- 1Department of Psychology, University of Michigan, Ann Arbor, MI, United States
- 2Department of Biology, Miami University, Oxford, OH, United States
- 3Department of Ecology and Evolutionary Biology, University of Michigan, Ann Arbor, MI, United States
Accurately recording the social and mating behavior of wild animals is necessary to test hypotheses regarding the evolution of monogamous behavior but documenting the behavior of most wild animals is challenging. Social network analyses can use patterns of spatial and temporal co-occurrence to describe the social associations of individuals within a population, such as which opposite-sex individuals are found together more frequently than others as an indicator of their degree of social monogamy. Social networks generated using automated radio frequency identification (RFID) tracking systems may provide insights into the social behavior of secretive animals because they enable the automated and continuous tracking of the social associations among individuals, which can address many of the limitations with studying these kinds of species. We assessed the potential for social networks generated using an automated RFID tracking system to describe the social behavior of prairie voles (Microtus ochrogaster) in semi-natural enclosures. Our aim was to assess whether social networks generated using the RFID system provided meaningful insights into the social behavior of voles by comparing this method to other methods that have been traditionally used in laboratory (partner preference tests) or field (degree of home range overlap) studies to study social monogamy in prairie voles. In partner preference tests conducted in the field, females spent more time with males with which they had stronger social network associations. Voles that had stronger social network associations also had home ranges that overlapped considerably more than dyads with lower social network associations. In addition, social networks generated from live-trapping and RFID data were comparable but social networks generated using data from our RFID system recorded almost twice as many social associations overall. Our results show that social association metrics derived from social networks generated using the RFID tracking system reflect other commonly used measures of social monogamy in prairie voles. Overall, this suggests that patterns of spatial and temporal co-occurrence are meaningful measures of social monogamy in wild animals.
Introduction
Understanding the evolution of social monogamy is a central aim in animal behavior research (Wittenberger and Tilson, 1980; Komers and Brotherton, 1997; Dobson et al., 2010; Lukas and Clutton-Brock, 2013; Opie et al., 2013; Klug, 2018). Definitions of social monogamy typically focus on how two opposite-sex individuals share a living space and interact with one another (Kleiman, 1977; Reichard, 2003; Tecot et al., 2016; Klug, 2018). Studying social monogamy therefore requires quantifying which opposite-sex individuals are more likely to be found together over time and the interactions between these individuals. This is possible in some species that are directly observable and able to be habituated to humans, but most species are secretive, nocturnal, or not readily habituated to human presence.
To date, many studies that characterize the socially monogamous behavior of secretive species do so indirectly such as by estimating nest sharing (Ribble and Salvioni, 1990), assessing patterns of home range overlap using telemetry (Böhm et al., 2008), or by bringing animals into the laboratory to conduct behavioral assays to quantify their socially monogamous or pair-bonding behavior (Williams et al., 1992; Salo and Dewsbury, 1995; Leese, 2012; Carp et al., 2016). However, there are potential complications with each of these methods. Estimates of home range overlap between two opposite-sex individuals are often based on methods that record an individual's location for a brief moment and may only reflect whether individuals generally use the same portions of their habitat. Although behavioral assays conducted in the laboratory may provide some insight into what can occur in a simplified environment, they may not reflect the natural behavior of wild animals.
Automated tracking systems are one way to deal with these limitations. In these systems, each individual has a passive integrated transponder (PIT) tag that corresponds to a unique number sequence for identification. Radio frequency identification (RFID) antennae then record the unique identification of any PIT tag within a given radius of the antenna for the time that the tag is within range. This information is then recorded and saved by a central reader. These systems have been used to address a number of questions (see Bonter and Bridge, 2011) such as habitat use (Harper and Batzli, 1996; Godsall et al., 2014; Soanes et al., 2015) or quantifying foraging behavior (Newey et al., 2009) and burrow use (Rehmeier et al., 2006; Smith et al., 2018). Because these systems record data continuously, they offer a larger, uninterrupted data set from throughout the day, which is useful for nocturnal species. Further, after initial setup and tagging, human presence is limited so animals do not have to be habituated.
Social network analyses, using data from RFID systems, may also be useful for quantifying socially monogamous behavior, but this has rarely been done (but see Leu et al., 2010; Streatfeild et al., 2011). These data can quantify the number and strength of social connections between opposite-sex conspecifics (Wasserman and Faust, 1994; Wey et al., 2008; Whitehead, 2008). Specifically, social network analyses allow estimation of the strength of the social interaction or association for each dyad (the association index) as well as the overall level of sociality and the total number of social connections per individual (weighted degree and unweighted degree, respectively: Wey et al., 2008; Whitehead, 2008; Farine and Whitehead, 2015).
We investigated whether social network metrics derived from an automated tracking system could describe the social behavior of free-living prairie voles (Microtus ochrogaster) in semi-natural enclosures. Prairie voles are a model system to document the causes of individual differences in social and mating behavior (Getz et al., 1987; Hammock and Young, 2002; Solomon and Jacquot, 2002; Ophir et al., 2008b) and to understand the evolution of social monogamy (Ophir et al., 2008a; Solomon et al., 2009; Streatfeild et al., 2011). Despite being a model system to study social monogamy, the methods used to measure monogamous behavior differ between studies conducted in the laboratory and those in the field. For example, partner preference tests are commonly used to measure pair-bonding of voles in laboratory studies (Williams et al., 1992; Donaldson et al., 2010), whereas field studies employ other methods such as live-trapping males and females together at nests (Solomon et al., 2009) or radio telemetry to document the space use of males and females (Solomon and Jacquot, 2002; Ophir et al., 2008b; Lambert, 2018).
We collected spatial and temporal co-occurrence data to develop social networks using an automated tracking system that monitored voles implanted with PIT tags living within enclosures that contained an array of RFID antennas (Figure 1). We then used social network metrics derived from this automated RFID tracking system to quantify the social relationships in our two enclosures. Our main goal was to assess if the social associations estimated from social network analyses using data recorded from the RFID system were also found using traditional methods of quantifying social connections, including behavioral assays (partner preference test: Williams et al., 1992), home range overlap, and social network analyses using live-trapping data.
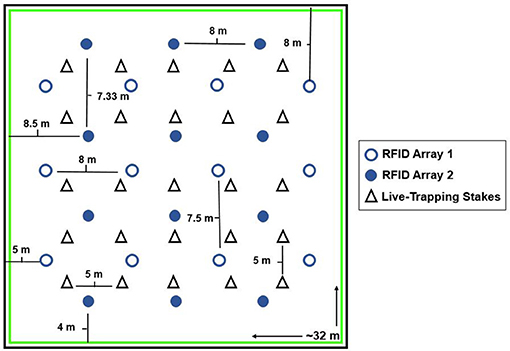
Figure 1. The layout of both RFID antenna arrays and the grid stakes used as locations for live-trapping in both enclosures. RFID Array 1 is depicted by open circles, (12 antenna each 7.5 or 8 m apart) whereas Array 2 is depicted by filled circles (12 antenna each 7.33 or 8 m apart). The 5 × 5 grid for live-trapping is shown by the triangles. Two Ugglan live-traps were placed at each of the 25 grid stakes (all stakes approximately 5 meters apart). The green box represents the 1 m that was mowed around the edge of the enclosure to prevent voles from digging near the walls. Note that we had two enclosures in this same format.
