- 1Shanghai Key Lab of Urban Ecological Processes and Eco-Restoration, School of Ecological and Environmental Sciences, East China Normal University, Shanghai, China
- 2School of Fishery, Zhejiang Ocean University, Zhoushan, China
- 3Shanghai Chenshan Plant Science Research Center, Chinese Academy of Sciences, Chenshan Botanical Garden, Shanghai, China
- 4Shanghai Institute of Pollution Control and Ecological Security, Shanghai, China
Fine-scale spatial genetic structure (SGS) is predominantly determined by gene flow. While sexually reproducing plants can disperse their genes through pollen and seed grains, clonal plants can additionally disperse genes through clonal growth. Plants' clonal reproduction strategy, however, often varies within and between species. Still, the effect of differential clonal reproduction strategy on fine-scale SGS remains somewhat unclear. Halophila ovalis is a fast-growing clonal seagrass, whose internode length (which defines a species' clonal reproduction strategy) varies among populations. Using eight polymorphic microsatellites, here we compare the genetic diversity, clonal structure and fine-scale SGS of two H. ovalis populations with contrasting internode lengths (Yingluo vs. Xialongwei populations). We found moderate to high genotypic and allelic richness and heterozygosities in both populations. Compared to Xialongwei population, genetic and genotypic diversity was significantly lower in Yingluo population. Although their internode length was relatively short, clones of Yingluo population spread farther than those of Xialongwei population. Sexual-to-vegetative dispersal variance ratios were 34.6 and 445.5 in Yingluo and Xialongwei populations, respectively. In both populations, clonal growth significantly intensified the SGS, especially in short distance classes. The SGS at small distance classes were weaker in Yingluo than Xialongwei, in part, due to more intermingled distribution of genets and more extensive clonal expansion in the former population. Our results indicate that vegetative dispersal variance/distance, rather than internode length, plays a crucial role in shaping the fine-scale genetic structure.
Introduction
Fine-scale spatial genetic structure (SGS) characterizes the spatial distribution of genetic composition within a population. Drift, selection and mating system can affect the SGS to some extent, but gene flow is considered as the main determinant of SGS in plant populations (Doligez et al., 1998). Spatially restricted gene flow (e.g., short-distance dispersal) leads to strong fine-scale SGS, while spatially extensive gene flow (e.g., long-distance dispersal) leads to weak or no SGS (Epperson, 2007). And for plants, gene flow may occur predominantly through pollen and seed dispersal (Browne et al., 2018; Kitamura et al., 2018). Of which pollen dispersal generally plays a stronger role than seed dispersal in shaping the distribution of genetic variation among and within populations (Petit et al., 2005; Liu et al., 2015). However, each dispersal event of a diploid seed disperses twice the number of genes as the dispersal of a pollen grain (Chen et al., 2008). Therefore, seed dispersal effects on fine-scale SGS may be more important than pollen dispersal effects (Doligez et al., 1998). And spatially restricted seed dispersal alone, irrespective of the patterns of pollen dispersal, may lead to strong SGS (Wang et al., 2011).
Beyond seed and pollen dispersal, clonal reproduction (or vegetative reproduction) is also an important mechanism through which many plants can disperse their genes. In clonal plants, a vegetatively produced offspring is called a ramet, and the whole plant is called as a genet (Harper, 1979). Clonal reproduction helps produce and disperse genes that are identical to the maternal plant. Genetic similarity among spatially adjacent neighbors is thus expected to be high. Accordingly, fine-scale SGS is expected to be strong in clonal plants (Alberto et al., 2005; Lundemo et al., 2010; Vallejo-Marín et al., 2010; Brzyski et al., 2018).
But plants often adopt different strategy for clonal reproduction (e.g., guerrilla vs. phalanx strategies), and these strategies can influence the pattern of spatial expansion of ramets. Plants with guerrilla strategy possess long internodes or spacers (internode length is defined as the rhizome length between two consecutive shoots), so that these plants can disperse their ramets over relatively large areas. By contrast, plants with phalanx strategy possess very short internodes or no spacers, so that ramets of these plants are often found densely clumped (Lovett-Doust, 1981). In nature, most plants' clonal reproduction strategy lies in between the two extremes (i.e., long vs. short intermodal length) and also vary with site environmental conditions (Vallejo-Marín et al., 2010). Variation in spatial expansion of ramets is likely to alter vegetative dispersal and clonal structure, even within the same species. It is still unclear, however, whether and to what extent clonal reproduction strategy may impact the spatial patterns of gene flow, and thereby impact the fine-scale SGS.
Seagrasses are marine flowering plants. They can reproduce both sexually and asexually (Kendrick et al., 2017). The number and spatial distribution of shoots in a seagrass bed are typically controlled by clonal reproduction via rhizome growth. Internode length is one of the most important determinants of rhizome growth and clonal reproduction in seagrasses (Marbà and Duarte, 1998). But rhizome length varies greatly among seagrass species: for example, the rhizome length of Cymodocea serrulata is 12.7 times of Posidonia oceanica (Marbà and Duarte, 1998). Rhizome length could also vary within the same species: horizontal internodal length in Cymodocea nodosa may vary from 6 to 53 mm and from 3 to 20 mm in Zostera noltii, for example (Marbà and Duarte, 1998). Considering the variation in rhizome length, some seagrasses (e.g., Zostera noltii) may look like phalanx with clumped clones and others (e.g., Cymodocea nodosa) may look like guerrilla with intermingled clones (Ruggiero et al., 2005a). All such differences in spatial configuration of clones may affect the SGS (e.g., Sinclair et al., 2014; Van Tussenbroek et al., 2016). Still, the relationship between clone configuration and SGS in seagrasses has been rarely studied, especially in populations with contrasting clonal traits.
Halophila ovalis (Hydrocharitace) is a small, dioecious, rhizomatous seagrass. This species is commonly distributed in the tropical Indo-West Pacific and some areas beyond the tropics (den Hartog and Kuo, 2006). Dense monospecific meadows of H. ovalis are relatively common along the coastal regions of southern China (Xu et al., 2011). This species can tolerate a wide range of water depths (Xu et al., 2011), salinities (Benjamina et al., 1999), temperatures (Hillman et al., 1995), sediment types (Xu, 2011), and can recover quickly after disturbance. Roots of this species grow from almost all nodes of its horizontal rhizomes; and thus its internode length is very similar to spacer length. Also, H. ovalis shows variation in clonal traits and biomass among different habitats. For example, internode length of this species varies with water depths, most likely due to difference in light availability (Xu et al., 2011).
In this study, we sampled two H. ovalis populations with contrasting internode length. We genotyped each sample using polymorphic microsatellite loci, which is particularly effective in detecting genetic diversity in clonal seagrasses (Arnaud-Haond et al., 2007). We then analyzed spatial autocorrelation of genetic composition to answer the following questions: (1) Do the two H. ovalis populations differ in clonal structure? (2) What is the contribution of clonal reproduction to the fine-scale SGS?
Materials and Methods
Species, Study Site and Sampling
We sampled H. ovalis ramets from two of its monospecific meadows (Xialongwei and Yingluo) in Hepu Dugong National Nature Reserve of Guangxi, China (N21°18′− 21°30′, E109° 34′ − 109° 47′). Total area of these two meadows were ~60 and ~38 ha, respectively. These two meadows were spatially separated from each other by ~18 km. Halophila ovalis populations in these two meadows were significantly different in some clonal traits (Xu et al., 2011): mean internode length of Xialongwei population (47.6 ± 2.0 mm) was approximately twice the length of Yingluo population (24.1 ± 1.1 mm) (Table 1); rhizome diameter (1.41 ± 0.04 vs. 0.84 ± 0.02 mm), leaf length (24.5 ± 0.6 vs. 19.3 ± 0.6 mm), and width (13.2 ± 0.3 vs. 11.0 ± 0.3 mm) were also significantly higher in Xialongwei population than in Yingluo population. Over time, Yingluo population had experienced dramatic changes in meadow area (133 ha in 1994 → 1.3 ha in 1999 → 20 ha in 2000 → 3.3 ha in 2001 → 45 ha in 2003), possibly due to anthropogenic disturbances (Fan et al., 2007).
We conducted our field sampling in the lower intertidal zone of each location (Xialongwei and Yingluo) in June 2009. For that, we used a grid of 20 m × 40 m. The interval grid spacing was 2 m, yielding a total of 231 sampling units (ramets) per population. For each sampling unit, we collected five to ten shoots from same rhizome to ensure sufficient sample for DNA extraction. After being washed with fresh water, all samples were dried and preserved using silica gels for DNA extraction.
In order to avoid the linkage disequilibrium induced by clonal reproduction, we also randomly collected additional sample of 33 and 36 individuals of H. ovalis from Xialongwei and Yingluo populations, respectively. In this case, we used a minimum sampling interval of 20 m. These additional samples were used to test the independence between loci and recent bottlenecks.
