- Department of Entomology, Purdue University, West Lafayette, IN, United States
Monarch butterflies are undergoing a long-term population decline, which has led to a search for potential causes underlying this pattern. One poorly studied factor is exposure to non-target pesticides on their primary host-plant, the common milkweed Asclepias syriaca, during larval development. This species frequently grows near agricultural fields in the Midwestern U.S., but the spectrum of pesticides encountered by monarch caterpillars on milkweed leaves is unknown. Further, it is unclear whether pesticide exposure can be avoided by isolating restored milkweed patches at sites far from cropland. Over 2 years, we analyzed 1,543 milkweed leaves across seven sites in northwestern Indiana for the presence and concentration of a range of commonly used agricultural insecticides, fungicides, and herbicides. Additionally, we tested the ability of local (i.e., nearest linear distance to crop field) and landscape-level (i.e., % of corn/soybean in 1 km radius) variables to predict the presence of pesticides on focal milkweeds. Overall, we detected 14 pesticides−4 insecticides, 4 herbicides, 6 fungicides—on milkweeds that varied widely in their prevalence and concentration. The neonicotinoid clothianidin, the only pesticide for which toxicity data are available in monarchs, was detected in 15–25% of plants in June with nearly 60% of milkweeds at some sites testing positive (mean conc. = 0.71 and 0.48 ng/g in 2015 and 2016, respectively); however, no samples from July or August contained clothianidin. The related neonicotinoid thiamethoxam and the pyrethroid deltamethrin were detected in most (>75%) samples throughout the season, but only in the second year of the study. For thiamethoxam, isolating milkweeds 50–100 m from the nearest corn or soybean field tended to decrease the concentration and likelihood of detecting residues, whereas landscape composition surrounding milkweed sites had comparatively weak predictive power. These data suggest that monarch caterpillars frequently consume a diversity of pesticides in their diet; the lethal or sublethal impacts of this exposure remain to be tested.
Introduction
Since 1960, agricultural intensification and a corresponding rise in pesticide use has been an environmental concern due to contamination of soil-water-air and movement of chemicals through the trophic chain (Carson, 1962; Krupke et al., 2007; Epstein, 2014; Douglas et al., 2015). Because broad-spectrum pesticides are, by nature, not specific to focal pests, they can affect non-target beneficial organisms (i.e., pollinators, parasitoids, predators) inhabiting crops, as well as unmanaged habitats neighboring agricultural land (Longley and Sotherton, 1997; Aktar et al., 2009). Routes of exposure are varied and challenging to track, but include direct contact with contaminated surfaces or spray droplets, residues remaining on the soil, and consumption via food resources such as leaves, nectar or pollen (Cilgi and Jepson, 1995; Longley and Stark, 1996). In many cases, only a small fraction of active ingredient makes contact with target pests, while the remainder is absorbed by the greater ecosystem. Pesticides applied by aircraft, for example, can reach as little as 50% of the target crop with the remainder moving to surrounding areas as far as 30 km downwind (Pimentel and Levitan, 1986; Pimentel, 1995). As a result, a range of insect pests, from aphids to caterpillars, are estimated to contact < 0.1% of insecticides applied for their control (Pimentel and Levitan, 1986). Even newer, more targeted technologies are vulnerable to this pesticide ‘loss'; namely, seed treatments that were once touted for their limited off-site drift (Jeschke and Nauen, 2008). New data estimate that only 1.3% of initial seed treatment is recovered from corn plants exposed to the neonicotinoid clothianidin, with the remaining 98–99% of material leached into the environment (Alford and Krupke, 2017).
Off-site exposure to mobile insecticides is particularly a concern for pollinators, many of which inhabit agricultural landscapes and are undergoing long-term population declines. Several studies provide evidence of lower abundance and/or diversity of butterflies in the field margins of insecticide-treated crops compared with unsprayed controls (Rands and Sotherton, 1986; Dover et al., 1990; De Snoo et al., 1998). In most cases, it is unknown whether effects are caused by exposure to adults nectaring on flowering plants or larvae developing on contaminated leaves. However, a field experiment exposing Pieris brassicae caterpillars at different distances downwind to spray drift from the insecticide diflubenzuron, showed higher mortality when developing on leaves of their host-plant up to 16 m from the field edge (Davis et al., 1991). Similarly, several studies illustrate that the nectar and/or pollen of wild flowering plants on crop field edges contain residues of neonicotinoid insecticides among other agrochemicals (Krupke et al., 2012; Botías et al., 2015, 2016; David et al., 2016; Mogren and Lundgren, 2016). Indeed, much of the recent focus of non-target impacts on pollinators centers on the neonicotinoids, due in large part to their widespread adoption in global agriculture (Douglas and Tooker, 2015). Although this work has primarily targeted bees, increasing evidence suggests that butterflies are also affected. Two recent time-scale analyses of reductions in butterfly diversity over the past several decades link these changes with the introduction and rise of neonicotinoids in the UK (Gilburn et al., 2015) and California (Forister et al., 2016). These correlative analyses were complimented by a few experimental lab studies showing strong negative effects on larval development for butterflies reared at field-realistic exposure levels for clothianidin (Pecenka and Lundgren, 2015) and imidacloprid (Whitehorn et al., 2018). Yet, due to the strong research emphasis on bees and pollen/nectar composition, we still lack field data on dietary exposure to pesticides for butterfly larvae developing on leaves of host-plants bordering cropland.
The common milkweed Asclepias syriaca L. is an abundant and opportunistic herbaceous plant growing in disturbed agricultural areas throughout the eastern United States (Woodson, 1954). It is notorious as being the primary larval food plant for the migratory monarch butterfly (Danaus plexippus L.) throughout its summer breeding range (Seiber et al., 1986; Wassenaar and Hobson, 1998). While Asclepias is a relatively diverse genus in North America and monarchs are capable of feeding on most, if not all, of these species, A. syriaca is by far the most widely available and used by monarchs in the Midwestern U.S. (Hartzler and Buhler, 2000; Zaya et al., 2017). Because A. syriaca grows in close proximity to corn and soybean fields and monarchs specialize on milkweed, this system offers a unique opportunity to examine the links between crop management, pesticide leaf concentrations, and butterfly development. Importantly, monarch populations have declined sharply over the last 20 years with censuses in overwintering sites reporting an 82% decrease in population size (Inamine et al., 2016; Semmens et al., 2016; Malcolm, 2018). Hypothesized contributors to this decline include: loss of overwintering forests in Mexico (Brower et al., 2012); reductions in milkweed host-plants due to widespread use of the herbicide glyphosate (Hartzler, 2010; Pleasants and Oberhauser, 2013; Stenoien et al., 2016; Thogmartin et al., 2017a); urban development (Brower et al., 2012); severe weather events (Swengel, 1995; Brower et al., 2012); climate change (Oberhauser and Peterson, 2003; Flockhart et al., 2015; Saunders et al., 2017); and parasites (Altizer and Oberhauser, 1999; Altizer et al., 2004, 2015).
Although pesticides have been considered as a factor underlying the monarch decline (see Oberhauser et al., 2006; Krischik et al., 2015; Pecenka and Lundgren, 2015; Thogmartin et al., 2017a), it is difficult to evaluate this hypothesis because we lack data on field exposure during larval development. Interestingly, monarch declines have temporally coincided with the increase in use of neonicotinoids throughout agricultural regions in their summer breeding habitat, leading some to speculate whether this is a correlative or causal relationship (Stone, 2013). A recent petition by the U.S. Fish & Wildlife Service to protect monarchs under the endangered species act highlights this point: “It is notable that the monarch decline has occurred during the same time period that the use of neonicotinoid insecticides in the key monarch breeding areas has dramatically increased, although, to our knowledge no one has tested the hypothesis that neonicotinoid use is a significant driver of monarch population dynamics.”
A lab toxicity assay of monarch larvae exposed to different concentrations of clothianidin—the main neonicotinoid seed treatment applied to corn—showed lethal effects with an LC50 at 15.6 ng/g and sub-lethal effects at as little as 1 ng/g (Pecenka and Lundgren, 2015). Despite the lack of data on realistic field exposure, some have taken proactive measures to protect monarchs against potential harm. In 2014, for instance, the U.S. Fish & Wildlife Service phased out neonicotinoid insecticides on crops grown on National Wildlife Refuge System lands. Further, the U.S. Department of Agriculture developed a wildlife habitat evaluation guide and decision support tool for monarch butterfly restoration in which a 125-foot-wide pesticide-free buffer around restored milkweed habitat is advocated (USDA-NRCS 2016). To our knowledge, these buffers have not been “ground truthed” by quantifying actual pesticide residues on milkweed plants varying in their distance from the edge of agricultural fields. Such data are critical for defining the validity of nearest-distance thresholds used by land managers creating monarch habitat. Given that recent monarch population models estimate that 1.6 billion milkweed stems need to be added to the Midwestern region to achieve future conservation goals, close proximity to agricultural land is unavoidable (Pleasants, 2017; Thogmartin et al., 2017b).
With this in mind, our primary aim in this study was to define and quantify the spectrum of pesticides exposed to potential consumption by monarch caterpillars on their host-plant, A. syriaca, in agricultural landscapes. Secondarily, we assessed how pesticide presence varies with linear distance between focal milkweeds and cropland. This was done to test the degree to which pesticide residues diminish with increasing spatial isolation at a local-scale, which is most relevant to land managers who often have some amount of flexibility over local habitat placement on their property. Pesticide-free buffers assume a proximity threshold beyond which exposure is minimal to non-existent. Last, we compared the effectiveness of nearest-distance buffer models with broader landscape-scale analyses of land use to determine which better predicts monarch exposure.
Methods
Study Areas
In 2015 and 2016, we sampled A. syriaca at seven sites across two counties—Tippecanoe and Newton—in northwestern Indiana, USA. Each site was separated from the nearest site by at least 2 km with the farthest two sites ca. 100 km apart. A site consisted of a patch of at least 30 milkweed plants growing in an area adjacent to a corn or soybean field. Although all milkweed patches were embedded within agricultural landscapes dominated by corn and soybean production (see Table 1 for land use data and SI Appendix, Figures S1 and S2 for reference GIS land use maps to visualize surrounding habitats for a representative agricultural and natural site, respectively), the local habitat varied widely from unmanaged crop field edges to large prairies used in restoration or conservation. As a result, the degree of isolation separating milkweeds from the nearest crop field varied widely, from 0 to > 2 km; however, most were within a 100 m buffer zone of the field edge. Because we were constrained by the location of existing milkweeds and site configuration, we had little control over min/max distances, as well as other factors that could affect pesticide movement, e.g., soil type, direction of milkweed patch relative to crop field (upwind vs. downwind). Data on number of plants sampled per site/year, distance range separating milkweeds from crop, size of neighboring crop field, and direction of milkweeds compared to crop are provided in Table 2. Sites included:
(i) Purdue Agronomy Center for Research & Education (ACRE), a 1,408 acre farm managed for row crop research, mainly corn and soybean. Within ACRE, we identified and sampled milkweed plants in the Peterson Prairie Plot, a 4 acre tall grass prairie restoration planting established in 2003.
