- 1Nicholas School of the Environment, Duke University, Durham, NC, United States
- 2Agence Nationale des Parcs Nationaux, Libreville, Gabon
- 3Institut de Recherche en Ecologie Tropicale, Libreville, Gabon
- 4African Forest Ecology Group, University of Stirling, Stirling, United Kingdom
As a keystone megafaunal species, African forest elephants (Loxodonta cyclotis) influence the structure and composition of tropical forests. Determining the links between food resources, environmental conditions and elephant movement behavior is crucial to understanding their habitat requirements and their effects on the ecosystem, particularly in the face of poaching and global change. We investigate whether fruit abundance or climate most strongly influence forest elephant movement behavior at the landscape scale in Gabon. Trained teams of “elephant trackers” performed daily fruit availability and dietary composition surveys over a year within two relatively pristine and intact protected areas. With data from 100 in-depth field follows of 28 satellite-collared elephants and remotely sensed environmental layers, we use linear mixed-effects models to assess the effects of sites, seasons, focal elephant identification, elephant diet, and fruit availability on elephant movement behavior at monthly and 3-day time scales. At the month-level, rainfall, and to a lesser extent fruit availability, most strongly predicted the proportion of time elephants spent in long, directionally persistent movements. Thus, even elephants in moist tropical rainforests show seasonal behavioral phenotypes linked to rainfall. At the follow-level (2–4 day intervals), relative support for both rainfall and fruit availability decreased markedly, suggesting that at finer spatial scales forest elephants make foraging decisions largely based on other factors not directly assessed here. Focal elephant identity explained the majority of the variance in the data, and there was strong support for interindividual variation in behavioral responses to rainfall. Taken together, this highlights the importance of approaches which follow individuals through space and time. The links between climate, resource availability and movement behavior provide important insights into the behavioral ecology of forest elephants that can contribute to understanding their role as seed dispersers, improving management of populations, and informing development of solutions to human-elephant conflict.
Introduction
Understanding the environmental factors that influence animal movements is fundamental to theoretical and applied research in the field of movement ecology (Nathan et al., 2008). Studies relating fine-scale movement paths to spatiotemporally structured landscape data can shed light on the determinants of movement, such as resource availability and the relative importance of different resources (Owen-Smith et al., 2010). The relationship between landscape dynamics and movement is particularly important to wide-ranging species, like elephants, whose mobility can be critical for persistence in the face of high temporal variability of local food resources (Hebblewhite and Merrill, 2008).
The African forest elephant (Loxodonta cyclotis) is a wide-ranging megaherbivore and keystone species for Afrotropical rainforests that influences the composition and structure of the ecosystem through movement and browsing (Turkalo and Fay, 2001; Blake, 2002; Poulsen et al., 2018; Cardoso et al., 2019). Forest elephants can move over 2800 km annually with average home ranges of ∼700 km2 (Mills et al., 2018). Like savanna elephants (Loxodonta africana), forest elephants show patterned preferences for various habitats, potentially moving to fulfill their energy and nutrition needs (Sach et al., 2019). The ranging behavior of forest elephants has been linked to resources such as browse abundance, mineral deposits, water resources, and the physical and seasonal distribution of ripe fruit (Blake, 2002; Blake et al., 2006; Buij et al., 2007; Mills et al., 2018), but the relative importance of these resources on their movements is largely unknown.
Although forest elephants have broad diets, they are highly frugivorous and foraging decisions likely track fruit resources (White, 1994b; Theuerkauf et al., 2000; Blake et al., 2009). The distribution of forest elephant trails, for example, is associated with high-nutrient food sources such as fruit and mineral deposits (Blake et al., 2006) and elephant trails frequently lead to important fruiting trees (Short, 1983; White, 1992). Although rainforests are often viewed as lush, productive ecosystems, ripe fruit is often patchily distributed and seasonally scarce (Wallace, 2005; Janmaat et al., 2014; Adamescu et al., 2018). Consistent with this, Central African forests demonstrate distinct fruiting fluctuations, linked with both annual and seasonal patterns (Tutin et al., 1993; White, 1994a; Takenoshita et al., 2008; Adamescu et al., 2018). Peak fruit production consistently falls within the wettest months of the year across geographic locations, particularly for fleshy fruits (Frankie et al., 1974; Chapman et al., 1999, 2005; van Schaik et al., 2003), at least in the forest interior (Cardoso et al., 2019). Studies of other frugivores (e.g., primates) have highlighted that the spatial distribution and temporal periodicity of flowering and fruiting often drive their ranging behavior and habitat use (Heithaus et al., 1975; Wallace, 2006), and that frugivores can show marked seasonal dietary shifts by switching to alternative diets when particular energy resources are scarce (Wallace, 2005, 2006). These dietary shifts lead to behavioral shifts and changes in activity budgets, including when, where, and how individuals move (Peres, 1994; Wallace, 2006).
Despite high rainfall in tropical forests, forest elephants, like savannah elephants, might also be limited by water (Loarie et al., 2009; Mills et al., 2018). As large-bodied mammals living in a tropical climate, forest elephants likely use a substantial amount of water in thermoregulation (Dunkin et al., 2013) and employ similar range of physiological and behavioral mechanisms as their savannah counterparts (e.g., Mole et al., 2016; Thaker et al., 2019). Moreover, proximity to wetlands often predicts elephant presence, and seasonal habitat occupation has been more closely linked with permanent wetlands than fruit availability (Buij et al., 2007). In Congo, forest elephants are hypothesized to have two distinct modes of ranging with dry season movements concentrated around rivers or swamps and wet season movements tracking the irregular distribution of fruit (Blake, 2002). Amongst these key environmental factors, the degree to which water availability, temperature and resource availability interact to shape forest elephant movement behavior is yet to be explored at the landscape scale.
Here we address the scarcity of information on the factors driving forest elephant movement behavior by asking: (i) What is the level of temporal and/or spatial variation in site-level availability and diversity of elephant-consumed fruits?; (ii) To what extent does variation in site-level fruit availability influence elephant diet?; (iii) What is the relative importance of fruit availability and/or climatic factors (e.g., temperature and rainfall) in explaining temporal variation in elephant movement behaviors (ratio of exploratory to encamped behavior) at two different temporal scales (monthly and follow-level); and (iv) Is there evidence for inter-individual differences in the responsiveness of elephants to the drivers of movement? We address these questions using a unique dataset obtained through over 2500 observer-hours of follows of 28 elephants of known identity in two national parks in Gabon. Most of the data was collected in relatively pristine locations, which are not highly fragmented and remain largely undisturbed by anthropogenic influences. As such, the results represent natural factors influencing elephant behavior.
Materials and Methods
Study Areas
We followed satellite collared forest elephants within and around the Ivindo National Park and the Wonga Wongué Presidential Reserve, from February 2017 to May 2018 (Figure 1). Located in northeastern Gabon, Ivindo National Park is 2990 km2, comprised of primary and secondary lowland forest, swamps, and a series of baïs (forest clearings containing water which are maintained by animal activity). The region has 160 species of trees, with primary forest having the highest species richness (Sassen and Wan, 2006). In coastal Gabon, Wonga Wongué covers 4282 km2, comprised of beaches, mangrove wetlands, tropical savanna and inland forest. The savanna-forest matrix is 85% lowland forest and 15% savanna (Mills et al., 2018).
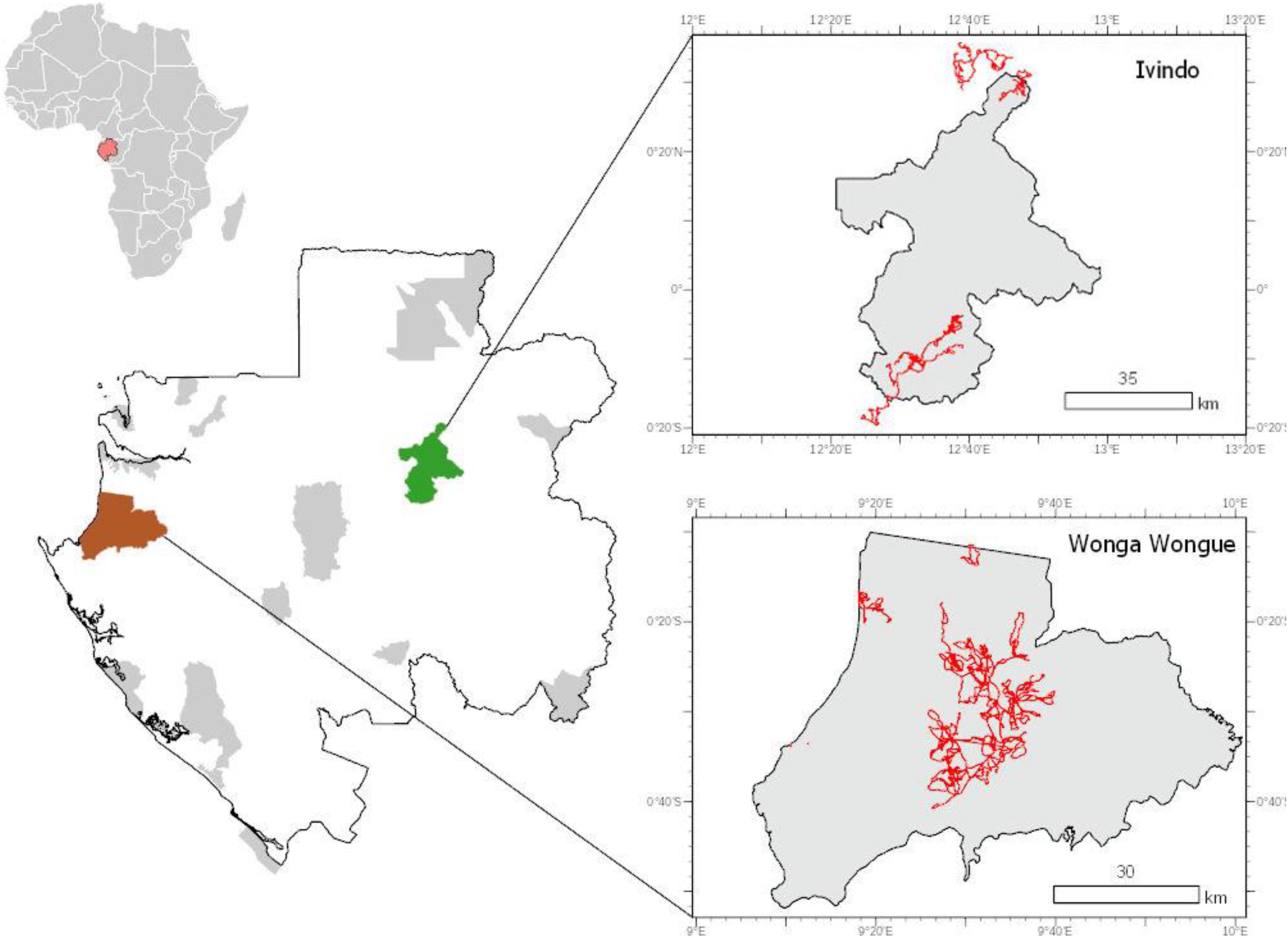
Figure 1. The location of study sites and distribution of follow effort within Gabon, central Africa. The top-left inset shows the location of Gabon (red) in Africa; the left hand panel shows the two focal protected areas (green = Ivindo; orange = Wonga Wongué); and the right hand panel shows the tracks of research teams during focal elephant follows (red lines) within and around each protected area (gray polygons).
