- 1Florida Museum of Natural History, University of Florida, Gainesville, FL, United States
- 2Norwegian Polar Institute, Fram Centre, Tromsø, Norway
- 3College of Science and Engineering, Flinders University, Adelaide, SA, Australia
- 4CSIRO National Research Collections Australia, Hobart, TAS, Australia
- 5KwaZulu-Natal Sharks Board, Umhlanga Rocks, South Africa
- 6School of Life Sciences, University of KwaZulu-Natal, Durban, South Africa
- 7Department of Conservation, Auckland, New Zealand
- 8Oceanographic Research Institute, University of KwaZulu-Natal, Durban, South Africa
- 9National Institute of Water and Atmospheric Research, Wellington, New Zealand
- 10South Australia Research and Development Institute – Aquatic Sciences, Henley Beach, SA, Australia
- 11Southwest Fisheries Science Center, National Marine Fisheries Service, La Jolla, CA, United States
- 12Gulf Elasmo Project, Dubai, United Arab Emirates
- 13Stick Figure Fish Illustration, Peregian Beach, QLD, Australia
- 14Centro de Investigação em Biodiversidade e Recursos Genéticos (CIBIO/InBIO), Universidade do Porto, Porto, Portugal
- 15Centro Tecnológico del Mar-Fundación CETMAR, Vigo, Spain
- 16Pepperell Research and Consulting Pty Ltd., Noosaville DC, QLD, Australia
In this paper we combine analyses of satellite telemetry and molecular data to investigate spatial connectivity and genetic structure among populations of shortfin mako (Isurus oxyrinchus) in and around Australian waters, where this species is taken in recreational and commercial fisheries. Mitochondrial DNA data suggest matrilineal substructure across hemispheres, while nuclear DNA data indicate shortfin mako may constitute a globally panmictic population. There was generally high genetic connectivity within Australian waters. Assessing genetic connectivity across the Indian Ocean basin, as well as the extent that shortfin mako exhibit sex biases in dispersal patterns would benefit from future improved sampling of adult size classes, particularly of individuals from the eastern Indian Ocean. Telemetry data indicated that Australasian mako are indeed highly migratory and frequently make long-distance movements. However, individuals also exhibit fidelity to relatively small geographic areas for extended periods. Together these patterns suggest that shortfin mako populations may be genetically homogenous across large geographical areas as a consequence of few reproductively active migrants, although spatial partitioning exists. Given that connectivity appears to occur at different scales, management at both the national and regional levels seems most appropriate.
Introduction
Implementing practical and effective management for highly migratory species (HMS) of pelagic sharks is challenging because they have vast ranges that are often spatiotemporally dynamic. For example, some HMS of pelagic sharks move among favorable foraging, breeding, and pupping grounds, sometimes using specific migration pathways (Hueter et al., 2005; Kinney and Simpfendorfer, 2009; Chapman et al., 2015). Recognizing such movement patterns is important for devising suitably scaled management plans, particularly when a species range spans multiple, or extends beyond, national jurisdictions (Worm and Vanderzwaag, 2007). However, propensity to migrate is multifaceted and different movement types variously influence population persistence. This means that management plans for HMS must consider more than simply their mobility. For instance, the extent of genetic and spatial connectivity among regions are each relevant for management, but are not always positively correlated (Palumbi, 2003). Some migration patterns are driven by prey availability or environmental change and are unrelated to gene flow (Heupel et al., 2003; Campana et al., 2011; Hueter et al., 2013; Doherty et al., 2017). Habitat preference, philopatric behavior or physical and ecological barriers to dispersal can promote genetic structure in species with high mobility (Schultz et al., 2008; Portnoy et al., 2010; Daly-Engel et al., 2012; Feldheim et al., 2014; Sandoval-Castillo and Beheregaray, 2015; Corrigan et al., 2016; Bester-Van Der Merwe et al., 2017; Guttridge et al., 2017). Conversely, regions may be genetically homogenized by a few reproductively active migrants despite considerable spatial partitioning (Waples, 1998; Gagnaire et al., 2015).
Combining analyses of satellite telemetry and molecular data can provide information about both spatial connectivity and genetic linkages among populations of HMS of pelagic sharks. Satellite telemetry methods are particularly useful for determining mobility and for identifying migration pathways or habitat preferences (Block et al., 2011). Complementing this with population genetic analysis can inform about connectivity via reproductively effective migration. The combination of these approaches allows assessments at a range of spatiotemporal scales, informing about contemporary population dynamics and dispersal patterns as well as connectivity that is relevant for long-term population fitness (Frankham et al., 2010; Gagnaire et al., 2015).
We employed both satellite telemetry and molecular approaches to study spatial connectivity and population genetic structure in shortfin mako Isurus oxyrinchus Rafinesque 1810 (Rogers et al., 2015a). Shortfin mako exhibit red myotomal endothermy and are able to maintain their body temperature above ambient levels. This adaptation is thought to allow this species to occupy a broad thermal niche, sustain high swimming speeds, and ultimately be very highly migratory (Carey, 1973; Dickson and Graham, 2004). Shortfin mako are oceanic, coastal, and pelagic. They are an economically lucrative fisheries resource, taken as bycatch and targeted both recreationally and commercially worldwide. Declines in relative abundance of shortfin mako have been recorded in the Mediterranean and the northern Atlantic Ocean (Chang and Liu, 2009). This prompted their listing on the Convention on the Conservation of Migratory Species of Wild Animals Appendix II (Dulvy et al., 2008) and as globally Vulnerable according to the International Union for Conservation of Nature Red List of Threatened Species criteria (Cailliet et al., 2009). Recent stock assessment confirmed that the North Atlantic stock remains overfished and that current regulations will neither promote future growth, nor prevent further decline (Sims et al., 2018).
Available fisheries and tracking data suggest that shortfin mako combine phases of fidelity in neritic regions with characteristic broad-scale, highly directional, transitory movements across both neritic, and oceanic environments. It also appears that warm water masses, such as thermal equatorial fronts, act as a dispersal barrier resulting in Northern and Southern Hemisphere stock differentiation (Holts and Bedford, 1993; Abascal et al., 2011; Block et al., 2011; Musyl et al., 2011; Sippel et al., 2011; Rogers et al., 2015a,b; Holdsworth and Saul, 2017). Consistent with these patterns, previous genetic studies have shown cross-equatorial matrilineal sub-structure (Heist et al., 1996; Schrey and Heist, 2003; Taguchi et al., 2015). Significant matrilineal sub-structure between the southeastern and southwestern Pacific has also been reported (Michaud et al., 2011; Taguchi et al., 2015). Nuclear data, on the other hand, indicate that shortfin mako are globally panmictic, possibly as a result of male-mediated gene flow (Schrey and Heist, 2003; Taguchi et al., 2015). While these studies have provided important insights regarding the movement ecology of shortfin mako, more information is needed to determine the appropriate spatial scale at which to manage this species. Specifically, the Southern Hemisphere has previously been sparsely sampled and the extent of connectivity among locations within this region is poorly understood. This region thus forms the geographical focus of the current study, particularly in and around Australian waters, where shortfin mako are regularly targeted by commercial and recreational fishers and proposed protection measures have been the subject of substantial conjecture.
Shortfin mako were previously listed in Australia under the Environment Protection and Biodiversity Conservation Act (EPBC Act 1999). Uncertainty regarding regional connectivity within Australian waters, and among Australian stocks and declining populations in the Northern Hemisphere, ultimately resulted in this listing being amended to allow recreational fishing for shortfin mako to continue. Developing appropriately scaled management strategies for shortfin mako in the Southern Hemisphere therefore requires further information regarding spatial and genetic connectivity within Australian waters, and among neighboring regions. We thus focused satellite tracking effort and intensified the geographic coverage of sampling for molecular analyses within these areas (Rogers et al., 2015a). Our specific aims were to (1) use genetic data to assess the extent of regional population genetic structure within Australian waters, and between Australian waters and neighboring regions within the Southern Hemisphere, and (2) compare the spatial scale of genetic connectivity with movement and dispersal patterns determined using satellite telemetry data collected over multiple years.
Materials and Methods
Satellite Telemetry
Thirteen dorsal-fin mounted satellite tags were deployed in continental shelf and slope waters of southern Australia between 2008 and 2013. These included position-only Sirtrack KiwiSat 202 and K2F161A tags, Wildlife Computers™ Smart Position or Temperature (SPOT) tags, and data-collecting Argos SPLASH and Mk10A tags. Sirtrack 202 and WC SPOT tags were programmed to transmit daily signals, whereas the SPLASH and Sirtrack K2F161A tags were duty-cycled to transmit at a 2-day frequency to optimize battery life. Capture and satellite tagging techniques are described in Rogers et al. (2015b). Shark total length, sex, and tag deployment locations were recorded (Table S1).
Satellite tags transmitted signals to the low polar orbiting environmental satellite network receiver stations, which were forwarded to Argos centers in France and the USA. Argos position estimates were accessed using Telnet and Tera Term Pro software, downloaded in seven location classes ranging from the highest to the lowest quality between 3, 2, 1, 0, A, B, and Z with predicted accuracies of 3 = < 250 m, 2 = 250–500 m, 1 = 500–1,500 m, classes 0 – B = >1,500 m, and Z = no position (http://www.argos-system.org). Raw data were mapped in MapInfo v. 11.5. to remove positions on land. Argos data were filtered by estimating locations using a Kalman filter under a continuous-time state-space framework using the (C)orrelated (RA)andom (W)alk (L)ibrary “CRAWL” package in R v. 2.15.2 (Johnson et al., 2008; R Core Team, 2013). Locations were interpolated along each filtered track to reduce sampling bias due to irregular transmission of Argos location data. To establish a set of spatial scale-based movement parameters, we estimated mean rate of movement (ROM) per day, minimum cumulative distance traveled and distal displacement distances (linear distance between tagging location and most distant location) for each individual (Table S1).
Population Genetics—Sample and Data Collection
Tissue samples were obtained from 389 shortfin mako collected opportunistically from recreational and commercial fisheries catches or through collaboration with international research organizations. Samples were collected from six regions throughout the Southern Hemisphere (N = 275: Indo-Pacific, eastern Australia, southern Australia, Western Australia, New Zealand, and South Africa; Figure 1). Two regions from the Northern Hemisphere (N = 114: Northern Atlantic and northern Indian Oceans) were also sampled to assess trans-equatorial connectivity. Individuals were sampled at several locations within these broad regions to ensure that fine-scale geographic structure would be detected, if present (Figure 1). Tissue was preserved in either 95% ethanol or salt-saturated 20% dimethyl sulfoxide, and genomic DNA was extracted using a modified salting out protocol (Sunnucks and Hales, 1996).