Methods
Study Site
Field work was conducted at the Ecology Research Center at Miami University in Oxford, Ohio (39° 53′N, 84° 73′W) between May and August 2017. We used two 0.1 ha (approximately 32 × 32 m) enclosures surrounded by a 20-gauge galvanized steel fence, 75 cm above and 45 cm below ground to prevent voles from moving between enclosures (Cochran and Solomon, 2000). Approximately 1 m was mowed around the edge of the enclosures to discourage voles from digging near the enclosure walls. Fences were regularly checked for any holes, gaps, or burrows near the edges to ensure that voles could not escape. Both enclosures contained a mix of goldenrod (Solidago spp.), bluegrass (Poa pratensis), clover (Trifolium spp.), fescue (Festuca spp.), timothy (Phleum spp.), and ryegrass (Elymus spp.: Solomon et al., 2009) that provided food and cover for voles. An electric wire was run across the top of the fence and turned on whenever researchers were not in the enclosures to prevent mammals such as raccoons from entering the enclosures and disturbing traps (although we did not encounter this during our study). Other predators, including predatory birds and snakes, could potentially enter the enclosures and we observed snakes within the enclosures (ACS and BD personal observations) and also observed predation due to avian predators (likely owls). Prior to releasing the voles inside the enclosures, we live-trapped for 3 days without catching any other small mammal species to ensure that none were in the enclosures at the start of the field season. We occasionally caught mice (n = 5 Peromyscus spp.) and shrews (n = 2) within the enclosures throughout the field season and released them outside the enclosures.
Study Animals
We used 7–8th generation laboratory-bred prairie voles descended from voles that were originally captured in Illinois. We released voles from this laboratory population into both enclosures in late May 2017. One enclosure started out at twice the density of the other (48 voles vs. 24 voles, both with equal sex ratios) but reached a similar density by the end of the field season. Populations were started with different densities due to another project conducted simultaneously. Densities in both enclosures were within the natural range found in wild prairie vole populations (Getz et al., 1993, 2001). No opposite-sex siblings or opposite-sex parents and offspring were released into the same enclosure to prevent inbreeding and provide the same number of non-sibling mating partners for all individuals. If any voles were found dead within the first week of the study, we replaced them with another same-sex vole from the laboratory colony (n = 2 females, 1 male).
Adult voles were identified by unique passive integrated transponder (PIT) tags (Biomark: Boise, Idaho, 12 mm HPT tags) implanted before release. In two cases where we trapped voles with PIT tags that were not working, we implanted new PIT tags. For one of these voles, we were able to determine which individual it was, while the other could not be identified as any individual from the initial population. All offspring produced were left in the enclosures but were not included in any of our analyses. Voles in the enclosures were not provided with any supplemental food besides small amounts of cracked corn used to bait traps.
Live Trapping
We used live trapping to collect social interaction data, assess reproductive condition of voles, and capture offspring produced in the enclosures (as we have done previously, see Solomon et al., 2009). We placed two live-traps at each stake in a 5 × 5 grid system (stakes approximately 5 m apart, see Figure 1) for a total of 50 live traps in each enclosure. We used Ugglan multiple capture traps (Granhab, Sweden) covered with sheet metal (to protect from sun or rain), which allow for multiple individuals to be captured per trap. To locate nests, we used both powder-tracking by dipping female voles in UV fluorescent powder, releasing them, and then following their powder trail with a black light back to their burrows or surface nests (Lemen and Freeman, 1985) and VHF radio telemetry (see below). Once we identified the location of a nest, we also set two traps at each burrow or nest entrance every time we set the trapping grid to increase our chances of catching adult voles and any offspring produced. We identified 15 surface and underground nests (burrows) that were actively used, 9 in enclosure 1 and 6 in enclosure 2, resulting in a maximum of 26 or 18 additional live traps for enclosures 1 and 2, respectively.
At the beginning of the experiment, we live-trapped nearly every day but after the first 2 weeks, we reduced live-trapping to allow the RFID system to collect more data overnight and to provide more time for locating voles using VHF radio telemetry. From weeks 3 to 14, we set traps three times per week (Monday, Wednesday, and Friday) alternating between the two enclosures so that voles in each enclosure were trapped three different days during each 2-week period. Live traps were set and checked on three different schedules depending on what data were needed: set in the late evening (between 22:30 and 23:00) and checked in the morning (starting at 7:00), set in the early evening traps (between 18:00 and 19:00) and checked 2 h later, and set in the morning (starting at 5:00) and checked 2 h later. Originally, we set traps in the evening and overnight, but stopped setting them overnight once it was likely that offspring had been born (Richmond and Conaway, 1969) and were moving independently outside the nest (N. Solomon, B. Keane, unpublished data) to prevent them from being separated from their parents for the entire night. Finally, we did not trap when there were thunderstorms but set live-traps on a different day during the week if possible. See Table S1 for full details on trapping schedule. Each time an individual was trapped we recorded its body mass (g), reproductive condition, other individuals in the same live trap, and the trap location.
Radio Frequency Identification (RFID) Data
We placed 12 radio frequency identification (RFID) antennas in two different 3 × 4 arrays throughout the enclosure (see Figure 1 for layout of both arrays), all connected to a central PIT tag reader system (Biomark, RM310/SM303 system). This system records the unique PIT tag numbers of any vole traveling within approximately 6 inches of the antenna (ACS personal observation), once per second. When multiple tags are present at the antenna, it alternates between recording the two (ACS personal observation), therefore to miss individuals present at an antenna at the same time both individuals would need to be within range of the antenna at the same time for >2 s. The antenna system was rotated every 3 days from enclosure 1 array 1, enclosure 1 array 2, enclosure 2 array 1, enclosure 2 array 2, so that the RFID system was in each enclosure for six continuous days.
VHF Radio Telemetry
To locate vole nests and record spatial movements, we tracked voles with VHF radio telemetry. We placed VHF collars (Holohil model PD-2C, approximately 3 g) on as many adult voles as possible, locating individuals four times per week between 11:00 and 16:00 h with at least an hour between each relocation. We recorded each location with a handheld GPS unit (Trimble Navigation Limited, Trimble Geo 7x) and searched around the location to identify burrows or surface nests that were actively in use (Lucia et al., 2008). Overall, each vole was located between 5 and 71 times with VHF telemetry depending on the duration they were wearing the collar, with an average of 22.36 ± 12.70 locations per vole.
Partner Preference Tests
To test how male-female associations generated from the social network analyses using the RFID system compared to measures of pair bond formation, we conducted partner preference tests in the field. Partner preference tests are an established laboratory method used to assess pair bonding in prairie voles (Williams et al., 1992). We modified a laboratory protocol to conduct partner preference tests in the field to determine if females preferred individuals they were associated with based on the social networks generated using the RFID system. These tests were conducted in a three chambered, clear plastic arena where the female was placed in the middle of the arena, with a male partner (or potential partner) on one side and a novel (or potentially less-familiar) male called a stranger) on the other side. The three different parts of the arena (76 × 21 × 30 cm) were separated by 5 cm clear plastic dividers with a 6 cm opening for movement of focal females.
Once initial data from the RFID system were available after the first few weeks of the study, we tested females when a male and a female vole were recorded at the same antenna within ≤ 1.5 min of each other. Because this pair was recorded at least once at the same place close in time and pairs often spatially co-occur (Hofmann et al., 1984), it is likely that they would have a high association index for the entire season. Therefore, these male-female dyads became our potential pairs for the partner preference tests. Following morning live-trapping, we conducted partner preference tests (from approximately 08:30 to 12:00 h) on any potential pairs that were both caught that morning. The female from this pair would be the focal female in the trial and the male would be the potential partner. We chose the potential stranger based on the available males caught that day that were closest to the male partner in age and body mass and not caught at an adjacent live-trapping location. We note that although we chose the potential pairs after only a small portion of the field season, the average association index from social network analyses generated using the RFID system from the entire field season supported our choices. Specifically, the average association index (the strength of their social association, see below for details on calculation) for focal females with their potential partners for the entire field season was 0.04, whereas the focal female's average association index with the potential stranger for the entire field season was 0.0042.
