- 1UCD Forestry, School of Agriculture and Food Sciences, University College Dublin, Dublin, Ireland
- 2Forest, Environment Research and Services (FERS) Ltd., Meath, Ireland
Sustainable Forest Management (SFM) has become an important pillar of modern forest management, and one way to evaluate the sustainability of forestry is to assess long-term supply of ecosystem services (ESs) indicators. The concept of sustainability also has come to include adapting to climate change and the associated dynamic timber markets. This study aims to: (1) incorporate several ESs indicators in a Forest Management Decision Support System (FMDSS) that can deal with climate change and dynamic timber markets; and (2) analyse the impact that intensified forest management, resulting from global change scenarios that represent different levels of climate change mitigation efforts, will have on forest ES indicators in the west of Ireland. A linear programming model that optimized Net Present Value (NPV) from mill-gate sales was previously developed in Remsoft Woodstock, a DSS framework used for strategic forest planning around the world. This Woodstock model was modified to include the effects of global scenarios that include climate change and dynamic timber prices. This model was further developed to include indicators for five ESs (carbon storage in the forest as well as in harvested wood products and carbon substitution, windthrow risk, biodiversity, water quality, and cultural values), to assess the impacts of these global scenarios on the forest landscape and the sustainability of forest management. The ES indicator values were mainly linked to forest age, forest type, and yield tables, and their inclusion in the FMDSS had almost no impact on total model run times. Intensified forest clearfelling, as a result of increasing timber prices associated with most global scenarios, led to increased phosphor emissions to waterbodies, and reductions in windthrow risk and carbon storage. The global scenarios only resulted in minor differences in the indicator values for biodiversity and cultural values. Besides the global scenarios, recent forest policy development and the poor soil conditions in the study area impacted on the results. The developed system, with its innovative method to incorporate climate change and associated market dynamics, could be applied to other forest landscapes in Ireland and Europe, or indeed by any forest company or organization that uses Remsoft Woodstock.
Introduction
The concept of sustainable forestry originates from the 18th century and concerned sustainable supply of charcoal required for the mining industry (Hofer, 2009). Since then, Sustainable Forest Management (SFM) has been expanded to include economic, ecological, and social values, as defined by the United Nations Conference on Environment and Development in Rio de Janeiro in 1992 (Forest Europe, 1993; Mulloy, 1997). Compliance with SFM has become a requirement for many forests around the world, Ireland included. The concept of Ecosystem Services (ESs) was originally introduced to raise awareness about the importance of nature protection by framing biodiverse habitat destruction in terms of economic loss (Gómez-Baggethun et al., 2010). The concept has since been expanded, and ES indicators specific to each region are now utilised to assess the status of the forest and the economic, environmental and social performance of the forest industry. ESs are defined as goods and services that contribute to human well-being (Reid et al., 2005) and they often depend on assets and functions of the world's natural capital (e.g., soil, air, freshwater, minerals etc.; Turner and Daily, 2008). The Millennium Ecosystem Assessment reported that intensified natural resource management rapidly accelerated the decline of many ESs globally (Reid et al., 2005). Implementing SFM could be simplified by having a set of measurable ES indicators tied to each SFM pillar (Biber et al., 2015; Nobre et al., 2016). However, since different methodologies are often applied across the world when assessing ESs, it is questionable if ES-values can be compared between countries (Biber et al., 2015), and utilizing internationally uniform methodologies to assess ESs could result in reduced relevance for local landscape ESs (Nordström et al., 2019). Quantifying ESs makes it possible to analyse the interactions and trade-offs between them under different forest management approaches (Raudsepp-Hearne et al., 2010). This can be done using Forest Management Decision Support Systems (FMDSSs), both at a stand and landscape level, which can then be upscaled (or modelled) to analyse regional or even global level ES trade-offs.
FMDSSs have been widely used since the 1980s to make better forest management decisions as well as to forecast the future forest condition to ensure the sustainability of timber harvesting (Reynolds, 2005; Reynolds et al., 2008). FMDSSs were initially developed to ensure sustainable strategic timber yield (Nobre et al., 2016), and timber production or Net Present Value (NPV) often remains the main focus to this day (Reynolds et al., 2008; Bettinger et al., 2017). Spatial aspects of forest planning have been developed for these systems, mainly at the tactical and operational planning level (Baskent and Keles, 2005). These aspects refer to avoiding too large adjacent clearfelled areas, i.e., green-up rules (Bettinger and Zhu, 2006), minimizing harvesting and transportation costs (Nieuwenhuis and Williamson, 1993), and maintaining large areas of un-fragmented old growth and valuable biodiverse forest (Öhman and Wikström (2008). FMDSSs have been developed to analyse the impacts of forestry operations on biodiversity, carbon sequestration, water quality, the long-term changes in forest composition and structure, as well as to analyse how pest, disease, windthrow and wildfire damage will affect the forest and the resulting timber supply and other ES-values (Eriksson and Borges, 2014; Vacik and Lexer, 2014; Biber et al., 2015; Nobre et al., 2016; Marques et al., 2017). With an increasing understanding of forest ecosystems, the concept of SFM has expanded to consider, inter alia, the impacts that changing climate may have on species suitability, forest productivity, forest ESs and the resilience to pests diseases and extreme weather events (Nordström et al., 2019). Forest ecosystems are increasingly under pressure—along with accommodating SFM principles and the pressure of climate change impacts, new policies that have been introduced as a response to climate change often emphasize increased biomass production to make societies more sustainable (Lindner et al., 2010; Söderberg and Eckerberg, 2013). Research in Europe has shown that increased harvesting levels often reduce the biodiversity levels in Europe (Verkerk et al., 2011; Duncker et al., 2012; Biber et al., 2015). High levels of biodiversity and tree species diversity have been found to be closely linked to other ESs and ecosystem functions (e.g., increasing resilience to disturbances and climate change, enhanced growth in certain species mixtures, high stocks of carbon stored in living biomass), making them strong indicators of ecosystem health (Balvanera et al., 2006; Gamfeldt et al., 2013; Brockerhoff et al., 2017).
Analyzing the long-term impacts of various global development scenarios on forest management approaches and forest ESs is crucial to avoid negative outcomes and conflicts between stakeholders. The ALTERFOR project is a collaboration between 9 countries (Germany, Ireland, Italy, Lithuania, The Netherlands, Portugal, Slovakia, Sweden, and Turkey; Marques et al., 2017; Marto et al., 2018; Schwaiger et al., 2018, 2019; Mozgeris et al., 2019; Nordström et al., 2019) that investigates the suitability of FMDSSs to analyse the complex dynamic interactions between climate change, global markets, and forest management practices to assess the suitability of current and alternative forest management systems to address future challenges and provide society with an optimal mix of ESs. Standardized ES indicators have been implemented in nine different FMDSSs to allow for comparisons across European landscapes and facilitated the large-scale analysis of long-term climate change and bioeconomy impacts on the provision of forest ESs in Europe (Nordström et al., 2019). The research presented in this article focusses on aspects of the ALTERFOR project that relate to the situation in Ireland.
Early industrialism and an increased demand for agricultural land from the rapidly increasing population in the 18th and 19th century nearly exhausted all Irish forests (OCarroll, 2004). Between 1908 and 2017 the forest cover in the Republic of Ireland increased from 1.5 to 11% or 770,020 ha (OCarroll, 2004; Forest Service, 2018). Much of this afforestation was done by the Irish state between the 1950s and 1990s, and large areas of inexpensive, mountainous, marginal agricultural and blanket peat land were planted with fast growing and hardy conifer species from Western North America (Gray, 1963; Neeson, 1991; Tiernan, 2007). The nutrient poor and excessively wet blanket peat sites were afforested using a combination of plowing, drainage, and application of rock phosphatic fertiliser to ensure stand survival (Renou-Wilson and Byrne, 2015). By 2012, about one third of all Irish afforestation had occurred on blanket peat sites (Forest Service, 2013). The main species used were Sitka spruce (Picea Sitchensis (Bong.) Carr.) and lodgepole pine (Pinus contorta Douglas), which now occupy 51.1% and 9.6% of the Irish forest estate, respectively (Forest Service, 2018). Lodgepole pine was planted on the least productive sites, while Sitka spruce was generally planted on the better sites, due to its ability to reach a high Yield Class (YC, maximum mean annual volume increment for the species on the site in m3 ha−1 yr−1) on a wide range of sites (Renou and Farrell, 2005). Sitka spruce is still the staple income producing species in Irish forestry and conifers in are clearfelled on a 35–50-year rotation, depending on species and site productivity. Since the 1990s, nearly all afforestation has been done on private land, with a greater focus on fertile and productive sites, while adhering to higher environmental standards, including the use of mandatory buffer zones, and, recently, with increased species diversification requirements (Byrne and Legge, 2008; Forest Service, 2016, 2017). Studies on the long-term impacts of climate change on Irish forestry found that the spruce trees utilised for sawlog production will likely suffer reduced growth in the future, causing reduced revenue for forest managers (Cabrera Berned and Nieuwenhuis, 2017; Keenan et al., 2017; Lundholm et al., 2019). However, Lundholm et al. (2019) found that increased demand for wood biomass would offset the negative growth impacts of climate change on NPV, causing a net increase in future profits due to higher timber assortment prices. Forest based ESs has been evaluated previously in Ireland, but this assessment was focused on finding the biophysical provision limits of the forest landscape (Corrigan and Nieuwenhuis, 2016), in order to find an optimal balance of ESs under future policy scenarios (Corrigan and Nieuwenhuis, 2017). Although these studies involved important development in adapting FMDSS to assess ESs, they did not consider the impact of climate change on their provision levels. Thus, it is important to investigate the impacts that increased harvesting, resulting from climate change and an increased timber demand caused by mitigation efforts, might have on biodiversity and other ESs, especially if the harvesting of forest biomass is introduced.
FMDSSs can be used to model severe biotic and abiotic disturbances, including disturbances with increased frequency and/or magnitude due to climate change (Hennigar et al., 2013). Wind is the largest abiotic disturbance causing mortality in Irish forestry; mortality from windthrow is expected to increase in boreal and temperate forests due to climate change. This increase is not only the result of increased wind speeds, but also from milder winters with less frozen soil, reducing root anchorage (Saad et al., 2017), and from excessively wet soils, limiting root growth (Ray et al., 2008). However, a lack of relevant information about future disturbances means that many potentially devastating impacts are difficult to model accurately (Cunniffe et al., 2015), and using Monte Carlo simulation to model disturbances can result in highly imprecise estimates, even if long time series are available (Armstrong, 1999). Remsoft Woodstock models using optimisation (the type of FMDSS used in this study) cannot accommodate stochastic disturbances (Walters, 1993), so these types of impacts were not included in this study.
The aims of this study were: (1) incorporate several ESs indicators in a FMDSS that can deal with climate change and dynamic timber markets; and (2) analyse the impact that intensified forest management, resulting from global change scenarios that represent different levels of climate change mitigation efforts, will have on forest ES indicators in the west of Ireland.
