biology eds R. F. Sage and R. K. Monson (San Diego, CA: Elsevier), 313–373. doi: 10.1016/B978-012614440-6/50011-2
Sankaran, M., Hanan, N. P., Scholes, R. J., Ratnam, J., Augustine, D. J., Cade, B. S., et al. (2005). Determinants of woody cover in African savannas. Nature 438, 846–849. doi: 10.1038/nature04070
Schefuß, E., Kuhlmann, H., Millenhauer, G., Prange, M., and Pätzold, J. (2011). Forcing of wet phases in southeast Africa over the past 17,000 years. Nature 480, 509–512. doi: 10.1038/nature10685
Scheiter, S., Higgins, S. I., Osborne, C. P., Bradshaw, C., Lunt, D., Ripley, B. S., et al. (2012). Fire and fire-adapted vegetation promoted C4 expansion in the late Miocene. New Phytol. 195, 653–666. doi: 10.1111/j.1469-8137.2012.04202.x
Schirnding, Y., Van Der Merwe, N. J., and Vogel, J. C. (1982). Influence of diet and age on carbon isotope ratios in ostrich eggshell. Archaeometry 24, 3–20. doi: 10.1111/j.1475-4754.1982.tb00643.x
Scott, A. C. (2000). The pre-quaternary history of fire. Palaeogeogr. Palaeoclimatol. Palaeoecol. 164, 281–329. doi: 10.1016/S0031-0182(00)00192-9
Sellers, P. J., Randall, D. A., Collatz, G. J., Berry, J. A., Field, C. B., Dazlich, D. A., et al. (1996). A revised land surface parameterization (SiB2) for atmospheric GCMs. Part I: model formulation. J. Clim. 9, 676–705. doi: 10.1175/1520-0442(1996)009<0676:ARLSPF>2.0.CO;2
Sheldon, N. D., and Tabor, N. J. (2009). Quantitative paleoenvironmental and paleoclimatic reconstruction using paleosols. Earth Sci. Rev. 95, 1–52. doi: 10.1016/j.earscirev.2009.03.004
Sitch, S., Smith, B., Prentice, I. C., Arneth, A., Bondeau, A., Cramer, W., et al. (2003). Evaluation of ecosystem dynamics, plant geography and terrestrial carbon cycling in the LPJ dynamic global vegetation model. Glob. Chang. Biol. 9, 161–185. doi: 10.1046/j.1365-2486.2003.00569.x
Smiley, T. M., Hyland, E. G., Cotton, J. M., and Reynolds, R. E. (2016). Evidence of early C4 grasses, habitat heterogeneity, and faunal response during the miocene climatic optimum in the mojave region. Palaeogeogr. Palaeoclimatol. Palaeoecol. 490, 415–430. doi: 10.1016/j.palaeo.2017.11.020
Smith, M. D., and Knapp, A. K. (2003). Dominant species maintain ecosystem function with non-random species loss. Ecol. Lett. 6, 509–517. doi: 10.1046/j.1461-0248.2003.00454.x
Spriggs, E. L., Christin, P.-A., and Edwards, E. J. (2014). C4 photosynthesis promoted species diversification during the Miocene grassland expansion. PLoS ONE 9:e97722. doi: 10.1371/journal.pone.0097722
Staver, A. C., Archibald, S., and Levin, S. A. (2011). The global extent and determinants of savanna and forest as alternative biome states. Science 334, 230–232. doi: 10.1126/science.1210465
Stern, L. A., Johnson, G. D., and Chamberlain, C. P. (1994). Carbon isotope signature of environmental change found in fossil ratite eggshells from a South Asian Neogene sequence. Geology 22, 419–422. doi: 10.1130/0091-7613(1994)022<0419:CISOEC>2.3.CO;2
Still, C. J., Berry, J. A., Collatz, G. J., and DeFries, R. S. (2003). Global distribution of C3 and C4 vegetation: carbon cycle implications. Global Biogeochem. Cycles 17:1006. doi: 10.1029/2001GB001807
Stinchcomb, G. E., Nordt, L. C., Driese, S. G., Lukens, W. E., Williamson, F. C., and Tubbs, J. D. (2016). A data-driven spline model designed to predict paleoclimate using paleosol geochemistry. Am. J. Sci. 316, 746–777. doi: 10.2475/08.2016.02
Strömberg, C. A. E. (2006). Evolution of hypsodonty in horses: testing a hypothesis of adaptation. Paleobiology 32, 236–258. doi: 10.1666/0094-8373(2006)32%5b236:EOHIET%5d2.0.CO;2
Strömberg, C. A. E. (2011). Evolution of grasses and grassland ecosystems. Annu. Rev. Earth Planet. Sci. 39, 517–544. doi: 10.1146/annurev-earth-040809-152402
Strömberg, C. A. E., and McInerney, F. A. (2011). The Neogene transition from C3 to C4 grasslands in North America: assemblage analysis of fossil phytoliths. Paleobiology 37, 50–71. doi: 10.1666/09067.1
Teeri, J. A., and Stowe, L. G. (1976). Climatic patterns and distribution of C4 grasses in North America. Oecologia 23, 1–12. doi: 10.1007/BF00351210
Tipple, B. J., Meyers, S. R., and Pagani, M. (2010). Carbon isotope ratio of Cenozoic CO2: a comparative evaluation of available geochemical proxies. Paleoceanography 25:PA3202.
