- 1Department of Primatology, Max Planck Institute for Evolutionary Anthropology, Leipzig, Germany
- 2German Centre for Integrative Biodiversity Research (iDiv) Halle-Leipzig-Jena, Leipzig, Germany
- 3Wild Chimpanzee Foundation (West Africa Representation), Abidjan, Côte d'Ivoire
- 4Tacugama Chimpanzee Sanctuary, Freetown, Sierra Leone
- 5Bumbuna Watershed Management Authority, Freetown, Sierra Leone
- 6Projets Biodiversité et Ressources Naturelles BRL Ingénierie, Nîmes, France
- 7The Biodiversity Consultancy Ltd., Cambridge, United Kingdom
- 8Faculty of Computing Science and Mathematics, University of Stirling, Stirling, United Kingdom
- 9Department of Wildlife and Range Management, Kwame Nkrumah University of Science and Technology, Kumasi, Ghana
- 10Independent Consultant, Nîmes, France
- 11Conservation International, Monrovia, Liberia
- 12Independent Biodiversity Consultant, Slinger, WI, United States
- 13West African Primate Conservation Action, Accra, Ghana
- 14Anthropology Center for Conservation, Environment and Development, Oxford Brookes University, Oxford, United Kingdom
- 15Chimbo Foundation, Amsterdam, Netherlands
- 16Behavioural Biology Unit, University of Liège, Liège, Belgium
- 17WWF Germany, Berlin, Germany
- 18RSPB Centre for Conservation Science, The Royal Society for the Protection of Birds, Sandy, United Kingdom
- 19School of Biological Sciences, Royal Holloway University of London, Egham, United Kingdom
- 20Université Jean Lorougnon Guédé, Daloa, Côte d'Ivoire
- 21Centre Suisse de Recherches Scientifiques en Côte d'Ivoire, Abidjan, Côte d'Ivoire
- 22Fauna and Flora International, Monrovia, Liberia
- 23World Wide Fund for Nature, Regional Office for Africa - Yaoundé Hub, Yaoundé, Cameroon
- 24Jane Goodall Institute Spain, Dindefelo Community Nature Reserve, Kédougou, Senegal
- 25International Union for Conservation of Nature, West and Central Africa Programs, Ouagadougou, Burkina Faso
- 26Regional Environmental Center for Central and Eastern Europe, Country Office Poland, Warsaw, Poland
With continued expansion of anthropogenically modified landscapes, the proximity between humans and wildlife is continuing to increase, frequently resulting in species decline. Occasionally however, species are able to persist and there is an increased interest in understanding such positive outliers and underlying mechanisms. Eventually, such insights can inform the design of effective conservation interventions by mimicking aspects of the social-ecological conditions found in areas of species persistence. Recently, frameworks have been developed to study the heterogeneity of species persistence across populations with a focus on positive outliers. Applications are still rare, and to our knowledge this is one of the first studies using this approach for terrestrial species conservation. We applied the positive deviance concept to the western chimpanzee, which occurs in a variety of social-ecological landscapes. It is now categorized as Critically Endangered due to hunting and habitat loss and resulting excessive decline of most of its populations. Here we are interested in understanding why some of the populations did not decline. We compiled a dataset of 17,109 chimpanzee survey transects (10,929 km) across nine countries and linked them to a range of social and ecological variables. We found that chimpanzees seemed to persist within three social-ecological configurations: first, rainforest habitats with a low degree of human impact, second, steep areas, and third, areas with high prevalence of hunting taboos and low degree of human impact. The largest chimpanzee populations are nowadays found under the third social-ecological configuration, even though most of these areas are not officially protected. Most commonly chimpanzee conservation has been based on exclusion of threats by creation of protected areas and law enforcement. Our findings suggest, however, that this approach should be complemented by an additional focus on threat reduction, i.e., interventions that directly target individual human behavior that is most threatening to chimpanzees, which is hunting. Although changing human behavior is difficult, stakeholder co-designed behavioral change approaches developed in the social sciences have been used successfully to promote pro-environmental behavior. With only a fraction of chimpanzees and primates living inside protected areas, such new approaches might be a way forward to improve primate conservation.
Introduction
With continued human population growth and the associated expansion of human-dominated areas, 75% of land surface areas have been anthropogenically modified (Ellis and Ramankutty, 2008). Human disturbance resulted in an average population decline of 25% for terrestrial vertebrates and of 45% for the majority of invertebrates, with the main drivers being habitat destruction, overexploitation, and invasive species (Dirzo et al., 2014). However, in some instances, species are able to persist and co-exist with humans (Gardner et al., 2009). Threats to species and the resulting species decline are relatively well studied, but considerably less is known about the social-ecological conditions under which some species tend to persist while others disappear.
One approach to understand why species are persisting at certain sites is to understand the heterogeneity across populations with a focus on analyzing positive outliers (Post and Geldmann, 2018). Similarities between areas where species are doing exceptionally well, called exceptional responders (Post and Geldmann, 2018), bright spots (Cinner et al., 2016), or positive deviants (Marsh et al., 2004), could highlight novel solutions to conservation challenges (Cinner et al., 2016; Post and Geldmann, 2018). While this approach has been used widely in medicine and social sciences, applications in ecology and conservation are still rare (Cinner et al., 2016; Frei et al., 2018). Applied to species conservation this approach entails identifying those social-ecological conditions in which a species is likely to persist.
Primates are a taxon that is strongly impacted by anthropogenic factors, and despite their social, cultural, and ecological importance, most populations are severely threatened (Estrada et al., 2017). However, evidence on the effectiveness of conservation interventions for primates remains scarce (Junker et al., 2017). Nonetheless, some primates, and great apes in particular, seem to be able to adapt to and persist in anthropologically impacted landscapes (Hockings et al., 2015; McCarthy et al., 2017; Spehar et al., 2018). The identification of conditions that are enabling species persistence can guide the design of conservation interventions that are mimicking these favorable conditions.
Here we focus on the western chimpanzee (Pan troglodytes verus), that occurs in a variety of social-ecological landscapes, meaning that different combinations of biophysical and socio-economic variables characterize their range (Liu et al., 2007). For example, western chimpanzees occur in isolated patches of intact habitat surrounded by human-dominated areas (e.g., Nimba mountains), protected primary rainforest (e.g., Taï National Park), or in agricultural landscapes with forest remnants (e.g., parts of Sierra Leone). A recent evaluation of their status showed a dramatic decline in abundance of 80% and a range reduction of 20% since 1990 (Kühl et al., 2017). This resulted in the up-listing of the species' IUCN status to Critically Endangered (Humle et al., 2016). However, from the 20 sites for which longitudinal data were available, Kühl et al. (2017) found that three sites were exceptions to the general trend of population decline, with two sites in Guinea and one in Côte d'Ivoire seeming to support stable populations. The aim of this study was to apply the positive deviance approach across the entire range of western chimpanzees, to identify social-ecological conditions that might enable chimpanzee persistence. For this we compiled a range-wide dataset of chimpanzee densities, identified drivers of chimpanzee density, and characterized social-ecological conditions across 66 sites.
Methods
General Workflow
We compiled a western chimpanzee density dataset covering the entire range of this taxon and extracted publicly available social-ecological data for all surveyed sites. We first determined which of the factors influenced chimpanzee densities significantly by fitting a Generalized Linear Mixed Model (McCullagh and Nelder, 1989; Baayen, 2008). After the model established which factors influenced chimpanzee densities significantly, we compared the configuration of significant social-ecological factors across a total of 66 sites.
Statistical Modeling
Model Response and Offset Term
Great ape density estimates are usually based on the counting of nests they built as resting places, instead of counting individuals themselves. Nests are more visible, more numerous, and do not move, meaning that there is no correlation between detectability of nests and intensity of threats (Kühl et al., 2008). Following a procedure that is commonly used for modeling ape densities, we used number of nests per transect as the model response and constructed an offset term to let the model output directly express chimpanzee densities (Murai et al., 2013; Wich et al., 2016; Voigt et al., 2018).
The number of nests was derived from chimpanzee nest surveys. In total we compiled 52 chimpanzee nest surveys across nine countries (Figure 1, Supplementary Table 1) via the IUCN SSC A.P.E.S. database (Kühl et al., 2007). These included line transect and reconnaissance surveys (Kühl et al., 2008) conducted between 2001 and 2015. We only included reconnaissance surveys for which the survey effort was known, based on GPS tracklog data. The entire dataset consisted of 17,109 transect or reconnaissance segments (hereafter referred to as “transect”) with a total survey effort of 10,929 km (mean transect length: 0.64 km, range: 0.02–14.00 km).
