- 1Conservation Ecology Group, Groningen Institute for Evolutionary Life Sciences, University of Groningen, Groningen, Netherlands
- 2Department of Biological Sciences, University of South Carolina, Columbia, SC, United States
- 3NIOZ Royal Netherlands Institute for Sea Research, Department of Coastal Systems and Utrecht University, Texel, Netherlands
Variation in migratory behavior is the result of different individual strategies and fluctuations in individual performances. A first step toward understanding these differences in migratory behavior among individuals is, therefore, to assess the relative contributions of inter- and intra-individual differences to this variation. We did this using light-level geolocators deployed on the breeding grounds to follow continental black-tailed godwits (Limosa limosa limosa) throughout their south- and northward migrations over multiple years. Based on repeated tracks from 36 individuals, we found two general patterns in godwit migratory behavior: First, migratory timing in black-tailed godwits varies mostly because individual godwits migrate at different times of the year. Second, individuals also exhibit considerable variation in timing within their respective migratory windows. Although the absolute amount of inter-individual variation in timing decreased over the course of northward migration, individual godwits still arrived at their breeding grounds across a span of more than 5 weeks. These differences in migratory timing among individuals are larger than those currently observed in other migratory bird species and suggest that the selective forces that limit the variation in migratory timing in other species are relaxed or absent in godwits. Furthermore, we could not attribute these individual differences to the sex or wintering location of an individual. We suggest that different developmental trajectories enabled by developmental plasticity likely result in these generally consistent, life-long annual routines. To investigate this possibility and to gain an understanding of the different selection pressures that could be acting during migration and throughout a godwit's life, future studies should track juvenile godwits and other migratory birds from birth to adulthood while also manipulating their spatiotemporal environment during development.
Introduction
Long-term mark-recapture studies and the rapid development of tracking technologies have revealed the migratory patterns of many avian migrants (Berthold, 2001; Newton, 2008; Bridge et al., 2011). These migratory patterns are always characterized by some degree of variation, such as individuals migrating at different times and toward different destinations (Berthold, 2001; Newton, 2008). Such population-level variation in migratory patterns is the result of both inter- and intra-individual differences (Vardanis et al., 2011; Conklin et al., 2013). The amount of consistent variation among individuals (i.e., inter-individual variation) is subject to selection: only those strategies that ensure survival will remain in the population and over the long-term those strategies that maximize fitness will be selected (Alerstam et al., 2003).
For instance, the timing of arrival on the breeding grounds, in particular, is thought to be under strong selection in migratory birds in order for individuals to procure high-quality breeding territories and breed in synchrony with consistently timed local resource peaks (Alerstam et al., 2003). Inter-individual variation in this component of migration is therefore usually expected to be small (Kokko, 1999; Bety et al., 2004; Both et al., 2006). However, selection can also favor multiple canalized strategies and thus lead to large inter-individual variation within a population. This can happen as a result of fluctuating environmental conditions (e.g., serial residency; Cresswell, 2014) or frequency-dependent processes (e.g., partial and differential migration; Lundberg, 1988; Chapman et al., 2011).
Most environments, however, are neither entirely consistent nor entirely predictable, which can affect the consistency with which individuals are able to perform their migrations (e.g., Studds and Marra, 2011). In addition, an individual can exhibit different migration strategies with increasing experience (e.g., individual improvement; Sergio et al., 2014), because the environment requires flexibility (e.g., nomadism; Pedler et al., 2018), or because the environment allows flexibility (e.g., the absence of carry-over effects; Senner et al., 2014). Differences in an individual's migratory behavior across years (i.e., intra-individual variation) therefore also contribute to migration variation at the population level (sensu Conklin et al., 2013). Thus, the amount of observed variation in migratory behavior within a population can result from (1) differences among individuals, which are consistent, and (2) differences within individuals, which are expected to vary according to the predictability and consistency of the environment and the individual's ability to respond to environmental changes.
A first step toward understanding why migratory patterns vary within populations is to consider the relative contributions of both inter- and intra-individual variation to the amount of variation at the population level (Senner et al., 2015b). To do this, the performances of multiple individuals must be measured across multiple years. These repeated measures allow for the calculation of repeatability (r), which reflects the proportion of population-level variation that can be attributed to inter-individual differences (Nakagawa and Schielzeth, 2010). The non-repeatable fraction (1-r) therefore reflects the contribution of intra-individual variation. However, a high r value—where inter-individual variation is proportionally larger than intra-individual variation—can result from either large variation among individuals, high consistency within individuals, or both (Conklin et al., 2013).
Black-tailed Godwits (Limosa limosa limosa; hereafter “godwits”) are long-distance migratory birds that breed in Europe and have a large non-breeding range—a quarter of the population winters north of the Sahara on the Iberian Peninsula (Márquez-Ferrando et al., 2014), while the majority winters south of the Sahara in the Sahel zone of West Africa (Hooijmeijer et al., 2013; Kentie et al., 2017). There is also large variation in the migratory timing of godwits (Lourenço et al., 2011; Senner et al., under review). This is especially true during northward migration: at the population level, variation in departure dates from the African wintering grounds and Iberian stopover sites span more than 10 weeks, and even arrival at the breeding grounds can vary by up to 5 weeks (Lourenço et al., 2011; Senner et al., under review). Inter-individual differences play an unexpectedly important role in this considerable variation—accounting for the majority of observed variation in departure to the north (r = 0.76) and nearly half the observed variation in arrival on the breeding grounds (r = 0.49; (Senner et al., under review).
Because selection determines the amount of variation in migratory timing among individuals, this raises two major questions about godwit migration: (1) Why isn't the role of inter-individual variation small, as it is in most other long-distance migrants (Newton, 2008; Stanley et al., 2012; Conklin et al., 2013)? and (2) What is the source of this surprisingly large amount of inter-individual variation? (Senner et al., under review) address the first question, suggesting that the large variation among individuals in godwits exists because of relaxed selection on migratory timing. This study addresses the second question, investigating the source of inter-individual variation in migratory timing in godwits. We describe the timing of migration and the wintering location of 70 individuals, of which 36 individuals were followed for multiple years. We calculate the repeatability of migratory timing and wintering location to assess whether individuals consistently winter either north or south of the Sahara and to identify the relative contributions of inter- and intra-individual variation to total population-level variation. Then, we use sex and wintering location to explain some, but not all, of the large amount of inter-individual variation. Ultimately, we are unable to account for the remaining variation that occurs between individuals and so we discuss in detail other sources—such as differences in developmental trajectories—that may be contributing to this phenomenon.