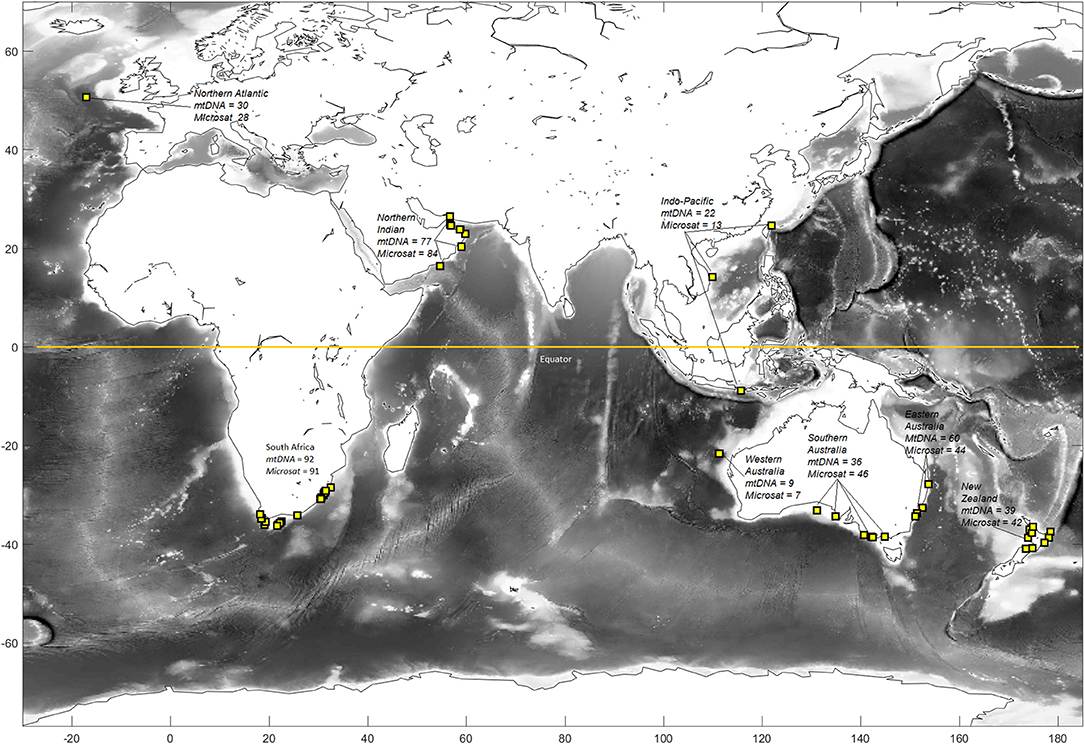
Figure 1. Sampling locations and sample sizes of shortfin mako for genetic analyses. Regions include the Northern Atlantic, South Africa, Northern Indian, Western Australia, Indo-Pacific, southern and eastern Australia, and New Zealand. Western and southern Australia were grouped to comprise southwestern Australasia and the Indo-Pacific and eastern Australia were grouped to comprise eastern Australia for some analyses.
The mitochondrial control region and portions of the flanking tRNAs (~1142 bp) were amplified by the Polymerase Chain Reaction (PCR) using primers Shark tPheR 5′-TYTCATCTTAGCATCTTCAGTGC-3′ and Shark tProF 5′-AGCCAAGATTCTGCCTAAACTG-3′. Reactions were conducted in 25 μL volumes comprised of 15–30 ng template DNA, 2.5 mM MgCl2, 1 × MangoTaq™ reaction buffer (Bioline, Taunton USA), 0.25 mM dNTPs, 30 pmol forward and reverse primers and 1.25 U MangoTaq™ DNA polymerase (Bioline, Taunton USA). PCR cycling consisted of initial denaturation at 94°C, followed by “touchdown” cycling of 30 s denaturation at 94°C, 45 s annealing, and 1 min extension at 72°C. Annealing temperatures began at 59°C and decreased by 2°C at each touchdown, stabilizing at 51°C for 30 cycles. Amplified products were purified using ExoSAP-IT (Affymetrix USB® Products, Affymetrix, Inc., Cleveland USA), according to the manufacturer's protocol. Sanger sequencing was performed bi-directionally using internal primers mako405F 5′-GCCCGCTAGTTCCCTTTAATG-3′ and mako572R 5′-CCTTTCAGTTATGGTCAACTTGACAATC-3′, and BigDye® Terminator chemistry on an ABI 3730xl genetic analyzer (Applied Biosystems®, Life Technologies, Grand Island USA). DNA sequences were edited and aligned using Geneious® Pro v. 6.1.7 (Biomatters Ltd, Auckland New Zealand http://www.geneious.com). Sequences were cropped to 791bp for downstream analyses due to variability in sequence quality on either end.
Ten microsatellite loci were amplified using primers Iox-12 and Iox-30 (Schrey and Heist, 2002) Iox-M01, Iox-M110, Iox-M115, Iox-M192, Iox-M36, Iox-M59, Iox-B3, and Iox-D123 (via GenBank accession numbers KJ454433, KJ454434, KJ454435, KJ454436, KJ454437, KJ454438, KJ454439, KJ454440, respectively). Forward primers were tailed with a fluorescently labeled M13 tag (Schuelke, 2000). Reactions were conducted in 5 μL volumes comprising 15–30 ng template DNA, 3 mM MgCl2, 1 × MangoTaq™ reaction buffer (Bioline, Taunton USA), 0.1 mM each dNTP, 0.1 pmol M13 tailed forward primer, 0.3 pmol reverse primer, 0.1 pmol fluorescently labeled M13 primer, 0.5 μg bovine serum albumin, and 0.25 U MangoTaq™ DNA polymerase (Bioline, Taunton USA). PCR cycling consisted of initial denaturation at 94°C, followed by “touchdown” cycling of 30 s denaturation at 94°C, 45 s annealing, and 1 min extension at 72°C. Annealing temperature began at 65°C and decreased by 2°C at each touchdown, stabilizing at 57°C for 30 cycles. Products were separated on an ABI 3730xl genetic analyzer (Applied Biosystems®, Life Technologies, Grand Island USA). Reference samples for each locus were included in all PCR programs and during capillary separation of fragments to ensure consistent genotype calling. Microsatellite alleles were visually inspected, binned, and sized according to the GeneScan™ 500 LIZ™ size standard (Applied Biosystems®, Life Technologies, Grand Island USA) using the Third Order Least Squares algorithm in the microsatellite plugin for Geneious® Pro v6.1.7 (Biomatters Ltd, Auckland New Zealand. http://www.geneious.com). Genotypes were checked for signatures of possible scoring errors due to null alleles, short allele dominance, and stutter peaks using Microchecker v. 2.2.3 (Van Oosterhout et al., 2004).
Population Genetics—Genetic Diversity and Structure
To avoid biases associated with limited sampling, samples from Western Australia and southern Australia, the Indo-Pacific and eastern Australia, were pooled for all frequency-based analyses of both mitochondrial and microsatellite data. Analysis of molecular variance (AMOVA) did not indicate any significant difference among these sampling locations, confirming the validity of this pooling scheme.
Mitochondrial DNA sequence variation and the extent of population differentiation were explored in Arlequin v. 3.5.1.2 (Excoffier and Lischer, 2010). Number of haplotypes, haplotypic, and nucleotide diversities were calculated assuming the Jukes and Cantor model of DNA substitution (Jukes and Cantor, 1969). An exact test of population differentiation was performed and population pairwise estimates of the parameters FST and ΦST were calculated. Significance was assessed via permutation (100,000 permutations) and interpreted following non-parametric Bonferroni correction (Rice, 1989). Hierarchical AMOVA was conducted partitioning total variance into within population, among population, and among regional covariance components (Cockerham, 1973) and testing for significance via permutation (10,100 permutations). A median-joining network (Bandelt et al., 1999) was constructed in Network v. 5.0 (Fluxus Technology Ltd) and illustrated in Network Publisher v. 2.0.0.1 (Fluxus Technology Ltd). Epsilon was set to 0 and hyper-variable sites were down weighted.
Microsatellite diversity was characterized using GenAlEx v. 6.5 (Peakall and Smouse, 2012) by calculating allele frequencies, number of alleles, effective number of alleles, and average observed, expected and unbiased expected heterozygosities per sampling location. Allelic richness was calculated in FSTAT v. 2.9.3.2 (Goudet, 2001). Genepop v. 4.2 (Raymond and Rousset, 1995) was used to assess whether the data conformed to expectations under Hardy-Weinberg and linkage equilibrium models. Bonferroni corrections for multiple comparisons were applied prior to interpretation.
Powsim v. 4.1 (Ryman and Palm, 2006) was used to determine the alpha error and statistical power with which significant genetic differentiation could be determined using our microsatellite dataset. Datasets were simulated with the same sample size, number of loci, and average allele frequencies as our observed and populations allowed to drift for a user-specified number of generations in order to attain a pre-defined level of differentiation (FST = 0.0005 to 0.05, 500 replicates per value). Statistical power was determined as the proportion of simulations for which Fisher's exact and Chi-square tests showed significant differentiation. Statistical α (type I) error was assessed by calculating the probability of rejecting H0 when it is true for simulations omitting the drift step (i.e., FST = 0).
Population differentiation was investigated in GenAlEx v. 6.5. Pairwise fixation indices, Nei's GST, and Hedrick's GST′′, were calculated following Meirmans and Hedrick (2011). Allelic differentiation, DEST, was calculated following Jost (2008). Arlequin v. 3.5.1.2 was used to conduct an AMOVA of microsatellite data, partitioning total variance into within population, among population, and among regional covariance components. Significance was assessed via permutation (10,100 permutations).
Model-based clustering of genotypic data was conducted using Structure v. 2.3.4 (Pritchard et al., 2000). Since mobility is high in shortfin mako, allele frequencies were assumed to be similar across populations (Falush et al., 2003) and individuals were assigned using the admixture model of ancestry. Prior information regarding sampling location was allowed to inform ancestry in order to assist clustering (Hubisz et al., 2009). Inference was conducted over one million iterations (100,000 burn-in). Five independent runs were performed for each value of K, which varied from one to the number of sampled localities. Priors for the average and standard deviation of F (drift within populations) were set to 0.01 and 0.05, respectively, following Falush et al. (2003). A uniform prior (0, 10) on α (the parameter shaping the distribution of admixture proportion) was assumed. Following Evanno et al. (2005), ΔK (the second order rate of change of the log probability of the data given K (Ln P(X|K)) was calculated using Structure Harvester v. 0.6.93 (Earl and Vonholdt, 2012) and used to guide inference regarding the number of populations. Replicate clustering analyses were aligned using CLUMPP v. 1.1.2 (Jakobsson and Rosenberg, 2007) and visualized using distruct v. 1.1 (Rosenberg, 2004).
