- 1Sea Mammal Research Unit, School of Biology, Scottish Oceans Institute, University of St Andrews, St Andrews, United Kingdom
- 2Biodiversity, Marine Ecology and Conservation Group, Department of Animal Biology, University of La Laguna, La Laguna, Spain
- 3Monterey Bay Aquarium Research Institute, Moss Landing, CA, United States
- 4Institute of Marine Sciences, University of California, Santa Cruz, Santa Cruz, CA, United States
- 5Southall Environmental Associates, Aptos, CA, United States
- 6Environmental Research Division, NOAA Southwest Fisheries Science Center, Monterey, CA, United States
- 7Department of Biology, Hopkins Marine Station, Stanford University, Monterey, CA, United States
- 8Vertebrate Ecology Lab, Moss Landing Marine Laboratories, Moss Landing, CA, United States
- 9Department of Mathematics and Statistics, Calvin College, Grand Rapids, MI, United States
- 10Cascadia Research Collective, Olympia, WA, United States
- 11Fundación Oceanogràfic, Valencia, Spain
- 12Woods Hole Oceanographic Institution, Woods Hole, MA, United States
Air-breathing marine predators must balance the conflicting demands of oxygen conservation during breath-hold and the cost of diving and locomotion to capture prey. However, it remains poorly understood how predators modulate foraging performance when feeding at different depths and in response to changes in prey distribution and type. Here, we used high-resolution multi-sensor tags attached to Risso's dolphins (Grampus griseus) and concurrent prey surveys to quantify their foraging performance over a range of depths and prey types. Dolphins (N = 33) foraged in shallow and deep habitats [seabed depths less or more than 560 m, respectively] and within the deep habitat, in vertically stratified prey features occurring at several aggregation levels. Generalized linear mixed-effects models indicated that dive kinematics were driven by foraging depth rather than habitat. Bottom-phase duration and number of buzzes (attempts to capture prey) per dive increased with depth. In deep dives, dolphins were gliding for >50% of descent and adopted higher pitch angles both during descent and ascents, which was likely to reduce energetic cost of longer transits. This lower cost of transit was counteracted by the record of highest vertical swim speeds, rolling maneuvers and stroke rates at depth, together with a 4-fold increase in the inter-buzz interval (IBI), suggesting higher costs of pursuing, and handling prey compared to shallow-water feeding. In spite of the increased capture effort at depth, dolphins managed to keep their estimated overall metabolic rate comparable across dive types. This indicates that adjustments in swimming modes may enable energy balance in deeper dives. If we think of the surface as a central place where divers return to breathe, our data match predictions that central place foragers should increase the number and likely quality of prey items at greater distances. These dolphins forage efficiently from near-shore benthic communities to depth-stratified scattering layers, enabling them to maximize their fitness.
Introduction
When animals are foraging, their efficiency can be defined as the difference in energy gained from ingesting prey relative to the energy expenditures associated with searching for and capturing prey (Parker et al., 1996). Therefore, to increase efficiency, animals must minimize the cost of prey capture (Williams et al., 2000) and/or increase the energetic benefits from prey (Watanabe and Takahashi, 2013; Watanabe et al., 2014). To minimize locomotor costs, aquatic animals adopt gliding gaits, and slow swimming speeds to decrease drag and the energetic requirements of searching for food (Williams, 2001; Fahlman et al., 2008, 2013; Watanabe et al., 2011). To increase energy intake, they can increase feeding rates by extending the time spent in a prey patch (Doniol-Valcroze et al., 2011; Wilson et al., 2011), or selecting prey patches of higher quality (Orians and Pearson, 1979). Higher quality prey can take the form of larger bodied prey for animals that take prey individually (i.e., particle feeders, hunting predators), greater densities of the same type for multiple-prey loaders (i.e., filter feeders or grazers), or other prey changes that decrease handling time or increase catch per unit effort. Moreover, the cumulative benefit from a foraging bout may increase due to a combination of these processes and mechanisms (Daniel et al., 2008; Doniol-Valcroze et al., 2011; Gibb et al., 2016). However, what remains poorly understood is how predators modulate foraging performance when faced with heterogeneous, dynamic prey-scapes (Friedlaender et al., 2016).
Many animals across taxonomic groups and environments are central place foragers where feeding bouts occur far from a return location (Houston and McNamara, 1985). Air-breathing divers can be described as central place foragers, where the sea surface is the return location that provides a critical source of oxygen replenishment (Kramer, 1988). In this context, divers face the conflicting demands of oxygen conservation during apnea and the energetic costs associated with diving, swimming, and feeding (Davis et al., 2004). Therefore, diving foragers must balance managing oxygen stores while maximizing net energy intake during feeding at depth (Hazen et al., 2015). If the high energetic costs of feeding deplete body oxygen stores, this in turn would reduce aerobic diving capacity and thus impose a potential constraint on foraging performance (Croll et al., 2001; Acevedo-Gutiérrez et al., 2002; Goldbogen et al., 2012).
Central place foraging theory predicts that predators should only swim farther from the central place to encounter increasing numbers or quality of prey (Carbone and Houston, 1996). When the same prey resource is targeted, diminishing energetic returns are expected at increasing distances from the central place, because of increased travel time. Thus, at greater distances animals should feed on higher value prey to optimize foraging efficiency (Schoener, 1969, 1979). It is known that toothed whales (Odontoceti) hunt and capture individual prey across a wide size range (Clarke, 1996; Andersen et al., 2016); however it remains unclear whether odontocetes selectively vary feeding rates when feeding at different depths and/or modulate their locomotor and diving performance in response to changes in prey distribution and availability. To test the above hypothesis, we explore the fine-scale foraging behavior and kinematics of the cephalopod-eating Risso's dolphin using motion-sensing and acoustic recording tag data to quantify the foraging performance of this species in the wild.
Materials and Methods
Dolphin Data Collection
Archival tags (Johnson and Tyack, 2003) were attached dorsally to free-ranging Risso's dolphins off Santa Catalina Island, California during field efforts from 2010 through 2016. The tags had a suction-cup system that released at local sunset, or unintentionally due to movement of the dolphins. They were positively buoyant and transmitted a VHF signal when at the surface, allowing tracking, and recovery. Stereo acoustic data were sampled with 16-bit resolution at a sampling rate of 240 kHz, except for tag gg11_216a where 120 kHz was used. Data from the other sensors were sampled at 200 Hz per channel and converted into depth, pitch, roll, and heading of the tagged animal, following methods described in Johnson and Tyack (2003). Pressure and accelerometer data were decimated to 20 and 5 Hz, respectively, before analysis. Analyses were carried out in Matlab 9.1.0 (http://www.mathworks.es/) using the DTAG toolbox (https://www.soundtags.org/dtags/dtag-toolbox/) and custom-made scripts. A subset of 18 tagged dolphins were subject to controlled acoustic playback exposure experiments (CEE) (Table 1). Detailed exposure protocols are described in Southall et al. (2012). Dives were classified as “non-CEE,” “pre-exposure,” “CEE,” or “post-exposure,” depending on the time overlap with the onset and end of the acoustic stimuli. Generalized additive mixed models (GAMMs) were used to assess the effect of CEE mode and type on two representative metrics of foraging performance. We fitted GAMMs using maximum dive depth and buzz rate as response variables, including CEE categorical data as a factor and individual dolphin as random effect, to identify potential behavioral responses as a function of CEE mode (non-CEE, pre-CEE, during-CEE, and post-CEE) and type (non-CEE, Control, PRN, Simul-MFA, Real MFA). As we were testing a specific hypothesis no model selection was needed. This statistical approach allowed us to assess whether there was a behavioral response as a consequence of CEE, which was important to determine if the full Grampus tag dataset could be pooled across CEE modes and types for further analysis. Foraging models were fitted as a functions of dive metrics and of CEE modes and types, using the mgcv 1.8–15 package (Wood, 2011) in R 3.3.2 “Sincere Pumpkin Patch” (http://www.R-project.org/).
Focal follows of tagged animals were conducted whenever possible, avoiding approaches closer than 25 m. Observations were made from the tag boat with the aid of binoculars and VHF radio tracking equipment. Individuals were identified via photos of their dorsal fin and scar pattern, which facilitated tagging different individuals every time. Location of the tagged animals was recorded with a GPS on board and seabed depth at dolphin sighting locations was extracted using NOAA's NGDC bathymetric charts in ArcMap 9.2 (Environmental Systems Resource Institute, Redlands, CA, USA) with 3-arc second resolution (https://www.ngdc.noaa.gov/mgg/coastal/grddas06/grddas06.htm). Average seabed depth was estimated from all positions gathered during each tag deployment and the maximum depth of the deepest dive recorded among the 33 dolphins. This was 560 m, considering that in the deep-water habitat the seabed must be deeper than the deepest dive recorded. In this way dolphins were broadly classified as foraging in a shallow or deep-water habitat (Table 1). Only two of the dolphins (data sets gg14_223a and gg14_253a) were close to the boundary defined by this depth threshold, as they remained above average seabed depths of 450 and 500 m, respectively. Those depths may have allowed deep diving but if so, foraging would likely have occurred close to the seabed, in the benthic boundary layer (Angel and Boxshall, 1990). Given the differences between benthopelagic and pelagic organisms, these dolphins were classified as in a shallow-water habitat. Moreover, data for each dive were analyzed with respect to which habitat the dolphin was in, based on the seabed depth of the closest focal follow position gathered within 15 min of the start or end of a given dive. We acknowledge the low accuracy of these estimates but the seabed depth at the nearest focal follow location was considered a representative measure of the type of foraging habitat used by the animal. Dives without focal follow information within the 15 min time window was not considered for kinematic analyses.
