- 1Access & Aboriginal Focus Programs, University of Manitoba, Winnipeg, MB, Canada
- 2Groupe de Recherche sur les Environnements Nordiques BORÉAS, Département de Biologie, Chimie et Géographie, Centre d'Études Nordiques, Centre de la Science de la Biodiversité du Québec, Université du Québec à Rimouski, Rimouski, QC, Canada
- 3Department of Biological Sciences, University of Windsor, Windsor, ON, Canada
- 4Department of Biological Sciences, University of Manitoba, Winnipeg, MB, Canada
- 5Bird Studies Canada, Port Rowan, ON, Canada
Nomadic movements of migratory birds are difficult to study, as the scale is beyond the capabilities of hand-held telemetry (10 s of kms) but too fine-scale for long-range tracking devices like geolocators (50–100 km accuracy). Recent widespread installation of automated telemetry receiving stations allowed us, for the first time, to quantify and test predictions about within-winter movements of a presumed nomadic species, the Snow Bunting (Pletrophenax nivalis). We deployed coded radio-transmitters on 40 individual Snow Buntings during two winters (2015-16 and 2016-17) in southern Ontario, Canada, and tracked movements over a 300 by 300 km area with 69–77 active radio-receiving stations (Motus Wildlife Tracking Network). To complement our tracking data, we also examined the influence of weather on the demographics of winter flocks at a single wintering site over 6 consecutive years (n = 9312 tagged birds). We recorded movements of 25 Snow Buntings from the deployment sites to 1–6 different radio recievers (mean 2.68 locations/bird). Birds traveled a minimum average distance of 49 km between detections (range: 3 to 490 km) in the core wintering period of Dec-Feb, and cumulative total movements ranged from 3 to 740 km (average 121 ± 46 km). In March distances between detections increased to an average of 110 km, suggesting an extended early-migration period. Overall, older birds (after-second year or older) tended to move more (higher cumulative distances traveled) than younger (first winter) birds, even during the Dec-Feb period. The long-term banding data revealed that larger, male birds were more likely to be captured in colder and snowier weather, relative to female and smaller birds, suggesting that they can withstand these conditions more easily owing to their body size. We have provided the first direct-tracking data on nomadic winter movements of Snow Buntings, and tested the hypothesis that winter weather drives flock composition at a single site. Site-specific banding data suggest that weather-related changes in flock composition could explain the nomadic, landscape-scale movements of Snow Buntings we observed by using automated telemetry. Future work should explore the importance of resource availability, competition, and predation risk as drivers of winter movements in Snow Buntings.
Introduction
Many avian species escape cold winters at breeding sites by migrating to lower latitudes (Newton, 2008). Birds that remain at high latitudes during winter must cope with energetically challenging conditions (Belda et al., 2007). Some individuals, especially when facing highly unpredictable environmental conditions, adopt a nomadic strategy (Stearns, 1976; Senar et al., 1992; Watts et al., 2018). The variability in nomadic movements, both within and across populations and species, remains poorly understood and difficult to study (McKinnon and Love, 2018). In a time of rapid environmental change, it is particularly important to determine factors that drive the within-winter movements of northern animals (Leblond et al., 2016) and the extent of landscape they use.
Variability in resources or access to resources across space and time generally correlates with higher rates of landscape-scale movements of animals (Newton, 2006a,b). At its extreme, unpredictable and patchily-distributed resources can lead migratory birds to exhibit nomadism throughout their life cycle, stopping to breed only when and where resources are most abundant (Stojanovic et al., 2015). Irruptive species exhibit nomadic movements during the non-breeding season in order to track irregular booms in resource abundance outside of their breeding range (Bock and Lepthien, 1976; Smith, 1986; Senar et al., 1992). However, irruptions of northern-breeding species may also be simply a consequence of high productivity in the previous breeding season, and not necessarily that individuals are flexibly tracking resources (Curk et al., 2018).
Weather can also directly impact food availability (e.g., rain/drought affecting arthropod abundance, or covering of seeds by snow or ice) triggering landscape-scale movements (Mittelhauser et al., 2012). Some migratory songbirds may hedge their bets by remaining as far north as possible (in otherwise marginal habitats), and only moving south in the case of extreme disruption of resources (i.e., “fugitive” migration patterns) (Terrill and Ohmart, 1984; Watts et al., 2018). Unfortunately, little is known about exactly how and why variability in weather parameters (i.e., temperature, snow cover, humidity, wind) trigger movement in wintering songbirds (Macdonald et al., 2016; Laplante et al., 2019).
A number of non-resource-based hypotheses have also been proposed to explain species-, population- and individual-based variation in winter movement strategies (Catry et al., 2003; Nebel and Ydenberg, 2005; Macdonald et al., 2016). For example, since social dominance hierarchies can affect access to resources among individuals (Ketterson and Nolan, 1979), higher-ranked individuals in flocking species have less to gain by moving since they can maintain priority over resources at a given site. High-ranking individuals may thus adopt a resident strategy relative to transient subordinates, even within the same population or species (Campos et al., 2011; Fudickar et al., 2013). However, these individual-level strategies have been difficult to explore, in part due to the inherent challenges associated with tracking species during the winter (McKinnon and Love, 2018).
Here, we investigate the within-winter, spatio-temporal dynamics of Snow Buntings (Plectrophenax nivalis), an Arctic-breeding migratory songbird, using automated radio-telemetry and a long-term banding dataset. Snow Buntings are long-distance migrants, traveling between breeding sites in the Arctic and wintering sites south of the boreal forest (Macdonald et al., 2012). Overall Snow Buntings show a complex migration pattern, with a migratory divide in North America at Hudson Bay (Figure 1A; Macdonald et al., 2012), and another in Greenland, where western and southeastern breeding birds migrate to eastern North America, and northeastern-breeding birds migrate to the Russian steppes (Lyngs, 2003). There are subspecies of Snow Buntings that are year-round residents in Alaska, USA, and Iceland, respectively (Montgomerie and Lyon, 2011).