Materials and Methods
Fieldwork
Fieldwork occurred from March through July 2012–2017, in our long-term study area in southwest Fryslân, The Netherlands (Senner et al., 2015a). This area, which encompasses 12,000 ha, stretches from 53.0672°N, 5.4021°E in the north to 52.8527°N, 5.4127°E in the south, and from 52.9715°N, 5.6053°E in the east to 52.8829°N, 5.3607°E in the west. In this area we located godwit nests and used the flotation method (Liebezeit et al., 2007) to determine lay dates. To reduce the chance of nest abandonment and increase the chance of capturing an adult, we caught breeding adults toward the end of their incubation period (24 ± 3.84 days after laying). In each of the six field seasons, we outfitted 42–69 individuals with geolocators; this corresponded to 26–61% of all captured adults each year. We used geolocators from Migrate Technology, Ltd: the 0.65 g Intigeo W65A9 model in 2012–2013, and the 1g Intigeo C65 model thereafter.
These geolocators were attached to colored flags and placed on the tibia. The total weight of this attachment was ~3.3 g in 2012–2013 and ~3.7 g in 2014–2017, resulting in a loading factor of 1–1.5% of an individual's body mass at capture. For molecular sexing, we took ~30 μl of blood from the brachial vein of each individual. We were able to use these blood samples to sex 67 of the 70 individuals; we sexed the remaining 3 individuals based on morphological measurements as in Schroeder et al. (2008). In the years following geolocator deployment, we put considerable effort into recapturing godwits carrying geolocators. Recapture probability was nonetheless low; over the course of six field seasons, we managed to recapture 92 out of 305 deployed geolocators. Some geolocators did not record full annual cycles. For this reason, our data contains more tracks of southward migration (n = 117) than northward migration (n = 79; see Table 1 for more details).
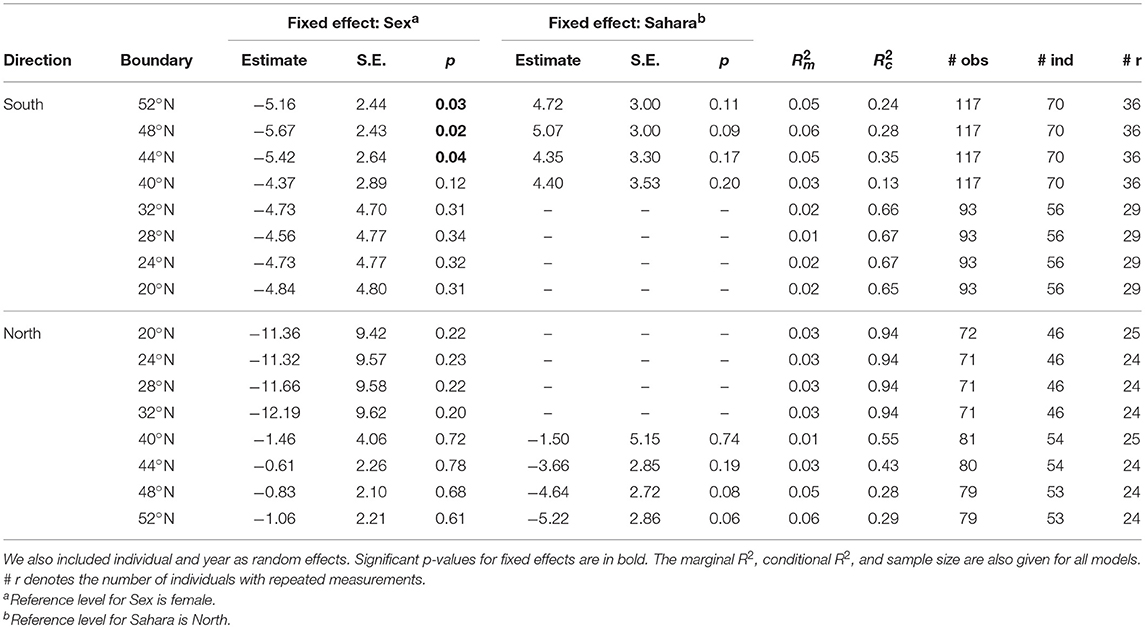
Table 1. Summary of the linear mixed effect models evaluating whether the timing of each crossing on both south- and northward migrations was a result of the sex (male/female) or wintering location (north/south of Sahara) of an individual.
Analyzing Geolocator Data
Using package “BAStag” (Wotherspoon et al., 2016) in Program R (R Core Team, 2017), we started with the function “preprocesslight,” which automatically detects sunrises and sunsets. We set the threshold light value to 2. Next, we visually inspected the slope of each sunrise and sunset and excluded those slopes that were strongly biased over time, i.e., showed abrupt changes in light level (Rakhimberdiev et al., 2016). We then used package “FLightR” (Rakhimberdiev et al., 2017) to reconstruct the annual schedules of godwits from this light-level data. Detailed examples of this analytical routine using our own godwit data can be found in Rakhimberdiev et al. (2016, 2017). These examples use data from a godwit that wintered north of the Sahara (≥28°N). Our sample also includes birds that wintered south of the Sahara (<28°N), with the only difference between the published examples and our own analyses being that we constrained the spatial extent of the particle filter to 18°W−13°E and 11–57°N instead of the 14°W−13°E and 30–57°N boundaries used by Rakhimberdiev et al. (2016, 2017).
Next, using the FLightR function “find.times.distribution” we estimated when individual godwits crossed certain arbitrary spatial boundaries. For this, we designated eight spatial boundaries which were spaced 4° of latitude apart across the entire godwit migration corridor, from 52 (the breeding grounds) to 20°N (just north of the southernmost African wintering grounds; Figure 1). We used the same eight spatial boundaries for both south- and northward migrations. In our analyses, we excluded the crossing of the spatial boundary at 36°N (the Strait of Gibraltar) because we could not distinguish between birds stopping in northern Morocco and birds stopping in southern Spain. In 26 out of 79 cases, we were also unable to estimate arrival at the breeding grounds (≥52°N) using this method. In these cases, migration and arrival on the breeding grounds coincided with the spring equinox, a period during which it is difficult to reliably estimate latitude from light-level data (Fudickar et al., 2012; Rakhimberdiev et al., 2015). Longitude, however, is much less affected (Rakhimberdiev et al., 2015, 2016), and godwits fortunately migrate from west to east as well as from south to north during their northward migration. For these 26 cases we could therefore use a spatial boundary of 5°E to estimate arrival on the breeding grounds (sensu Rakhimberdiev et al., 2015, 2016). Similarly, in 16 of 79 cases, we were unable to estimate the crossing of 48°N due to the spring equinox, and used a boundary of 0.75°E instead.