GPS Collar Data
The Gabonese National Park Agency (ANPN) initiated a forest elephant collaring program in October 2015. To date, 96 elephants have been collared with Inmarsat satellite GPS devices, fabricated by Africa Wildlife Tracking, and set to record hourly location data. For full collaring procedures see Mills et al. (2018). This study analyses data from a subset of 28 individuals for which we also conducted complimentary “focal follows” (described below) between February 16th, 2017 and May 25th, 2018. The average number of GPS locations per elephant during this period was 16,674 (min = 9,576; max = 24,436; the variation being driven by several focal elephants being collared during the study period). The mean interval between successive GPS locations was 65.6 min, representing a fix success rate of ∼92%. Location data were explored for impossible fix locations or movement speeds between successive points (e.g., movement speeds >10 km–1h–1), however, none were found. Focal elephants consisted of 14 male and 14 female adults, although their exact ages are unknown. All focal elephants spent the vast majority of their time apart from other collared elephants (>200 m from another collared individual for 99.5% of fixes, with an average separation distance of 9.5 km), thus we consider them to be ecologically and statistically independent units. Focal elephants may have associated with other non-collared elephants during the study period, research conducted elsewhere in Gabon suggests that mean group size is between 1.8 and 2.2 individuals (Morgan and Lee, 2007). However, it was not possible to directly determine the extent and duration which such events occurred in this case as observations of the focal individuals were extremely rare in forested environments.
Field Data
During focal follows (n = 100), a field research team tracked an elephant on foot for 3 days (mode = 3 days; mean = 2.8 days; sd = 0.6 days). We used the hourly GPS locations and assistance from local BaAka elephant trackers to best follow the focal elephants “true” path, and to minimize the probability of sampling dung from non-focal individuals. Occasionally follows had to be abandoned early due to weather or logistical constraints (n = 22). To reduce the possibility of disturbing the focal animal and to ensure the safety of the field team, follows were conducted a minimum of 1 h behind the focal elephant. As it was not logistically possible to follow elephants continuously during the focal period, each day of a focal follow started at a different GPS collar location to ensure follow coverage across the entirety of an elephant’s daily activity cycle. For example, during a 3-day follow sampling would start at the temporal GPS locations of 13:00 h, 20:00 h and 6:00 h. As a rule, follows would start at a location no sooner than 1 h and no later than 24 h from the focal elephant’s last known location.
To characterize elephant diet, during elephant follows we searched every third fresh dung to determine its composition. Only fresh dung was searched to minimize the possibility that it came from a non-focal elephant. The relative abundance of fiber, leaf fragments, fruit and seeds was quantified on a 4-point scale following White et al. (1993) and Blake et al. (2009); we counted and identified all seeds greater than 1 cm to species or morpho-species.
To quantify fruit availability, we conducted “fruit-walks” twice a day, at the start of each daily follow (see above) and at the last waypoint visited at the end of the follow: if the field team moved less than 100 m total only one fruit walk was conducted. Assessing fruit availability at the first and last waypoints provided randomized temporal coverage depending on how far the field team moved each day. On each fruit walk, all fallen fleshy fruits encountered on a 2 × 50 m transect were counted and identified to species, or morpho-species when the species was unknown (with all fruits of a morpho-species given the same unique code, msp#). Each transect ran perpendicular to the elephant’s trail, or if no trail was present, perpendicular to the cardinal direction to the next GPS point. We further categorized fruits as mature or immature; ripe or unripe; and intact, chewed or partial.
Elephant Movement Behavior
We used hidden Markov chain models to infer latent behavioral states from the GPS collar location data of all focal elephants using the “momentuHMM” package (Mcclintock et al., 2018) in R. Hidden Markov models use time series analysis to classify movement tracks into behavioral modes – they account for the high degree of autocorrelation in telemetry data and are robust when observational error is low (as in this case). A gamma distribution was used to describe the step lengths, a von Mises distribution described the turning angles, and the Viterbi algorithm was used to estimate the most likely sequence of movement states to have generated the observations (as in Mcclintock et al., 2018). Each movement was either characterized as “encamped” (small movements with high turning angles – resting or foraging) or “exploratory” (longer movements with directional persistence – movement between patches or habitats). The amount of time each focal elephant spent in each behavioral category was summed, then divided by the total length of the time step in question (monthly or follow duration) to get the proportion of time spent in a given behavioral state.
Remotely Sensed Covariates
We used remote sensing to characterize climatic variables thought to influence elephant movement. Temperature data were derived from daily MODIS Aqua Satellite land surface temperature at 1 km2 resolution from LP DAAC via Google Earth Engine (Schwantes and Nuñez, 2019). Precipitation data were derived from the CHIRPS database (Funk et al., 2015) at 0.05° resolution (5.6 km at the equator). The CHIRPS dataset models precipitation from infrared and validates it with fixed rain stations. Both datasets were averaged across each park to create a daily mean. All data of insufficient quality were removed from analysis. Wet and dry seasons were derived from transitions in slope of the anomalous accumulation of pentad means of rainfall started at the beginning of the year from park daily means (Liebmann et al., 2007) – see Figures 2A,B for graphical representations.
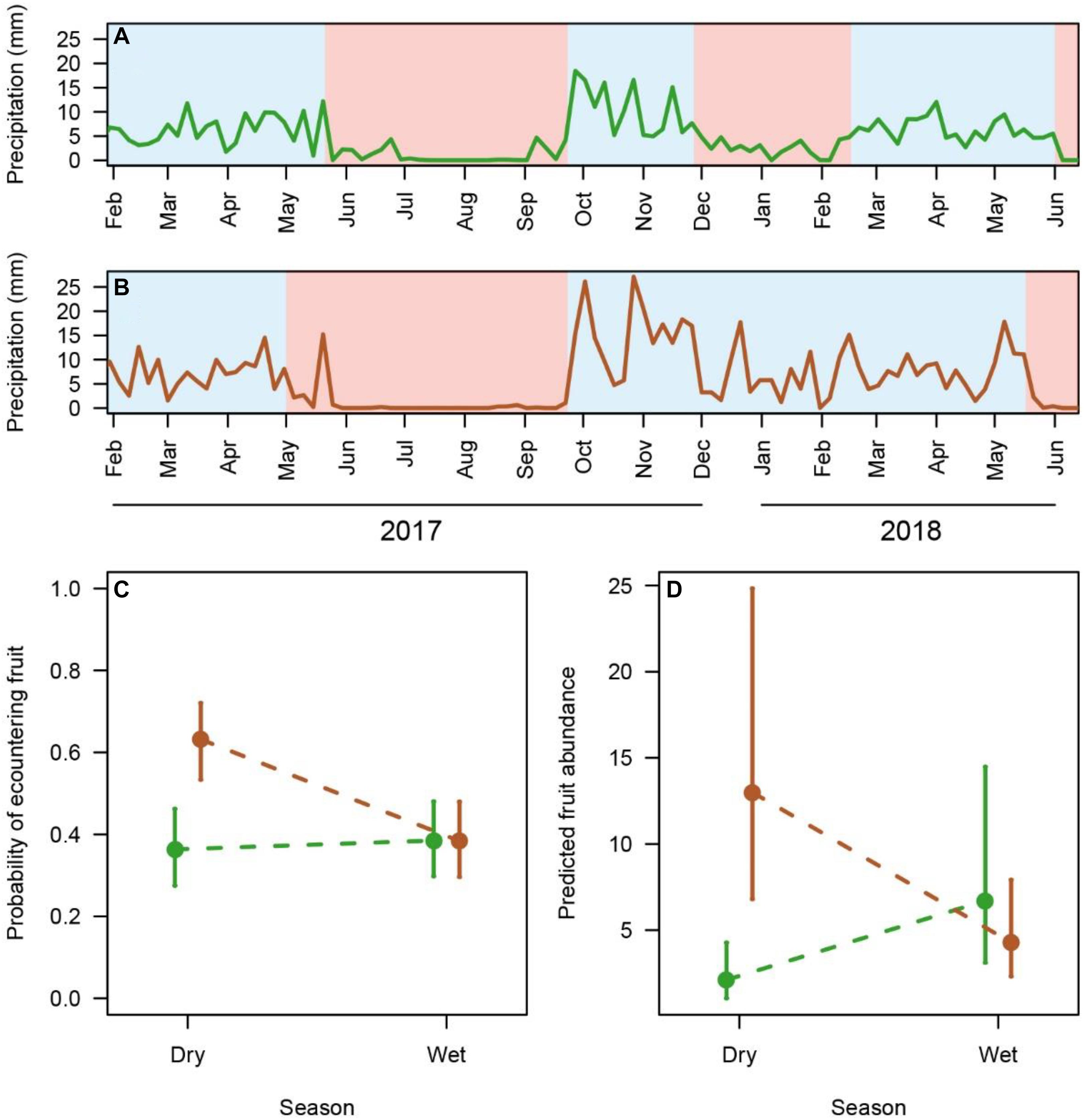
Figure 2. Pentad mean daily rainfall trends for Ivindo (A) and Wonga Wongué (B) and predicted fruit encounter probability (C), and fruit abundance (D) from the best supported models across sites and seasons. Where: green = Ivindo; brown = Wonga Wongué; blue sections (A,B) = dates assigned as wet seasons; pink sections (A,B) = dates assigned as dry season; points (C,D) = estimated fruit presence or abundance from the best supported model; bars = 95% confidence intervals around the fixed effects.