Each female was only tested once (n = 10). The arena was placed in an opaque plastic bin in the shade next to the enclosures. We observed trials from a distance of approximately 1 m to ensure that trials were not disturbed. For half of the trials the partner was on the left side of the arena and for half the partner was on the right side of the arena. Which side the partner was on for each given trial was randomized. Both males were placed on their respective sides of the arena, loosely secured with a tether made from a cable-tie and monofilament line (Castelli et al., 2011) and given ad libitum access to water. The males were given 20 min to habituate to the tethers and the arena before the focal female was placed in the arena. The first 4 trials lasted 3 h after habituation (Curtis et al., 2001), but to conduct more trials and reduce the time that voles were kept out of the enclosures, the trial length was reduced to 1 h after a 20 min habituation period for males. The trial was recorded from above in real time by a video camera. Videos were scored by 4 observers using JWatcher (Blumstein and Daniel, 2007) to quantify the amount of time females spent on the partner's side of the arena, the stranger's side of the arena, or the neutral middle part. We defined “time spent” based on when the female's head crossed the border into a new area. For the few trials that lasted 3 h, we only used data from the first hour in order to make it more comparable to the 1-h trials. Observers could not be blind to which male vole was the potential partner and which male vole was the potential stranger because they assigned the males to each trial. However, our response variable for these trials (time spent in each part of the enclosure) should be unaffected by any observer biases.
Patterns of Vole Activity
We also investigated when voles were most active based on what time of day the RFID system recorded the most PIT tags. The circadian activity cycle of prairie voles has been suggested to be diurnal, crepuscular, nocturnal, or ultradian (Madison, 1985), although there seems to be more support for a crepuscular rhythm (Calhoun, 1945). To investigate prairie vole activity, we identified how many hits the RFID system recorded per hour. We omitted days in which live traps were set in the same enclosure as the RFID system to control for reduced activity if voles were in live traps.
Construction of Social Networks
Although social networks provide measures of the associations among conspecifics and of an individual's level of sociality, we were specifically interested in the following two measures:
1. Association index, or the strength of the association of each dyad (female-male, female-female, male-male) of voles in each enclosure;
2. Unweighted degree, or the number of unique voles each individual was connected to.
We constructed social networks and conducted social network analyses using the R package asnipe (version 1.1.4, Farine, 2017). To construct the social networks from the RFID system data, we ran the raw RFID system data through a Gaussian mixture model in R, which creates groups based on the distribution of voles at each antenna through time (Psorakis et al., 2012). These groups were then used to form a network where voles placed in the same group have a connection. The number of these connections (the unweighted degree) represents the number of unique individuals with which voles had associations. Each individual connection is weighted by a simple ratio index, a type of association index, which is the number of times that dyad was observed together divided by the sum of the number of the times they were observed together or separately, representing the association rate of that dyad (Cairns and Schwager, 1987). We used a simple ratio index instead of a half-weight index because we did not break key assumptions, like individuals being more likely to be recorded when associated or not recording all associations at a given time (Whitehead, 2008). Further, Krackhardt (1988) suggest that using the half-weight index may over-correct for any biases in the data. Live-trapping networks were made by creating a group-by-individual matrix, where individuals caught at the same location (the same grid stake or nest) when traps were checked were placed in the same group (Solomon et al., 2009; Streatfeild et al., 2011). The social network was then created from the group by individual matrix in asnipe and association indices were weighted using a simple ratio index as well. Network figures were created using the R package igraph (version 1.1.2, Csardi and Nepusz, 2006).
Statistical Analyses
All analyses were conducted in R version 3.4.1 (R Core Team, 2017). We visually examined the distributions of the response variables to check for normality and visually examined the residuals to check for normality and homoscedasticity when appropriate (Zuur et al., 2010).
The full live-trapping and RFID system social networks were compared using a multiple regression quantitative assignment procedure (MRQAP: Krackhardt, 1988) in the R package asnipe. Specifically, we used an ordinary least squared (OLS) network regression with the live-trapping network as the predictor and the RFID network as the response variable. We used random social networks generated through network permutations on the group by individual matrix (produced from the Gaussian mixture model conducted using the RFID data) as the random networks used in the MRQAP. We also used a Spearman's rank correlation to compare how individuals were ranked based on their unweighted degree in both networks. Because the RFID system generated substantially more spatial and temporal co-occurrence data for the social networks compared to those generated using the live-trapping data (see Results), our assessments of how the social network metrics compared to the results from the partner preference tests and home range overlap were conducted using only the social network metrics generated from the RFID system and not the live-trapping data.
Using spatial coordinates from the locations generated by the RFID system, live-trapping, and VHF radio telemetry we calculated minimum convex polygon (mcp) home range area estimates in the R package adehabitatHR (version 0.4.15; Calenge, 2006). As required by this package, we removed any individuals that had fewer than 5 locations with each method and all individuals that were only ever located at one location (see the sample size for each method in Table 1). We compared home range estimates generated by the three methods using a linear mixed-effects model with method (live-trapping, telemetry, or RFID system), sex, and enclosure as fixed effects and vole ID as a random effect (R package lme4 version 1.1–1; Bates et al., 2015, P-values calculated by lmerTest, version 2.0.36, Kuznetsova et al., 2017). Home range estimates were transformed by taking the square root before running the model to improve normality of the residuals.
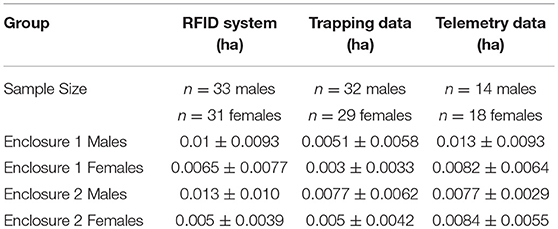
Table 1. The mean and standard deviation for the minimum convex polygon home range area estimates in hectares calculated from each of the three methods from adult prairie voles in two enclosures at the Miami University Ecology Research Center.
We also compared the degree of home range overlap and social network association index between the dyads for all voles for which we could estimate home range size with VHF telemetry. To do so, we calculated home range overlap (based on the mcp home range estimates) for all dyads in the same enclosure using the VHF radio telemetry data using the gIntersection function of the package rgeos (version 0.3–28; Bivand and Rundel, 2018). We used these estimates to calculate the proportion of home range overlap for each dyad in our two enclosures. We then used a multiple regression quantitative assignment procedure (MRQAP: Krackhardt, 1988) in the R package asnipe to compare the matrix of the proportion of home range overlap to the social network matrix (only including voles for which we had home range overlap estimates) based on data from the RFID system in each enclosure separately. Specifically, we used an OLS network regression with the matrix of proportion of home range overlap as the predictor and the matrix of association indices based on social network analyses of data from the RFID system as the response variable. We used random social networks generated through network permutations on the group by individual matrix of the individuals included in this analysis (produced from the Gaussian mixture model run on the RFID data) as the random networks used in the MRQAP.
For our partner preference tests, we compared how much time the female spent in each part of the arena using a binomial generalized linear model with the proportion of time the female spent in each third of arena as the response variable (stranger, partner, or middle) and the corresponding chamber (stranger, partner, or middle) as the predictor variable. We included female ID as a fixed effect since the model would not converge with female ID as a random effect. We used a generalized linear model to compare the time that the female spent on the side with each male (as the response variable) to the association index of the focal female and that specific male from the social network generated from RFID data (as the predictor variable). We included trial number as a fixed effect (to control for repeated observations in each of the three sections of the arena, from the same trial) rather than a random effect because the model would not converge when trial number was included as a random effect. We standardized the predictor variable (scaled with a mean of 0 and variance of 1) to allow for easier comparison and interpretation of effect sizes (Schielzeth, 2010). Data are shown as mean ± 1 SD unless stated otherwise.
Ethical Note
All methods were approved by the Institutional Animal Care and Use Committee of Miami University (protocol number 979) because this was where data collection with live animals occurred.