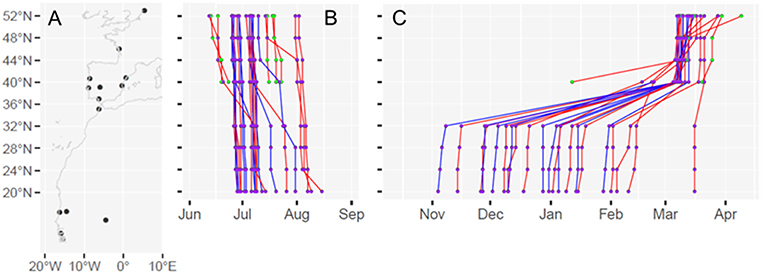
Figure 1. Presentation of (A) the entire godwit migration corridor with the breeding location and wintering and stopover locations as identified by satellite transmitters (MAV, AHJL, and TP unpubl. data) and the nine arbitrary latitudinal boundaries used to quantify the variation in migratory timing; (B) the variation among individuals in the timing of southward migration in 2013*, and (C) the variation among individuals in the timing of northward migration in 2014*. Red lines represent females and blue lines represent males. Individuals that crossed the Sahara are shown with purple dots, while individuals that did not cross the Sahara are shown with green dots. Note that the timing of crossing the spatial boundary at 36°N is excluded from the analyses and figures. *Similar graphs for all years, 2012–2017, can be found in Figure S2.
Lastly, we used the FLightR-function “stationary.migration.summary” to provide an overview of the stationary periods occurring throughout an individual's annual schedule. This allowed us to infer whether an individual wintered north (≥28°N) or south of the Sahara (<28°N).
Analyzing Annual Schedules
We first grouped individual godwits according to where they spent the non-breeding period. We considered individuals that crossed the Sahara (<28°N) during migration to have wintered “South” of the Sahara, and individuals that never crossed the Sahara (≥28°N) to have wintered “North” of the Sahara. To determine whether individuals were flexible in their over-wintering behavior, we looked at whether they consistently wintered on the same side of the Sahara from year to year. We also used a binomial Generalized Linear Model (GLM) with wintering area as the dependent variable and sex as the independent variable to test whether the proportion of males and females that crossed the Sahara differed. Because some individuals winter north and others winter south of the Sahara, our sample sizes differed among spatial boundaries (Table 1).
For both south- and northward migrations, we calculated population-level variation in the timing of each crossing of our arbitrary spatial boundaries. We did this by calculating the difference between the earliest crossing and all subsequent crossings, and then calculating the 5 year mean and standard deviation of this difference (Figures 2A,B). We then used a GLM to test whether the amount of variation differed between the spatial boundaries. Our data includes repeated measures of 26 individuals followed for 2 years, nine individuals followed for 3 years, and one individual followed for 4 years. We calculated individual variation in the timing of crossings by identifying the largest difference between the crossings of each individual over the course of the time that they were tracked. Next, we calculated the mean and standard deviation across all individuals (Figures 2C,D) and used a GLM to test for differences in the amount of intra-individual variation among spatial boundaries. When differences among spatial boundaries were found, we used a Tukey post-hoc test with a 95% confidence level to establish how the timing differed between pairs of boundaries. Additionally, we calculated the repeatability of each barrier crossing during south- and northward migration (Figures 2E,F). We did this by including individual as a random effect in the linear mixed model method of the function “rpt,” which is part of the R package “rptR” (Stoffel et al., 2017). To evaluate whether individuals consistently shifted their timing earlier or later over the course of our study, we plotted for every spatial boundary the first observed timing of crossing vs. the last observed timing of crossing for each individual godwit (Figure S1).
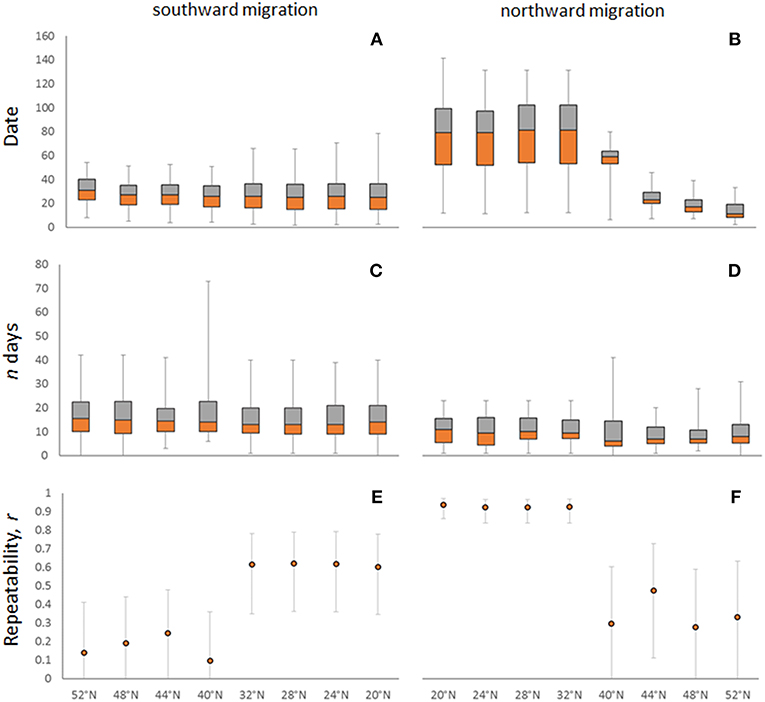
Figure 2. For both south and northward migrations the spatial boundaries (x-axis) are in chronological order from left to right. (A) Observed population variation during southward migration 2012–2016 and (B) during northward migration 2012–2017. Boxplots show 25, 50, and 75th percentiles; whiskers indicate 5 and 95th percentiles (day 1 = earliest observation for each crossing). (C) Intra-individual variation in the timing of southward migration and (D) of northward migration. Boxplots show 25, 50, and 75th percentiles; whiskers indicate the entire range of values. (E) Individual repeatability of timing on southward migration and (F) on northward migration. Plots show the repeatability estimate and the 95% confidence interval. The different colors are used for visual purposes only.