DNA Extraction and Microsatellite Genotyping
The Plant Genomic DNA Kit (Tiangen, Beijing, China) was used for total genomic DNA extraction according to the protocol provided by the manufacturer. Eight polymorphic microsatellite loci (HO2, HO3, HO5, HO8, HO20, HO36, HO48, HO51) (Xu et al., 2010) were employed to identify genetically distinct individuals, i.e., to discriminate genets from ramets based on their multilocus genotypes (MLGs). Forward primers of all loci were labeled with either 5'HEX, 5'TAMRX, 5'ROX or 5'6-FAM (Sangon, Shanghai, China), and each locus was PCR-amplified singly using a Mastercycler ep gradient S (Eppendorf, Hamburg, Germany) in a 15 μl reaction volume containing the following components: 50–75 ng of genomic DNA, 0.2 mM of each dNTPs, 0.2 μM of each primer, 1× PCR buffer (Mg2+ free), 2.5 mM Mg 2+ and 1 U of Taq DNA polymerase (Sangon) or 0.75 U of Taq DNA polymerase (Takara, Dalian, China). PCR cycles were as follow: 5 min denaturation at 95°C; 35 cycles containing 30 s at 94°C, 45 s at 55–63.5°C, 45 s at 72°C; and a final extension of 8 min at 72°C for loci HO2, HO3, HO5, HO8, HO20, HO48; or 5 min denaturation at 95°C; 35 cycles of 30 s at 94°C, 60 s at 46°C, 60 s at 72°C; and a final extension of 40 min at 72°C for loci HO36 and HO51. Fragment length was scored on an ABI 3130 automated sequencer using an internal lane standard [GS500 (−250) Liz]. Allele binning and calling was performed using the GENEMAPPER 4.0 software, and then checked manually.
Clone Discrimination and Clonal Analysis
Genotypic linkage disequilibrium was tested based on randomly-collected samples in the two populations using GENEPOP v4.0 software (Raymond and Rousset, 1995). A permutation procedure (1,000 permutations) was used and followed by the sequential Bonferroni calibration (Rice, 1989). No significant linkage disequilibrium was observed between any pair of loci in the two populations using samples randomly collected beyond the grid (Table S1), and thus all loci can be used in the subsequent analyses. Na, HE and FIS values were estimated for every locus within two plots at ramet and genet levels using GenAlEx software (Peakall and Smouse, 2006).
In order to quantify the resolution power of the 8 loci, we estimated the unbiased probability of identity [PID(unbiased)] and the PID among sibs [PID(sib)] (Waits et al., 2001) over all loci using software GIMLET v1.3.3 (Valière, 2002).
When identifying clonality, it is necessary to consider the possibility that multiple different ramets sharing identical multilocus genotypes (MLGs) may result from sexual reproduction (Arnaud-Haond et al., 2007). Therefore, we used the GenClone v2.0 (Arnaud-Haond and Belkhir, 2007) to calculate index Psex to estimate the probability of a given MLG occurring more than once due to different recombination events. MLGsim 2.0 (http://www.rug.nl/fmns-research/theobio/downloads) was used to evaluate the likelihood of Psex values for every MLG observed more than once and tested the significance via a Monte Carlo simulation (106 iterations) approach as proposed by Stenberg et al. (2003). The significant Psex value suggested that those samples were ramets of the same genet by clonal propagation, and non-significant Psex value suggested that those samples sharing genotype were output of sexual reproduction (Vorwerk and Forneck, 2006). Thus, we also calculated the number of corrected MLGs (G*), i.e., number of distinct zygotes by different sexual events. Furthermore, due to mutation or scoring errors, slightly distinct MLGs may be derived from the same genet, and should be recognized as the same multilocus lineage (Arnaud-Haond et al., 2007). If the distribution of the number of distinct alleles among sampling units follows a bimodal distribution and shows high peaks at low distances, mutation or scoring errors should be considered. We used GenClone software to obtain the relationship between frequency distribution and pairwise number of distinct alleles.
Once the clones were discriminated, clonal richness of each population was quantified at ramet level using the number of multilocus genotypes (G) and proportion of distinct clones (Pd). However, clonal richness indices only show the proportion of different clones and do not describe the clonal heterogeneity which indicates the distribution of the samples. So we used the adapted Simpson index (D*), which can be interpreted as the probability that two random samples from the whole would be part of the same clone to measure clonal heterogeneity. This index ranges from 0 to 1, and 0 indicates any two random samples belonging to the same clone. Because heterogeneity indices could not reflect equitability, we used Simpson's complement index (ED*) to estimate clonal evenness. This index varies from 0, when all samples belong to one clone, to 1, when all clones have equal abundance (Arnaud-Haond et al., 2007). The Pareto distribution, a power-law probability distribution, is frequently used to describe the inequalities in allocation across discrete types. We also used the Pareto parameter (β), ranging from 0 to infinity, to describe the scaling of the partitioning of the ramets among MLG size classes (Arnaud-Haond et al., 2007). Larger values of β indicate more ramets belonging to the few largest clones.
To describe the spatial components of clonal growth, we estimated the clonal subrange (CR), i.e., the maximum distance of clonal dispersal (Alberto et al., 2005). To quantify the extent of intermingling between MLGs, we calculated clonal dominance (Dc) for each MLG that had at least three ramets using Ohsako's 2010 method: Dc = (NR-1)/(NT-1), where NR is number of ramets per genet and NT is the total number of individuals included with the minimal convex envelope containing all ramets. A high Dc value indicates that a genet dominates the spatial range enclosed by its ramets. To estimate the bias induced by the sampling scheme, i.e., possible overestimation of clonal diversity due to rare MLGs distributing more at the edges of the plot, we calculated edge effect (Ee) using Arnaud-Haond et al.'s 2007 method: Ee = (Du-Da)/Da, where Du is the average geographic distance between unique MLGs and the center of plot and Da is the average geographic distance between all sampls and the center of plot. We can test the significance of Ee considering the null hypothesis as random distribution of MLGs using 10,000 permutations of the positions of the samples. We also determined aggregation index (Ac) (Arnaud-Haond et al., 2007) to evaluate the spatial aggregation of clone mates. This index varies from 0, which indicates nearest neighbors are not different on average from the global one, to 1, which indicates all nearest neighbors share the same MLG. Significant P-value indicates the existence of spatial aggregation of clonemates. All clonal diversity and structure parameters were calculated using GenClone v2.0 (Arnaud-Haond and Belkhir, 2007), and number of permutations was 10,000.
Population Genetic Statistics
We estimated parameters of genetic diversity at both ramet and genet levels. The observed number of alleles per locus (Na), expected heterozygosity (HE), and inbreeding coefficient (FIS) were calculated. Hardy-Weinberg equilibrium was tested to determine whether a particular estimate of the overall inbreeding coefficient was significantly different from 0 (P < 0.01). All calculations and tests were performed using the GENEPOP v4.0 software (Raymond and Rousset, 1995). Difference in the number of alleles per locus between the two populations was tested using a pairwise Student's t-test in R (R Development Core Team, 2012). Based on the randomly collected samples, we detected sign of recent bottlenecks using Bottleneck 1.2 (Piry et al., 1999). We employed Wilcoxon tests under both two-phase model (TPM) and stepwise mutation model (SMM). The TPM was believed to be the most appropriate model for the mutation of microsatellites (Ellegren, 2004). Parameters for the TPM were set as 79% SMM with a variance of 9% (Piry et al., 1999). One thousand simulations were performed for each population.
Spatial Autocorrelation Analysis and Heterogeneity Test
To quantify the fine-scale SGS within each population and to assess the potential effect of clonality on SGS, we performed spatial autocorrelation analysis at both ramet and genet levels. At the genet level, position of each multi-ramet genet was identified using both central and random methods (Alberto et al., 2005). In the central method, the central coordinates of each clone (average of the x and y coordinates of the clone mates) were set as the position of the genet. In the random method, position of a genet was randomly assigned to one of its observed geographic positions of its ramets. We used re-sampling approach to study autocorrelation at the genet level. Significance tests were conducted by comparing the observed distribution with the simulated distribution. For each plot, geographic locations were randomly permuted 10,000 times to test if the observed mean kinship coefficients were different from those expected simulated distribution for each distance class. The simulated distribution can be obtained by assuming that all genotypes distributed randomly in space. Spatial genetic structure was analyzed using the SPAGEDI v1.3 software (Hardy and Vekemans, 2002). Kinship coefficients (Fij) between each two samples were calculated following the method of Loiselle et al. (1995). Multilocus kinship coefficients per distance interval were computed for the following distance classes: 0–2, 2–4, 4–6, 6–8, 8–10, 10–12, 12–14, 14–16, 16–18, 18–20, 20–25, 25–30, and 30–45 m. The significance of these kinship coefficients was tested using 10,000 permutations.