Materials and Methods
Case Study Area
The Barony of Moycullen was chosen as the Case Study Area (CSA); it is located around the Cloosh Valley forest and the Derrada forest, just west of Galway city, county Galway, in western Ireland (Figure 1). The area contains 10,230 ha of forest, including Ireland's largest continuous forest, at almost 4,600 ha. Coillte, the Irish semi-state forestry company, owns 81.1% of the forests in the CSA, with the remainder privately owned. Atlantic blanket peat soils occupy 82% of forest area, with the remainder mainly consisting of heavy wet gley soils and shallow lithosol soils. Most of the forest was established through afforestation in the 1970s and 1980s, using plowing, drainage, fertilisation and planting, and using hardy and fast-growing tree species from western North America. Sitka spruce and lodgepole pine occupy 41.0% and 29.1% of the CSA's forests, respectively, other conifers and broadleaves occupy 10.4%, and the remainder, 19.5%, is made up of open, unstocked forest area. The CSA contains one of Ireland's eight priority Freshwater Pearl Mussel (Margaritifera margaritifera L) catchments (Moorkens et al., 2013), and is frequented by many visitors, both locals from Galway, as well as tourists from Ireland and abroad. As blanket peat soils are often waterlogged, poor in nutrients, and allow only shallow root growth, the forests growing on them are very susceptible to windthrow. Windthrow is further exacerbated by the CSA's proximity to the Atlantic Ocean and the associated strong winds. Additionally, peatlands had to be drained prior to afforestation, causing the peat to oxidize and release CO2. Thus, the landscape in the CSA has multiple uses and complex ES interactions are taking place, making it an interesting study object for the analysis of the long-term sustainability impacts of climate change and the associated anticipated changes in timber prices. Many of these multiple use conflicts and ES interactions are also present in afforested peatland landscapes all along the western European seaboard, thus making the results relevant for forest managers and policy makers in a wider area.
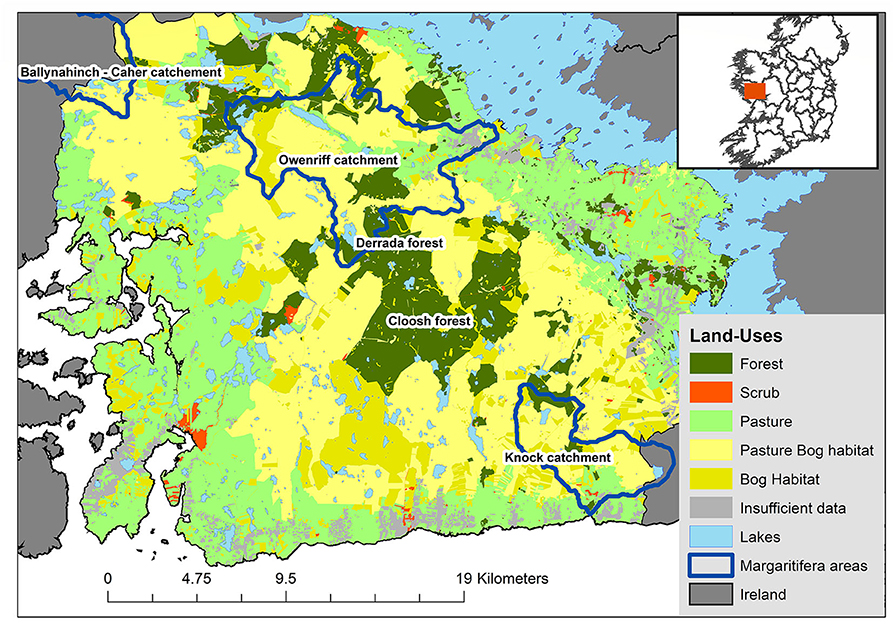
Figure 1. The Barony of Moycullen CSA in County Galway, Ireland, delineated by its land-uses. Margaritifera areas are catchments with freshwater pearl mussel populations. The Cloosh Forest is the forest area at the center of the CSA, Derrada is located north of Cloosh. The Owenriff catchment contains much of the Derrada forest and is located just north of Cloosh. Source: Lundholm et al. (2019).
Decision Support System (DSS)
The core model for the DSS was developed in Remsoft Woodstock (Remsoft, Fredericton, Canada), a software system used worldwide for strategic forest planning and management (Walters, 1993). The model used linear programming optimisation, with an objective function that maximises NPV from mill-gate timber sales over a 100-year planning horizon, using a 5% discount rate, commonly used in Irish forestry (Tiernan, 2007; Corrigan and Nieuwenhuis, 2016; Teagasc, 2019). This core model was developed specifically for Irish forestry, incorporating country-specific forest management prescriptions, and to be compliant with Irish forest policy and environmental policy. The model used Irish growth and yield tables to forecast stand development and timber production, as well as the relevant costs and revenues associated with forest management actions (Lundholm et al., 2019). The core model was then expanded to include both climate change, through changes in tree species productivity, and an expanding bioeconomy, represented by dynamic wood assortment prices that reflect varying levels of mitigation efforts, for three global scenarios that were down-scaled to the national level. Since a changing climate and wood demand affect other ESs than harvest volumes and assortments, the DSS model was further expanded and customized to include indicators for five ESs: carbon storage, regulatory services, biodiversity, water quality, and cultural services. With the exception of the cultural RAFL-index, all ESs presented in this study were outputs produced by the Woodstock DSS; the cultural ES attributes were DSS outputs that were combined to produce the RAFL-index post-optimisation. The final DSS model is called the ALTERFOR model, after the research project for which is was developed.
Modelled Scenarios
Three global scenarios and a control scenario were modelled, with the global scenarios including the effect of climate change on tree growth and dynamic timber prices, based on regional and global demand for wood, affected by different levels of climate change mitigation effort. The global scenarios narratives (Forsell and Korosuo, 2016) were derived from the Global Biosphere Management Model (GLOBIOM) (Havlík et al., 2014) and were provided by the International Institute for Applied Systems Analysis. The basis for the global scenarios were combinatory analyses of the EU policy scenarios (Forsell et al., 2016) and the Representative Concentration Pathways (RCP)—Shared Socio-economic Pathways (Fricko et al., 2017), developed for the International Panel for Climate Change. Thus, GLOBIOM provided dynamic timber prices on a decennial basis and their associated climate change scenario narratives. The Irish software Climadapt (Ray et al., 2009) was used to obtain species-specific climate change impact factors which were implemented in the ALTERFOR model, bringing the global scenario narratives to the Irish level. Climadapt uses a combination of ecological site classifications, current climate, and future climate in 2080 to predict the current and future site productivity for 20 tree species used in Irish forestry, 11 of these species and species groups which were modelled in this study: alder (Alnus glutinosa (L.) Gaertn.), ash (Fraxinus excelsior L.), beech (Fagus sylvatica L.), birches (Betula pubescens Ehrh. and Betula pendula Roth), Japanese larch (Larix kaempferi (Lamb.) Carr.), lodgepole pine (Pinus contorta Douglas), Norway spruce (Picea abies (L.) H. Karst.), oaks (Quercus robur L. and Quercus petraea (Matt.) Liebl.), Scots pine (Pinus sylvestris L.), Sitka spruce (Picea sitchensis (Bong.) Carr.), and sycamore (Acer pseudoplatanus L.). The Climadapt predictions of future climate only included the average climatic factors and did not consider the increased severity of storms. The exact global scenario factors used can be found in the study by Lundholm et al. (2019), and the four modelled scenarios were:
• BAU—Business as usual. Control scenario with no climate change or dynamic prices implemented.
• S1—Reference: Temperature increase of 3.7°C by 2100, compared to pre-industrial values. Climate scenario: RCP8.5. No effort to mitigate climate change. Early increase in sawlog prices in the first 30 years, then prices remain static at 29% higher than the start year. Early increase in pulpwood price by the first 20 years, then slight decline around year 30, after which prices were mostly static at 21% higher than the start year.
• S2—EU Bioenergy: Temperature increase of 2.5°C by 2100, compared to pre-industrial values. Climate scenario: RCP4.5. EU effort to mitigate climate change through expanded bioeconomy. Steep increase in sawlog prices by 38% around year 60–90. Slight pulpwood price increase by 22% followed by decline all within the first 50 years, followed by static prices at 14% higher than the start year.
• S3—Global Bioenergy: Temperature increase of 1.5–2.0°C by 2100, compared to pre-industrial values. Climate scenario: RCP2.6. Global effort to mitigate climate change through increased bioeconomy. Steady increase in sawlog prices throughout the planning horizon to a level 42% higher than in the start year. Pulpwood prices increase by 84% over the 100-year planning horizon.
Both the climate change productivity impacts and the dynamic timber prices were converted to annual change values to avoid sharp increases and decreases between years, as those would greatly influence the model solution. The climate change productivity impacts were implemented in the DSS by scaling the growth and yield for all tree sizes, and the dynamic wood assortment prices were implemented as factors that were multiplied with the default wood assortment price.
Ecosystem Service Indicators
Carbon
The carbon ES indicator includes five categories of forest related carbon: (1) stand living carbon (above and below ground), (2) deadwood carbon (from harvesting and natural mortality), (3) carbon stored in harvested wood-products (HWP), (4) substitution of fossil fuels from using wood fiber for biofuel and in construction, and (5) carbon emissions from drained peat soil. The deadwood carbon and HWP were subjected to a decay function to represent decomposition of deadwood and degradation of HWP. The carbon ES assessment focused on the cumulative changes in total carbon stock from the start of the planning horizon (Equation 1). The absolute stock would be difficult to estimate since the historic harvest assortments and historic storage in HWP were unknown.
where CBiha−1 is the carbon balance per ha in year i, in units tons carbon ha−1; ΔCpoolj,i is the change in carbon for category j (i.e., stand living carbon, deadwood carbon, and HWP carbon), in year i, given in tons carbon; Psub(ff)totali and Psub(P)totali are the total carbon substitution for fossil fuels and products, respectively, in year i, in tons carbon; OSC is the organic soil carbon loss in tons carbon ha−1; peatfori is the area of drained peatland forest in year i; forest areai is the total area of forest, for which the carbon balance was calculated.
Stand living carbon
Stand living carbon accounts for both above and below ground stocks of carbon and was calculated based on biomass expansion factors, carbon fractions, merchantable standing volume, and root ratio, i.e., ratio of belowground biomass to aboveground biomass, in Equation (2).
where SLCi is the stand living carbon, in year i, in tons carbon; BCEFS is the biomass conversion and expansion factor for growing stock (hence the subscript s) (Supplementary Table 1); CF is the carbon fraction in tons carbon (ton dry mass)−1 (Supplementary Table 2); standvoli is the total merchantable stand volume in year i, in m3; and R is the ratio of belowground biomass to aboveground biomass (Supplementary Table 1).