Population Genetics—Sex-Biased Movement
Analyses of sex-biased dispersal were conducted on a reduced dataset consisting only of individuals for which sex data were available (152 females (F) and 151 males (M) total; northern Indian 41 F: 40 M, South Africa 34 F: 57 M, eastern Australia 28 F: 20 M, southern Australia 21 F: 22 M, and New Zealand 28 F: 12 M).
The likelihood that an individual originates from its sampled location was calculated following Paetkau et al. (1995) using GeneClass2 v.2.0 (Piry et al., 2004). Log transformed likelihood values were corrected for population effects following Favre et al. (1997) resulting in corrected Assignment Indices (AIc) that averaged zero per population and whereby negative values indicate lower than average probability of being born locally (migrants). The distributions of AIc were calculated and compared for males and females, with the expectation that the more dispersive sex would show a more negative frequency distribution (Favre et al., 1997; Mossman and Waser, 1999). Following Goudet et al. (2002), the parameters FST, FIS, and the mean (mAIc) and variance (vAIc) of AIc were calculated and compared among sexes by taking the difference between the more dispersive and philopatric sex for FIS (FISd – FISp), the difference between the more philopatric and dispersive sex for mAIc and FST (mAIcp – mAIcd, FSTp – FSTd); or the ratio of the more dispersive to philopatric sex for vAIc (vAIcd / vAIcp). Significant bias was detected using a randomization approach in FSTAT v. 2.9.3.2.
Following Banks and Peakall (2012), multivariate spatial autocorrelation analyses (Smouse and Peakall, 1999; Peakall et al., 2003) were compared across sexes to look for any sex-bias in fine-scale spatial patterns of genetic structure. Pairwise genetic distances were calculated following Peakall et al. (1995) and Smouse and Peakall (1999). Autocorrelation coefficients (Smouse and Peakall, 1999) were calculated across a range of distance classes that varied so as to incorporate comparisons within sampling localities, among adjacent localities, and more distant comparisons. Confidence intervals (95% CIs) about r were calculated by bootstrapping (Peakall et al., 2003) and the null hypothesis of no sex-bias was accepted if there was overlap in the CIs between sexes. The alternative hypothesis predicts that r values are significantly greater in the more philopatric sex. Heterogeneous autocorrelation across sexes was also assessed using single- (t2) and multi-distance (ω) class criteria as implemented in the non-parametric heterogeneity tests described by Smouse et al. (2008). These analyses were conducted in GenAlEx v. 6.5 and assessed for significance using 10,000 permutations and 10,000 bootstrap replicates.
Results
Movement Patterns Based on Satellite Telemetry
Shortfin mako exhibited fidelity to the neritic waters of the Great Australian Bight, Bass Strait, southern Western Australia and the broad oceanic area to the west of Tasmania along the Sub-Tropical Front (Figure 2). Some sharks exhibited oceanic transit phases, leaving continental shelf waters to travel northward into the tropical waters of the northeastern Indian Ocean. During these migrations, three sharks traveled via the Perth and Carnarvon Canyons to the Bartlett and Karma Seamounts, located to the south of Indonesia. Other long-distance movements included four sharks that traveled southward to the Sub-Tropical Front. One shark traveled to the Coral Sea and another individual crossed the Tasman Sea to New Zealand shelf waters, followed by a northward migration to tropical waters near New Caledonia. A single individual moved as far west as the Crozet Plateau in the Indian Ocean.
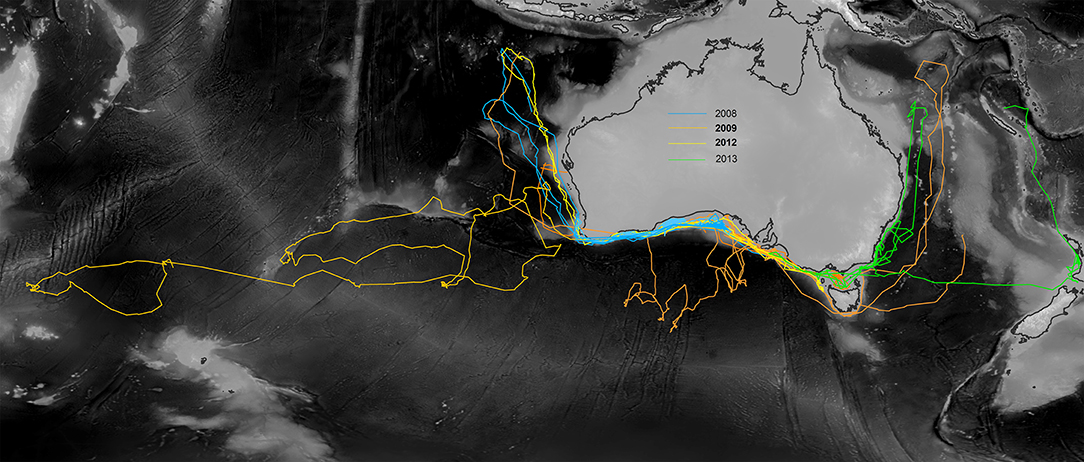
Figure 2. CRAWL model fits to tag data showing the spatial range occupied by shortfin mako over 249–672 days. Individuals were tagged in continental shelf and slope waters of southern Australia between 2008 and 2013.
Thirteen individuals were tracked for 249–672 days (mean 418 ± 37 per individual). Six individuals were tracked for more than one year. Figure 2 shows the spatial scale of movements by all individuals according to CRAWL model fits to the Argos data. Total cumulative distances traveled by shortfin mako ranged from 8,776 km in 262 days to 24,213 km in 551 days (Table S1). Distal displacement distances ranged from 1,500 to 7,520 km (mean 3356 ± 509 km per individual), with 69% of individuals exhibiting distal displacements greater than 2,000 km and 38% of individuals moving more than 4,000 km from their tagging locations. Many of these movements however, represented return migration events (Figure 2). There were no apparent sex-biases in scale of movement. The two longest (M5, M8) and shortest (M9, M12) movements were undertaken by both a male and a female. Males and females traveled an average of 40.8 and 37.5 km, respectively, per day.
Population Genetics—Genetic Diversity and Structure
Mitochondrial DNA data suggest matrilineal substructure across hemispheres, while nuclear DNA data indicate shortfin mako may constitute a globally panmictic population. There was generally high genetic connectivity within Australian waters.
The mitochondrial control region was sequenced for 365 shortfin mako resulting in 48 unique haplotypes, defined by 31 polymorphic sites, sampled across eight broad geographic regions (Table 1, Figure 1). Overall, haplotypic diversity was high (0.894 ± 0.013) while nucleotide diversity was very low (0.004 ± 0.003). Diversity metrics including sample size, number of haplotypes, haplotypic, and nucleotide diversity are shown in Table 1.
The haplotype network (Figure 3A) is characterized by a single, abundant haplotype that was sampled from all locations and in approximately 30% (108/365) of individuals. Other haplotypes are closely related, mostly separated by only a single substitution. Three, or fewer, substitutions were required to link any two haplotypes using parsimony. The network does not indicate any apparent geographic partitioning of haplotypes. Frequencies differ across sampling sites but most haplotypes are found at several, often geographically disparate, locations. One third of haplotypes (16/48) were unique to a single location, 13 of which were singletons.
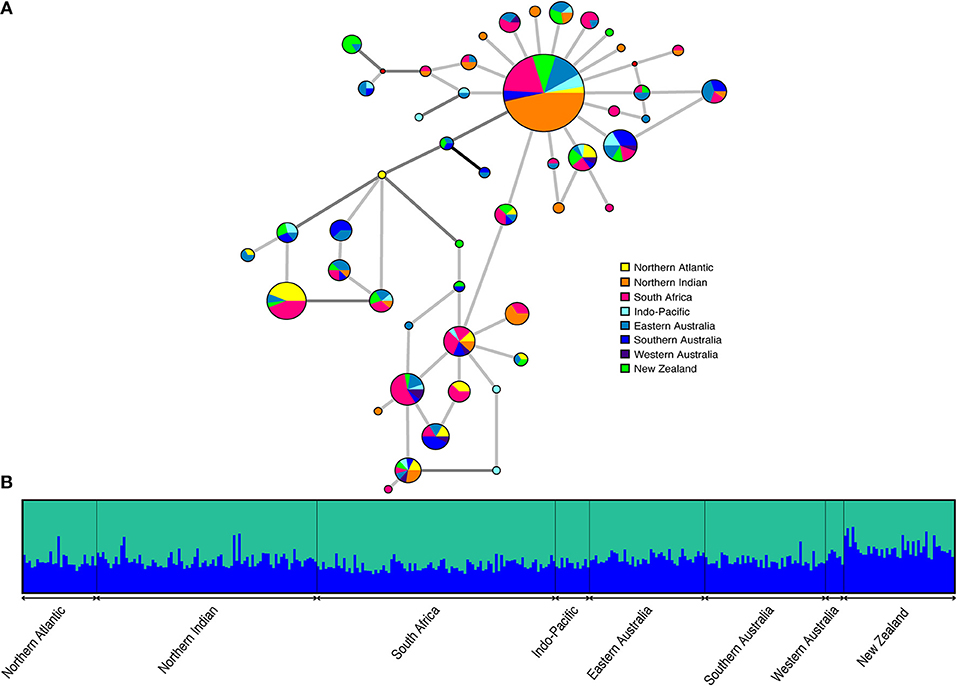
Figure 3. (A) Median joining network containing 10 equally parsimonious trees. Haplotypes are shown as pie charts indicating geographical distribution with size proportional to observed haplotype frequency. Small solid red circles are intermediate states that were not observed. Light gray, dark gray, and black lines represent 1, 2, and 3 mutational steps between haplotypes, respectively (B) Plot of the estimated membership coefficients for each individual in each of two genetic clusters (K = 2). Individuals are represented by vertical columns and grouped according to sampling region.
Pairwise values of FST and ΦST based on mitochondrial data were low to moderate. There was significant differentiation among both Northern Hemisphere locations (Northern Atlantic and Northern Indian Ocean) and all Southern Hemisphere localities. There was significant differentiation between South Africa and all other locations based on exact tests of population differentiation. Weak but significant differentiation was also detected between South Africa and southern Australia based on FST, but this result was not corroborated by ΦST estimates, which showed no significant differentiation between South Africa and any of the Australasian locations (southern Australia, eastern Australia, or New Zealand). Within Australasia, significant differentiation was detected between southern Australia and New Zealand based on FST, ΦST and exact tests of population differentiation (Table 2A).