Pair-wise kinship coefficients were then regressed on the logarithm of spatial distance, and the significance of this regression slope (blog) was tested using a permutation test in SPAGEDI v1.3. The strength of SGS in each population was quantified using the Sp statistic according to Vekemans and Hardy's Vekemans and Hardy (2004) method: , where was the mean between individuals belonging to the first distance interval that should include all pairs of neighbors, and was the slope of regression. The absolute value of Sp statistic indicates the rate of decrease of pairwise kinship with spatial distance. This statistic allows comparisons among different species, and a higher Sp value suggests a stronger spatial autocorrelation. Life history, such as mating system, life form and population density, may significantly affect Sp statistic (Vekemans and Hardy, 2004).
To examine the impacts of clonal growth on the SGS, we performed heterogeneity tests for SGS patterns between ramet and genet levels in each population and between the two populations at both ramet and genet levels using the GenAlEx software (Peakall and Smouse, 2006). We used the approach proposed by Smouse et al. (2008), which compares the real differentiation among the spatial autocorrelation analyses with permutated values from the pooled data set and computes Fisher's combined probabilities to determine the validity of null hypothesis. Autocorrelation coefficient is defined by rij, which shows the genetic correlation between the ith and jth individuals. The coefficient rij is a scale-free measure of genetic similarity between pair of individuals, and is closely related to “kinship” (Loiselle et al., 1995). The criteria ω and t2 were used to quantify the total divergences between entire correlograms and the divergences in each distance class, respectively. In the present study, all distance classes mentioned above were involved in the heterogeneity test, and number of bootstrap resamplings was set to 9999.
Neighborhood Size and Dispersal Variance
We used software SPAGEDI (Hardy and Vekemans, 2002) to estimate neighborhood size (Nb) in each population. Under Wright's isolation-by-distance model, values of kinship coefficients in two-dimensional space are expected to decline linearly with the logarithm of geographic distance within a range from σ to 50σ under a moderate rate of mutation (Hardy and Vekemans, 1999; Heuertz et al., 2003). Under this assumption, slope (blog) of the linear regression function can be adopted to evaluate Nb from the formula: Nb = –(1-F(1))/blog, where F(1) is the mean Fij for all individuals in the first distance class (Fenster et al., 2003; Heuertz et al., 2003). In this study, we considered genotype density as population density.
For clonal plants, variance of dispersal can be rewritten as the following equation (Gliddon et al., 1987): , where , and are variances of pollen, seed and clonal dispersal, respectively. We calculated the variance of clonal dispersal () following Alberto et al.'s 2005 method:, where N is number of genets, NG is the number of genets having two or more ramets, ni is number of ramets of genet i, dij is distance of ramet j to central coordinate of genet i. For genets with only one ramet, dispersal distance was calculated as half of the distance between two consecutive samples, i.e., 1 m in our case. Therefore, we calculated the ratio of sexual-to-vegetative dispersal variance (rd), i.e., , to evaluate the relative contributions of sexual and vegetative dispersal (Alberto et al., 2005).
Results
Genotypic Richness and Clonal Structure
Based on the eight loci, PID(unbiased) and PID(sib) values were 8.70e−8 and 1.78e−3. These values confirmed that the resolution was sufficient to identify genetically unique genets. Equal number (160) of genotypes was found in both populations. All Psex-values were smaller than 0.01. However, Psex values for five (from Xialongwei population) and eight (from Yingluo population) MLGs with multiple samples were non-significant (Table S2). Each of the MLGs that could have arisen as an independent sexual event was found in two individuals. Therefore, the corrected MLGs in Xialongwei and Yingluo populations were 165 and 171, respectively (Table 1). Results of the relationship between frequency distribution and pairwise number of distinct alleles indicated that no somatic mutation or scoring errors occur in our analysis.
About 16.4% (26 genets) and 12.7% (21 genets) of the total genets had two or more ramets in Yingluo and Xialongwei populations, respectively (Figure 1). Proportion of distinct clones (Pd), based on corrected MLGs, was 0.825 and 0.747, and the values of the Pareto index β were 2.822 and 2.440 for Xialongwei and Yingluo populations, respectively. Surprisingly, the adapted Simpson index for genotypic diversity (D*) was almost identical in the two populations (Table 1). No significant effect of edge was observed in any population, suggesting that edge played a negligible role in clonal diversity.
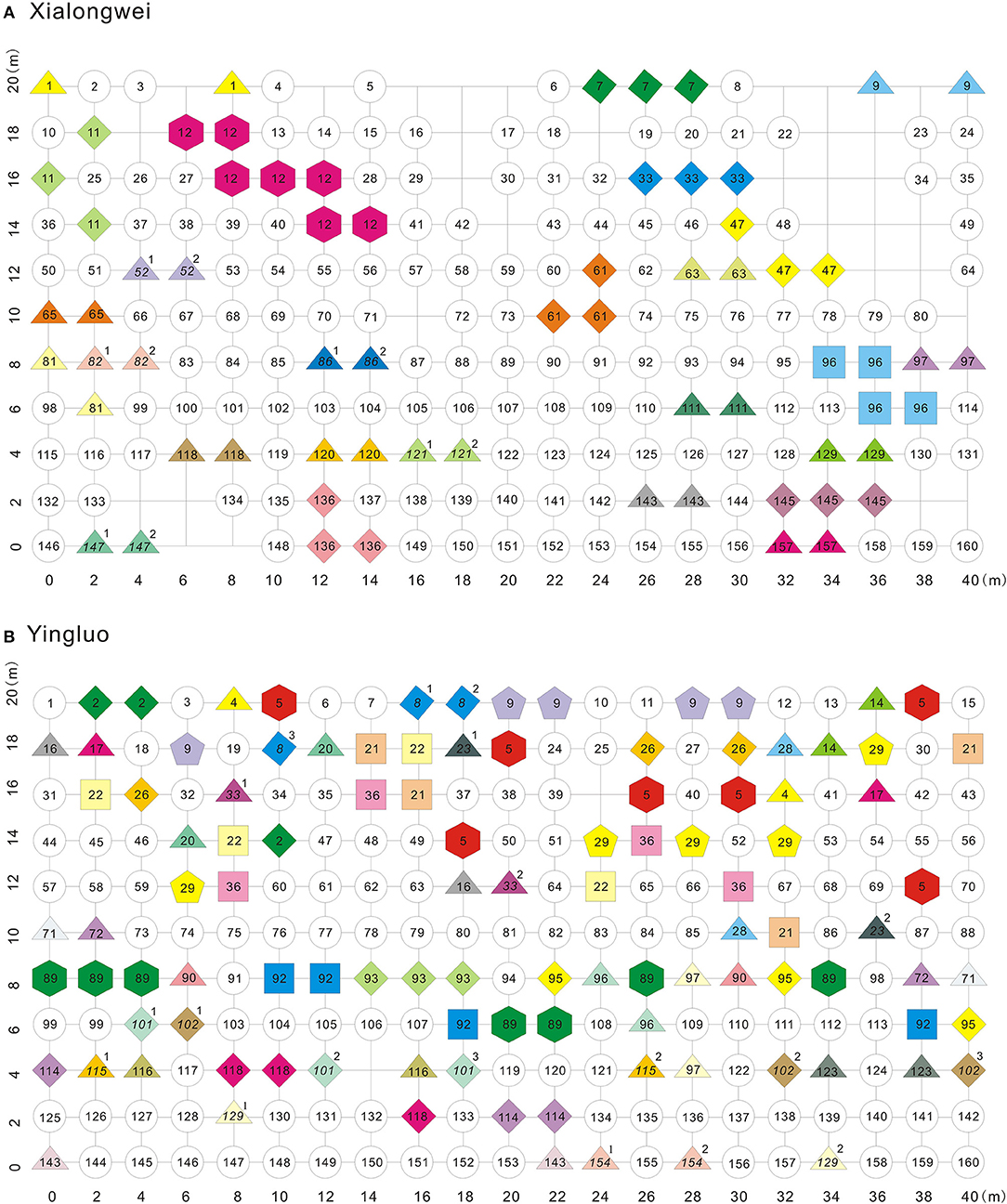
Figure 1. Sampling grids and clone distribution of two Halophila ovalis populations. Circle, triangle, diamond, square, pentagon, and hexagon indicate the clones of 1, 2, 3, 4, 5, and 7 ramets, respectively. The numbers are codes of MLGs. Italic numbers with the superscript of some ramets indicate that they shared the same MLG but were distinct zygotes via distinct sexual events. Same color and same number indicate ramets belonging to the same MLG.