(ii) Kankakee Sands, a 20,000 acre protected savannah-prairie owned and managed by The Nature Conservancy. Because of its large area, we identified two sites within this location; one directly abutting a soybean field named “Kankakee close” and another that was in the core area, at least 1,500 m from the nearest agricultural land designated as “Kankakee far.”
(iii) Meigs-Purdue Agricultural Center, a 145 acre research farm used primarily for fruit and vegetable production, but also including row crop agriculture. Because we could only identify 28 naturally growing milkweeds at this site, we supplemented by transplanting an additional 38 plants. Seedlings from two milkweed species (A. syriaca and A. incarnata) were transplanted in the field in April 2015 in six rows, each of which contained five plants along a distance transect from the corn field edge: 0, 5, 10, 20, and 30 m. Transects were separated by 10 m. An additional eight plants at 0 m were placed along the northern and western borders of the field. It is unclear whether the lack of milkweed at this site, as well as site (vi) below, was due to the high local use of glyphosate or because these field margins were occasionally mowed, which likely reduced milkweed stand establishment.
(iv) Prophetstown State Park, a 900 acre restored prairie. Milkweeds in this area were within a grassland close to a large corn field.
(v) Purdue Wildlife Area (PWA), a 159 acre property that includes forest, wetlands, and early successional habitat. Milkweeds were adjacent to a corn field on the western border.
(vi) Throckmorton-Purdue Agricultural Center (TPAC), an 830 acre research farm managed for row crop research, mainly corn and soybean. Similar to the Meigs site described above, we used milkweed transplants along distance transects running perpendicular to the corn field edge. In 2015, 36 plants were placed around the corn field; four transects at 0, 5, 10, 20, and 30 m along the western field edge, four transects at 0, 5, 10 m along the eastern edge, and four individual plants at 0 m along the north and south field edges.
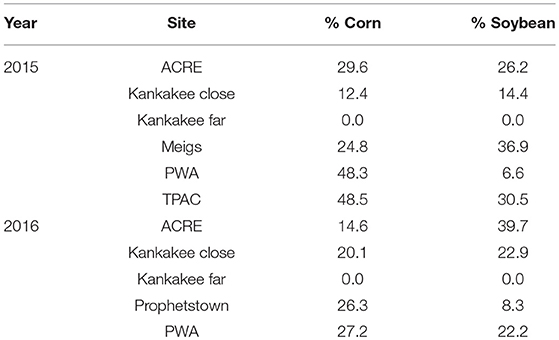
Table 1. Area of corn and soybean, expressed as percent of total land use, planted in a 1 km radius around milkweed sites sampled in 2015 and 2016.
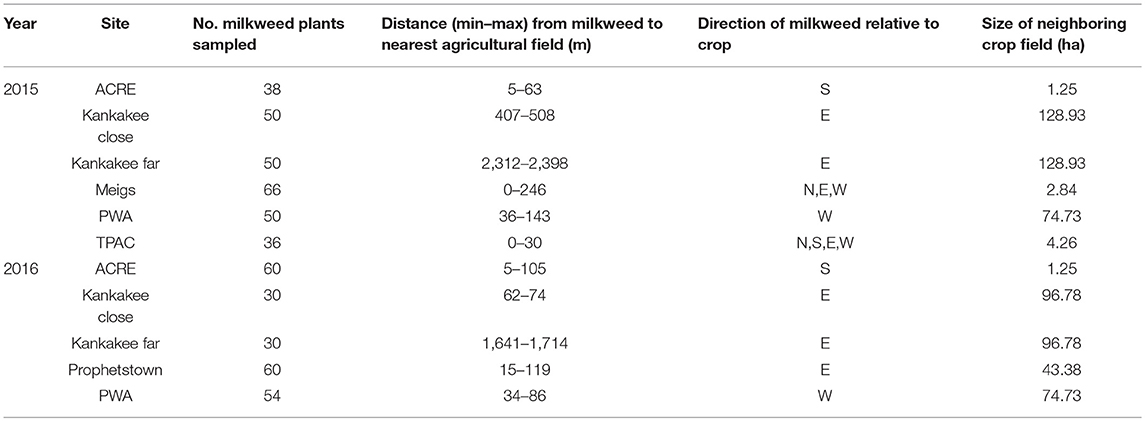
Table 2. Local site characteristics and number of plant replicates for milkweeds sampled in 2015 and 2016.
Field Sampling
Milkweed Leaf Samples
During June, July, and August in both years, leaf tissue was collected from milkweed plants for chemical analysis. On average, we sampled 48 plants per site each year, with 524 total milkweed plants sampled over the 2-year period across all sites. Within a given site, sampled plants were semi-randomly chosen to span a distance gradient along a transect extending out from the crop field edge. Each month, two leaves were removed to provide at least one-gram of tissue for analysis. The two leaves were located in the central portion of the plant, avoiding the new growth in the apical meristem and older senescent leaves at the bottom of the stem. This leaf position roughly coincides with where we often observe monarch larvae feeding in the field. Leaves were sealed in plastic bags and kept in a cooler with ice before they were transferred to a −80°C freezer in the laboratory. Because we collected whole-leaf samples, we do not know whether residues were on plant surfaces or inside of plant tissues. Similarly, due to the large number of pesticides measured and logistical challenges with sampling from multiple field sites over time, we did not attempt to control for variation in other factors that undoubtedly impact pesticide detection, e.g., rainfall, time since application, half-life. However, our sampling design over two years with several samples at different time points within a given year, using multiple sites, and a relatively large number of plant replicates per site, was in part intended to account for this inevitable background “noise” and provide a reasonable estimate for average exposure at a given time and place.
Plants were labeled with colored flagging tape to sample the same individuals in subsequent months and georeferenced to calculate the linear distance between focal milkweeds and the nearest corn/soybean field in the study area. To calculate the distance of each individual plant to the crop fields we used an ArcGIS model for each individual site. The tools used in the model include: “Project” that converts data from one coordinate system (WGS_1984) to another (NAD_1983_UTM_Zone_16N); “Near” which calculates the distance between the input feature (milkweeds) and the near feature (crop field); “Add field” that adds a new field to a table, in our case the distance from milkweeds to the crop; and “Calculate field” which calculates the values within the new field in the table (SI Appendix, Figure S3).
Soil Samples
We collected five soil samples per site during June, July and August 2016, resulting in 15 total samples per site. Soil was collected from random locations in the same approximate area where milkweeds were growing at different distances from the crop (SI Appendix, Figure S4). To do so, we used a soil core (2 cm diameter), sampling the top ca. 18 cm, although the sampling depth varied with soil compaction across sites. Because soil type plays an important role in the retention or degradation of pesticides, we identified the types of soils at each site using the USDA Soil Survey Geographic Database (SSURGO) map data, which contains information for 3,200 soil surveys (SI Appendix, Table S1).
Land Use Analysis
Although we measured the linear distance of each plant to a specific crop field within our study areas, distance alone may be a poor predictor of variation in pesticide residues. Thus, we quantified the area of corn and soybean in a 1 km radius buffer around the milkweed sampling sites since most of the pesticide inputs are compounds applied to these two crops, which dominate land use in our region. To do so, we used the ArcGIS buffer geoprocessing tool with a 1 km radius, extracted by mask to obtain the crops just within the buffer and tabulated area to calculate the percent of corn, soybean and other crops as a fraction of total land use. Land use data were obtained from USDA NASS Cropland Data Layer for Indiana (www.indianamap.org).
We also estimated corn and soybean pesticide use at a broader geographical scale (county-level) to assess the relationship between pesticide inputs in those crops and the residues associated with our plants. We used the USGS pesticide database, which estimates pesticide applications per crop per state; we used Epest-low values, which are more conservative and tend to better match other estimates. To quantify the amount of pesticides applied in the two counties (Tippecanoe and Newton) where milkweeds were sampled, we first divided the total amount of each corn or soybean pesticide applied at the state-level (i.e., for Indiana only) by state-wide acreage to provide a per area use rate in each year. This approach assumes that state-wide averages are reflected in local grower practices, which may not always be the case. The per-unit rate was then multiplied by the area of corn or soybean planted per county in that year to estimate how much of each pesticide was applied near milkweed sites (SI Appendix, Table S2). Because USGS datasets stopped including seed treatments in their pesticide surveys after 2014, we unfortunately could not include neonicotinoids and some fungicides using this approach. However, virtually all corn (> 90%) in our area is seed treated with clothianidin and thus total corn acreage is a good proxy for neonicotinoid input (Douglas and Tooker, 2015). In addition to the information provided by USGS, a list of the pesticides applied during our sampling to corn or soybean close to the milkweeds was provided by the staff managers at the different sites (SI Appendix, Table S3).
Laboratory Analysis
Leaf Pesticide Residue Analysis
QuEChERS (Quick-Easy-Cheap-Effective-Rugged-Safe) extraction method was used to identify pesticide residues associated with milkweed samples. We screened 65 commonly used pesticides following the approach by Long and Krupke (2016). Multiple leaves within a sampled plant/date were combined and chopped with scissors to obtain a roughly homogenized 1 g sample. All plant tissues were processed with scissors and forceps, cleaned in a 70% alcohol solution before processing each sample and latex gloves were used to avoid contamination between samples. Each 1 g sample was transferred into a 7 ml homogenizer tube (Bertin-technologies) with 2 g of zirconium oxide beads (2 mm diameter; Bertin-technologies). To homogenize the tissue, 2 ml of double deionized (dd) water was added to each tube, after which tubes were set in a Precellys 24 lysis homogenizer, which processed samples using four cycles at 5,000 rpm. Homogenized samples were transferred to 15 ml tubes, and 2 ml dd water and 4 ml of the extraction solvent acetonitrile were added. The 15 ml tubes contained the 1 g plant tissue, 4 ml dd water and 4 ml acetonitrile. Ten μl of an isotopically labeled internal standard mix containing the pesticides screened was added to the 15 ml tubes. The standards help in the quantification of the pesticides in the samples, because a calibration curve is then created to assign a concentration value to peaks obtained from the processed samples.