Deadwood carbon
Deadwood carbon inflow originated from both natural mortality and harvesting, recording carbon stored in logs aboveground and all belowground roots. Natural mortality was only obtained from the yield tables and therefore did not include the impacts of extreme events such as droughts, windthrow, pests and diseases. The annual inflow of deadwood went into four different stocks (aboveground and belowground stocks for both natural mortality and harvest residue deadwood carbon) that were subjected to annual decay functions. Natural mortality carbon (NMC) was calculated using Equation (3), Harvest Residue Carbon (HRC) was calculated using Equation (4).
where inflowNMCiis the total input of aboveground and belowground natural mortality carbon in year i, in tons carbon; CF is the carbon fraction in tons carbon (ton dry mass)−1 (Supplementary Table 2); NMvoli is the natural mortality of merchantable volume in year i, in m3; D is the density of the tree species in tons m−3 (Supplementary Table 2); BCEFS is the biomass conversion and expansion factor for growing stock (Supplementary Table 1); and R is the ratio of belowground biomass to aboveground biomass (Supplementary Table 1).
where inflowHRCi is the total inflow of harvest residue carbon and belowground deadwood due to harvesting in year i, in tons carbon; CF is the carbon fraction in tons carbon (ton dry mass)−1 (Supplementary Table 2); Harvvoli is the harvested volume in year i, in m3; D is the density of the tree species in tons m−3 (Supplementary Table 2); HF is the harvest fraction left on sites and can be calculated from the average tree volume in m3, based on the calculation of F in Equation (5), which is the currently used Irish industry standard; BCEFS is the biomass conversion and expansion factor for growing stock (Supplementary Table 1); and R is the ratio of belowground biomass to aboveground biomass (Supplementary Table 1).
If the value of F < 0.03, HF is given the calculated value of F, otherwise HF = 0.03, which only happens when the average tree volume is larger than 1.35 m3.
The decay function was applied to all deadwood carbon pools, using Equation (6) (Bond-Lamberty and Gower, 2008).
where DWij is deadwood carbon stock in category j (i.e., NMC and HRC) in year i, in tons carbon; k is the constant for first order decay which is dependent on the product half-life given in units yr−1 (Equation 7); FF is the fragmentation loss factor set at 0.85 (i.e., 15% is the proportion of annually lost deadwood soil carbon due to fragmentation); and Inflowijis the inflow of particulate deadwood carbon from category j in year i, in tons carbon yr−1.
where HL is the half-life in years for the deadwood carbon category (aboveground or belowground). Half-life for logs is 12 years (Yatskov et al., 2003; Olajuyigbe et al., 2011; Lundmark et al., 2016). Roots have a half-life of 19 years, stumps have a half-life of 14 years, and stumps make up about 30% of the total mass of stumps and roots larger than 10 cm in diameter (Olajuyigbe et al., 2011). This gives all belowground deadwood carbon a weighted mean half-life of 17.5 years. Thus, k is 0.0577 and 0.0396 for aboveground and belowground deadwood carbon pools, respectively. In the calculation, aboveground and belowground carbon were kept separate, so that of the different decay functions could be applied.
Harvested wood-products carbon
Utilisation of harvested wood and processing it into different products affects the storage of carbon outside the forest. In Ireland, HWP consist mainly of sawnwood and wood-based panels, while small amounts of the pulpwood assortment are utilised for biofuel. The inflow of carbon to each HWP category depends on tree species, log diameter, and wood allocation in each global scenario (Equation 8).
where WInflowij is the inflow of stored carbon in year i in HWP product category j (i.e., wood-based panels or sawnwood) given in tons carbon; Hproductijis the wood allocated in year i to HWP category j, given in m3 (Equation 9); PL is the processing loss factor, set to 0.43 for Ireland; D is the HWP category density, in tons m−3(Supplementary Table 2); and CF is the carbon conversion factor in tons carbon (ton dry mass)−1 (Supplementary Table 2).
where Harvvoli is the harvested volume in year i, in m3; HF is the harvest fraction left on site (Equation 5); AFh,i is the assigned fraction of harvested wood removed from site that is allocated to assortment h, for each year i, AF varies by species and tree size and was derived from the yield tables; FsFPh,jis the utilisation fraction of assortment h to HWP category j and varies between three species categories (i.e., conifers excluding lodgepole pine, lodgepole pine, and broadleaves), and by global scenario (“normal utilisation” for BAU and S1, “climate change mitigation” for S2 and S3) (Supplementary Table 3).
The carbon stock in each HWP category increased from the inflow of processed wood in subsequent years, but the inflow and previous year's stock was subject to decayed over time, Equation (10).
where HWPCij is the carbon stock in HWP category j, in year i, in units tons carbon; k is the decay constant, using 0.027726 for sawnwood and 0.019804 for wood-based panels, based on half-lives of 25 and 35 years (IPCC, 2014), respectively, calculated according to Equation (7); and WInflowijis the inflow of carbon to HWP category j, in year i, in tons carbon units.
Fossil fuel substitution
Utilisation of harvested wood can substitute the use of emission-heavy construction materials or fossil fuels when wood fiber is used for energy production. These were considered as one-off substitutions happening in the year of harvesting. All the substitution factors excluded forest carbon dynamics, to avoid double counting of forest carbon. Fossil fuel carbon substitution was calculated according to Equation (11), and product carbon substitution was calculated according to Eqution (13).
where Psub(ff)i,jare the emission savings due to substitution of fossil fuels in year i, for fossil fuel category j, in tons carbon; Harvvolenergy(i) is the harvested volume utilised for bioenergy in year i, given in m3 (Equation 12); D is the species wood density in tons m−3 (Supplementary Table 2); CF is the carbon fraction (Supplementary Table 2); Fmix(j) is the ratio of fossil fuel category j being replaced over the total fossil fuels being replaced, based on Ireland's fossil fuel mix of natural gas: 0.49, oil: 0.35, and coal: 0.16 (Duffy et al., 2018); and DFi is the product substitution displacement factor for category j, in units ton carbon (emission ton carbon wood)−1 (Supplementary Table 4). The equation used to account for burning of firewood was a modification of Equation (11), but instead of multiplying with the Fmix(j) factor and DFi, the estimated emission was multiplied with −1. This was done since firewood in Ireland is largely burnt in inefficient domestic stoves, resulting in immediate oxidization and a net emission, as opposed to burning wood in combined heat and power plants.
where Harvvolenergy(i) is the harvested volume in year i, in m3; HF is the harvest fraction left on site (Equation 5); AFhi is the assigned fraction of harvested wood removed from site that is allocated to each assortment h, for each year i, AF varies by species and tree diameter and was sourced from the yield tables; FsubEh is the fraction of each wood assortment (h) assigned to fossil fuel energy replacement (Supplementary Table 5).
where Psub(P)I,j is the emission savings due to product category j in year i, in tons carbon; Harvvolsubs(I, j) is the harvest volume of wood used for semi-finished substitution products for category j, in year i, given in m3, calculated according to Equation (12), but with FsubEh replaced with FsubPh,j, the fraction of each wood assortment (h) assigned to product substitution of category j (Supplementary Table 5); D is the species wood density in tons m−3 (Supplementary Table 2); CF is the carbon fraction (Supplementary Table 2); PL is the processing loss factor, set to 0.43 for Ireland; and DFj is the product substitution displacement factor for product category j, in units ton carbon emission ton carbon wood−1 (Supplementary Table 4).
Soil carbon
Studies of the soil carbon balance in mineral soils are largely inconclusive on the magnitude and direction of stock changes due to forest management and forest types (IPCC, 2006). Thus, changes in mineral soil carbon stock were not included in the FMDSS and all soil carbon refers only to drained and forested organic soils, where there is significant carbon loss. The IPCC default emission factor for drained organic soils in the temperate zone is 0.61 tons C ha−1 yr−1, with an additional loss of 0.31 tons C ha−1 yr−1 due to runoff emission from dissolved organic carbon (IPCC, 2006). These values were incorporated for all forested peatland since drainage at afforestation was a necessary practice to ensure crop survival.
Regulatory—Windthrow Risk
Regulatory ESs refers to risk management, which mainly means windthrow in Ireland. A windthrow risk model was developed for Ireland by Ní Dhubháin et al. (2009) which calculates the probability that a stand has experienced windthrow with more than 3% of stems windthrown, based on several site and stand characteristics (Supplementary Table 6). The windthrow risk probability was calculated for the total forest area at ≥70% windthrow risk, and fellable forest area at ≥70% windthrow risk, using the general structure of a logistic model. This model only measured the risk of windthrow having affected the stand, it did not make any prediction on the damage impact.
Biodiversity
The biodiversity ES assessment was based on measuring multiple stand structural features that contribute to improving biodiversity (Nieuwenhuis and Nordström, 2017) and some of the cultural attributes that were relevant for biodiversity assessment. These features were reported separately on a landscape level, and because they affect different aspects of biodiversity, they were not deemed equivalent, which is why no average biodiversity indicator score was calculated. These features were:
• Volume of large diameter trees, with Diameter at Breast Height (DBH) > 30 cm, > 40 cm, and > 50 cm, all in m3 ha−1.
• Volume of natural mortality logs and volume of large diameter (DBH > 30 cm) natural mortality logs, both in m3 ha−1.
• Volume of native Irish trees and broadleaves, in m3 ha−1.
• Area of buffer zones, in ha.
• Area of forest aged 61–80 and area of forest older than 80 years, in ha.
• Cultural attributes for the percentage final felling area, Hemeroby index, Shannon species diversity, and DBH evenness (Table 1).
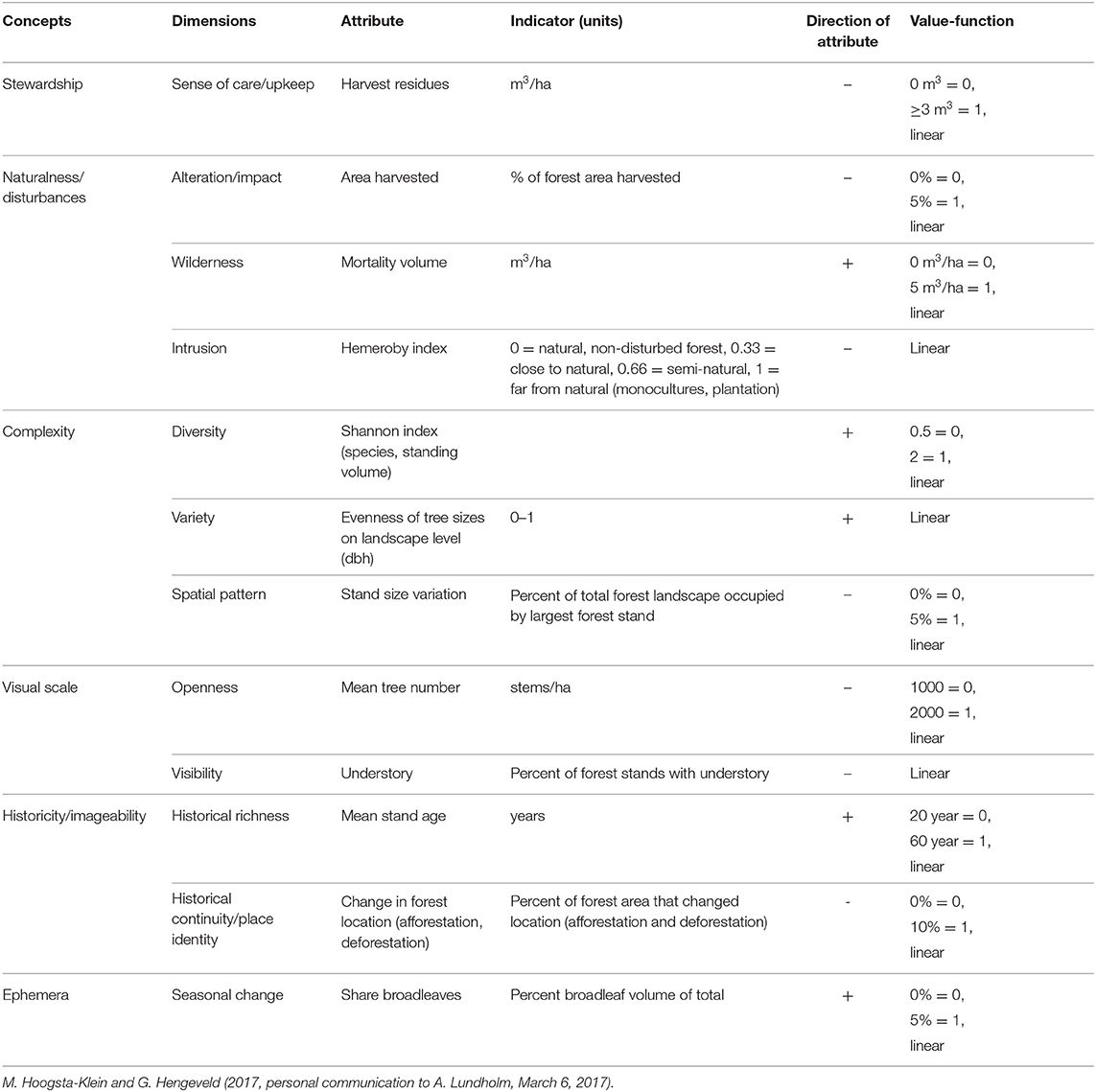
Table 1. Overview of operationalisation of indicators and attributes for all dimensions and concepts, including the specific value functions to determine upper and lower limits before averaging the score to create the RAFL-index.