Clone mates were spatially aggregated in both Xialongwei (Ac = 0.162, P < 0.001) and Yingluo populations (Ac = 0.052, P < 0.001) (Table 1). Genets of H. ovalis in Xialongwei population were smaller in size and exhibited more restricted spatial spread than those in Yingluo population. Only nine genets at Xialongwei possessed more than two sample units, and only one genet spread more than 5 m. In contrast, 14 genets at Yingluo had more than two ramets and 26 genets had a spreading distance of >5 m (Table 2). The maximum clone dispersal distance was much shorter at Xiaongwei (CR = 8.9 m) than at Yingluo (CR = 40.1 m) (Table 1). Clonal dominance was significantly lower at Yingluo than at Xialongwei (P < 0.001) with Dc values ranging from 0.11 to 1.0 (Table 2). Clonal dominance was negatively related with clone size (area covered by a genet, in natural logarithm, adjusted R2 = 0.1952, P = 0.006) and clone distance (adjusted R2 = 0.6227, P < 0.001).
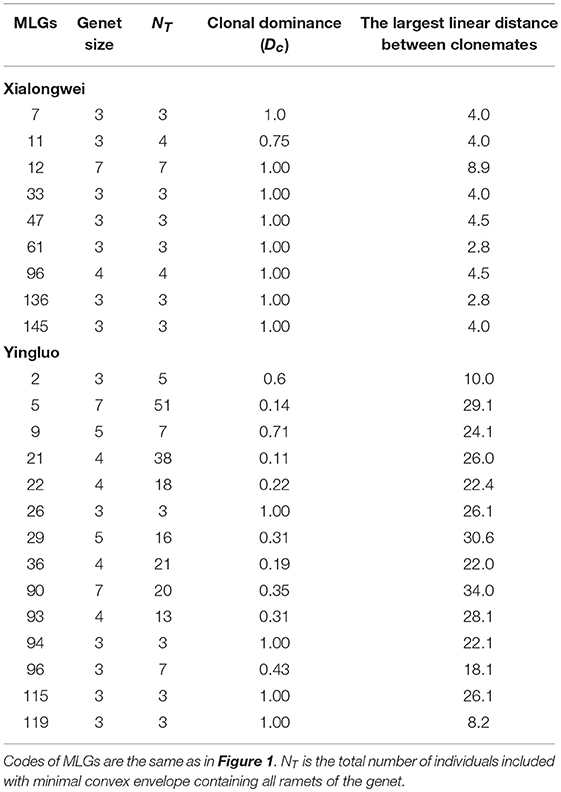
Table 2. Genet size (the number of sampling ramets per genet) and clonal dominance of Halophila ovalis in Xialongwei and Yingluo sites.
Genetic Diversity
Significant departures from Hardy-Weinberg equilibrium were observed in the both populations. Xialongwei population showed an excess of heterozygotes (FIS = −0.062 and −0.052 at ramet and genet levels, respectively, all P < 0.001), while Yingluo population showed a heterozygote deficiency (FIS = 0.230 and 0.248 at ramet and genet levels, respectively, all P < 0.001) (Table 3). No significant associations were observed between heterozygosity and clone size, i.e., area covered by a genet, in the two populations. Both at ramet and genet levels, all measures showed higher genetic diversity in Xialongwei population than Yingluo population (Table 3). The pair-wise Student's t-test indicated that the number of alleles per locus (P = 0.0398), and the expected heterozygosity at ramet (P = 0.0317) and genet (P = 0.0254) levels were significantly higher in Xialongwei population than in Yingluo population.
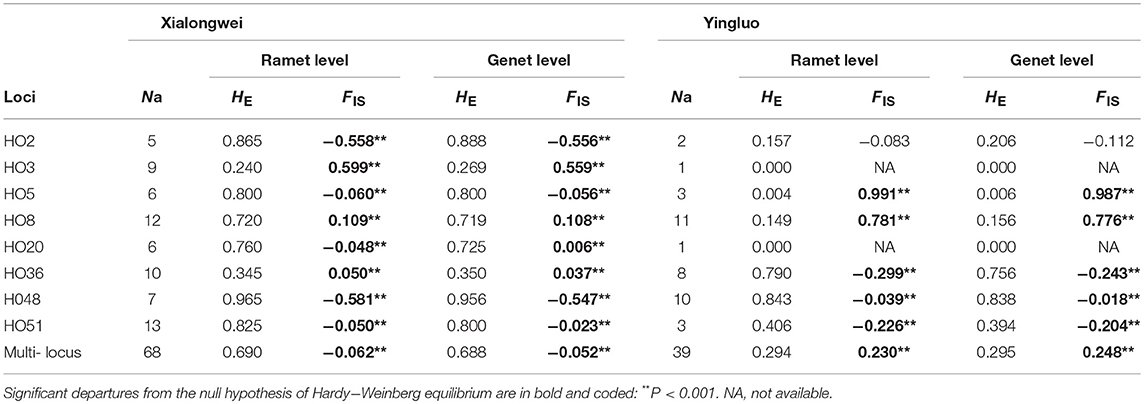
Table 3. Number of alleles (Na), expected heterozygosity (HE), and inbreeding coefficient (FIS) at ramet and genet levels of Halophila ovalis populations in Xialongwei and Yingluo.
Using randomly collected samples, there was a sign of recent bottleneck events in Yingluo population but not in Xialongwei population under TPM and SMM models (Table S3).
Spatial Genetic Structure
For both populations, the average kinship coefficient (Fij) both at ramet and genet (positioned by central method) levels decreased linearly with increasing logarithm of spatial distance (Table 4). In both populations, significant co-ancestry kinship was present within 0–6 m both at ramet and genet levels (all P < 0.001 for each distance class) with an extra extension to 8 m at the ramet level in Yingluo population (Figure 2). Similar pattern was detected through correlogram analysis, using rij. Heterogeneity tests indicated that the overall pattern of SGS differed marginally between ramet vs. genet levels in Yingluo population (ω = 18.836, P = 0.054), but not in Xialongwei population (Table 5). In both populations, the ramet-level SGS was significantly stronger than the genet-level SGS up to first or second distance classes, suggesting that clonal growth strengthens the SGS at short distances (Table 5).
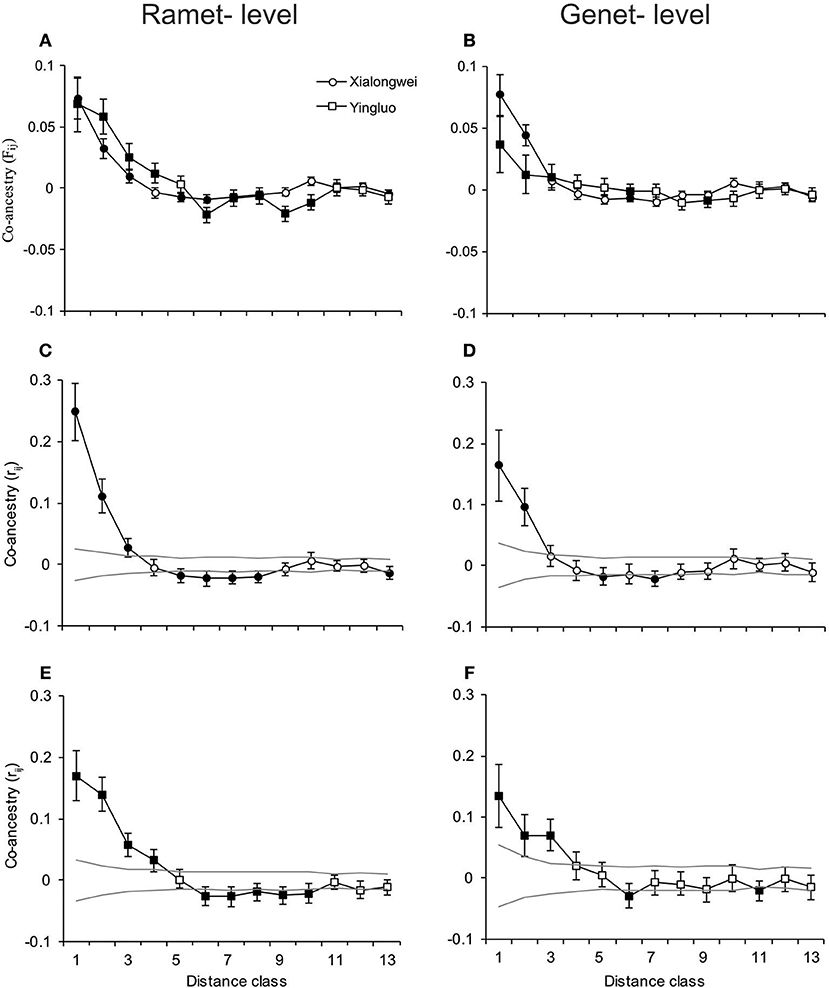
Figure 2. Correlograms of (mean) pairwise co-ancestry parameter as a function of spatial distance for two Halophila ovalis populations. The first column of the correlograms was conducted at ramet level by including all sampling units, and the second column of the correlograms was produced at genet level by using central coordinates as spatial coordinates of genet. The first row correlograms showed co-ancestry (Fij) based on estimations from the software SPAGEDI v.1.3. The second and third row correlograms showed co-ancestry (rij), based on GenAlex, of Xialongwei and Yingluo populations, respectively. The error bar for each index values in (A–F) is the standard error obtained from jackknifing over eight loci, and the dotted lines in (C–F) are 95% confidence interval of no association from 9,999 permutations. The values significant from zero were presented with filled circles and squares for Xialongwei and Yingluo, respectively.