The anhydrous salts magnesium sulfate (1.2 g) and sodium acetate (0.3 g) were added to enhance the extraction efficiency and induce phase separation with acetonitrile. Each 15 ml tube was agitated for 1 min with a S8220 Deluxe Mixer Vortex (Scientific Products) and shaken on a VWR W-150 Waver Orbital Shaker at speed 10 for 10 min. The tubes were centrifuged at 4°C, 2,500 rpm for 10 min, for phase separation. One ml of supernatant was added to 2 ml Agilent dispersive Solid Phase Extraction tubes (part no: 5982-5321), containing 25 mg PSA, 7.5 mg GCB and 150 mg MgSO4, cleaning up the samples before the analysis by liquid chromatography. The dispersive SPE tubes with the 1 ml supernatant were spun in a vortex (Labnet VX100) for 10 min and centrifuged at 15,000 rpm for 5 min in an Eppendorf Centrifuge 5424. The supernatant was then transferred into 2 ml Eppendorf tubes, which evaporated overnight in a speed vacuum (SC250EXP, ThermoFisher Scientific). The dry residue at the bottom of the tubes was mixed with 100 μl of acetonitrile, spun for 10 min in a vortex, centrifuged for 5 min, and the supernatant was transferred to liquid chromatography mass spectrometry (LC-MS) autosampler vials. The identification, quantification and separation of the pesticide residues were carried out in an Agilent 1200 rapid resolution liquid chromatography with a triple quadrupole mass spectrometry (Agilent 6460 series) and an Agilent Zorbax SB-Phenyl 4.6 × 150 mm, 5 μm column (Agilent technologies, Santa Clara, CA). Both the QuEChERS method modification and LC-MS analysis were performed at the Bindley Bioscience Center at Purdue University.
Soil Pesticide Residue Analysis
QuEChERS extraction method was modified and used to identify pesticide residues in soil, similar to the above-described protocol. Seven grams of wet soil were weighed on a scale (Mettler Toledo model MS3001S). The samples were dried for 2 days at 105°C in individual aluminum baking cups. The dry weight of each individual sample was recorded to calculate the pesticide concentration in ng/g per sample; dry weight varied between 5.04 and 6.96 g. Dry soil was sieved and slowly added and mixed to avoid clumping with 5 ml dd water in a 50 ml falcon tube. The 50 ml tubes were agitated for 1 min, then 5 ml of acetonitrile (ACN) at 99% and acetic acid at 1% were added, followed by 10 μl of an isotopically labeled internal standards mix containing the 65 pesticides targeted for screening. The tubes were agitated in a vortex for 7 min and then 4 g of magnesium sulfate (MgSO4) and 1 g of sodium acetate (NaOAC) were added slowly, shaking regularly in a vortex to facilitate the incorporation of the salts with the soil and avoid clumps. Upon adding salts, tubes were agitated in a vortex for another 2 min to dissolve any clumps. The samples were centrifuged for 5 min at 4,000 rpm and 1.4 ml of supernatant was transferred into dispersive Solid Phase Extraction tubes (part no: 5982-5122), containing 50 mg PSA, 50 mg C18EC and 150 mg MgSO4, to clean up the samples before the analysis by liquid chromatography. The dispersive SPE tubes were spun for 5 min and centrifuged at 5,000 rpm for 3 min; 1 ml of supernatant was then transferred to 2 ml Eppendorf tubes and left to dry overnight in a speed vacuum (SC250EXP, ThermoFisher Scientific). The next day, samples were resuspended in 100 μl of acetonitrile, spun for 5 min and centrifuged for 7 min at 13,000 rpm before transferring the supernatant into an LC-MS vial. Pesticide identification and quantification were carried out as described above for leaf samples.
Statistical Analysis
We only targeted pesticides for statistical analysis and figures if they were detected in > 1% of milkweed samples with overall concentrations > 1 ng/g. Pesticides that fell below these thresholds were considered either too sporadic or diffuse to cause significant ecological impacts on monarch populations.
The effects of year, month and site on pesticide presence in milkweed tissue were evaluated with a mixed model logistic regression, with binary data (SI Appendix, Table S4). When pesticide residues were found in association with milkweed tissue we assigned a value of 1 and when pesticide residues were below the detection limit we gave a value of 0. Site was considered as a random factor, and year and month were fixed factors. For this analysis, we only used 0/1 data, rather than the actual concentrations due to the large number of samples below the detection limit.
We used a correlation analysis to test the relationship between pesticide concentrations found in soil vs. corresponding values in milkweed leaves. To do so, we created a 5 m buffer around the points where soil samples were collected and selected the plants inside the buffer (SI Appendix, Figure S4). These soil-plant samples were paired together as spatially co-occurring to test for a correlative pattern. In cases where multiple plants were within the soil buffer we averaged the plant data to create a single mean value for each pesticide at that location.
To evaluate the effects of land use on pesticide residues associated with milkweed leaves we used a three-tiered approach, starting with local habitat placement and ending with landscape-scale crop pesticide use. For local habitat placement, we used a two-part hurdle model with logistic regression using binary data based on detection frequency, followed by a secondary analysis using the continuous concentration data with non-detections removed. For this analysis, we focused on the three insecticides—thiamethoxam, clothianidin, deltamethrin—since the impacts of fungicides/herbicides on monarchs at this point are unknown. Because distance to field is confounded with site, we were unable to include both factors in the model. In working with naturally occurring milkweed patches we were constrained by existing plant distributional patterns (see Table 2), resulting in some sites with all milkweeds clustered relatively close to the field margin (0–30 m for 2015 TPAC) and other sites that were far further away (2,300–2,400 m for 2015 Kankakee far). Thus, we developed site-specific models that include the factors year (when appropriate; some pesticides were mostly detected one of the 2 years), month, and distance separating milkweed plants from the nearest agricultural field. This allows us to test the effects of spatial isolation, while controlling for temporal variation. We only analyzed sites in which the distance gradient spanned the 125 ft distance threshold proposed for milkweed restoration. Several of our sites (see Table 2) included milkweeds that far exceeded this distance threshold, even at the closest proximity, and, consequently, distance from nearest crop field is biologically less relevant in these cases.
Next, simple linear regressions per year and pesticide active ingredient were used to quantify the relationship between percent of corn and soybean planted in a 1 km radius around milkweed habitats and the frequency of milkweed leaves with pesticide residues. For this analysis, we took advantage of natural variation in land use surrounding our sites, which varied widely from no agriculture to ca. 80% cropland (see Table 1). Last, we used correlations to determine whether corn or soybean pesticides applied at the county-level reflected the frequency of residues associated with milkweed leaves. This analysis used Tippecanoe as the focal county since this housed the majority of our milkweed sites and has a similar agricultural backdrop to the other county (Newton) surveyed. Also, we focused this county analysis only on fungicides for two reasons: one, given the chemical and application differences across pesticide classes, we wanted to avoid directly comparing, for example, insecticides and herbicides; and two, fungicides had the most active ingredients-−6 compounds—which allowed us to make this comparison (i.e., we were unable to use a correlation with only 2 or 3 data points in the case of insecticides and herbicides).
All statistical analyses were conducted with R software 3.5.1 using the packages car, ggplot2, lmer4, and multicomp, except for local habitat use (i.e., distance from crop), for which we employed the Proc Genmod and Proc GLM functions in SAS, V. 9.4.
Results
Leaf Pesticides
Across both years of the study, 14 pesticides commonly used in crops in Indiana were detected on milkweed leaves (Table 3). It is important to note, however, that this is not a comprehensive list. While we screened a relatively large number of pesticides, focusing on ones that we know are ubiquitous components of row crop pest management in our region, some compounds are difficult to detect due to factors such as high volatility (e.g., dicamba) or require a different, more specialized analytical approach for quantification (e.g., glyphosate).
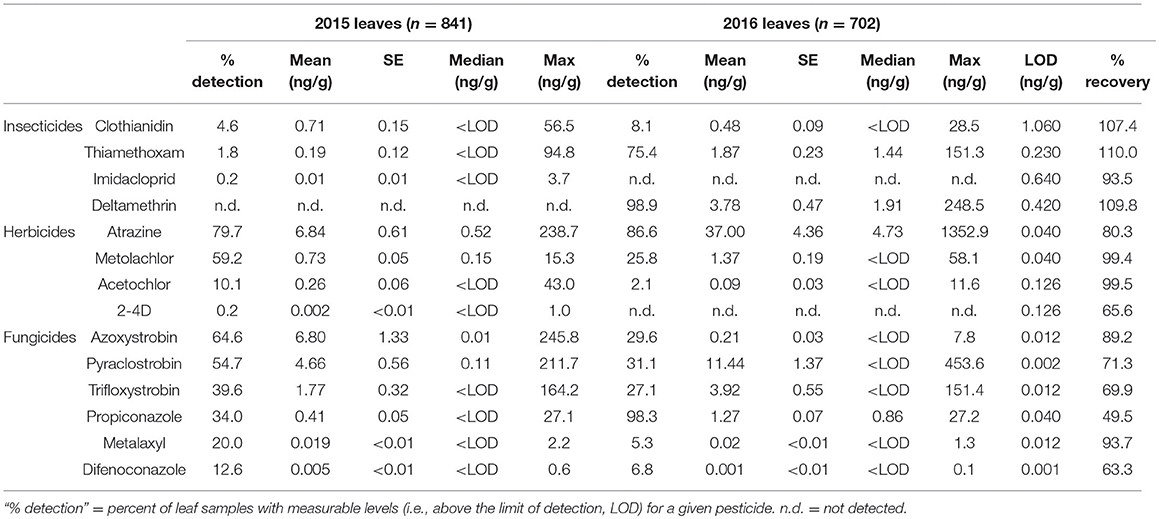
Table 3. Summary data for pesticides detected in milkweed leaf samples across both years of the study.
Clothianidin, the insecticide that to date has received the most attention for potential non-target impacts on monarchs, was only detected in 4–8% of total samples; however, those values are somewhat misleading since it averages across all sites and dates. As a general pattern for both sampling years, we almost exclusively detected clothianidin in June, but not in July or August (Figures 1A,D). During these early season samples, clothianidin was detected in ca. 15–25% of plants with nearly 60% of milkweeds at some sites testing positive. Interestingly, both thiamethoxam (neonicotinoid) and deltamethrin (pyrethroid) varied dramatically in their detection rates across years (SI Appendix, Table S4), with both compounds occurring at high frequencies in 2016 (75–99%) while being virtually absent from samples in 2015 (Figures 1A,D). Imidacloprid (neonicotinoid) was only found in a small number of plants (0.2%) in the first year of this study.
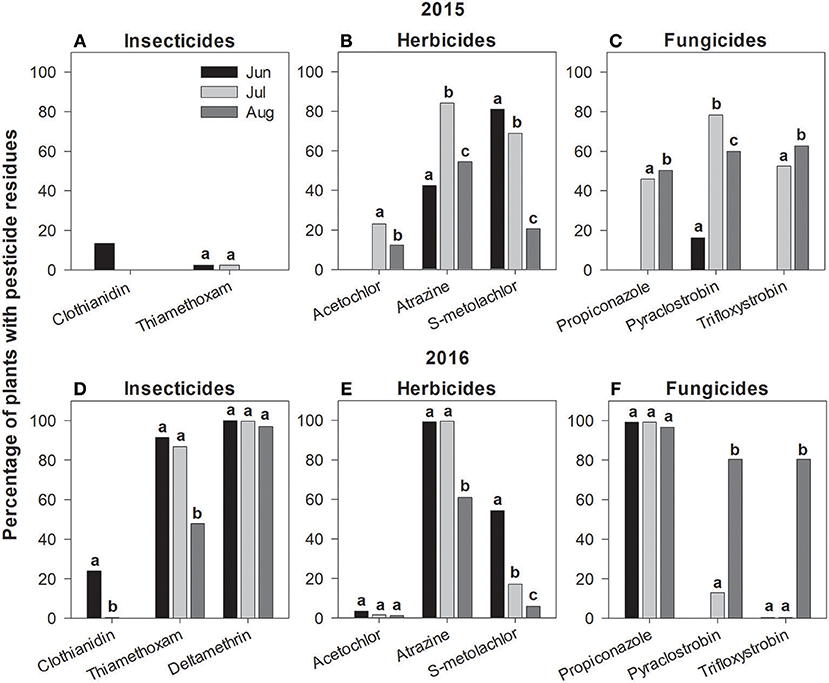
Figure 1. Frequency of pesticide residues detected from milkweed leaf tissue during June, July and August 2015 (A–C) and 2016 (D–F). Percentages are calculated from samples summed across all study sites. Months with different letters, by pesticide, indicate significant differences (P < 0.05).