Results
Partner Preference Test Compared to Social Network Metrics
The outcome of the partner pair preference tests supported the results from the social networks generated with the RFID tracking system. Overall female voles spent significantly more time in the area of the test arena with their potential partner (Figure 2), than their potential stranger (effect of the stranger side of the arena compared to the partner side, b = −3.46, z = −2.72, P = 0.0066) or the empty middle chamber (effect of middle compared to the partner side, b = −3.89, z = −2.73, P = 0.0063). Females did not spend more time with the potential stranger than the empty middle of the arena (effect of the stranger side of the arena compared to the middle, b = 0.44, z = 0.28, P = 0.78). Further, the amount of time the focal females spent with each male during the trial was related to the association index estimated from the social network using the RFID system data (effect of RFID association index, b = 899.56, t(17) = 3.68, P = 0.0019, Figure 3).
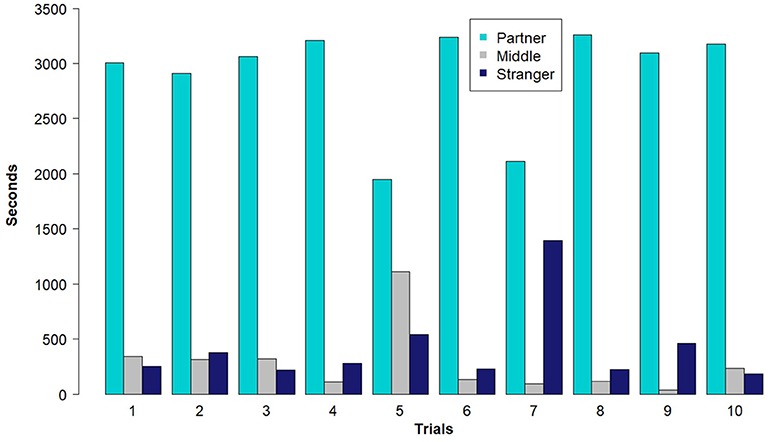
Figure 2. The number of seconds that female prairie voles (n = 10) in each trial spent on the three sides of the partner preference arena: middle of the arena, potential partner, and potential stranger (with a possible maximum of 3,600 s). Note that trial number refers to the order trials were conducted during the field season.
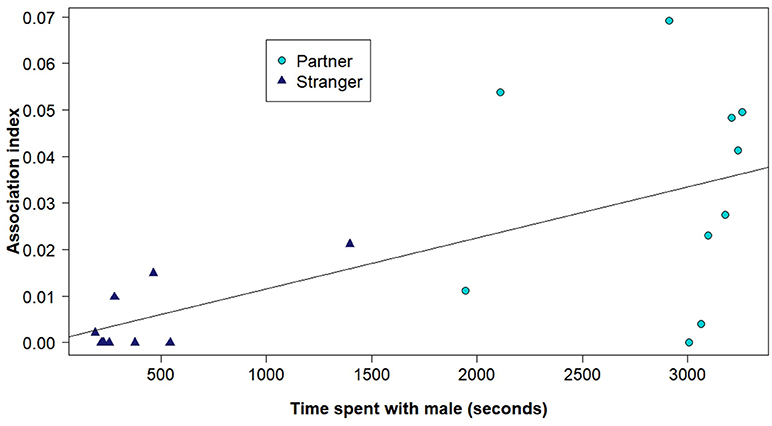
Figure 3. The time that the focal female spent with each male during the partner preference test (with a possible maximum of 3,600 s) and the corresponding association index of that pair based on the social network analyses using the RFID system. The points representing either the potential partners or the potential strangers from the partner preference trials are represented as different shapes and colors.
Degree of Home Range Overlap vs. Social Network Association Index
The proportion of home-range overlap between each dyad based on VHF radio telemetry data was a significant predictor of the strength of their association index in both enclosures (enclosure 1 OLS network regression: b = 0.017, P = 0.026, model adjusted R2 = 0.055, model residual standard error = 0.016, Figure 4; enclosure 2 OLS network regression: b = 0.035, P = 0.0056, model adjusted R2 = 0.42, model residual standard error = 0.0088, Figure 4). Overall, home range size estimates generated by the RFID system were significantly larger than estimates produced by live-trapping (effect of live-trapping method, b = −0.024, t = −3.43, P < 0.001, Figure 5) but not VHF telemetry (effect of telemetry method, b = 0.0049, t = 0.58, P = 0.56, Figure 5). Males had significantly larger home range sizes than females overall (effect of sex, b = 0.020, t = 2.54, P = 0.014, Figure 5) and there were no differences between enclosures (effect of enclosure, b = 0.012, t = 1.40, P = 0.17).
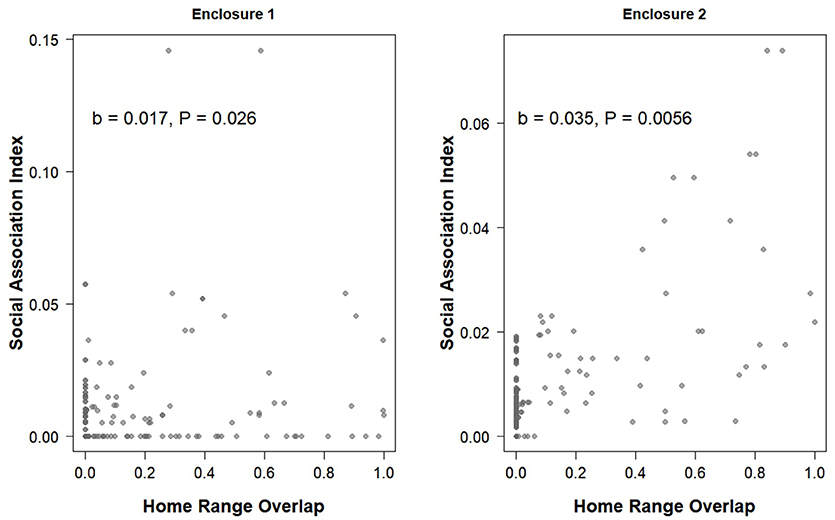
Figure 4. The home range overlap between each pair of prairie voles based on the minimum convex polygon from VHF radio telemetry data and the social association index for that pair (the strength of the association) from social network analyses generated using data from the RFID system. Note that the x-axes are the same on both panels but the y-axes are different.
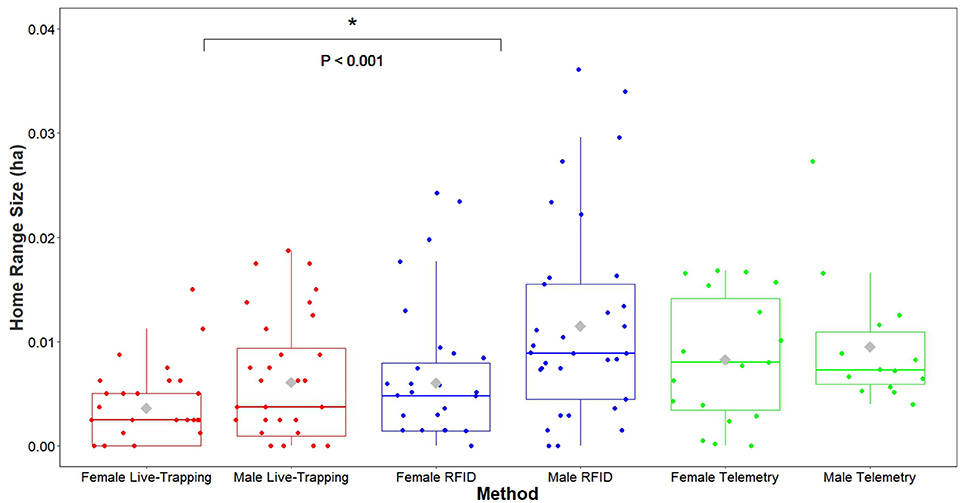
Figure 5. Home range size estimates (minimum convex polygons) of adult prairie voles estimated using our automated RFID system were significantly larger than those estimated with live-trapping. Females and males were combined in these models, so P-values refer to the difference in home range estimates between methods for all adult male and female voles. *P < 0.05.