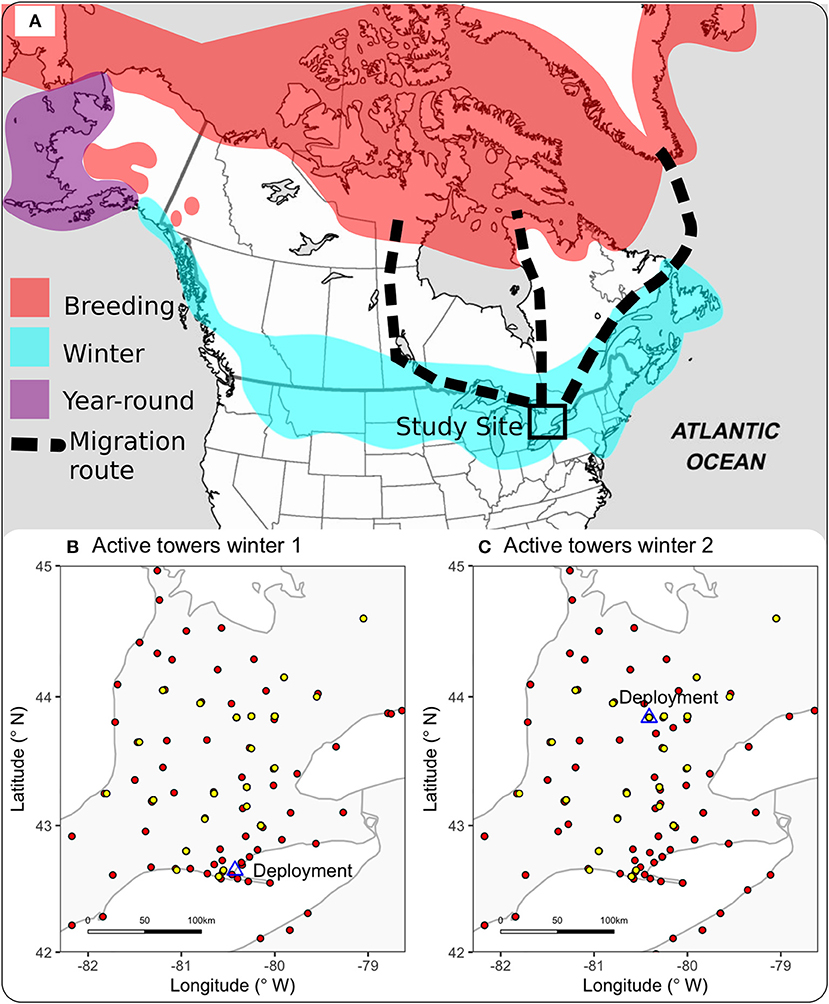
Figure 1. North American range of Snow Bunting and study site. (A) Breeding grounds, wintering sites and year-round resident sites are indicated by color (range based on Montgomerie and Lyon, 2011); most birds in our study population likely breed in Greenland (based on band recoveries; summarized in Macdonald et al., 2012); however, it is possible that some individuals breed in the Canadian Arctic, thus three potential spring flyways are shown. (B,C) Show the ~300 × 300 km region of our study, with active Motus receiving stations as red points, tags deployment sites as blue triangles and stations that detected Snow Buntings as yellow dots.
During the winter, Snow Buntings form flocks and forage in agricultural fields, natural prairie, rocky coasts, and shores of the great lakes in southern Canada and the northern US (Figure 1A; Montgomerie and Lyon, 2011). Vincent and Bédard (1976) described a flock of about 100 individual Snow Buntings occupying a 4 km2 home range from Dec-Mar; although they did not individually mark these birds. In contrast, banded Snow Buntings have been recaptured within the winter over 150 km from initial banding sites, and geolocator tracking suggested large scale movements by individual birds (Macdonald et al., 2012). Overall there is little quantitative information on the scale and drivers of winter movements in Snow Buntings. Here, we combined data from a collaborative network of automated radio-telemetry stations (Motus Wildlife Tracking Network) with a large, single-site winter banding dataset (n = 9,312 individuals captured over 6 years) collected by a participant in a citizen scientist program, to test several hypotheses regarding drivers of within-winter movements in Snow Buntings.
Snow Buntings are granivorous ground-feeders during the winter, thus variability in snow cover results in dynamic availability of food over time and space. Early snowfalls or late spring snow-storms directly affect how much seed is available for foraging buntings. Bird-banders that rely on baited traps to catch Snow Buntings are well-aware that a significant amount of snow is necessary before the birds are attracted to the bait, indicating that the snow prevents birds from accessing “wild” foods (CSBN, pers. comm.).
Snow Buntings also exhibit male-biased sexual size dimorphism and previous work has suggested that larger individuals may have a thermoregulatory advantage in colder weather, losing less heat per unit mass than small individuals (Macdonald et al., 2016; Laplante et al., 2019). Harsh winter weather could thus have a different impact on the energy budgets and movement decisions of males and females, due to differential thermoregulatory constraints among the sexes, potentially also explaining why females carry larger energy reserves per unit size than males (Macdonald et al., 2016; Laplante et al., 2019). Furthermore, since buntings are highly gregarious in winter and exhibit dominance hierarchies within flocks (Smith and Metcalfe, 1997a,b), social hierarchies could also generate differential pressure to move in interaction with weather (Ketterson and Nolan, 1979).
We hypothesized that if winter movements are a flexible response to maximize access to food, then deterioration in weather conditions at a single site should result in changes in flock composition as birds move around the landscape. Differences in structural size and social dominance by sex- and age-classes should result in: (1) larger-sized individuals, (2) males, and (3) younger individuals, forming the main constituents of flocks on days of severe weather (i.e., colder, snowier days). We predicted that birds with these three phenotypes should be able to remain at the site and feed during difficult weather conditions because (1) larger-sized individuals should have better capacity to withstand adverse weather, and because (2) males and younger birds (within a sex) are generally dominant over food resources (Smith and Metcalfe, 1997b). Smaller and subordinate individuals (female, older birds) are also predicted to engage more frequently in winter movements, to compensate for a relatively higher daily energy cost of thermoregulation and reduced access to food.
Methods
Animal Care Statement
All methods followed the Canadian Council for Animal Care recommendations, as reviewed by Environment and Climate Change Canada. Bird banding and handling permission was obtained from the Canadian Bird Banding Office. Tracking and tag-deployment protocols were reviewed and approved by the University of Windsor's Animal Utilization Committee (protocol AUPP # 9-14).
Field Methods—Tracking
We used commercially available cracked corn as bait to capture 40 individual Snow Buntings in custom-made walk-in traps (Love et al., 2012), at two long-term study sites in southern Ontario, Canada in February 2016 (Fergus; 43.838° N, −80.40806° W) and December 2016 (Long Point; 42.5792° N, 80.4309° W). Birds <1 year old were classed as “second-year” (SY) and birds >1 year old as “after-second-year” (ASY) by examining plumage characteristics. We deployed 20 coded radio Nanotags (NTQB-1, Lotek, Inc., attached with a polypropylene string or elastic cord leg-loop harness) each year, and aimed to evenly tag birds from all representative demographic groups (Winter 1: n = 2 ASY males, 8 ASY females, 5 SY males, 5 SY females; Winter 2: n = 8 ASY males, 4 ASY females, 4 SY males, 4 SY females). All 20 birds were tagged on the same day in each winter, and banded (including assessment of age-class and sex) by experienced members of the Canadian Snow Bunting Network (D. Okines and D. Lamble). We tagged birds within their wintering range (Figure 1A) in the center of the Ontario Motus Wildlife Tracking System (Motus, www.motus-wts.org, Taylor et al., 2017), a network of hundreds of receiving stations (Figures 1B,C for location of tagging in Winter 1 and Winter 2). Worldwide, there were 359 Motus stations active for at least part of the tracking period in Winter 1, and 522 stations active for at least part of the tracking period in Winter 2.