Finally, using the R package “lme4” (Bates et al., 2015), we fitted linear mixed effect models for each crossing during both south- and northward migrations. In these models we used the timing of crossing of a spatial boundary as the response variable, and the wintering location (north/south of Sahara) and sex of an individual (male/female) as fixed effects. We also included individual and year as random effects. We assessed whether the fixed effects improved the model significantly by means of a likelihood ratio test. We also calculated the marginal R2 to describe the amount of variance that is explained by the fixed effects using package MuMIn (Barton, 2016), following the method established by Nakagawa and Schielzeth (2013).
Results
Among all individuals (n = 70), 30 females and 26 males crossed the Sahara (80%), whereas 9 females and 5 males did not (20%). The proportion of males and females that crossed the Sahara did not differ (χ2 = 0.53, df = 1, p = 0.47). Of the 36 individuals for which we obtained repeated measures—23 females and 13 males—all 36 were consistent in wintering either north (n = 7) or south (n = 29) of the Sahara over the course of the time they were tracked.
The smallest difference among individuals between the earliest and latest crossings during southward migration was 62 days for the barriers at both 48 and 44°N, whereas the largest difference was 106 days for crossing 20°N (Figures 1B, 2A; Figure S2). During northward migration, the smallest difference was 38 days for crossing 52°N, and the largest difference was 153 days for crossing 28°N (Figures 1C, 2B; Figure S2). The average amount of variation among individuals did not vary among spatial boundaries during southward migration [F(7,832) = 0.96; p = 0.46; Figures 1B, 2A; Figure S2], but did vary during northward migration [F(7,596) = 108.4; p < 0.001; Figures 1C, 2B; Figure S2]. A Tukey post-hoc test with a 95% confidence level found that population-level variation was greatest for crossing 20–32°N (the Sahara), decreased for crossing 40°N (departing the Iberian Peninsula), and was smallest for crossing 44–52°N (France, Belgium, and The Netherlands; Figures 1C, 2B; Figure S2).
Intra-individual differences between years for timing at the same latitude varied from 0–73 days during southward migration (Figure 2C). The biggest differences, 62 and 73 days, occurred when crossing 40°N (Figure 2C). This was due to two individuals stopping over north of this boundary 1 year and south of it the other. The intra-individual differences in timing between those years includes the durations of these stopovers and is deceptively large as a result. During northward migration, intra-individual differences varied from 0 to 42 days (Figure 2D). The biggest difference, 42 days when crossing 40°N, was again the result of an individual stopping over on opposite sides of the boundary in different years (Figure 2D). Thus, the average amount of intra-individual differences did not vary between spatial boundaries during either southward [F(7,252) = 0.70; p = 0.68; Figure 2C] or northward migration [F(7,186) = 0.57; p = 0.78; Figure 2D]. Furthermore, individuals did not consistently shift their timing earlier or later over the course of our study during either southward or northward migration (Figure S1).
Individual repeatability during southward migration varied from 0.1–0.6 and was highest when crossing the Sahara (20–32°N; Figure 2E). During northward migration, repeatability varied between 0.3–0.9 and was again highest when crossing the Sahara (Figure 2F). Repeatability therefore increased over the course of southward migration and decreased over the course of northward migration (Figures 2E,F). This could be the result of individuals wintering south of the Sahara being more consistent in their timing than individuals wintering north of the Sahara. However, the amount of intra-individual variation is non-significantly larger during Sahara crossings (Figures 2C,D); this indicates that the repeatability is higher because inter-individual differences are larger for Sahara crossings, not because these individuals are more consistent.
During southward migration, males departed the Netherlands (52°N) on average 5 d earlier than females (χ2 = 4.47, df = 1, p = 0.03, n = 117; Table 1). This difference held true for the crossing of 48 and 44°N, but not for more southerly boundaries (40–20°N; p > 0.1; Table 1). Whether or not an individual crossed the Sahara did not explain a significant amount of the variation in the timing of southward migration (Table 1). During northward migration, neither the sex of the individual (p > 0.1 for all spatial boundaries; Table 1) nor whether it crossed the Sahara (p > 0.05; Table 1) explained a significant amount of the variation in their timing. Thus, the amount of variance explained by our fixed effects—as indicated by the marginal R2—was never higher than 0.06 (Table 1). The marginal R2 was highest when crossing 44–52°N during both southward and northward migration (Table 1). Not surprisingly, these were the southward migration boundary crossings for which a significant amount of the variation was explained by sex, and the northward migration crossings for which at least some of the variation (p = 0.06; Table 1) was explained by whether an individual crossed the Sahara or not.
Discussion
We found that the large amount of population-level variation in the migratory timing of continental black-tailed godwits is mostly the result of individual godwits exhibiting consistent differences from one another in the timing of their movements during both north- and southward migration. In addition, we found that a given individual can exhibit considerable flexibility while still adhering to its own particular schedule. These inter-individual and intra-individual differences in timing are large compared to other species of migratory birds (e.g., Alerstam et al., 2006; Vardanis et al., 2011; Stanley et al., 2012; Conklin et al., 2013). This suggests that the selective forces that limit the variation in migratory timing in other species are likely relaxed or absent in godwits (see also Senner et al., under review) and that the unexplained but consistent differences among godwits may be the result of different developmental trajectories.
Population Variation
We found that approximately 80% of black-tailed godwits breeding in Fryslân cross the entirety of the Sahara Desert during migration, whereas 20% do not cross any portion of it, and that this was a consistent behavior across years. Furthermore, although the repeatability in the timing of flights across the Sahara was higher than that of other migratory flights, this was driven by the relative influence of inter-individual variation, which was also highest at this point in the migration. In other words, individual godwits consistently time their Sahara crossings differently from one another. This suggests both that this major ecological barrier is traversable for a long period of time and that other temporal constraints—for example, the availability of resources at sites to the north of the Sahara—do not influence the time at which individuals make this flight (Moore and Yong, 1991; Baker et al., 2004). This is surprising, as the crossing of the Sahara during both south- and northward migrations in most other migratory bird species takes place over a shorter period of time (e.g., Vardanis et al., 2011, max = 64 days; Lindström et al., 2015, max = 33 days; Briedis et al., 2016, max = 25 days; Jacobsen et al., 2017, max = 35 days; Ouwehand and Both, 2017, max = 37 days), although Sergio et al. (2014) found that black kites (Milvus migrans) also cross the Sahara over a 5 month period as a result of the sequential departure from the wintering grounds by different age classes. It is not clear why the Sahara crossing of the other migratory birds appears to be under generally stronger temporal selection, but these species must either face stronger temporal constraints in relation to the crossing itself or during subsequent events in their annual cycle.