Water Quality
The Source Load Apportionment Model framework, developed by Mockler et al. (2017), to measure nutrient emissions of N and P from different land-use areas in Ireland was utilised for the water quality ES indicator. The published framework, as well as unpublished work by Mockler, was implemented in the FMDSS to model long-term forestry impacts on water quality as well as background emission levels (Supplementary Table 7). The emission values were landscape-level average values, regardless of where in the landscape the land parcel was located, e.g., adjacent to or remote from watercourses. The FMDSS reported on emission rates both as total nutrient loads year−1 and average nutrient loads ha−1 year−1, for the forest and for the entire CSA, respectively.
Cultural
The Recreation Aesthetics Forest Landscape (RAFL) index was used as the cultural ESs indicator (Nieuwenhuis and Nordström, 2017). The index framework was largely based on four abstraction levels: concept—dimension—attribute—indicator, identified by Tveit et al. (2006). The concepts were based on perceived preferred forest structures to recreationalists, drawing from findings on scenic quality of landscapes (Tveit et al., 2006; Ode et al., 2008) and scenic beauty of forests (Edwards et al., 2012; Giergiczny et al., 2015). The attribute indicators were scaled to have equal impact on the RAFL index, by determining upper and lower limits of the indicator, and if they had a negative of positive impact on the index (Table 1). The attributes belonging to the same concept were then averaged to a concept score, and, finally, all concepts were averaged into the RAFL-index. Most attributes were determined by the forest average values from the yield tables, the Hemeroby index and understory attributes were landscape averages based on values assigned to different forest stand types, the Shannon Index and evenness of tree size on landscape level were calculated using landscape average values (Shannon, 1948; Whittaker, 1972; Mouillot and Leprêtre, 1999).
The Shannon index was calculated by multiplying the percentage merchantable volume of each species in the landscape with the natural logarithm of itself, these values were then added up and multiplied by −1 Equation (14).
where pvoli is the percentage of the merchantable volume of species i; plnvoli is the percentage of merchantable volume of species i multiplied by the natural logarithm of itself; and I is the number of forest species in the landscape at the start of the planning horizon.
The evenness of tree sizes on the landscape level was calculated by getting a percentage logarithmic estimate of each DBH class (Equation 15). These percentage logarithmic DBH class values were summed and divided by the natural logarithm of the number of diameter classes (Equation 16).
where DBH evenness is the evenness of tree sizes on the landscape level; I is the number of DBH classes; plnpDBHi is the proportion of the total volume in DBH class i multiplied by the natural logarithm of the proportion of the total volume in DBH class i; volDBHi is the volume in DBH class i; and VOLtot is the total volume in the forest landscape.
Results
Forest Composition and Age-Class
The main change in forest composition over the planning horizon was the replacement of Sitka spruce and other conifer stands with lodgepole pine on blanket peat sites (Figure 2). The area of lodgepole pine monocultures increased from around 26.0% in 2017 to 58.0, 62.2, 57.6, and 60.0% of the forest area by 2070 for BAU, S1, S2, and S3, respectively, and there was little or no change in forest composition after 2070. In the scenarios in which a smaller area was converted to lodgepole pine (i.e., BAU and S2), a larger area of non-lodgepole pine conifer stands was maintained on blanket peat. There was also a large change in total buffer zone area, which increased from 0.9% in 2017 to 5.3, 6.8, 6.4, and 6.8% of the forest area in 2070 for BAU, S1, S2, and S3, respectively (Figure 2). The age class distribution was largely affected by two major harvesting events around the years 2020 and 2070, which happened in all scenarios, but to a lesser degree in S3 (Figure 3). The area of old forest also differed between scenarios, being larger in the BAU scenario and in S2 (Figure 3 and Table 2).
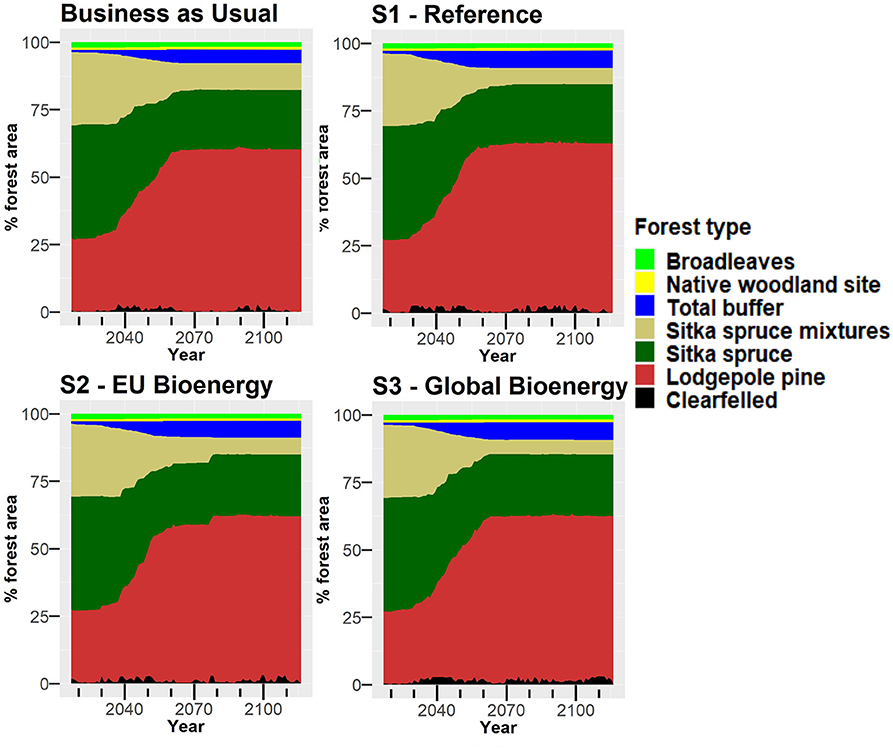
Figure 2. Percentage area by forest type for the four scenarios over the planning horizon. The Broadleaves group contains managed broadleaf forests. Native Woodland Sites are mainly unmanaged native broadleaf stands retained for their high biodiversity values. Sitka spruce mixtures contain all stands dominated by non-lodgepole pine conifers (including Sitka spruce), with broadleaves and/or non-lodgepole pine conifers as secondary or tertiary species. The Sitka spruce and lodgepole pine groups refers to monoculture stands of the respective species. Source: Lundholm et al. (2019).
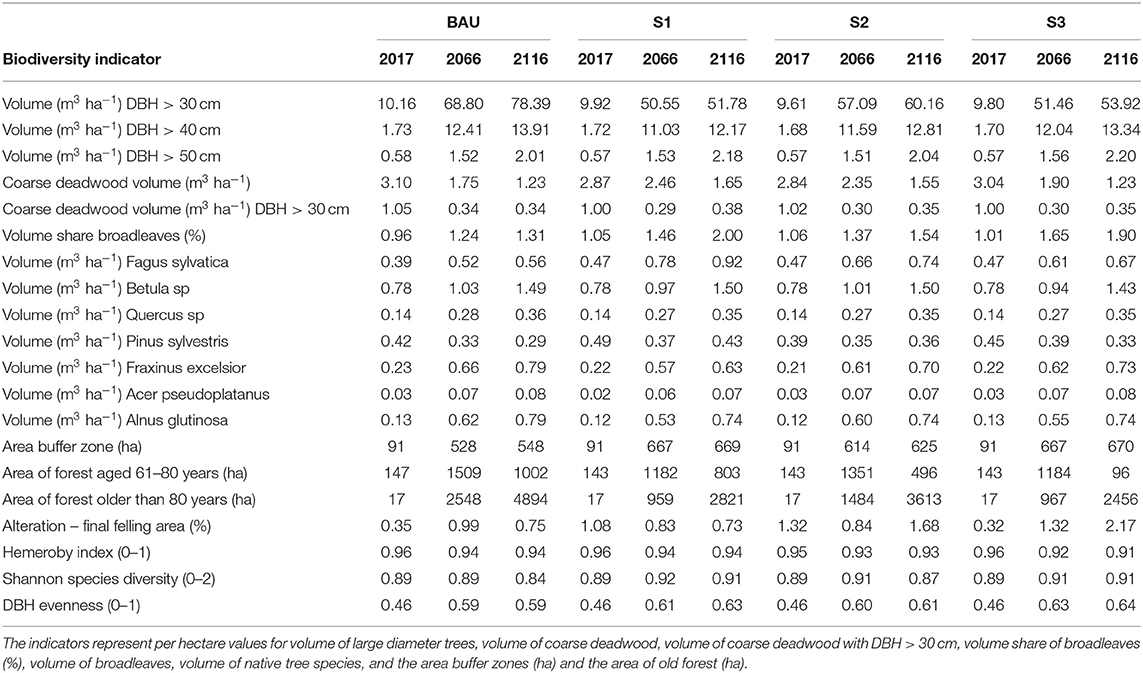
Table 2. Biodiversity indicators for the four scenarios, BAU, S1, S2, and S3, at three time points: 2017, 2066, and 2116.