Prey Data Collection
Data on the distribution of prey in the Catalina Basin, off the eastern coast of Santa Catalina Island, California, comprising the deep-water habitat of the dolphins, were obtained from ship and underwater autonomous vehicle (AUV) based hydroacoustic surveys. The ship transects covered seabed depths ranging from 300 to 900 m and the AUV sampled pre-defined depths between 50 and 500 m, depending on the location of prey layers. Both platforms were equipped with Simrad EK60 echo sounders at 38 and 120 kHz. The AUV survey provides a 15 × 10 cm sampling resolution in horizontal and vertical planes, respectively. This allowed individual animals to be observed within scattering features, whereas the ship-based sensors provided a view of entire features. Comprehensive explanation of the sensors and platforms used is provided by Moline et al. (2015) while Benoit-Bird et al. (2017) provide a detailed description of biological sampling and active acoustics methodology. Briefly, acoustic scattering data were processed using Echoview and individual prey detected from the AUV within scattering features were identified as single targets (Sawada et al., 1993), providing measurements of target strength at two frequencies. Frequency differencing was used to facilitate coarse taxonomic classification (i.e., fish, squid, or crustacean) while target strength was used as a proxy of length within each taxonomic class. Calculation of inter-individual spacing of prey in layers was measured using the nearest neighbor distance for each individual target sampled by the AUV, both in the beam and along the track. The dominant composition of layers identified from the active acoustics was determined using a net towed at relatively high speed (1–2.2 m s−1) that captured mobile organisms between 1 and 35 cm body length. This catch matched the composition identified acoustically (Benoit-Bird et al., 2017). Sampling of the deep-water habitat of the dolphins was coincident in space and time with tagging of two of the 33 dolphins (tag id gg13_266b and gg13_267a, Table 1). The spatial coverage of the prey mapping and its overlap with the tag data is presented in Figure 2 in Arranz et al. (2018). Another 15 dolphins were tagged in the same general habitat, 6 of them in the same year. Prey fields in the shallow-water habitat were not sampled in this study. Dolphins in shallow-water habitat were mostly tagged in 2013, when increased upwelling productivity conditions may have influenced prey availability, particularly of market squid (Doryteuthis opalescens) in coastal waters off the island. Hence the dolphin's foraging opportunities were higher close to shore (Vanderzee et al. pers. comm.). This was supported by visual observations during tagging in 2013, when market squid were observed at the surface and in the mouths of Risso's dolphins. Dives occurring within the shallow-water habitat were classified based on their depth distribution as Shallow (<100 m depth) or Intermediate (>100 m), for comparison of dive parameters.
Kinematic Analyses
Dives were defined as vertical excursions exceeding 20 m depth. Dives recorded within 15 min of tag-on were excluded from the analysis, to remove data potentially affected by the tagging procedure (the 15 min duration is roughly equivalent to two deep dives or 5 shallow dives by this species). Incomplete dives at the start or end of the record (caused by a delay in triggering the salt water switch or release at depth) were also excluded. Dives performed in the deep-water habitat were classified according to their maximum depth and the prey feature targeted in the bottom phase (sensu Arranz et al., 2018). Echolocation clicks and buzzes from the tagged dolphin, respectively, indicative of prey search and capture attempts within dives, were isolated using a supervised click detector together with spectrogram visualizations of acoustic recordings, following the methods described in Arranz et al. (2016). The interpretation that buzzes are associated with prey capture attempts has been confirmed for several echolocating marine mammals, including sperm whales (Miller et al., 2004), beaked whales (Johnson et al., 2004), pilot whales (Aguilar de Soto et al., 2008), porpoises (Deruiter et al., 2009), and belugas (Ridgway et al., 2014). Foraging dives were defined as including one or more buzzes. For each dive, the mean descent and ascent depth rates and the proportion of descent time spent gliding were computed. Fluke strokes were identified in the recordings as cyclic variations in the pitch with a magnitude >3 degrees and with a period between 0.4 and 5 s, estimated from a nominal fluking period of 1.5 s for Risso's dolphin. The stroke count was verified visually by checking the pitch angle in random sections of the record. Glides were defined as 10 s time intervals with no stroking. Descent, bottom and ascent phases in dives were defined following a 70% rule, as in Arranz et al. (2016). The descent phase of each dive was considered the period from when the dolphin left the surface to the first time the depth exceeded 70% of the maximum dive depth. The ascent phase started at the last time the depth exceeded 70% of maximum dive depth and ended when the dolphin reached the surface. The bottom phase was defined as the period from the first to the last time the depth exceeded 70% of the maximum dive depth. The inter-buzz interval (IBI) was estimated as the time elapsed since the end of a buzz and the start of the next one. The vertical swim speed was computed as the rate of change in depth (as in Aguilar de Soto et al., 2008).
Energetic Analyses
As a proxy for the activity level of the dolphins we computed the overall dynamic body acceleration (ODBA, Wilson et al., 2006) as the norm of the high-pass filtered acceleration resulting from the movement of the animal and recorded by the tag sensors (ODBA tool from the soundtags toolbox). Cut-on frequency required for ODBA estimation was set to 0.35 Hz (about half of the normal stroking rate for these dolphins). Previous studies have shown that ODBA is a reasonable proxy for metabolic rate in marine mammals (Fahlman et al., 2013) although other studies report difficulty in observing such a relationship (Halsey et al., 2011; Halsey, 2017). An exploratory analysis of this relationship in Risso's dolphins is presented here, assuming that for each individual dolphin, the lowest ODBA recorded on each dive (ODBAmin) corresponded to the , or estimated resting metabolic rate (RMR) and the highest ODBA for each dive (ODBAmax) corresponded to its or estimated maximum metabolic rate.
For comparative purposes, we modeled in three ways (Table S1 in Data Sheet S1): (1) using the measured from the smaller bottlenose dolphin (Tursiops truncatus) (Equation 3.2 in Fahlman et al., 2018) and scaled allometrically for the larger Risso's dolphin, (2) assuming an average mass-specific of 3.9 ml O2 kg min−1 (Figure 3B in Fahlman et al., 2018) and multiplying by the estimated body mass (Mb), or (3) using Kleiber's equation (Equation 1) for terrestrial mammals and the estimated Mb of the tagged dolphin (Kleiber, 1947). The Mb of tagged Risso's dolphins was approximated from individual age class (Table 1), implying a mass of 500 kg for adults and 300 kg for sub-adults and juveniles (Jefferson et al., 2008).
was modeled in two different ways (Table S1 in Data Sheet S1): scaling up (1) by 5 times or Metabolic Equivalents of Task (MET's, Savage et al., 2007), a level of exercise that one would expect animals to undertake routinely; or (2) by the Hoppeler equation (Equation 2) (Weibel and Hoppeler, 2005).
A regression equation was built using estimated , a for each dolphin, in one of the ways described above, estimated ODBAmin and ODBAmax for a given dive. We acknowledge reasonable concerns about generating a regression equation from two data points, but it allowed us to estimate the (or field metabolic rate) of the dive based on the overall activity. Regression lines were re-calculated for each dive to account for different tag placements within the same individual (due to potential tag moves) and likely variation of minimum and maximum ODBA values amongst dolphins (Figure S1 in Data Sheet S1). The resulting intercept and slope of the regression line obtained for a given dive were multiplied either by the average ODBA of the dive+ surface interval, to estimate the average per dive, or by the average ODBA of the dive phase (descent, bottom and ascent) to estimate the average of each phase, as follows:
Recorded ODBAmin and ODBAmax per dive were regressed as a function of duration of the deployment, to rule out correlation between these variables (Figure S2 in Data Sheet S1). The ratio between the estimated metabolic costs of each dive phase in different dive types was used comparatively, to assess relative differences in dolphin metabolic costs when diving to different depths.
Statistical Modeling
To assess differences in dive parameters by dive type, we used generalized linear mixed-effects models fitted in R, using the package glmmTMB (Brooks et al., 2017). We modeled each dive parameter as a function of habitat and dive type, controlling for effects of age class (via fixed effects) and individual differences and temporal autocorrelation (via a random intercept of tag ID). We used Gaussian models with identity link, except for the following parameters. Inter-buzz interval and buzz duration were modeled using gamma family with log link, to account for right-skew and overdispersion of residuals, and for number of buzzes a negative binomial type I with log link and an offset for dive duration, since data are count data, and to account for right skew and overdispersion of residuals. We used ANOVA to compare models with and without habitat and dive type as predictors, and in the case of a significant result, we used Tukey's method to assess pairwise differences between dive types [using R package emmeans (Lenth, 2018)].
Results
A total of 124 h of acoustic and movement data from 33 Risso's dolphins (20 adults, 5 sub-adults, 1 juvenile, and 7 individuals of unknown age) resulted in 331 dives during 22 deployments as analyzed here. Most tags recorded data during daytime only but two documented 2 h 19 min and 3 h 30 min after local sunset, respectively, resulting in an overall time coverage from 7:25 a.m. to 9:57 p.m. local (UTC−7) (Table 1, Figure 1). No clear time-of-day dependent pattern of feeding behavior or inshore-offshore movements were detected from plots of the seabed depth at the focal follow positions of the dolphins in relation to time of day. These results are based on a small sample size without full day/night periods from tag records. GAMMs revealed no significant effect of CEEs on the buzz rate per dive nor the maximum dive depth in any of the 18 out of 33 tagged dolphins subject to the experiments (Table 2).