We expected that Snow Buntings would be traveling distances in the range of 10–100 km in the winter, based on coarse-scale data obtained previously by tracking using geolocators (Macdonald et al., 2012). We focused on southern Ontario as our main tracking site, which had an area of approximately 300 × 300 km (Figures 1B,C), and contained 69 active stations in Winter 1 and 77 stations active in Winter 2. The Motus Wildlife Tracking System had never before been tested for winter tracking, nor for a ground-foraging bird such as Snow Buntings. We anticipated that the ground-foraging behavior might reduce the number of detections received by stations, for two reasons (Lotek Inc., staff, pers. comm.): (1) the antenna would be in regular contact with the ground or snow, and (2) birds on the ground would be underneath the typical detection range of mounted receiving antennae. Therefore, we expected that most detections would be from birds in flight. Despite the above limitations, the location of the Motus array was ideal: well within the Snow Bunting winter range, and most stations embedded directly in agricultural habitat that Snow Buntings prefer. Southern Ontario is also bordered on three sides by large bodies of water, and Snow Buntings also use lakeshore habitats. In the north, our focal study area is bordered by the boreal forest—which is functionally a migratory barrier to Snow Buntings (Macdonald et al., 2012).
Field Methods—Banding
From 2009 to 2014; Snow Buntings were captured (by D. Lamble) using the same methods at a banding site in Fergus, Ontario (same as deployment site for Nanotags in Year 2; 43.838° N, −80.40806° W, Figure 1C). Winter banding efforts at Fergus occur every day of the winter between 9 a.m. and 2 p.m., starting when birds are first sighted in the area and continuing until they depart. For example, in 2015, D. Lamble banded 7038 Snow Buntings between 2 January and 10 March (note that we only used birds with measurements in analysis, so our sample size was only a fraction of the birds banded). Duration of banding session (hours traps were open) and number of captured individuals varied dependent on weather, presence of predators, and other factors; thus, the total number of captured individuals could not be analyzed as a response variable and we focused instead of characteristics of the birds captured (i.e., sex, age-class, size). Each bird was banded with a uniquely-numbered aluminum band (issued by the Canadian Bird Banding Office), and sex and age-class were determined morphologically according to Canadian Snow Bunting Network guidelines (Love et al., 2012). Unflattened wing chord was measured (for every bird possible) to the nearest 1 mm as an approximation of structural body size; Snow Buntings carry varying fat levels and muscle mass varies seasonally and would confound using weight as a measure of overall size (Macdonald et al., 2016). All individuals were measured by the same bander (D. Lamble). Only individuals for which a complete set of information was available were kept in the dataset (wing chord, sex, age-class).
Weather Data
All weather variables except snow depth were obtained from the Environment and Climate Change Canada (ECCC) weather station nearest to Fergus, Ontario (12.9 km away) for the day of capture for each individual bird. We obtained snow depth data from the National Snow and Ice Data Center (NSIDC) (Brasnett, 1999; Brown and Brasnett, 2010) using a grid cell (24 km x 24 km) that included the banding site in Fergus. In total, we extracted eight daily weather variables, which based on previous work on Snow Buntings and others, are predicted to have an influence on snow bunting behavior (Orlowski and Gebski, 2007; Macdonald et al., 2016; Laplante et al., 2019): minimum temperature (°C), mean temperature (°C), maximum temperature (°C), snow depth (cm), total snowfall (cm), absolute humidity (g/m3), maximum wind gust (km/h), and cloud cover (0–10).
Data Analyses—Tracking
For Motus tracking data, we removed potential false detections (e.g., detections outside the bunting winter range [latitude < 35 N, longitude > −50 W], detections in months outside the lifetime of the tags [May-Nov], run length of detections < 2, frequency standard deviation > 0.1, signal > −30) following protocols recommended by researchers in the Motus community and the Motus RBook (Crewe et al., 2018). We then calculated cumulative minimum distances traveled per individual by using the R package “geosphere” (Hijmans, 2017), summing the Haversine (great-circle) distance between consecutive detections at different receiver stations. We compared the cumulative distance traveled by sex and age-class classes by using a two-factor analysis of variance, with an interaction between sex and age-class. We examined these sex-age-class differences in movements by using the full dataset as well as specifically during the core wintering period of Dec-Feb, as we noted some larger movements in March that could be considered early spring migration.
Data Analyses—Banding
We used banding data collected between November 1st and March 20th for analyses of weather correlates of flock composition. This time period covers the wintering period for Snow Bunting populations within eastern North America (Vincent and Bédard, 1976; Montgomerie and Lyon, 2011; McKinnon et al., 2016). To avoid conflicts with subsequent model selection, models included only banding entries for which a complete set of weather variables was available (i.e., no missing data; final sample size for day of capture dataset, n = 9,312). We tested for the effect of daily weather on 3 response variables: structural size (i.e., wing length), sex, and age-class of birds, by using linear and logistic mixed-effect models with the prediction that structurally large birds, males, and younger (juvenile) birds would be found in the flock on cold/harsh days.
For logistic mixed-effects models for sex and age-class, females and young birds were the reference (dummy) categories. Banding year was included as a random variable in all models. To bring all numerical variables on the same scale, each predictor was standardized as a z-score using the R package “arm” (Gelman et al., 2018). Standardizing predictors was essential to interpret the relative importance of parameter estimates for subsequent model averaging in our analyses (Grueber et al., 2011).
We checked for multicollinearity between weather variables by calculating the Variance Inflation Factor (VIF) for each predictor in the full models using a threshold value of 10 (Quinn and Keough, 2002). One variable (mean temperature) had to be removed due to multicollinearity. Additionally, we calculated the average VIF across predictors to ensure the value was not substantially >1 (Chatterjee and Price, 1991; average VIF = 2.44).
Using the full models, we derived all possible sub-models from each set of predictors. We calculated an Akaike information criterion value (AICc) for each model. Models differed in their AICc only by small amounts, therefore we opted for model averaging (Grueber et al., 2011) using the list of models that fell within Δ AICc < 2 (Burnham and Anderson, 2003), especially as one of our objectives was to predict the relative importance of each variable in explaining variation of the dependent variable. We assessed the importance of each predictor by calculating the sum of the Akaike weights over all the models in the subset of Δ AICc < 2 where each predictor occurred (Burnham et al., 2011). Variables were considered significant when their confidence intervals did not include zero.
Statistical analyses were conducted using R 3.2.1 (R Development Core Team, 2018). The functions “lmer” (linear models) and “glmer” (generalized linear models) (Bates et al., 2014) and lmerTest (Kuznetsova et al., 2014) were used to run mixed-effects models. The function “dredge” and the function “model.avg” in the package “MuMIn” was used for model selection and averaging (Barton, 2019). All coefficients for parameter estimates are reported ± conditional SEM.