Statistical Analysis
To investigate the ecological and environmental drivers influencing elephant movement behavior, we employed a four step approach: Step 1 – we assessed the degree of spatiotemporal variation in availability and diversity of elephant consumed fruits; Step 2 – we explored how fruit availability influenced forest elephant diet; Step 3 – we determined how fruit availability and climate factors (e.g., temperature and rainfall) affected variation in elephant movement behavior; and Step 4 – we assessed the evidence for individual-level sensitivity to fruit availability and climatic covariates. All analyses were conducted using the glmmTMB package (Brooks et al., 2017) in the R statistical environment 3.6.2 (R Core Team, 2013).
For each step, we defined a suite of candidate models containing all combinations of our explanatory variables (defined below). The candidate models were ranked by their Akaike Information Criterion corrected for small sample size (AICc) and all models with a ΔAICc ≤ 6 from the best supported model were included in the “top model set.” To avoid over interpretation of spurious candidate variables, we removed all models from the “top model set” which had a simpler nested-model with more relative support (Richards et al., 2011; Harrison et al., 2018). Informative covariates are discussed in terms of their relative support, defined here as the sum of Akaike weights (Aw) across all of the “top-set” models in which the covariate occurs, and in terms of their absolute importance, defined here as their standardized effect-size on the response term in each step (Galipaud et al., 2017). We selected the top model in each step and calculated the variance explained (R2) by both fixed and random effects (Nakagawa and Schielzeth, 2013).
Step 1: What Is the Level of Temporal and/or Spatial Variation in the Site-Level Availability and Diversity of Elephant Consumed Fruits?
To characterize fruit availability, we evaluated fruit abundance and diversity. To account for zero inflation in the fruit abundance data, we employed a hurdle model. We first modeled the probability of encountering fruit on a fruit-walk. Then, given that fruit were encountered, we modeled the abundance of fruit (number of fruits observed per fruit-walk). Candidate models (n = 5) included all combinations of site, season and their interaction term. To account for the temporal non-independence of fruit-walks conducted on the same follow, the follow identity was included as a random intercept. To compare fruit diversity across sites and seasons with different levels of survey effort, we used the iNEXT package (Hsieh et al., 2016) to extrapolate diversity to 135 fruit-walks. Sites were deemed to have statistically significant differences in species richness estimates if the 95% confidence intervals did not overlap. Community structure was compared using rank-abundance (Whittaker) plots across sites and seasons with the vegan package (Oksanen et al., 2011). Rank-abundance plots compare the evenness of a community whereby shallow curves represent a community of many species of similar abundance and steep curves represent a skewed assemblage with one or more species in substantially higher relative abundance than the others.
Step 2: To What Extent Does Variation in Site-Level Fruit Availability Influence Elephant Diet?
To determine if estimates of fruit availability had a measurable impact on forest elephant diet, we modeled the proportional seed composition (ratio of seeds to fiber) of elephant dung as a function of site, average fruit availability in a given month and their interaction. Five candidate models were created from these combinations of variables including a null, intercept only model. To account for temporal non-independence of dungs encountered on the same follow and for repeat follows of the same elephant through time, we included the identities of the focal follow and focal elephant as random intercepts. We used the iNEXT package and rank-abundance plots, as above, to compare dietary composition between sites and seasons, although in this instance species richness was extrapolated to 150 sampling units (the maximum number of dung piles searched at a site in a given season).
Step 3: What Is the Relative Importance of Fruit Availability and/or Climatic Factors (e.g., Temperature and Rainfall) in Explaining Temporal Variation in Elephant Movement Behaviors?
To determine the relative importance of fruit availability and/or climate factors on movement behavior, we examined how the monthly ratio of encamped behavior to directed movement varied as a function of site, monthly mean fruit availability (average number of fruits encountered on transects in a given month), monthly mean precipitation, and monthly mean temperature. Candidate models included all combinations of fruit availability, precipitation, and temperature (n = 8) as the predictors were not strongly correlated (Appendix 1). To account for individual behavioral differences and spatial heterogeneity, we included focal elephant identity and site as random intercepts.
We repeated the analysis above for elephant movement at the follow-level to examine if the factors driving monthly movement patterns had consistent effects at finer temporal scales. In this instance, fruit availability represents the average of all fruit-walks performed on a follow, rainfall represents average daily rainfall across a follow, and temperature represents the 2-week averaged temperature for a follow.
Step 4: Is There Evidence for Inter-Individual Differences in the Responsiveness to the Drivers of Elephant Movement?
To explore plasticity in the responses of elephants to resource availability, we assessed the strength of evidence for individual-variation in random effect slopes (Dingemanse and Dochtermann, 2013). Using the best supported monthly model from Step 3 as the null model, we used AICc to determine if the addition of a random slope term for each of the putative covariates increased the amount of information explained by the model.
Results
We conducted 100 elephant follows on 28 focal individuals, representing a total of 278 follow-days. The mean number of follows per elephant was 3.5 (min = 1; max = 9). The elephant follows were evenly distributed throughout the calendar year, with a median of 8 follows per month (min = 3; max = 14) and only 1 month with less than six follows (August).
(i) What Is the Level of Temporal and/or Spatial Variation in the Site-Level Availability and Diversity of Elephant Consumed Fruits?
A total of 499 fruit walks were conducted across both sites (Ivindo = 253, Wonga Wongué = 246), with a 44% chance of encountering fruit on a transect. There was strong evidence for a site × season interaction (Table 1): the probability of encountering fruits in Ivindo was higher in the wet season and lower in the dry season; whereas in Wonga Wongué, it was lower in the wet season and higher in the dry season (Figure 2C). Fixed effects explained 6% of the variance in encountering fruit and the random effect (follow ID) explained 3%. There was also some support for a site × season interaction in the number of fruits conditional upon fruit being present (Table 2 and Figure 2D), but the relative strength of support for the interaction between sites relative to the null model decreased (Table 2). In the fruit abundance model, fixed effects explained 27% of the variance and the random effect (follow ID) explained 61%.
The wet seasons in Ivindo and Wonga Wongué and the dry season in Ivindo had similar estimated fruit species richness (26, 19, and 25 species), whereas just six species were encountered in Wonga Wongué in the dry season (Figures 3A,B). Rank-abundance plots show diverse and relatively even community composition of fruit in Ivindo year-round and in Wonga Wongué in the wet season, whereas the dry season assemblage in Wonga Wongué is skewed due to an extremely high abundance of Sacoglottis gabonensis (Figure 3C). Sacoglottis gabonensis is also the most abundant fruit species in the wet season in Wonga Wongué, but other fruits are also highly available.
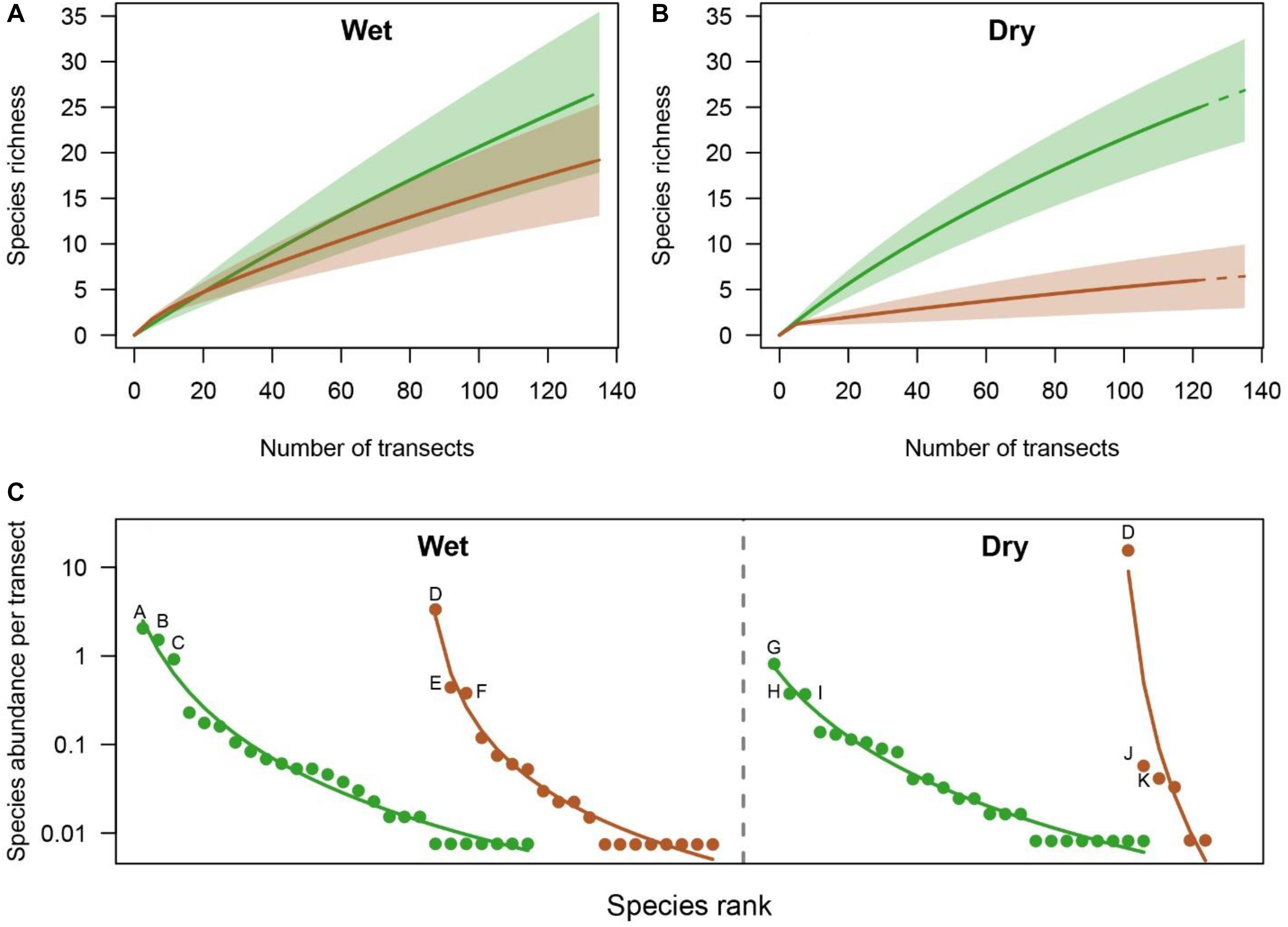
Figure 3. Sample-based species accumulation curves (A,B) and rank-abundance plots (C) for fruits found on transect walks by site (green = Ivindo; brown = Wonga Wongué) and season. In (A,B): lines denote the mean species richness for each site and shaded areas represent 95% confidence intervals. In (C): points = species specific abundances; lines = fitted values from a mandlebrot model of species abundances; letters = the three most abundant fruit species in each site by season (A = Ivindo msp16; B = Tetrapleura tetraptera; C = Nauclea diderrichii; D = Sacoglottis gabonensis; E = Pachypodanthium staudtii; F = Uapaca guineensis G = Dacryodes buettneri; H = Irvingia robur; I = Ivindo msp2; J = Wonga Wongué msp2; K = Batunga spp.).