Atrazine was the most commonly detected (80–87% on average, although some months approached 100% of samples) and occurred at the highest mean concentrations (6.84 and 37.0 ng/g) of any pesticide surveyed in either year, followed by s-metolachlor and acetochlor among the herbicides (Table 3). Notably, s-metolachlor displayed consistent within-season patterns in both sampling years whereby detection rates were several-fold higher early in the season before gradually declining in July and August (Figures 1B,E; SI Appendix, Table S4).
Overall, fungicides were the most omnipresent of pesticides detected on milkweed with 6 compounds consistently occurring on leaves. Several fungicides, most notably propiconazole (98% detection rate in 2016), were somewhat commonly detected, but only at trace (< 1 ng/g) amounts. The compounds that combined relatively high concentrations and detection rates included pyraclostrobin (31–55%) and trifloxystrobin (27–40%; Table 3). In contrast with the herbicide s-metolachlor, which decreased throughout the season, the two strobilurin fungicides showed the opposite pattern, gradually increasing from June to August in both years (Figures 1C,F; SI Appendix, Table S4). Propiconazole detection frequency displayed a nearly 3-fold increase between years one and two, from 34 to 98% of samples.
Soil Pesticides
We found 7 pesticides in soil across the sites sampled in 2016 (Table 4), which were a subset of the 14 pesticides recorded from milkweed leaves. Clothianidin was the only insecticide detected and it was found in all samples consistently throughout the summer (Figure 2A), in contrast with leaf presence, which was restricted to only June. Thus, clothianidin was far more ubiquitous in the soil than leaves. Importantly, soil concentrations of clothianidin were highly correlated with levels in co-occurring milkweed leaves (Figure 3; r = 0.763, p < 0.0001). This was the only pesticide showing a soil-plant association.
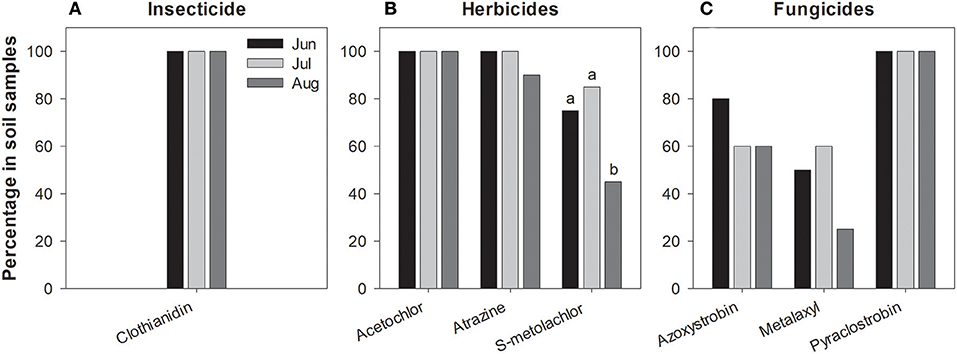
Figure 2. Frequency of pesticide residues detected in soil samples during June, July and August 2016 (A–C). Percentages are calculated from samples summed across all study sites. Significant differences were detected only in s-metolachlor (P < 0.05).
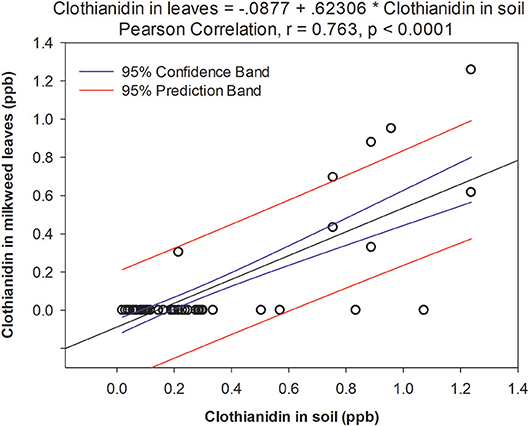
Figure 3. Relationship between clothianidin concentration detected in soil and leaves from adjacent milkweed plants. Data points represent a paired soil-leaf sample for a given site/month.
We detected three herbicides—atrazine, s-metolachlor, acetochlor—and three fungicides—azyoxystrobin, pyraclostrobin, metalaxyl. Similar to clothianidin, soil concentrations of these compounds tended to be far more stable over time, i.e., leaf values fluctuated dramatically across months when the same soil values remained relatively constant (compare Figure 1 vs. Figure 2) even though half-life of pesticides vary with soil physical and chemical characteristics and our plants grow under different soil types (SI Appendix, Table S1).
Land Use
Linear distance separating milkweed plants from agricultural fields was a strong predictor of thiamethoxam detection frequency at all of the sites evaluated (Table 5). However, distance frequently interacted with sampling month, resulting in variation in the nature of the relationship over time. In 6 of 9 cases (3 sites × 3 months), detection rates declined with increasing distance separating milkweed from crop field up to 150 m, although the shape of this relationship varied (Figure 4). The other two insecticides either showed no spatial patterning (clothianidin; no significant main or interactive effects of distance from crop) or were detected in nearly 100% of samples and thus did not have sufficient variation in detection frequency to statistically evaluate using binary data (deltamethrin in 2016, Table 3). When continuous concentration data were used after removing samples below the detection threshold, one of the three sites also showed a distance relationship involving thiamethoxam (Figure 5; distance x month, F = 11.35, P < 0.0001). Similar to detection data, concentrations were higher in milkweeds growing closer to field edges. As with binary data, no relationships were observed for clothianidin or deltamethrin.
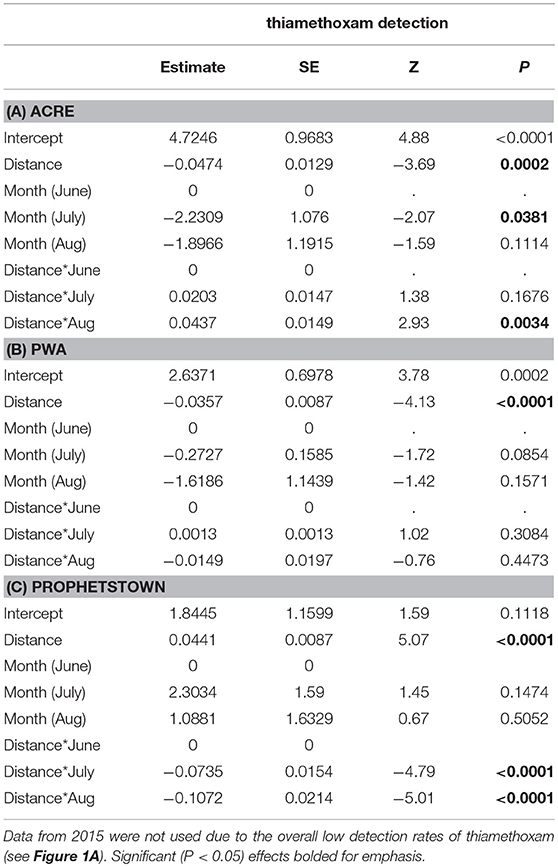
Table 5. The effects of month and distance separating focal plants from the nearest cropland on thiamethoxam detection associated with milkweed leaves at three sites (A–C) sampled in 2016.
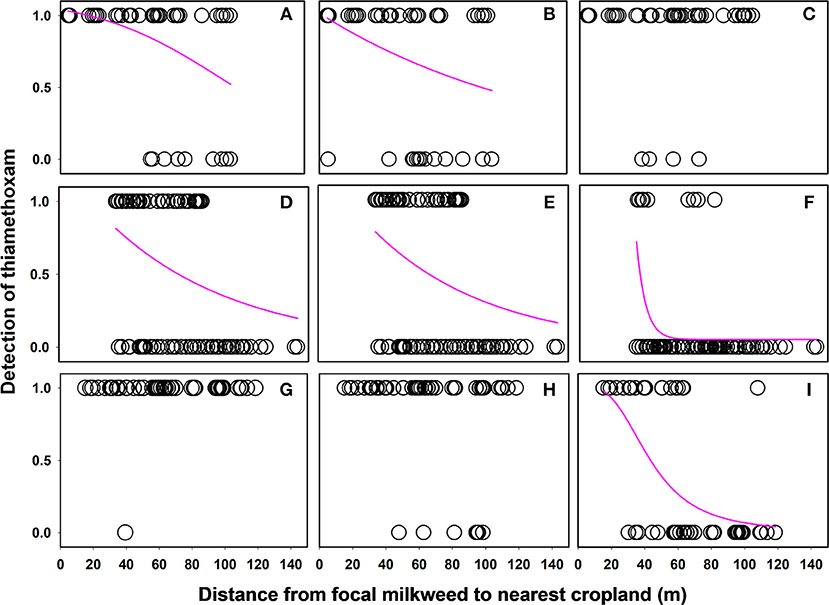
Figure 4. Relationship between distance separating focal plants from cropland and detection of the neonicotinoid thiamethoxam associated with milkweed leaves. Each data point is an individual plant sample with “1” values = thiamethoxam detected and “0” values = thiamethoxam not detected. Best fit curves plotted to the data using sigmoidal or exponential decay functions. Data are for June, July, and August, respectively, for three sites in Indiana: ACRE (A–C), PWA (D–F), and Prophetstown (G–I).
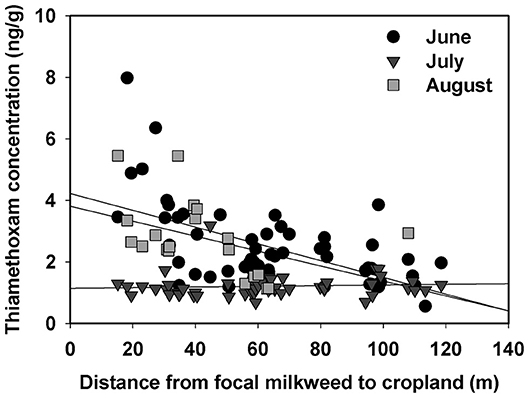
Figure 5. Relationship between distance separating focal plants from cropland and concentration of the neonicotinoid thiamethoxam associated with milkweed leaves at one of our sites, Prophetstown. Each data point is an individual plant sample for June, July, or August.