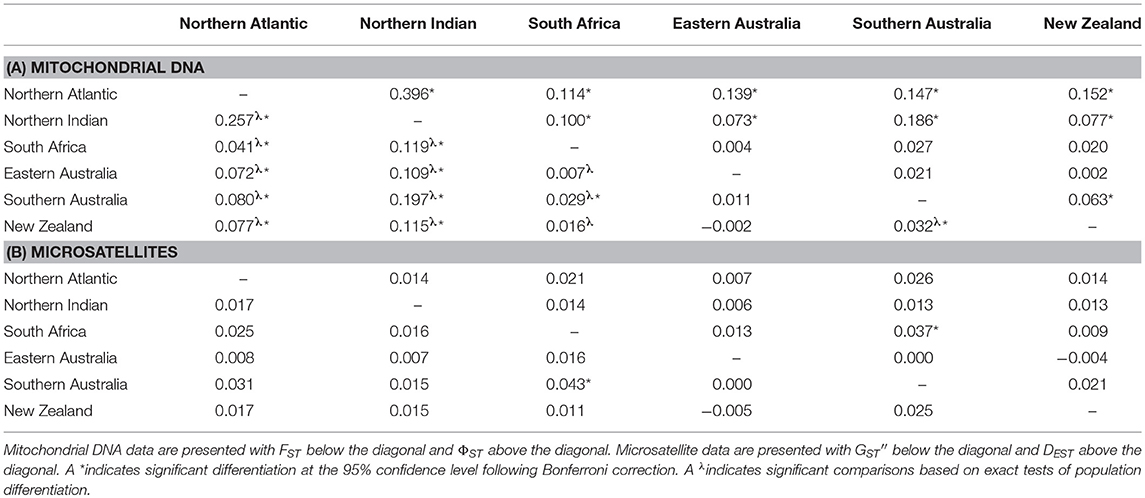
Table 2. Pairwise measures of population differentiation based on (A) mitochondrial DNA and (B) microsatellite data.
The results from AMOVA based on FST and ΦST were qualitatively similar. Interpretations presented herein are thus based on ΦST only. The global ΦST estimate was low, but significant (ΦST = 0.080; P = 0.000). Total variation in the dataset could be separated into five major regions: the northern Atlantic, the northern Indian, South Africa, western Australasia (western and southern Australia), and eastern Australasia (Indo-Pacific, eastern Australia, and New Zealand). While most of the total variation in the dataset was found within populations (91.5%, ΦST = 0.085, P = 0.000), among region variance accounted for a significant 8.2% (ΦCT = 0.082, P = 0.009).
Ten microsatellite loci were genotyped for 355 shortfin mako sampled across the eight broad geographic locations (Figure 1, Table 1). There was no evidence of scoring errors, although Iox-12 and Iox-D123 showed evidence of null alleles in samples from a single location. The frequency of null alleles was low overall (< 10%) and all loci and populations conformed to Hardy-Weinberg expectations following Bonferroni correction. Linkage disequilibrium was detected between Iox-M110 and Iox-B3, Iox-12 and Iox-30, and Iox-M192, and Iox-D123, also in samples from a single location. All loci were therefore included in final analyses.
Genetic diversity at microsatellite loci was moderate to high. The number of alleles per locus ranged between 9 and 30, with means per population ranging from 7.1 to 15.8. The effective number of alleles per locus ranged between 5.3 and 9.4 across populations. Allelic richness was relatively consistent across populations, ranging between 6.7 and 7.2. Observed heterozygosity ranged from 0.75 to 0.86 (unbiased expected heterozygosity = 0.80–0.87; Table 1).
The microsatellite dataset has good statistical power with a 100% probability of detecting a true FST as low as 0.0025, and a high probability (65–70%) of detecting an FST as low as 0.001. The alpha error was ≤ 5%. The majority of microsatellite variation was within populations (99.8%) and the global multilocus FST estimate was very low (FST = 0.002), but significant (P = 0.020). This result was driven by significant FST values at just two of the 10 loci (Iox-M192, FST = 0.005, P = 0.004; Iox-M36 FST = 0.009, P = 0.001). Population pairwise estimates of GST, GST′′, and DEST were low and only a single pairwise comparison, South Africa vs. southern Australia, indicated significant differentiation (Table 2B, Table S2). The among-region variance component of AMOVA was not significant (FCT = 0.004, P = 0.060), accounting for < 1% of total variation in the dataset.
High connectivity among sampling locations was also supported by model-based clustering analyses (Figure 3B). The mean estimated log probability of the data was highest for K = 1, while the modal value of the distribution of ΔK (Evanno et al., 2005) suggested that two clusters could be identified in the data. The ΔK metric cannot be estimated for K = 1 and so panmixia could not be assessed as a possible scenario using this approach. Further, this metric does not take into account the scale of ΔK. Observed values were two orders of magnitude smaller than is typical of cases of real structure and bar plots of the estimated cluster membership coefficients for each individual did not support K = 2 (Figure 3B). There was considerable variance in parameter estimates across runs for each individual K, suggesting non-convergence of the analysis despite running for a sufficient length of time. Together these observations are consistent with there being no signal of population structure in the data.
Population Genetics—Sex-Biased Movement
Patterns of molecular variation across sexes trended toward a signal of male-biased dispersal, however, this was not statistically supported.
Pairwise fixation indices (FST) based on microsatellite markers were low overall, but higher in females (FST = 0.003) than males (FST = 0.000). This difference bordered on significance (P = ~0.050), however the observed values of the test statistics for these parameters were within the range of the null distribution that dispersal is not biased by sex (Figure S1). FIS was higher in males (FIS = 0.009) than females (FIS = 0.001), but this difference was not statistically significant (P = 0.203) and the observed value of the test statistic was also within the null distribution. Corrected assignment (AIc) values ranged between −8.0 and 7.9 for males and −6.2 and 10.8 for females. The frequency distributions of AIc values for males and females were largely overlapping and both sexes showed a similar proportion of negative values (54% for females and 52% for males). The mean and variance of AIc were higher for females (mAIc = 0.19, vAIc = 11.87) than for males (mAIc = −0.19, vAIc = 9.11), however, these differences were not statistically significant (P = 0.158 and P = 0.838, respectively). The observed value of the test statistics for both mAIc and vAIc fell within the range of the null distribution representing the probability that dispersal is not biased by sex (Figure S1).
Spatial patterns of genetic structure were similar across sexes. The male and female 95% bootstrap confidence intervals about r overlapped in all distance classes (Figure 4). The single distance class t2-tests were all non-significant, as was the multi-class ω test of overall correlogram heterogeneity (ω = 6.2, P = 0.411). Low but significant positive autocorrelation among genotypes was detected for both males and females at small (100 km) distance classes (Table S3; Figure 4).

Figure 4. Correlogram plot of the spatial autocorrelation coefficient, r, as a function of geographic distance for males (dark gray) and females (light gray). Black dotted lines represent the 95% confidence interval for the null hypothesis of no spatial structure (r = 0) based on 10,000 random permutations of the data among distance classes. 95% confidence intervals about r were determined using 10,000 bootstrap replicates. Geographic distances (km) are the maximum distance of each class.
Discussion
The vast ranges of pelagic HMS make it challenging to assess population connectivity at spatial scales that are appropriate for informing policy. The pelagic ocean is consequently one of the most under-regulated ecosystems on the planet (Wood, 2008; Game et al., 2009), evidenced by widespread declines in pelagic biodiversity (Verity et al., 2002; Dulvy et al., 2008; Worm and Tittensor, 2011). We aimed to address knowledge gaps regarding connectivity among populations of highly migratory shortfin mako from the Southern Hemisphere, particularly in and around Australian waters. We improved sampling throughout the region and examined spatial and genetic connectivity based on information from long-term satellite telemetry and molecular data.
Movement Patterns Based on Satellite Telemetry
Satellite telemetry data indicated that shortfin mako in Australian waters exhibit multiple movement phases. Periods of residency in neritic habitats are probably indicative of time spent foraging. These contrasted with highly directional transitory movements, within neritic waters, and across vast oceanic expanses including among seamounts, ridges, and adjoining basins (Figure 2). For example, we hypothesize that features such as the eastward flowing South Indian Current, Sub-Tropical Front, and east–west running bathymetric features such as the Naturaliste Plateau, Diamantina Fracture Zone, and Broken Ridge may have facilitated the long-distance movement into the Indian Ocean that was recorded for one individual (Figure 2).
Overall, the geographical scale of the telemetry dataset spanned over 10,700 km from east to west. Observed movement patterns aligned closely with those documented in a previous study of juveniles (Rogers et al., 2015b) and both males and females exhibited similar scales of movement. While movement at these spatial scales indicate that shortfin mako in the Australian region are among the most HMS of pelagic sharks (Benavides et al., 2011; Rogers et al., 2013a,b; Holmes et al., 2014), it is important to also note that many of these movements were return events and some individuals exhibited fidelity to particular areas for extended periods.
Notably, no satellite tagged individuals traversed the lower tropical latitudes, nor passed through equatorial thermal frontal systems. Observed northernmost turning points of directional migrations aligned with surface water temperatures of 28–29°C. Southernmost latitudinal turning points coincided with the Southern subtropical frontal zone and were generally characterized by 9–11°C surface water temperatures. Although a single individual tagged with a standard tag as part of the New South Wales Department of Primary Industries Game Fish Tagging Program was recaptured after having apparently traversed the equator from the east coast of Australia to the Philippines (Rogers et al., 2015a), such transequatorial migration events appear to be uncommon in this species. Long-term telemetry studies of shortfin mako in the northeast Pacific Ocean also reported tropical thermal fronts aligned with turning points during similarly vast return migrations to shelf waters of the California Current ecosystem (Block et al., 2011). This apparent thermal preference has been documented by other studies (Holts and Bedford, 1993; Abascal et al., 2011; Musyl et al., 2011; Rogers et al., 2015b), providing further evidence that warm water may act as a potential barrier to dispersal among hemispheres.
Trans-Equatorial Matrilineal Substructure
We found considerable mitochondrial DNA diversity in shortfin mako. Haplotypic diversity was close to, or higher than, 0.9 at most sampling sites (Figure 3, Table 1), which is similar to that observed in previous studies of this species (Heist et al., 1996; Michaud et al., 2011; Taguchi et al., 2011, 2015) and toward the higher end of the range typically observed for elasmobranchs (Hoelzel et al., 2006; Dudgeon et al., 2008; Schultz et al., 2008; Chabot and Allen, 2009; Benavides et al., 2011; Blower et al., 2012; Corrigan et al., 2016). Also consistent with previous work, our mitochondrial DNA data showed evidence of trans-equatorial matrilineal substructure. Although haplotype sharing was observed among all locations, both Northern Hemisphere sampling locations (North Atlantic and northern Indian) showed significant differentiation from all other sampling sites (Table 2A). This indicates reduced matrilineal gene flow between hemispheres, consistent with the observation that trans-equatorial migration events appear to be infrequent according to tracking data.