(ii) To What Extent Does Variation in Fruit Availability Influence Elephant Diet?
We examined 820 dungs, evenly split between Ivindo and Wonga Wongué. Across both sites, seeds constituted 18.3% (sd = 13.2) of dung. The proportion of seeds in dung increased with increasing fruit availability on transects (Table 3 and Figure 4), with a 64% increase from low to high fruit availability. There was weak support for site-specific seed proportions (Table 3): elephants in Wonga Wongué had, on average, a slightly higher proportion of seed than elephants in Ivindo (22% and 19%). There was no support for a site × fruit availability interaction on the proportion of seeds in dung. Fixed effects explained 6% of the variation in the proportion of seeds in dung, and the random effects explained 40% of the variation (100% explained by elephant identity and none by follow identity). Rank-abundance plots of the composition of seeds in dung revealed qualitatively similar trends to those observed in fruit availability: species richness and community composition are similar in both wet and dry seasons in Ivindo, but species richness is substantially lower in Wonga Wongué and community composition is markedly skewed in the dry season compared to the wet season (see Appendix 2).
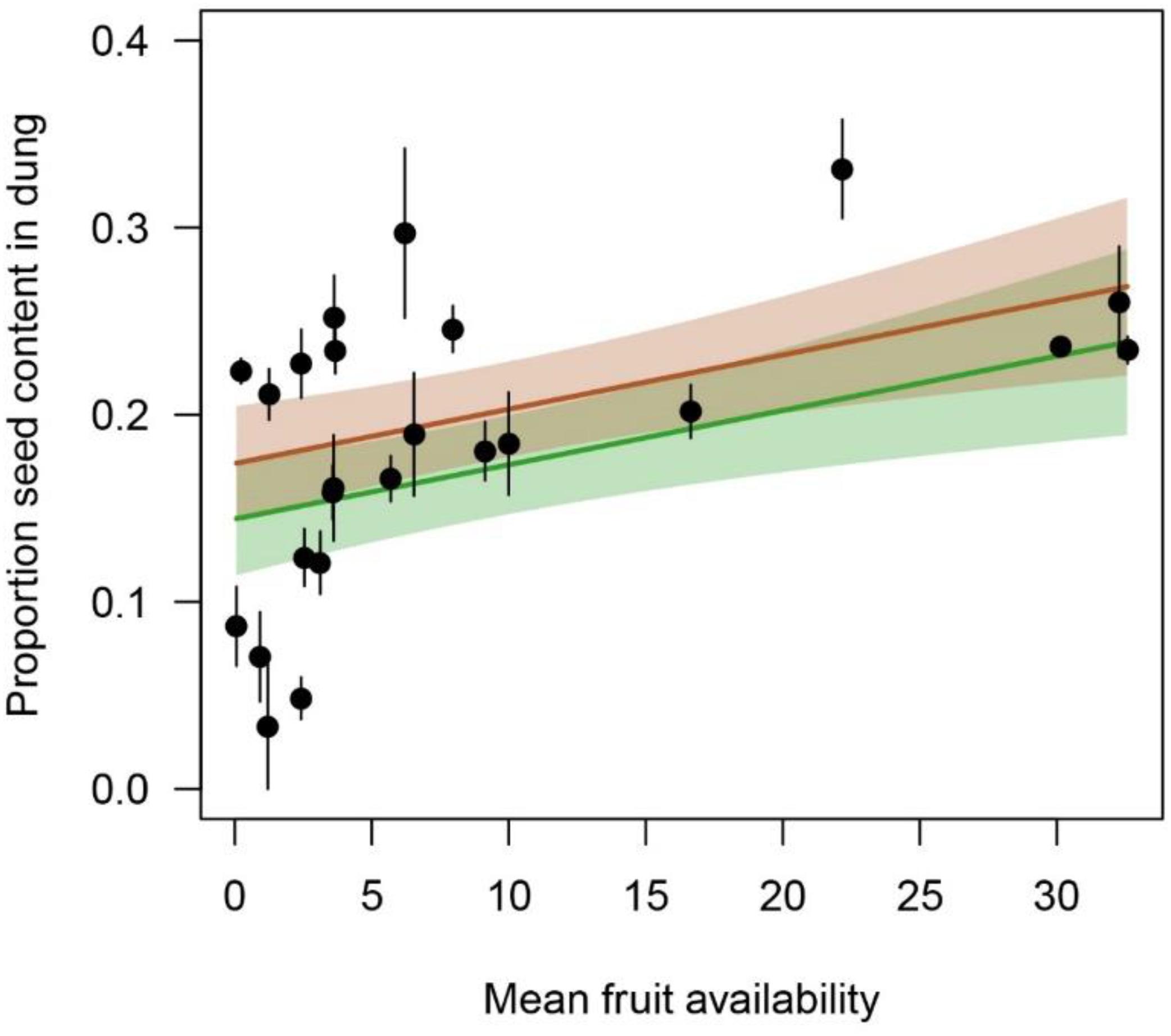
Figure 4. Predicted relationship between the proportion seed content of dung and average fruit availability on fruit walks at the month level. Where: solid colored lines = site level predictions for Ivindo (green) and Wonga Wongué (brown); colored polygon = 95% confidence interval around the fixed effect of fruit abundance; black points = average proportion of seed content in dung by month from the raw data; black bars = standard error of the mean for the raw data.
(iii) What Is the Relative Importance of Fruit Availability and/or Climate Factors (e.g., Temperature and Rainfall) in Explaining Temporal Variation in Elephant Movement Behaviors (The Ratio of Directed to Encamped Behavior).
The elephant movement dataset consisted of 216,341 locations during the study period, with 48% classified as encamped and 52% classified as directed. There was substantial inter-individual variation in the proportion of time spent in each latent behavioral state (Appendix 3). At the monthly scale, there was strong support for an increase in time spent in a “directed” movement state with increasing rainfall (Table 4 and Figure 5A): time in a directed state increased 32% from low (0 mm day–1) to high rainfall (40 mm day–1). There was weak support for time spent in a directed state to increase with fruit availability (Table 4 and Figure 5B): it increased 10% from low (0 fruits per transect) to high fruit availability (33 fruits per transect). There was no support for temperature (Table 4) or the sex of the focal elephant (Appendix 4) influencing elephant movement behavior. Fixed effects accounted for 4.3% of the variation in the best supported model and random effects accounted for 56% (43% of which explained by site and 57% explained by focal elephant identity).
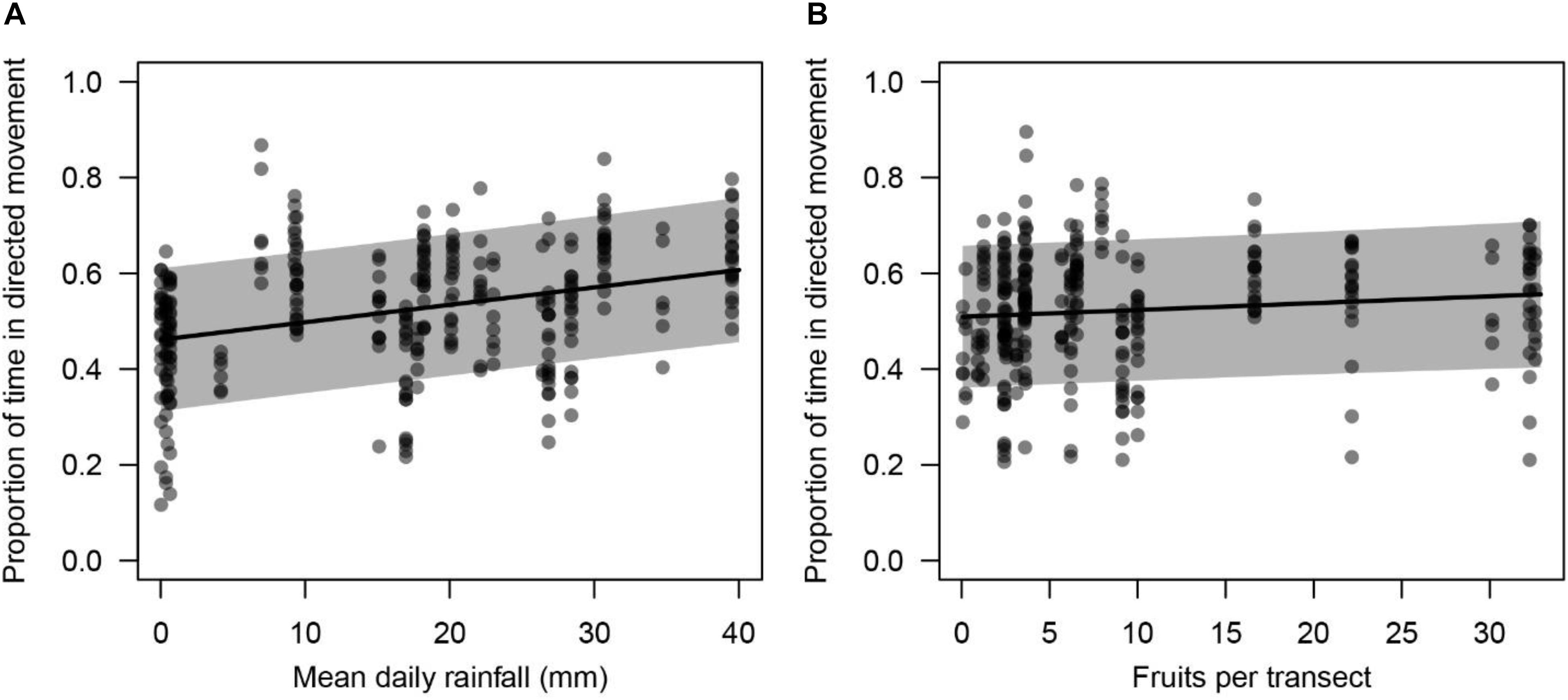
Figure 5. Average relationship between directed movement and rainfall (A) and fruit abundance (B) at monthly scales. Where: black lines = population-average proportion of time in directed movement from the top model in Table 4; gray polygon = 95% confidence interval around the fixed effects; points = partial residuals from the best supported model.