Carbon
Relative to the model start year, the cumulative storage of carbon increased in the first 10 years to about 25 tons of carbon ha−1 for all scenarios. The increase was followed by an overall slow decline for the remainder of the planning horizon for all global scenarios (Figure 4). In the BAU scenario 37.0 tons of carbon was stored cumulatively in the first 20 years before the overall slow decline. The overall slow decline included a small increase in cumulatively stored carbon starting around 2060, which lasted until 2087, 2078, 2087, and 2070, for the BAU, S1, S2, and S3 scenarios, respectively. The final cumulatively stored carbon was 21.1, 7.6, 9.9, and −12.1 tons carbon ha−1 for the BAU, S1, S2, and S3 scenarios, respectively. To visualize the impact of drained peatlands on the cumulative carbon storage, the cumulative stored carbon indicator was also reported excluding the drained peat emissions. In that case, the cumulatively stored carbon per hectare increased in all scenarios, ending at 96.5, 83.0, 85.3, and 63.3 tons ha−1 over the 100-year planning horizon for the BAU, S1, S2, and S3 scenarios, respectively (Figure 4). The cumulative carbon storage by pools fluctuated over the planning horizon and differed slightly between scenarios, but the cumulative living carbon pool increased by 60.0, 32.7, 38.1, and 6.4 tons ha−1 for the BAU, S1, S2, and S3 scenarios, respectively. The cumulative storage in deadwood carbon increased by 5.3, 4.7, 5.8, and 6.9 tons ha−1 for the BAU, S1, S2, and S3 scenarios, respectively, and the cumulative storage of carbon in HWP was 13.2, 19.1, 15.8, and 18.7 tons ha−1 by 2116 for the four scenarios. The total displacement and total fossil fuel substitution over the planning horizon was 21.3, 30.1, 29.3, and 34.6 tons ha−1 for the BAU, S1, S2, and S3 scenarios, respectively, while the total loss of carbon due to drained peatlands over the planning horizon was 75.4 tons ha−1 for all four scenarios.
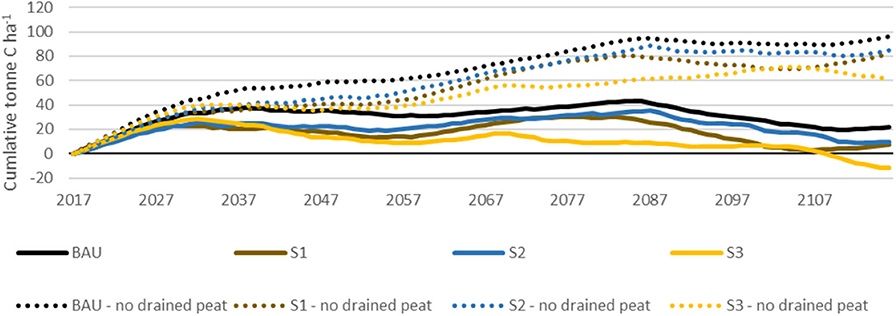
Figure 4. Cumulative stored carbon by year, in tons ha−1, for the four scenarios (solid lines), as well as for the four scenarios when emissions from drained peat soils are excluded (dashed lines).
Regulatory—Windthrow Risk
The analysis of the area of forest at high windthrow risk (≥70% probability that >3% of stems are windthrown, based on the windthrow risk model) showed a steep increase in the first decade for all scenarios, as much of the forest grew taller before being clearfelled (Figure 5). The scenarios started to diverge in terms of the high risk area around the year 2030 due to different harvest levels. Clearfelling was the only method to reduce the windthrow risk of a stand, and not all stands were eligible for clearfelling due to environmental regulations. The ‘fellable area’ with stands at high windthrow risk exhibited a similar pattern in terms of which scenarios resulted in the largest high risk area, but the total at risk area was lower (Figure 5). Based on the results for S3, circa 2,130 ha of non-fellable forests with a high risk of experiencing windthrow were present at the end of the planning horizon (Figure 5).
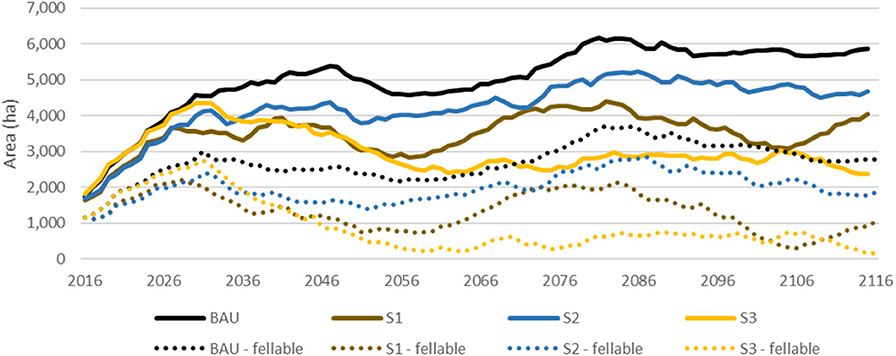
Figure 5. Forest area (solid) and fellable forest area (dashed) with a critical windthrow risk over 70%, for the BAU, S1, S2, and S3 scenarios.
Biodiversity
Volume Stored in Large Diameter Trees
The volume of large diameter trees per hectare increased in all scenarios, but more so in the BAU scenario compared to the scenarios where global impacts were implemented (Table 2). Around 80% of the total large diameter volume was stored in trees with DBH 30–40 cm, regardless of scenario. All volume measurements (DBH > 30 cm, DBH > 40 cm, DBH > 50 cm) increased by at least a factor of four in each scenario, and most of this increase had taken place by the planning horizon midpoint, the year 2066. The BAU scenario resulted in a greater volume per ha for trees with DBH > 30 cm than the other scenarios by the end of the planning horizon, i.e., 94.31, 66.13, 75.01, and 69.49 m3 ha−1 for the BAU, S1, S2, and S3 scenarios, respectively. However, at the end of the planning horizon all four scenarios produced almost an equal volume in trees with DBH > 40 cm (11.03-12.81 m3 ha−1). All scenarios resulted in a volume of trees with DBH > 50 cm between 2.01 and 2.18 m3 ha−1 at the end of the planning horizon.
Coarse Deadwood Volume From Natural Mortality
The total volume of coarse deadwood originating from natural mortality was more than halved in all scenarios over the planning horizon (Table 2). Most of this total decrease had already taken place by the planning horizon midpoint, i.e., year 2066. The same was true for large diameter (DBH >30 cm) coarse deadwood from natural mortality, i.e., the volume per hectare decreased by more than half in all scenarios. Total deadwood volume decreased from around 3.0 m3 ha−1 to 1.2–1.5 m3 ha−1, and the volume of large diameter deadwood decreased from around 1.0 m3 ha−1 to around 0.3 m3 ha−1, indicating very low levels of deadwood in the forest landscape, according to the model. Almost all the natural mortality volume originated from conifers, mainly Sitka spruce and lodgepole pine.
Broadleaf Volume and Native Tree Volume
The birch (Betula L.) volume increased steadily in all scenarios from 0.78 m3 ha−1 to around 1.45 m3 ha−1 by the end of the planning horizon, due to birch being planted in buffer zones (see section Area of Buffer Zones below). The other broadleaf species present in the forest landscape at least doubled their total volumes per hectare in all scenarios, or in the case of alder (Alnus glutinosa (L.) Gaertn.), quintupled it. Alder increased its volume from 0.12–0.13 m3 ha−1 to 0.74–0.79 m3 ha−1 due to this species being planted in buffer zones (see section Area of Buffer Zones below). The increase in volume for other broadleaf species was not the result of new planting but occurred due to existing stands growing older. However, apart from alder and birch, none of the other broadleaves ever reached more than 0.92 m3 ha−1 (beech (Fagus sylvatica L.) in year 2116 in the S1 scenario). Compared to a combined average conifer volume of around 200 m3 ha−1, broadleaves will have a very minor presence in the forest landscape. Ireland's only native commercial conifer, Scots pine (Pinus sylvestris L.), had its volume reduced in all scenarios and it was never higher than 0.5 m3 ha−1.
Area of Buffer Zones
The buffer zone area increased in all scenarios, from 91 ha in 2017 to 548, 669, 625, and 670 ha in 2116 for the BAU, S1, S2, and S3 scenarios, respectively (Table 2). The buffer zone area peaked around the year 2060 in all scenarios and was maintained for the remainder of the planning horizon. The requirement to establish buffer zones did not exist when most of the forest stands in CSA were established and they are thus retrofitted during subsequent management actions, mainly as 10–25 m wide water setbacks, sparsely planted with birch and alder, with varying width depending on soil type and slope.
Area of Old Forests
The area of forest older than 80 years increased in all scenarios but to very different levels at the end of the planning horizon: the total area increased from 17 ha in 2017, to 4,894 ha, 2,821 ha, 3,613 ha, and 2,456 ha in 2116 for BAU, S1, S2, and S3, respectively (Table 2). More forest area entered this older age class in the second half of the planning horizon (i.e., year 2066–2116) than in the first half.
Cultural Attributes
Alteration, i.e., the percent of forest clearfelled, varied over time, and the cultural attribute Hemeroby index decreased in all scenarios, from 0.96 to 0.91–0.94, indicating a slightly more natural forest landscape due to the buffer zones. The Shannon species diversity index did not change much but showed a slight reduction in the BAU and S2 scenarios, but a small increase in S1 and S3, due to more broadleaf volumes in buffer zones. DBH Evenness increased more in scenarios with more clearfelling (i.e., S1 and S3), as the distribution between volume stored in small and large diameter trees became more even.
Water Quality
P emissions from a site increased in the years following a clearfell, while N emissions remained static. Thus, the total P emission loads were higher in the scenarios with greater total clearfell area. Although forest stands emitted more nutrients per hectare, other land parcels in the landscape contributed to N and P loads in watercourses (Table 3). The S3 scenario resulted in the highest amount of P emissions, followed by the S1, S2, and BAU scenarios, in that order.
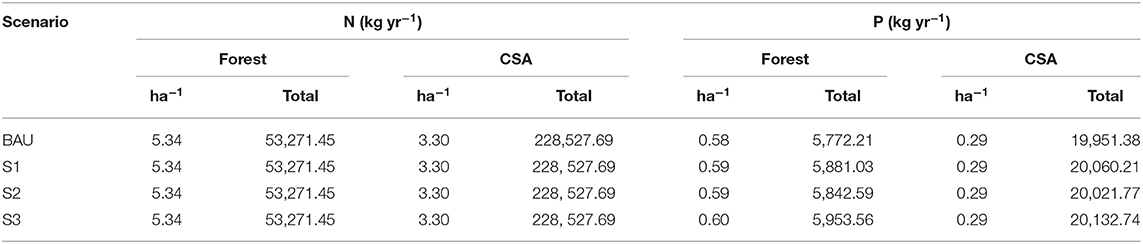
Table 3. Emission levels for N and P, for the forest (in kg yr−1 ha−1and kg yr−1) and the entire CSA (in kg yr−1 ha−1and kg yr−1), for the BAU, S1, S2, and S3 scenarios.
Cultural
All scenarios resulted in slight increases in the RAFL-index over the planning horizon, and although the index fluctuated over time, there were no large differences in the final index values between the scenarios. The RAFL-index increased from 0.50 in 2016 to 0.58, 0.58, 0.53, and 0.52 for BAU, S1, S2, and S3, respectively (Figure 6). The RAFL-index scores mainly changed due to a combination of changes in forest composition, clearfell areas, and the volumes of harvest residue in the forest landscape. Overall, all scenarios experienced very similar changes in forest composition but the total clearfell area differed greatly between scenarios—with respectively 61, 40, and 102% more total clearfell area in S1, S2, and S3 than in the BAU scenario.