Although we found substantial site-level variation in pesticide presence on milkweed, landscape composition—namely, amount of corn and soybean—within a 1 km radius surrounding focal sites was a poor predictor of our data. Across both 2015 (Figure 6) and 2016 (Figure 7), only one pesticide—pyraclostrobin in 2015 (Figure 6D; F = 8.61, P < 0.05)—showed a relationship between land use and detection frequency (SI Appendix, Table S5). In this case, percent of plants with measurable amounts of pyraclostrobin increased from ca. 40 to 70% when comparing the least to most agricultural sites.
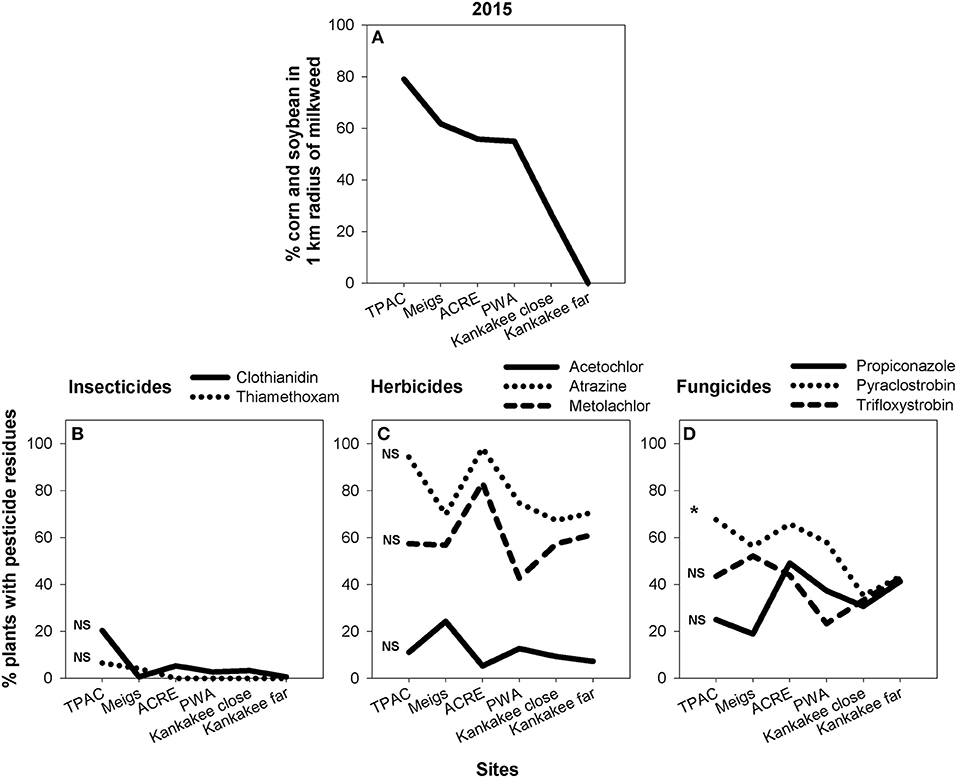
Figure 6. Area of corn and soybean, expressed as percent of total land use, in 1 km buffer surrounding the six milkweed sites sampled in 2015 (A) and corresponding site-level changes in insecticide (B), herbicide (C), and fungicide (D) residues associated with milkweed leaves. Statistical analyses compare, within each pesticide, the relationship between agricultural intensification and pesticide detection frequency. *p < 0.05, ns = not significant.
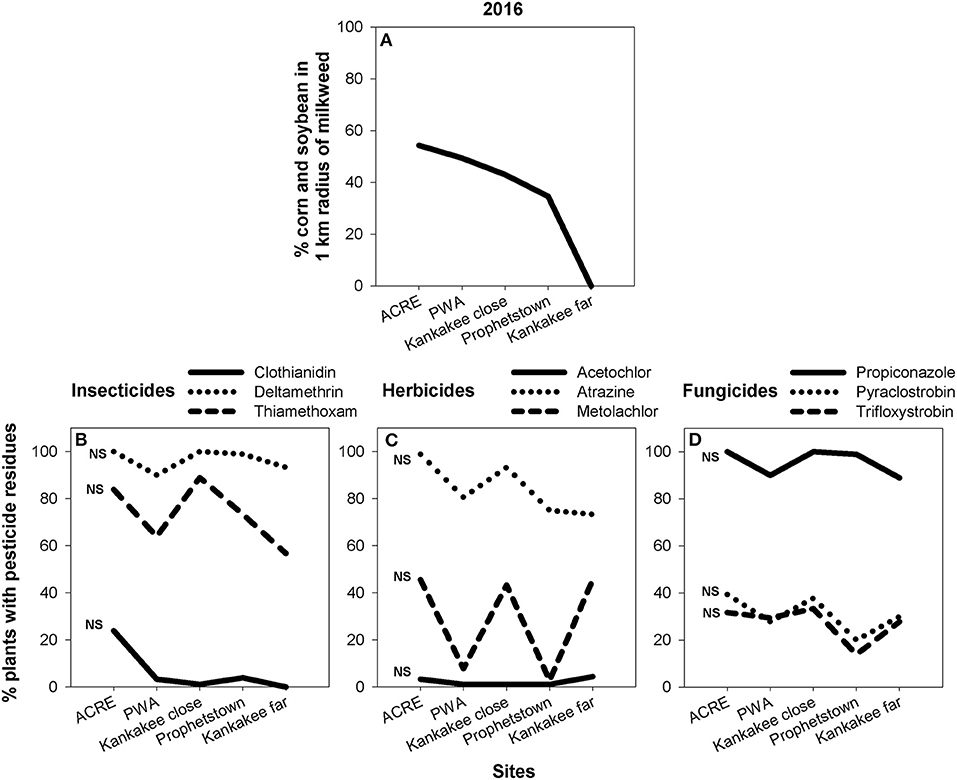
Figure 7. Area of corn and soybean, expressed as percent of total land use, in 1 km buffer surrounding the five milkweed sites sampled in 2016 (A) and corresponding site-level changes in insecticide (B), herbicide (C), and fungicide (D) residues associated with milkweed leaves. Statistical analyses compare, within each pesticide, the relationship between agricultural intensification and pesticide detection frequency. ns = not significant.
Finally, at the county-level, which encompasses the broadest spatial scale employed (for reference, Tippecanoe Co. is ca. 1,300 km2), the total amount of fungicides applied to soybean had a marginally significant (r = 0.85, P = 0.06) effect on the percent detection frequency of fungicides for milkweed leaves in 2015 (Figure 8B). However, other relationships were not significant (corn 2015, r = 0.38, P = 0.28; corn 2016, r = 0.18, P = 0.77).
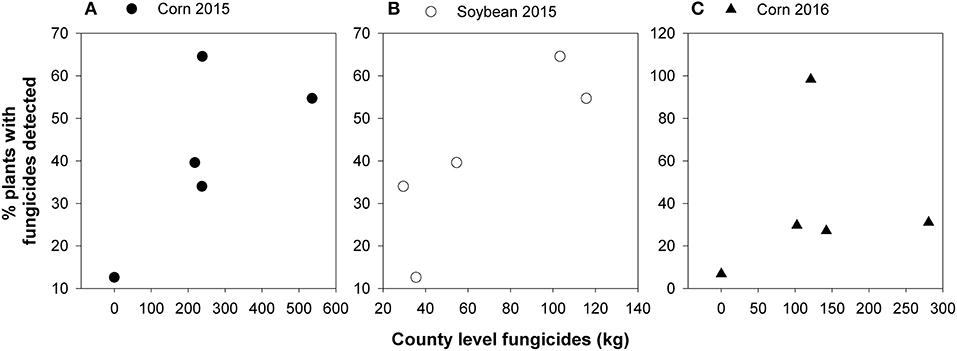
Figure 8. Relationship between amounts of corn (A,C) and soybean (B) fungicides used in Tippecanoe County in 2015 and 2016 vs. the percent of plants with detectable levels of fungicides. Data points show each of the five fungicides detected in our analysis.
Discussion
Our study clearly shows that the foliage of milkweed growing in prairies and unmanaged habitats neighboring cropland contains residues from a wide variety of agricultural pesticides, primarily those applied to corn and soybean. The actual risk of these pesticides, however, depends on how frequently milkweeds contain those levels in the field. Our data reveal strong spatiotemporal variation in pesticide occurrence across sites, months, and years, which means that the threat posed by these chemicals depends on if, when, and where they coincide with monarch colonization and phenology. Below we highlight the implications of these findings for each of the three major pesticide classes and discuss whether pesticide exposure can be avoided based on local and landscape-level habitat placement.
Insecticides
Contamination of non-target plants by neonicotinoids used in agriculture is widely reported, but almost exclusively for pollen or nectar samples taken from flowers (Greatti et al., 2003; Krupke et al., 2012; Bonmatin et al., 2014; Botías et al., 2015, 2016; Mogren and Lundgren, 2016). Consistent with this literature, our study found neonicotinoid residues associated with milkweed leaves around farmland, specifically the active ingredients clothianidin and thiamethoxam. Although seed treatment data are no longer reported for U.S. row crops, most corn in our region is seed treated, primarily with clothianidin, and much of the soybean acreage also employs a seed treatment, mainly thiamethoxam (Douglas and Tooker, 2015). Corn and soybean dominate land use in the areas surrounding each of our milkweed sites, and thus it is not surprising that these two insecticides were among the ones most commonly detected.
Importantly, the leaf concentrations we recorded (up to 56.5 and 151.3 ng/g for clothianidin and thiamethoxam, respectively) are within the range previously reported from other studies. For example, a recent analysis of clothianidin on the leaves of plants used in pollinator strips bordering seed-treated corn fields reported values that were comparable to our milkweed data (Mogren and Lundgren, 2016), including sunflower (max. 81 ng/g), buckwheat (max. 54 ng/g), and phacelia (max. 33 ng/g). Interestingly, some milkweed concentrations were also roughly similar to those reported from the leaves of seed-treated crops such as corn (7–86 ng/g at 20–34 days post planting; Alford and Krupke, 2017) and soybean (105 ng/g in V1 stage and 1.7 ng/g in V4 stage after 17 and 56 days; Magalhaes et al., 2009). Perhaps most relevant to our study, Pecenka and Lundgren (2015) documented clothianidin in 36–64% of milkweed leaves surveyed in South Dakota at mean concentrations of 1.24 and 1.11 ng/g. By comparison, we detected clothianidin at a far lower rate (4.6 and 8.1%, overall, for the 2 years), but with comparable mean values (0.71 and 0.48 ng/g). Pecenka and Lundgren (2015) used dose-response curves for monarch larvae to clothianidin, which revealed the LC50 at 15.63 ng/g and sublethal effects at as little as 1 ng/g. Based on extrapolating these calculations to our field data, sublethal effects should be observed for monarchs on 5–8% of leaves surveyed (averaged across all sites, months, and years; risk varies seasonally), whereas lethal effects (i.e., > LC50) are limited to 1.4% of samples. It is important to note that our assessment is based solely on clothianidin, for which data exist on monarch growth and survival. Our second sampling year revealed that thiamethoxam can be much more prevalent—detected in 75% of samples—but its toxicity to monarchs is unknown at present.