Results
Tracking Results
We obtained detections of 25 of the 40 birds outfitted with Nanotags (12 males, 13 females; 10 SY, 15 ASY). Individuals were detected at up to 5 individual Motus stations in Winter 1 and up to 6 Motus stations in Winter 2 (Figure 2). Signals were received by stations only during the day time, with the exception of one bird detected at 21:00 h on 1 April 2016 (Figure 3C; clearly migration). The average distance between sequential detections at different stations was 75.87 ± 16 km (n = 66 detections across all individuals for the whole tracking period). In the core winter period (Dec-Feb), the average distance between sequential detections was 49.27 ± 13.19 km (n = n = 37 detections), while in March and April the distances between detections were on average 109.81 ± 32.36 km (n = 29).
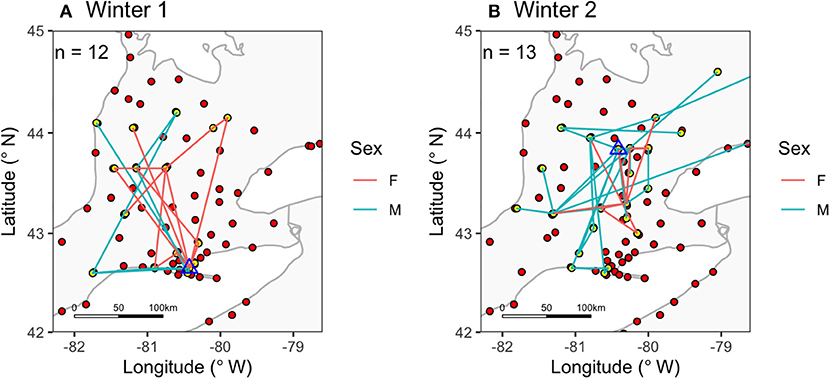
Figure 2. Within-winter movements of snow buntings (A) Winter 1, 2015/2016, and (B) Winter 2, 2016/2017, by using automated radio-telemetry (Motus Wildlife Tracking Network). Red circles show the location of receiver stations and yellow circles show stations where snow buntings were detected; blue triangles indicate deployment sites. Blue (males) and red (females) lines connect detections for individuals.
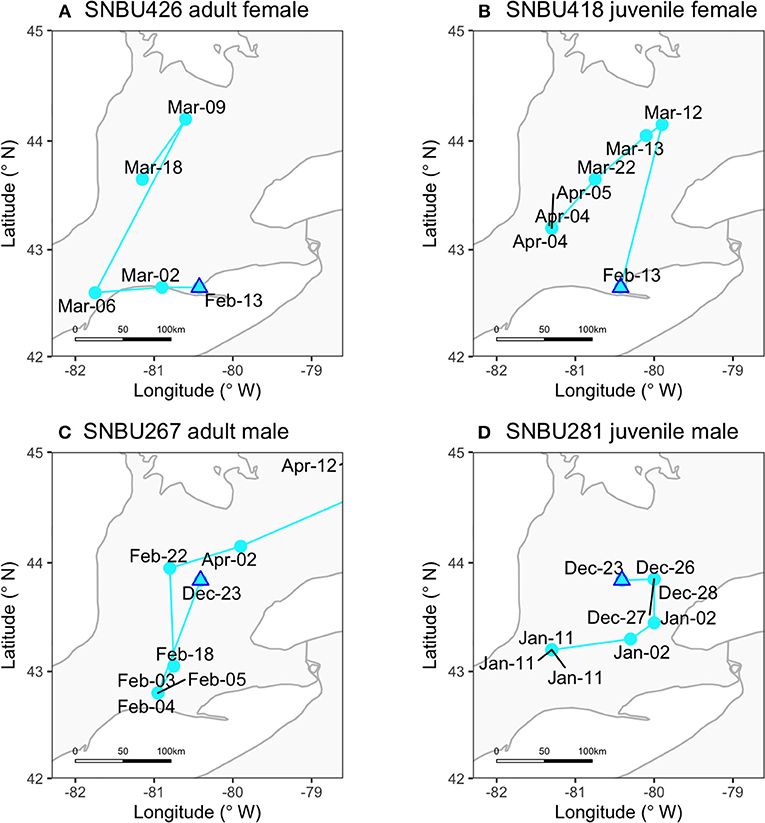
Figure 3. Examples of individual bird movements in the winter. Labels for points indicate detections per hour (i.e., when the same date appears more than once the bird was detected over >1-h period). The females shown here (A,B) were from Winter 1, and the males (C,D) were from Winter 2. “Adult” refers to birds that have had 1 breeding season and “juvenile” to birds that have yet to return to their breeding grounds.
Signals received by the Motus stations were mostly typical of a “fly-by” (Crewe et al., 2018), indicating the individual birds were not remaining within the immediate station area (with some exceptions; see Appendix A—Bird 268 was detected 17 times in 20 days at a landfill station). For example, SY male #274 was first detected on January 4th, with 6 detections from one station in a 1-min period between 9:57 and 9:58 h. This individual was next detected at another station on the 30th of January, with 18 detections between 10:10 and 10:13 h. In total we detected SY male #274 at 4 different stations, each for short bursts, and the bird returned to the vicinity of one station 3 separate times after being detected elsewhere. Since detections at a given station were usually only over a span of a few minutes, in further analysis, we summarized data into hourly detections. For most birds this meant 1 detection per day, for a total of 66 detections for 25 birds.
Throughout the entire tracking period, cumulative distances traveled were longer for males (mean for males: 308.18 km, females: 100.71 km, F(1, 21) = 5.85, P = 0.02), but not significantly different by age-class (SY: 110.16 km, ASY: 260.39 km, F(1, 21) = 2.81, P = 0.10) and the interaction between sex and age-class was not significant (F(1, 21) = 3.14, P = 0.09). We counted the total number of days in the period within which birds were detected (i.e., last detection minus deployment date). Here we found a significant age-class effect (F(1, 21) = 7.52, P = 0.01) and age-sex-interaction (F(1, 21) = 5.35, P = 0.03) that we explored further with a Tukey post-hoc test. Adult males were detected over a significantly longer period (mean for adult males: 75 days) compared to juvenile males (22 days, P = 0.008) and juvenile females (35 days, P = 0.05) but not compared to adult females (40, days P = 0.06). Tracking period was not significantly different by age-class for females (P = 1.00), between juveniles by sex (P = 0.85), or between juvenile males and adult females (P = 0.59).
Because of our smaller sample size within the core Dec-Feb winter period (n = 15 individual birds, only 6 females), we compared sexes by using a t-test. Males traveled a cumulative distance of 152.68 km ± 76 SEM and females 74.79 km ± 20 SEM but the difference was not significant (t = −0.99, df = 9.04, P = 0.35). In Dec-Feb, we documented birds moving as far as 738 km in total (one adult male, who was tracked over almost the entire core Dec-Feb period, and picked up at 5 receiving stations). The average cumulative distance traveled during Dec-Feb was 121 km ± 46 SEM (n = 15 individuals). By March and April, cumulative distances increased to an average of 183.91 km ± 35.64 SEM.