When using random selection method at the genet level, mean kinship coefficient Fij at Xialongwei (0.0639, CI: 0.0616–0.0663) was lower than at Yingluo (0.0889, CI: 0.0793–0.0983). The mean observed blog of regression between Fij and the logarithm of spatial distance was−0.0191±0.0012 (mean±SD) at Xialongwei and −0.0322 ± 0.0015 at Yingluo; and a larger value of Sp was found in Yingluo than that in Xialongwei population (Table 4). Both at ramet and genet levels, there were significant differences in the overall SGS, up to several distance classes, between the two populations (Table 5).
The estimated variances of vegetative dispersal () were 0.8 and 9.3 in Xialongwei and Yingluo populations, respectively. When the effective population density was set as 0.0185 (about 1/11D), the dispersal distances were 18.9 m and 18.2 m in Xialongwei and Yingluo populations, respectively, and corresponding neighborhood sizes (Nb) were 85.9 and 116.3 individuals. The sexual dispersal variances () were 356.4 m2 and 321.9 m2 in Xialongwei and Yingluo populations, respectively; and the corresponding sexual-to-vegetative dispersal variance ratios (rd) were 445.5 and 34.6.
Discussion
We compared the genetic diversity, clonal structure and fine-scale SGS of H. ovalis between two (monospecific) populations. The Yingluo population had relatively shorter internodes and lower genetic and genotypic diversity than Xialongwei population. Clones of Yingluo population, however, spread farther than those of Xialongwei population, with sexual-to-vegetative dispersal variance ratios being 34.6 and 445.5 in Yingluo and Xialongwei populations, respectively. Clonal growth intensified the SGS in both populations, but the SGSs at small distance classes were relatively weak in Yingluo population.
Clonal Structure of Halophila ovalis
We have detected moderate to high levels of genetic variation in H. ovalis populations, using polymorphic microsatellites. Hence, polymorphic microsatellite is an effective tool for identifying plants' clonal structure. We found shorter internode length, but larger clone size, larger neighborhood size and larger variance of vegetative dispersal in Yingluo population, compared to Xialongwei population (Table 1). Halophila ovalis forms roots at each of its nodes. A short internode length would mean clones to have many roots per area. That is, roots in clones of Yingluo population should be denser than the roots of Xialongwei population. A dense root system could help a plant anchor in the sediment and reduce the risk of being broken and washed away by waves or sea currents, thereby increase the resilience of a seagrass bed. Despite having short internode and possibly dense root system, seagrass bed at Yingluo had experienced dramatic fluctuations in area; and when population size was reduced, most of the ramets were eliminated. Perhaps, the shorter internodes were not strong enough to resist the type of disturbances that Yingluo population experienced.
Alternatively, the large decrease in seagrass bed of Yingluo (Fan et al., 2007) was possibly associated with anthropogenic disturbances, beyond the typical disturbance like sea waves and sea currents. On the other hand, strong clonal growth of H. ovalis might have facilitated their quick recovery (i.e., increase in meadow size). Indeed, we have found a sign of recent bottlenecks in Yingluo population. Halophila ovalis has the fastest vegetative extension rate reported in seagrass species (Marbà and Duarte, 1998). Rapid recovery through vegetative extension would permit some genotypes, which is close to the damaged areas at Yingluo, to increase quickly and form large clones. The clonal subrange data of H. ovalis supports this assentation. The clonal subranges of H. ovalis were relatively low to intermediate (8.9 m in Xialongwei −40.1 m in Yingluo), compared to other seagrass species such as in Cymodocea nodosa (20–35 m) (Alberto et al., 2005), Posidonia oceanica (12.7–76.6 m) (Diaz-Almela et al., 2007). The higher clonal subrange in Yingluo population (40.1 m) compared to that of Xialongwei population (8.9 m) could be attributed to the presence of disturbance in the former population. Disturbance-induced increase in clonal subranges has also been observed in the slowly, vegetatively extending Posidonia oceanica (Diaz-Almela et al., 2007) and in the moderately extending Cymodocea nodosa (Alberto et al., 2005).
In theory, plants often allocate more resources to sexual than clonal reproduction in frequently disturbed habitats. Hence, the diversity of clonal genotypes is expected to be higher in disturbed than in relatively stable habitats (Eckert et al., 2016). Contrary to this expectation, proportion of distinct clones (Pd) was lower in Yingluo than in Xialongwei population (Tables 1, 3). This could be the result of rapid expansions of some clones close to the damaged area after the disturbances. However, direct observations on the sexual reproduction are needed.
Horizontal internode length can impact the intermixing of clones. For example, internode length of C. nodosa (25 mm) is approximately twice the length of Zostera noltii (12 mm) (Marbà and Duarte, 1998); and an intermingled configuration of genets is observed in C. nodosa, while Z. noltii is characterized by a clumped, phalanx-type distribution of clones (Ruggiero et al., 2005a). In H. ovalis, a more intermingled clonal pattern was observed in Yingluo population, which had a shorter internode than that of Xialongwei population. Clonal dominance was significantly lower at Yingluo than at Xialongwei. Ohsako (2010) argued that increasing ramet density could in part increase the clonal dominance. But surprisingly, ramet density was higher at Yingluo than at Xialongwei (Table 1). The low clonal dominance at Yingluo is perhaps associated with the presence of many large clones, which increased the probability of clonal mixture.
Genetic Structure of Halophila ovalis
An increasing rate of clonal reproduction increases the heterozygosity via fixed heterozygosity within individuals (Balloux et al., 2003; Navascues et al., 2010; Jolivet and Degen, 2011), though inbreeding and effective size can increase or decrease (Yonezawa et al., 2004). The inbreeding index (FIS) is thus expected to be negative, and the variance of FIS among loci is expected to increase with increasing rate of asexuality (Stoeckel and Masson, 2014). Clonality-driven heterozygote excess has been confirmed in partially asexual plants, such as in a tree species Prunus avium (Stoeckel et al., 2006), in seagrasses Zostera marina (Kamel et al., 2012) and Cymodocea serrulata (Arriesgado et al., 2015), and in fungus Puccinia triticina (Goyeau et al., 2007). Consistent with those observations, moderate to high levels of genetic diversity were found in the studied H. ovalis populations. Negative FIS was found in Xialongwei population, and large variance of FIS among loci was observed in both populations.
However, allelic richness, heterozygosity and FIS differed between the two populations. Xialongwei population had significantly higher allelic richness and expected heterozygosity than Yingluo population (Table 3). Such differences may reflect the impacts of demography on genetic composition. After bottleneck, genetic diversity is expected to be low. Clonal reproduction permits rapid expansion of the remnant genotypes via vegetative reproduction, while propagule recruitment from other populations can stabilize the genetic diversity over time (Harper, 1979; Becheler et al., 2014; Oliva et al., 2014). At an initial stage of population recovery, the rapid clonal expansion of genotypes ought to be determined mainly by chance, rather than by competition among genotypes. But in undisturbed population, strong competition between genotypes tends to exclude disadvantageous genotypes, which are commonly related with homozygotes (Hansson and Westerberg, 2002), and thus heterozygote excess is expected due to selection.
Our study suggested that genetic diversity is higher in relatively stable (Xialongwei) than in fluctuating (Yingluo) populations. This result contradicts with Reusch (2006), who found higher genetic diversity of Z. marina in disturbed than undisturbed plots, due to stimulated sexual recruitment at a fine scale (~1 m2). Jiang et al. (2018) also observed high genetic diversity in Zostera japonica populations, though those populations experienced dramatically fluctuation in size. However, our result is consistent with Diaz-Almela et al. (2007), who found reduced genetic diversity in the disturbed populations of a seagrass Posidonia oceanica. McMahon et al. (2017) found that intermediate disturbance increases clonal richness, but high disturbance decreases clonal richness. It is likely that the dramatic fluctuations of the seagrass meadow area may affect the sexual reproduction, while strong clonal extension may enable the rapid recovery (Hughes and Stachowicz, 2004; Park et al., 2011; Macreadie et al., 2014).