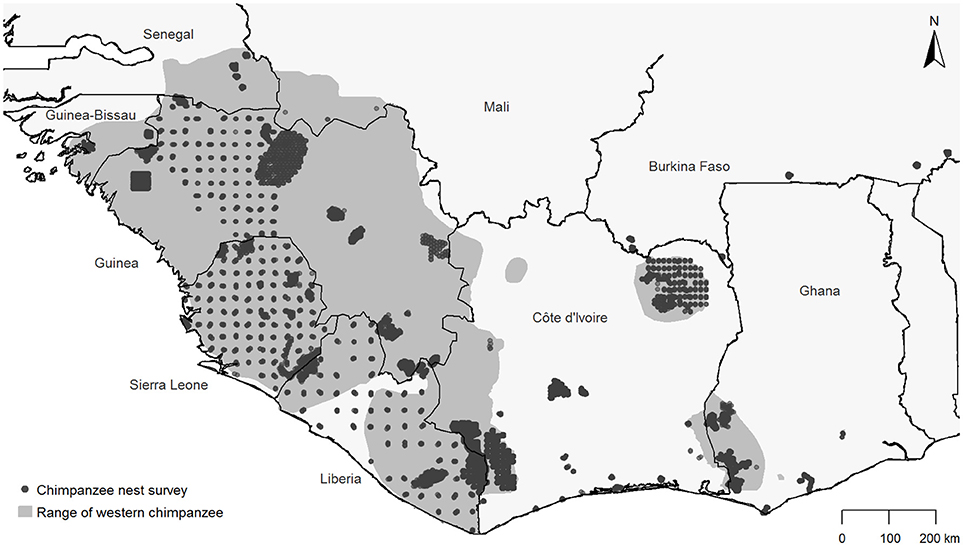
Figure 1. Location of the 52 chimpanzee nest surveys compiled for this study and the geographic range of western chimpanzees (Humle et al., 2016).
The offset term (McCullagh and Nelder, 1989) was calculated as D = N/(2*L*ESW*p*r*t) where D is chimpanzee density, N number of nests, L transect length, ESW effective strip width, p proportion of nest builders, r nest production rate, and t nest decay time (Kühl et al., 2008). Hence, the offset term in the model was the log of the denominator of the above equation [i.e., log(2*L*ESW*p*r*t)].
To determine the ESW we only used nests for which the perpendicular distance from the transect line was recorded (n = 12,728 nests), meaning that we did not use nest observations from reconnaissance surveys for this specific analysis. We determined the ESW separately for different habitat types to account for varying nest detectability due to habitat type. For nest observations the habitat type was typically recorded during the survey. To standardize habitat types across datasets we assigned them to the land cover categories defined by the Global land cover dataset (Friedl et al., 2010), namely “evergreen broadleaf forest,” “mixed forest,” “permanent wetlands,” “woody savanna,” “savanna,” “croplands,” “cropland natural vegetation mosaic.” For < 10% of nest observations the habitat was not recorded during the survey, and we extracted habitat type from satellite data (Global land cover dataset, Friedl et al., 2010). To get approximately balanced sample sizes for each habitat type we pooled habitat type to three categories: forest (“evergreen broadleaf forest,” “mixed forest,” “permanent wetlands”), savanna (“savannah,” “woody savannah,” “closed shrubland”), and cropland (“cropland,” “cropland/natural vegetation mosaic”). We determined the ESW using DISTANCE (6.2 Release 1, Thomas et al., 2010, further details in Supplementary Material, Supplementary Tables 2, 3, Supplementary Figure 1). We then extracted the habitat type for an area of 0.5 km around each transect from the Global land cover dataset (Friedl et al., 2010), and used the same assignment to the three habitat categories as above. Lastly, we assigned the habitat-specific ESW to each transect. ESW assignment for transects that traversed more than one habitat type was based on a majority vote.
Nest decay times vary between sites and seasons and are influenced by rainfall (Walsh and White, 2005; Kühl et al., 2008; Kouakou et al., 2009). Hence, we first fitted models to determine the mean nest decay time separately for each of the eight nest decay datasets and then fitted a separate model to estimate decay time as a function of rainfall. We then assigned a nest decay time to each transect based on that model and the mean rainfall at the respective transect. The model revealed a minimum fitted decay time of 85.45 days and a maximum fitted decay time of 229.03 days. Model uncertainty was assessed by deriving 10,000 bootstraps (additional details on nest decay methods and results in Supplementary Tables 4, 5, Supplementary Figures 2, 3). We used a proportion of nest builders of 0.83 (Plumptre and Cox, 2006) and a nest production rate of 1.143 (Kouakou et al., 2009).
Model Predictors
We modeled chimpanzee density as a function of different social-ecological factors relevant for chimpanzee abundance, such as habitat, climate, topography, and socio-economic context. For this we used 19 predictor datasets that were available for the entire study area (predictor descriptions, temporal and spatial resolution of datasets detailed in Table 1). For quantitative predictors we calculated the mean of values within a fixed extraction radius around each transect, and for categorical predictors we determined the proportion of each category within the extraction radius. We transformed predictors when necessary to derive approximately symmetric distributions (details on predictor data extraction, transformation, and post-processing in Supplementary Table 6).
Spearman correlations among predictors indicated that some predictors were highly interrelated (Supplementary Table 7); hence, we used factor analyses to reduce redundancy among them, which resulted in three factors. On the factor that we termed “environment” tree cover and vegetation height loaded negatively, while savanna, temperature, and rainfall seasonality loaded positively (Supplementary Table 8). On the factor termed “socio-economic status” education and corruption control loaded positively, while poverty and malnourishment loaded negatively. On the factor termed “human activity” settlements, human density, nighttime light, and conflicts loaded positively (details on factor analyses in Supplementary Table 9). We did not include the variables cropland, forest loss, hunting taboo, slope, river, and road in the factor analyses either because they did not load strongly on any factor with Eigenvalue ≥1, or because it was the only predictor that loaded strongly on a factor. We therefore included them as separate predictors in the model.
We differentiated between test and control predictors (Mundry, 2014), and included 13 model terms as test predictors into the model (details on anticipated effects, also for interactions and squared terms, in Table 2). Distance to the closest river and road were included as control predictors, because they are known to influence animal densities (Boesch et al., 2017). All predictors were standardized to a mean of zero and a standard deviation of one to facilitate comparability of model parameters and ease interpretation of interactions (Schielzeth, 2010).
Model Implementation
We fitted a Generalized Linear Mixed Model (McCullagh and Nelder, 1989; Baayen, 2008) with a negative binomial error distribution and log link function, because the response was highly skewed with no nest observations on most transects (90.24%) and a large number of nests on some transects (range number of nests per transect: 0–430). The latter speaks against a Poisson distribution. With ape surveys being very cost and time intensive, they are usually targeted toward areas with possible chimpanzee presence, and hence we decided against a zero-inflated error distribution.
We included an autocorrelation term into the model to account for spatial autocorrelation, i.e., nest counts from transects that were closer to one another were more similar, even after accounting for the predictors in the model, and therefore the model residuals were not independent (details on implementation in Supplementary Material). The full model was:
number of nests per transect ~ cropland + date + environment + forest loss + human activity + hunting taboo + slope + socio-economic status + socio-economic status 2 + human activity:hunting taboo + human activity:slope + human activity:socio-economic status + human activity:socio-economic status 2 + hunting taboo:slope + hunting taboo:socio-economic status + hunting taboo:socio-economic status 2 + river + road + spatial autocorrelation + offset term.
Chimpanzee densities are likely to differ among countries. This can be due to past events, for example chimpanzee densities are likely to be lower in Sierra Leone due to excessive chimpanzee captures in the 70's and 80's (Hanson-Alp et al., 2003). To account for these between-country differences and to control for the non-independence of data points from the same country, i.e., pseudoreplication, we included country as a random effect.
Additionally, the strength of effects can differ among countries, i.e., the slopes of the response against the predictor. For example, in a country with high poaching intensity, forests have lower than expected mammal densities, so that the positive effect of forests on mammal density will be smaller than in a country with less intense poaching. Such country-specific differences in poaching intensity can have many reasons among which could be differences in law enforcement capacity, or access to alternative protein sources or livelihoods. Consequently, we included the random slopes of all predictors within country (Schielzeth and Forstmeier, 2009; Barr et al., 2013).
The check of model assumptions revealed overdispersion (dispersion parameter = 1.71), causing standard errors to be underestimated. We corrected for this by adjusting the estimated standard errors and then re-determining z- and p-values (Gelman and Hill, 2007). We also tested for multicollinearity and found that it was not an issue (details on implementation in Supplementary Material, Supplementary Table 10, Supplementary Figure 4).
To test the significance of fixed effects as a whole, we compared the fit of the full model with that of a null model lacking all test predictors, but comprising the same control predictors and random effects structure as the full model (Forstmeier and Schielzeth, 2011) using a likelihood ratio test (Dobson and Barnett, 2008). All models were fitted with the R function “glmer.nb” of the R package “lme4” (version 1.1–11, Bates et al., 2015). Model stability was assessed by comparing model estimates based on all data, with model estimates based on data excluding countries one at a time. The model was stable regarding the effects of all significant predictors (minimum and maximum estimates in Table 3). To derive confidence limits, we fitted parametric bootstraps. For this, we randomly selected one nest decay bootstrap, determined the fitted nest decay rate, and derived an adjusted offset term. We then fitted the full model with the new offset term and derived one bootstrap with the R function “bootMer” (package “lme4”). This was repeated 1,000 times. Unless specified otherwise, all analyses were implemented in R (version 3.2.3, R Core Team, 2015).