Comparing Social Networks Generated by Live-Trapping and the RFID System
Overall, social networks generated from RFID and live-trapping data were similar, although the number of social connections varied between the two methods. On average, voles were recorded 12.78 times through live-trapping and 68.95 times through the Gaussian mixture model for the RFID system. The Gaussian mixture model produced 4,564 events from the raw RFID data. These events ranged from 0 s (i.e., voles recorded simultaneously) to 66,161 s with 655.2 ± 3,352.84 s being the average (or about 10 min). The strength of association indices between voles generated by live-trapping was positively related to the strength of association indices between voles from the social network generated by RFID data (OLS network regression: b = 0.13, P = 0.0007, model adjusted R2 = 0.105, model residual standard error = 0.0093, Figure 6). Therefore, the network of social interactions collected through live-trapping was a significant and positive predictor of the network of social interactions collected by the RFID system. The average unweighted degree (the number of unique conspecifics each individual is connected to in the network) was 8.88 when generated from RFID data while it was 4.52 when generated from live-trapping data. Individuals were similarly ranked in terms of unweighted degree in both types of networks (Spearman's rank correlation: P < 0.001, rho = 0.64, Figure 7), suggesting that individuals were recorded as highly social (i.e., more connections with different individuals) or less social by both the social networks generated from live-trapping and our RFID system.
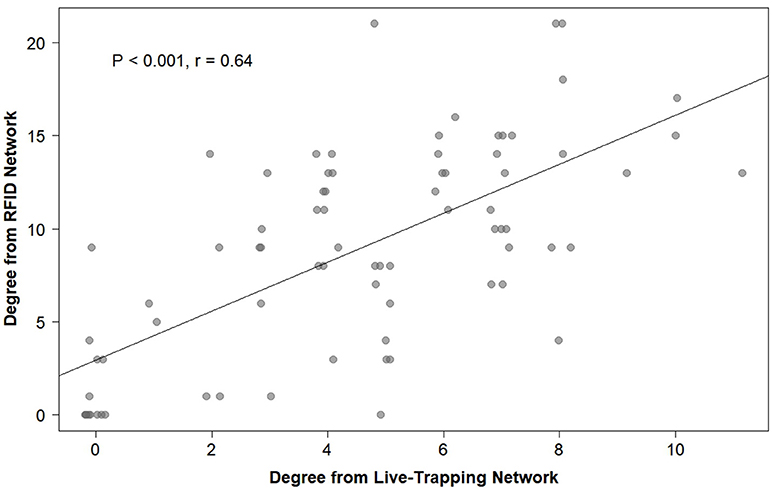
Figure 6. The relationship between each individual prairie vole's unweighted degree (the number of unique conspecifics each individual is connected to in either social network) generated from the social network using the live-trapping data and the unweighted degree generated from the social network using the automated RFID tracking system.
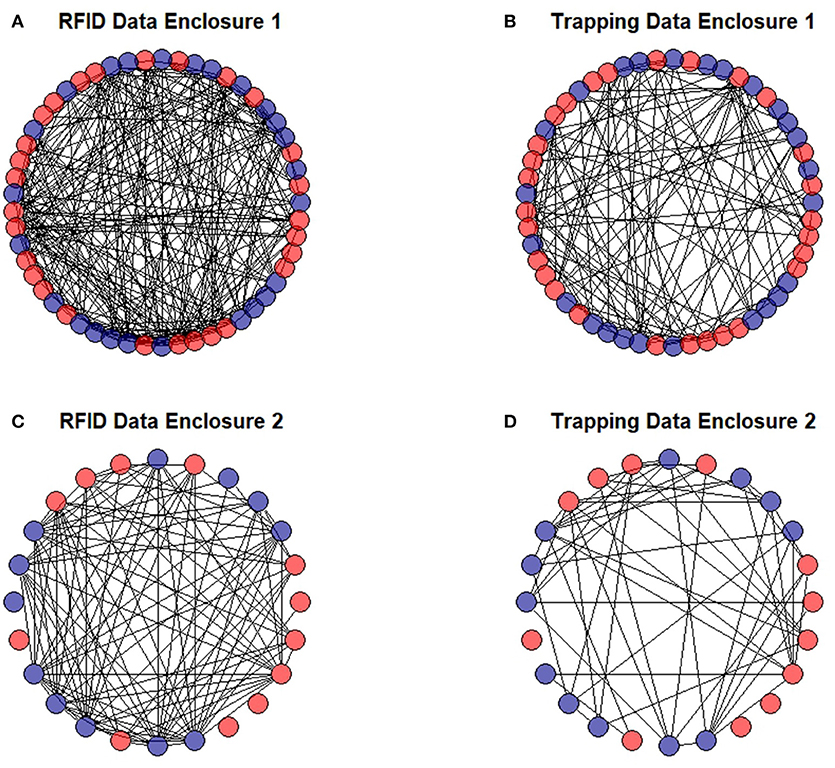
Figure 7. Social networks of prairie voles in two separate enclosures generated by our RFID system (A,C) and multiple capture live-trapping (B,D). Males are shown in blue and females are shown in red. Individuals with no connections to other individuals likely died soon after they were released into the enclosures.
Patterns of Vole Activity
The highest peak of activity (29.75% of all times voles were recorded) was around dusk (from 18:00 to 20:00), which supports the hypothesis that prairie voles are crepuscular and not nocturnal or diurnal, at least in this habitat during this time of year (Figure 8). However, there was not a similarly-high peak around dawn, as we would expect in a crepuscular species.
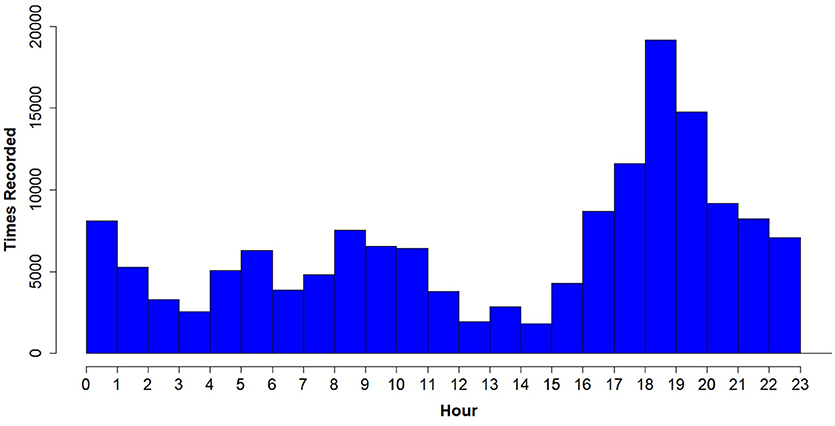
Figure 8. Total number of times PIT tagged prairie voles were recorded (hits) from all RFID antennas per hour of the day (days where live-trapping took place, which may bias activity results, were removed). Note the peak in readings in the evening, from hours 18:00 to 20:00 h.
Discussion
Can Social Network Metrics Describe the Socially Monogamous Behavior of Secretive Animals?
Our results provide some support that the male-female social network metrics calculated from spatial or temporal co-occurrence data provide insight into the social mating system of prairie voles. Male-female associations derived from the social network analyses using the automated RFID system were consistent with results from the partner preference tests. Specifically, female voles showed a preference for their potential partner and the time spent with each male within the trial was related to the strength of the dyad's association index estimated from the social networks using the RFID system. The degree of home range overlap for each dyad was also related to the strength of their association index derived from the social networks in both populations. Additionally, we found that social networks generated by live-trapping and the RFID system were statistically similar but that social networks generated by the RFID system provided more data per individual and thus allowed us to detect more associations.
Our results show that a small amount of spatiotemporal data generated from a RFID system can predict the behavioral preference of females in partner preference tests. This indicates that this method can be used in future studies to provide investigators with information about a female's social partner under natural conditions. This is an important result because few studies where social networks have been constructed using data from wild animals have attempted to use other methods to test the hypothesis that the spatial-temporal co-occurrence data are consistent with our knowledge of the social behavior of their study species.