Once past the Sahara Desert during northward migration, inter-individual variation in timing decreased toward the breeding grounds and was smallest when crossing the region between 44–52°N (France, Belgium and The Netherlands). Levels of intra-individual variation did not decrease simultaneously, but were smallest when crossing 44°N. As a result, the repeatability of timing for these stages (40–52°N) differed from zero only when crossing 44°N. Individuals are thus relatively consistent in their timing of departure from the Iberian Peninsula (44°N), but not their timing of arrival at the breeding grounds. Given that the intra-individual variation increased for the two most northerly crossings, 48 and 52°N, this is probably due to the flexible adjustment of their migratory schedule in response to environmental conditions encountered en route. For instance, in 2013, a rare spring snowstorm delayed the arrival of godwits to the breeding grounds by an average of 19 days (Senner et al., 2015a).
Both the tightening of migratory schedules toward the breeding grounds (e.g., Hasselquist et al., 2017; Wellbrock et al., 2017) and the flexible adjustment of migratory schedules (e.g., Nuijten et al., 2014; Briedis et al., 2017) have been shown in other migratory bird species. Nonetheless, the arrival of godwits at the breeding grounds spans more than 5 weeks—which is a larger range than that currently observed in other migratory bird species (e.g., Senner et al., 2014; Lindström et al., 2015; Briedis et al., 2016; Jacobsen et al., 2017; Ouwehand and Both, 2017). Potentially, the absence of a strong temporal constraint on arrival at the breeding grounds is what allows godwits to cross the Sahara over such a long period of time. If this is true, other species making similar flights, but over a shorter period of time, may not face stronger temporal constraints for the crossing of the Sahara itself, but rather for their arrival at the breeding grounds. Accordingly, in other species, individuals from different breeding populations wintering in the same region of sub-Saharan Africa depart their wintering areas at different times, and these departure windows are correlated with their breeding-site specific reproductive timing (Briedis et al., 2016; Ouwehand et al., 2016).
The Relative Importance of Intra-Individual Variation
Although each godwit appeared to migrate within its own migratory window, individual godwits also displayed considerable flexibility in their timing of migration within their own specific windows. This degree of flexibility was not the result of directional changes in migratory timing, and is greater than the amount of intra-individual variation reported in other studies (e.g., Conklin et al., 2013, <5 days; Senner et al., 2014, <5 days; Hasselquist et al., 2017, <20 days; Wellbrock et al., 2017, <15 days). The relatively large intra-individual variation during migration can therefore be interpreted as an individual decision that balances migrating at a specific time and leaving when endogenous and exogenous conditions are best (e.g., Senner et al., 2015a). For example, crossing the Sahara is possible over a long period of time, but the right conditions might not present themselves consistently each year at the same time; waiting for the right conditions could thus result in considerable intra-individual variation in the timing of the initial portion of northward migration. If godwits lack a strong temporal constraint during northward migration, this might enable them to exhibit such flexibility without fitness consequences (Senner et al., under review). In this scenario, it is important that godwits be able to reliably predict the conditions characterizing the flight ahead of them (Winkler et al., 2014). However, Senner et al. (under review) found that in three of the 5 years studied, the survival of godwits was reduced while crossing the Sahara during northward migration; this could indicate that godwits cannot always reliably predict the conditions for this crossing or that the Sahara crossing invariably has a survival cost (see also Klaassen et al., 2010).
The Control of Migratory Timing
How can individual godwits consistently depart West Africa at different times? Individual godwits could depart at different times as a result of variation in their speed of migratory preparation or as a result of variation in their condition when they begin preparing for migration. Both options are likely to occur in godwits, through consistent differences in individual and environmental quality (Studds and Marra, 2005; Paxton and Moore, 2015). However, it is highly unlikely that these options could result in a difference of up to 5 months in migratory timing among individuals. Alternatively, unpredictable cues or a less rigid endogenous programme could also lead to variation among individuals within a given year (Aloni et al., 2017). However, if the cue or programme were so variable as to lead to a difference of up to 5 months in a given year, it is improbable that differences in migratory timing among individuals would be consistent across years, as is observed in godwits. For these reasons, we believe that godwits must make use of a predictable cue or have a relatively rigid circannual programme, or that both factors apply (Gwinner, 1989, 1996).
If we assume that godwits, like other migratory birds, use photoperiod to reliably keep track of time, then individual godwits must be responding differently from each other to the same photoperiod cues in order to maintain their differences in migratory timing (Gwinner, 1996). For instance, some godwits begin their northward migrations while day length is still decreasing, whereas others migrate once day length has begun increasing again. Thus, the inter-individual variation in the migratory timing of godwits from the same wintering location must be the result of individually-specific reaction norms to the same environmental stimuli. What might be the source of these large inter-individual differences in reaction norms? They are unlikely to be the result of inheritance or adaptation, as they appear to have no fitness consequences (Kentie et al., 2017; Senner et al., under review). They are also not likely to be the result of inter-individual differences in experience, since godwits did not shift their migration earlier or later over the course of our study. Instead, different developmental trajectories are likely the source. For instance, godwits have shifted their spring staging site through developmental plasticity (Verhoeven et al., 2018), which makes it plausible that the observed individual differences in migration are also the result of different developmental trajectories (Senner et al., 2015b).
Future Directions
Future research should therefore investigate whether differences in developmental trajectories are the source of the large inter-individual differences observed, and whether the variation in migratory timing in other migratory bird species is limited by stronger temporal constraints. To accomplish this, researchers could track godwits and other migratory bird species from birth to adulthood while also performing translocation and delay experiments (Perdeck, 1958; Chernetsov et al., 2004; Thorup et al., 2007). Additionally, researchers could simultaneously perform a captive study during development in which selective disappearance is absent and photoperiod is manipulated (Helm and Gwinner, 2006; Maggini and Bairlein, 2012). All of these experiments should manipulate the spatiotemporal environment during development, thus enabling an evaluation of whether the environment does or does not affect the migratory behavior of juveniles. If it does not, this would be evidence for innate migratory behavior (Perdeck, 1958; Thorup et al., 2007). If it does, this would suggest that environmental variation brings about differences in migratory behavior (Chernetsov et al., 2004; Piersma, 2011; Meyburg et al., 2017). Tracking these individuals into adulthood would then show whether these environmentally-induced differences are plastic or flexible and whether there is selective disappearance as a result of temporal constraints. The combination of these results would allow researchers to discern whether the narrower window of migratory timing in other bird species is the result of stronger innate control, stronger temporal constraints, or both.