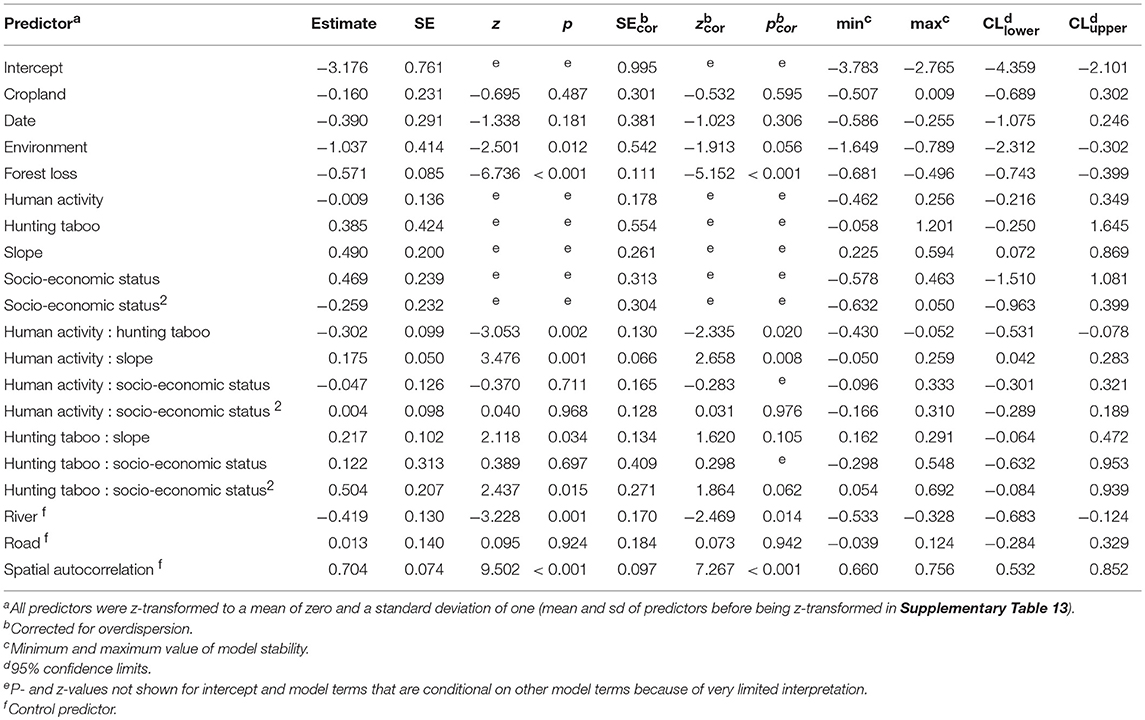
Table 3. Results of the full model to identify drivers of chimpanzee densities (Generalized Linear Mixed Model, n = 17,109).
Characterization of Positive Deviant Sites
We assigned the transects to 19 of the 20 sites delineated in the previous study on western chimpanzee population trends (Kühl et al., 2017). We did not have data for Mount Péko in Côte d'Ivoire, but it is thought that chimpanzees are now extirpated there (Kühl et al., 2017). The remaining transects covered 47 additional sites, usually according to protected area delineation. For each of the total 66 sites and each factor that was significant in the statistical model we calculated the median, lower and upper quartile of the predictor variable. We then compared characteristics for the three sites that were previously identified as having stable populations, namely Fouta Djallon and Sangaredi in Guinea and Cavally in Côte d'Ivoire (Kühl et al., 2017). We also analyzed sites with transects that deviated by more than two standard deviations from the mean chimpanzee density of transects with chimpanzee presence, as suggested by Post and Geldmann (2018). Due to lower densities of feeding trees, chimpanzee densities are naturally lower in savanna-mosaics than in rainforest habitat (Pruetz and Bertolani, 2009). As chimpanzees have adapted to these challenging conditions (Wessling et al., 2018a,b), low chimpanzee densities in these habitats do not imply population decline. We therefore, focus interpretation of results on populations which have been shown to have remained stable (Kühl et al., 2017) and also ordered sites in Figure 3 according to environmental conditions.
Results
In total, 13,464 nests were recorded. Estimated chimpanzee density ranged between 0.00 and 46.33 individuals/km2, with average densities of 0.14 ± 0.93 individuals/km2 (mean ± SD) across all transects and average densities of 1.42 ± 2.67 individuals/km2 on transects with chimpanzee presence.
Statistical Model
The full model explained chimpanzee density significantly better than the null model (likelihood ratio test comparing full model and null model without test predictors: χ2 = 40.28, df = 16, p < 0.001, model results in Table 3, random effects and random slopes in Supplementary Tables 11, 12). The factor “environment” had a marginally significant negative effect, with lower chimpanzee densities in dry and sparsely forested areas, and higher chimpanzee densities in rainforest habitat (Table 3). “Forest loss” had a significant negative effect on chimpanzee density. Except for two transects in Marahoué National Park, Côte d'Ivoire, chimpanzee nests were only found on transects with < 10% forest loss (Figure 2A). We also found a positive synergistic effect of human activity and slope. Chimpanzee densities were higher in areas with low intensities of human activity. However, in areas characterized by relatively high intensities of human activity, chimpanzee densities were higher in steeper terrain (Figure 2B). There was also a positive synergistic effect of human activity and hunting taboo, with the highest chimpanzee densities in areas with a high prevalence of hunting taboos and low degree of human activity. Socio-economic status had no significant effect (Table 3), i.e., chimpanzee density was not significantly influenced by education, poverty or malnourishment levels. Cropland did not have a significant effect on chimpanzee densities, but highest densities were recorded on transects with <25% cropland. Overall, absolute model estimates showed that the factor “environment” had the strongest influence on chimpanzee densities, followed by “forest loss” and “slope”.
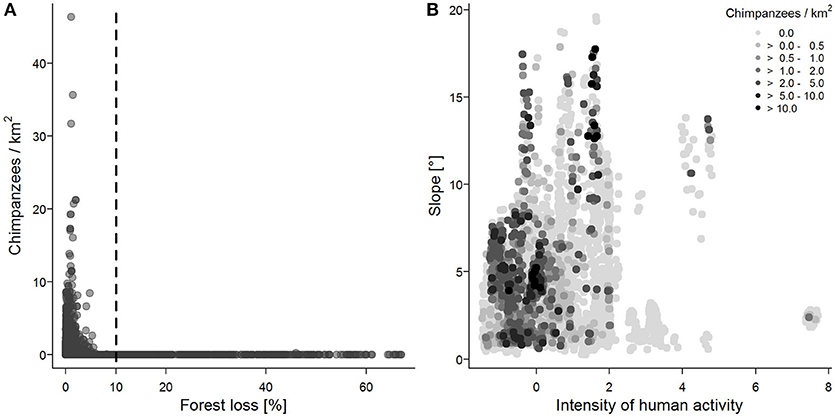
Figure 2. Chimpanzee density as a function of (A) forest loss (data points drawn in transparent colors to visualize overlapping points), and (B) slope and human activity. Chimpanzee nests were found on transects with <10% forest loss (dashed vertical line). Chimpanzees mainly occurred on transects with low intensity of human activity, but in areas with higher human activity higher chimpanzee densities were found in steeper areas, i.e., a refuge effect.
Characteristics of Positive Deviant Sites
In addition to the three sites identified as exceptional based on stable populations in a previous study (Kühl et al., 2017), we identified five sites with transects with very high estimated chimpanzee densities (>6.76 individuals/km2 corresponding to the mean+2SD), namely Tai (Côte d'Ivoire), Grebo (Liberia), Nimba (Côte d'Ivoire and Guinea part), and Boé (Guinea-Bissau, Figure 3, Supplementary Figure 5). Characterization of those sites could be grouped into three social-ecological configurations. The first group was characterized by rainforest habitats with low degree of forest loss and low intensity of human activity, e.g., Cavally (Côte d'Ivoire), Grebo (Liberia), and Tai (Côte d'Ivoire). The second group was characterized by steep terrain, e.g., Nimba mountains in Guinea and Côte d'Ivoire. The third was characterized by a high prevalence of hunting taboos and low intensity of human activity, e.g., Boé in Guinea-Bissau, and Fouta Djallon and Sangaredi in Guinea (Figure 3). Nimba (Liberian part), Gola (Sierra Leone), and Goin-Débé (Côte d'Ivoire) also each had one transect with exceptionally high chimpanzee densities, but as this was only one transect each, we did not base any conclusions on these three sites.