Banding Results: Structural Size
Snow depth, snowfall, cloud cover, and maximum temperatures were the variables that best predicted variation in structural size of birds composing the flock on a given day (Table 1). Wing length of captured birds was longer on days where snow depth was higher (z = 2.52, β = 0.35 ± 0.14), snowfall was greater (z = 3.35, β = 0.24 ± 0.07), and the sky was cloudier (z = 4.82, β = 0.37 ± 0.07). Captured birds were also larger (i.e. longer wings) when maximum ambient temperature was lower (z = −3.93, β = −0.33 ± 0.07).
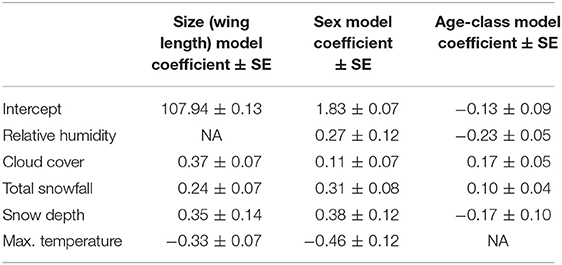
Table 1. Summary of standardized model coefficients for top models (females and juveniles are the reference categories in the sex and age-class models, respectively).
Banding Results: Sex and Age
Snow depth, snowfall, humidity, and maximum temperatures predicted the occurrence of sexes forming the flock at a single wintering site over the long-term (2009–2014; Table 1). Males tended to be captured more often on days where weather was snowier (effect of snow depth on sex: z = 3.34, β = 0.38 ± 0.12; effect of snowfall on sex: z = 3.99, β = 0.31 ± 0.08), more humid (z = 2.29, β = 0.27 ± 0.12) and colder (effect of maximum temperature on sex : z = −4.42, β = −0.46 ± 0.12).
Cloud cover, humidity, snow depth and snowfall were significant predictors of the age-class of birds captured on a given day. ASY (older) birds were more likely to be captured on days when weather was cloudier (z = 3.38, β = 0.17 ± 0.05), snowier (z = 2.32, β = 0.10 ± 0.04), less humid (z = −3.68, β = −0.23 ± 0.05). However, the influence of weather on daily variation in the age-class of birds forming the flock was not as strong as for structural size or sex. In fact, maximum temperature was not present in the final age-class model because it did not appear in the top model set, indicating that it is not a useful predictor of age-class. Furthermore, most variables that were retained in the top age models had relatively low β values (i.e., <0.22).
Discussion
The extent of winter movements has only recently been revealed for many species with the use of miniaturized tracking technology (McKinnon et al., 2013a; McKinnon and Love, 2018), and the ecological and behavioral drivers of these movements remain poorly understood. The data we present here on winter movements of Snow Buntings are an important first step in understanding space-use year-round, which is critical for effective conservation assessment and actions (Marra et al., 2015). We also analyzed weather and patterns of winter movements, by using long-term banding data from a single site. Climate change is affecting high-latitude habitats at a greater rate than other environments in North America (IPCC, 2014), which may pose new challenges to Arctic-breeding, temperate-wintering bird populations (Rodenhouse et al., 2009; Princé and Zuckerberg, 2015; Williams et al., 2015). Understanding flexibility in patterns of movements of Snow Buntings may provide insight into how they could respond to warming temperatures.
Because of the sparse “fly-by” nature of our Motus detections, we were not able to analyze habitat use of buntings, because we could not pinpoint the exact location of the bird. We also could not analyze weather data as a correlate of movements, for several reasons. First, as above, we could not pinpoint the final destination of the bird. Detections indicate that the bird traveled near the station and thus justifies measuring a minimum distance traveled; however, we could not ascertain whether the bird stayed near the Motus receiver in most cases. Further, weather stations are irregularly spaced around southern Ontario, and for many Motus receivers, the nearest source of weather information would be the same, even if the receivers were fairly distant from each other. Finally, we had no control group, i.e., information on birds that did not move, therefore we could not assess whether individual birds were tracking weather or moving for some other reason. Despite the limitations of these tracking data, we were able to quantify for the first time with direct tracking, the extent of Snow Bunting movements across a large area.
Our tracking data indicate that most Snow Buntings use a “nomadic” strategy in winter (Figure 3), moving around an area of several hundred square-kilometers. Space-use of buntings was much larger than winter home-ranges reported for other flocking migratory songbirds (Shizuka et al., 2014; Weinkam et al., 2017). A recent geolocator study of Snow Buntings from a population breeding on Svalbard, Norway, suggested wintering birds were either completely stationary, or traveled to 1–3 separate sites, remaining locally at each place (Snell et al., 2018). Our previous geolocator work also suggested extensive winter movements (Macdonald et al., 2012), although it was difficult to quantify given the error range of geolocators (McKinnon et al., 2013b). Here, we found that 25 of 40 tagged birds moved 20–50 km every few days (see Figure 3 for examples), which fits more clearly with the idea of “nomadic” or “wandering” movements (Rappole et al., 1989) than with birds occupying multiple stationary sites within a large home range. With our banding dataset, we found support for the prediction that birds move in response to changes in daily weather conditions, which in turn correlates with landscape-level demographic patterns of Snow Buntings (Macdonald et al., 2016).
Our results showing non-breeding season movements in the temperate zone are comparable to movements of some songbirds overwintering at tropical latitudes. For example, recent direct-tracking using geolocators has revealed that species expected to be stationary in winter are in fact making relatively large, within-season movements during the non-breeding season (McKinnon et al., 2013a; Stutchbury et al., 2016). In some cases, these movements have been connected to patterns of rainfall associated with resource availability (Heckscher et al., 2015; Thorup et al., 2017). At the local scale, we found that for Snow Buntings, snow depth and temperature correlated with flock composition, suggesting individual birds are “wandering” to new sites when conditions become energetically unfavorable. Eastern Bluebirds (Sialis sialis) wintering in temperate areas diet-switched (from arthropods to fruit) when weather deteriorated, and flock size increased (Weinkam et al., 2017). Snow Buntings do not have the option of diet-switching in winter, as they only consume seeds and grains; selection may instead have favored a strategy of moving on when conditions are poor. However, for Snow Buntings and other species that form large overwintering flocks, the role of predators on flock composition and movements (Nebel and Ydenberg, 2005), intraspecific competition, or other social factors should be considered in future as potential proximate drivers of movement patterns.
The long-term banding data analyses revealed maximum temperatures and snow depth were the most important weather variables correlated with changes in flock composition by sex and size. These results are consistent with our earlier study showing that these variables were also central in informing daily fattening patterns in Snow Buntings (Laplante et al., 2019). Snow depth may rank foremost, considering that snow buntings are granivorous ground-feeders which can have more difficulty accessing resources in areas of high snow depth, and record-high numbers of Snow Buntings have been observed during warmer (less snowy) winters in Central Europe (Orlowski and Gebski, 2007).