Data Availability
The raw data supporting the conclusions of this manuscript will be stored on the University of Groningen data repository and made available by the authors to any qualified researcher upon reasonable request.
Author Contributions
All authors designed and carried out the study. MAV, AHJL, and ADM performed the analyses. MAV, AHJL, NRS, and ADM wrote the paper with contributions from all authors.
Funding
Funding for geolocators and their analysis was provided by NWO-ALW TOP grant Shorebirds in space (854.11.004) and the Spinoza Premium 2014 of the Netherlands Organization for Scientific Research (NWO), both awarded to TP. The long-term godwit research project was funded by the Kenniskring weidevogels of the former Ministry of Agriculture, Nature Management and Food Safety (2012, 2016) and the Province of Fryslân (2013–2017). Additional financial support came from the Prins Bernhard Cultuurfonds (through It Fryske Gea), the Van der Hucht de Beukelaar Stichting, the Paul and Louise Cook Endowment Ltd., the University of Groningen, BirdLife-Netherlands, and WWF-Netherlands.
Conflict of Interest Statement
The authors declare that the research was conducted in the absence of any commercial or financial relationships that could be construed as a potential conflict of interest.
Acknowledgments
We thank the members of our field crews for recapturing godwits, Eldar Rakhimberdiev for teaching us how to use FLightR, Jos Hooijmeijer for curating our research database and Julie Thumloup, Marco van der Velde and Yvonne Verkuil for their help with the molecular sexing. We are grateful to many farmers, most of whom are organized in the Collectief Súdwestkust, and the conservation management organizations It Fryske Gea and Staatsbosbeheer for cooperation and granting us access to their properties. This work was done under license number 6350A following the Dutch Animal Welfare Act Articles 9 and 11.
Supplementary Material
The Supplementary Material for this article can be found online at: https://www.frontiersin.org/articles/10.3389/fevo.2019.00031/full#supplementary-material
References
Alerstam, T., Hake, M., and Kjellen, N. (2006). Temporal and spatial patterns of repeated migratory journeys by ospreys. Anim. Behav. 71, 555–566. doi: 10.1016/j.anbehav.2005.05.016
Alerstam, T., Hedenström, A., and Åkesson, S. (2003). Long-distance migration: evolution and determinants. Oikos 103, 247–260. doi: 10.1034/j.1600-0706.2003.12559.x
Aloni, I., Markman, S., and Ziv, Y. (2017). February precipitation in the wintering grounds of the lesser whitethroat, Sylvia curruca: is it a cue for migration onset? R. Soc. Open Sci. 4:160755. doi: 10.1098/rsos.160755
Baker, A. J., Gonzalez, P. M., Piersma, T., Niles, L. J., de Lima Serrano do Nascimento, I., Atkinson, P. W., et al. (2004). Rapid population decline in red knots: fitness consequences of decreased refuelling rates and late arrival in Delaware Bay. Proc R Soc B. 271, 875–882. doi: 10.1098/rspb.2003.2663
Barton, K. (2016). MuMIn: Multi-Model Inference. R Package Version 1.15.6. Available online at: https://CRAN.R-project.org/package=MuMIn
Bates, D., Maechler, M., Bolker, B., and Walker, S. (2015). Fitting linear mixed-effects models using lme4. J. Stat. Soft. 67, 1–48. doi: 10.18637/jss.v067.i01
Bety, J., Giroux, J. F., and Gauthier, G. (2004). Individual variation in timing of migration: causes and reproductive consequences in greater snow geese (Anser caerulescens atlanticus). Behav. Ecol. Sociobiol. 57, 1–8. doi: 10.1007/s00265-004-0840-3
Both, C., Bouwhuis, S., Lessells, C. M., and Visser, M. E. (2006). Climate change and population declines in a long-distance migratory bird. Nature 441, 81–83. doi: 10.1038/nature04539
Bridge, E. S., Thorup, K., Bowlin, M. S., Chilson, P. B., Diehl, R. H., Fléron, R. W., et al. (2011). Technology on the move: recent and forthcoming innovations for tracking migratory birds. BioScience 61, 689–698. doi: 10.1525/bio.2011.61.9.7
Briedis, M., Hahn, S., and Adamík, P. (2017). Cold spell en route delays spring arrival and decreases apparent survival in a long-distance migratory songbird. BMC Ecol. 17:11. doi: 10.1186/s12898-017-0121-4
Briedis, M., Hahn, S., Gustafsson, L., Henshaw, I., Träff, J., Král, M., et al. (2016). Breeding latitude leads to different temporal but not spatial organization of the annual cycle in a long-distance migrant. J. Avian Biol. 47, 743–748. doi: 10.1111/jav.01002
Chapman, B.B., Bronmark, C., Nilsson, J., and Hansson, L. (2011). The ecology and evolution of partial migration. Oikos 120, 1764–1775. doi: 10.1111/j.1600-0706.2011.20131.x
Chernetsov, N., Berthold, P., and Querner, U. (2004). Migratory orientation of first-year white storks (Ciconia ciconia): inherited information and social interactions. J. Exp. Biol. 207, 937–943. doi: 10.1242/jeb.00853
Conklin, J. R., Battley, P. F., and Potter, M. A. (2013). Absolute consistency: individual versus population variation in annual-cycle schedules of a long-distance migrant bird. PLoS ONE 8:e54535. doi: 10.1371/journal.pone.0054535
Cresswell, W. (2014). Migratory connectivity of Palaearctic-African migratory birds and their responses to environmental change: the serial residency hypothesis. Ibis 156, 493–510. doi: 10.1111/ibi.12168
Fudickar, A. M., Wikelski, M., and Partecke, J. (2012). Tracking migratory songbirds: accuracy of light-level loggers (geolocators) in forest habitats. Methods Ecol. Evol. 3, 47–52. doi: 10.1111/j.2041-210X.2011.00136.x
Gwinner, E. (1989). Einfluß der Photoperiode auf das circannuale System des Halsbandschnäppers (Ficedula albicollis) und des Trauerschnäppers (F. hypoleuca). J. Ornithol. 130, 1–13. doi: 10.1007/BF01647157
Gwinner, E. (1996). Circadian and circannual programmes in avian migration. J. Exp. Biol. 19, 39–48.