Tipple, B. J., and Pagani, M. (2007). The early origins of terrestrial C4 photosynthesis. Annu. Rev. Earth Planet. Sci. 35, 435–461, doi: 10.1146/annurev.earth.35.031306.140150
Urban, M. A., Nelson, D. M., Street-Perrott, F. A., Verschuren, D., and Hu, F. S. (2015). A late-Quaternary perspective on atmospheric pCO2, climate, and fire as drivers of C4-grass abundance. Ecology 96, 642–653.
Valdes, P. J., Armstrong, E., Badger, M. P., Bradshaw, C. D., Bragg, F., Crucifix, M., et al. (2017). The BRIDGE HadCM3 family of climate models: HadCM3@ Bristol v1. 0. Geosci. Model Dev. 10, 3715–3743. doi: 10.5194/gmd-10-3715-2017
Visser, V., Clayton, W. D., Simpson, D. A., Freckleton, R. P., and Osborne, C. P. (2014). Mechanisms driving an unusual latitudinal diversity gradient for grasses. Global Ecol. Biogeogr. 23, 61–75. doi: 10.1111/geb.12107
Visser, V., Woodward, F. I., Freckleton, R. P., and Osborne, C. P. (2012). Determinants of the phylogenetic structure of C4 grass communities: testing the role of environmental factors. J. Biogeogr. 39, 232–246. doi: 10.1111/j.1365-2699.2011.02602.x
von Fischer, J. C., Tiezsen, L. L., and Schimel, D. S. (2008). Climate controls on C3 vs. C4 productivity in North American grasslands from carbon isotope composition of soil organic matter. Global Change Biol. 14, 1141–1155. doi: 10.1111/j.1365-2486.2008.01552.x
Wang, Y., and Cerling, T. E. (1994). A model of fossil tooth and bone diagenesis: implications for paleodiet reconstruction from stable isotopes. Palaeogeogr. Palaeoclimatol. Palaeoecol. 107, 281–289. doi: 10.1016/0031-0182(94)90100-7
Watcharamongkol, T., Christin, P.-A., and Osborne, C. P. (2018). C4 photosynthesis evolved in warm climates but promoted migration to cooler ones. Ecol. Lett. 21, 376–383. doi: 10.1111/ele.12905
Woodward, F., and Lomas, M. (2004). Vegetation dynamics–simulating responses to climatic change. Biol. Rev. 79, 643–670. doi: 10.1017/S1464793103006419
Woodward, F. I., Lomas, M. R., and Lee, S. E. (2001). “Predicting the future productivity and distribution of global terrestrial vegetation,” in Terrestrial Global Productivity, eds J. Roy, B. Saugier, and H. A. Mooney (San Diego, CA: Academic Press), 521–541.
Zamanian, K., Pustovoytov, K., and Kuzyakov, Y. (2016). Pedogenic carbonates: forms and formation processes. Earth Sci. Rev. 157, 1–17. doi: 10.1016/j.earscirev.2016.03.003
Zazzo, A., Lecuyer, C., and Mariotti, A. (2004). Experimentally-controlled carbon and oxygen isotope exchange between bioapatites and water under inorganic and microbially-mediated conditions. Geochim. Cosmochim. Acta 68, 1–12. doi: 10.1016/S0016-7037(03)00278-3
Keywords: Miocene, Pliocene, C4 grasses, carbon isotopes, model-data comparison, vegetation models
Citation: Fox DL, Pau S, Taylor L, Strömberg CAE, Osborne CP, Bradshaw C, Conn S, Beerling DJ and Still CJ (2018) Climatic Controls on C4 Grassland Distributions During the Neogene: A Model-Data Comparison. Front. Ecol. Evol. 6:147. doi: 10.3389/fevo.2018.00147
Received: 21 May 2018; Accepted: 04 September 2018;
Published: 24 September 2018.
Edited by:
Oana Moldovan, Emil Racovita Institute of Speleology, RomaniaReviewed by:
David Nelson, University of Maryland Center for Environmental Science (UMCES), United StatesLydie M. Dupont, Zentrum für Marine Umweltwissenschaften, Universität Bremen, Germany
Copyright © 2018 Fox, Pau, Taylor, Strömberg, Osborne, Bradshaw, Conn, Beerling and Still. This is an open-access article distributed under the terms of the Creative Commons Attribution License (CC BY). The use, distribution or reproduction in other forums is permitted, provided the original author(s) and the copyright owner(s) are credited and that the original publication in this journal is cited, in accordance with accepted academic practice. No use, distribution or reproduction is permitted which does not comply with these terms.
*Correspondence: David L. Fox, dlfox@umn.edu
†Present Address: Catherine Bradshaw, Met Office Hadley Centre, Exeter, United Kingdom
Stephen Conn, School of Earth and Ocean Sciences, Cardiff University, Cardiff, United Kingdom