Conducting model selection at the follow-level weakened the relative support for all covariates (Table 5). Some support remained for fruit availability being positively correlated with the proportion of time spent in an exploratory state (Table 5), with the relative effect of fruit availability being 2.5 times greater than in the monthly models: this model, however, had a similar amount of support as the null model. There was no support for follow-level rainfall or temperature influencing the proportion of time spent in an exploratory state.
(iv) Is There Evidence for Inter-Individual Differences in the Responsiveness to the Drivers of Elephant Movement Behavior?
We assessed evidence for inter-individual variation in responsiveness of directed vs. encamped behavior to rainfall and fruit availability using random slope models. There was strong support for inter-individual variation in the magnitude of behavioral shifts due to rainfall (Table 6 and Figure 6A). Some individuals appear relatively insensitive to rainfall, whereas others (particularly within Wonga Wongué) dramatically change their behavioral phenotype with rainfall patterns. There was no support for individual variation in responsiveness to fruit availability (Figure 6B).
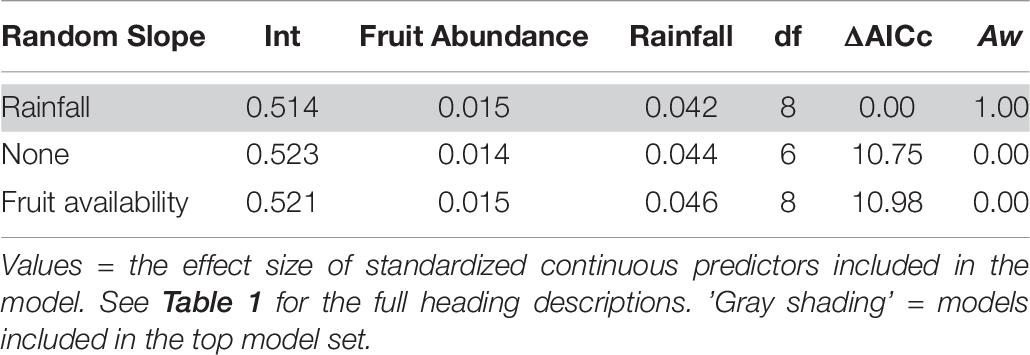
Table 6. Assessment of evidence for between individual variation in sensitivity to rainfall and fruit availability.
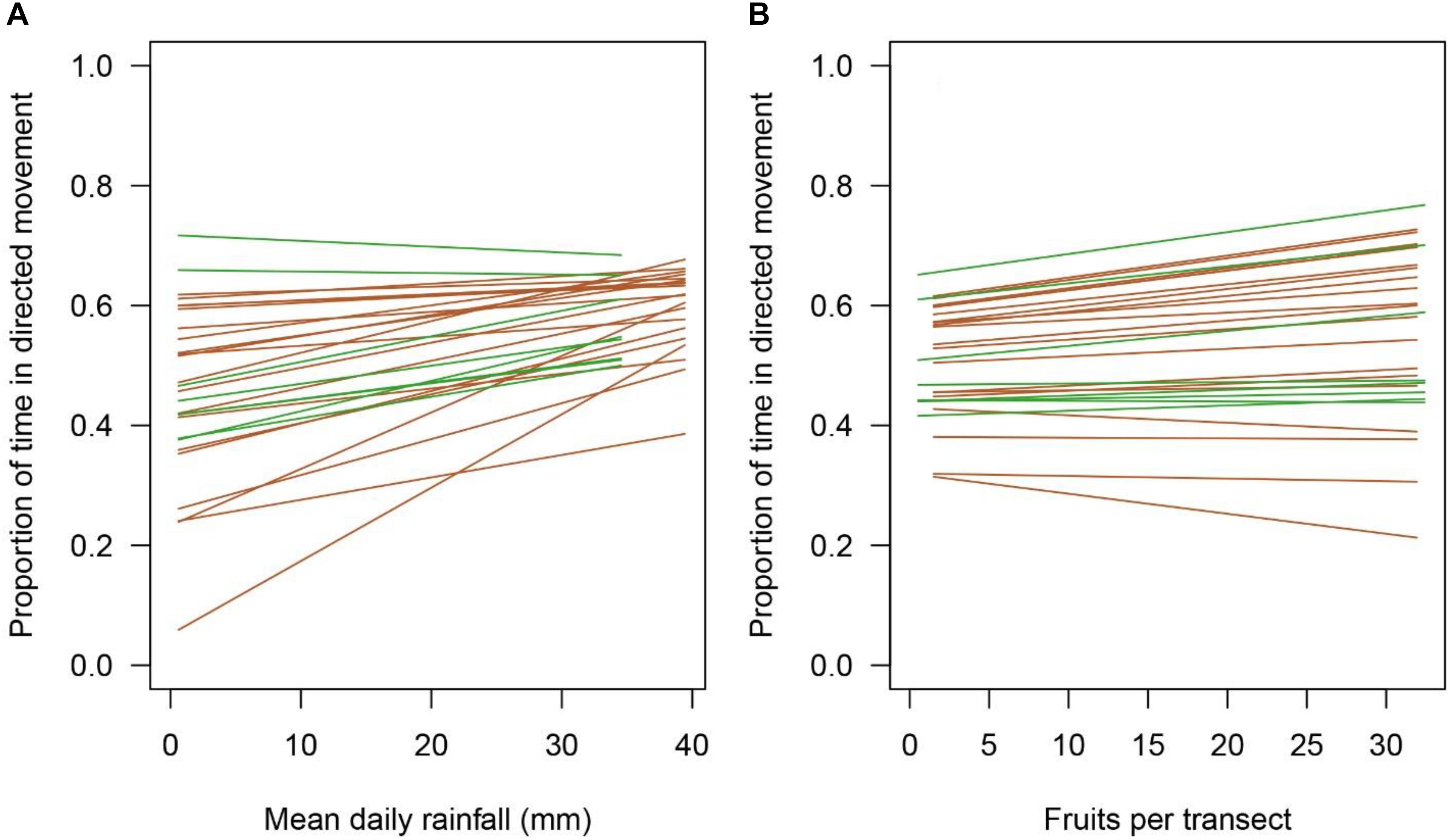
Figure 6. Individual-level relationship between directed movement and rainfall (A) and fruit abundance (B) at monthly scales. Where: lines = individual-level proportion of time in directed movement in relation to rainfall (A) and fruit abundance (B); green = elephants in Ivindo; brown = elephants in Wonga Wongué.
Discussion
For the first time, matching diet information from dung piles of known forest elephants with their actual movements, we demonstrate that spatiotemporal variation in resource availability influences both the dietary composition and movement behavior of forest elephants. The strength of effects of resource availability – specifically fruit and water – on movement behavior varies depending on the temporal scale of analyses. However, across both scales, focal elephant identity accounts for most of the variation observed. Below we discuss the implications of the links between resource availability, climate and individual identity on elephant movement behavior.
The spatiotemporal heterogeneity in fruit availability observed here confirms previous research demonstrating significant seasonal and spatial variation in the Central African tropics (Chapman et al., 1999, 2005; Morgan, 2009; Cardoso et al., 2019). The high abundance of fruit in the dry season in Wonga Wongué is particularly noteworthy as it is often assumed that fruit abundance is highest in the wet season in Central Africa, although a similar pattern was observed in Loango National Park (Morgan, 2009) and a recent synthesis of long-term phenological studies showed marked intersite variation in seasonal fruiting (Adamescu et al., 2018). Within sites, fruit species richness and availability were relatively consistent in Ivindo across seasons, albeit with compositional shifts; whereas in Wonga Wongué, dry season fruit availability was markedly higher than the wet season because of the abundance of Sacoglottis gabonensis fruits. The factors underpinning spatiotemporal heterogeneity in fruit resource availability are complex, including landscape-scale shifts in fruiting-plant composition and species-specific responses to environmental cues (Frankie et al., 1974; Van Schaik et al., 1986; Tutin et al., 1993; Chapman et al., 1999, 2005). Importantly, although our samples of fruit availability were randomly assigned, they were situated on the tracks of followed elephants, and thus reflect fruit availability in elephant used areas. Savannah elephants (Loxodonta africana) preferentially select nutrient rich patches (Pretorius et al., 2011) and forest elephant trails occur in fruit rich locations (Blake et al., 2006), thus the spatiotemporal variation in fruit abundance may also reflect spatiotemporal variation in elephant foraging behavior and habitat selection.
If spatiotemporal variation in fruit availability influences elephant foraging behavior, dietary composition should be correlated with fruit availability. We found strong evidence that fruit consumption is positively correlated with fruit availability: the proportion of seeds in the diet increases as fruit availability increases at both sites. The scaling of fruit consumption with availability likely reflects a preference of fruit over other resources, as other resources (e.g., leaves and/or bark) are available in similar quantities year-round. Forest elephants also likely prefer different fruits in different seasons (e.g., Buij et al., 2007) – the most abundant fruit species may not be the most favored (Kitamura et al., 2002; Wallace, 2005). Despite quantifying seed species abundance and identity in focal elephant dungs, assessing preference was not possible for three reasons. (i) There is not a linear relationship between fruit abundance and seed number because we could not reliably fully count and identify all seeds smaller than 1 cm in the field. (ii) Different fruits have different numbers of seeds. (iii) There are likely species-specific probabilities of seeds surviving the digestive tract. For example, despite being the third most common fruit in Ivindo during the wet season, Nauclea diderrichii was not recorded in elephant diets owing to the small size of its seeds (0.06 g: Seed Information Database, Kew Gardens). Ultimately, robust assessment of dietary preference is challenging without direct experimentation. Also, we only considered the number of fruits available on a given transect, not the biomass/energetic value of each species. Fruit species vary morphologically, nutritionally and visually, and such traits will likely interact in complex ways when acting as cues for foraging elephants (Valido et al., 2011; Schmitt et al., 2018).