Based on observed haplotype sharing between the Atlantic ocean basin and Australia/New Zealand, Michaud et al. (2011) hypothesized that gene flow between Pacific and Atlantic populations of shortfin mako occurs primarily via the Indian Ocean. Taguchi et al. (2011) were similarly unable to distinguish western Indian Ocean sampling sites from those in the eastern Indian or Pacific Oceans. Our analyses provide some support for this hypothesis. Pairwise ΦST estimates between South Africa and the Australasian sampling sites were low relative to comparisons between Northern and Southern Hemisphere sampling sites and not statistically significant, indicating gene flow across the Indian Ocean basin (Table 2A). A distal displacement distance of 7,520 km was recorded for one tracked individual tagged off southern Australia, representing a return westward movement to a location about 2,000 km east of South Africa. This suggests that suitable oceanic migratory pathways exist that could facilitate trans-Indian Ocean linkages between Australasian and South African populations (Figure 2, Table S1). However, exact tests of population differentiation indicated significant differentiation between Australasian and South African sampling sites, and a single pairwise comparison between South Africa and southern Australia was also statistically significant based on FST (Table 2A).
Taguchi et al. (2011) reported that the eastern Indian Ocean sample was highly differentiated from most other sampling sites, although this was based on limited sampling from the region. We are unable to comment on the validity of their finding because, despite extensive efforts, we too obtained only few samples from the eastern Indian Ocean off Western Australia. Taguchi et al. (2011) also indicated possible population structure between the eastern and western coasts of Australia. The Bassian Isthmus in southern Australia is a well-characterized biogeographic barrier that is thought to have promoted bicoastal population subdivision in several marine taxa (Teske et al., 2017). For example, Blower et al. (2012) reported matrilineal subdivision between eastern and southwestern coastal regions of Australia in the white shark, Carcharodon carcharias, a close relative of shortfin mako (Naylor et al., 2012). In contrast, we did not find any evidence of matrilineal population structure in shortfin mako sampled from around the Australian continent (Table 2A). Interestingly, however, the comparison between southern Australia and New Zealand indicated significant differentiation. The ΦST estimate between southern Australia and New Zealand is lower than those observed between Northern and Southern Hemisphere sampling sites, indicating that gene flow between these locations is less constrained than across the equator, but nevertheless restricted enough to represent significant differentiation (Table 2A). It is possible that matrilineal gene flow occurs in a “stepping stone” fashion throughout the region whereby southern Australia and New Zealand are connected via the east coast of Australia. Alternatively, the statistical significance of this single comparison may be artefactual (discussed below).
Further investigations into the extent of connectivity between Australian and South African waters would benefit from tracking information from additional adult individuals, as this may uncover links between neritic habitats on either coastline and reveal how these animals may use bathymetric features during transoceanic movements. Genetic data from an improved sampling of individuals from the southeastern Indian Ocean region would also help clarify the extent of gene flow across the Indian Ocean Basin and between the east and west coasts of Australia.
Nuclear DNA Data Suggest Global Panmixia
Schrey and Heist (2003) report very weak evidence of population structure between the North Atlantic and North Pacific Oceans according to their analysis of microsatellite DNA. Based on an analysis of a larger number of microsatellite markers, Taguchi et al. (2015) report that shortfin mako lack differentiation across their Pacific Ocean range. Sampling from Australasia and the Indian Ocean were limited in both of their studies, allowing little prior inference regarding nuclear genetic structure across the region.
Similar to Schrey and Heist (2003) and Taguchi et al. (2015), we inferred little evidence of population structure based on our microsatellite data. Only a single pairwise comparison, South Africa vs. southern Australia, indicated significant differentiation (Table 2B). The model-based clustering analysis suggested only subtle differences in allele frequencies across regions (Figure 3) and no apparent population structure.
The biological relevance of significant pairwise comparisons of fixation indices should generally be interpreted with caution given their observed small magnitude. FST and analogs measure the effects of gene flow on population differentiation and are thus influenced by both population size and migration rate. The magnitude of FST and analogs decreases non-linearly with increasing migration rate, such that a similar signal of weak to no genetic differentiation can be produced under a scenario of panmixia as well as when populations are large but sufficiently independent to warrant separate management (Waples and Gaggiotti, 2006; Waples et al., 2008; Gagnaire et al., 2015). This makes it difficult to precisely estimate these parameters, and interpret the significance of their magnitude, when population sizes are large and dispersal potential is high. This is likely the case of most marine species, including shortfin mako. Moreover, restricted sampling from a highly diverse set of genotypes can mean that some low estimates of pairwise differentiation are spuriously rendered statistically significant due to minor allele frequency differences (Waples, 1998; Waples et al., 2008).
Given that a high percentage of our tracked individuals showed long distance movements and that there was no evidence of genetic structure based on clustering analyses, it seems plausible that the statistically significant differentiation we detected based on fixation indices between Southern Australia and New Zealand in the mitochondrial data, and South Africa and Southern Australia in the microsatellite data, are artefactual (Waples, 1998; Waples et al., 2008; Gagnaire et al., 2015). However, it is worth noting that many long-distance movements by shortfin mako are return events and this could potentially promote genetic differentiation at smaller geographic scales than their mobility predicts. Additionally, the South Africa vs. Southern Australia comparison for the microsatellite data was also statistically significant based on Jost's DEST, a complementary measure of population structure that quantifies allelic differentiation rather than nearness to population fixation and is less influenced by demographic variables (Jost et al., 2017). This may indicate some potentially biologically relevant partitioning of allelic diversity between these areas, although the magnitude of this parameter was still low.
Methods for collecting genomic-scale data are becoming readily available and the possibility to analyze data from thousands of markers may allow better estimates of low values of genetic differentiation in the future. However, this will likely remain challenging for many marine species with large population sizes (Waples et al., 2008; Gagnaire et al., 2015; Waples, 2015). Quantifying adaptive divergence between locations offers a solution for assessing differentiation even when population sizes are large and gene flow is high. Unlike genetic drift, selection counteracts the homogenizing effect of migration more efficiently in large populations. Genome scans of large marker datasets make it easier than ever to identify loci under selection for this purpose. Applying such methods to studying shortfin mako in the future may offer the possibility to delineate locally adapted units that require independent management even though they may be highly connected (Allendorf et al., 2015; Gagnaire et al., 2015; Jost et al., 2017). Contrasting patterns of neutral vs. adaptive variation may also be particularly relevant to make future predictions regarding how populations may respond to changing environmental conditions or fishing pressure.
Sex-Biased Dispersal
Understanding biases in dispersal patterns can reveal ecologically important areas such as feeding or breeding grounds. This information can also guide fisheries management to avoid selective overharvest of a more philopatric sex (Hueter et al., 2005; Chapman et al., 2015). Male-biased dispersal has been demonstrated in a number of elasmobranch species (Schultz et al., 2008; Daly-Engel et al., 2012; Portnoy et al., 2015). This includes other Lamniformes such as white sharks (Pardini et al., 2001; Blower et al., 2012) in which both sexes are known to undertake oceanic scale movements (Bonfil et al., 2005; Bruce et al., 2006). Skewed sex ratios in shortfin mako catches indicate regional and seasonal sexual segregation (Mucientes et al., 2009; Francis, 2013) and Schrey and Heist (2003) propose male-biased dispersal as a possible mechanism to explain differing patterns of matrilineal vs. nuclear genetic structure in this species.
Under a scenario of sex-biased dispersal, allele frequencies should be more similar across sampling sites among individuals of the dispersing sex than those of the more philopatric sex. Because of this, the dispersing sex will show greater variance in assignment index and lower probability of local assignment, while FST will be higher among the more philopatric sex (Goudet et al., 2002). Observed values for these parameters were consistent with these expectations, suggesting that dispersal may be male-biased in shortfin mako. However, the difference in these parameters between sexes was not statistically significant (Figure S1).
There are several caveats to the interpretation of these results. These tests lack power when dispersal occurs at intermediate rates and sex-bias is subtle (< 80:20; Goudet et al., 2002). Tracking data, low pairwise fixation indices and the clustering analysis based on genetic data suggest that both male and female shortfin mako are highly mobile. Given their mobility and pelagic habit, it seems more likely that any female philopatry will be weak, perhaps at the scale of hemispheres given that we detected a signature of trans-equatorial matrilineal substructure and warm water at the equator appears to represent a physical barrier to dispersal. These tests are also only applicable if sampling occurs during the dispersed phase (Goudet et al., 2002). This assumption is likely violated here given that we sampled multiple cohorts of mostly juveniles and sub-adults. It is possible that violation of these assumptions is masking any signal of sex-bias in these particular analyses, although the trend indicates a male bias.
We also did not detect any statistically supported differences in spatial genetic structure across sexes based on spatial autocorrelation analysis. Detecting a sex bias using this method similarly requires large sample sizes and the development of strong spatial genetic structure in the more philopatric sex (Banks and Peakall, 2012). Banks and Peakall (2012) stress the importance of sampling and concentrating pairwise data points at the scale at which dispersal is restricted in the more philopatric sex. This analysis and our inferences regarding sex-biased dispersal in general, would thus benefit greatly from more information regarding the movements and mating behavior of adult individuals of both sexes, particularly identifying regions that are used for mating and parturition. Satellite tracking together with genetic analysis of a large sample of mature sharks collected during the breeding season from both hemispheres will be required.
Conservation and Management Implications
Inferences of high connectivity based on analyses of our long-term telemetry and molecular datasets spanning six key regions support defining shortfin mako as a pelagic HMS in Australia and neighboring regions of the Southern Hemisphere. Although highly mobile, molecular data presented herein and elsewhere (Michaud et al., 2011; Taguchi et al., 2011, 2015) indicate separation between the Northern and Southern Hemispheres and weaker evidence of separation within the Southern Hemisphere. There appears to be distinct populations in the southeastern and southwestern Pacific (Michaud et al., 2011; Taguchi et al., 2015), and potentially southern Australia and the western Indian Ocean, though connectivity across the Indian Ocean is somewhat complicated to interpret. From a management perspective, it is most important to determine whether inferred differentiation is biologically meaningful such that units warrant management as independent stocks. Only a small number of migrants are required to homogenize allele frequencies across regions (Spieth, 1974; Mills and Allendorf, 1996). Significant spatial partitioning may occur despite high genetic connectivity and the number of migrants per generation required to allow stock rebuilding may be much higher than is required to produce genetic homogeneity (Waples, 1998; Waples et al., 2008).