Although the proportion of home range overlap was related to the strength of the association index in both populations (Figure 4), the R2-value was quite low in one of the enclosures. This could be due to the fact that this enclosure has more individuals with some level of home range overlap but an association index of zero (Figure 4). This was the enclosure with the higher population density at the start of the study, so voles in this enclosure may have been more space-limited resulting in home range overlap, but that they were preferentially choosing to associate with certain social partners over others, although we did not directly test this. Conversely, several dyads in both enclosures had non-zero association indices from the RFID data (suggesting some level of association) yet had no home range overlap based on telemetry data (Figure 4). Although the overall pattern we found in both enclosures suggests that association index and home range overlap are related, the presence of these particular data points suggests that home range overlap alone does not fully represent all social relationships or that VHF telemetry misses some relationships due to limitations on the amount of data that can be collected per individual and the fact that home ranges are calculated by constructing polygons, which may leave out some areas where associations may occur. As we have illustrated, RFID systems provide useful information about the spatial behavior of individuals by taking into account space use and movement during all hours of the day and night instead of many traditional methods that are only point samples during one part of the day or night. Thus, social network analyses can provide additional insight into inter-individual interactions in studies of social monogamy, in addition to traditional methods like spatial overlap. Further, after individuals have initially been PIT tagged, RFID systems eliminate most human disturbance when collecting these data.
Home Range Estimates
Automated tracking systems can also provide information about individual differences in social monogamy. Studying the spatial behavior of prairie voles in particular may show us which alternative mating tactic an individual is displaying (Ophir et al., 2008b; Blondel et al., 2016), either a “resident” (a paired individual that has established a territory) or a “wanderer” (an un-paired individual that does not have only one particular nest and tends to have a larger home range, Getz et al., 1987, 1990; Solomon and Jacquot, 2002). In fact, in a previous study, reproductive success of wandering males varied with the individual's space use (Ophir et al., 2008b). Home range overlap can also tell us whether or not there are multiple potential mates and potential rivals with overlapping territories (Ophir et al., 2008b).
Comparing Social Networks Generated by Live-Trapping and the RFID System
Social networks generated from two different methods of data collection detected similar social associations among individuals and similar levels of sociality, although our automated RFID system generated much larger data sets and detected more social associations than live-trapping. Although the maximum duration of an event for our Gaussian mixture model is quite long (66,161 s), the mean value (655.2 ± 3,352.84 s) is much lower than live-trapping (which lasts several hours to overnight), suggesting that the RFID data is recording associations at a much finer scale than live-trapping. As Krause et al. (2013) indicated, data collected by repeated live-captures or focal observations at set intervals may miss weaker associations or transitions in social associations due to the much longer intervals over which focal observations, live-trapping, or VHF telemetry are spread. Because we expect socially monogamous individuals to have one main, stronger relationship, missing these weaker associations may not detract from our general understanding of their social behavior. However, because non-socially monogamous individuals may have several weaker or more transient social associations, we may miss important social connections for these individuals without the continuous finer-scale data provided by RFID systems, making comparisons between individuals incomplete. Passive animal-tracking systems, like the RFID system used here, are more likely to detect these thanks to the generally much shorter interval between recordings and less restricted time over which data are recorded.
Our results comparing the social networks generated using live-trapping and the RFID system are similar to those of Farine (2015), who showed that social networks based on proximity data and interaction data may have some structural differences (like the number of social connections) but still detect a similar pattern of sociality when comparing between individuals (e.g., whether individuals had a high or low unweighted degree). Nomano et al. (2014) also found that social networks based on passive recording data were comparable to social networks generated from direct and video observations, suggesting that this system is also beneficial in species where direct observations are possible. Overall, the automated RFID tracking system we used provide a magnitude more data per individual. This is important because it has been suggested that at least 20 observations per individual are needed to increase reliability and stability in social network metrics (Farine and Strandburg-Peshkin, 2015; Hoppitt and Farine, 2018). Despite our intensive live-trapping sessions, only 19 of 77 (24.68%) voles crossed this threshold for live-trapping data, while 48 of 77 (62.34%) voles crossed this threshold when RFID data was used. This indicates that RFID systems provide more powerful assessments of social networks for animals that are difficult to observe.
Patterns of Vole Activity
The ability of the RFID system to record when animals are active may allow researchers to tailor their field methods to specific times for optimal data collection. For example, our results (Figure 8) indicate that the optimal time to set live traps is from 18:00 to 20:00, when voles are at peak activity. Alternatively, if the aim is to find burrows where voles sleep or interact with their offspring, 12:00–15:00 would be the optimal time because this is when voles were least active (Figure 8). Therefore, reviewing data from an RFID system early in a study can provide useful information for refining field methods.
Potential Limitations of Automated Tracking Systems
Automated tracking systems and social networks derived from such data may not be ideal for every species and question. Generating meaningful social network data requires recording a sufficient amount of social associations. This method will likely not provide a good representation of the social system if a sufficient amount of the population cannot be tagged. Further, the type of automated tracking system we used in this study will likely be less effective on larger animals with large home ranges given that the range at which an RFID antennae can detect a PIT tag is quite low. Although these types of systems can run on 12 V batteries that can be recharged using solar panels, applications to animals that live in “off grid” or in remote areas may also be limited. Therefore, researchers should consider if automated tracking systems will collect sufficient, representative data for each species and question.
Conclusions
Recording socially monogamous behavior is difficult in most wild animals. Social network analyses based on passive spatial and temporal co-occurrence data, like the data recorded by our automated RFID system, offers a useful way to record and quantify the degree of socially monogamous behavior individuals exhibit. We show that data recorded by RFID systems provide more data across all hours of the day with less human disturbance than traditional methods like live-trapping and VHF telemetry. We also show social network metrics describing indicators of social monogamy generated by this method are consistent with other methods of assessing social monogamy in prairie voles such as partner preference tests or the degree of home range overlap. Therefore, generating social networks with an automated RFID system can be useful to describe the social mating system of species that are difficult to observe.
Data Availability
Raw data for this manuscript is available from the senior author on FigShare at https://figshare.com/projects/Prairie_vole_social_network_data_for_Frontiers_in_Ecology_and_Evolution/56114
Author Contributions
BD designed the study and experiments, AS collected data under the guidance of BD, AS conducted statistical analyses, AS and BD wrote the manuscript with contributions from NS. All authors approved of the final manuscript.
Conflict of Interest Statement
The authors declare that the research was conducted in the absence of any commercial or financial relationships that could be construed as a potential conflict of interest.
Acknowledgments
We thank Miami University for allowing us to conduct the study at their Ecology Research Center. We also thank Jeremy Fruth, field manager at the Ecology Research Center, for his help with field work and Connor Lambert, James Lichter, Sage Sparks, Caleb Collins, Tony Leon, Meghan Charles, and Staci Barrow for their work on the project. Thanks to David Fisher for suggestions on statistical analyses, Brian Keane for helpful suggestions on the field research, and two reviewers for helpful comments. We would also like to thank the American Society of Mammalogists (Grant-in-Aid of Research to AS) and the University of Michigan (BD) for funding.
Supplementary Material
The Supplementary Material for this article can be found online at: https://www.frontiersin.org/articles/10.3389/fevo.2018.00178/full#supplementary-material
References
Bates, D., Mächler, M., Bolker, B., and Walker, S. (2015). Fitting linear mixed-effects models using lme4. J. Stat. Softw. 67, 1–48. doi: 10.18637/jss.v067.i01
Bivand, R., and Rundel, C. (2018). Rgeos: Interface to Geometry Engine–Open Source (‘geos'). R package version 0.3-28. Available online at: https://CRAN.R-project.org/package=rgeos.