Another critical aspect of our neonicotinoid data is that during both years of the study, residues diminished dramatically over the course of the summer. We virtually only detected clothianidin in June, and thiamethoxam detection in year 2 dropped by ~50% from June-July to August. This within-season decline would be consistent with pesticide degradation from the putative time of exposure (i.e., when seed-treated fields are planted in late spring) to the timing of when milkweeds were sampled. More importantly, the data suggest that early-season monarchs are at greater risk from neonicotinoid exposure than subsequent generations occurring later in the season. Similarly, our data suggest strong annual fluctuations in risk, indicating that monarchs likely encounter a different suite of pesticides each year. Thiamethoxam and deltamethrin, for example, were more prevalent in the second sample year. This is likely a result of local or regional differences in pest management approaches employed by farms. Active ingredients for foliar sprays such as deltamethrin can vary greatly across years, depending on factors such as price and availability. Thiamethoxam is more likely to be a reflection of seed treatments, which vary with the relative acreage of corn vs. soybean in the landscape. Further, in corn/soy rotations, the insecticides used will change on an alternate year basis. Overlaying temporal variation in pesticide presence with the timing of non-target insect colonization and development is a key component to risk assessment that, to our knowledge, is rarely incorporated into such studies.
While we did not document the mechanism by which neonicotinoids moved from cropland to milkweeds in this study, for clothianidin we found a strong positive relationship between soil and leaf concentrations (Figure 3). This could be simply correlative (i.e., areas with high neonicotinoid deposition result in correspondingly higher concentrations both in soil and on plant surfaces), or indicative of systemic uptake from soil into nearby plants. In all cases, we analyzed whole tissue samples so, unfortunately, do not know whether pesticides are on the leaf surface or inside the plant, for systemic compounds. Overall, the clothianidin concentrations in our soil samples (range: 0.88–8.59 ng/g; mean: 1.75 ng/g) were comparable with those reported in other studies of agricultural soils, i.e., 6.57 ng/g (Botías et al., 2015), 7.0 ng/g (Xu et al., 2016), 2.1 and 6.3 ng/g (Krupke et al., 2012).
Last, in 2016 we frequently detected the pyrethroid deltamethrin in milkweed samples. Although pyrethroids are considered highly toxic to lepidopterans in general, nothing is known specifically about the deltamethrin-monarch relationship. A few studies have found negative non-target effects of the related pyrethroids, permethrin, and resmethrin, used in mosquito control on monarch caterpillars (Oberhauser et al., 2006, 2009). Similarly, field applications of deltamethrin in the UK increased mortality of Pieris butterfly larvae developing in hedgerows bordering cereals (Cilgi and Jepson, 1995). Topical application of 20 ng was sufficient to kill 50% of P. brassicae individuals after 2 weeks of exposure (Cilgi and Jepson, 1995); however, host plants influence caterpillar susceptibility to deltamethrin (Tan and Guo, 1996), and thus it is difficult to extrapolate these values for milkweed.
Herbicides and Fungicides
Monarch decline is often attributed to an indirect effect from glyphosate reducing milkweed abundance (Hartzler, 2010; Pleasants and Oberhauser, 2013; Pleasants, 2017). Yet, the direct effects of herbicides on monarchs (i.e., those not merely caused by a reduction in milkweed availability) are unknown, and likely dismissed since herbicides are considered non-toxic to insects (but see Russell and Schultz, 2009; Stark et al., 2012). Potential non-target pathways could occur via herbicide exposure, either topically or orally, changing some aspect of caterpillar physiology or altering the milkweed-monarch interaction by e.g., interfering with or amplifying the induced defense pathways employed by milkweeds (Boutin et al., 2004, 2014). For instance, the herbicide 2,4D functions as a plant defense elicitor, resulting in resistance to herbivorous insects on plants exposed to low doses (Xin et al., 2012). Also, drift of the herbicide dicamba into field margins reduced pollinator visitation rates (Bohnenblust et al., 2016), impacted the abundance of several arthropods in the community (Egan et al., 2014), and decreased caterpillar development (Bohnenblust et al., 2016). Herbicides such as glyphosate can even act directly on pollinators by disrupting their gut microbiome (Motta et al., 2018).
Of the herbicides sampled, atrazine was the most commonly detected and at the highest concentrations. Much of what is known about atrazine's impacts on invertebrates comes from aquatic food webs where run-off into streams or lakes alters community structure (Dewey, 1986; Gruessner and Watzin, 1996). While these are mostly indirect effects via reductions in the population of algae or related macrophytes, direct effects of atrazine on insects are documented (Miota et al., 2000; Graymore et al., 2001), as well as their role in synergizing insecticides such as organophosphates (Anderson and Lydy, 2002).
For fungicides, the compounds we detected in milkweed leaves largely match those reported from pollen, honey, nectar, wax, and foliage of wildflowers or crops (Krupke et al., 2012; Sanchez-Bayo and Goka, 2014; David et al., 2016; Long and Krupke, 2016). Fungicides inhibiting ergosterol biosynthesis, like propiconazole, act as synergists for neonicotinoid insecticides, increasing their toxicity to bees by inhibiting cytochrome P450s that function in detoxification (Pilling and Jepson, 1993; Pilling et al., 1995; Johnson et al., 2013). There is also the potential for additive toxicity when insects are exposed to mixtures of pesticides. Propiconazole was detected in 98% of milkweed samples in 2016, in many cases co-occurring with insecticides like deltamethrin and thiamethoxam. The high frequency of the fungicides propiconazole, pyraclostrobin, and trifloxystrobin compared with metalaxyl and azoxystrobin could be related to the high use of these fungicides to increase yield in hybrid corn and soybean (Paul et al., 2011; Mahoney et al., 2015).
Land Use
For the neonicotinoid thiamethoxam, we found that detection frequency and concentrations tended to be higher on milkweeds growing in closer proximity to agricultural land. This suggests that spatially isolating milkweed restoration sites from crop fields could be an effective approach to reduce risk. To our knowledge, the proposed 125 ft buffer distance is a somewhat arbitrary value that is not based on specific criteria; however, our data nevertheless suggest that milkweed habitat restoration abiding by this rule would likely result in fewer plants containing thiamethoxam and at lower concentrations (see values > 38.1 m on Figures 4, 5). What remains unclear is the degree to which these reductions result in enhanced survival and/or performance of monarch caterpillars, which is the ultimate goal. This would require experimentally rearing larvae on plants in the field along a distance transect extending from a crop field edge. In fact, bypassing high quality monarch habitat on land that is relatively close to a corn or soybean field could have a net detrimental effect on monarch conservation if the benefits of additional milkweed stems exceed the detrimental impact of higher pesticide load; a scenario that is entirely plausible, depending on the factor(s) most limiting monarch fitness. This is particularly true for pesticides such as clothianidin that already occur at relatively low frequencies. Deltamethrin also occurred at frequencies and concentrations that were independent of distance to crop. This could be due to the fact that this insecticide is likely applied via aerially spraying, which may result in greater propensity for drift beyond the immediate surrounding of crop fields.
At the landscape level, amount of row crop production in the 1 km radius around milkweed sites was generally a poor predictor of pesticide presence on milkweeds. Only one of the pesticides tested—pyraclostrobin—showed a significant relationship whereby prevalence increased on milkweeds with increasing agricultural intensity. That being said, several of the pesticides, including clothianidin in both years, were most prevalent at the most heavily agricultural site while showing the lowest occurrence at the least agricultural site. We suspect that the lack of statistical power due to low site replication (n = 6 and 5 sites in 2015 and 2016, respectively) played a role in these outcomes, especially for a coarse predictor variable like total crop acres that does not account for variation in local site factors. A similar conclusion was drawn from a recent study of pesticide residues on native bees; despite trends, land cover in a 1 km radius around sites was non-significant, likely due to low site replication (Hladik et al., 2018). Our county-level analysis led to an analogous conclusion. Correlations suggested that greater use by farmers at the regional scale increased prevalence of fungicides on milkweeds, but statistical effects were equivocal (i.e., marginal significance) due to low replication (Figure 8).
Conclusions
Risk assessment evaluating the potential impacts of pesticides on monarchs entails a two-step process; first, documenting the chemicals that larvae and/or adults are exposed to in the environment, and second, experimentally testing those chemicals most commonly encountered to assess lethal and sub-lethal effects. Here, we take the first step in this process, documenting the spectrum of pesticides encountered by monarch larvae on the most critical host-plant in their summer breeding range, A. syriaca. We strongly emphasize, however, that pesticide presence does not necessarily translate into impact. Unlike honeybees, for which LD50 data are widely available on most compounds, at present such information is only available for clothianidin in the monarch system. Clearly, a major emphasis of future research efforts should be to close this knowledge gap by quantifying monarch larval responses to a range of pesticides under controlled lab settings. Based on our field data, obvious starting points for these trials would be insecticides such as thiamethoxam and several of the ubiquitous fungicides that occur on milkweed leaves.
Assuming pesticide presence is undesirable for land managers focused on restoring milkweed for monarch conservation, our data secondarily point to local habitat placement—namely, site isolation—as an effective tool for reducing non-target exposure. Additional work to help refine these recommendations could focus on site-specific factors that contribute to off-site pesticide drift beyond simple linear distance, e.g., wind direction, slope, soil type.
Author Contributions
PO-A wrote the manuscript, collected, and analyzed the data. IK guided the research, reviewed, and edited the manuscript.
Funding
This research was supported by a dissertation fellowship from the Colombian government-Colciencias, and partial grants from the Nature Conservancy, Xerces Society, Experiment.com, and Purdue AgSeed.
Conflict of Interest Statement
The authors declare that the research was conducted in the absence of any commercial or financial relationships that could be construed as a potential conflict of interest.
Acknowledgments
We are grateful to the many collaborators who made this project possible. Specifically, we thank those students involved in lab/field assistance for sample collection and analysis (Ivy Widick, Ashley Kirtman, Carolina Zamorano, Javier Lenzi, Rosario Uribe, Sylvia Bonilla, Rachel Thomas, Andrés Sandoval, Megan McCarty, Kayleigh Hauri); ArcGIS professional guidance (Mayra Rodriguez and Mariam Valladares); staff members at each field location for their help with site logistics (Pete Illingworth, Jay Young, Nate Linder, Brian Baheler, John Shuey, James Beaty, Jason Getz, Kile Westerman); personnel who assisted with pesticide residue analysis at the Metabolite Profiling Facility of the Bindley BioScience Center at Purdue (Amber Hopf Jannasch, Bruce Cooper, Jackeline Franco, Elizabeth Long, Christian Krupke, Mercedes Laland, Spencer Bockover); Laura Cruz for statistical guidance; and Maggie Douglas for help with interpreting state- and county-level pesticide records. A special thanks to Larry Bledsoe who provided detailed information related to corn and soybean at the beginning of this project and tools necessary for the success of the data collection in the field. Finally, thanks to thesis committee members—Cliff Sadof, Michael Scharf, Jen Zaspel, and Kevin Gibson—and students/postdocs in the Kaplan Lab—Wadih Ghanem, Laura Ingwell, Jacob Pecenka, Christie Shee, John Ternest—for their valuable comments on the manuscript.