Previous studies of forest elephants suggest that fruit abundance is an important driver of movement behavior (e.g., White, 1994b). At the monthly level, we find rainfall a stronger predictor of movement than fruit availability. The best supported model suggests that although greater fruit availability is related to an increase in directed movement state, forest elephants, like savanna elephants, appear to be highly sensitive to water availability. The 32% increase in time spent in directed movement from low to high rainfall could indicate that more abundant small, ephemeral water sources allow forest elephants to move farther from permanent water supplies. Similarly, previous research shows that forest elephants occur farther from perennial water sources in the rainy season (Mills et al., 2018). The marked rainfall seasonality in Gabon indicates that water availability, like fruit and other ephemeral resources, varies temporally. Research in both Congo and Gabon support water availability as a key determinant of elephant presence and movement (Blake, 2002; Buij et al., 2007; Mills et al., 2018).
Although the link between fruit availability and rainfall was decoupled in our two sites, rainfall and fruiting phenology are not independent. Phenological patterns show marked seasonality in Gabon (White, 1994a) and other Central African locations (Poulsen et al., 2002; Adamescu et al., 2018), although there is likely to be substantial inter-annual variation in the links between rainfall and phenology when examined at longer temporal scales (Dunham et al., 2018). Elephants, like other species of frugivores, may use small-scale environmental cues like daily rainfall to predict local, patchy fruit production (Blake, 2002; Takenoshita et al., 2008; Ban et al., 2014; Janmaat et al., 2014). In this case, rainfall could act as a cue for fruit availability while creating ephemeral water resources, both of which might increase directed movement by forest elephants.
Our study demonstrates the importance of evaluating movement behavior at multiple temporal and spatial scales. At finer temporal scales (follow-level rather than monthly), the effect size of rainfall decreased and the effect size of fruit availability increased, although support for the effect of fruit availability was relatively weak in comparison to the null model. This suggests that water availability may determine elephant movements at relatively long time intervals, whereas fine-scale daily movements may be related to fruit availability or other factors not measured in this study. In the analyses presented here, we examined environmental covariates averaged across the temporal period of interest (monthly or follow-level), however, we recommend future studies collect movement and environmental data at finer temporal scales to give step-level insights into the factors influencing elephant movement behavior. Increasing satellite collar emissions to every 15 min, for example, would enable higher intensity sampling of environmental variables while providing more reliable information on the true path the focal elephant took. Higher resolution information on fruit and water availability, slope, vegetation density and ecosystem type could distinguish the drivers of the extreme individual-level variation in elephant movement behaviors. Future studies should also aim to collect environmental data at random sites not visited by focal elephants to evaluate resource selection by comparing the use and availability of resources in the environment (Thurfjell et al., 2014) and/or collect higher resolution fruit availability data along the “true” path of the elephant (rather than at the start and end of each follow day) potentially using novel technologies (e.g., drones; Morellato et al., 2016). Such approaches may increase the relative proportion of variation in elephant movement behavior explained by fruit availability. Additionally, coupling GPS collars with accelerometers would help deduce specific behaviors, such as foraging on fruit on the ground or leaves and branches at shoulder height, through movement and body posture (Brown et al., 2013; Soltis et al., 2016).
Combining follows of known elephants and mixed-effects modeling facilitated teasing apart repeatable individual variation from overall averaged responses. Across all models (dietary composition and movement behavior), focal elephant identity accounted for a substantial proportion of the variation in the data with little repeatable variation attributable to study site or fixed effects. For the dung composition models, between-individual variation suggests that individual elephants adopt different foraging strategies resulting in specialization on different resources, potentially in response to intra and interspecific competition (Araújo et al., 2011). For the movement behavior models, between-individual variation suggests that individual elephants invest different amounts of time and energy to “encamped” and “directed” behaviors, and that these differences are repeatable through time. Determining the proximate mechanisms underpinning individual differences and their implications represents a key challenge for understanding forest elephant movement. For example, is the cost (e.g., greater energy consumption) of moving long distances offset by the increasing probability of encountering higher quality resource patches? We also examined whether focal elephants were differentially sensitive to both fruit availability and rainfall, finding that this is the case, at least for rainfall. Our study supports work on savannah elephants demonstrating that strong individual-level heterogeneity in movement behaviors makes it difficult to draw general conclusions (e.g., Pozo et al., 2018). Note that some of the individual heterogeneity observed here could be derived from variables not included in our models, such as elephant age, reproductive status, and social environment (McComb et al., 2011; Webber and Vander Wal, 2018). Although we took steps to minimize the probability of surveying dung from non-focal elephants, it is remains a possibility that this occurred, particularly if the focal-elephant was associating with a group of un-collared individuals. The extent to which this influences our results is difficult to assess as reliable estimates of group size are near impossible to determine in forested environments, and one off chance visual encounters may not represent the typical group size a given individual associates with. It is reasonable to assume that individuals traveling together as a group would experience the same environmental conditions and show similar movement behaviors as the focal elephant, however, this assumption should be tested in the future. Where possible, future work should strive to collar multiple elephants in a group and use genetic approaches to unambiguously assign dung piles to specific individuals to better assess within-group variation. Research is necessary across longer periods of time, at different sites, and including additional variables to fully understand the drivers of forest elephant movement behavior.
Forest elephants are important seed dispersers that move many intact seeds relatively long distances (Campos-Arceiz and Blake, 2011; Poulsen et al., 2018). In just 1 year at two sites, elephants dispersed in excess of 60 species (Appendix 5), with rarefication suggesting that additional species would be encountered with greater survey effort (see Appendix 2). Across all study sites, African elephants are known to disperse seeds from at least 335 species (Campos-Arceiz and Blake, 2011). An important component of seed dispersal is the distance that seeds are moved from their parent plant: dispersal outside the parent canopy usually increases seed and seedling survival and long-distance dispersal can facilitate genetic diversity within a population or colonization of new habitat (Nathan and Muller-landau, 2000). Seasonal differences in movement behavior could mean that elephants move seeds farther during seasons when they make more directed movements – the wet season in this study. Substantial variation in individual movement behavior also suggests that dispersal distances could depend largely on individual identity. The implications of inter-individual variation in movement patterns on seed dispersal distance have yet to be explored in forest elephants. While not the sole dispersers for most tropical seeds, the distance and quantity of seeds moved by forest elephants supersedes most other species (Bunney et al., 2017). Loss of elephant dispersal from poaching or habitat loss (Poulsen et al., 2017) could lead to reduced forest diversity, inability of forests to colonize new or deforested areas, and potentially reduced carbon stocks (Nathan, 2006; Jordano et al., 2007; Poulsen et al., 2018; Berzaghi et al., 2019).
Human-elephant conflict increasingly threatens both elephants and the livelihoods of rural people (Ngama et al., 2019). Understanding the spatiotemporal availability of resources and elephants responses to changes in their availability represents an important step in identifying drivers of human-wildlife conflict and designing effective solutions. Our observed changes in diet composition and movement behavior in response to fruit and water availability are consistent with more frequent crop raiding (e.g., Buij et al., 2007) and use of edge habitats (Cardoso et al., 2019) in the dry season. Elephants tend to be attracted to agricultural fields with fruiting trees (Ngama et al., 2019), which could be compounded when fruits are seasonally scarce in the forest. With knowledge of forest fruiting phenology and the fruiting cycle of their crops, farmers could predict when crop raiding is most likely to occur and focus elephant deterrent activities at these times (e.g., patrolling fields at night or turning on electrical fences). At the same time, agricultural fields should be developed in areas where elephant diet trees do not occur, while active maintenance of fruiting trees in protected areas or logging concessions might reduce elephant visitation to plantations. Similarly, knowledge of key stands of fruiting trees visited by elephants could orient seasonal anti-poaching patrols and efforts. Most elephant follows occurred within relatively pristine protected areas: future work should investigate drivers of elephant movement around settlements as forest elephants may alter their foraging behavior at human-wildlife interfaces (see Branco et al. (2019) where this was demonstrated for savannah elephants). In the same way that responses to fruit availability and rainfall vary among individuals, elephants may also demonstrate individual behavior in their tendency to approach settlements and raid crops (Swan et al., 2017).
Linking resource availability and environmental factors to animal behavior is key to understanding the movement ecology of forest elephants. This study corroborates previous studies showing that important resources, such as fruit or water, drive elephant movement. We provide novel insight into the strong effects of rainfall at broad temporal scales and suggest that movement at finer temporal scales may be governed by other factors. We also highlight a key challenge to effective elephant management and conservation – strong individual variation that complicates drawing general conclusions about movement behavior.
Data Availability Statement
All the datasets analyzed within this article are available on FigShare: https://doi.org/10.6084/m9.figshare.12081837.
Ethics Statement
The animal study was reviewed and approved by the Duke Institutional Animal Care and Use Committee, Duke University.
Author Contributions
JP, MF, and AM conceived the ideas and designed the methodology. LJ-S, ML, and JM collected the data. CB, GB, KM, and AM analyzed the data. CB, AM, and JP led the writing of the manuscript. All authors contributed critically to the drafts and gave final approval for publication.
Funding
This research was generously supported by the United States Fish and Wildlife Service African Elephant Conservation Fund.
Conflict of Interest
The authors declare that the research was conducted in the absence of any commercial or financial relationships that could be construed as a potential conflict of interest.
Acknowledgments
We are grateful to the Agence Nationale des Parcs Nationaux (ANPN) for collaboration on the elephant collaring project, logistical support, and permission to conduct this work within the national parks. Thank you to the Centre National de la Recherche Scientifique et Technologique (CENAREST) for permission to conduct this work. We thank everyone in the ANPN’s salle d’opération and cellule scientifique, and in particular: Alan Nkoghe Nge, Aurelie Flore Koumba Pambo, Stéphanie Bourgeois, Wynand Viljoen, Norbert Pradel, Roland Zinga Koumba, Anicet Mejne, and Daniel Nzame. There were many ANPN ecoguards who supported us in the field, including: Antoine Djague, Jimmy Ndjangue, Michel Malouata, Bebe Emil, Steed Ntoutoume, Marius Edang, Charles Bikong, Alain Djessy Banguiya, Martial Bouyedi, and Minko Marius Miki. We had additional elephant tracking support from Ongain Thurel, Aymard Tangha, Ambass Awoubazo, and local support from Pacome Mapeko Kambou, Blaise Bazza, Roger Kowe, Jeannot Mbelassanga, and Irma Ngoboutseboue.