Blondel, D. V., Wallace, G. N., Calderone, S., Gorinshteyn, M., St. Mary, C. M., and Phelps, S. M. (2016). Effects of population density on corticosterone levels of prairie voles in the field. Gen. Comp. Endocr. 225, 13–22. doi: 10.1016/j.ygcen.2015.09.002
Blumstein, D. T., and Daniel, J. C. (2007). Quantifying Behavior the JWatcher Way. Sunderland, MA: Sinauer.
Böhm, M., Palphramand, K., Newton-Cross, G., Hutchings, M. R., and White, P. (2008). Dynamic interactions among badgers: implications for sociality and disease transmission. J. Anim. Ecol. 77, 735–745. doi: 10.1111/j.1365-2656.2008.01377.x
Bonter, D., and Bridge, E. (2011). Applications of radio frequency identification (RFID) in ornithological research: a review. J. Field Ornithol. 82, 1–10. doi: 10.1111/j.1557-9263.2010.00302.x
Cairns, S. J., and Schwager, S. J. (1987). A comparison of association indexes. Anim. Behav. 35, 1454–1469. doi: 10.1016/S0003-3472(87)80018-0
Calenge, C. (2006). The package adehabitat for the R software: a tool for the analysis of space and habitat use by animals. Ecol. Model. 197, 516–519. doi: 10.1016/j.ecolmodel.2006.03.017
Calhoun, J. (1945). Diel activity rhythms of the rodents, Microtus ochrogaster and Sigmodon hispidus hispidus. Ecology 26, 251–273. doi: 10.2307/1932405
Carp, S. B., Rothwell, E. S., Bourdon, A., Freeman, S. M., Ferrer, E., and Bales, K. L. (2016). Development of a partner preference test that differentiates between established pair bonds and other relationships in socially monogamous titi monkeys (Callicebus cupreus). Am. J. Primatol. 78, 326–339. doi: 10.1002/ajp.22450
Castelli, F. R., Kelley, R. A., Keane, B., and Solomon, N. G. (2011). Female prairie voles exhibit social and sexual preferences for males with longer avpr1a microsatellite alleles. Anim. Behav. 82, 1117–1126. doi: 10.1016/j.anbehav.2011.08.006
Cochran, G. R., and Solomon, N. G. (2000). Effects of food supplementation on the social organization of prairie voles (Microtus ochrogaster). J. Mammal. 81, 746–757. doi: 10.1644/1545-1542(2000)081<0746:EOFSOT>2.3.CO;2
Csardi, G., and Nepusz, T. (2006). The igraph software package for complex network research, Int. J. Complex Syst. 1695, 1–9. Available Online at: http://igraph.sf.net
Curtis, J. T., Liu, Y., and Wang, Z. (2001). Lesions of the vomeronasal organ disrupt mating-induced pair bonding in female prairie voles (Microtus ochrogaster). Brain Res. 901, 167–174. doi: 10.1016/S0006-8993(01)02343-5
Dobson, F. S., Way, B. M., and Baudoin, C. (2010). Spatial dynamics and the evolution of social monogamy in mammals. Behav. Ecol. 21, 747–752. doi: 10.1093/beheco/arq048
Donaldson, Z. R., Spiegel, L., and Young, L. J. (2010). Central vasopressin V1a receptor activation is independently necessary for both partner preference formation and expression in socially monogamous male prairie voles. Behav. Neurosci. 124, 159–163. doi: 10.1037/a0018094
Farine, D. R. (2015). Proximity as a proxy for interactions: issues of scale in social network analysis. Anim. Behav. 104, e1–e5. doi: 10.1016/j.anbehav.2014.11.019
Farine, D. R. (2017). asnipe: Animal Social Network Inference and Permutations for Ecologists. R package version 1.1.4. Available online at: https://CRAN.R-project.org/package=asnipe
Farine, D. R., and Strandburg-Peshkin, A. (2015). Estimating uncertainty and reliability of social network data using Bayesian inference. R. Soc. Open. Sci. 2:150367. doi: 10.1098/rsos.150367
Farine, D. R., and Whitehead, H. (2015). Constructing, conducting and interpreting animal social network analysis. J. Anim. Ecol. 84, 1144–1163. doi: 10.1111/1365-2656.12418
Getz, L. L., Hofmann, J. E., and Carter, C. S. (1987). Mating system and population fluctuations of the prairie vole, Microtus ochrogaster. Integr. Compar. Biol. 27, 909–920. doi: 10.1093/icb/27.3.909
Getz, L. L., Hofmann, J. E., McGuire, B., and Dolan, T. W. III. (2001). Twenty-five years of population fluctuations of Microtus ochrogaster and M. pennsylvanicus in three habitats in east-central Illinois. J. Mammal. 82, 22–34. doi: 10.1644/1545-1542(2001)082<0022:TFYOPF>2.0.CO;2
Getz, L. L., McGuire, B., Hofmann, J., Pizzuto, T., and Frase, B. (1990). “Social organization and mating system of the prairie vole, Microtus ochrogaster,” in Social Systems and Population Cycles in Voles, eds R. H. Tamarin, R. S. Ostfeld, S. R. Pugh, and G. Bujalska (Birkhäuser; Basel: Advances in Life Sciences), 69–80.
Getz, L. L., McGuire, B., Pizzuto, T., Hofmann, J. E., and Frase, B. (1993). Social organization of the prairie vole (Microtus ochrogaster). J. Mammal. 74, 44–58. doi: 10.2307/1381904
Godsall, B., Coulson, T., Malo, A. F., and Montgomery, I. (2014). From physiology to space use: energy reserves and androgenization explain home-range size variation in a woodland rodent. J. Anim. Ecol. 83, 126–135. doi: 10.1111/1365-2656.12116
Hammock, E. A., and Young, L. J. (2002). Variation in the vasopressin V1a receptor promoter and expression: implications for inter- and intraspecific variation in social behaviour. Eur. J. Neurosci. 16, 399–402. doi: 10.1046/j.1460-9568.2002.02083.x
Harper, S. J., and Batzli, G. O. (1996). Monitoring use of runways by voles with passive integrated transponders. J. Mammal. 77, 364–369.
Hofmann, J. E., Getz, L. L., and Gavish, L. (1984). Home range overlap and nest cohabitation of male and female prairie voles. Am. Midland Natur. 112, 314–319.
Hoppitt, W., and Farine, D. R. (2018). Association indices for quantifying social relationships: how to deal with missing observations of individuals or groups. Anim. Behav. 136, 227–238. doi: 10.1016/j.anbehav.2017.08.029
Klug, H. (2018). Why monogamy? A review of potential ultimate drivers. Front. Ecol. Evol. 6:30. doi: 10.3389/fevo.2018.00030
Komers, P. E., and Brotherton, P. N. (1997). Female space use is the best predictor of monogamy in mammals. Proc. R. Soc. B 264, 1261–1270. doi: 10.1098/rspb.1997.0174
Krackhardt, D. (1988). Predicting with networks: nonparametric multiple regression analysis of dyadic data. Soc. Netw. 10, 359–381. doi: 10.1016/0378-8733(88)90004-4
Krause, J., Krause, S., Arlinghaus, R., Psorakis, I., Roberts, S., and Rutz, C. (2013). Reality mining of animal social systems. Trends Ecol. Evol. 28, 541–551. doi: 10.1016/j.tree.2013.06.002
Kuznetsova, A., Brockhoff, P. B., and Christensen, R. H. B. (2017). lmerTest package: Tests in linear mixed effects models. J. Stat. Softw. 82, 1–26. doi: 10.18637/jss.v082.i13
Lambert, C. (2018). ERα Expression and Monogamy in Prairie Voles: An Experimental Field Study. master's thesis, Miami University (Oxford, OH).