Supplementary Material
The Supplementary Material for this article can be found online at: https://www.frontiersin.org/articles/10.3389/fevo.2019.00223/full#supplementary-material
References
Aktar, M. W., Sengupta, D., and Chowdhury, A. (2009). Impact of pesticides use in agriculture: their benefits and hazards. Interdiscip. Toxicol. 2, 1–12. doi: 10.2478/v10102-009-0001-7
Alford, A., and Krupke, C. H. (2017). Translocation of the neonicotinoid seed treatment clothianidin in maize. PLoS ONE 12:e0186527. doi: 10.1371/journal.pone.0186527
Altizer, S. M., Hobson, K. A., Davis, A. K., De Roode, J. C., and Wassenaar, L. I. (2015). Do healthy monarchs migrate farther? Tracking natal origins of parasitized vs. uninfected monarch butterflies overwintering in Mexico. PLoS ONE 10:e0141371. doi: 10.1371/journal.pone.0141371
Altizer, S. M., and Oberhauser, K. S. (1999). Effects of the protozoan parasite ophryocystis elektroscirrha on the fitness of monarch butterflies (Danaus plexippus). J. Invert. Path. 74, 76–88. doi: 10.1006/jipa.1999.4853
Altizer, S. M., Oberhauser, K. S., and Geurts, K. A. (2004). “Transmission of the protozoan parasite, ophryocystis elektroscirrha in monarch butterfly populations: implications for prevalence and population-level impacts,” in The Monarch Butterfly: Biology and Conservation (Ithaca, NY: Cornell University Press), 203–218.
Anderson, T. D., and Lydy, M. J. (2002). Increased toxicity to invertebrates associated with a mixture of atrazine and organophosphate insecticides. Environ. Toxicol. Chem. 21, 1507–1514. doi: 10.1002/etc.5620210724
Bohnenblust, E. W., Vaudo, A. D., Egan, J. F., Mortensen, D. A., and Tooker, J. F. (2016). Effects of the herbicide dicamba on nontarget plants and pollinator visitation. Environ. Toxicol. Chem. 35, 144–151. doi: 10.1002/etc.3169
Bonmatin, J. M., Giorio, C., Girolami, V., Goulson, D., Kreutzweiser, D. P., et al. (2014). Environmental fate and exposure; neonicotinoids and fipronil. Environ. Sci. Pollut. Res. 22, 35–67. doi: 10.1007/s11356-014-3332-7
Botías, C., David, A., Hill, E. M., and Goulson, D. (2016). Contamination of wild plants near neonicotinoid seed-treated crops, and implications for non-target insects. Sci. Total Environ. 566, 269–278. doi: 10.1016/j.scitotenv.2016.05.065
Botías, C., David, A., Horwood, J., Abdul-Sada, A., Nicholls, E., Hill, E. M., et al. (2015). Neonicotinoid residues in wildflowers, a potential route of chronic exposure for bees. Environ. Sci. Tech. 49, 12731–12740. doi: 10.1021/acs.est.5b03459
Boutin, C., Elmegaard, N., and Kjaer, C. (2004). Toxicity testing of fifteen non-crop plant species with six herbicides in a greenhouse experiment: implications for risk assessment. Ecotoxicology 13, 349–369. doi: 10.1023/B:ECTX.0000033092.82507.f3
Boutin, C., Strandberg, B., Carpenter, D., Mathiassen, S. K., and Thomas, P. J. (2014). Herbicide impact on non-target plant reproduction: what are the toxicological and ecological implications? Environ. Pollut. 185, 295–306. doi: 10.1016/j.envpol.2013.10.009
Brower, L. P., Taylor, O. R., Williams, E. H., Slayback, D. A., Zubieta, R. R., and Ramírez, M. I. (2012). Decline of monarch butterflies overwintering in México: is the migratory phenomenon at risk? Insect Conserv. Div. 5, 95–100. doi: 10.1111/j.1752-4598.2011.00142.x
Cilgi, T., and Jepson, P. C. (1995). The risks posed by deltamethrin to hedgerow butterflies. Environ. Pollut. 87, 378–386. doi: 10.1016/S0269-7491(99)80001-3
David, A., Botías, C., Abdul-Sada, A., Nicholls, E., Rotheray, E. L., Hill, E. M., et al. (2016). Widespread contamination of wildflower and bee-collected pollen with complex mixtures of neonicotinoids and fungicides commonly applied to crops. Environ. Internat. 88, 169–178. doi: 10.1016/j.envint.2015.12.011
Davis, B. N. K., Lakhani, K. H., and Yates, T. J. (1991). The hazards of insecticides to butterflies of field margins. Agric. Ecosyst. Environ. 36, 151–161. doi: 10.1016/0167-8809(91)90012-M
De Snoo, G. R., van der Poll, R. J., and Bartels, J. (1998). Butterflies in sprayed and unsprayed field margins. J. Appl. Entomol. 122, 157–161. doi: 10.1111/j.1439-0418.1998.tb01478.x
Dewey, S. L. (1986). Effects of the herbicide atrazine on aquatic insect community structure and emergence. Ecology 67, 148–162. doi: 10.2307/1938513
Douglas, M. R., Rohr, J. R., and Tooker, J. F. (2015). Neonicotinoid insecticide travels through a soil food chain, disrupting biological control of non-target pests and decreasing soya bean yield. J. Appl. Ecol. 52, 250–260. doi: 10.1111/1365-2664.12372
Douglas, M. R., and Tooker, J. F. (2015). Large-scale deployment of seed treatments has driven rapid increase in use of neonicotinoid insecticides and preemptive pest management in U.S. field crops. Environ. Sci. Tech. 49, 5088–5097. doi: 10.1021/es506141g
Dover, J. W., Sotherton, N., and Gobbett, K. (1990). Reduced pesticide inputs on cereal field margins: The effects on butterfly abundance. Ecol. Entomol. 15, 17–24. doi: 10.1111/j.1365-2311.1990.tb00779.x
Egan, J. F., Bohnenblust, E., Goslee, S., Mortensen, D., and Tooker, J. F. (2014). Herbicide drift can affect plant and arthropod communities. Agric. Ecosys. Environ. 185, 77–87. doi: 10.1016/j.agee.2013.12.017
Epstein, L. (2014). Fifty years since Silent Spring. Annu. Rev. Phytopathol. 52, 377–402. doi: 10.1146/annurev-phyto-102313-045900
Flockhart, D. T. T., Pichancourt, J. –B., Norris, D. R., and Martin, T. G. (2015). Unravelling the annual cycle in a migratory animal: breeding-season habitat loss drives population declines of monarch butterflies. J. Anim. Ecol. 84, 155–165. doi: 10.1111/1365-2656.12253
Forister, M. L., Cousens, B., Harrison, J. G., Anderson, K., Thorne, J. H., Waetjen, D., et al. (2016). Increasing neonicotinoid use and the declining butterfly fauna of lowland California. Biol. Lett. 12:20160475. doi: 10.1098/rsbl.2016.0475
Gilburn, A. S., Bunnefeld, N., Wilson, J. M., Botham, M. S., Brereton, T. M., Fox, R., et al. (2015). Are neonicotinoid insecticides driving declines of widespread butterflies? PeerJ 3:e1402. doi: 10.7717/peerj.1402
Graymore, M., Stagnitti, F., and Allinson, G. (2001). Impacts of atrazine in aquatic ecosystems. Environ. Internat. 26, 483–495. doi: 10.1016/S0160-4120(01)00031-9
Greatti, M., Sabatini, A. G., Barbattini, R., Rossi, S., and Stravisi, A. (2003). Risk of environmental contamination by the active ingredient imidacloprid used for corn seed dressing. Preliminary results. Bullet. Insect. 56, 69–72.