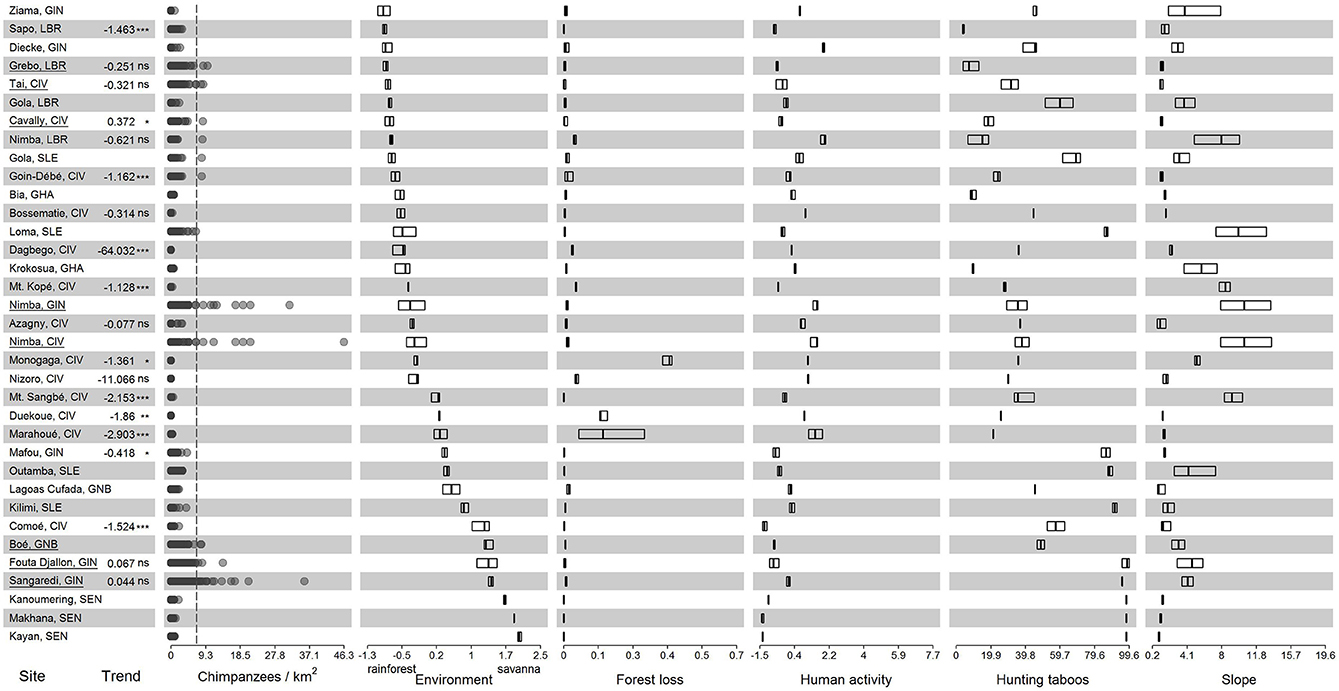
Figure 3. Chimpanzee densities and significant factors from statistical model plotted for all sites analyzed in Kühl et al. (2017) and additional sites included in our dataset (additional sites Supplementary Figure 5) all sorted according to environment from rainforest habitat (top) to savanna habitat (bottom). Positive deviant sites are underlined. Population trend estimates are based on Kühl et al. (2017) and were only available for certain sites. In the chimpanzee density panel data points are drawn in transparent gray, so that overlapping data points appear in a darker shade, meaning sites with low chimpanzee density do not necessarily have less data points. The vertical dashed line in the chimpanzee density panel marks the threshold for exceptional density [6.76 individuals/km2 corresponding to the mean + 2SD as suggested by Post and Geldmann (2018)]. Due to the data being based on nest count surveys individual transects with very high chimpanzee densities likely indicate favored sleeping sites, while surrounding areas are likely characterized by less favorable conditions. Horizontal axes depict the range of values in the dataset. Bars represent the lower and upper quartiles and thick vertical lines the median. Country names are abbreviated as CIV, Côte d'Ivoire; GHA, Ghana; GIN, Guinea; GNB, Guinea-Bissau; LBR, Liberia; SEN, Senegal and SLE, Sierra Leone.
Discussion
In our study we found that three configurations of social-ecological factors enabled chimpanzee persistence: rainforest habitat with low degree of human impact, steep areas, and areas with a high prevalence of hunting taboos and low degree of human impact. While the conditions of the first and second configuration are mirrored in conservation interventions aiming at threat exclusion, such as the expansion of protected areas and law enforcement, conservation interventions focusing on threat reduction, as reflected in the third configuration, are still very rare in primate conservation.
Social-Ecological Conditions Enabling Chimpanzee Persistence
The results underlined the importance of intact habitat for chimpanzee persistence, as chimpanzees seemed to only be able to tolerate a surprisingly low threshold of a maximum of 10% forest loss (Figure 2A). Habitat loss not only implies a loss of feeding and nesting trees, but often also an increase in other disturbances, such as hunting or human-wildlife conflicts (Estrada et al., 2017). This is in line with previous findings that chimpanzees are sensitive to habitat disturbance (Junker et al., 2015; Morgan et al., 2018). At those sites in our dataset for which we found high forest loss levels, such as Monogaga (median forest loss: 40.88%), Duékoué (14.19%) and Marahoué (15.20%) in Côte d'Ivoire, chimpanzees are now thought to be extirpated (Campbell et al., 2008; Kühl et al., 2017). In contrast, median forest loss ranged between 0.09 and 1.51% at sites with exceptional chimpanzee densities. Consequently, very low levels of forest loss seem to be a prerequisite for chimpanzee persistence.
Regarding the three social-ecological configurations enabling chimpanzee persistence, we first found exceptionally high chimpanzee densities at rainforest sites with low human activity. The low level of human activity in some of these areas is due to conservation interventions, such as law enforcement, presence of researchers and NGOs, which have a scientifically proven positive effect on great ape persistence (Campbell et al., 2011; Tranquilli et al., 2012; Tagg et al., 2015). For other sites in this category, the relative remoteness and the large distances to the next city (Weiss et al., 2018) might have enabled chimpanzee persistence, as it has been shown that increased market integration has a negative influence on chimpanzee densities (Boesch et al., 2017).
Second, exceptionally high chimpanzee densities were found in steep terrain, especially when surrounding areas were strongly impacted by humans (Figure 2B). The steep terrain likely reduced access for humans, as has been found elsewhere (Adanu et al., 2011; Sesink Clee et al., 2015), and such areas are less favorable for conversion to other land-uses (Kinnaird et al., 2003; Silva et al., 2007), so that they seem to function as refuge areas for chimpanzees. In savanna environments, steep terrain also seem to be favored sleeping sites due to the higher tree cover and access to water sources (Pintea and Plumptre, 2006). Consequently, individual transects with very high densities may indicate favored sleeping sites, while surrounding areas are likely characterized by less favorable conditions. For those sites in our dataset with steep terrain and high chimpanzee densities, including the Nimba mountains in Guinea and Côte d'Ivoire, and to a lesser extent Loma mountains in Sierra Leone, the population trend is not known. Chimpanzees might be restricted to small refuge areas, and their long-term survival at those refuges could be constrained due to reduced dispersal possibilities and increased vulnerability, for example to diseases. This is illustrated by examples from Côte d'Ivoire such as Mount Kopé and Mount Sangbé for which strong population declines have been shown (Kühl et al., 2017). While these mountains are isolated areas of steep terrain, there are regions where extensive areas are characterized by relatively steep slopes, for example the Fouta Djallon (Figure 3, Supplementary Figure 6). Here, loss in dispersal ability is less likely to be of concern for chimpanzee survival. In addition, mineral exploitation poses a threat as mountains often contain mineral deposits, and several mining sites operate in the Nimba mountains, entailing further threats to wildlife such as infrastructure expansion (Edwards et al., 2014).
The third social-ecological configuration was characterized by relatively low levels of human activity in combination with a high prevalence of hunting taboos at sites characterized by higher proportions of savanna habitat. It was surprising to find that this group of sites not only has seemingly stable populations (Kühl et al., 2017), but also exceptionally high chimpanzee densities, despite the fact that our analysis showed that overall chimpanzee densities are lower in savanna areas compared to rainforest areas (Table 3). It appears that the adherence to hunting taboos by humans reduced hunting pressure on chimpanzees, and thereby the key threat to chimpanzees was removed. This is in accordance with a site-based sociological study from Côte d'Ivoire that showed that people adhering to hunting taboos generally do not eat primate meat (Bachmann et al. submitted). These important chimpanzee areas are now partly protected by the recently established Boé National Park (Guinea-Bissau) and the Moyen Bafing National Park (Guinea) that is currently being created. However, large-scale open pit mining is underway at Sangaredi (Guinea), and the current status of the chimpanzee communities there is unclear.