Structurally larger individuals and/or male snow buntings were predominantly found in a flock on days of severe weather conditions (i.e., cold, snowy, cloudy, humid). We found a similar pattern in a study of sex ratio in wintering flocks across a broad range of wintering sites, where larger birds of both sexes were found at colder, snowier sites (Macdonald et al., 2016). Large individuals have less body surface area relative to their volume and therefore should loose less heat per unit mass than small individuals, suggesting that on cold days, smaller birds may “wander” until they find a more energetically favorable foraging area.
Movements may also be influenced by male dominance over food resources, where females may be forced to move away when weather is severe because they are simply unable to access food and accumulate the required fat store to survive cold nights. Laplante et al. (2019) reported that for their body size, females were carrying more fat than males. In Snow Buntings wintering in Scotland, females were more likely to move when flock sizes were larger, presumably because they were excluded by dominant individuals when intraspecific competition increased (Smith and Metcalfe, 1997a).
In contrast, our tracking data seemed to suggest that males, and particularly adult males, were moving more often. We detected adult (ASY) male movements over a longer time period, and covering the longest cumulative distances, which suggests these individuals are moving around the landscape more than other age-class-sex groups. Given that stations were 30–40 km apart and the range of each station was up to 20 km, we assume that birds that moved more and over longer distances would be more likely to be detected. Tag detections are biased toward capturing birds in flight, as opposed to foraging on the ground. Fifteen of the 40 tagged birds were never detected after the tag deployment. These birds could have either remained locally at the tagging area until the tag batteries died in late spring, or moved in such a way as to avoid detection by any of the 69–77 active stations within the ~300 × 300 km study area. Alternatively, batteries on the tags could have failed, or the birds been taken by predators or otherwise succumbed. Given the novelty of tracking using these methods during the winter, and on a ground-foraging bird, much remains to be learned about the effective range of the tags and capabilities of the tags and receivers.
Some adult males were clearly departing on spring migration during the tracking period (see individual maps in Appendix), similar to findings of an earlier study of migratory phenology in Snow Buntings that found older males left wintering sites prior to all other demographic groups (McKinnon et al., 2016). However, even within the Dec-Feb period, males (especially older males) tended to move more than females (cumulative distance in Dec-Feb: 190 km for adult males, 84 km adult females, 13 km juvenile males, 37 km juvenile females), although the differences were not significant. If a cold/snowy weather system moves in across a large enough area, it may be that males move into sites previously occupied by more females, which would account for the correlation between weather and sex-ratio (Macdonald et al., 2016), as well as the increased movements we observed from males by direct tracking. Our tracking site in southern Ontario experiences winters that are in general, milder than in other areas of the Snow Bunting wintering range, such as the northern Prairies in Manitoba, Canada, where winter temperatures are regularly below −30°C and where mostly adult (i.e., ASY) males are found (B. Maciejko, pers. comm.). Further direct-tracking or even experimental work in more extreme wintering sites might shed additional insight into flock dynamics and their association with weather.
There was a weak tendency for older (ASY) birds to be found in the flock on days with more snow cover and snowfall (Table 1). Although younger birds have been shown to be dominant in flocks, older birds have improved feeding efficiency (higher peck rate) (Smith and Metcalfe, 1994, 1997b). We also found that younger birds tended to be detected less than adult birds. First-winter birds might have higher mortality rates than experienced adults, which could be an important driver of overall population demographics (Robinson et al., 2004). It could also be that younger birds with less experience tend to remain longer at a given site, thus resulting in few detections at stations away from the initial deployment site. Confidence intervals for most weather variables included zero in the age-class model and the size of variable effects (Beta values) were relatively low, thus we cannot be confident that age-class is an important factor influencing movements in Snow Buntings until further work is conducted.
Conclusion
Factors that drive the nomadic movements of temperate wintering species, as well as the characteristics of those movements, have been difficult to study. We combined local scale flock dynamics with regional tracking to show that weather influences site tenure by sex and structural size, and that movements in the non-breeding season vary by sex and, in some cases, age-class. We showed that Snow Buntings are weather-sensitive and that winter conditions can influence their behavior and distribution. While nomadism seems to be one of the strategies used by birds to respond and adjust to winter conditions, it is unknown whether it could be sustained if winter weather becomes more extreme, as predicted by climate models. Further, experimental work is required to strengthen understanding of the energetic challenges faced by male and female buntings. Improving our understanding in general of the nomadic movements of small species that travel widely remains a frontier of movement ecology studies, one which will continue to be aided by the miniaturization of tracking technology that does not require retrieval for data recovery.
Data Availability
The datasets generated for this study are available on request to the corresponding author.
Ethics Statement
We followed animal care guidelines of the Canadian Council for Animal Care, the Canadian Bird Banding Office regulations (Environment and Climate Change Canada), and the Univ. of Windsor Animal Utilization Project Proposal (AUPP#9-14).
Author Contributions
EM and MP-L: ideas, fieldwork, analysis, interpretation, and writing. OL: ideas, writing, field support, analysis, and interpretation. KF: ideas, writing, analysis, and interpretation. SM: fieldwork, analysis, and interpretation. FV: ideas, field support, writing, analysis, and interpretation.
Funding
Funding and other support was provided by the University of Windsor, the Université du Québec à Rimouski, NSERC, FRQNT, the Canada Research Chairs Program, Bird Studies Canada and the Mitacs Postdoctoral Fellowship Program.
Conflict of Interest Statement
The authors declare that the research was conducted in the absence of any commercial or financial relationships that could be construed as a potential conflict of interest.
Acknowledgments
For field assistance and logistical support, we thank David Lamble, David Okines, the Canadian Snow Bunting Network, Chris Harris, and Matilda Fraser. We also thank Alain Caron and Nicolas Casajus for their help with statistical analyses of banding data.
Supplementary Material
The Supplementary Material for this article can be found online at: https://www.frontiersin.org/articles/10.3389/fevo.2019.00329/full#supplementary-material
References
Barton, K. (2019). Mumin: Multi-Model Inference, Version 1.43.6. Available online at: https://cran.r-project.org/package=MuMIn
Bates, D., Maechler, M., Bolker, B. M., and Walker, S. (2014). lme4: Linear Mixed-Effects Models Using Eigen and S4, Version R Package Version 1.1-7. Available online at: http://cran.r-project.org/package=lme4
Belda, E. J., Barba, E., and Monros, J. S. (2007). Resident and transient dynamics, site fidelity and survival in wintering Blackcaps Sylvia atricapilla: evidence from capture-recapture analyses. Ibis 149, 396–404. doi: 10.1111/j.1474-919X.2007.00657.x
Bock, C. E., and Lepthien, L. W. (1976). Synchronous eruptions of boreal seed-eating birds. Am. Nat. 110, 559–571. doi: 10.1086/283091
Brasnett, B. (1999). A global analysis of snow depth for numerical weather prediction. J. Appl. Meteorol. 38, 726–740. doi: 10.1175/1520-0450(1999)038<0726:AGAOSD>2.0.CO;2
Brown, R. D., and Brasnett, B. (2010). Canadian Meteorological Center (CMC) Daily Snow Depth Analysis Data, Version 1. Boulder, CL: NASA National Snow and Ice Data Center Distributed Active Archive Center.