Comparison of Ecosystem Services
The average supply of the ES indicators over the planning horizon was determined for the four modelled scenarios to evaluate and compare the levels of ESs, and to see if there were positive or negative correlations between them. Since the linear programming model operated on maximising NPV, the comparison of ES indicators was best made in relation to the NPV and clearfelling intensity in the scenarios, based on the results from a previous study (Lundholm et al., 2019), affected carbon storage, windthrow risk, broadleaf volume, P emissions, and RAFL-index (Table 4). The general trends were that cumulative carbon storage, windthrow risk area and RAFL-index decreased as clearfelling intensity increased, e.g., when comparing the BAU scenario with S3, a 61% increase in harvest volume resulted in 35% less carbon storage, 65% less fellable area at windthrow risk and a 7% lower RAFL-index. Although the scenarios that involved more harvesting had slightly higher P emissions, the absolute differences were small (3% higher P emission in S3 compared to the BAU scenario). More standing broadleaf volume was found in the scenarios with more harvesting (i.e., 15 and 17% more in S1 and S3, respectively, compared to the BAU scenario), although the actual differences were small.
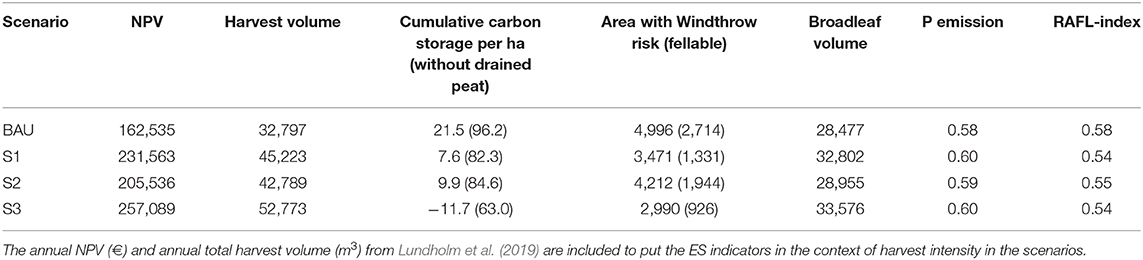
Table 4. Annual average ES indicator values for the four scenarios: cumulative carbon storage (tons ha−1) with and without (in brackets) the impact of drained peat soils, the total area at 70% windthrow risk (ha) and the fellable area (in brackets), total broadleaf volume (m3), average P emissions from forests (kg ha−1) and average RAFL index per hectare.
Discussion
This study integrated the external global factors climate change and dynamic timber prices, as well as ES indicators in a FMDSS, using an approach that modified yield tables already used in traditional forest management planning. The FMDSS Remsoft Woodstock is widely used around the world, and the modelling approach presented in this study could be integrated in the model of any forest company without requiring additional software or significant model overhauls, since the approach can simply be built into any existing Woodstock model that is oriented toward the optimisation of NPV and harvest volume. Although this model was applied to a CSA in Ireland, and locally relevant ES indicators were used, making the model results specific to the CSA and relevant to a wide group of local and national stakeholders, the basic methodology can be applied in any country or region. Of course, locally relevant ES indicators should be used wherever this approach is applied, e.g., local utilisation rates for HWP, prioritized regulatory services, relevant biodiversity indicators, etc.
External Impacts and Forest Composition
Climate change impacted on the growth rates of tree species and affected ES indicators that are based on stand volume measurements, e.g., many of biodiversity indicators and carbon, but the overall climate change impact on ES indicators was small. Determining the exact impact of external factors on ES indicators by comparing scenarios is difficult. Forest management in the scenarios differed as a response to the external factors and the largest impact on ESs was the level of clearfelling in the scenarios, which was mainly determined by the dynamic timber prices (Lundholm et al., 2019), a finding also confirmed using the same global scenario narratives in Lithuania (Mozgeris et al., 2019). Some correlations were found, where the greatest clearfell area (in the S3 scenario) resulted in more P emissions (which reduced water quality), and reductions in the area at windthrow risk, cumulative carbon storage, biodiversity indicators and RAFL-index. The opposite trend in ESs indicators was observed in the BAU scenario, which resulted in the smallest clearfell area. The results for the S1 and S2 scenarios fell somewhere between those for the BAU and S3 scenarios, both in terms of harvest level and the provision levels of the assessed ES indicators. Changes in forest composition also affected ES provision, but these changes were not only managerial responses the external factors (Dymond et al., 2016), forest policy also had a large influence. Due to certification rules and increased environmental considerations, peat sites could no longer be reforested using fertiliser. This was the reason for the landscape changing from dominated by Sitka spruce to dominated by lodgepole pine, as this is the only species that can be established on blanket peat without fertiliser (Figure 2). The other large change in landscape composition was the establishment of buffer zones. Stands were historically planted right up to the waterbodies, but since adopting SFM in 1996, buffer zones are being retrofitted during subsequent harvesting (DAFF, 1996). Differences in the age class distribution were due to clearfelling, which was a direct response to the external factors. Although additional afforestation, with enhanced biodiversity consideration, would be beneficial for most ES indicators, this was not a realistic option since the CSA is not suitable for afforestation (i.e., poor soils and many Natura2000 areas). Even if the land had been suitable for afforestation, studies have shown that the barriers to private landowners establishing forests are inflexibility of land management, lack of information, and the associated values and attitudes of farming and food production, rather than a lack of expected revenue (Duesberg et al., 2014a,b). Thus, increasing timber prices would have been unlikely to expand afforestation in Ireland. Furthermore, the uncertainties associated with the impacts of climate change on forestry may have a negative effect on landowners' willingness to afforest. On the other hand, potential new government policy to reduce Ireland's carbon emissions may result in the mandatory establishment of a forest area on every farm that receives subsidies.
Landscape-level management planning is the preferred and required scale for the modelling of the provision of multiple ES indicators when both the spatial and temporal interaction between stand types and forest management actions are included, as well as to allow for the involvement of multiple stakeholders (Marto et al., 2018). The objective of this study was to analyse the potential impacts on forest ESs from climate change and dynamic timber prices, rather than finding an optimal management schedule for the future that produces the best combination of ESs possible. Therefore, linear programming was considered a useful tool, as it allowed for the optimisation of a specific ES indicator (i.e., NPV) in a forest landscape, while also evaluating the associated provision levels of other ESs.
Carbon
Large amounts of carbon were sequestered in the BAU scenario, as large forest areas grew beyond normal clearfell age, proving that set-aside is an effective method for short-term carbon sequestration (Schwaiger et al., 2019). The forests became a carbon source in the S3 scenario, due to heavy clearfelling throughout the planning horizon. The other scenarios, S1 and S2, produced sequestration levels somewhere in the middle. Carbon emissions from drained blanket peat resulted in lowering the cumulative storage of carbon by 78, 91, 88, and 119% for the BAU, S1, S2, and S3 scenarios, respectively. Additionally, the normal utilisation scenarios (BAU and S1) stored more cumulative carbon than the climate change mitigation scenarios (S2 and S3). The carbon stocks were impacted by different utilisation rates; for instance, 10% of all pulpwood was utilised for bioenergy in the BAU and S1 scenarios, while the corresponding value for the S2 and S3 scenarios was 30%. Although this higher level resulted in less carbon being stored long-term in wood-based panels, it contributed to a reduction in the use of fossil fuels for heating and energy production, reducing Ireland's high dependency on imported fossil fuels, although biomass only supplies 2.3% of Ireland's total energy needs (Dineen et al., 2016). Based on the analysis of 21 studies, Sathre and O'Connor (2010) found that displacement factors for using wood in construction varied from −2.3 to 15, with a mean on 2.1 tons carbon per ton carbon in wood. Factors that determine the actual displacement factors were mainly end-of life use, i.e., bioenergy or landfill, but also harvest and processing efficiencies. Furthermore, differences in landfill management have a large impact on released CO2 and methane (Micales and Skog, 1997), which in some of the analyzed studies determined whether using wood products was a net sink or net source. Methodological differences mean there is a shortage of comparative studies for determining accurate carbon displacement factors, especially from utilizing wood for construction (Smyth et al., 2017). Therefore, there is some uncertainty associated with the results on fossil fuel substitution presented in this study, especially for the BAU and S1 scenarios, in which more wood in HWP was used.
Increased harvesting of biomass fuel could lead to shorter rotation periods if bioenergy species are planted, and extraction of more harvest residues, which decreases forest biodiversity (Verkerk et al., 2011; Duncker et al., 2012; Söderberg and Eckerberg, 2013). Since old-growth forests and coarse deadwood volume are important contributors to habitat provision and an indicator of forest health (Lassauce et al., 2012; Brockerhoff et al., 2017), increased biomass extraction for bioeconomy and climate change mitigation must be carefully considered against the potential trade-off of forest biodiversity. Verkerk et al. (2011) estimated that intensified bioenergy harvesting could cause a 5.5% reduction of deadwood in European forests between 2005 and 2030, whereas a business as usual scenario would increase deadwood volumes by 6.4% over the same time period. Utilizing European agricultural land for short rotation biomass crops would likely lead to increased food imports from developing countries, causing global biodiversity loss, as intensified land-use would remove species-rich habitats in the tropics (Di Fulvio et al., 2019). Alternatively to increasing bioenergy extraction, paying forest owners for creating carbon offset credits and accounting for carbon storage in HWP leads to longer rotation periods (Asante and Armstrong, 2012). However, if forest owners are also penalized for carbon emissions, there is a stronger incentive to clearfell old-growth forests to avoid natural disturbances (van Kooten, 2018), which would reduce the area of high biodiversity habitat. Thus, the trade-offs of mitigating climate change through utilizing wood products must be carefully considered, so as not to cause short-term habitat destruction and a reduction in biodiversity. Depending on how unmanaged forests will be affected by a changing climate will also determine whether it is a better climate mitigation strategy to harvest forests: will biomass growth increase as a result of more atmospheric CO2 acting as a fertiliser of forests (Houghton et al., 2001), or will increased catastrophic windthrow events, pests and diseases, wildfire (La Porta et al., 2008), and increased decomposition rates cause these forests to become carbon sources (Bradford et al., 2014)? Cannell (1999) acknowledged that although storing carbon in living trees increases the time to find other carbon storage and mitigation solutions, it does create a problem in that the reservoir of carbon can be released in the future through catastrophic events, and it limits the future management options for those forests.