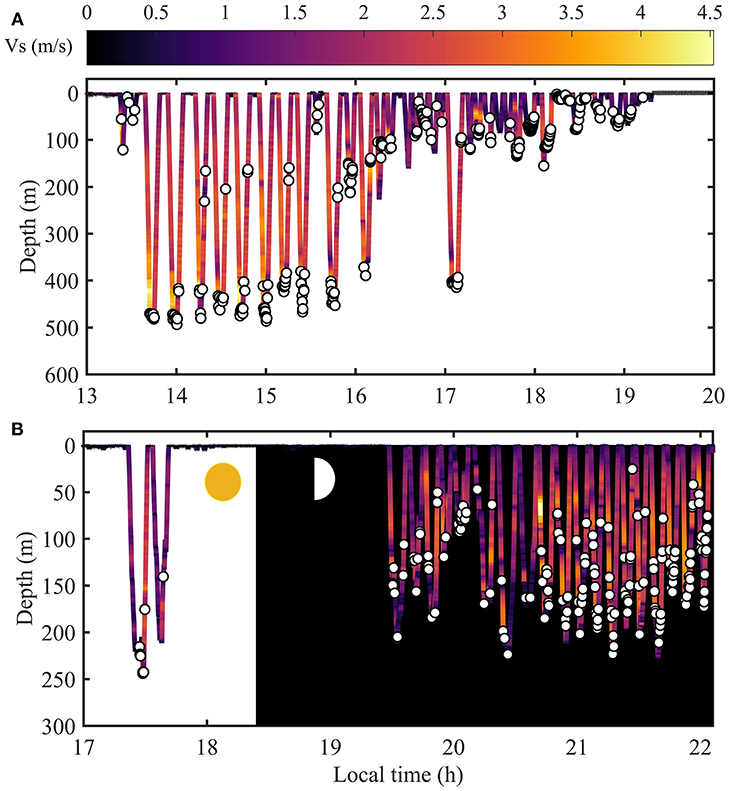
Figure 1. Dive profiles from two Risso's dolphins with (A) the longest period of time with consistent deep dives and (B) the longest night time foraging period recorded, among 33 tagged individuals. Prey capture attempts are represented by white circles. Dive profile is colored according to vertical swim speed (Vs).
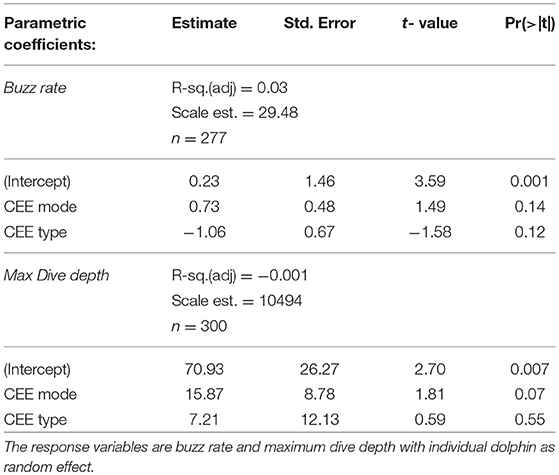
Table 2. Generalized Additive Mixed Model (GAMM) estimates for the effects of mode and type of Controlled Exposure Experiments (CEE) on behavioral criteria of 33 Risso's dolphins.
Habitat
Hydroacoustic sampling of the biotic structure in the water column throughout the Santa Catalina Basin >560 m seabed depth, i.e., deep-water habitat, revealed prey fields vertically stratified into four prey features comprising three sound-scattering layers. Each prey layer was horizontally identified as follows: Shallow [30–90 m], Midwater [200–300 m], and Deep [350–450 m], and a zone of scattered patches between 100 and 200 m depth named Intermediate (see Figure 2 in Arranz et al., 2018). The shallow layer was dominated by larval fish and small crustaceans, the midwater layer by myctophids and krill, and the deep layer primarily by dragonfish, squid, shrimp, and large krill (Benoit-Bird et al., 2017). Scattered patches were not directly sampled to confirm potential composition but echo characteristics were consistent with small schooling fish. Measures of the horizontal patch structure are reported by Benoit-Bird et al. (2017). Prey patch aggregations had similar topological extent, 100 individuals across, irrespective of taxonomic composition, animal size, and depth. Inter-individual spacing increased with relative animal size within each taxonomic group. When Grampus were detected by AUV echosounder sampling in the deep-water habitat of the dolphins, they were found mostly within the boundaries of monospecific aggregations with a frequency response corresponding to squid (Benoit-Bird et al., 2017). About half of the dolphins (N = 17) stayed within the deep-water habitat during most of the recording time, whereas the other animals (N = 16) stayed in shallow-water habitat (Table 1). In the deep-water habitat, 39% of the dives performed by the dolphins were shallow (to a max depth of 30–90 m), 35% intermediate (to a maximum depth of 90–200 m), 15% midwater (to a maximum depth of 200–300 m), and 8% were deep dives (to a maximum depth >350 m).
Foraging Behavior
Dolphins spent 83 (SD 48)% of the time near (<20 m) or at the surface and 17 (SD 35)% of the time diving. The pattern of dive cycles differed among individuals, with variable-duration bouts of foraging dives interspersed with periods at or near the surface (entailing behaviors like resting, traveling, socializing) (Figure 1). Tagged dolphins foraged throughout the day, with search clicks recorded in 76% of dives and buzzes recorded in 62% of dives. Foraging dives lasted 5.0 [0.7–10] min on average [range], covering a broad range of depths up to 588 m (Figure 1). Duration of dives recorded from adult dolphins (N = 279 dives) was 4.3 [1.2–10. 7] min, whereas in non-adults (i.e., juveniles and sub-adults, N = 25 dives) dive duration was 3.8 [1.5–5.7] min. Deep-water foraging was only recorded in adult dolphins (average maximum dive depth 137 ± 119 m [mean ± SD]), all recorded dives by non-adult dolphins were shallower than 141 m depth (average maximum dive depth 59 ± 34 m). Deep dives occurred mostly in the afternoon, since before noon most data were recorded within the shallow habitat. Diurnal patterns of inshore-offshore movement or vice versa were absent when visualizing plots of seabed depth in relation to time of day (average Pearson's correlation across tags R = −0.14). Seabed depths from focal follows revealed that dolphins were foraging near the seabed when in shallow-waters, although benthopelagic feeding may also take place in the deep-water habitat (Figure 2).
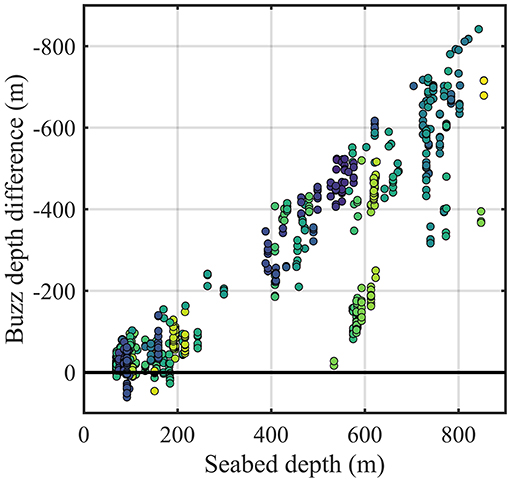
Figure 2. Foraging activity of Risso's dolphins relative to the seabed. Seabed depth from the closest focal follow position gathered within 6 min of the given buzz (x-axis) and difference between buzz depth and seabed depth (y-axis), colored according to individual. Positive differences are the result of the offset between focal follow position from where seabed depth is extrapolated and depth where dolphins are feeding.
Dive Kinematics
Dolphins' descent and ascent rates were up to twice as fast when diving deeper (Spearman's correlation ρ = 0.59, p-value < 0.005, and ρ = 0.65, p-value < 0.005, respectively). They also tended to adopt higher absolute body pitch angles (Spearman's correlation ρ = 0.51, p-value < 0.005) with active stroking on ascent from deeper dives (Table 3). The proportion of time spent gliding relative to the duration of the descent phase of each dive increased up to 5 times with maximum depth of the dive (Spearman's correlation ρ = 0.53, p-value < 0.005), with an associated dramatic drop in fluke stroking rate (Figure 4C). Stroke frequency decreased over the first 30 s of the descent during deep dives, from 15 to < 5 strokes every 20 s, after which they glided for about a minute. After that, they increased stroke frequency again, resulting in high vertical velocities (up to 4.5 m s−1) during the final phase of most descents of deep dives (Figures 1, 4E). Stroking patterns during descents of shallow, intermediate and midwater dives were more variable, with fewer episodes of high vertical swim speed.