Spatial Genetic Structure
In H. ovalis, sexual-to-vegetative dispersal variance ratios were 445.5 and 34.6, for the two populations. These values are larger than those of C. nodosa (0.065 and 1.16) (Alberto et al., 2005), a seagrass whose horizontal internode length is greater than that of H. ovalis (Marbà and Duarte, 1998). Nevertheless, vegetative dispersal played an essential role in shaping the SGSs of H. ovalis populations. Our results of significant SGS both at ramet and genet levels, especially in short distance classes, indicate that gene dispersal was spatially restricted. The genet-level SGS of H. ovalis was similar to other seagrasses such as Posidonia oceanica (Diaz-Almela et al., 2007), Zostera marina (Becheler et al., 2010), Halophila beccarii (Phan et al., 2017), and Cymodocea nodosa in Italy (Ruggiero et al., 2005b), but weaker than that of Cymodocea nodosa in Spain (Alberto et al., 2005). The contribution of seed dispersal to SGS is likely to be higher in H. ovalis than other seagrasses, given that the rate of horizontal rhizome elongation is very high in H. ovalis (Marbà and Duarte, 1998).
Dispersal can heavily affect the strength of SGS. A strong SGS is expected in plants with limited dispersal ability (Epperson, 2007). Clonal growth spreads out ramets of the same genotype as that of maternal ramet. Genetic similarity among spatially adjacent neighbors is thus expected to be high; and this may lead to a strong SGS to a short distance. Such effect of clonal reproduction on the strength of SGS has been found in high-density populations of P. avium (Jolivet et al., 2011). Slight increase, albeit non-significant, in the SGS at ramet level compared to that of genet-level has also been observed in Cymodocea nodosa (Alberto et al., 2005; Ruggiero et al., 2005b) and in Z. marina var. angustifolia (Becheler et al., 2010). The SGS of H. ovalis was significant only in small distance classes (Figure 2), indicating that clonal growth can strengthen the SGS over relatively short distances. Interestingly, the genet-level Sp statistic differed greatly between central and random coordinate in Yingluo population, but not in Xialongwei population. This discrepancy can be attributed to the presence of many large clones in Yingluo population (Table 2). Estimates of central and random coordinates are usually similar in a small clone, but those estimates differ greatly in a large clone. Estimates of clone position are used in the classification of distance classes. If the frequency of large clone is high, then the results of central coordinate will underestimate the F(1). Random coordinates, on the other hand, reflect the autocorrelation coefficient for the first distance class. The observed short-distance impact of clonal growth in H. ovalis is perhaps related to the vegetative dispersal ability of the species. Being a small species with short internodes, long-distance vegetative dispersal is very rare for H. ovalis. In fact, the farthest dispersal distance of H. ovalis was no more than 40.1 m, and only few genets (only 14 genets in Yingluo population) dispersed over 10 m.
The overall SGSs differed significantly between Yingluo and Xialongwei populations, for both genet and ramet (Table 5). At short distance classes, the SGS (rij) of Yingluo population was relatively weaker than the SGS of Xialongwei population (Table 5, Figures 2C–F), perhaps due to an intermingled pattern of clone intermixing in Yingluo population. Our result of positive association between clone size and the degree of intermixing indicates that larger clones of H. ovalis can intermingled with other clones to a higher degree than smaller clones. Intermixing of clones decreases the genetic relatedness up to a certain distance (2 m in our study), thereby weakens the SGS. Longer vegetative dispersal distance may also contribute to the weaker SGS in Yingluo population. In a simulation study, Epperson (2007) observed that mean kinship value at the shortest distance decreases with increasing distance of seed and/or pollen dispersal. We suggest that extensive vegetative expansion, like seed and pollen dispersal, can also weaken SGS.
Water depth may also affect the fine-scale SGS by changing the resource allocation from vegetative to sexual reproduction. Frequent tidal and anthropogenic disturbances in shallow marine habitats may thus favor sexual reproduction (Orth et al., 2006). Increasing light availability at relatively shallow water may also favor sexual reproduction. Although it remains untested, relatively low water depth at Yingluo may contribute to the weaker SGS. Nevertheless, seedling recruitment in deeper seagrass beds might not be dramatically affected by the reduction of sexual reproduction, given that seeds from adjacent shallower habitats can easily arrive at a deeper habitat.
Conclusions
The two H. ovalis populations with contrasting internode lengths showed moderate to high levels of genetic diversity. Yingluo population with shorter internodes had larger clone sizes, leading to larger neighborhood sizes and larger variance of vegetative dispersal. Historical bottleneck events might contribute to the lower genetic diversity in this population, compared to Xialongwei population. Clonal growth significantly intensified the fine scale SGS in H. ovalis populations. Overall SGS as well as kinship at small distance classes were weaker in Yingluo population than those in Xialongwei population, most likely due to intermingled distribution of genets induced by extensive vegetative expansion in the former population. We concluded that, like seed and pollen dispersal, extensive vegetative expansion can also impact the SGS.
Data Availability
The data used in this study are available at DYRAD. doi: 10.5061/dryad.68s80k7.
Author Contributions
X-YC conceived the study. X-YC and N-NX designed the experiments. N-NX performed the experiments. N-NX, KJ, XT, RW, SB, and X-YC performed the analyses. N-NX, KJ, SB, RW, and X-YC wrote the manuscript. All the authors approved the final version of the manuscript.
Funding
This work was funded by the National Key Research & Development Program (2016YFC0503102) and the National High-tech Research and Development Program (2007AA09Z432) to X-YC, and the China Postdoctoral Science Foundation (2012M511355) to N-NX.
Conflict of Interest Statement
The authors declare that the research was conducted in the absence of any commercial or financial relationships that could be construed as a potential conflict of interest.
Acknowledgments
We thank the crew of the Management Station of Hepu Dugong National Natural Reserves for their professional assistance during the field surveys; Chao Zhang, Ning Yu and Jing Du for help in samples collections; Min Liu and Yuan-Yuan Li for support in the experiments; Miao-Miao Shi for help in statistical analyses.
Supplementary Material
The Supplementary Material for this article can be found online at: https://www.frontiersin.org/articles/10.3389/fevo.2019.00170/full#supplementary-material
References
Alberto, F., Gouveia, L., Arnaud-Haond, S., Pérez-Lloréns, J. L., Duarte, C. M., and Serrão, E. A. (2005). Within-population spatial genetic structure, neighbourhood size and clonal subrange in the seagrass Cymodocea nodosa. Mol. Ecol. 14, 2669–2681. doi: 10.1111/j.1365-294X.2005.02640.x
Arnaud-Haond, S., and Belkhir, K. (2007). GENCLONE: a computer program to analyse genotypic data, test for clonality and describe spatial clonal organization. Mol. Ecol. Notes 7, 15–17. doi: 10.1111/j.1471-8286.2006.01522.x
Arnaud-Haond, S., Duarte, C. M., Alberto, F., and Serrão, E. A. (2007). Standardizing methods to address clonality in population studies. Mol. Ecol. 16, 5115–5139. doi: 10.1111/j.1365-294X.2007.03535.x
Arriesgado, D. M., Kurokochi, H., Nakajima, Y., Matsuki, Y., Uy, W. H., Fortes, M. D., et al. (2015). Genetic diversity and structure of the tropical seagrass Cymodocea serrulata spanning its central diversity hotspot and range edge. Aquat. Ecol. 49, 357–372. doi: 10.1007/s10452-015-9529-0
Balloux, F., Lehmann, L., and de Meeus, T. (2003). The population genetics of clonal and partially clonal diploids. Genetics 164, 1635–1644.
Becheler, R., Benkara, E., Moalic, Y., Hily, C., and Arnaud-Haond, S. (2014). Scaling of processes shaping the clonal dynamics and genetic mosaic of seagrasses through temporal genetic monitoring. Heredity 112, 114–121. doi: 10.1038/hdy.2013.82
Becheler, R., Diekmann, O., Hily, C., Moalic, Y., and Arnaud-Haond, S. (2010). The concept of population in clonal organisms: mosaics of temporally colonized patches are forming highly diverse meadows of Zostera marina in Brittany. Mol. Ecol. 19, 2394–2407. doi: 10.1111/j.1365-294X.2010.04649.x
Benjamina, K. J., Walker, D. I., McComb, A. J., and Kuo, J. (1999). Structural response of marine and estuarine plants of Halophila ovalis (R. Br.) Hook. f. to long-term hyposalinity. Aquat. Bot. 64, 1–17. doi: 10.1016/S0304-3770(98)00103-X
Browne, L., Ottewell, K., Sork, V. L., and Karubian, J. (2018). The relative contribution of seed and pollen dispersal to gene flow and genetic diversity in seedlings of a tropical palm. Mol. Ecol. 27, 3159–3173. doi: 10.1111/mec.14768
Brzyski, J. R., Stieha, C. R., and Nicholas Mcletchie, D. (2018). The impact of asexual and sexual reproduction in spatial genetic structure within and between populations of the dioecious plant Marchantia inflexa (Marchantiaceae). Ann. Bot. 122, 993–1003. doi: 10.1093/aob/mcy106
Chen, X. Y., Fan, X. X., and Hu, X. S. (2008). Roles of seed and pollen dispersal in natural regeneration of Castanopsis fargesii (Fagaceae): implications for forest management. Forest Ecol. Manag. 256, 1143–1150. doi: 10.1016/j.foreco.2008.06.014
den Hartog, C., and Kuo, J. (2006). “Taxonomy and biogeography of seagrasses,” in Seagrass: Biology, Ecology and Conversation, eds A. W. D. Larkum, R. J. Orth, and C. M. Duarte (AA Dordrecht: Springer), 1–23.