Hasselquist, D., Montras-Janer, T., Tarka, M., and Hansson, B. (2017). Individual consistency of long-distance migration in a songbird: significant repeatability of autumn route, stopovers and wintering sites but not in timing of migration. J. Avian Biol. 48, 91–102. doi: 10.1111/jav.01292
Helm, B., and Gwinner, E. (2006). Migratory restlessness in an equatorial nonmigratory bird. PLoS Biol. 4, 611–614 doi: 10.1371/journal.pbio.0040110
Hooijmeijer, J. C. E. W., Senner, N. R., Tibbitts, T. L., Gill, R. E. Jr., Douglas, D. C., Bruinzeel, L. W., et al. (2013). Post-breeding migration of Dutch-breeding black-tailed godwits: timing, routes, use of stopovers, and nonbreeding destinations. Ardea 101, 141–152. doi: 10.5253/078.101.0209
Jacobsen, L. B., Jensen, N. O., Willemoes, M., Hansen, L., Desholm, M., Fox, A. D., et al. (2017). Annual spatiotemporal migration schedules in three larger insectivorous birds: European nightjar, common swift and common cuckoo. Anim. Biotelem. 5:4. doi: 10.1186/s40317-017-0119-x
Kentie, R., Márquez-Ferrando, R., Figuerola, J., Gangoso, L., Hooijmeijer, J. C. E. W., Loonstra, A. H. J., et al. (2017). Does wintering north or south of the Sahara correlate with timing and breeding performance in black-tailed godwits? Ecol. Evol. 7, 2812–2820. doi: 10.1002/ece3.2879
Klaassen, R. H. G., Strandberg, R., Hake, M., Olofsson, P., Tøttrup, A. P., and Alerstam, T. (2010). Loop migration in adult marsh harriers Circus aeruginosus as revealed by satellite telemetry. J. Avian Biol. 41, 200–207. doi: 10.1111/j.1600-048X.2010.05058.x
Kokko, H. (1999). Competition for early arrival in migratory birds. J. Anim. Ecol. 68, 940–950. doi: 10.1046/j.1365-2656.1999.00343.x
Liebezeit, J. R., Smith, P. A., Lanctot, R. B., Schekkerman, H., Tulp, I., Kendall, S. J., et al. (2007). Assessing the development of shorebird eggs using the flotation method: species-specific and generalized regression models. Condor 109, 32–47. doi: 10.1650/0010-5422(2007)109[32:ATDOSE]2.0.CO;2
Lindström, A., Alerstam, T., Bahlenberg, P., Ekblom, R., Fox, J. W., Råghall, J., et al. (2015). The migration of the great snipe Gallinago media: intriguing variations on a grand theme. J. Avian Biol. 46, 1–14. doi: 10.1111/jav.00829
Lourenço, P. M., Kentie, R., Schroeder, J., Groen, N. M., Hooijmeijer, J. C. E. W., and Piersma, T. (2011). Repeatable timing of northward departure, arrival and breeding in black-tailed godwits Limosa l. limosa, but no domino effects. J. Ornithol. 152, 1023–1032. doi: 10.1007/s10336-011-0692-3
Lundberg, P. (1988). The evolution of partial migration in birds. Trends Ecol. Evol. 3, 172–175. doi: 10.1016/0169-5347(88)90035-3
Maggini, I., and Bairlein, F. (2012). Innate sex differences in the timing offspring migration in a songbird. PLoS ONE 7:e31271. doi: 10.1371/journal.pone.0031271
Márquez-Ferrando, R., Figuerola, J., Hooijmeijer, J. C. E. W., and Piersma, T. (2014). Recently created man-made habitats in Doñana provide alternative wintering space for the threatened Continental European black-tailed godwit population. Biol. Cons. 171, 127–135. doi: 10.1016/j.biocon.2014.01.022
Meyburg, B.-U., Bergmanis, U., Langgemach, T., Graszynski, K., Hinz, A., Börner, I., et al. (2017). Orientation of native versus translocated juvenile lesser spotted eagles (Clanga pomarina) on the first autumn migration. J. Exp. Biol. 220, 2765–2776. doi: 10.1242/jeb.148932
Moore, F. R., and Yong, W. (1991). Evidence of food-based competition among passerine migrants during stopover. Behav. Ecol. Sociobiol. 28, 85–90. doi: 10.1007/BF00180984
Nakagawa, S., and Schielzeth, H. (2010). Repeatability for Gaussian and non-Gaussian data: a practical guide for biologists. Biol. Rev. 85, 935–956. doi: 10.1111/j.1469-185X.2010.00141.x
Nakagawa, S., and Schielzeth, H. (2013). A general and simple method for obtaining R2 from generalized linear mixed-effects models. Methods Ecol. Evol. 4, 133–142. doi: 10.1111/j.2041-210x.2012.00261.x
Nuijten, R. J., Kölzsch, A., van Gils, J. A., Hoye, B. J., Oosterbeek, K., de Vries, P. P., et al. (2014). The exception to the rule: retreating ice front makes Bewick's Swans Cygnus columbianus bewickii migrate slower in spring than in autumn. J. Avian Biol. 45, 113–122. doi: 10.1111/j.1600-048X.2013.00287.x
Ouwehand, J., Ahola, M. P., Ausems, A. N. M. A., Bridge, E. S., Burgess, M., Hahn, S., et al. (2016). Light-level geolocators reveal migratory connectivity in European populations of pied flycatchers Ficedula hypoleuca. J. Avian Biol. 47, 69–83. doi: 10.1111/jav.00721
Ouwehand, J., and Both, C. (2017). African departure rather than migration speed determines variation in spring arrival in pied flycatchers. J. Anim. Ecol. 86, 88–97. doi: 10.1111/1365-2656.12599
Paxton, K. L., and Moore, F. R. (2015). Carry-over effects of winter habitat quality on en route timing and condition of a migratory passerine during spring migration. J. Av. Biol. 46, 495–506. doi: 10.1111/jav.00614
Pedler, R. D., Ribot, R. F. H., and Bennett, A. T. D. (2018). Long-distance flights and high-risk breeding by nomadic waterbirds on desert salt lakes. Cons. Biol. 32, 216–228. doi: 10.1111/cobi.13007
Perdeck, A. C. (1958). Two types of orientation in migrating starlings Sturnus vulgaris L. and Chaffinches Fringilla coelebs L., as revealed by displacement experiments. Ardea 46, 1–37.