Burnham, K. P., and Anderson, D. R. (2003). Model Selection and Multimodel Inference: A Practical Information-Theoretic Approach. New York, NY: Springer-Verlag.
Burnham, K. P., Anderson, D. R., and Huyvaert, K. P. (2011). AIC model selection and multimodel inference in behavioral ecology: some background, observations, and comparisons. Behav. Ecol. Sociobiol. 65, 23–35. doi: 10.1007/s00265-010-1029-6
Campos, A. R., Catry, P., Ramos, J., and Robalo, J. (2011). Competition among European Robins Erithacus rubecula in the winter quarters: sex is the best predictor of priority of access to experimental food resources. Ornis Fenn. 88, 226–233. Available online at: https://www.ornisfennica.org/
Catry, P., Catry, I., Catry, T., and Martins, T. (2003). Within and between-year winter-site fidelity of chiffchaffs Phylloscopus collybita. Ardea 91, 213–219. Available online at: http://hdl.handle.net/10400.12/1450
Crewe, T. L., Crysler, A., and Taylor, P. D. (2018). Motus R Book, Version 1.0. Available online at: https://motus.org/MotusRBook/
Curk, T., McDonald, T., Zazelenchuk, D., Weidensaul, S., Brinker, D., Huy, S., et al. (2018). Winter irruptive Snowy Owls (Bubo scandiacus) in North America are not starving. Can. J. Zool. 96, 553–558. doi: 10.1139/cjz-2017-0278
Fudickar, A. M., Schmidt, A., Hau, M., Quetting, M., and Partecke, J. (2013). Female-biased obligate strategies in a partially migratory population. J. Anim. Ecol. 82, 863–871. doi: 10.1111/1365-2656.12052
Gelman, A., Su, Y.-S., Masanao, Y., Zheng, T., and Dorie, V. (2018). arm: Data Analysis Using Regression and Multilevel/Hierarchical Models, Version 1.10-1. Available online at: https://cran.r-project.org/package=arm
Grueber, C. E., Nakagawa, S., Laws, R. J., and Jamieson, I. G. (2011). Multimodel inference in ecology and evolution: challenges and solutions. J. Evol. Biol. 24, 699–711. doi: 10.1111/j.1420-9101.2010.02210.x
Heckscher, C. M., Halley, M. R., and Stampul, P. M. (2015). Intratropical migration of a Nearctic-neotropical migratory songbird (Catharus fuscescens) in South America with implications for migration theory. J. Trop. Ecol. 31, 285–289. doi: 10.1017/S0266467415000024
Hijmans, R. J. (2017). geosphere, Version 1.5-7. Available online at: https://cran.r-project.org/package=geosphere
Ketterson, E. D., and Nolan, V. (1979). Seasonal, annual, and geographic-variation in sex-ratio of wintering populations of Dark-Eyed Juncos (Junco hyemalis). Auk 96, 532–536.
Kuznetsova, A., Brockhoff, P. B., and Christensen, R. H. B. (2014). Package 'lmerTest': Tests in Linear Mixed Effects Models, Version R package Version 2.0-20. Available online at: http://CRAN.R-project.org/package=lmerTest
Laplante, M. P., McKinnon, E. A., Love, O. P., and Vezina, F. (2019). Flexible response to short-term weather in a cold-adapted songbird. J. Avian Biol. 50:e01766. doi: 10.1111/jav.01766
Leblond, M., St-Laurent, M. H., and Côté, S. D. (2016). Caribou, water, and ice - fine-scale movements of a migratory arctic ungulate in the context of climate change. Mov. Ecol. 4:14. doi: 10.1186/s40462-016-0079-4
Love, O. P., Macdonald, C., and McKinnon, E. (2012). Canadian Snow Bunting Banding Network Protocol. doi: 10.6084/m9.figshare.1588581
Lyngs, P. (2003). Migration and wintering ranges of birds in Greenland: an analysis of ringing recoveries. Dans. Ornithol. Foren. Tidsskr. 97, 1–167. Available online at: https://rc.ku.dk/publikationer/traekfugleatlas/Greenland_DOFT_2003_1_1.pdf
Macdonald, C. A., Fraser, K. C., Gilchrist, H. G., Kyser, T. K., Fox, J. W., and Love, O. P. (2012). Strong migratory connectivity in a declining Arctic passerine. Anim. Migr. 1, 23–30. doi: 10.2478/ami-2012-0003
Macdonald, C. A., McKinnon, E. A., Gilchrist, H. G., and Love, O. P. (2016). Cold tolerance, and not earlier arrival on breeding grounds, explains why males winter further north in an Arctic-breeding songbird. J. Avian Biol. 47, 7–15. doi: 10.1111/jav.00689
Marra, P. P., Cohen, E. B., Loss, S. R., Rutter, J. E., and Tonra, C. M. (2015). A call for full annual cycle research in animal ecology. Biol. Lett. 11, 1–4. doi: 10.1098/rsbl.2015.0552
McKinnon, E. A., Fraser, K. C., and Stutchbury, B. J. M. (2013a). New discoveries in landbird migration using geolocators and a flight plan for the future. Auk, 130, 1–12. doi: 10.1525/auk.2013.12226
McKinnon, E. A., and Love, O. P. (2018). Ten years tracking the migrations of small landbirds: lessons learned in the golden age of bio-logging. Auk 135, 834–856. doi: 10.1642/AUK-17-202.1
McKinnon, E. A., Macdonald, C. M., Gilchrist, H. G., and Love, O. P. (2016). Spring and fall migration phenology of an Arctic-breeding passerine. J. Ornithol. 157, 681–693. doi: 10.1007/s10336-016-1333-7
McKinnon, E. A., Stanley, C. Q., Fraser, K. C., MacPherson, M., Casbourn, G., Marra, P. P., et al. (2013b). Estimating geolocator accuracy for a migratory songbird using live ground-truthing in tropical forest. Anim. Migr. 1, 31–38. doi: 10.2478/ami-2013-0001
Mittelhauser, G. H., Tudor, L., and Connery, B. (2012). Within-year movements and site fidelity of Purple Sandpipers during the nonbreeding season. J. Field Ornithol. 83, 32–40. doi: 10.1111/j.1557-9263.2011.00353.x
Montgomerie, R., and Lyon, B. (2011). “Snow bunting (plectrophenax nivalis),” in Birds of North America Online, ed A. Poole (Ithaca: Cornell Lab of Ornithology).