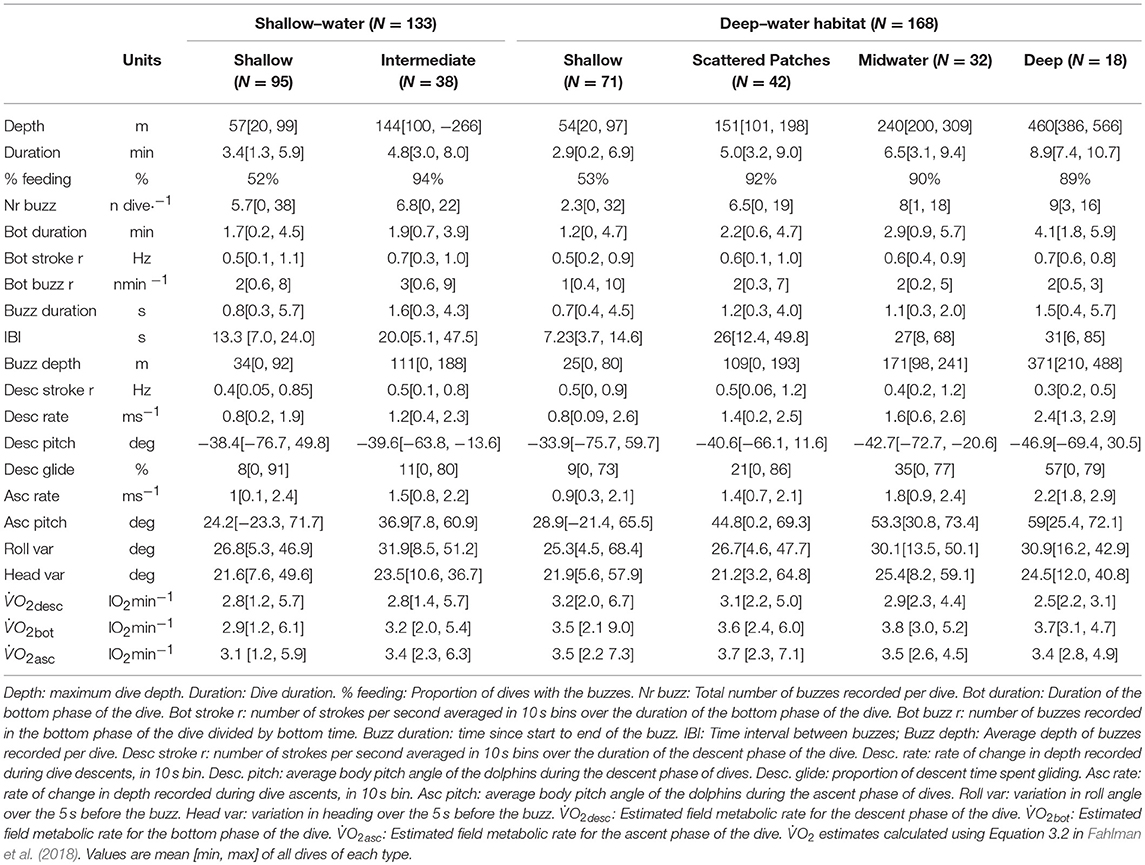
Table 3. Kinematic data for Risso's dolphins foraging dives, pooling dives from all tagged dolphins by habitat type and depth layer.
Deep dives featured on average twice the number of prey capture attempts in the bottom phase (7 ± 3 buzzes) as shallow dives (3 ± 6 buzzes), whereas in scattered patches and midwater dives dolphins featured 5 ± 4 prey capture attempts, respectively. There was a significant correlation between maximum dive depth and number of buzzes performed at the bottom phase of the dive (Spearman's correlation ρ = 0.40, p < 0.0001 N = 307 dives). Considering only bottom phase foraging rates, estimated as the ratio between the number of prey capture attempts at the bottom of dives and the duration of the bottom phase, these ranged between 79 and 134 prey capture attempts h−1 as a function of dive type. Bottom phase foraging rates were on average 79 prey capture attempts h−1 in shallow dives [30–90 m], 134 attempts h−1 in intermediate dives [100–200 m], 112 attempts h−1 in midwater dives [200–300 m], and 102 capture attempts h−1 in deep dives [350–450 m].
Feeding during descent and/or ascent of dives increased the number of prey items targeted in shallow dives by 17% (4 ± 7 buzzes), 40% in scattered patches (6 ± 5 buzzes), 60% in midwater dives (8 ± 3 buzzes), and 28% in deep dives (9 ± 3 buzzes). Overall foraging rates, i.e., the cumulative number of buzzes emitted per dive divided by duration of dives, revealed between 28 and 55 prey attempts per hour, depending on the foraging depth. Dolphins pursued on average 32 ± 52 prey items h−1 in shallow dives, 36 ± 26 items h−1 in intermediate dives, 55 ± 40 items h−1 in midwater dives and 28 ± 20 items h−1 in deep dives.
GLMMs revealed significant differences in buzz rate for different dive types (chisq = 9.83, p-value = 0.02), although pairwise comparisons indicated significant differences only for shallow vs. intermediate dives (Data Sheet S2). Differences in the duration of the bottom-phase of the dive were significant across dive types (chisq = 110.01, p-value = 1.08−23) with differences between intermediate and deep dives, as well as shallow dives and all other dive types. Similarly, mean stroke rate at the bottom phase of the dive differ significantly for all dive types (chisq = 3, p-value = 3.62−8). According to the full model, IBI did not differ by dive type (chisq = 2.69, p-value = 0.44), however pairwise comparison for individual dive types reported significant differences between shallow and deep dives. There was also evidence of a difference in roll variance by dive type (chisq = 8.56, p-value = 0.03), with shallow dives being different from midwater and deep dives. Buzz duration did not vary among dive types (chisq = 4.28, p-value = 0.23). None of the models indicated significant differences in dive response variables when fitted with habitat type as predictor. Figure 3 shows kernel density of the above modeled parameters separated by age class, habitat and dive type.
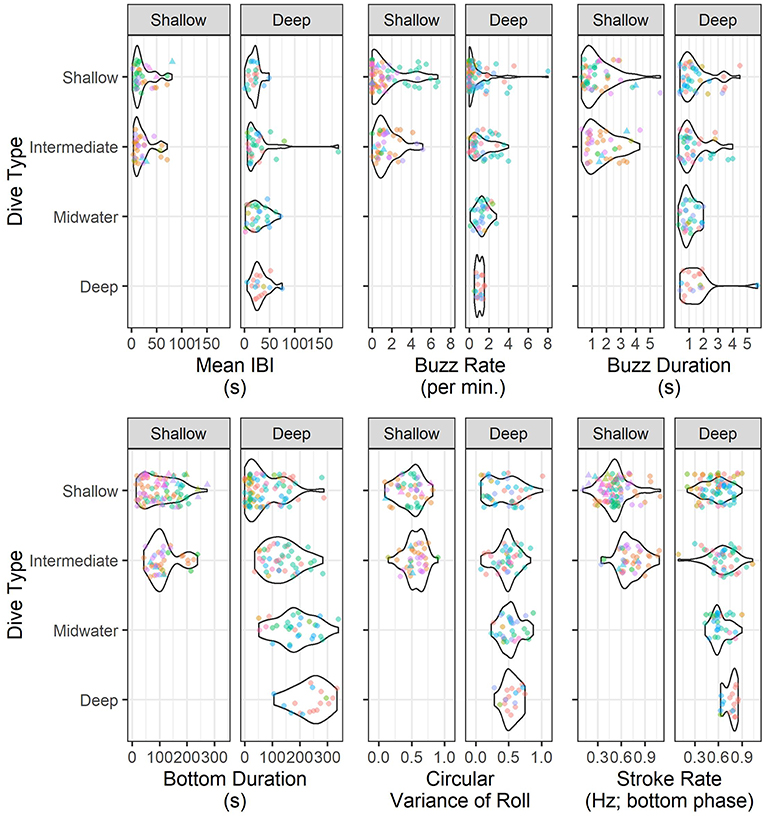
Figure 3. Summary statistics of Risso's dolphins foraging parameters by dive type. Multi-panel violin plot of kernel probability density colored by individual dolphin, different shapes correspond to age class (dots represent adults and triangles non-adults) and separate panels correspond to each habitat. Results of the GLMM (Generalized linear mixed model) fitted for the above parameters with and withouth habitat and dive type as predictors are reported in the text and Data Sheet S2.
Metabolic Costs
GLM results indicated that estimated per dive was similar across dive types and habitats (Figure S3 in Data Sheet S1) but differed between dive phases (chisq = 120.92, p-value = 4.88−26), with descent being different from bottom and ascent estimates (Figure 5, Table 3). Estimated average per dive was 1.22 ± 0.18 l O2 min−1 for shallow dives, 1.19 ± 0.12 l O2 min−1 for scattered patch dives, 1.18 ± 0.12 l O2 min−1 for midwater dives and 1.11 ± 0.09 l O2 min−1 for deep dives, calculated using Equation 3.2 in Fahlman et al. (2018). The second method used, based on Figure 3B in Fahlman et al. (2018), rendered values that doubled or tripled the previous results but were again consistent across dive types: 1.69 ± 0.25 l O2 min−1 for shallow dives, 1.65 ± 0.17 l O2 min−1 for scattered patches dives, 1.63 ± 0.17 l O2 min−1 for midwater dives and 1.53 ± 0.13 l O2 min−1 for deep dives. Estimates using Kleiber's equation resulted in slightly higher estimates but were also similar among dive types: 3.14 ± 0.46 l O2 min−1 for shallow dives, 3.06 ± 0.32 l O2 min−1 for scattered patch dives, 3.03 ± 0.32 l O2 min−1 for midwater dives and 2.85 ± 0.24 l O2 min−1 for deep dives. Hoppeler's equation yielded an upper bound for of 16.8 l O2 min−1 for a 300 kg dolphin and 26.3 l O2 min−1 for a 500 kg dolphin.
Discussion
The Risso's dolphins in this study appear to employ several mechanisms to maximize foraging efficiency, depending on their feeding depth. The dolphins varied their diving kinematics and prey quantity when foraging in prey layers with different composition. They foraged throughout the day in shallow and deep-water habitats, attempting to capture prey at depths up to 488 m. Foraging dives had maximum dive depths up to 560 m and occurred in bouts representing about 20% of a dolphin's daytime activity, although tag-on durations averaged only a few hours and at most 13.5 h. These foraging dives targeted epipelagic to mesopelagic prey resources and may have also included near-benthic and coastal water foraging. Foraging bouts were interspersed by traveling/resting/socializing periods of variable length at the surface, which may be needed for recovery. Diving patterns were stereotyped when foraging at particular depths irrespective of the habitat type. They altered their activity for different types of dives in response to metabolic demands, indicating that their foraging tactics were influenced by foraging costs and benefits.