Gruessner, B., and Watzin, M. C. (1996). Response of aquatic communities from a Vermont stream to environmentally realistic atrazine exposure in laboratory microcosms. Environ. Toxicol. Chem. 15, 410–419. doi: 10.1002/etc.5620150402
Hartzler, R. G. (2010). Reduction in common milkweed (Asclepias syriaca) occurrence in Iowa cropland from 1999 to 2009. Crop Prot. 29, 1542–1544. doi: 10.1016/j.cropro.2010.07.018
Hartzler, R. G., and Buhler, D. D. (2000). Occurrence of common milkweed (Asclepias syriaca) in cropland and adjacent areas. Crop Prot. 19, 363–366. doi: 10.1016/S0261-2194(00)00024-7
Hladik, M. L., Main, A. R., and Goulson, D. (2018). Environmental risks and challenges associated with neonicotinoid insecticides. Environ. Sci. Technol. 52, 3329–3335. doi: 10.1021/acs.est.7b06388
Inamine, H., Ellner, S. P., Springer, J. P., and Agrawal, A. A. (2016). Linking the continental migratory cycle of the monarch butterfly to understand its population decline. Oikos 125, 1081–1091. doi: 10.1111/oik.03196
Jeschke, P., and Nauen, R. (2008). Neonicotinoids-from zero to hero in insecticide chemistry. Pest Manag. Sci. 64, 1084–1098. doi: 10.1002/ps.1631
Johnson, R. M., Dahlgren, L., Siegfried, B. D., and Ellis, M. D. (2013). Acaricide, fungicide and drug interactions in honey bees (Apis mellifera). PLoS ONE 8:e54092. doi: 10.1371/journal.pone.0054092
Krischik, V., Rogers, M., Gupta, G., and Varshney, A. (2015). Soil-applied imidacloprid translocates to ornamental flowers and reduces survival of adult Coleomegilla maculata, Harmonia axyridis, and Hippodamia convergens lady Beetles, and larval Danaus plexippus and Vanessa cardui Butterflies. PLoS ONE 10:e0119133. doi: 10.1371/journal.pone.0119133
Krupke, C. H., Hunt, G. J., Eitzer, B. D., Andino, G., and Given, K. (2012). Multiple routes of pesticide exposure for honey bees living near agricultural fields. PLoS ONE 7:e29268. doi: 10.1371/journal.pone.0029268
Krupke, C. H., Prasad, R. P., and Anelli, C. M. (2007). Professional entomology and the 44 noisy years since Silent Spring part 2: response to Silent Spring. Amer. Entomol. 53, 16–26. doi: 10.1093/ae/53.1.16
Long, E. Y., and Krupke, C. H. (2016). Non-cultivated plants present a season-long route of pesticide exposure for honey bees. Nat. Comm. 7:11629. doi: 10.1038/ncomms11629
Longley, M., and Sotherton, N. W. (1997). Factors determining the effects of pesticides upon butterflies inhabiting arable farmland. Agric. Ecosyst. Environ. 61, 1–12. doi: 10.1016/S0167-8809(96)01094-8
Longley, M., and Stark, J. D. (1996). Analytical techniques for quantifying direct, residual, and oral exposure of an insect parasitoid to an organophosphate insecticide. Bull Environ. Contam. Toxicol. 57, 683–690. doi: 10.1007/s001289900244
Magalhaes, L. C., Hunt, T. E., and Siegfried, B. D. (2009). Efficacy of neonicotinoid seed treatments to reduce soybean aphid populations under field and controlled conditions in Nebraska. J. Econ. Entomol. 102, 187–195. doi: 10.1603/029.102.0127
Mahoney, K. J., Vyn, R. J., and Gillard, C. L. (2015). The effect of pyraclostrobin on soybean plant health, yield, and profitability in Ontario. Can. J. Plant Sci. 95, 285–292. doi: 10.4141/cjps-2014-125
Malcolm, S. B. (2018). Anthropogenic impacts on mortality and population viability of the monarch butterfly. Annu. Rev. Entomol. 63, 277–302. doi: 10.1146/annurev-ento-020117-043241
Miota, F., Siegfried, B. D., Scharf, M. E., and Lydy, M. J. (2000). Atrazine induction of cytochrome P-450 in Chironomus tentans. Chemosphere 40, 285–291. doi: 10.1016/S0045-6535(99)00257-X
Mogren, C. L., and Lundgren, J. G. (2016). Neonicotinoid-contaminated pollinator strips adjacent to cropland reduce honey bee nutritional status. Sci. Rep. 6:29608. doi: 10.1038/srep29608
Motta, E. V. S., Raymann, K., and Moran, N. A. (2018). Glyphosate perturbs the gut microbiota of honey bees. Proc. Nat. Acad. Sci. U.S.A. 115, 10305–10310. doi: 10.1073/pnas.1803880115
Oberhauser, K., and Peterson, A. T. (2003). Modeling current and future potential wintering distributions of eastern North American monarch butterflies. Proc. Natl. Acad. Sci. U.S.A. 100, 14063–14068. doi: 10.1073/pnas.2331584100
Oberhauser, K. S., Brinda, S. J., Weaver, S., Moon, R. D., Manweiler, S. A., and Read, N. (2006). Growth and survival of monarch butterflies (Lepidoptera: Danaidae) after exposure to permethrin barrier treatments. Environ. Entomol. 35, 1626–1634. doi: 10.1093/ee/35.6.1626
Oberhauser, K. S., Manweiler, S., Lelich, R., Blank, M., et al. (2009). Impacts of ULV resmethrin applications on non-target insects. J. Am. Mosquito Contr. 25, 83–93. doi: 10.2987/08-5788.1
Paul, P. A., Madden, L. V., Bradley, C. A., Robertson, A. E., Munkvold, G. P., et al. (2011). Meta-analysis of yield response of hybrid field corn to foliar fungicides in the US corn belt. Phytopathology 101, 1122–1132. doi: 10.1094/PHYTO-03-11-0091
Pecenka, J. R., and Lundgren, J. G. (2015). Non-target effects of clothianidin on monarch butterflies. Sci. Nat. 102:19. doi: 10.1007/s00114-015-1270-y
Pilling, E. D., Bromley-Challenor, K. A. C., Walker, C. H., and Jepson, P. C. (1995). Mechanism of synergism between the pyrethroid insecticide alpha-cyhalothrin and the imidazole fungicide prochloraz in the honeybee (Apis mellifera L.). Pestic. Biochem. Physiol. 51:1e11. doi: 10.1006/pest.1995.1001
Pilling, E. D., and Jepson, P. C. (1993). Synergism between EBI fungicides and a pyrethroid insecticide in the honeybee (Apis mellifera). Pestic. Sci. 39, 293–297. doi: 10.1002/ps.2780390407
Pimentel, D. (1995). Amounts of pesticides reaching target pests: environmental impacts and ethics. J. Agric. Environ. Ethics 8, 17–29. doi: 10.1007/BF02286399
Pimentel, D., and Levitan, L. (1986). Pesticides: amounts applied and amounts reaching pests. BioScience 36, 86–91. doi: 10.2307/1310108
Pleasants, J. M. (2017). Milkweed restoration in the Midwest for monarch butterfly recovery: estimates of milkweeds lost, milkweeds remaining and milkweeds that must be added to increase the monarch population. Insect Conserv. Div. 10, 42–53. doi: 10.1111/icad.12198
Pleasants, J. M., and Oberhauser, K. S. (2013). Milkweed loss in agricultural fields because of herbicide use: effect on the Monarch butterfly population. Insect Conserv. Div. 6, 135–144. doi: 10.1111/j.1752-4598.2012.00196.x
Rands, M. R. W., and Sotherton, N. W. (1986). Pesticide use on cereal crops and changes in the abundance of butterflies on arable farmland. Biol. Conserv. 36, 71–82. doi: 10.1016/0006-3207(86)90102-3
Russell, C., and Schultz, C. B. (2009). Effects of grass-specific herbicides on butterflies: an experimental investigation to advance conservation efforts. J. Insect Conserv. 14:53e63. doi: 10.1007/s10841-009-9224-3
Sanchez-Bayo, F., and Goka, K. (2014). Pesticide residues and bees—a risk assessment. PLoS ONE 9:e94482. doi: 10.1371/journal.pone.0094482
Saunders, S. P., Ries, L., Oberhauser, K. S., Thogmartin, W. E., and Zipkin, E. F. (2017). Local and cross-seasonal associations of climate and land use with abundance of monarch butterflies (Danaus plexippus). Ecography 40, 1–12. doi: 10.1111/ecog.02719
Seiber, J. N., Brower, L. P., Lee, S. M., McChesney, M. M., Cheung, H. T. A., Nelson, C. J., et al. (1986). Cardenolide connection between overwintering monarch butter?ies from Mexico and their larval foodplant, Asclepias syriaca. J. Chem. Ecol. 12, 1157–1170. doi: 10.1007/BF01639002
Semmens, B., Semmens, D., Thogmartin, W., Wiederholt, R., Lopez-Hoffman, L., Diffendorfer, J. E., et al. (2016). Extinction risk and population targets for the Eastern, migratory population of monarch butterflies (Danaus plexippus). Sci. Rep. 6:23265. doi: 10.1038/srep23265
Stark, J. D., Chen, X. D., and Johnson, C. (2012). Effects of herbicides on Behr's metalmark butterfly, a surrogate species for the endangered butterfly, Lange's metalmark. Environ. Poll. 164, 24–27. doi: 10.1016/j.envpol.2012.01.011
Stenoien, C., Nail, K. R., Zalucki, J. M., Parry, H., Oberhauser, K. S., and Zalucki, M. P. (2016). Monarchs in decline: a collateral landscape-level effect of modern agriculture. Insect Sci. 25, 528–541. doi: 10.1111/1744-7917.12404
Stone, W. W. (2013). Estimated annual agricultural pesticide use for counties of the conterminous United States, 1992–2009. U.S. Geol. Survey Data Series 2013:752. doi: 10.3133/ds752
Swengel, A. B. (1995). Population fluctuations of the monarch (Danaus plexippus) in the 4th of July butterfly count 1977–1994. Amer. Midl. Nat. 134, 205–214. doi: 10.2307/2426291
Tan, W. J., and Guo, Y. Y. (1996). Effects of host plant on susceptibility to deltamethrin and detoxication enzymes of Heliothis armigera (Lepidoptera: Noctuidae). J. Econ. Entomol. 89, 11–14. doi: 10.1093/jee/89.1.11
Thogmartin, W. E., López-Hoffman, L., Rohweder, J., Diffendorfer, J. E., Drum, R., et al. (2017b). Restoring monarch butterfly habitat in the Midwestern US: ‘all hands on deck'. Environ. Res. Lett. 12:074005. doi: 10.1088/1748-9326/aa7637
Thogmartin, W. E., Wiederholt, R., Oberhauser, K., Drum, R. G., Diffendorfer, J. E., et al. (2017a). Monarch butterfly population decline in North America: identifying the threatening processes. R. Soc. Open. Sci. 4:170760. doi: 10.1098/rsos.170760
Wassenaar, L. I., and Hobson, K. A. (1998). Natal origins of migratory monarch butter?ies at wintering colonies in Mexico: new isotopic evidence. Proc. Nat. Acad. Sci. U.S.A. 95, 15436–15439. doi: 10.1073/pnas.95.26.15436
Whitehorn, P. R., Norville, G., Gilburn, A., and Goulson, D. (2018). Larval exposure to the neonicotinoid imidacloprid impacts adult size in the farmland butterfly Pieris brassicae. PeerJ 6:e4772. doi: 10.7717/peerj.4772
Woodson, R. E. (1954). The North American species of Asclepias L. Ann. Missouri Bot. Garden. 41, 1–211. doi: 10.2307/2394652
Xin, Z., Yu, Z., Erb, M., Turlings, T. C., Wang, B., Qi, J., et al. (2012). The broad-leaf herbicide 2,4-dichlorophenoxyacetic acid turns rice into a living trap for a major insect pest and a parasitic wasp. New. Phytol. 194, 498–510. doi: 10.1111/j.1469-8137.2012.04057.x
Xu, T., Dyer, D. G., McConnell, L. L., Bondarenko, S., Allen, R., and Heinemann, O. (2016). Clothianidin in agricultural soils and uptake into corn pollen and canola nectar after multiyear seed treatment applications. Environ. Toxicol. Chem. 35, 311–321. doi: 10.1002/etc.3281
Keywords: milkweeds, agriculture, pesticides, monarchs, landscape
Citation: Olaya-Arenas P and Kaplan I (2019) Quantifying Pesticide Exposure Risk for Monarch Caterpillars on Milkweeds Bordering Agricultural Land. Front. Ecol. Evol. 7:223. doi: 10.3389/fevo.2019.00223
Received: 31 December 2018; Accepted: 29 May 2019;
Published: 14 June 2019.
Edited by:
Jay E. Diffendorfer, United States Geological Survey, United StatesReviewed by:
Christina Mogren, University of Hawaii at Manoa, United StatesPhilip Neil Smith, Texas Tech University, United States
Copyright © 2019 Olaya-Arenas and Kaplan. This is an open-access article distributed under the terms of the Creative Commons Attribution License (CC BY). The use, distribution or reproduction in other forums is permitted, provided the original author(s) and the copyright owner(s) are credited and that the original publication in this journal is cited, in accordance with accepted academic practice. No use, distribution or reproduction is permitted which does not comply with these terms.
*Correspondence: Paola Olaya-Arenas, cG9sYXlhYXJAcHVyZHVlLmVkdQ==