Leese, J. M. (2012). Sex differences in the function of pair bonding in the monogamous convict cichlid. Anim. Behav. 83, 1187–1193. doi: 10.1016/j.anbehav.2012.02.009
Lemen, C. A., and Freeman, P. W. (1985). Tracking mammals with fluorescent pigments: a new technique. J. Mammal. 66, 134–136. doi: 10.2307/1380966
Leu, S. T., Bashford, J., Kappeler, P. M., and Bull, C. M. (2010). Association networks reveal social organization in the sleepy lizard. Anim. Behav. 79, 217–225. doi: 10.1016/j.anbehav.2009.11.002
Lucia, K. E., Keane, B., Hayes, L. D., Lin, Y. K., Schaefer, R. L., and Solomon, N. G. (2008). Philopatry in prairie voles: an evaluation of the habitat saturation hypothesis. Behav. Ecol. 19, 774–783. doi: 10.1093/beheco/arn028
Lukas, D., and Clutton-Brock, T. H. (2013). The evolution of social monogamy in mammals. Science 341, 526–530. doi: 10.1126/science.1238677
Madison, D. M. (1985). “Activity rhythms and spacing In Tamarin,” in Biology of New World Microtus, ed R. H. Tamarin (Stillwater, OK: American Society of Mammalogists), 373–419.
Newey, S., Allison, P., Thirgood, S. J, Smith, A. A., and Graham, I. M. (2009). Using PIT-tag technology to target supplementary feeding studies. Wildlife Biol. 15, 405–411. doi: 10.2981/08-083
Nomano, F., Browning, L., Nakagawa, S., Griffith, S., and Russell, A. (2014). Validation of an automated data collection method for quantifying social networks in collective behaviours. Behav. Ecol. Sociobiol. 68, 1379–1391. doi: 10.1007/s00265-014-1757-0
Ophir, A. G., Phelps, S. M., Sorin, A. B., and Wolff, J. O. (2008a). Social but not genetic monogamy is associated with greater breeding success in prairie voles. Anim. Behav. 75, 1143–1154. doi: 10.1016/j.anbehav.2007.09.022
Ophir, A. G., Wolff, J. O., and Phelps, S. M. (2008b). Variation in neural V1aR predicts sexual fidelity and space use among prairie voles in semi-natural settings. Proc. Natl. Acad. Sci. U.S.A. 105, 1249–1254. doi: 10.1073/pnas.0709116105
Opie, C., Atkinson, Q. D., Dunbar, R. I. M., and Shultz, S. (2013). Male infanticide leads to social monogamy in primates. Proc. Natl. Acad. Sci. U.S.A. 110, 13328?13332. doi: 10.1073/pnas.1307903110
Psorakis, I., Roberts, S. J., Rezek, I., and Sheldon, B. C. (2012). Inferring social network structure in ecological systems from spatio-temporal data streams. J. R. Soc. Interface 9, 3055–3066. doi: 10.1098/rsif.2012.0223
R Core Team (2017). R: A Language and Environment for Statistical Computing. Vienna: R Foundation for Statistical Computing. Available online at: https://www.R-project.org/.
Rehmeier, R., Kaufman, G., and Kaufman, D. (2006). An automatic activity-monitoring system for small mammals under natural conditions. J. Mammal. 87, 628–634. doi: 10.1644/05-MAMM-A-220R2.1
Reichard, U. H. (2003). “Monogamy: past and present,” Monogamy: Mating Strategies and Partnerships in Birds, Humans and Other Mammals, in ed U. H. Reichard and C. Boesch (Cambridge, UK: Cambridge University Press), 3–25.
Ribble, D. O., and Salvioni, M. (1990). Social organization and nest co-occupancy in Peromyscus californicus, a monogamous rodent. Behav. Ecol. Sociobiol. 26, 9–15. doi: 10.1007/BF00174020
Richmond, M., and Conaway, C. H. (1969). Management, breeding, and reproductive performance of the vole, Microtus ochrogaster, in a laboratory colony. Lab. Anim. Care 19, 80–87.
Salo, A. L., and Dewsbury, D. A. (1995). Three experiments on mate choice in meadow voles (Microtus pennsylvanicus). J. Comp. Psychol. 109, 42–46.
Schielzeth, H. (2010). Simple means to improve the interpretability of regression coefficients. Methods Ecol. Evol. 1, 103–113. doi: 10.1111/j.2041-210X.2010.00012.x
Smith, J. E., Gamboa, D. A., Spencer, J. M., Travenick, S. J., Ortiz, C. A., Hunter, R. D., et al. (2018). Split between two worlds: automated sensing reveals links between above- and belowground social networks in a free-living mammal. Philos. Trans. R. Soc. Lond. B Biol. Sci. 373:20170249. doi: 10.1098/rstb.2017.0249
Soanes, K., Vesk, P. A., and van der Ree, R. (2015). Monitoring the use of road-crossing structures by arboreal marsupials: insights gained from motion-triggered cameras and passive integrated transponder (PIT) tags. Wildlife Res. 42, 241–256. doi: 10.1071/WR14067
Solomon, N. G., and Jacquot, J. J. (2002). Characteristics of resident and wandering prairie voles, Microtus ochrogaster. Can. J. Zool. 80, 951–955. doi: 10.1139/z02-053
Solomon, N. G., Richmond, A. R., Harding, P. A., Fries, A., Jacquemin, S., Schaefer, R. L., et al. (2009). Polymorphism at the avpr1a locus in male prairie voles correlated with genetic but not social monogamy in field populations. Mol. Ecol. 18, 4680–4695. doi: 10.1111/j.1365-294X.2009.04361.x
Streatfeild, C. A., Mabry, K. E., Keane, B., Crist, T. O., and Solomon, N. G. (2011). Intraspecific variability in the social and genetic mating systems of prairie voles, Microtus ochrogaster. Anim. Behav. 82, 1387–1398. doi: 10.1016/j.anbehav.2011.09.023
Tecot, S. R., Singletary, B., and Eadie, E. (2016). Why “monogamy” isn't good enough. Am. J. Primatol. 78, 340–354. doi: 10.1002/ajp.22412
Wasserman, S., and Faust, K. (1994). Social Network Analysis: Methods and Applications. New York, NY: Cambridge University Press.
Wey, T., Blumstein, D. T., Shen, W., and Jordán, F. (2008). Social network analysis of animal behaviour: a promising tool for the study of sociality. Anim. Behav. 75, 333–344. doi: 10.1016/j.anbehav.2007.06.020
Whitehead, H. (2008). Analyzing Animal Societies: Quantitative Methods for Vertebrate Social Analysis. Chicago, IL: University of Chicago Press.
Williams, J. R., Catania, K. C., and Carter, C. S. (1992). Development of partner preferences in female prairie voles (Microtus ochrogaster): the role of social and sexual experience. Horm. Behav. 26, 339–349. doi: 10.1016/0018-506X(92)90004-F
Wittenberger, J. F., and Tilson, R. L. (1980). The evolution of monogamy: hypotheses and evidence. Ann. Rev. Ecol. Syst. 11, 197–232.
Keywords: association index, social monogamy, social network analyses, RFID system, social behavior, vole
Citation: Sabol AC, Solomon NG and Dantzer B (2018) How to Study Socially Monogamous Behavior in Secretive Animals? Using Social Network Analyses and Automated Tracking Systems to Study the Social Behavior of Prairie Voles. Front. Ecol. Evol. 6:178. doi: 10.3389/fevo.2018.00178
Received: 30 June 2018; Accepted: 16 October 2018;
Published: 09 November 2018.
Edited by:
Ann Valerie Hedrick, University of California, Davis, United StatesReviewed by:
Inger Suzanne Prange, Ohio University, United StatesDaizaburo Shizuka, University of Nebraska-Lincoln, United States
Copyright © 2018 Sabol, Solomon and Dantzer. This is an open-access article distributed under the terms of the Creative Commons Attribution License (CC BY). The use, distribution or reproduction in other forums is permitted, provided the original author(s) and the copyright owner(s) are credited and that the original publication in this journal is cited, in accordance with accepted academic practice. No use, distribution or reproduction is permitted which does not comply with these terms.
*Correspondence: Ben Dantzer, dantzer@umich.edu