When feeding in the shallow-water habitat, buzz depth and seabed depth at focal follow positions point to dolphins targeting benthic organisms during some of these dives. Opportunistic observations report Risso's dolphins feeding on non-gregarious benthic prey, like octopods in very shallow-waters (Ruiz et al., 2011). Stomach contents from stranded specimens from another location reveal that the benthic octopus Eledone cirrosa can represent up to 55% of the cephalopod prey consumed by this species (Clarke and Pascoe, 1985; Blanco et al., 2006), which would support the potential benthic feeding strategy inferred from data presented here. Nevertheless, foraging patterns were comparable when diving at similar depths but in different habitats.
Furthermore, dolphins feeding in deep-water exhibited several adaptations to reduce the energy cost of locomotion when searching for food at different depths, such as the use of gliding gaits and higher pitch angles during ascent and descent (Table 3). Therefore, despite the longer transit distance to forage for deep-water resources, the dolphins managed to keep their average field metabolic rate similar across dive types (i.e., shallow, intermediate, midwater, and deep). While our approach to estimate field metabolic rate relies on the assumption that the lowest and highest ODBA for each dive represent resting and maximal metabolic rate. This assumption may not be true for each individual dive, but this analysis allows us to explore the relative metabolic costs within dives, and accounts for possible changes in tag placement on overall ODBA. In addition, the heterogeneity in the regression estimate between ODBA and metabolic rate within an individual between dives was acceptable (C.V. of 32% for slope and of 0.7% for intercept), suggesting that there were not large variation in estimated costs between dives. It has been suggested that glide and stroke behavior helps conserve energy (Williams et al., 2017). Gliding during dive descent may help to reduce the metabolic costs of deep dives up to 40% to balance their need to perform long ascents while fluking actively. Similarly, Steller sea-lions diving to artificial prey patches from 5 to 50 m in experimental studies have been observed to decrease their overall (average) diving metabolic rate and activity level with dive depth (Fahlman et al., 2009, 2013). These adaptations may allow them to extend their time at depth in deeper dives and to make a greater number of prey attempts at the bottom of the dive; although bottom buzz rates remained comparable for shallow vs. deep dives. Foraging during transits from (and to a lesser extent to) foraging depths slightly increases the overall foraging rate during midwater dives compared to intermediate dives, but not in deep dives. This represented about a quarter (25%) of prey caught in deep dives, however if dolphins were still diving deep to forage, it suggests intermediate and midwater prey is of lower quality, with respect to prey energy content and/or prey density per unit volume, compared to deeper prey.
Hydroacoustic sampling within the same general area did not find greater prey density at depth (Benoit-Bird et al., 2017). Active acoustics data revealed that inter-individual distances in schools targeted by Risso's dolphins increased with total body length of prey items (Benoit-Bird et al., 2017). Moreover, the scattering layers were stratified vertically in terms of composition and size. The average length of individuals constituting mono-specific prey patches in the Catalina basin generally increased with depth, while the average density of prey in patches decreased. It therefore seems that the dolphins benefit from deeper foraging trips through accessing prey of varied quality rather than greater prey density. The longer IBI associated with deeper buzzes is consonant with dolphins targeting more widely spaced prey items (i.e., larger distance between individuals) during deep dives or that it requires longer handling times, an interpretation compatible with prey data reported here.
The records of higher in the bottom phase of deep dives is consistent with the registered increase in body roll and stroke rate and suggest a greater effort involved in the pursuit of deep-water prey items. These results support the notion of shift in prey type with increasing foraging depth and could be related to the observed escalation of prey length as a function of depth. Stomach contents from stranded Risso's dolphins reveal consumption of large mesopelagic cephalopods, including the family of muscular squid Ommastrephiidae. Examples are the jumbo squid Dosidicus gigas (García-Godos and Cardich, 2010; Yates and Palavecino-Sepúlveda, 2011) or the neon flying squid Ommastrephes bartramii (Fernández et al., 2017). Such squid, including species from genera Todarodes, have strong locomotor abilities (O'dor and Webber, 1991) and present high energy density per unit of wet weight (3–4.5 kJ g−1 wet weight Clarke et al., 1985), constituting a potential high-quality target for Risso's dolphins during deep dives.
Data on swimming speeds of the dolphins while foraging at the bottom could not be estimated reliably in this study (but see Cade et al., 2017), however the relatively high vertical swimming speeds recorded in the bottom phase of deep dives (up to 4.5 m s−1) (Figure 4), are coherent with dolphins chasing prey at depth. Deep-water sprints associated with capturing large squid have been described in short-finned pilot whales (Globicephala macrorhynchus) (Aguilar de Soto et al., 2008). Similarly, Aoki et al. (2012) reported bursts of speed in sperm whales (Physeter macrocephalus), suggesting that they performed these bursts to catch powerful and nutritious deep-water prey (i.e., large and/or muscular). In deep dives, we found no strong evidence of longer buzzes that have been associated with the capture of larger prey items in beaked whales (Johnson et al., 2008), although it may depend on the clicking rate and closing speed at which targets are approached. Nevertheless, the apparently high levels of exercise undertaken by Risso's dolphins during deep-water feeding recorded here are highly significant, together with the measured increase of total body length of prey items in schools targeted by these dolphins as a function of depth. This evidence points to a potential common strategy of some deep-diving toothed whales, in which the capture of larger, more nutritious prey in deep-waters may compensate for the higher metabolic costs of performing longer foraging dives at depth.
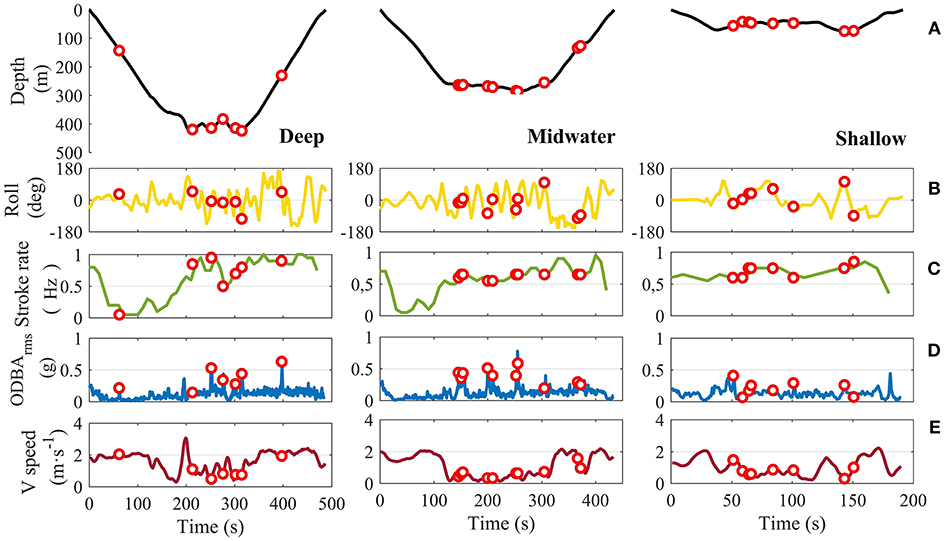
Figure 4. Representative examples of fine-scale kinematics of a Risso's dolphin feeding in a deep-water habitat during deep, midwater, and shallow dives (dolphin with tag id gg13_267a). Dive profiles with buzzes in red (A) and associated measurements of body roll (B), fluke stroke rate (C), ODBArms (D) and vertical swim speed (E) during the first dive of each type recorded on this tag (15 min after the tag on). Red dots indicate prey capture attempts (buzzes). ODBArms corresponds to the root-mean-square (rms) of the 2-norm overall dynamic body acceleration (ODBA). Shallow and midwater dives started 5 min before and 12 min after the start of the deep dive, respectively, by the same dolphin and within the same general habitat. Note the gliding periods during the second part of the descent during deep and midwater dives.
Some deep diving species, such as sperm whales and pilot whales, adapt to circadian migrations of prey distribution (Watwood et al., 2006; Aoki et al., 2007; Aguilar de Soto et al., 2008) and exploit deep-water resources when they are available at shallower depths at night. Other species, like Blainville's beaked whales (Mesoplodon densirostris) seem to adjust little (Arranz et al., 2011). All prey layers in the deep-water habitat of Risso's dolphins, other than the deepest, have been described to perform diel vertical migrations (Benoit-Bird et al., 2017). It is therefore possible that these dolphins benefit from the availability of deep-water prey in shallower waters at night, so as to reduce the apparently higher energetic costs involved in foraging at depth. Soldevilla et al. (2010) reported higher click detection rates of Risso's dolphins at night within the Catalina basin, suggesting either a higher feeding rate or shallower foraging depths. A few data about night-time foraging behavior of Risso's dolphins presented here are inconclusive, limiting interpretation of whether, and to what extent, this species adapts to circadian changes in prey distribution. Collection of night-time tag data on foraging dolphins would be needed to better understand the feeding patterns of this species.