Supplementary Material
The Supplementary Material for this article can be found online at: https://www.frontiersin.org/articles/10.3389/fevo.2020.00096/full#supplementary-material
References
Adamescu, G. S., Plumptre, A. J., Abernethy, K. A., Polansky, L., Bush, E. R., Chapman, C. A., et al. (2018). Annual cycles are the most common reproductive strategy in African tropical tree communities. Biotropica 50, 418–430. doi: 10.1111/btp.12561
Araújo, M. S., Bolnick, D. I., and Layman, C. A. (2011). The ecological causes of individual specialisation. Ecol. Lett. 14, 948–958. doi: 10.1111/j.1461-0248.2011.01662.x
Ban, S. D., Boesch, C., and Janmaat, K. R. L. (2014). Taï chimpanzees anticipate revisiting high-valued fruit trees from further distances. Anim. Cogn. 17, 1353–1364. doi: 10.1007/s10071-014-0771-y
Berzaghi, F., Longo, M., Ciais, P., Blake, S., Bretagnolle, F., Vieira, S., et al. (2019). Carbon stocks in central African forests enhanced by elephant disturbance. Nat. Geosci. 12, 725–729. doi: 10.1038/s41561-019-0395-6
Blake, S. (2002). The Ecology of Forest Elephant Distribution and its Implications for Conservation. Ph.D. thesis, University of Edinburgh, Edinburgh.
Blake, S., Deem, S. L., Mossimbo, E., Maisels, F., and Walsh, P. (2009). Forest Elephants: Tree Planters of the Congo. Biotropica 41, 459–468. doi: 10.1111/j.1744-7429.2009.00512.x
Blake, S., Inkamba-Nkulu, C., Stephen, B., Clement, I.-N., Blake, S., and Inkamba-Nkulu, C. (2006). Fruit, minerals, and forest elephant trails: Do All Roads Lead to Rome? Biotropica 36, 392–401. doi: 10.1646/03215
Branco, P. S., Merkle, J. A., Pringle, R. M., Pansu, J., Potter, A. B., Reynolds, A., et al. (2019). Determinants of elephant foraging behaviour in a coupled human-natural system: Is brown the new green? J. Anim. Ecol. 88, 780–792. doi: 10.1111/1365-2656.12971
Brooks, M. E., Kristensen, K., van Benthem, K. J., Magnusson, A., Berg, C. W., Nielsen, A., et al. (2017). Modeling zero-inflated count data with glmmTMB. bioRxiv [Preprint]. doi: 10.1101/132753
Brown, D. D., Kays, R., Wikelski, M., Wilson, R., and Klimley, A. P. (2013). Observing the unwatchable through acceleration logging of animal behavior. Anim. Biotelemetry 1:20. doi: 10.1186/2050-3385-1-20
Buij, R., McShea, W. J., Campbell, P., Lee, M. E., Dallmeier, F., Guimondou, S., et al. (2007). Patch-occupancy models indicate human activity as major determinant of forest elephant Loxodonta cyclotis seasonal distribution in an industrial corridor in Gabon. Biol. Conserv. 135, 189–201. doi: 10.1016/j.biocon.2006.10.028
Bunney, K., Bond, W. J., and Henley, M. (2017). Seed dispersal kernel of the largest surviving megaherbivore—the African savanna elephant. Biotropica 49, 395–401. doi: 10.1111/btp.12423
Campos-Arceiz, A., and Blake, S. (2011). Megagardeners of the forest - the role of elephants in seed dispersal. Acta Oecol. 37, 542–553. doi: 10.1016/j.actao.2011.01.014
Cardoso, A. W., Malhi, Y., Oliveras, I., Ndong, E., Dimoto, E., Bush, E., et al. (2019). The Role of Forest Elephants in Shaping Tropical Forest – Savanna Coexistence. Ecosystems doi: 10.1007/s10021-019-00424-3
Chapman, C. A., Chapman, L. J., Struhsaker, T. T., Zanne, A. E., Clark, C. J., and Poulsen, J. R. (2005). A long-term evaluation of fruiting phenology: importance of climate change. J. Trop. Ecol. 21, 31–45. doi: 10.1017/s0266467404001993
Chapman, C. A., Wrangham, R. W., Chapman, L. J., Kennard, D. K., and Zanne, A. E. (1999). Fruit and flower phenology at two sites in Kibale National Park, Uganda. J. Trop. Ecol. 15, 189–211. doi: 10.1017/s0266467499000759
Dingemanse, N. J., and Dochtermann, N. A. (2013). Quantifying individual variation in behaviour: mixed-effect modelling approaches. J. Anim. Ecol. 82, 39–54. doi: 10.1111/1365-2656.12013
Dunham, A. E., Razafindratsima, O. H., Rakotonirina, P., and Wright, P. C. (2018). Fruiting phenology is linked to rainfall variability in a tropical rain forest. Biotropica 50, 396–404. doi: 10.1111/btp.12564
Dunkin, R. C., Wilson, D., Way, N., Johnson, K., and Williams, T. M. (2013). Climate influences thermal balance and water use in African and Asian elephants: physiology can predict drivers of elephant distribution. J. Exp. Biol. 216, 2939–2952. doi: 10.1242/jeb.080218
Frankie, G. W., Baker, H. G., and Opler, P. A. (1974). Comparative phenological studies of treelet and shrub species in tropical wet and dry forests in the lowlands of costa rica. J. Ecol. 62, 881–919.
Funk, C., Peterson, P., Landsfeld, M., Pedreros, D., Verdin, J., Shukla, S., et al. (2015). The climate hazards infrared precipitation with stations - A new environmental record for monitoring extremes. Sci. Data 2, 1–21. doi: 10.1038/sdata.2015.66
Galipaud, M., Gillingham, M. A. F. F., and Dechaume-Moncharmont, F.-X. X. (2017). A farewell to the sum of Akaike weights: the benefits of alternative metrics for variable importance estimations in model selection. Methods Ecol. Evol. 8, 1668–1678. doi: 10.1111/2041-210X.12835
Harrison, X. A., Donaldson, L., Correa-Cano, M. E., Evans, J., Fisher, D. N., Goodwin, C. E. D., et al. (2018). A brief introduction to mixed effects modelling and multi-model inference in ecology. PeerJ 6:e4794. doi: 10.7717/peerj.4794
Hebblewhite, M., and Merrill, E. H. (2008). Modeling wildlife-human relationship for social species with mixed-effects resource selection models. J. Appl. Ecol. 45, 834–844. doi: 10.1111/j.l365-2664.2008.01466.x
Heithaus, E. R., Fleming, T. H., and Opler, P. A. (1975). Foraging Patterns and Resource Utilization in Seven Species of Bats in a Seasonal Tropical Forest. Ecology 56, 841–854. doi: 10.2307/1936295
Hsieh, T. C., Ma, K. H., and Chao, A. (2016). iNEXT: an R package for rarefaction and extrapolation of species diversity (Hill numbers). Methods Ecol. Evol. 7, 1451–1456. doi: 10.1111/2041-210X.12613
Janmaat, K. R. L., Polansky, L., Ban, S. D., and Boesch, C. (2014). Wild chimpanzees plan their breakfast time, type, and location. Proc. Natl. Acad. Sci. U.S.A. 111, 16343–16348. doi: 10.1073/pnas.1407524111
Jordano, P., Garcia, C., Godoy, J. A., and Garcia-Castano, J. L. (2007). Differential contribution of frugivores to complex seed dispersal patterns. Proc. Natl. Acad. Sci. U.S.A. 104, 3278–3282. doi: 10.1073/pnas.0606793104
Kitamura, S., Yumoto, T., Poonswad, P., Chuailua, P., Plongmai, K., Maruhashi, T., et al. (2002). Interactions between fleshy fruits and frugivores in a tropical seasonal forest in Thailand. Oecologia 133, 559–572. doi: 10.1007/s00442-002-1073-7
Liebmann, B., Camargo, S. J., Seth, A., Marengo, J. A., Carvalho, L. M. V., Allured, D., et al. (2007). Onset and end of the rainy season in South America in observations and the ECHAM 4.5 atmospheric general circulation model. J. Clim. 20, 2037–2050. doi: 10.1175/JCLI4122.1
Loarie, S. R., Van Aarde, R. J., and Pimm, S. L. (2009). Fences and artificial water affect African savannah elephant movement patterns. Biol. Conserv. 142, 3086–3098. doi: 10.1016/j.biocon.2009.08.008
Mcclintock, B. T., Fisheries, A., and Michelot, T. (2018). momentuHMM: R package for generalized hidden Markov models of animal movement. Methods Ecol. Evol. 9, 1518–1530. doi: 10.1111/2041-210X.12995
McComb, K., Shannon, G., Durant, S. M., Sayialel, K., Slotow, R., Poole, J., et al. (2011). Leadership in elephants: The adaptive value of age. Proc. R. Soc. B Biol. Sci. 278, 3270–3276. doi: 10.1098/rspb.2011.0168
Mills, E. C., Poulsen, J. R., Fay, J. M., Morkel, P., Clark, C. J., Meier, A., et al. (2018). Forest elephant movement and habitat use in a tropical forest-grassland mosaic in Gabon. PLoS One 13:e0199387. doi: 10.1371/journal.pone.0199387
Mole, M. A., Rodrigues DÁraujo, S., van Aarde, R. J., Mitchell, D., and Fuller, A. (2016). Coping with heat: behavioural and physiological responses of savanna elephants in their natural habitat. Conserv. Physiol. 4:cow044. doi: 10.1093/conphys/cow044
Morellato, L. P. C., Alberton, B., Alvarado, S. T., Borges, B., Buisson, E., Camargo, M. G. G., et al. (2016). Linking plant phenology to conservation biology. Biol. Conserv. 195, 60–72. doi: 10.1016/j.biocon.2015.12.033
Morgan, B. J. (2009). Sacoglottis gabonensis- a keystone fruit for forest elephants in the Réserve de Faune du Petit Loango. Gabon. Afr. J. Ecol. 47, 154–163. doi: 10.1111/j.1365-2028.2007.00897.x
Morgan, B. J., and Lee, P. C. (2007). Forest elephant group composition, frugivory and coastal use in the Réserve de Faune du Petit Loango, Gabon. Afr. J. Ecol. 45, 519–526. doi: 10.1111/j.1365-2028.2007.00762.x
Nakagawa, S., and Schielzeth, H. (2013). A general and simple method for obtaining R2 from generalized linear mixed-effects models. Methods Ecol. Evol. 4, 133–142. doi: 10.1111/j.2041-210x.2012.00261.x
Nathan, R. (2006). Long-distance dispersal of plants. Science 313, 786–788. doi: 10.1126/science.1124975
Nathan, R., Getz, W. M., Revilla, E., Holyoak, M., Kadmon, R., Saltz, D., et al. (2008). A movement ecology paradigm for unifying organismal movement research. Proc. Natl. Acad. Sci. U.S.A. 105, 19052–19059. doi: 10.1073/pnas.0800375105
Nathan, R., and Muller-landau, H. C. (2000). Spatial patterns of seed dispersal. Trends Ecol. Evol. 15, 278–285.