Windthrow and Modelling Risk
Although, the methodology used to assess the carbon ES was a comprehensive and science-based method, it does require a closer investigation in relation to the windthrow ES indicator. Higher carbon storage was achieved by less clearfelling rather than storing carbon in HWP, e.g., compare the cumulative carbon storage and windthrow risk area in the BAU and S3 scenarios (Table 4). Most of Ireland's forests are heavily production oriented and carbon stored in HWP provides a substantial positive contribution to Ireland's greenhouse gas accounting (Green et al., 2006). Other forest carbon storage calculations have also found that more carbon was stored by utilizing wood for products with long storage lives than to indefinitely store carbon in unmanaged forests (Cannell, 1999). Over time, strong wind coupled with overall increased disturbances from climate change would likely cause endemic and catastrophic windthrow, not only in unmanaged western peatland forests but also in many European forests, resulting in a loss of productivity and decaying deadwood that releases carbon, instead of large stocks of living carbon (Senf and Seidl, 2020). Thus, the BAU scenario most likely overestimated the amount of sequestered carbon stock in living biomass. Since tree height, soil type, elevation, and exposure are important factors in determining windthrow risk (Lynch, 1985; Miller, 1985; Ní Dhubháin et al., 2009), it is very unlikely that indefinite retention of coniferous blanket peat forests should be part of a successful carbon storage strategy (Seidl et al., 2014). However, including the initial and subsequent impact of windthrow damage in the model is not simple, and stand volume cannot be reduced by windthrow risk alone. Subsequent windthrow damage increases as stand edges are reshaped and the internal structure of the stand changes, both as a result of natural disturbances and management actions, such as thinning and clearfelling of adjacent stands (Montoro Girona et al., 2019). Even though the current (i.e., at model start year) stand stocking, and indirectly stand volume, in Coillte's Woodstock model is reduced based on windthrow recorded during forest inventories, and these data are continuously updated, the potential impacts of future windthrow damage is not included in their model. The windthrow risk model, used in this study, only estimated the probability that at least 3% of the stems in a stand have been damaged by windthrow—it made no assumptions on the actual proportion of windblown trees or how a stand with high windthrow risk would be affected during subsequent years.
Monte Carlo simulation is often used in forest modelling to evaluate the potential impact of natural disturbances (Davis and Keller, 1997). A Canadian study that modelled the average annual forest area affected by wildfire found that Monte Carlo simulation resulted in highly imprecise annual estimates, even though long time series were available (Armstrong, 1999), and this might also be true for windthrow damage. However, Monte Carlo simulation can only be utilised in Woodstock models that use simulation, and not those that use linear programming (Walters, 1993). To properly include the impact of windthrow, it might be better to adjust the yield tables or include a mandatory windthrow action, where in a certain percentage of the stands the stocking is lowered. However, this method would need to utilise a generalised damage level, instead of the irregular nature of catastrophic windthrow events and the individualised windthrow damage at a stand level (Scott and Mitchell, 2005). On the other hand, spatial specificity to reflect increased windthrow damage in stands adjacent to clearcuts or heavily wind damaged stands would likely increase the accuracy in modelling such damage at the landscape level (Seidl et al., 2009).
Biodiversity Impacts
Except for coarse deadwood volume, all biodiversity indicators increased in all modelled scenarios. The biodiversity indicators were not greatly impacted by the global scenarios since they were not directly influenced by the objective function. The fact that the biodiversity indicators were not greatly reduced in any of the global scenarios indicates that initial indicator values in the forest landscape were low to begin with, which is often the case in production oriented forest landscapes dominated by exotic tree species (Marto et al., 2018). The increases were largely due to additional broadleaf volumes resulting from the creation of buffer zones and more large diameter trees in older stands, either due to their protection status or as a result of the unprofitability of their clearfelling and future management. Unprofitable forests and protection status also caused the area of old forest to increase in all scenarios. Natural mortality volumes decreased in all scenarios, and almost all coarse deadwood in the landscape originated from coniferous trees. The yield tables used for broadleaves did not include natural mortality as they were based on intensively managed forests where trees were thinned out before natural mortality could take place. The yield tables used for conifers included more natural mortality associated with unthinned Sitka spruce stands (which most of the Sitka spruce stands in the CSA were), whereas lodgepole pine stands produced more harvest residue during clearfelling, for stands of the same age on the same site. Thus, most of the reduction in coarse deadwood volume was due to the replacement of Sitka spruce with lodgepole pine, since aboveground deadwood from harvest residues accounted for only around 10% of all aboveground deadwood. High levels of biodiversity ES have been found to contribute to improvements in the provision of many other ESs (Lefcheck et al., 2015), especially with regards to overall ecosystem multifunctionality rather than individual ESs (Hector and Bagchi, 2007; Gamfeldt et al., 2008). Although this blanket peat dominated study landscape is very limited in its ability to grow a wide range of tree species, studies have found that even small increases in tree diversity contribute to increased ecosystem multifunctionality (Van Der Plas et al., 2016). Further, Duncker et al. (2012) found that modified forest management can have positive effects on biodiversity at fairly low costs. Thus, sacrificing only a small amount of NPV by implementing relatively minor management changes can lead to increased biodiversity and multifunctionality of Ireland's western peatland forests.
Water Quality
Water quality was assessed based on N and P emissions. The N emissions were not changed by forest management actions, but the P emissions were assumed to increase for 4 years after clearfelling before returning to previous levels. Thus, water quality was indirectly negatively affected by higher timber assortment prices, as these led to an increase in the total clearfell area in the S1 and S3 scenarios. However, even in the absence of clearfelling, the forests and all other land-use parcels in the CSA emit a background amount of P. Whether the emitted P would actually significantly impact the ecological status of downstream rivers and lakes depends on water discharge rates, other diffuse and point sources of P in the landscape, which catchments were affected, the temporal distribution of P, as well as the ecological threshold and current status of the waterbody receiving the additional P (Cummins and Farrell, 2003; Mockler et al., 2017). Some of these factors could be included in a Woodstock landscape management model, but others are much more difficult to capture, especially since most P is emitted from forests during heavy rainfall events (Rodgers et al., 2012). The P emission values from the Source Load Apportionment Model framework were area averages and applied to all land parcels, regardless of slope and distance to watercourses. In reality, harvesting sites close to watercourses release more P into the watercourse, but these additional P emissions could be avoided by increasing the width of buffer zones, especially in areas receiving more overland water flow (Ó hUallacháin, 2014). However, buffer zones on blanket peat sites, which dominate in the CSA, are unlikely to sequester large amount of nutrients, especially P (Kelly-Quinn et al., 2016). Proper planning and implementation of forest operations in sensitive catchments, such as avoiding tracks near the watercourses, are paramount to limiting nutrient emission runoffs. The methodology presented here can easily be integrated into Coillte's Woodstock model to produce rough estimates of the long-term P emissions at a catchment and sub-catchment level, which are required for FSC certification (FSC, 2012). Furthermore, governmental authorities implementing the Water Framework Directive could utilise this method to assess total nutrient emissions from all land-uses in a catchment (forestry, agriculture, and other point and diffuse sources), not just the current level, but also future emissions based on rural development scenarios.
Cultural Services
Overall there was a small increase in RAFL-index values over the planning horizon in all scenarios, but there were no large differences between the global scenarios. The increase was mainly due to increased buffer zone areas that also increased the broadleaf volume, and an increased area of over-mature forest. The change from Sitka spruce to lodgepole pine caused no major change in either landscape aesthetics or Hemeroby-index, as both species are exotic conifers, but it did affect the stewardship score. The volume of harvest residue from clearfelled lodgepole pine is higher than the volume of harvest residue from Sitka spruce according to the yield tables, and this factor negatively affects the aesthetics of forests (Edwards et al., 2012). In contrast, unthinned Sitka spruce contained more natural mortality volume than unthinned lodgepole pine, and as natural mortality decreased in the landscape, the wilderness score decreased. Changes in the stewardship score were the main reason for the fluctuations in RAFL-index changes over the planning horizon and were due to differences in the size and temporal distribution of clearfell areas between the scenarios. Clearfelling followed by reforestation increased the number of trees per hectare, reduced the stand age and increased the volume of harvest residues—factors that all contributed to temporarily lowering the RAFL-index. It is important to note that the limits for the RAFL-index attributes were set subjectively and were based on achievable values within the CSA. For example, the maximum share of broadleaves was set to 5% as this is what would be biophysically possible to achieve in the CSA, since the blanket peats, wet mineral soils, mountainous areas and marginal agricultural land are not suitable for broadleaves. The CSA is representative for the western half of Ireland, where much public afforestation was done in the 20th century. For Ireland as a whole, the maximum share of broadleaves would more appropriately be set somewhere in the range of 55–67%, based on the soil types in the current forest estate, although only around 33% of the national estate would have commercial potential for broadleaves (Forest Service, 2018). Finally, the RAFL-index was based on a landscape average and ignored the likelihood that local areas might have high aesthetic values, where recreation activities could be concentrated.
Management Implications and the Improvement of ES Provision Levels
High levels of carbon, regulatory, biodiversity, and cultural ES indicators and low levels of P emissions were all achieved by not clearfelling any trees, allowing forests to mature, as in the BAU scenario. However, due to the windthrow instability of blanket peat forests and the fact that stands that have been opened up by initial windthrow are likely to experience more windthrow in subsequent years, many of these stands can be expected to have their standing volume reduced (Montoro Girona et al., 2019), which would reduce the provision levels of most ESs, and possibly shorten rotation periods due to salvage felling. Therefore, it is necessary, for a better utilisation of the land, for forest managers to look outside the box for new types of management intervention in these stands. Management actions such as planting low stocked forests, restoring bog habitat and promoting natural regeneration of native vegetation could be used to redesign many blanket peatland forests. Such actions could result in long-term increases in biodiversity, cultural services, and water quality from the forest landscape, compared to the results of this study. Additionally, this would reduce the overall windthrow risk by clearfelling more forest stands, which would avoid the negative impacts of having over-mature conifer trees falling into watercourses and impacting water ecology (Lynch et al., 1985), or unmanaged stands becoming a breeding ground for bark beetles in the future (Weslien and Schroeder, 1999). Therefore, it is advisable to decommission most Sitka spruce stands on blanket peat and harvest most standing Sitka spruce timber, recovering most of the extractable value (Lundholm et al., 2019). Sitka spruce is expected to suffer reduced growth due to climate change, and restrictive use of fertiliser in forestry makes it less likely as a reforestation option on peatlands in the future, further driving the argument to replace Sitka spruce with other species and, perhaps, move away from commercial forest management of many blanket peat forests. However, the profitability of peatland forests could improve if demand for biomass increases as part of climate change mitigation practices. In such a case, the best strategy could well be the continued management of forests on blanket peat sites with medium to high productivity. The future development of economically marginal peatland forests, their increasing windthrow risk, and how they should be managed are relevant issues to address for all Irish western peatland forest (Tiernan, 2008) and also for many forests along the Atlantic seaboard of western Europe.
Future Research
Some potential future research areas are: (1) inclusion of disturbance impacts; (2) investigation of the impact of the spatial resolution of climate change on the results; and (3) assessment of alternative forest management systems on peatlands. Not including the impact of disturbances risks skewing the results, so a better understanding of their long-term abiotic and biotic impacts on mortality and stand development is necessary to properly assess the future provision of ESs. Climate change impacts vary locally and regionally, meaning large-scale climate models often have too low a resolution to provide detailed enough information for proper decision making (Koca et al., 2006). The Climadapt climate change data, used in this study, is scaled down from low-resolution projections (Ray et al., 2009). Thus, the forecasting precision and accuracy of the climate change impacts on species suitability and productivity could be improved by using higher resolution climate data. This aspect refers not only to the CSA, but generally to the resolution of climate change data that should be used in forecasting studies in the whole of Ireland, in Europe, and globally. Alternative management of Ireland's peatlands has been proposed both in this study and by other authors (Tiernan, 2008; Renou-Wilson and Byrne, 2015). Before initiating the redesign of the forested landscape, the expected ES provision resulting from the use of alternative forest management systems should be carefully modelled. It is also be important to establish tests sites to increase our knowledge of suitable alternative management systems, especially regarding the natural development of clearfelled sites, the development of low-stocked stands, and the cost-effective potential to seed or plant areas with native broadleaf species for biodiversity.