However, there are exceptions to these patterns, i.e., there are sites which are characterized by one of the above-mentioned combination of factors but still have low chimpanzee densities or decreasing populations. This shows that additional factors for which data were not available across the entire study area and could therefore not be included in our analysis might influence chimpanzee persistence, for example absence of diseases (Ryan and Walsh, 2011). Also, historic events, such as intensive hunting in the past at specific sites (Hanson-Alp et al., 2003), might influence current chimpanzee densities. Due to their slow life history apes are especially susceptible to such threats and communities can take decades to recover from single mortality events (Ryan and Walsh, 2011). In addition, due their behavioral flexibility, chimpanzees might be able to adapt to savanna and anthropogenic habitat mosaics and persist there long-term, though likely at lower densities (Hockings et al., 2015). For example, it has been shown that although chimpanzees at Fongoli, Senegal, a site with strong seasonality in temperature and rainfall, experience heat and dehydration stress, chimpanzees likely developed mechanisms for avoiding costs of energetic constraint (Wessling et al., 2018b). However, these types of landscapes are still less surveyed, and longitudinal data from more sites are needed to determine the population trend, especially from Guinea-Bissau, Mali, Senegal, and Sierra Leone for which such data are lacking. Consequently, this study can only be regarded as a first step and follow-up studies are needed to substantiate our findings.
Threat Reduction Through Behavioral Change
Our study revealed three factors having a positive effect on chimpanzee densities; habitat protection, reduced accessibility, and hunting taboos. The first two factors are already reflected in commonly implemented conservation interventions, such as protected areas, law enforcement, and the presence of researchers, NGOs and tourism activities, which have also been shown to have a positive effect on ape persistence (Campbell et al., 2011; Tranquilli et al., 2012; Strindberg et al., 2018). The mechanism underlying those activities is threat exclusion, meaning threats are excluded from delineated areas. In contrast, the positive effect of hunting taboos is based on a different mechanism, namely the reduction of a threat, in this case due to a particular human behavior. While threat exclusion addresses the symptoms of conservation challenges, threat reduction aims to focus on the root causes. Previous studies argued that for conservation to be successful, threats need to be actively reduced (Allison et al., 1998; Clout, 2001; Challender and MacMillan, 2014; Crees et al., 2016). Considering that only a small proportion of chimpanzees are living in protected areas (Kühl et al., 2017), conservation interventions reducing threats outside of protected areas are needed, that thereby also reduce the pressure on protected areas. However, in a recent compilation of available evidence for the effectiveness of conservation interventions for primates, the majority of interventions was aimed at threat exclusion, for example through protected areas, law enforcement, and species management (Junker et al., 2017). There is considerably less evidence for interventions targeting threat reduction (Junker et al., 2017).
While the positive effect of hunting taboos we found for chimpanzees cannot be directly transferred to other areas, conservation interventions mimicking these conditions could complement current conservation efforts. The positive effect of hunting taboos is a challenge for conservationists, because they generally have a religious or a supernatural basis, both in their origin and in their maintenance (Colding and Folke, 2001). While taboos can be strengthened or reinforced where they already exist (Junker et al., 2017), they cannot simply be introduced to other areas, where they never existed or disappeared. An additional concern is the loss of power of traditional taboos through modernization and migration, with people from different cultural or religious background being less likely to accept local taboos (Golden and Comaroff, 2015).
Mimicking hunting taboos would mean to reduce the demand for chimpanzees by consumers and discourage the supply by hunters and traders. From a consumer perspective, chimpanzees are not a notable protein source, and the provision of alternative protein sources is a common intervention aimed at reducing the economic incentive to consume bushmeat, including chimpanzee. Junker et al. (2015) have, for example, shown that affordable fish protein correlated positively with chimpanzee densities. Another important conservation intervention includes awareness raising activities, especially because in certain areas medicinal or magical properties are assigned to chimpanzee parts and chimpanzee bone powder (Hanson-Alp et al., 2003). There are studies that have shown a positive effect of such interventions on bushmeat consumption, for example in the context of repeated multimedia campaigns (Kouassi et al., 2017) and Ebola information campaigns (Ordaz-Németh et al., 2017). However, hunting chimpanzees is also strongly driven by a demand for chimpanzee parts and live animals from urban areas and even international markets (Kuehl et al., 2009; Greengrass, 2016; Strindberg et al., 2018). Awareness raising activities at national or even regional scale specifically targeting urban consumers is absent from West Africa, but could be an important tool to reduce the acceptability of chimpanzee consumption. Evidence from China suggests that an ambitious nation-wide awareness raising campaign championed by the most popular Chinese athlete, Yao Ming, resulted in a change in government policy and a strong decrease in shark fin demand across China (Whitcraft et al., 2014). In general, research on behavioral change in conservation highlights the need to go beyond awareness raising because often a change in awareness alone is not enough to lead to pro-environmental behavior (Schultz, 2011; Amel et al., 2017). Stakeholder co-designed behavioral change tools therefore try to identify barriers to behavioral change as well as providing benefits (Schultz, 2014). Successful examples of behavioral change interventions aimed at reducing bushmeat consumption include the so-called community-based social marketing tool, that has been implemented to reduce consumer demand for wild meat in a Brazilian town, and that explicitly identified and then reduced barriers to the consumption of domesticated meat (Chaves et al., 2018).
From a supplier perspective, chimpanzees are mostly killed or captured opportunistically, but because of their large size, hunters make high profits from a single catch, and young chimpanzees can be sold for the pet trade (Hanson-Alp et al., 2003). Even such single catches can have detrimental effects on chimpanzee populations due to their long time to maturation and long inter-birth intervals. As discussed above, law enforcement aiming to exclude hunters from certain areas often seems not to be sufficient, mainly due to the virtual impossibility of stopping every single hunter. Conservation interventions aiming at reducing chimpanzee supply are scarce, and here again stakeholder co-designed behavioral change tools might be a way forward to first understand what is driving certain behaviors and how hunters could be motivated to not kill or capture chimpanzees despite their high monetary value. While there is evidence that monetary and non-monetary benefits can have a positive effect on primate populations, there are also studies showing no effect (Junker et al., 2017). In addition, studies looking at the entire supply chain from individual hunters via traders to sellers have identified multiple entry points for conservation interventions (Bachmann et al., submitted).
Application of the Positive Deviance Approach to Other Study Systems and Challenges
The positive deviance approach can be a useful tool for conservation science because it focuses on identifying conditions or mechanisms that have already proven to work. While understanding threats to species is a prerequisite for conservation planning, solutions are often a lot less understood. The positive deviance approach allows directing research toward possible answers to conservation challenges. In general, this approach can be applied to any taxon, region and at different spatial scales, if matched with data of corresponding resolution and quality. Importantly, the spatial scale needs to be chosen so that there is sufficient variation along multiple predictor variables.
Similarly to Frei et al. (2018) who applied this approach to agricultural landscapes, we found that applying it to a specific species comes with several challenges. First, it is difficult to differentiate between the influence of historic and current conditions, i.e., past events such as disease outbreaks might have long-lasting effects on a population independent of current conditions. This is of particular concern for species with slow life histories. Second, the data, especially when it pertains to human behavior or socio-economic context, might not be available at a small resolution for a large area, which makes large-scale analyses difficult. Here, multi-scale studies might give additional insights. In general, many more studies using the positive deviance approach would be needed to determine whether this is truly a useful approach that can provide novel insights for species conservation.
Conclusions
Conservation interventions, especially for the conservation of primates, still largely focus on habitat protection and reducing accessibility for humans through protected areas and law enforcement. However, with about 80% of western chimpanzees living outside of high-level protected areas, i.e., national parks and strict nature reserves, the focus on excluding threats from delineated areas might not be sufficient to ensure the long-term survival of western chimpanzees. By using the positive deviance approach, we found high chimpanzee densities and seemingly stable population trends for sites with a high prevalence of hunting taboos, even though those areas were not set aside under any high-level protective status. This suggests that these enabling conditions can be mimicked by using stakeholder co-designed behavioral change approaches (Schultz, 2011, 2014; Chaves et al., 2018) to reduce hunting pressure and thereby complement current conservation interventions. While new behavioral change tools have been applied to different environmental problems, they remain largely absent from primate conservation (Junker et al., 2017). With a lot of organizations already working for the protection of chimpanzees across West Africa and the relatively strong support that chimpanzee protection garners within and outside its range, this might be an opportunity to pioneer and test new conservation approaches, which, if successful, could inform protection of other primates. Applications of the positive deviance approach to species conservation are still rare, and many more studies and methodological advancements would be needed to establish this method as a useful conservation science tool.
Data Availability
The raw data will be made available via the IUCN SSC A.P.E.S. database (http://apesportal.eva.mpg.de).
Author Contributions
HK, SH, RM, and CB conceived and designed the study; SH and TS curated the data; SH and RM analyzed the data; SH and HK wrote the initial draft; all remaining authors contributed data, and provided comments on model formulation. All authors contributed to the reviewing and editing of the manuscript, and approved its submission.
Conflict of Interest Statement
GC was affiliated with the Max Planck Institute for Evolutionary Anthropology, Leipzig, Germany during the time of research and is now employed by company The Biodiversity Consultancy Ltd., Cambridge, UK.