Ngama, S., Bindelle, J., Poulsen, J. R., Hornick, J. L., Linden, A., Korte, L., et al. (2019). Do topography and fruit presence influence occurrence and intensity of crop-raiding by forest elephants (Loxodonta africana cyclotis)? PLoS One 14:e0213971. doi: 10.1371/journal.pone.0213971
Oksanen, J., Blanchet, F. G., Kindt, R., Legendre, P., Minchin, P. R., O’Hara, R. B., et al. (2011). vegan: Community Ecology Package. Available online at: http://cran.r-project.org/package=vegan (accessed January, 2019).
Owen-Smith, N., Fryxell, J. M., and Merrill, E. H. (2010). Foraging theory upscaled: The behavioural ecology of herbivore movement. Philos. Trans. R. Soc. B Biol. Sci. 365, 2267–2278. doi: 10.1098/rstb.2010.0095
Peres, C. A. (1994). Composition, Density, and Fruiting Phenology of Arborescent Palms in an Amazonian Terra Firme Forest. Biotropica 26, 285–294.
Poulsen, J. R., Clark, C. J., Connor, E. F., and Smith, T. B. (2002). Differential resource use by primates and hornbills: implications for seed dispersal. Ecology 83, 228–240. doi: 10.1890/0012-9658(2002)083
Poulsen, J. R., Koerner, S. E., Moore, S., Medjibe, V. P., Blake, S., Clark, C. J., et al. (2017). Poaching empties critical Central African wilderness of forest elephants. Curr. Biol. 27, R134–R135. doi: 10.1016/j.cub.2017.01.023
Poulsen, J. R., Rosin, C., Meier, A., Mills, E., Nuñez, C. L., Koerner, S. E., et al. (2018). Ecological consequences of forest elephant declines for Afrotropical forests. Conserv. Biol. 32, 559–567. doi: 10.1111/cobi.13035
Pozo, R. A., Cusack, J. J., McCulloch, G., Stronza, A., Songhurst, A., and Coulson, T. (2018). Elephant space-use is not a good predictor of crop-damage. Biol. Conserv. 228, 241–251. doi: 10.1016/J.BIOCON.2018.10.031
Pretorius, Y., de Boer, F. W., van der Waal, C., de Knegt, H. J., Grant, R. C., Knox, N. M., et al. (2011). Soil nutrient status determines how elephant utilize trees and shape environments. J. Anim. Ecol. 80, 875–883. doi: 10.1111/j.1365-2656.2011.01819.x
R Core Team (2013). R: A Language and Environment for Statistical Computing. Vienna: R Foundation for Statistical Computing.
Richards, S., Whittingham, M., and Stephens, P. (2011). Model selection and model averaging in behavioural ecology: the utility of the IT-AIC framework. Behav. Ecol. Sociobiol. 65, 77–89. doi: 10.1007/s00265-010-1035-8
Sach, F., Dierenfeld, E. S., Langley-Evans, S. C., Watts, M. J., and Yon, L. (2019). African savanna elephants (Loxodonta africana) as an example of a herbivore making movement choices based on nutritional needs. PeerJ 7:e6260. doi: 10.7717/peerj.6260
Sassen, M., and Wan, M. (2006). Biodiversity and Local Priorities in a Community Near the Ivindo National Park Makokou, Gabon, Makokou/Ipassa, Gabon. Makokou: CIFOR.
Schmitt, M. H., Shuttleworth, A., Ward, D., and Shrader, A. M. (2018). African elephants use plant odours to make foraging decisions across multiple spatial scales. Anim. Behav. 141, 17–27. doi: 10.1016/j.anbehav.2018.04.016
Short, J. C. (1983). Density and seasonal movements of forest elephant (Loxodonta africana cyclotis, Matschie) in Bia National Park, Ghana. Afr. J. Ecol. 21, 175–184. doi: 10.1111/j.1365-2028.1983.tb01179.x
Soltis, J., King, L., Vollrath, F., and Douglas-Hamilton, I. (2016). Accelerometers and simple algorithms identify activity budgets and body orientation in African elephants Loxodonta africana. Endanger. Species Res. 31, 1–12. doi: 10.3354/esr00746
Swan, G. J. F., Redpath, S. M., Bearhop, S., and McDonald, R. A. (2017). Ecology of Problem Individuals and the Efficacy of Selective Wildlife Management. Trends Ecol. Evol. 32, 518–530. doi: 10.1016/j.tree.2017.03.011
Takenoshita, Y., Ando, C., Iwata, Y., and Yamagiwa, J. (2008). Fruit Phenology of the Great Ape Habitat in the. Afr. Study Monogr. 39, 23–39.
Thaker, M., Gupte, P. R., Prins, H. H. T., Slotow, R., and Vanak, A. T. (2019). Fine-Scale Tracking of Ambient Temperature and Movement Reveals Shuttling Behavior of Elephants to Water. Front. Ecol. Evol. 7:4. doi: 10.3389/fevo.2019.00004
Theuerkauf, J., Waitkuwait, W. E., Guiro, Y., Ellenberg, H., and Porembski, S. (2000). Diet of forest elephants and their role in seed dispersal in the Bossematié forest reserve. Ivory Coast. Mamm. 64, 447–460. doi: 10.1515/mamm.2000.64.4.447
Thurfjell, H., Ciuti, S., and Boyce, M. S. (2014). Applications of step-selection functions in ecology and conservation. Mov. Ecol. 2, 1–12. doi: 10.1186/2051-3933-2-4
Turkalo, A. K., and Fay, J. M. (2001). “Forest elephant behavior and ecology,” in African Rain Forest Ecology and Conservation: An Interdisciplinary Perspective, eds W. Weber, L. White, A. Vedder, and L. Naughton-Treves (New Haven, CT: Yale University press), 207–213.
Tutin, C. E. G., Fernandez, M., and Journal, S. (1993). Relationships between minimum temperature and fruit production in some tropical forest trees in gabon. J. Trop. Ecol. 9, 241–248. doi: 10.1017/s0266467400007239
Valido, A., Schaefer, H. M., and Jordano, P. (2011). Colour, design and reward: Phenotypic integration of fleshy fruit displays. J. Evol. Biol. 24, 751–760. doi: 10.1111/j.1420-9101.2010.02206.x
Van Schaik, C. P. (1986). Phenological changes in a sumatran rain forest. J. Trop. Ecol. 2, 327–347. doi: 10.1017/S0266467400000973
van Schaik, C. P., Terborgh, J. W., and Wright, S. J. (2003). The phenology of tropical forests: adaptive significance and consequences for primary consumers. Annu. Rev. Ecol. Syst. 24, 353–377. doi: 10.1146/annurev.es.24.110193.002033
Wallace, R. B. (2005). Seasonal variations in diet and foraging behavior of Ateles chamek in a southern Amazonian tropical forest. Int. J. Primatol 26, 1053–1075. doi: 10.1007/s10764-005-6458-4
Wallace, R. B. (2006). Seasonal Variations in Black-Faced Black Spider Monkey (Ateles chamek) Habitat Use and Ranging Behavior in a Southern Amazonian Tropical Forest. Am. J. Primatol. 68, 313–332. doi: 10.1002/ajp.20227
Webber, Q. M. R., and Vander Wal, E. (2018). An evolutionary framework outlining the integration of individual social and spatial ecology. J. Anim. Ecol. 87, 113–127. doi: 10.1111/1365-2656.12773
White, L. J. T. (1994a). Patterns of fruit-fall phenology in the Lopé Reserve, Gabon. J. Trop. Ecol. 10, 289–312. doi: 10.1017/s0266467400007975
White, L. J. T. (1994b). Sacoglottis gabonensis fruiting and the seasonal movements of elephants in the Lopé Reserve, Gabon. J. Trop. Ecol. 10, 121–125. doi: 10.1017/s0266467400007768
White, L. J. T., Tutin, C. E., and Fernandez, M. (1993). Group composition and diet of forest elephants Loxodonta africana cyclotis. Afr. J. Ecol. 31, 181–189.
Keywords: behavior, path segmentation, resource availability, human-elephant conflict, individual variation
Citation: Beirne C, Meier AC, Brumagin G, Jasperse-Sjolander L, Lewis M, Masseloux J, Myers K, Fay M, Okouyi J, White LJT and Poulsen JR (2020) Climatic and Resource Determinants of Forest Elephant Movements. Front. Ecol. Evol. 8:96. doi: 10.3389/fevo.2020.00096
Received: 22 October 2019; Accepted: 26 March 2020;
Published: 17 April 2020.
Edited by:
Andrew James Jonathan MacIntosh, Kyoto University, JapanReviewed by:
Monique De Jager, Netherlands Institute of Ecology (NIOO-KNAW), NetherlandsLuke Evans, Arizona State University, United States
Copyright © 2020 Beirne, Meier, Brumagin, Jasperse-Sjolander, Lewis, Masseloux, Myers, Fay, Okouyi, White and Poulsen. This is an open-access article distributed under the terms of the Creative Commons Attribution License (CC BY). The use, distribution or reproduction in other forums is permitted, provided the original author(s) and the copyright owner(s) are credited and that the original publication in this journal is cited, in accordance with accepted academic practice. No use, distribution or reproduction is permitted which does not comply with these terms.
*Correspondence: John R. Poulsen, am9obi5wb3Vsc2VuQGR1a2UuZWR1