Tagging data support the separation of Northern and Southern Hemisphere populations of shortfin mako, with only one tagged shark known to have crossed the Equator (Sippel et al., 2011; Rogers et al., 2015a,b; Holdsworth and Saul, 2017). Most movements of tagged mako have occurred within half-ocean basins (e.g., the southwest Pacific). Although Australasian mako frequently make long-distance movements, they also often return to near their tagging location, and importantly, show fidelity to specific areas of continental shelf and slope over several to many months (Rogers et al., 2015a,b; M. Francis unpublished data; data presented herein). Hundreds of mako tagged by gamefishers with standard tags in Australian and New Zealand waters all remained within the southwest Pacific Ocean (Sippel et al., 2011; Rogers et al., 2015a; Holdsworth and Saul, 2017). Fourteen mako tagged with electronic tags in New Zealand and tracked for 34–588 days (mean 251 days, all but one longer than 120 days) spent 42–100% (median 77%) of their time in the New Zealand Exclusive Economic Zone (EEZ; M. Francis, unpublished data). Those results, in combination with our own telemetry data from southern Australia that also showed fidelity to Australian waters, indicate that mako do not wander randomly across the globe, but instead may be resident in comparatively small areas for extended periods. Mako do cross international boundaries and the high seas, however, such that management at the scale of Regional Fisheries Management Organizations is important. But the propensity for mako to spend extended periods within national EEZs means that the homogenizing effect of large-scale movements likely occurs at a rate that is too slow to combat differing levels of fishing mortality across the entire genetic stock. This means that effective fisheries management of shortfin mako must occur at national as well as international levels.
Data Availability Statement
Mitochondrial DNA sequences are available via GenBank® (www.ncbi.nlm.nih.gov/genbank/) Accession numbers MH759795–MH760159. Microsatellite data are provided in Supplementary Data Tables 1–3 in the Supplementary Material.
Author Contributions
SC, PJR, and SDG conceived and designed the study. SC and PJR collected the data. ADL, LBB, BDB, GC, CAD, AF, MPF, SDG, JRH, RWJ, DK, LM, GRM, GJPN, JGP, NQ, WTW, and SPW contributed samples, laboratory infrastructure, and analysis tools. SC, PJR, and ADL performed the analysis. SC, PJR, ADL, LBB, GC, MPF, RWJ, WTW, and SPW wrote the paper.
Conflict of Interest Statement
JGP was employed by Pepperell Research and Consulting Pty Ltd. LM was employed by Stick Figure Fish Illustration.
The remaining authors declare that the research was conducted in the absence of any commercial or financial relationships that could be construed as a potential conflict of interest.
Acknowledgments
Funding for this research was provided by the Fisheries Research and Development Corporation Tactical Research Fund (Shark Futures: 2011-077) on behalf of the Australian Government. Aspects of this research were reported in Rogers et al. (2015a) and are reproduced with permission. Additional support was provided by the SeaWorld Research and Rescue Foundation, Nature Foundation SA Inc., Department for Environment and Water (DEW), Australian Geographic Society, SARDI Aquatic Sciences, the Victorian Department of Primary Industries Recreational Fishing License Trust Account Large Grants Program and Flinders University. LBB acknowledges financial support from the Australian Research Council (FT130101068). GRM was supported by the Isabel Barreto Human Resources Plan of the Government of Galicia. Procedures were undertaken under SARDI/PIRSA Ministerial exemptions (Section 115; 9902094, and S59; 9902064), DEW Permit U25570, Environment Australia, EPBC Act 1999 Permit E20120068 and Flinders University Animal Welfare Committee approval (Project 309). We thank the international participants of the FRDC funded workshop, Shark futures - a synthesis of available data on mako and porbeagle sharks in Australasian waters: Current status and future directions for constructive input and support of this project. Andrew Oxley, Nicole Patten, an FRDC assigned reviewer, Viorel Popescu and Melissa Millar provided valuable comments that improved the final version of this manuscript. We also thank the following people for their assistance during satellite tag deployments or tissue sample collection: John Collinson, Anton Blass, Callan Henley, Shane Gill (FV Rahi Aroha), Dennis and Kerry Heineke, Adam Todd (FV Shaka-Zura), Paul Irvine, Steve Toranto, Phil Stroker, Clinton Adlington (FV Home Strait), Shane Sanders and Brodie Carter (FV Baitwaster), Ashley and Neville Dance, Greg Barea, Charlie Huveneers (Flinders University), Matt Heard, Mick Drew, Crystal Beckmann (SARDI), Slavko Kolega, Chris Meletti (Sekol, MV Lucky-S), Mark Lewis, Bruce Barker (CSIRO), staff of the KwaZulu-Natal Sharks Board, The South African Institute for Aquatic Biodiversity, Matias Braccini, and Rory McAuley.
Supplementary Material
The Supplementary Material for this article can be found online at: https://www.frontiersin.org/articles/10.3389/fevo.2018.00187/full#supplementary-material
References
Abascal, F., Quintans, M., Ramos-Cartelle, A., and Mejuto, J. (2011). Movements and environmental preferences of the shortfin mako, Isurus oxyrinchus, in the southeastern Pacific Ocean. Mar. Biol. 158, 1175–1184. doi: 10.1007/s00227-011-1639-1
Allendorf, F. W., Hohenloe, P. A., and Luikart, G. (2015). Genomics and the future of conservation genetics. Nat. Rev. Genet. 11, 697–709. doi: 10.1038/nrg2844
Bandelt, H., Forster, P., and Rohl, A. (1999). Median-joining networks for inferring intraspecific phylogenies. Mol. Biol. Evolu. 16, 37–48. doi: 10.1093/oxfordjournals.molbev.a026036
Banks, S. C., and Peakall, R. (2012). Genetic spatial autocorrelation can readily detect sex-biased dispersal. Mol. Ecol. 21, 2092–2105. doi: 10.1111/j.1365-294X.2012.05485.x
Benavides, M. T., Feldheim, K. A., Duffy, C. A., Wintner, S. P., Braccini, J. M., Boomer, J., et al. (2011). Phylogeography of the copper shark (Carcharhinus brachyurus) in the Southern Hemisphere: implications for the conservation of a coastal apex predator. Mar. Freshwater Res. 62, 861–869. doi: 10.1071/MF10236
Bester-Van Der Merwe, A. E., Bitalo, D., Cuevas, J. M., Ovenden, J. R., Hernández, S., Dasilva, C., et al. (2017). Population genetics of Southern Hemisphere tope shark (Galeorhinus galeus): intercontinental divergence and constrained gene flow at different geographical scales. PLoS ONE 12:e0184481. doi: 10.1371/journal.pone.0184481
Block, B. A., Jonsen, I. D., Jorgensen, S. J., Winship, A. J., Shaffer, S. A., Bograd, S. J., et al. (2011). Tracking apex marine predator movements in a dynamic ocean. Nature 475, 86–90. doi: 10.1038/nature10082
Blower, D. C., Pandolfi, J. M., Bruce, B. D., Gomez-Cabrera, M., and Ovenden, J. R. (2012). Population genetics of Australian white sharks reveals fine-scale spatial structure, transoceanic dispersal events and low effective population sizes. Mar. Ecol. Prog. Ser. 455, 229–244. doi: 10.3354/meps09659
Bonfil, R., Meyer, M., Scholl, M., Johnson, R., O'brien, S., Oosthuizen, H., et al. (2005). Transoceanic migration, spatial dynamics, and population linkages of white sharks. Science 310, 100–103. doi: 10.1126/science.1114898
Bruce, B. D., Stevens, J. D., and Malcolm, H. (2006). Movements and swimming behaviour of white sharks (Carcharodon carcharias) in Australian waters. Mar. Biol. 150, 161–172. doi: 10.1007/s00227-006-0325-1
Cailliet, G. M., Cavanagh, R. D., Kulka, D. W., Stevens, J. D., Soldo, A., Clo, S., et al. (2009). Isurus oxyrinchus. The IUCN Red List of Threatened Species 2009:e.T39341A10207466. doi: 10.2305/IUCN.UK.2009-2.RLTS.T39341A10207466.en
Campana, S. E., Dorey, A., Fowler, M., Joyce, W., Wang, Z., Wright, D., et al. (2011). Migration pathways, behavioural thermoregulation and overwintering grounds of blue sharks in the northwest Atlantic. PLoS ONE 6:e16854. doi: 10.1371/journal.pone.0016854
Carey, F. G. (1973). Fishes with warm bodies. Sci. Am. 228, 36–44. doi: 10.1038/scientificamerican0273-36
Chabot, C. L., and Allen, L. G. (2009). Global population structure of the tope (Galeorhinus galeus) inferred by mitochondrial control region sequence data. Mol. Ecol. 18, 545–552. doi: 10.1111/j.1365-294X.2008.04047.x
Chang, J., and Liu, K. (2009). Stock assessment of the shortfin mako shark (Isurus oxyrinchus) in the northwest Pacific Ocean using per recruit and virtual population analyses. Fish. Res. 98, 92–101. doi: 10.1016/j.fishres.2009.04.005
Chapman, D. D., Feldheim, K. A., Papastamatiou, Y. P., and Hueter, R. E. (2015). There and back again: a review of residency and return migrations in sharks, with implications for population structure and management. Ann. Rev. Mar. Sci. 7, 547–570. doi: 10.1146/annurev-marine-010814-015730
Corrigan, S., Huveneers, C., Stow, A. J., and Beheregaray, L. B. (2016). A multilocus comparative study of dispersal in three codistributed demersal sharks from eastern Australia. Can. J. Fish. Aquat. Sci. 73, 406–506. doi: 10.1139/cjfas-2015-0085
Daly-Engel, T. S., Seraphin, K. D., Holland, K. N., Coffey, J. P., Nance, H. A., Toonen, R. J., et al. (2012). Global phylogeography with mixed-marker analysis reveals male-mediated dispersal in the endangered scalloped hammerhead shark (Sphyrna lewini). PLoS ONE 7:e29986. doi: 10.1371/journal.pone.0029986
Dickson, K. A., and Graham, J. B. (2004). Evolution and consequences of endothermy in fishes. Physiol. Biochem. Zool. 77, 998–1018. doi: 10.1086/423743
Doherty, P. D., Baxter, J. M., Gell, F. R., Godley, B. J., Graham, R. T., Hall, G., et al. (2017). Long-term satellite tracking reveals variable seasonal migration strategies of basking sharks in the north-east Atlantic. Sci. Rep. 7:e42837. doi: 10.1038/srep42837
Dudgeon, C. L., Broderick, D., and Ovenden, J. R. (2008). IUCN classification zones concord with, but underestimate, the population genetic structure of the zebra shark Stegostoma fasciatum in the Indo-West Pacific. Mol. Ecol. 18, 248–261. doi: 10.1111/j.1365-294X.2008.04025.x
Dulvy, N. K., Baum, J. K., Clarke, S., Compagno, L. J. V., Cortes, E., Domingo, A., et al. (2008). You can swim but you can't hide: the global status and conservation of oceanic pelagic sharks and rays. Aquat. Conserv. 18, 459–482. doi: 10.1002/aqc.975
Earl, D. A., and Vonholdt, B. M. (2012). Structure Harvester: a website and program for visualizing STRUCTURE output and implementing the Evanno method. Conserv. Genet. Resour. 4, 359–361. doi: 10.1007/s12686-011-9548-7
Evanno, G., Regnaut, S., and Goudet, J. (2005). Detecting the number of clusters of individuals using the software STRUCTURE: a simulation study. Mol. Ecol. 14, 2611–2620. doi: 10.1111/j.1365-294X.2005.02553.x
Excoffier, L., and Lischer, H. E. L. (2010). Arlequin suite Ver 3.5: a new series of programs to perform population genetics analyses under Linux and Windows. Mol. Ecol. Resour. 10, 564–567. doi: 10.1111/j.1755-0998.2010.02847.x
Falush, D., Stephens, M., and Pritchard, J. K. (2003). Inference of population structure using multilocus genotype data: linked loci and correlated allele frequencies. Genetics 164, 1567–1587. doi: 10.3410/f.1015548.197423
Favre, L., Balloux, F., Goudet, J., and Perrin, N. (1997). Female-biased dispersal in the monogamous mammal Crocidura russula: evidence from field data and microsatellite patterns. Proc. R. Soc. Lond. Ser. B 264, 127–132. doi: 10.1098/rspb.1997.0019
Feldheim, K. A., Gruber, S. H., Dibattista, J. D., Babcock, E. A., Kessel, S. T., Hendry, A. P., et al. (2014). Two decades of genetic profiling yields first evidence of natal philopatry and long-term fidelity to parturition sites in sharks. Mol. Ecol. 23, 110–117. doi: 10.1111/mec.12583
Francis, M. P. (2013). Commercial Catch Composition of Highly Migratory Elasmobranchs. New Zealand Fisheries Assessment Report 2013/68.