A number of methods have been developed to estimate metabolic rate in free-ranging marine mammals. In pinnipeds and smaller cetaceans, indirect measurements have been made using doubly labeled water, or species-specific calibrations of proxies of energy expenditure, such as heart rate and activity (Butler et al., 2004; Maresh et al., 2014). In larger cetaceans, heart rate has seldom been measured in situ, nor has the relationship between activity and metabolic cost been validated. However, activity correlates reasonably well with diving metabolic rate in Steller sea-lions (Fahlman et al., 2008, 2013). Recent work has also determined the RMR during restrained near-shore shallow diving or off-shore deep-diving in bottlenose dolphins undergoing capture-release health assessments (Fahlman et al., 2018). Indeed, there is a relative similarity in the deep diving capacity, anatomy, morphology, and body structure of bottlenose dolphins and Risso's dolphins. Despite the fact bottlenose dolphins are within Delphininae and Risso's dolphins within Globicephalinae, groups separated by about 10 million years of evolution, we hypothesize that a comparable relationship exists in Risso's dolphin. While we have made the assumption that the maximal and minimal ODBA represent maximal and resting , respectively, we cannot confirm that these are true estimates of these two measures. This is especially true for the resting ODBA, where the variability was higher for tag durations that were < 4 h (Figures S1, S2). However, for maximal ODBA and minimal ODBA for tag durations > 4 h and up to 13.5 h there were no systematic trends with tag duration. We therefore argue that if these estimated minimal or maximal values were grossly over- or underestimated, we would have seen at least one outlier for 13 dolphins with an overall tag duration of 92 h. We also used two independent methods, based on Kleiber's equation (Kleiber, 1947) and Fahlman et al. (2018), to estimate the RMR in free-ranging Risso's dolphins. This was to address the caveats associated with the application of respirometry data from restrained bottlenose dolphins in relatively warm water, since temperature differences have a potential impact. Consequently, the estimated absolute metabolic rate is higher in the larger Risso's dolphin, whereas the mass-specific metabolic rate is lower (Lavigne et al., 1986). Although the results of the three methods varied between 1 and 3 l O2 min−1, they all reveal a consistent overall consumption rate across dive types (Figure 5), indicating that Risso's dolphins compensate for energetic costs associated with diving, swimming and feeding at different depths (Davis et al., 2004).
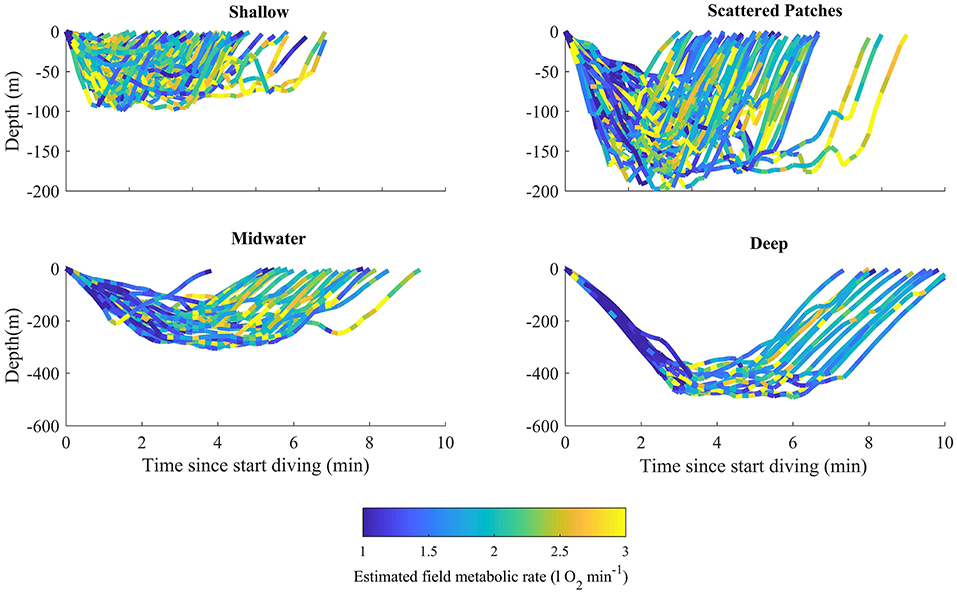
Figure 5. Estimated field metabolic rate of Risso's dolphins in foraging dives performed within the deep-water habitat. Colored lines represent estimates of the field metabolic rate as a function of dive time and depth of the dolphin for each dive type, showing maximum estimated value per every 10 s window.
The calculated aerobic dive limit (cADL), the total O2 stores divided by the diving , provides a useful index to indicate whether the animal mainly forages aerobically or possibly uses anaerobic pathways (Butler and Jones, 1997). The majority of diving animals are thought to perform most dives well within their cADL, since this maximizes long-term foraging efficiency (Costa, 2001). Nevertheless, there are some notable examples like fur seals that appear to regularly dive outside their cADL (Costa et al., 2001). In the current study, we used the estimated mass-specific O2 stores from the bottlenose dolphin [36 ml kg−1, see Table 5 in Ponganis (2011)] and the average diving for each dive type (via Equation 3.2 in Fahlman et al., 2018) to estimate cADL. This resulted in a cADL ranging between 14.8 and 16.2 min and 8.9–9.7 min for adult and non-adult Risso's dolphins, respectively. While our estimated diving entail a number of uncertainties, they provide an initial estimate of energy use and indicate that all dives in the current study are apparently within the estimated cADL.
The Risso's dolphins in this study fed in shallow and deep-water habitats, foraging in different depth layers in deep-waters (occasionally within the same dive) and possibly benthically near the shore when in shallow-waters. In order to balance the energetic needs and costs of foraging at increased distances from the surface, these dolphins altered their dive kinematics to minimize energy consumption during longer transits and extended feeding time with increased depth. They thus allowed for a higher number of prey encounters per dive. Moreover, in spite of the increased overall cost of deep dives, they left longer intervals between prey capture attempts during deep dives. Even faced with the increased need to manage oxygen during deeper and longer dives, prey capture appeared to be more energetic during deep foraging. To maintain energetic returns at increasing distances from the surface, Risso's dolphins appear to choose to feed on larger prey at increasing depth, which points to a potential increased prey size-distance relationship for these predators. This strategy is however difficult to measure in free-ranging diving animals. This adaptive strategy appears to increase their foraging efficiency both within and between foraging bouts, thus enhancing their fitness throughout different habitats. Together with this, these predators show an apparent flexibility in using a variety of resources while maintaining their average metabolic rates per dive similar across foraging depths.
Data Availability
Datasets are available on request. The raw data supporting the conclusions of this manuscript will be made available by the authors, without undue reservation, to any qualified researcher.
Author Contributions
ASF, JC, BS, EH, JG, AS, SD, KB-B, and PA carried out the experiments. KB-B, BS, PT, JG, ASF, AF, PA conceived the presented idea. KB-B, EH, ASF, AS, SD, and PA performed the computations. PA wrote the manuscript with support from JG, AF, and PT. The project was supervised and funding provided by KB-B, BS, PT, and JC. All authors discussed the results and contributed to the final manuscript.
Funding
Funding for the SOCAL-BRS project was provided by the Chief of Naval Operations Environmental Readiness Division, the US Navy's Living Marine Resources Program, and the Office of Naval Research Marine Mammal Program. Experiments were performed under the US National Marine Fisheries Service (NMFS) (Permit # 14534-2), Channel Islands National Marine Sanctuary (Permit # 2010-003) (BS principal investigator for both) and IACUC permits issued to the project investigators. The Strategic Environmental Research and Development Program via an Army Corps of Engineers Contract (KB-B and BS) provided funding for data collection and prey analysis. PT acknowledges support from ONR grant N00014-15-1-2553 and from the MASTS pooling initiative (Marine Alliance for Science and Technology for Scotland; supported by the Scottish Funding Council, grant reference HR09011, and contributing institutions).
Conflict of Interest Statement
The authors declare that the research was conducted in the absence of any commercial or financial relationships that could be construed as a potential conflict of interest.
The handling editor is currently co-organizing a Research Topic with one of the authors, AF, and confirms the absence of any other collaboration.
Acknowledgments
We would like to thank Selene Fregosi for endless logistical support and effort in processing visual data, the crew of the R/V Truth for their help in the field and the two reviewers for their critical review of this manuscript. The authors wish to acknowledge the University of St Andrews Library Open Access Fund for support with Open Access costs.
Supplementary Material
The Supplementary Material for this article can be found online at: https://www.frontiersin.org/articles/10.3389/fevo.2019.00053/full#supplementary-material
References
Acevedo-Gutiérrez, A., Croll, D. A., and Tershy, B. R. (2002). High feeding costs limit dive time in the largest whales. J. Exp. Biol. 205, 1747–1753.