Piersma, T. (2011). Flyway evolution is too fast to be explained by the modern synthesis: proposals for an ‘extended' evolutionary research agenda. J. Ornithol. 152(Suppl. 1), S.151–S.159. doi: 10.1007/s10336-011-0716-z
R Core Team (2017). R: A Language and Environment for Statistical Computing. Vienna: R Foundation for Statistical Computing. Available online at: http://www.R-project.org
Rakhimberdiev, E., Saveliev, A., Piersma, T., and Karagicheva, J. (2017). FLightR: an R package for reconstructing animal paths from solar geolocation loggers. Methods Ecol. Evol. 8, 1482–1487. doi: 10.1111/2041-210X.12765
Rakhimberdiev, E., Senner, N. R., Verhoeven, M. A., Winkler, D. W., Bouten, W., and Piersma, T. (2016). Comparing inferences of solar geolocation data against high-precision GPS data: annual movements of a double-tagged black-tailed godwit. J. Avian Biol. 47, 589–596. doi: 10.1111/jav.00891
Rakhimberdiev, E., Winkler, D. W., Bridge, E., Seavy, N. E., Sheldon, D., Piersma, T., et al. (2015). A hidden Markov model for reconstructing animal paths from solar geolocation loggers using templates for light intensity. Mov. Ecol. 3:25. doi: 10.1186/s40462-015-0062-5
Schroeder, J., Lourenço, P. M., van der Velde, M., Hooijmeijer, J. C. E. W., Both, C., and Piersma, T. (2008). Sexual dimorphism in plumage and size in black-tailed godwits Limosa limosa limosa. Ardea 96, 25–37. doi: 10.5253/078.096.0104
Senner, N. R., Conklin, J. R., and Piersma, T. (2015b). An ontogenetic perspective on individual differences. Proc. R. Soc. B 282:20151050. doi: 10.1098/rspb.2015.1050
Senner, N. R., Hochachka, W. M., Fox, J. W., and Afanasyev, V. (2014). An exception to the rule: carry-over effects do not accumulate in a long-distance migratory bird. PLoS ONE 9:e86588. doi: 10.1371/journal.pone.0086588
Senner, N. R., Verhoeven, M. A., Abad-Gómez, J. M., Gutiérrez, J. S., Hooijmeijer, J. C. E. W., Kentie, R., et al. (2015a). When Siberia came to The Netherlands: the response of black-tailed godwits to a rare spring weather event. J. Anim. Ecol. 84, 1164–1176. doi: 10.1111/1365-2656.12381
Sergio, F., Tanferna, A., De Stephanis, R., Jiménez, L. J., Blas, J., Tavecchia, G., et al. (2014). Individual improvements and selective mortality shape lifelong migratory performance. Nature 515, 410–413. doi: 10.1038/nature13696
Stanley, C. Q., MacPherson, M., Fraser, K. C., McKinnon, E. A., and Stutchbury, B. J. M. (2012). Repeat tracking of individual songbirds reveals consistent migration timing but flexibility in route. PLoS ONE 7:e40688. doi: 10.1371/journal.pone.0040688
Stoffel, M. A., Nakagawa, S., and Schielzeth, H. (2017). rptR: repeatability estimation and variance decomposition by generalized linear mixed-effects models. Methods Ecol. Evol. 8, 1639–1644. doi: 10.1111/2041-210X.12797
Studds, C. E., and Marra, P. P. (2005). Nonbreeding habitat occupancy and population processes: an upgrade experiment with a migratory bird. Ecology 86, 2380–2385. doi: 10.1890/04-1145
Studds, C. E., and Marra, P. P. (2011). Rainfall-induced changes in food availability modify the spring departure programme of a migratory bird. Proc. R. Soc. B. 278, 3437–3443. doi: 10.1098/rspb.2011.0332
Thorup, K., Bisson, I.-A., Bowlin, M. S., Holland, R. A., Wingfield, J. C., Ramenofsky, M., et al. (2007). Evidence for a navigational map stretching across the continental US in a migratory songbird. Proc. Natl. Acad. Sci. U.S.A. 104, 18115–18119. doi: 10.1073/pnas.0704734104
Vardanis, Y., Klaassen, R. H. G., Strandberg, R., and Alerstam, T. (2011). Individuality in bird migration: routes and timing. Biol. Lett. 7, 502–505. doi: 10.1098/rsbl.2010.1180
Verhoeven, M. A., Loonstra, A. H. J., Hooijmeijer, J. C. E. W., Masero, J. A., Piersma, T., and Senner, N. R. (2018). Generational shift in spring staging site use by a long-distance migratory bird. Biol. Lett. 14:20170663. doi: 10.1098/rsbl.2017.0663
Wellbrock, A. H. J., Bauch, C., Rozman, J., and Witte, K. (2017). ‘Same procedure as last year¿Repeatedly tracked swifts show individual consistency in migration pattern in successive years. J. Av. Biol. 48, 897–903. doi: 10.1111/jav.01251
Winkler, D. W., Jørgensen, C., Both, C., Houston, A. I., McNamara, J. M., Levey, D. J., et al. (2014). Cues, strategies, and outcomes: how migrating vertebrates track environmental change. Mov. Ecol. 2:10. doi: 10.1186/2051-3933-2-10
Wotherspoon, S., Sumner, M., and Lisovski, S. (2016). Basic Data Processing for Light Based Geolocation Archival Tags. Available online at: http://lme4.r-forge.r-project.org
Keywords: migratory behavior, repeatability, shorebird, developmental plasticity, light-level geolocators
Citation: Verhoeven MA, Loonstra AHJ, Senner NR, McBride AD, Both C and Piersma T (2019) Variation From an Unknown Source: Large Inter-individual Differences in Migrating Black-Tailed Godwits. Front. Ecol. Evol. 7:31. doi: 10.3389/fevo.2019.00031
Received: 16 October 2018; Accepted: 28 January 2019;
Published: 26 February 2019.
Edited by:
Carlos Alonso Alvarez, Spanish National Research Council (CSIC), SpainReviewed by:
James Dale, Massey University, New ZealandWendy Hood, Auburn University, United States
Copyright © 2019 Verhoeven, Loonstra, Senner, McBride, Both and Piersma. This is an open-access article distributed under the terms of the Creative Commons Attribution License (CC BY). The use, distribution or reproduction in other forums is permitted, provided the original author(s) and the copyright owner(s) are credited and that the original publication in this journal is cited, in accordance with accepted academic practice. No use, distribution or reproduction is permitted which does not comply with these terms.
*Correspondence: Mo A. Verhoeven, bS5hLnZlcmhvZXZlbkBydWcubmw= orcid.org/0000-0002-2541-9786
†A.H. Jelle Loonstra orcid.org/0000-0002-5694-7581
Nathan R. Senner orcid.org/0000-0003-2236-2697