Frankham, R., Ballou, J. D., and Briscoe, D. A. (2010). Introduction to Conservation Genetics. Cambridge: Cambridge Universty Press.
Gagnaire, P. A., Broquet, T., Aurelle, D., Viard, F., Souissi, A., Bonhomme, F., et al. (2015). Using neutral, selected, and hitchhiker loci to assess connectivity of marine populations in the genomic era. Evol. Appl. 8, 769–786. doi: 10.1111/eva.12288
Game, E. T., Grantham, H. S., Hobday, A. J., Pressey, R. L., Lombard, A. T., Beckley, L. E., et al. (2009). Pelagic protected areas: the missing dimension in ocean conservation. Trends Ecol. Evol. (Amst). 24, 360–369. doi: 10.1016/j.tree.2009.01.011
Goudet, J. (2001). FSTAT, a Program to Estimate and Test Gene Diversities and Fixation Indices (Version 2.9.3). Available online at: https://www2.unil.ch/popgen/softwares/fstat.htm
Goudet, J., Perrin, N., and Waser, P. (2002). Tests for sex biased dispersal using genetic markers. Mol. Ecol. 11, 1103–1114. doi: 10.1046/j.1365-294X.2002.01496.x
Guttridge, T. L., Van Zinnicq Bergmann, M. P. M., Bolte, C., Howey, L. A., Finger, J. S., Kessel, S. T., et al. (2017). Philopatry and regional connectivity of the great hammerhead shark, Sphyrna mokarran in the U.S. and Bahamas. Front. Mar. Sci. 4, 2296–7745. doi: 10.3389/fmars.2017.00003
Heist, E. J., Musick, J. A., and Graves, J. E. (1996). Genetic population structure of the shortfin mako (Isurus oxyrinchus) inferred from restriction fragment length polymorphism analysis of mitochondrial DNA. Can. J. Fish. Aquat. Sci. 53, 583–588. doi: 10.1139/f95-245
Heupel, M. R., Simpfendorfer, C. A., and Hueter, R. E. (2003). Running before the storm: blacktip sharks respond to falling barometric pressure associated with tropical storm Gabrielle. J. Fish Biol. 63, 1357–1363. doi: 10.1046/j.1095-8649.2003.00250.x
Hoelzel, A. R., Shivji, M., Magnussen, J., and Francis, M. P. (2006). Low worldwide genetic diversity in the basking shark (Cetorhinus maximus). Biol. Lett. 2, 639–642. doi: 10.1098/rsbl.2006.0513
Holdsworth, J. C., and Saul, P. J. (2017). New Zealand Billfish and Gamefish Tagging, 2013–14 to 2015–16. New Zealand Fisheries Assessment Report 2017/14.
Holmes, B. J., Pepperell, J. G., Griffiths, S. P., Jaine, F. R. A., Tibbetts, I. R., and Bennett, M. B. (2014). Tiger Shark (Galeocerdo cuvier) movement patterns and habitat use determined by satellite tagging in eastern Australian waters. Mar. Biol. 11, 2645–2658.doi: 10.1007/s00227-014-2536-1
Holts, D. B., and Bedford, D. W. (1993). Horizontal and vertical movements of the shortfin mako shark, Isurus oxyrinchus, in the southern California Bight. Aust. J. Mar. Freshwater Res. 44, 901–909.
Hubisz, M., Falush, D., Stephens, M., and Pritchard, J. K. (2009). Inferring weak population structure with the assistance of sample group information. Mol. Ecol. Resour. 9, 1322–1332. doi: 10.1111/j.1755-0998.2009.02591.x
Hueter, R. E., Heupel, M. R., Heist, E. J., and Keeney, D. B. (2005). Evidence of philopatry in sharks and implications for the management of shark fisheries. J. Northwest Atl. Fish. Sci. 35, 239–247. doi: 10.2960/J.v35.m493
Hueter, R. E., Tyminski, J. P., and De La Parra, R. (2013). Horizontal movements, migration patterns and population structure of whale sharks in the Gulf of Mexico and northwestern Caribbean Sea. PLoS ONE 8:e71883. doi: 10.1371/journal.pone.0071883
Jakobsson, M., and Rosenberg, N. A. (2007). CLUMPP: a cluster matching and permutation program for dealing with label switching and multimodality in analysis of population structure. Bioinformatics 23, 1801–1806. doi: 10.1093/bioinformatics/btm233
Johnson, D., London, J., Lea, M. A., and Durban, J. (2008). Continuous-time correlated random walk model for animal telemetry data. Ecology 89, 1208–1215. doi: 10.1890/07-1032.1
Jost, L. (2008). GST and its relatives do not measure differentiation. Mol. Ecol. 17, 4015–4026. doi: 10.1111/j.1365-294X.2008.03887.x
Jost, L., Archer, F., Flanagan, S., Gaggiotti, O., Hoban, S., and Latch, E. (2017). Differentiation measures for conservation genetics. Evol. Appl. 11, 1139–1148. doi: 10.1111/eva.12590
Jukes, T. H., and Cantor, C. R. (1969). “Evolution of protein molecules,” in Mammalian protein metabolism, ed H.M. Munro (New York, NY: Academic Press), 21–132.
Kinney, M. J., and Simpfendorfer, C. A. (2009). Reassessing the value of nursery areas to shark conservation and management. Conserv. Lett. 2, 53–60. doi: 10.1111/j.1755-263X.2008.00046.x
Meirmans, P. G., and Hedrick, P. W. (2011). Assessing population structure: FST and related measures. Mol. Ecol. Resour. 11, 5–18. doi: 10.1111/j.1755-0998.2010.02927.x
Michaud, A., Hyde, J. R., Kohin, S., and Vetter, R. (2011). “Mitochondrial DNA sequence data reveals barriers to dispersal in the highly migratory shortfin mako shark (Isurus oxyrinchus),” in Report of the Shark Working Group Workshop. Annex 4. International Scientific Committee for Tuna and Tuna-like Species in the North Pacific Ocean. (La Jolla, CA), 38.
Mills, L. S., and Allendorf, F. W. (1996). The one-migrant-per-generation rule in conservation and management. Conserv. Biol. 10, 1509–1518.
Mossman, C. A., and Waser, P. M. (1999). Genetic detection of sex-biased dispersal. Mol. Ecol. Notes 8, 1063–1067.
Mucientes, G. R., Queiroz, N., Sousa, L. L., Tarroso, P., and Sims, D. W. (2009). Sexual segregation of pelagic sharks and the potential threat from fisheries. Biol. Lett. 5, 156–159. doi: 10.1098/rsbl.2008.0761
Musyl, M. K., Brill, R. W., Curran, D. S., Fragoso, N. M., Mcnaughton, L. M., Nielsen, A., et al. (2011). Postrelease survival, vertical and horizontal movements, and thermal habitats of five species of pelagic sharks in the central Pacific Ocean. Fish. Bull. 109, 341–368.
Naylor, G. J. P., Caira, J. N., Jensen, K., Rosana, K. M., Straube, N., and Lakner, C. (2012). “Elasmobranch phylogeny: A mitochondrial estimate based on 595 species,” in Biology of sharks and their relatives, 2nd Edn., eds J. C. Carrier, J. A. Musick and M. R. Heithaus (Boca Raton, FL: CRC Press), 31–56.
Paetkau, D., Calvert, W., Stirling, I., and Strobeck, C. (1995). Microsatellite analysis of population structure in Canadian polar bears. Mol. Ecol. 4, 347–354.
Palumbi, S. R. (2003). Population genetics, demographic connectivity, and the design of marine reserves. Ecol. Appl. 13, 146–158. doi: 10.1890/1051-0761(2003)013[0146:PGDCAT]2.0.CO;2
Pardini, A. T., Jones, C. S., Noble, L. R., Kreiser, B., Malcolm, H., Bruce, B. D., et al. (2001). Sex-biased dispersal of great white sharks. Nature 412, 139–140. doi: 10.1038/35084125
Peakall, R., Ruibal, M., and Lindenmayer, D. B. (2003). Spatial autocorrelation analysis offers new insights into gene flow in the Australian bush rat, Rattus fuscipes. Evolution 57, 1182–1195. doi: 10.1111/j.0014-3820.2003.tb00327.x
Peakall, R., and Smouse, P. E. (2012). GenAlEx 6.5: genetic analysis in Excel. Population genetic software for teaching and research – an update. Bioinformatics 28, 2537–2539. doi: 10.1093/bioinformatics/bts460
Peakall, R., Smouse, P. E., and Huff, D. R. (1995). Evolutionary implications of allozyme and RAPD variation in diploid populations of dioecious buffalo grass, Buchloe dactyloides. Mol. Ecol. 4, 135–147.