The remaining authors declare that the research was conducted in the absence of any commercial or financial relationships that could be construed as a potential conflict of interest.
The handling editor declared a collaboration with two of the authors, JJ and HK.
Acknowledgments
We would like to thank all governments and national authorities in the chimpanzee range countries in West Africa for providing permission for data collection. We also thank the field staff of Chimbo Foundation, Conservation International, Conservation Society of Sierra Leone, Fauna and Flora International, Jane Goodall Institute Spain, Royal Society for the Protection of Birds, Pan African Programme, Society for the Conservation of Nature of Liberia, Tacugama Chimpanzee Sanctuary, West African Primate Conservation Action, Wild Chimpanzee Foundation, and World Wide Fund for Nature for collecting survey data, and all funding organizations for funding these surveys. We further thank Thomas Esch for providing Global Urban Footprint data for West Africa, Julia Riedel for help with data curation and Mona Bachmann for insightful discussions on drivers of bushmeat hunting. We thank Marc Ancrenaz and Alex Piel for their helpful comments on the manuscript. We would like to thank the Max Planck Society and Robert Bosch Foundation for funding and support.
Supplementary Material
The Supplementary Material for this article can be found online at: https://www.frontiersin.org/articles/10.3389/fevo.2019.00016/full#supplementary-material
References
Adanu, J., Sommer, V., and Fowler, A. (2011). “Hunters, fire, cattle: conservation challenges in Eastern Nigeria, with special reference to chimpanzees,” in Primates of Gashaka: Socioecology and Conservation in Nigeria's Biodiversity Hotspot Developments in Primatology: Progress and Prospects, eds V. Sommer and C. Ross (New York, NY: Springer), 55–99. doi: 10.1007/978-1-4419-7403-7_3
Alkire, S., and Robles, G. (2015). Multidimensional Poverty Index 2015: Brief Methodological Note and Results. OPHI Brief. 31 Univ. Oxf
Allison, G. W., Lubchenco, J., and Carr, M. H. (1998). Marine reserves are necessary but not sufficient for marine conservation. Ecol. Appl. 8, S79–S92. doi: 10.1890/1051-0761(1998)8[S79:MRANBN]2.0.CO;2
Amel, E., Manning, C., Scott, B., and Koger, S. (2017). Beyond the roots of human inaction: fostering collective effort toward ecosystem conservation. Science 356, 275–279. doi: 10.1126/science.aal1931
Baayen, R. H. (2008). Analyzing Linguistic data: A Practical Introduction to Statistics Using R. Cambridge: Cambridge University Press doi: 10.1017/CBO9780511801686
Barr, D. J., Levy, R., Scheepers, C., and Tily, H. J. (2013). Random effects structure for confirmatory hypothesis testing: Keep it maximal. J. Mem. Lang. 68, 255–278. doi: 10.1016/j.jml.2012.11.001
Bates, D., Maechler, M., Bolker, B., and Walker, S. (2015). Fitting linear mixed-effects models using lme4. J. Stat. Softw. 67, 1–48. doi: 10.18637/jss.v067.i01
Boesch, L., Mundry, R., Kühl, H. S., and Berger, R. (2017). Wild mammals as economic goods and implications for their conservation. Ecol. Soc. 22:36. doi: 10.5751/ES-09516-220436
Campbell, G., Kuehl, H., Diarrassouba, A., N'Goran, P. K., and Boesch, C. (2011). Long-term research sites as refugia for threatened and over-harvested species. Biol. Lett. 7, 723–726. doi: 10.1098/rsbl.2011.0155
Campbell, G., Kuehl, H., N'Goran Kouam,é, P., and Boesch, C. (2008). Alarming decline of West African chimpanzees in Côte d'Ivoire. Curr. Biol. 18, R903–R904. doi: 10.1016/j.cub.2008.08.015
CCAPS (2013). Sub-national African Education and Infrastructure Access Data, Climate Change and African Political Stability Program, Robert S. Strauss Center for International Security and Law, University of Texas, Austin, TX. Available online at: https://www.strausscenter.org/ccaps-content/climate-vulnerability-model.html (Accessed October 30, 2015).
Challender, D. W. S., and MacMillan, D. C. (2014). Poaching is more than an enforcement problem. Conserv. Lett. 7, 484–494. doi: 10.1111/conl.12082
Chaves, W. A., Valle, D. R., Monroe, M. C., Wilkie, D. S., Sieving, K. E., and Sadowsky, B. (2018). Changing wild meat consumption: an experiment in the central amazon, Brazil. Conserv. Lett. 11:e12391. doi: 10.1111/conl.12391
Cinner, J. E., Huchery, C., MacNeil, M. A., Graham, N. A. J., McClanahan, T. R., Maina, J., et al. (2016). Bright spots among the world's coral reefs. Nature 535, 416–419. doi: 10.1038/nature18607
Clout, M. (2001). Where protection is not enough: active conservation in New Zealand. Trends Ecol. Evol. 16, 415–416. doi: 10.1016/S0169-5347(01)02225-X
Colding, J., and Folke, C. (2001). Social taboos: “invisible” systems of local resource management and biological conservation. Ecol. Appl. 11, 584–600. doi: 10.1890/1051-0761(2001)011[0584:STISOL]2.0.CO;2
Crees, J. J., Collins, A. C., Stephenson, P. J., Meredith, H. M. R., Young, R. P., Howe, C., et al. (2016). A comparative approach to assess drivers of success in mammalian conservation recovery programs. Conserv. Biol. 30, 694–705. doi: 10.1111/cobi.12652
Danielson, J. J., and Gesch, D. B. (2011). Global Multi-Resolution Terrain Elevation Data 2010 (GMTED2010), U.S. Geological Survey Open-File Report 2011–1073. Available online at: https://lta.cr.usgs.gov/GMTED2010 (Accessed August 23, 2015). doi: 10.3133/ofr20111073
Davis, J. T., Mengersen, K., Abram, N. K., Ancrenaz, M., Wells, J. A., and Meijaard, E. (2013). It's not just conflict that motivates killing of orangutans. PLoS ONE 8:e75373. doi: 10.1371/journal.pone.0075373
de Onis, M., and Blössner, M. (2003). The World Health Organization global database on child growth and malnutrition: methodology and applications. Int. J. Epidemiol. 32, 518–526. doi: 10.1093/ije/dyg099
DiMiceli, C. M., Carroll, M. L., Sohlberg, R. A., Huang, C., Hansen, M. C., and Townshend, J. R. G. (2011). Vegetation Continuous Fields MOD44B, Collection 5, Percent Tree Cover. University of Maryland, College Park, MD. Available online at: http://landcover.org/data/vcf/ (Accessed August 19, 2015)
Dirzo, R., Young, H. S., Galetti, M., Ceballos, G., Isaac, N. J. B., and Collen, B. (2014). Defaunation in the Anthropocene. Science 345, 401–406. doi: 10.1126/science.1251817
Dobson, A. J., and Barnett, A. (2008). An Introduction to Generalized Linear Models, 3rd Ed. Boca Raton: CRC Press.
Edwards, D. P., Sloan, S., Weng, L., Dirks, P., Sayer, J., and Laurance, W. F. (2014). Mining and the African environment. Conserv. Lett. 7, 302–311. doi: 10.1111/conl.12076
Ellis, E. C., and Ramankutty, N. (2008). Putting people in the map: anthropogenic biomes of the world. Front. Ecol. Environ. 6, 439–447. doi: 10.1890/070062
Esch, T., Taubenböck, H., Roth, A., Heldens, W., Felbier, A., Thiel, M., et al. (2012). TanDEM-X mission—new perspectives for the inventory and monitoring of global settlement patterns. J. Appl. Remote Sens. 6, 061702–1. doi: 10.1117/1.JRS.6.061702
Estrada, A., Garber, P. A., Rylands, A. B., Roos, C., Fernandez-Duque, E., Fiore, A. D., et al. (2017). Impending extinction crisis of the world's primates: why primates matter. Sci. Adv. 3:e1600946. doi: 10.1126/sciadv.1600946
FAO (2005). Roads of the World (Vmap0). Available online at: http://www.fao.org/geonetwork (Accessed February 11, 2016).
FAO (2007). RWDB2 River-Surface Water Body Network. Available online at: http://www.fao.org/geonetwork (Accessed January 8, 2016).
Forstmeier, W., and Schielzeth, H. (2011). Cryptic multiple hypotheses testing in linear models: overestimated effect sizes and the winner's curse. Behav. Ecol. Sociobiol. 65, 47–55. doi: 10.1007/s00265-010-1038-5
Frei, B., Renard, D., Mitchell, M. G. E., Seufert, V., Chaplin-Kramer, R., Rhemtulla, J. M., et al. (2018). Bright spots in agricultural landscapes: Identifying areas exceeding expectations for multifunctionality and biodiversity. J. Appl. Ecol. 55, 1–13. doi: 10.1111/1365-2664.13191
Friedl, M. A., Sulla-Menashe, D., Tan, B., Schneider, A., Ramankutty, N., Sibley, A., et al. (2010). Global Land Cover MCD12Q1, Collection 5.1, IGBP Land Cover. Boston University, Boston, MA. Available online at: http://landcover.org/data/lc/ (Accessed August 20, 2015).