Diaz-Almela, E., Arnaud-Haond, S., Vliet, M. S., Alvarez, E., Marba, N., Duarte, C. M., et al. (2007). Feed-backs between genetic structure and perturbation-driven decline in seagrass (Posidonia oceanica) meadows. Conserv. Genet. 8, 377–1391. doi: 10.1007/s10592-007-9288-0
Doligez, A., Baril, C., and Joly, H. I. (1998). Fine-scale spatial genetic structure with nonuniform distribution of individuals. Genetics 148, 905–920.
Eckert, C. G., Dorkern, M. E., and Barrett, S. C. H. (2016). Ecological and evolutionary consequences of sexual and clonal reproduction in aquatic plants. Aquat. Bot. 135, 46–61. doi: 10.1016/j.aquabot.2016.03.006
Ellegren, H. (2004). Microsatellites: simple sequences with complex evolution. Nat. Rev. Genet. 5, 435–445. doi: 10.1038/nrg1348
Epperson, B. K. (2007). Plant dispersal, neighbourhood size and isolation by distance. Mol. Ecol. 16, 3854–3865. doi: 10.1111/j.1365-294X.2007.03434.x
Fan, H. Q., Peng, S., Shi, Y. J., and Zheng, X. W. (2007). The situations of seagrass resources and researches along Guangxi coasts of Beibu Gulf. Guangxi Sci. 14, 289–295. doi: 10.3969/j.issn.1005-9164.2007.03.026
Fenster, C. B., Vekemans, X., and Hardy, O. J. (2003). Quantifying gene flow from spatial genetic structure data in a metapopulation of Chamaecrista fasciculata (Leguminosae). Evolution 57, 995–1007. doi: 10.1111/j.0014-3820.2003.tb00311.x
Gliddon, C., Belhassen, E., and Gouyon, P. H. (1987). Genetic neighbourhoods in plants with diverse systems of mating and different patterns of growth. Heredity 59, 29–32. doi: 10.1038/hdy.1987.93
Goyeau, H., Halkett, F., Zapater, M. F., Carlie, J., and Lannou, C. (2007). Clonality and host selection in the wheat pathogenic fungus Puccinia triticina. Fungal Genet. Biol. 44, 474–483. doi: 10.1016/j.fgb.2007.02.006
Hansson, B., and Westerberg, L. (2002). On the correlation between heterozygosity and fitness in natural populations. Mol. Ecol. 11, 2467–2474. doi: 10.1046/j.1365-294X.2002.01644.x
Hardy, O. J., and Vekemans, X. (1999). Isolation by distance in a continuous population: reconciliation between spatial autocorrelation analysis and population genetics models. Heredity 83, 145–154. doi: 10.1046/j.1365-2540.1999.00558.x
Hardy, O. J., and Vekemans, X. (2002). SPAGEDI: a versatile computer program to analyse spatial genetic structure at the individual or population levels. Mol. Ecol. Notes 2, 618–620. doi: 10.1046/j.1471-8286.2002.00305.x
Heuertz, M., Vekemans, X., Hausman, J. F., Palada, M., and Hardy, O. J. (2003). Estimating seed vs. pollen dispersal from spatial genetic structure in the common ash. Mol. Ecol. 12, 2483–2495. doi: 10.1046/j.1365-294X.2003.01923.x
Hillman, K., McComb, A. J., and Walker, D. I. (1995). The distribution, biomass and primary production of the seagrass Halophila ovalis in the Swan/Canning Estuary, Western Australia. Aquat. Bot. 51, 1–54. doi: 10.1016/0304-3770(95)00466-D
Hughes, A. R., and Stachowicz, J. J. (2004). Genetic diversity enhances the resistance of a seagrass ecosystem to disturbance. Proc. Natl. Acad. Sci. U S A. 101, 998–9002. doi: 10.1073/pnas.0402642101
Jiang, K., Tsang, P.-K. E., Xu, N.-N., and Chen, X.-Y. (2018). High genetic diversity and strong differentiation in dramatically fluctuating populations of Zostera japonica (Zosteraceae): implication for conservation. J. Plant Ecol. 11, 789–797. doi: 10.1093/jpe/rtx053
Jolivet, C., and Degen, B. (2011). Spatial genetic structure in wild cherry (Prunus avium L.): II. Effect of density and clonal propagation on spatial genetic structure based on simulation studies. Tree Genet. Genom. 7, 541–552. doi: 10.1007/s11295-010-0354-2
Jolivet, C., Höltken, A., Liesebach, H., Steiner, W., and Degen, B. (2011). Spatial genetic structure in wild cherry (Prunus avium L.): I. variation among natural populations of different density. Tree Genet. Genom. 7, 271–283. doi: 10.1007/s11295-010-0330-x
Kamel, S. J., Hughes, A. R., Grosberg, R. K., and Stachowicz, J. J. (2012). Fine-scale genetic structure and relatedness in the eelgrass Zostera marina. Mar. Ecol. Prog. Seri. 447, 127–137. doi: 10.3354/meps09447
Kendrick, G. A., Orth, R. J., Statton, J., Hovey, R., Montoya, L. R., Lowe, R. J., et al. (2017). Demographic and genetic connectivity: the role and consequences of reproduction, dispersal and recruitment in seagrasses. Biol. Rev. 92, 921–938. doi: 10.1111/brv.12261
Kitamura, K., Nakanishi, A., Lian, C., and Goto, S. (2018). Distinctions in fine-scale spatial genetic structure between growth stages of Picea jezoensis Carr. Front. Genet. 9:940. doi: 10.3389/fgene.2018.00490
Liu, M., Compton, S. G., Peng, F. E., Zhang, J., and Chen, X. Y. (2015). Movements of genes between populations: are pollinators more effective at transferring their own or plant genetic markers? Proc. R. Soc. B Biol. Sci. 282:20150290. doi: 10.1098/rspb.2015.0290
Loiselle, B. A., Sork, V. L., Nason, J., and Graham, C. (1995). Spatial genetic structure of a tropical understory shrub, Psychotria officinalis (Rubiaceae). Am. J. Bot. 82, 1420–1425. doi: 10.1002/j.1537-2197.1995.tb12679.x
Lovett-Doust, L. (1981). Population dynamics and local specialization in a clonal perennial (Ranunculus repens). I. The dynamics of ramets in contrasting habitats. J. Ecol. 69, 743–755. doi: 10.2307/2259633
Lundemo, S., Stenøien, H. K., and Savolainen, O. (2010). Investigating the effects of topography and clonality on genetic structuring within a large Norwegian population of Arabidopsis lyrata. Ann. Bot. 106, 243–254. doi: 10.1093/aob/mcq102
Macreadie, P. I., York, P. H., and Sherman, C. D. H. (2014). Resilience of Zostera muelleri seagrass to small-scale disturbances: the relative importance of asexual versus sexual recovery. Ecol. Evol. 4, 450–461. doi: 10.1002/ece3.933
Marbà, N., and Duarte, C. M. (1998). Rhizome elongation and seagrass clonal growth. Mar. Ecol. Prog. Ser. 174, 269–280. doi: 10.3354/meps174269
McMahon, K. M., Evans, R. D., van Dijk, K., Hernawan, U., Kendrick, G. A., Lavery, P. S., et al. (2017). Disturbance is an important driver of clonal richness in tropical seagrasses. Front. Plant Sci. 8:2026. doi: 10.3389/fpls.2017.02026
Navascues, M., Stoeckel, S., and Mariette, S. (2010). Genetic diversity and fitness in small populations of partially asexual, self-incompatible plants. Heredity 104, 482–492. doi: 10.1038/hdy.2009.159
Ohsako, T. (2010). Clonal and spatial genetic structure within populations of a coastal plant, Carex kobomugi (Cyperaceae). Am. J. Bot. 97, 458–470. doi: 10.3732/ajb.0900262
Oliva, S., Romero, J., Perez, M., Manent, P., Mascaro, O., Serrao, E. A., et al. (2014). Reproductive strategies and isolation-by-demography in a marine clonal plant along an eutrophication gradient. Mol. Ecol. 23, 5698–5711. doi: 10.1111/mec.12973
Orth, R. J., Harwell, M. C., and Inglis, G. J. (2006). “Ecology of seagrass seeds and seagrass dispersal processes,“ in Seagrass: Biology, Ecology and Conversation, eds A. W. D. Larkum, R. J. Orth, and C. M. Duarte (AA Dordrecht: Springer), 111–133.