Aguilar de Soto, N., Johnson, M., Madsen, P. T., Díaz, F., Domínguez, I., Brito, A., et al. (2008). Cheetahs of the deep sea: deep foraging sprints in short finned pilot whales off tenerife (Canary Islands). J. Anim. Ecol. 77, 936–947. doi: 10.1111/j.1365-2656.2008.01393.x
Andersen, K. H., Berge, T., Gonçalves, R. J., Hartvig, M., Heuschele, J., Hylander, S., et al. (2016). Characteristic sizes of life in the oceans, from bacteria to whales. Ann. Rev. Mar. Sci. 8, 217–241. doi: 10.1146/annurev-marine-122414-034144
Angel, M. V., and Boxshall, G. A. (1990). Life in the benthic boundary layer: connections to the mid-water and sea floor. Philo. Transac. R. Soc. Lond. Series A 331, 15–28. doi: 10.1098/rsta.1990.0053
Aoki, K., Amano, M., Mori, K., Kourogi, A., Kubodera, T., and Miyazaki, N. (2012). Active hunting by deep-diving sperm whales: 3d dive profiles and maneuvers during bursts of speed. Mar. Ecol. Progr. Ser. 444, 289–301. doi: 10.3354/meps09371
Aoki, K., Amano, M., Yoshioka, M., Mori, K., Tokuda, D., and Miyazaki, N. (2007). Diel diving behavior of sperm whales off japan. Mar. Ecol. Progr. Ser. 349, 277–287. doi: 10.3354/meps07068
Arranz, P., Aguilar de Soto, N., Madsen, P. T., Brito, A., Bordes, F., and Johnson, M. (2011). Following a foraging fish-finder: diel habitat use of blainville's beaked whales revealed by echolocation. PLoS ONE 6:E28353. doi: 10.1371/journal.pone.0028353
Arranz, P., Benoit-Bird, K. J., Brandon Southall, L., John, C., Ari, S., Friedlaender, A., et al. (2018). Risso's Dolphins Plan Foraging Dives. J. Exp. Biol. 221:jeb165209. doi: 10.1242/jeb.165209
Arranz, P., Deruiter, S. L., Stimpert, A. K., Neves, S., Friedlaender, A. S., Goldbogen, J. A., et al. (2016). Discrimination of fast click series produced by tagged risso's dolphins (Grampus Griseus) for echolocation or communication. J. Exp. Biol. 219, 2898–2907. doi: 10.1242/jeb.144295
Benoit-Bird, K. J., Moline, M. A., and Southall, B. L. (2017). Prey in oceanic sound scattering layers organize to get a little help from their friends. Limnol. Oceanogr. 62, 2788–2798. doi: 10.1002/lno.10606
Blanco, C., Raduán, M. A., and Raga, J. A. (2006). Diet of risso's dolphin (Grampus Griseus) in the western mediterranean sea. Sci. Mar. 70, 407–411.
Brooks, M. E., Kristensen, K., Van Benthem, K. J., Magnusson, A., Berg, C. W., Nielsen, A., et al. (2017). Glmmtmb balances speed and flexibility among packages for zero-inflated generalized linear mixed modeling. R J. 9, 378–400. doi: 10.32614/RJ-2017-066
Butler, P. J., Green, J. A., Boyd, I. L., and Speakman, J. R. (2004). Measuring metabolic rate in the field: the pros and cons of the doubly labelled water and heart rate methods. Func. Ecol. 18, 168–183. doi: 10.1111/j.0269-8463.2004.00821.x
Butler, P. J., and Jones, D. R. (1997). Physiology of diving birds and mammals. Physiol. Rev. 77 837–899. doi: 10.1152/physrev.1997.77.3.837
Cade, D. E., Barr, K. R., Calambokidis, J., Friedlaender, A. S., and Goldbogen, J. A. (2017). Determining forward speed from accelerometer jiggle in aquatic environments. J. Exp. Biol. 221(Pt 2):jeb170449. doi: 10.1242/jeb.170449
Carbone, C., and Houston, A. I. (1996). The optimal allocation of time over the dive cycle: an approach based on aerobic and anaerobic respiration. Anim. Behav. 51, 1247–1255. doi: 10.1006/anbe.1996.0129
Clarke, A., Clarke, M. R., Holmes, L. J., and Waters, T. D. (1985). Calorific values and elemental analysis of eleven species of oceanic squids (Mollusca: Cephalopoda). J. Mar. Biol. Assoc. U.K. 65, 983–986. doi: 10.1017/S0025315400019457
Clarke, M., and Pascoe, P. (1985). The stomach contents of a risso's dolphin (Grampus Griseus) stranded at thurleston, south devon. J. Mar. Biol. Assoc. U.K. 65, 663–665. doi: 10.1017/S0025315400052504
Clarke, M. R. (1996). Cephalopods as prey. part 3. cetaceans. philosophical transactions of the royal society of london. Ser. B. Biol. Sci. 352, 1105–1112.
Costa, D. P. (2001). “Diving Physiology of Marine Vertebrates,” in eLS (Chichester: John Wiley and Sons, Ltd.) doi: 10.1002/9780470015902.a0004230
Costa, D. P., Gales, N. J., and Goebel, M. E. (2001). Aerobic dive limit: how often does it occur in nature? Compar. Biochem. Physiol. 129, 771–783. doi: 10.1016/S1095-6433(01)00346-4
Croll, D. A., Acevedo-Gutiérrez, A., Tershy, B. R., and Urbán-Ramírez, J. (2001). The Diving Behavior Of Blue And Fin Whales: Is Dive Duration Shorter Than Expected Based On Oxygen Stores? Compar. Biochem. Physiol. Part A. 129, 797–809. doi: 10.1016/S1095-6433(01)00348-8
Daniel, S., Korine, C., and Pinshow, B. (2008). Central-place foraging in nursing, arthropod-gleaning bats. Can. J. Zool. 86, 623–626. doi: 10.1139/Z08-041
Davis, R. W., Polasek, L., Watson, R., Fuson, A., Williams, T. M., and Kanatous, S. B. (2004). The diving paradox: new insights into the role of the dive response in air-breathing vertebrates. Compar. Biochem. Physiol. Part A. 138, 263–268. doi: 10.1016/j.cbpb.2004.05.003
Deruiter, S. L., Bahr, A., Blanchet, M.-A., Hansen, S. F., Kristensen, J. H., Madsen, P. T., et al. (2009). Acoustic behaviour of echolocating porpoises during prey capture. J. Exp. Biol. 212, 3100–3107. doi: 10.1242/jeb.030825
Doniol-Valcroze, T., Lesage, V., Giard, J., and Michaud, R. (2011). Optimal foraging theory predicts diving and feeding strategies of the largest marine predator. Behav. Ecol. 22, 880–888. doi: 10.1093/beheco/arr038
Fahlman, A., Brodsky, M., Wells, R., Mchugh, K., Allen, J., Barleycorn, A., et al. (2018). Field energetics and lung function in wild bottlenose dolphins Tursiops truncatus in sarasota bay florida. R. Soc. Open Sci. 17:171280. doi: 10.1098/rsos.171280
Fahlman, A., Hooker, S. K., Olszowka, A., Bostrom, B. L., and Jones, D. R. (2009). Estimating the effect of lung collapse and pulmonary shunt on gas exchange during breath-hold diving: the scholander and kooyman legacy. Res. Physiol. Neurobiol. 165, 28–39. doi: 10.1016/j.resp.2008.09.013
Fahlman, A., Svärd, C., Rosen, D. A. S., Wilson, R. P., and Trites, A. W. (2013). Activity as a proxy to estimate metabolic rate and to partition the metabolic cost of diving vs. breathing in pre- and post-fasted steller sea lions. Aquat. Biol. 18, 175–184. doi: 10.3354/ab00500
Fahlman, A., Wilson, R., Svärd, C., Rosen, D. A. S., and Trites, A. W. (2008). Activity and diving metabolism correlate in steller sea lion Eumetopias Jubatus. Aquat. Biol. 2, 75–84. doi: 10.3354/ab00039
Fernández, A., Sierra, E., Díaz-Delgado, J., Sacchini, S., Sánchez-Paz, Y., Suárez-Santana, C., et al. (2017). Deadly acute decompression sickness in risso's dolphins. Sci. Rep. 7:13621. doi: 10.1038/s41598-017-14038-z
Friedlaender, A. S., Johnston, D. W., Tyson, R. B., Kaltenberg, A., Goldbogen, J., Stimpert, A. K., et al. (2016). Multiple-stage decisions in a marine central-place forager. R. Soc. Open Sci. 3:160043. doi: 10.1098/rsos.160043
García-Godos, I., and Cardich, C. (2010). First mass stranding of risso's dolphins (Grampus griseus) in peru and its destiny as food and bait. Mar. Biodiver. Records 3, 1–4. doi: 10.1017/S1755267209991084
Gibb, H., Andersson, J., and Johansson, T. (2016). Foraging loads of red wood ants: Formica Aquilonia (Hymenoptera: Formicidae) in relation to tree characteristics and stand age. Peerj 4:E2049. doi: 10.7717/peerj.2049
Goldbogen, J. A., Calambodkis, J., Croll, D. A., Mckenna, M. F., Oleson, E., Potvin, J., et al. (2012). Scaling of lunge feeding performance in rorqual whales: mass-specific energy expenditure increases with body size and progressively limits diving capacity. Func. Ecol. 26, 216–226. doi: 10.1111/j.1365-2435.2011.01905.x
Halsey, L. G. (2017). Relationships grow with time: a note of caution about energy expenditure-proxy correlations, focussing on accelerometry as an example. Func. Ecol. 31, 1176–1183. doi: 10.1111/1365-2435.12822
Halsey, L. G., Shepard, E. L. C., and Wilson, R. P. (2011). Assessing the development and application of the accelerometry technique for estimating energy expenditure. Compar. Biochem. Physiol. Part A 158, 305–314. doi: 10.1016/j.cbpa.2010.09.002
Hazen, E. L., Friedlaender, A. S., and Goldbogen, J. A. (2015). Blue whales (balaenoptera musculus) optimize foraging efficiency by balancing oxygen use and energy gain as a function of prey density. Sci. Adv. 1:e1500469. doi: 10.1126/sciadv.1500469
Houston, A. I., and McNamara, J. M. (1985). A general theory of central-place foraging for single-prey loaders. Theor. Popul. Biol. 28, 233–262. doi: 10.1016/0040-5809(85)90029-2
Jefferson, T. A., Webber, M. A., Pitman, R., and Jarrett, B. (2008). Marine Mammals of the World: a Comprehensive Guide to Their Identification. London: Academic Press/Elsevier, 573.