Gardner, T. A., Barlow, J., Chazdon, R., Ewers, R. M., Harvey, C. A., Peres, C. A., et al. (2009). Prospects for tropical forest biodiversity in a human-modified world. Ecol. Lett. 12, 561–582. doi: 10.1111/j.1461-0248.2009.01294.x
Gelman, A., and Hill, J. (2007). Data Analysis Using Regression and Multilevel/Hierarchical Models. Cambridge: Cambridge University Press.
Golden, C., and Comaroff, J. (2015). Effects of social change on wildlife consumption taboos in northeastern Madagascar. Ecol. Soc. 20:41. doi: 10.5751/ES-07589-200241
Greengrass, E. (2016). Commercial hunting to supply urban markets threatens mammalian biodiversity in Sapo National Park, Liberia. Oryx 50, 397–404. doi: 10.1017/S0030605315000095
Ham, R., and Carter, J. (1998). Population size and distribution of chimpanzees in the Republic of Guinea, West Africa. Rep. Eur. Commun. 29, 569–574.
Hansen, M. C., Potapov, P. V., Moore, R., Hancher, M., Turubanova, S. A., Tyukavina, A., et al. (2013). High-resolution global maps of 21st-century forest cover change. Science 342, 850–853. doi: 10.1126/science.1244693
Hanson-Alp, R., Bakarr, M. I., Lebbie, A., and Bangura, K. I. (2003). “Sierra Leone - Status surveys and recommendations,” in Regional Action Plan for the Conservation of Chimpanzees in West Africa (Washington DC: IUCN/SSC Primate Specialist Group and Conservation International), 77–87.
Hockings, K. J., McLennan, M. R., Carvalho, S., Ancrenaz, M., Bobe, R., Byrne, R. W., et al. (2015). Apes in the Anthropocene: flexibility and survival. Trends Ecol. Evol. 30, 215–222. doi: 10.1016/j.tree.2015.02.002
Humle, T., Boesch, C., Campbell, G., Junker, J., Koops, K., Kühl, H., et al. (2016). Pan troglodytes ssp. verus. The IUCN Red List of Threatened Species. Available online at: http://www.iucnredlist.org (Accessed February 15, 2017).
Johnson, T. M., and Grim, B. J. (2008). World Religion Database, Brill. Leiden; Boston, MA. Available online at: http://www.worldreligiondatabase.org (Accessed August 23, 2015).
Junker, J., Boesch, C., Mundry, R., Stephens, C., Lormie, M., Tweh, C., et al. (2015). Education and access to fish but not economic development predict chimpanzee and mammal occurrence in West Africa. Biol. Conserv. 182, 27–35. doi: 10.1016/j.biocon.2014.11.034
Junker, J., Kühl, H. S., Orth, L., Smith, R. K., Petrovan, S. O., and Sutherland, W. J. (2017). Primate Conservation: Global Evidence for the Effects of Interventions. Cambridge: University of Cambridge.
Kinnaird, M. F., Sanderson, E. W., O'Brien, T. G., Wibisono, H. T., and Woolmer, G. (2003). Deforestation trends in a tropical landscape and implications for endangered large mammals. Conserv. Biol. 17, 245–257. doi: 10.1046/j.1523-1739.2003.02040.x
Kouakou, C. Y., Boesch, C., and Kuehl, H. (2009). Estimating chimpanzee population size with nest counts: validating methods in Taï National Park. Am. J. Primatol. 71, 447–457. doi: 10.1002/ajp.20673
Kouassi, J. A. K., Normand, E., Koné, I., and Boesch, C. (2017). Bushmeat consumption and environmental awareness in rural households: a case study around Taï National Park, Côte d'Ivoire. Oryx First View. doi: 10.1017/S0030605317000333. [Epub ahead of print].
Kuehl, H. S., Nzeingui, C., Yeno, S. L. D., Huijbregts, B., Boesch, C., and Walsh, P. D. (2009). Discriminating between village and commercial hunting of apes. Biol. Conserv. 142, 1500–1506. doi: 10.1016/j.biocon.2009.02.032
Kühl, H. S., Maisels, F., Ancrenaz, M., and Williamson, E. A. (2008). Best Practice Guidelines for the Surveys and Monitoring of Great Ape Populations. Gland: IUCN. doi: 10.2305/IUCN.CH.2008.SSC-OP.36.en
Kühl, H. S., Sop, T., Williamson, E. A., Mundry, R., Brugière, D., Campbell, G., et al. (2017). The Critically Endangered western chimpanzee declines by 80%. Am. J. Primatol. 79:e22681. doi: 10.1002/ajp.22681
Kühl, H. S., Williamson, L., Sanz, C., Morgan, D., and Boesch, C. (2007). A.P.E.S., IUCN SSC A.P.E.S. database. Available online at: http://apesportal.eva.mpg.de/ (Accessed August 30, 2016)
Linard, C., Gilbert, M., Snow, R. W., Noor, A. M., and Tatem, A. J. (2012). Population distribution, settlement patterns and accessibility across Africa in 2010. PLoS ONE 7:e31743. doi: 10.1371/journal.pone.0031743
Liu, J., Dietz, T., Carpenter, S. R., Folke, C., Alberti, M., Redman, C. L., et al. (2007). Coupled human and natural systems. AMBIO J. Hum. Environ. 36, 639–649. doi: 10.1579/0044-7447(2007)36[639:CHANS]2.0.CO;2
Marsh, D. R., Schroeder, D. G., Dearden, K. A., Sternin, J., and Sternin, M. (2004). The power of positive deviance. BMJ Br. Med. J. Lond. 329:1177. doi: 10.1136/bmj.329.7475.1177
McCarthy, M. S., Lester, J. D., and Stanford, C. B. (2017). Chimpanzees (Pan troglodytes) flexibly use introduced species for nesting and bark feeding in a human-dominated habitat. Int. J. Primatol. 38, 321–337. doi: 10.1007/s10764-016-9916-y
McCullagh, P., and Nelder, J. A. (1989). Generalized Linear Models, 2nd edn. London: Chapman and Hall. doi: 10.1007/978-1-4899-3242-6
Mills, J. H., and Waite, T. A. (2009). Economic prosperity, biodiversity conservation, and the environmental Kuznets curve. Ecol. Econ. 68, 2087–2095. doi: 10.1016/j.ecolecon.2009.01.017
Morgan, D., Mundry, R., Sanz, C., Ayina, C. E., Strindberg, S., Lonsdorf, E., et al. (2018). African apes coexisting with logging: comparing chimpanzee (Pan troglodytes troglodytes) and gorilla (Gorilla gorilla gorilla) resource needs and responses to forestry activities. Biol. Conserv. 218, 277–286. doi: 10.1016/j.biocon.2017.10.026
Mundry, R. (2014). “Statistical issues and assumptions of phylogenetic generalized least squares,” in Modern Phylogenetic Comparative Methods and Their Application in Evolutionary Biology, ed L. Z. Garamszegi (Berlin, Heidelberg: Springer), 131–153. doi: 10.1007/978-3-662-43550-2_6
Murai, M., Ruffler, H., Berlemont, A., Campbell, G., Esono, F., Agbor, A., et al. (2013). Priority areas for large mammal conservation in Equatorial Guinea. PLoS ONE 8:e75024. doi: 10.1371/journal.pone.0075024
NOAA (2013). DMPS-OLS Nighttime Lights Time Series Imagery, Version 4. National Oceanic and Atmospheric Administration, Washington DC. Available online at: http://ngdc.noaa.gov/eog/dmsp/downloadV4composites.html (Accessed January 8, 2016).
Ordaz-Németh, I., Arandjelovic, M., Boesch, L., Gatiso, T., Grimes, T., Kuehl, H. S., et al. (2017). The socio-economic drivers of bushmeat consumption during the West African Ebola crisis. PLoS Negl. Trop. Dis. 11:e0005450. doi: 10.1371/journal.pntd.0005450
Pintea, L., and Plumptre, A. J. (2006). “Prediction of suitable habitat for chimpanzees using remote sensing and GIS,” in Surveys of Chimpanzees and Other Biodiversity in Western Tanzania (Report submitted to USFWS, Great Apes Fund).