Park, S. R., Kim, Y. K., Kim, J. H., Kang, C. K., and Lee, K. S. (2011). Rapid recovery of the intertidal seagrass Zostera japonica following intense Manila clam (Ruditapes philippinarum) harvesting activity in Korea. J. Exp. Mar. Biol. Ecol. 407, 275–283. doi: 10.1016/j.jembe.2011.06.023
Peakall, R., and Smouse, P. E. (2006). GENALEX 6: genetic analysis in Excel. Population genetic software for teaching and research. Mol. Ecol. Notes 6, 288–295. doi: 10.1111/j.1471-8286.2005.01155.x
Petit, R. J., Duminil, J., Fineschi, S., Hampe, A., Salvini, D., and Vendramin, G. (2005). Comparative organization of chloroplast, mitochondrial and nuclear diversity in plant populations. Mol. Ecol. 14, 689–701. doi: 10.1111/j.1365-294X.2004.02410.x
Phan, T. T. H., Raeymaeker, M. D., Luong, Q. D., and Triest, L. (2017). Clonal and genetic diversity of the threatened seagrass Halophila beccarii in a tropical lagoon: resilience through short distance dispersal. Aquat. Bot. 142, 97–104. doi: 10.1016/j.aquabot.2017.07.006
Piry, S., Luikart, G., and Cornuet, J. M. (1999). BOTTLENECK: a computer program for detecting recent reductions in the effective size using allele frequency data. J. Hered. 90, 502–503. doi: 10.1093/jhered/90.4.502
R Development Core Team (2012). R: A Language and Environment for Statistical Computing. R Foundation for Statistical Computing, Vienna.
Raymond, M., and Rousset, F. (1995). GENEPOP (version 1.2): population genetics software for exact tests and ecumenicism. J. Hered. 86, 248–249. doi: 10.1093/oxfordjournals.jhered.a111573
Reusch, T. B. H. (2006). Does disturbance enhance genotypic diversity in clonal organisms? A field test in the marine angiosperm Zostera marina. Mol. Ecol. 15, 277–286. doi: 10.1111/j.1365-294X.2005.02779.x
Rice, W. R. (1989). Analyzing tables of statistical tests. Evolution 43, 223–225. doi: 10.1111/j.1558-5646.1989.tb04220.x
Ruggiero, M. V., Capone, S., Pirozzi, P., Reusch, T. B. H., and Procaccini, G. (2005a). Mating system and clonal architecture: a comparative study in two marine angiosperms. Evol. Ecol. 19, 487–499. doi: 10.1007/s10682-005-0292-x
Ruggiero, M. V., Reusch, T. B. H., and Procaccini, G. (2005b). Local genetic structure in a clonal dioecious angiosperm. Mol. Ecol. 14, 957–967. doi: 10.1111/j.1365-294X.2005.02477.x
Sinclair, E. A., Gecan, I., Krauss, S. L., and Kendrick, G. A. (2014). Against the odds: complete outcrossing in a monoecious clonal seagrass Posidonia australis (Posidoniaceae). Ann. Bot. 113, 1185–1196. doi: 10.1093/aob/mcu048
Smouse, P. E., Peakall, R., and Gonzales, E. (2008). A heterogeneity test for fine-scale genetic structure. Mol. Ecol. 17, 3389–3400. doi: 10.1111/j.1365-294X.2008.03839.x
Stenberg, P., Lundmark, M., and Saura, A. (2003). mlgsim: a program for detecting clones using a simulation approach. Mol. Ecol. Notes 3, 329–331. doi: 10.1046/j.1471-8286.2003.00408.x
Stoeckel, S., Grange, J., Fernández-Manjarres, J. F., Bilger, I., Frascaria-Lacoste, N., and Mariette, S. (2006). Heterozygote excess in a self-incompatible and partially clonal forest tree species - Prunus avium L. Mol. Ecol. 15, 2109–2118. doi: 10.1111/j.1365-294X.2006.02926.x
Stoeckel, S., and Masson, J. P. (2014). The exact distributions of FIS under partial asexuality in small finite populations with mutation. PLoS ONE 9:e85228. doi: 10.1371/journal.pone.0085228
Valière, N. (2002). gimlet: a computer program for analysing genetic individual identification data. Mol. Ecol. Notes 2, 377–379. doi: 10.1046/j.1471-8286.2002.00228.x-i2
Vallejo-Marín, M., Dorken, M. E., and Barrett, S. C. H. (2010). The ecological and evolutionary consequences of clonality for plant mating. Annu. Rev. Ecol. Evol. System. 41, 193–213. doi: 10.1146/annurev.ecolsys.110308.120258
Van Tussenbroek, B., Valdivia-Carrillo, T., Rodríguez-Virgen, I. T., Sanabria-Alcaraz, S. N. M., Jiménez-Durán, K., Van Dijk, K. J., et al. (2016). Coping with potential bi-parental inbreeding: limited pollen and seed dispersal and large genets in the dioecious marine angiosperm Thalassia testudinum. Ecol. Evol. 6, 5542–5556. doi: 10.1002/ece3.2309
Vekemans, X., and Hardy, O. J. (2004). New insights from fine-scale spatial genetic structure analyses in plant populations. Mol. Ecol. 13, 921–935. doi: 10.1046/j.1365-294X.2004.02076.x
Vorwerk, S., and Forneck, A. (2006). Reproductive mode of grape phylloxera (Daktulosphaira vitifoliae, Homoptera: Phylloxeridae) in Europe: molecular evidence for predominantly asexual populations and a lack of gene flow between them. Genome 49, 678–687. doi: 10.1139/g06-028
Waits, L. P., Luikart, G., and Taberlet, P. (2001). Estimating the probability of identity among genotypes in natural populations: cautions and guidelines. Mol. Ecol. 10, 249–256. doi: 10.1046/j.1365-294X.2001.01185.x
Wang, R., Compton, S. G., and Chen, X. Y. (2011). Fragmentation can increase spatial genetic structure without decreasing pollen-mediated gene flow in a wind-pollinated tree. Mol. Ecol. 20, 4421–4432. doi: 10.1111/j.1365-294X.2011.05293.x
Xu, N. N. (2011). Clonality and Its Population Genetic and Ecological Consequences in Halophila ovalis (Hydrocharitaceae). Ph.D. Thesis, East China Normal University.
Xu, N. N., Tong, X., Tsang, P. K. E., Deng, H., and Chen, X. Y. (2011). Effects of water depth on clonal characteristics and biomass allocation of Halophila ovalis (Hydrocharitaceae). J. Plant Ecol. 4, 283–291. doi: 10.1093/jpe/rtq038
Xu, N. N., Yu, S., Zhang, J. G., Tsang, P. K. E., and Chen, X. Y. (2010). Microsatellite primers for Halophila ovalis and cross-amplification in H. minor (Hydrocharitaceae). Am. J. Bot. 97, e56–e57. doi: 10.3732/ajb.1000111
Keywords: clonal growth, Halophila ovalis, spatial genetic structure, internode length, microsatellites
Citation: Xu N-N, Jiang K, Biswas SR, Tong X, Wang R and Chen X-Y (2019) Clone Configuration and Spatial Genetic Structure of Two Halophila ovalis Populations With Contrasting Internode Lengths. Front. Ecol. Evol. 7:170. doi: 10.3389/fevo.2019.00170
Received: 19 February 2019; Accepted: 26 April 2019;
Published: 14 May 2019.
Edited by:
Tian Tang, Sun Yat-sen University, ChinaReviewed by:
Ming Yang, University of Washington, United StatesLudwig Triest, Vrije University Brussel, Belgium
Copyright © 2019 Xu, Jiang, Biswas, Tong, Wang and Chen. This is an open-access article distributed under the terms of the Creative Commons Attribution License (CC BY). The use, distribution or reproduction in other forums is permitted, provided the original author(s) and the copyright owner(s) are credited and that the original publication in this journal is cited, in accordance with accepted academic practice. No use, distribution or reproduction is permitted which does not comply with these terms.
*Correspondence: Xiao-Yong Chen, eHljaGVuQGRlcy5lY251LmVkdS5jbg==
†These authors have contributed equally to this work