Nebel, S., and Ydenberg, R. C. (2005). Differential predator escape performance contributes to a latitudinal sex ratio cline in a migratory shorebird. Behav. Ecol. Sociobiol. 59, 44–50. doi: 10.1007/s00265-005-0007-x
Newton, I. (2006a). Advances in the study of irruptive migration. Ardea 94, 433–460. Available online at: http://ardea.nou.nu/
Newton, I. (2006b). Movement patterns of common crossbills Loxia curvirostra in europe. Ibis 148, 782–788. doi: 10.1111/j.1474-919X.2006.00585.x
Orlowski, G., and Gebski, P. (2007). Effect of weather change on flock size of the Snow Bunting (Plectrophenax nivalis L.) wintering in south-western Poland. Pol. J. Ecol. 55, 181–185. Available online at: https://miiz.waw.pl/index.php/pl/wydawnictwa/polish-journal-of-ecology
Princé, K., and Zuckerberg, B. (2015). Climate change in our backyards: the reshuffling of North America's winter bird communities. Glob. Chang. Biol. 21, 572–585. doi: 10.1111/gcb.12740
Quinn, G., and Keough, M. (2002). Experimental Design and Data Analysis for Biologists, New York, NY: Cambridge University Press. doi: 10.1017/CBO9780511806384
R Development Core Team (2018) R: A Language and Environment for Statistical Computing, Version 3.1.1. Vienna: R Foundation for Statistical Computing. Available online at http://www.r-project.org/.
Rappole, J. H., Ramos, M. A., and Winker, K. (1989). Wintering wood thrush movements and mortality in southern Veracruz. Auk 106, 402–410.
Robinson, R. A., Green, R. E., Baillie, S. R., Peach, W. J., and Thomson, D. L. (2004). Demographic mechanisms of the population decline of the song thrush Turdus philomelos in Britain. J. Anim. Ecol. 73, 670–682. doi: 10.1111/j.0021-8790.2004.00841.x
Rodenhouse, N. L., Christenson, L. M., Parry, D., and Green, L. E. (2009). Climate change effects on native fauna of northeastern forests. Can. J. For. Res. 39, 249–263. doi: 10.1139/X08-160
Senar, J. C., Camerino, M., and Metcalfe, N. B. (1992). Fighting as a subordinate in finch flocks - escalation is effective but risky. Anim. Behav. 43, 862–864. doi: 10.1016/S0003-3472(05)80210-6
Shizuka, D., Chaine, A. S., Anderson, J., Johnson, O., Laursen, I. M., and Lyon, B. E. (2014). Across-year social stability shapes network structure in wintering migrant sparrows. Ecol. Lett. 17, 998–1007. doi: 10.1111/ele.12304
Smith, K. G. (1986). Winter population-dynamics of 3 species of mast-eating birds in the eastern United States. Wilson Bull. 98, 407–418.
Smith, R. D., and Metcalfe, N. B. (1994). Age, sex and prior site experience have independent effects on the foraging success of wintering Snow Buntings. Behaviour 129, 99–111. doi: 10.1163/156853994X00370
Smith, R. D., and Metcalfe, N. B. (1997a). Where and when to feed: sex and experience affect access to food in wintering snow buntings. Behaviour 134, 143–160. doi: 10.1163/156853997X00322
Smith, R. D., and Metcalfe, N. B. (1997b). Why does dominance decline with age in wintering Snow Buntings? Anim. Behav. 53, 313–322. doi: 10.1006/anbe.1996.0403
Snell, K. R. S., Stokke, B. G., Moksnes, A., Thorup, K., and Fossøy, F. (2018). From Svalbard to Siberia: passerines breeding in the high arctic also endure the extreme cold of the Western Steppe. PLoS ONE 13:e0202114. doi: 10.1371/journal.pone.0202114
Stearns, S. C. (1976). Life-history tactics - review of ideas. Q. Rev. Biol. 51, 3–47. doi: 10.1086/409052
Stojanovic, D., Terauds, A., Westgate, M. J., Webb, M. H., Roshier, D. A., and Heinsohn, R. (2015). Exploiting the richest patch has a fitness pay-off for the migratory swift parrot. J. Anim. Ecol. 84, 1194–1201. doi: 10.1111/1365-2656.12375
Stutchbury, B. J., Siddiqui, R., Applegate, K., Hvenegaard, G. T., Mammenga, P., Mickle, N., et al. (2016). Ecological causes and consequences of intratropical migration in temperate-breeding migratory birds. Am. Nat. 188, S28–S40. doi: 10.1086/687531
Taylor, P. D., Tara, L. C, Mackenzie, S. A., Lepage, D., Aubry, Y., Crysler, Z., et al. (2017). The Motus Wildlife Tracking System: a collaborative research network to enhance the understanding of wildlife movement. Avian Conserv. Ecol. 12:8. doi: 10.5751/ACE-00953-120108
Terrill, S. B., and Ohmart, R. D. (1984). Facultative extension of rall migration by Yellow-Rumped Warblers (Dendroica coronata). Auk 101, 427–438.
Thorup, K., Tøttrup, A. P., Willemoes, M., Klaassen, R. H., Strandberg, R., Vega, M. L., et al. (2017). Resource tracking within and across continents in long-distance bird migrants. Sci. Adv. 3:e1601360. doi: 10.1126/sciadv.1601360
Vincent, J., and Bédard, J. (1976). Fat reserves in snow buntings. Can. J. Zool. 54, 1051–1063. doi: 10.1139/z76-119
Watts, H. E., Cornelius, J. M., Fudickar, A. M., Pérez, J., and Ramenofsky, M. (2018). Understanding variation in migratory movements: a mechanistic approach. Gen. Comp. Endocrinol. 256, 112–122. doi: 10.1016/j.ygcen.2017.07.027
Weinkam, T. J., Janos, G. A., and Brown, D. R. (2017). Habitat use and foraging behavior of Eastern Bluebirds (Sialia sialis) in relation to winter weather. Northeast. Nat. 24, B1–B18. doi: 10.1656/045.024.s704
Keywords: nomadic migration, irruptive migration, differential migration, telemetry, songbird, movement ecology, weather
Citation: Mckinnon EA, Laplante M-P, Love OP, Fraser KC, Mackenzie S and Vézina F (2019) Tracking Landscape-Scale Movements of Snow Buntings and Weather-Driven Changes in Flock Composition During the Temperate Winter. Front. Ecol. Evol. 7:329. doi: 10.3389/fevo.2019.00329
Received: 30 April 2019; Accepted: 16 August 2019;
Published: 03 September 2019.
Edited by:
Brett K. Sandercock, Norwegian Institute for Nature Research (NINA), NorwayReviewed by:
Davide Scridel, Museo delle Scienze, ItalySissel Sjöberg, Lund University, Sweden
David Douglas, Royal Society for the Protection of Birds (RSPB), United Kingdom
Copyright © 2019 Mckinnon, Laplante, Love, Fraser, Mackenzie and Vézina. This is an open-access article distributed under the terms of the Creative Commons Attribution License (CC BY). The use, distribution or reproduction in other forums is permitted, provided the original author(s) and the copyright owner(s) are credited and that the original publication in this journal is cited, in accordance with accepted academic practice. No use, distribution or reproduction is permitted which does not comply with these terms.
*Correspondence: Emily A. Mckinnon, ZW1pbHkubWNraW5ub25AdW1hbml0b2JhLmNh