Johnson, M., Hickmott, L. S., Aguilar Soto, N., and Madsen, P. T. (2008). Echolocation behaviour adapted to prey in foraging blainville's beaked whale (Mesoplodon Densirostris). Proc. R. Soc. B 275, 133–139. doi: 10.1098/rspb.2007.1190
Johnson, M., Madsen, P. T., Zimmer, W. M. X., Aguilar Soto, N., and Tyack, P. L. (2004). Beaked whales echolocate on prey. Biol. Lett. 271, S383–S386. doi: 10.1098/rsbl.2004.0208
Johnson, M., and Tyack, P. L. (2003). A digital acoustic recording tag for measuring the response of wild marine mammals to sound. IEEE J. Oceanic Eng. 28, 3–12. doi: 10.1109/JOE.2002.808212
Kleiber, M. (1947). Body size and metabolic rate. Physiol. Rev. 27, 511–541. doi: 10.1152/physrev.1947.27.4.511
Kramer, D. L. (1988). The behavioral ecology of air breathing by aquatic animals. Can. J. Zool. 66, 89–94. doi: 10.1139/z88-012
Lavigne, D. M., Innes, S., Worthy, G. A. J., Kovacs, K. M., Schmitz, O. J., and Hickie, J. P. (1986). Metabolic rates of seals and whales. Can. J. Zool. 64, 279–284. doi: 10.1139/z86-047
Lenth, R. (2018). Emmeans: Estimated Marginal Means, Aka Least-Squares Means. R Package Version 1.3.0. Available online at: https://cran.r-project.org/package=emmeans
Maresh, J. L., Simmons, S. E., Crocker, D. E., Mcdonald, B. I., Williams, T. M., and Costa, D. P. (2014). Free-swimming northern elephant seals have low field metabolic rates that are sensitive to an increased cost of transport. J. Exp. Biol. 217, 1485–1495. doi: 10.1242/jeb.094201
Miller, P. J. O., Johnson, M. P., Tyack, P. L., and Terray, E. A. (2004). Swimming gaits, passive drag and buoyancy of diving sperm whales Physeter macrocephalus. J. Exp. Biol. 207, 1953–1967. doi: 10.1242/jeb.00993
Moline, M. A., Benoit-Bird, K., O'Gorman, D., and Robbins, I. C. (2015). Integration of scientific echo sounders with an adaptable autonomous vehicle to extend our understanding of animals from the surface to the bathypelagic. J. Atmos. Ocean. Technol. 32, 2173–2186. doi: 10.1175/JTECH-D-15-0035.1
O'dor, R. K., and Webber, D. M. (1991). Invertebrate athletes: trade-offs between transport efficiency and power density in cephalopod evolution. J. Exp. Biol. 160, 93–112.
Orians, G. H., and Pearson, N. E. (1979). “On The Theory Of Central Place Foraging,” in Analysis of Ecological Systems, eds D. J. Horn, R. D. Mitchell, and G. R.Stairs (Columbus, OH: The Ohio State University Press), 154–177.
Parker, K. L., Gillingham, M. P., Hanley, T. A., and Robbins, C. T. (1996). Foraging efficiency: energy expenditure versus energy gain in free-ranging black-tailed deer. Can. J. Zool. 74, 442–450. doi: 10.1139/z96-051
Ridgway, S. H., Moore, P. W., Carder, D. A., and Romano, T. A. (2014). Forward shift of feeding buzz components of dolphins and belugas during associative learning reveals a likely connection to reward expectation, pleasure and brain dopamine activation. J. Exp. Biol. 217, 2910–2919. doi: 10.1242/jeb.100511
Ruiz, L., Neves, S., Martín, V., Pérez-Gil, M., Tejedor, M., Pérez-Gil, E. Brederlau, et al. (2011). Risso's Dolphin (Grampus Griseus) Population Characteristics Of Canary Islands With An Observation On Octopus Predation. dissertation, Society For The Study Of Cetaceans In The Canary Archipelago (Secac).
Savage, P. D., Toth, M. J., and Ades, P. A. (2007). A re-examination of the metabolic equivalent concept in individuals with coronary heart disease. J. Cardiopulm. Rehabil. Preven. 27, 143–148. doi: 10.1097/01.HCR.0000270693.16882.d9
Sawada, K., Furusawa, M., and Williamson, M. (1993). Conditions for the precise measurement of fish target strength in situ. Fish. Sci. 20, 15–21.
Schoener, T. W. (1969). Models of optimal size for solitary predators. Am. Nat. 103, 277–313. doi: 10.1086/282602
Schoener, T. W. (1979). Generality of the size-distance relation in models of optimal feeding. Am. Nat. 114, 902–914. doi: 10.1086/283537
Soldevilla, M. S., Wiggins, S. M., and Hildebrand, J. A. (2010). Spatial and temporal patterns of risso's dolphin echolocation in the southern california bight. J. Acoust. Soc. Am. 127, 120–132. doi: 10.1121/1.3257586
Southall, B., Moretti, D., Abraham, B., Calambokidis, J., Deruiter, S., and Tyack, P. L. (2012). Marine mammal behavioral response studies in southern california: advances in technology and experimental methods. Mar. Tech. Soc. J. 46, 48–59. doi: 10.4031/MTSJ.46.4.1
Watanabe, Y. Y., Ito, M., and Takahashi, A. (2014). Testing optimal foraging theory in a penguin–krill system. Proc. R. Soc. B 281:20132376. doi: 10.1098/rspb.2013.2376
Watanabe, Y. Y., Sato, K., Watanuki, Y., Takahashi, A., Mitani, Y., Amano, M., et al. (2011). Scaling of swim speed in breath-hold divers. J. Anim. Ecol. 80, 57–68. doi: 10.1111/j.1365-2656.2010.01760.x
Watanabe, Y. Y., and Takahashi, A. (2013). Linking animal-borne video to accelerometers reveals prey capture variability. Proc. Natl. Acad. Sci. U.S.A. 110, 2199–2204. doi: 10.1073/pnas.1216244110
Watwood, S. L., Miller, P. J. O., Johnson, M. P., Madsen, P. T., and Tyack, P. L. (2006). Deep-diving foraging behavior of sperm whales (Physeter macrocephalus). J. Anim. Ecol. 75, 814–825. doi: 10.1111/j.1365-2656.2006.01101.x
Weibel, E. R., and Hoppeler, H. (2005). Exercise-induced maximal metabolic rate scales with muscle aerobic capacity. J. Exp. Biol. 208, 1635–1644. doi: 10.1242/jeb.01548
Williams, T. M. (2001). Intermittent swimming by mammals: a strategy for increasing energetic efficiency during diving1. Am. Zool. 41, 166–176. doi: 10.1668/0003-1569(2001)041[0166:ISBMAS]2.0.CO;2
Williams, T. M., Davis, R. W., Fuiman, L. A., Francis, J., Le Boeuf, B. J., Horning, M., et al. (2000). Sink or swim: strategies for cost-efficient diving by marine mammals. Science 288, 133–136. doi: 10.1126/science.288.5463.133
Williams, T. M., Kendall, T. L., Richter, B. P., Ribeiro-French, C. R., John, J. S., Odell, K. L., et al. (2017). Swimming and diving energetics in dolphins: a stroke-by-stroke analysis for predicting the cost of flight responses in wild odontocetes. J. Exp. Biol. 220, 1135–1145. doi: 10.1242/jeb.154245
Wilson, R. P., Mcmahon, C. R., Quintana, F., Frere, E., Scolaro, A., Hays, G. C., et al. (2011). N-Dimensional animal energetic niches clarify behavioural options in a variable marine environment. J. Exp. Biol. 214, 646–656. doi: 10.1242/jeb.044859
Wilson, R. P., White, C. R., Quintana, F., Halsey, L. G., Liebsch, N., Martin, G. R., et al. (2006). Moving towards acceleration for estimates of activity-specific metabolic rate in free-living animals: the case of the cormorant. J. Anim. Ecol. 75, 1081–1090. doi: 10.1111/j.1365-2656.2006.01127.x
Wood, S. N. (2011). Fast stable restricted maximum likelihood and marginal likelihood estimation of semiparametric generalized linear models. J. R. Stat. Soc. B 73, 3–36. doi: 10.1111/j.1467-9868.2010.00749.x
Keywords: deep diving odontocete, foraging energetics, marine mammal, Grampus griseus, activity level, prey value, central place foraging theory
Citation: Arranz P, Benoit-Bird KJ, Friedlaender AS, Hazen EL, Goldbogen JA, Stimpert AK, DeRuiter SL, Calambokidis J, Southall BL, Fahlman A and Tyack PL (2019) Diving Behavior and Fine-Scale Kinematics of Free-Ranging Risso's Dolphins Foraging in Shallow and Deep-Water Habitats. Front. Ecol. Evol. 7:53. doi: 10.3389/fevo.2019.00053
Received: 25 July 2018; Accepted: 14 February 2019;
Published: 12 March 2019.
Edited by:
Thomas Wassmer, Siena Heights University, United StatesReviewed by:
Stephen J. Trumble, Baylor University, United StatesKagari Aoki, University of Tokyo, Japan
Copyright © 2019 Arranz, Benoit-Bird, Friedlaender, Hazen, Goldbogen, Stimpert, DeRuiter, Calambokidis, Southall, Fahlman and Tyack. This is an open-access article distributed under the terms of the Creative Commons Attribution License (CC BY). The use, distribution or reproduction in other forums is permitted, provided the original author(s) and the copyright owner(s) are credited and that the original publication in this journal is cited, in accordance with accepted academic practice. No use, distribution or reproduction is permitted which does not comply with these terms.
*Correspondence: Patricia Arranz, arranz@ull.es