Piry, S., Alapetite, A., Cornuet, J. M., Paetkau, D., Baudouin, L., and Estoup, A. (2004). GeneClass2: a software for genetic assignment and first-generation migrant detection. J. Hered. 95, 536–539. doi: 10.1093/jhered/esh074
Portnoy, D. S., Mcdowell, J. R., Heist, E. J., Musick, J. A., and Graves, J. E. (2010). World phylogeography and male-mediated gene flow in the sandbar shark, Carcharhinus plumbeus. Mol. Ecol. 19, 1994–2010. doi: 10.1111/j.1365-294X.2010.04626.x
Portnoy, D. S., Puritz, J. B., Hollenbeck, C. M., Gelsleichter, J., Chapman, D., and Gold, J. R. (2015). Selection and sex-biased dispersal in a coastal shark: the influence of philopatry on adaptive variation. Mol. Ecol. 24, 5877–5885. doi: 10.1111/mec.13441
Pritchard, J. K., Stephens, M., and Donnelly, P. (2000). Inference of population structure using multilocus genotype data. Genetics 155, 945–959.
R Core Team (2013). R: A Language and Environment for Statistical Computing. Vienna: RFoundation for Statistical Computing.
Raymond, M., and Rousset, F. (1995). Population genetics software for exact tests and ecumenicism. J. Hered. 86, 248–249.
Rogers, P. J., Corrigan, S., and Lowther, A. D. (2015a). Using Satellite Tagging and Molecular Techniques to Improve the Ecologically Sustainable Fisheries Management of Shortfin Makos (Isurus oxyrinchus) in the Australasian region. Final Report FRDC Tactical Research Fund Project No. 2011/077.
Rogers, P. J., Huveneers, C., Goldsworthy, S. D., Cheung, W. W. L., Jones, G. K., Mitchell, J. G., et al. (2013a). Population metrics and movements of two carcharhinids: a comparison of the vulnerability of pelagic sharks of the southern Australian gulfs and shelves. Mar. Freshwater Res. 64, 20–30. doi: 10.1071/MF11234
Rogers, P. J., Huveneers, C., Goldsworthy, S. D., Mitchell, J. G., and Seuront, L. (2013b). Broad-scale movements and pelagic habitat of the dusky shark Carcharhinus obscurus off Southern Australia determined using pop-up satellite archival tags. Fish. Oceanogr. 22, 102–112. doi: 10.1111/fog.12009
Rogers, P. J., Huveneers, C., Page, B., Goldsworthy, S. D., Coyne, M., Lowther, A. D., et al. (2015b). Living on the continental shelf edge: habitat use of juvenile shortfin makos Isurus oxyrinchus in the Great Australian Bight, southern Australia. Fish. Oceanogr. 24, 205–218. doi: 10.1111/fog.12103
Rosenberg, N. A. (2004). Distruct: a program for the graphical display of population structure. Mol. Ecol. Notes 4, 137–138. doi: 10.1046/j.1471-8286.2003.00566.x
Ryman, N., and Palm, S. (2006). POWSIM: a computer program for assessing statistical power when testing for genetic differentiation. Mol. Ecol. 6, 600–602. doi: 10.1111/j.1471-8286.2006.01378.x
Sandoval-Castillo, J., and Beheregaray, L. B. (2015). Metapopulation structure informs conservation management in a heavily exploited coastal shark (Mustelus henlei). Mar. Ecol. Prog. Ser. 533, 191–203. doi: 10.3354/meps11395
Schrey, A. W., and Heist, E. J. (2002). Microsatellite markers for the shortfin mako and cross-species amplification in Lamniformes. Conserv. Genet. 3, 459–461. doi: 10.1023/A:1020583609967
Schrey, A. W., and Heist, E. J. (2003). Microsatellite analysis of population structure in the shortfin mako (Isurus oxyrinchus). Can. J. Fish. Aquat. Sci. 60, 670–675. doi: 10.1139/f03-064
Schuelke, M. (2000). An economic method for the fluorescent labeling of PCR fragments. Nat. Biotechnol. 18, 233–234. doi: 10.1038/72708
Schultz, J. K., Feldheim, K. A., Gruber, S. H., Ashley, M. V., Mcgovern, T. M., and Bowen, B. W. (2008). Global phylogeography and seascape genetics of the lemon sharks (genus Negaprion). Mol. Ecol. 17, 5336–5348. doi: 10.1111/j.1365-294X.2008.04000.x
Sims, D. W., Mucientes, G. R., and Queiroz, N. (2018). Shortfin mako sharks threatened by inaction. Science 359:1342. doi: 10.1126/science.aat0315
Sippel, T., Wraith, J., Kohin, S., Taylor, V., Holdsworth, J., Taguchi, M., et al. (2011). A Summary Of Blue Shark (Prionace glauca) and Shortfin Mako Shark (Isurus oxyrinchus) Tagging Data Available From the North and Southwest Pacific Ocean. Working document submitted to the ISC Shark Working Group Workshop ISC/11/SHARKWG-2/04 (La Jolla, CA).
Smouse, P. E., and Peakall, R. (1999). Spatial autocorrelation analysis of individual multiallele and multilocus genetic structure. Heredity 82, 561–573. doi: 10.1038/sj.hdy.6885180
Smouse, P. E., Peakall, R., and Gonzales, E. (2008). A heterogeneity test for fine-scale genetic structure. Mol. Ecol. 17, 3389–3400. doi: 10.1111/j.1365-294X.2008.03839.x
Sunnucks, P., and Hales, D. (1996). Numerous transposed sequences of mitochondrial cytochrome oxidase in aphids of the genus Sitobion (Hemiptera: Aphididae). Mol. Biol. Evol. 13, 510–523. doi: 10.1093/oxfordjournals.molbev.a025612
Taguchi, M., Kitamura, T., and Yokawa, K. (2011). Genetic Population Structure of Shortfin Mako (Isurus oxyrinchus) Inferred From Mitochondrial DNA on Inter-Oceanic Scale. Working document submitted to the ISC Shark Working Group Workshop ISC/11/SHARKWG-1/02" (Keelung).
Taguchi, M., Oshimo, S., and Yokawa, K. (2015). Genetic Stock Structure of Shortfin Mako (Isurus oxyrinchus) in the Pacific Ocean. Working document submitted to the ISC Shark Working Group Workshop ISC/15/SHARKWG-1/ (Shimizu-Ku: National Research Institute of Far Seas Fisheries).
Teske, P. R., Sandoval-Castillo, J., Waters, J. M., and Beheregaray, L. B. (2017). An overview of Australia's temperate marine phylogeography, with new evidence from high-dispersal gastropods. J. Biogeogr. 44, 217–229. doi: 10.1111/jbi.12783
Van Oosterhout, C., Hutchinson, W. F., Wills, D. P. M., and Shipley, P. (2004). Microchecker: software for identifying and correcting genotyping errors in microsatellite data. Mol. Ecol. Notes 4, 535–538. doi: 10.1111/j.1471-8286.2004.00684.x
Verity, P. G., Smetacek, V., and Smayda, T. J. (2002). Status, trends and the future of the marine pelagic ecosystem. Environ. Conserv. 29, 207–237. doi: 10.1017/S0376892902000139
Waples, R. S. (1998). Separating the wheat from the chaff – patterns of genetic differentiation in high gene flow species. J. Hered. 89, 438–450. doi: 10.1093/jhered/89.5.438
Waples, R. S. (2015). Testing for Hardy-Weinberg proportions: have we lost the plot? J. Hered. 106, 1–19. doi: 10.1093/jhered/esu062
Waples, R. S., and Gaggiotti, O. (2006). What is a population? An empirical evaluation of some genetic methods for identifying the number of gene pools and their degree of connectivity. Mol. Ecol. 15, 1419–1439. doi: 10.1111/j.1365-294X.2006.02890.x
Waples, R. S., Punt, A. E., and Cope, J. M. (2008). Integrating genetic data into management of marine resources: how can we do it better? Fish and Fisheries 9, 423–449. doi: 10.1111/j.1467-2979.2008.00303.x
Wood, L. J. (2008). Assessing progress towards global marine protection targets: shortfalls in information and action. Oryx 42, 340–351. doi: 10.1017/S003060530800046X
Worm, B., and Tittensor, D. P. (2011). Range contraction in large pelagic predators. Proc. Natl. Acad. Sci. U.S.A. 108, 11942–11947. doi: 10.1073/pnas.1102353108
Worm, B., and Vanderzwaag, D. (2007). High-seas fisheries: troubled waters, tangled governance, and recovery prospects. Behind Headlines 64, 2–32. Available online at: https://ssrn.com/abstract=2101249
Keywords: telemetry, tracking, population structure, mitochondrial DNA, microsatellites, conservation, fisheries
Citation: Corrigan S, Lowther AD, Beheregaray LB, Bruce BD, Cliff G, Duffy CA, Foulis A, Francis MP, Goldsworthy SD, Hyde JR, Jabado RW, Kacev D, Marshall L, Mucientes GR, Naylor GJP, Pepperell JG, Queiroz N, White WT, Wintner SP and Rogers PJ (2018) Population Connectivity of the Highly Migratory Shortfin Mako (Isurus oxyrinchus Rafinesque 1810) and Implications for Management in the Southern Hemisphere. Front. Ecol. Evol. 6:187. doi: 10.3389/fevo.2018.00187
Received: 18 August 2018; Accepted: 26 October 2018;
Published: 20 November 2018.
Edited by:
David Jack Coates, Department of Biodiversity, Conservation and Attractions (DBCA), AustraliaReviewed by:
Viorel Dan Popescu, Ohio University, United StatesMelissa Ann Millar, Department of Biodiversity, Conservation and Attractions (DBCA), Australia
Copyright © 2018 Corrigan, Lowther, Beheregaray, Bruce, Cliff, Duffy, Foulis, Francis, Goldsworthy, Hyde, Jabado, Kacev, Marshall, Mucientes, Naylor, Pepperell, Queiroz, White, Wintner and Rogers. This is an open-access article distributed under the terms of the Creative Commons Attribution License (CC BY). The use, distribution or reproduction in other forums is permitted, provided the original author(s) and the copyright owner(s) are credited and that the original publication in this journal is cited, in accordance with accepted academic practice. No use, distribution or reproduction is permitted which does not comply with these terms.
*Correspondence: Shannon Corrigan, c2NvcnJpZ2FuQGZsb3JpZGFtdXNldW0udWZsLmVkdQ==