Plumptre, A. J., and Cox, D. (2006). Counting primates for conservation: primate surveys in Uganda. Primates 47, 65–73. doi: 10.1007/s10329-005-0146-8
Post, G., and Geldmann, J. (2018). Exceptional responders in conservation. Conserv. Biol. 32, 576–583. doi: 10.1111/cobi.13006
Pruetz, J. D., and Bertolani, P. (2009). Chimpanzee (Pan troglodytes verus) behavioral responses to stresses associated with living in a savanna-mosaic environment: Implications for Hominin adaptations to open habitats. PaleoAnthropology 33, 252–262. doi: 10.4207/PA.2009.ART33
R Core Team (2015). R: A Language and Environment for Statistical Computing, R Foundation for Statistical Computing. Vienna. Available online at: https://www.R-project.org/
Raleigh, C., Linke, A., Hegre, H., and Karlsen, J. (2010). Introducing ACLED: an armed conflict location and event dataset special data feature. J. Peace Res. 47, 651–660. doi: 10.1177/0022343310378914
Ryan, S. J., and Walsh, P. D. (2011). Consequences of non-intervention for infectious disease in African great apes. PLoS ONE 6:e29030. doi: 10.1371/journal.pone.0029030
Schielzeth, H. (2010). Simple means to improve the interpretability of regression coefficients. Methods Ecol. Evol. 1, 103–113. doi: 10.1111/j.2041-210X.2010.00012.x
Schielzeth, H., and Forstmeier, W. (2009). Conclusions beyond support: overconfident estimates in mixed models. Behav. Ecol. 20, 416–420. doi: 10.1093/beheco/arn145
Schultz, P. W. (2011). Conservation means behavior. Conserv. Biol. 25, 1080–1083. doi: 10.1111/j.1523-1739.2011.01766.x
Schultz, P. W. (2014). Strategies for promoting proenvironmental behavior - lots of tools but few instructions. Eur. Psychol. 19, 107–117. doi: 10.1027/1016-9040/a000163
Sesink Clee, P. R., Abwe, E. E., Ambahe, R. D., Anthony, N. M., Fotso, R., Locatelli, S., et al. (2015). Chimpanzee population structure in Cameroon and Nigeria is associated with habitat variation that may be lost under climate change. BMC Evol. Biol. 15:2. doi: 10.1186/s12862-014-0275-z
Silva, W. G., Metzger, J. P., Simões, S., and Simonetti, C. (2007). Relief influence on the spatial distribution of the Atlantic Forest cover on the Ibiúna Plateau, SP. Braz. J. Biol. 67, 403–411. doi: 10.1590/S1519-69842007000300004
Spehar, S. N., Sheil, D., Harrison, T., Louys, J., Ancrenaz, M., Marshall, A. J., et al. (2018). Orangutans venture out of the rainforest and into the Anthropocene. Sci. Adv. 4:e1701422. doi: 10.1126/sciadv.1701422
Strindberg, S., Maisels, F., Williamson, E. A., Blake, S., Stokes, E. J., Aba'a, R., et al. (2018). Guns, germs, and trees determine density and distribution of gorillas and chimpanzees in Western Equatorial Africa. Sci. Adv. 4:eaar2964. doi: 10.1126/sciadv.aar2964
Tagg, N., Willie, J., Duarte, J., Petre, C.-A., and Fa, J. E. (2015). Conservation research presence protects: a case study of great ape abundance in the Dja region, Cameroon. Anim. Conserv. 18, 489–498. doi: 10.1111/acv.12212
Thomas, L., Buckland, S. T., Rexstad, E. A., Laake, J. L., Strindberg, S., Hedley, S. L., et al. (2010). Distance software: design and analysis of distance sampling surveys for estimating population size. J. Appl. Ecol. 47, 5–14. doi: 10.1111/j.1365-2664.2009.01737.x
Tranquilli, S., Abedi-Lartey, M., Amsini, F., Arranz, L., Asamoah, A., Babafemi, O., et al. (2012). Lack of conservation effort rapidly increases African great ape extinction risk. Conserv. Lett. 5, 48–55. doi: 10.1111/j.1755-263X.2011.00211.x
TRMM GES DISC (2011). Tropical Rainfall Measuring Mission (TRMM) 3B43, Version 7, Goddard Space Flight Center Distributed Active Archive Center, Greenbelt, MD. Goddard Earth Sci. Data Inf. Serv. Cent. Available online at: http://mirador.gsfc.nasa.gov/collections/TRMM_3B43__007.shtml (Accessed January 6, 2016).
Voigt, M., Wich, S. A., Ancrenaz, M., Meijaard, E., Abram, N., Banes, G. L., et al. (2018). Global demand for natural resources eliminated more than 100,000 Bornean orangutans. Curr. Biol. 28, 761–769.e5. doi: 10.1016/j.cub.2018.01.053
Walsh, P. D., and White, L. J. T. (2005). Evaluating the steady state assumption: Simulations of gorilla nest decay. Ecol. Appl. 15, 1342–1350. doi: 10.1890/03-5283
Wan, Z., and Hulley, G. C. (2015). MOD11B3 MODIS/Terra Land Surface Temperature/Emissivity Monthly L3 Global 6km SIN Grid V006, NASA EOSDIS Land Processes DAAC. doi: 10.5067/MODIS/MOD11B3.006 (Accessed April 4, 2016).
Weiss, D. J., Nelson, A., Gibson, H. S., Temperley, W., Peedell, S., Lieber, A., et al. (2018). A global map of travel time to cities to assess inequalities in accessibility in 2015. Nature 553, 333–336. doi: 10.1038/nature25181
Wessling, E. G., Deschner, T., Mundry, R., Pruetz, J. D., Wittig, R. M., and Kühl, H. S. (2018a). Seasonal variation in physiology challenges the notion of chimpanzees (Pan troglodytes verus) as a forest-adapted species. Front. Ecol. Evol. 6:60. doi: 10.3389/fevo.2018.00060
Wessling, E. G., Kühl, H. S., Mundry, R., Deschner, T., and Pruetz, J. D. (2018b). The costs of living at the edge: Seasonal stress in wild savanna-dwelling chimpanzees. J. Hum. Evol. 121, 1–11. doi: 10.1016/j.jhevol.2018.03.001
Whitcraft, S., Hofford, A., Hilton, P., O'Malley, M., Jaiteh, V., and Knights, P. (2014). Evidence of Declines in Shark Fin Demand China. San Francisco, CA: WildAid.
Wich, S. A., Singleton, I., Nowak, M. G., Atmoko, S. S. U., Nisam, G., Arif, S. M., et al. (2016). Land-cover changes predict steep declines for the Sumatran orangutan (Pongo abelii). Sci. Adv. 2:e1500789. doi: 10.1126/sciadv.1500789
Woods Hole Research Center (2007). Vegetation Height Estimates. Available online at: http://whrc.org/publications-data/datasets/detailed-vegetation-height-estimates-across-the-tropics/ (Accessed January 7, 2016).
World Bank (2015). Worldwide Governance Indicators. Available online at: http://data.worldbank.org/data-catalog/worldwide-governance-indicators (Accessed August 14, 2015).
Keywords: behavioral change, conservation planning, hunting, mimicking, positive deviance, West Africa, Pan troglodytes verus
Citation: Heinicke S, Mundry R, Boesch C, Amarasekaran B, Barrie A, Brncic T, Brugière D, Campbell G, Carvalho J, Danquah E, Dowd D, Eshuis H, Fleury-Brugière M-C, Gamys J, Ganas J, Gatti S, Ginn L, Goedmakers A, Granier N, Herbinger I, Hillers A, Jones S, Junker J, Kouakou CY, Lapeyre V, Leinert V, Marrocoli S, Molokwu-Odozi M, N’Goran PK, Normand E, Pacheco L, Regnaut S, Sop T, Ton E, van Schijndel J, Vendras E, Vergnes V, Welsh A, Wessling EG and Kühl HS (2019) Characteristics of Positive Deviants in Western Chimpanzee Populations. Front. Ecol. Evol. 7:16. doi: 10.3389/fevo.2019.00016
Received: 03 October 2018; Accepted: 18 January 2019;
Published: 19 February 2019.
Edited by:
Serge Wich, Liverpool John Moores University, United KingdomReviewed by:
Marc Ancrenaz, HUTAN-Kinabatangan Orangutan Conservation Project, MalaysiaAlex Kenneth Piel, Liverpool John Moores University, United Kingdom
Copyright © 2019 Heinicke, Mundry, Boesch, Amarasekaran, Barrie, Brncic, Brugière, Campbell, Carvalho, Danquah, Dowd, Eshuis, Fleury-Brugière, Gamys, Ganas, Gatti, Ginn, Goedmakers, Granier, Herbinger, Hillers, Jones, Junker, Kouakou, Lapeyre, Leinert, Marrocoli, Molokwu-Odozi, N'Goran, Normand, Pacheco, Regnaut, Sop, Ton, van Schijndel, Vendras, Vergnes, Welsh, Wessling and Kühl. This is an open-access article distributed under the terms of the Creative Commons Attribution License (CC BY). The use, distribution or reproduction in other forums is permitted, provided the original author(s) and the copyright owner(s) are credited and that the original publication in this journal is cited, in accordance with accepted academic practice. No use, distribution or reproduction is permitted which does not comply with these terms.
*Correspondence: Stefanie Heinicke, c3RlZmFuaWVfaGVpbmlja2VAZXZhLm1wZy5kZQ==