Conclusion
The Remsoft Woodstock based ALTERFOR FMDSS was used to model climate change and dynamic prices in Ireland by using modifiers on volume and price outputs, meaning that yield tables did not have to be changed, but the availability of reliable data is essential to get realistic results. Although the modelling framework presented here can be used to compare long-term ES provisions between regions and countries, the model results presented in this study are only applicable to Ireland's western peatland forest landscape. The model objective was to maximise NPV, and, as a result, this indicator was most affected by the global scenarios. The ES indicator values varied between the scenarios, mainly due to the level of clearfelling, which was affected by the global scenario impacts, especially the changes in assortment prices. The largest differences in ES indicator values between scenarios were observed in carbon storage and windthrow risk, with smaller differences for biodiversity, water quality and cultural services. The scenarios exhibited the same overall trends, due to the nature of the linear programming model and its objective function. Biophysical limitations, e.g., the poor soil conditions, and policy restrictions, e.g., the prohibition on aerial fertilisation, made lodgepole pine the only eligible reforestation species on blanket peat soils, which dominated the results for the scenarios. Recently introduced forest policy led to larger buffer zone areas and, consequently a smaller productive forest area, but impacted positively on several ES indicators. Single objective optimisation is not the best method to analyse the complex interactions between the ES indicators. However, the aims of this study were to analyse forest management impacts on ESs indicators under global scenarios and not to find the best possible combination of ES provision levels. Therefore, linear programming was an appropriate tool to use in this study, as well for the subsequent analysis of the impact of alternative management actions on ESs.
Data Availability Statement
All datasets generated for this study are included in the article/Supplementary Material.
Author Contributions
MN: conceptualization and funding acquisition. AL, KB, EC, and MN: methodology. AL, KB, and EC: software. AL: formal analysis, data curation, writing–original draft preparation, and visualization. AL and EC: investigation. AL, KB, EC, and MN: writing–review and editing. All authors: contributed to the article and approved the submitted version.
Funding
This project has received funding from the European Union's Horizon 2020 research and innovation programme under grant agreement No 676754. Responsibility for the information and views set out in this article/publication lies entirely with the authors.
Conflict of Interest
The authors declare that the research was conducted in the absence of any commercial or financial relationships that could be construed as a potential conflict of interest.
Acknowledgements
We would like to thank our colleagues in the ALTERFOR project, specifically the Ecosystem Service coordinators (Matts Lindbladh and Adam Fenton) for their advice on Ecosystem Service indicators, as well as Eva Mockler for help on the water quality methodology. We would also like to thank the Coillte staff who contributed with forest data, growth and yield tables, forest management action eligibility, and associated costs: Liam Malone, John Landy, Paul Ruane, Tony Clarke and Frank Flanagan.
Supplementary Material
The Supplementary Material for this article can be found online at: https://www.frontiersin.org/articles/10.3389/fevo.2020.00200/full#supplementary-material
References
Armstrong, G. W. (1999). A stochastic characterisation of the natural disturbance regime of the boreal mixedwood forest with implications for sustainable forest management. Canadian J. Forest Res. 29, 424–433. doi: 10.1139/x99-010
Asante, P., and Armstrong, G. W. (2012). Optimal forest harvest age considering carbon sequestration in multiple carbon pools: a comparative statics analysis. J. Forest Econ. 18, 145–156. doi: 10.1016/j.jfe.2011.12.002
Balvanera, P., Pfisterer, A. B., Buchmann, N., He, J. S., Nakashizuka, T., Raffaelli, D., et al. (2006). Quantifying the evidence for biodiversity effects on ecosystem functioning and services. Ecol. Lett. 9, 1146–1156. doi: 10.1111/j.1461-0248.2006.00963.x
Baskent, E. Z., and Keles, S. (2005). Spatial forest planning: a review. Ecol. Modell. 188, 145–173. doi: 10.1016/j.ecolmodel.2005.01.059
Bettinger, P., Boston, K., Siry, J. P., and Grebner, D. L. (2017). Forest Management and Planning. London, UK: Academic Press.
Bettinger, P., and Zhu, J. (2006). A new heuristic method for solving spatially constrained forest planning problems based on mitigation of infeasibilities radiating outward from a forced choice. Silva Fennica 40, 315–333. doi: 10.14214/sf.477
Biber, P., Borges, J. G., Moshammer, R., Barreiro, S., Botequim, B., Brodrechtová, Y., et al. (2015). How sensitive are ecosystem services in european forest landscapes to silvicultural treatment? Forests 6, 1666–1695. doi: 10.3390/f6051666
Bond-Lamberty, B., and Gower, S. T. (2008). Decomposition and fragmentation of coarse woody debris: re-visiting a boreal black spruce chronosequence. Ecosystems 11, 831–840. doi: 10.1007/s10021-008-9163-y
Bradford, M. A., Warren, R. J. II., Baldrian, P., Crowther, T. W., Maynard, D. S., Oldfield, E. E., et al. (2014). Climate fails to predict wood decomposition at regional scales. Nat. Clim. Chang. 4, 625–630. doi: 10.1038/nclimate2251
Brockerhoff, E. G., Barbaro, L., Castagneyrol, B., Forrester, D. I., Gardiner, B., González-Olabarria, J. R., et al. (2017). Forest biodiversity, ecosystem functioning and the provision of ecosystem services. Biodivers. Conserv 66, 3005–3035. doi: 10.1007/s10531-017-1453-2
Byrne, K. A., and Legge, T. (2008). Sustainability of Irish forestry–current status and future prospects. Irish Forestry 65, 47–49. Available online at: https://journal.societyofirishforesters.ie/index.php/forestry/article/view/10009
Cabrera Berned, A., and Nieuwenhuis, M. (2017). The potential impact of intensification on forest productivity under different climate change scenarios. Irish Forestry 74, 40–60. Available online at: https://journal.societyofirishforesters.ie/index.php/forestry/article/view/10817
Cannell, M. (1999). Growing trees to sequester carbon in the UK: answers to some common questions. Forestry 72, 237–247. doi: 10.1093/forestry/72.3.237
Corrigan, E., and Nieuwenhuis, M. (2016). A linear programming model to biophysically assess some ecosystem service synergies and trade-offs in two irish landscapes. Forests 7, 128–149. doi: 10.3390/f7070128
Corrigan, E., and Nieuwenhuis, M. (2017). Using goal-programming to model the effect of stakeholder determined policy and industry changes on the future management of and ecosystem services provision by ireland's Western peatland forests. Sustainability 9, 11–29. doi: 10.3390/su9010011
Cummins, T., and Farrell, E. P. (2003). Biogeochemical impacts of clearfelling and reforestation on blanket peatland streams i. Phosphorus. Forest Ecol. Managem. 180, 545–555. doi: 10.1016/S0378-1127(02)00648-5
Cunniffe, N. J., Koskella, B., Metcalf, C. J. E., Parnell, S., Gottwald, T. R., and Gilligan, C. A. (2015). Thirteen challenges in modelling plant diseases. Epidemics 10, 6–10. doi: 10.1016/j.epidem.2014.06.002
DAFF (1996). Growing For The Future—A Strategic Plan for the Development of the Forestry Sector in Ireland. Dublin: Stationery office.
Davis, T. J., and Keller, C. P. (1997). Modelling uncertainty in natural resource analysis using fuzzy sets and Monte Carlo simulation: slope stability prediction. Intern. J. Geograph. Inform. Sci. 11, 409–434. doi: 10.1080/136588197242239
Di Fulvio, F., Forsell, N., Korosuo, A., Obersteiner, M., and Hellweg, S. (2019). Spatially explicit LCA analysis of biodiversity losses due to different bioenergy policies in the European Union. Sci. Total Environ. 651, 1505–1516. doi: 10.1016/j.scitotenv.2018.08.419
Dineen, D., Howley, M., Holland, M., Cotter, E., and Glynn, J. (2016). Energy Security in Ireland: A Statistical Overview 2016 Report. Wilton Place, Dublin: Sustainabile Energy Authority of Ireland.
Duesberg, S., Dhubháin, Á. N., and O'Connor, D. (2014a). Assessing policy tools for encouraging farm afforestation in Ireland. Land Use Policy 38, 194–203. doi: 10.1016/j.landusepol.2013.11.001
Duesberg, S., O'Connor, D., N.í, Dhubháin, Á., and Upton, V. (2014b). Factors Influencing Irish Farmers' Afforestation Intentions. Forest Policy Econ. 39, 13–20. doi: 10.1016/j.forpol.2013.11.004
Duffy, P., Black, K., Hyde, B., Ryan, A. M., Ponzi, J., and Alam, S. (2018). Ireland National Inventory Report 2018. Wexford: EPA. Johnston Castle, Co.
Duncker, P. S., Raulund-Rasmussen, K., Gundersen, P., Katzensteiner, K., De Jong, J., Ravn, H. P., et al. (2012). How forest management affects ecosystem services, including timber production and economic return: Synergies and trade-offs. Ecol. Soc. 17, 50–67. doi: 10.5751/ES-05066-170450
Dymond, C. C., Beukema, S., Nitschke, C. R., Coates, K. D., and Scheller, R. M. (2016). Carbon sequestration in managed temperate coniferous forests under climate change. Biogeosciences 13, 1933–1947. doi: 10.5194/bg-13-1933-2016
Edwards, D. M., Jay, M., Jensen, F. S., Lucas, B., Marzano, M., Montagné, C., et al. (2012). Public preferences for structural attributes of forests: towards a pan-European perspective. Forest Policy Econ. 19, 12–19. doi: 10.1016/j.forpol.2011.07.006
Eriksson, L. O., and Borges, G. J. (2014). Computerized Decision Support Tools to Address Forest Management Planning Problems: History and Approach for Assessing the State of Art World-Wide Computer-Based Tools for Supporting Forest Management. The Experience and the Expertise World-Wide. (Umeå: Swedish University of Agricultural Sciences), 503. Available online at: https://pub.epsilon.slu.se/11417/ (accessed January 30 2018).
Forest Europe (1993). “Resolution H1 - general guidelines for sustainable forest management of forests in Europe,” in Second Ministerial Conference on the Protection of Forests in Europe.
Forest Service (2013). National Forest Inventory – Republic of Ireland – Results. Wexford: Forest Service. Johnstown Castle Estate.
Forest Service (2016). Environmental Requirements for Afforestation. Wexford: Department of Agriculture, Food and the Marine. Johnstown Castle Estate, Co.
Forest Service (2017). Felling and Reforestation Requierments. Wexford: Forest Service, Department of Agriculture, Food and the Marine, Ireland. Johnstown Castle Estate, Co.
Forest Service (2018). Ireland's National Forest Inventory 2017 – Results. Wexford: Forest Service, Department of Agriculture, Food and the Marine Johnstown Castle Estate.
Forsell, N., and Korosuo, A. (2016). Global and Country Specific Prospective Scenarios. ALTERFOR project website, 20. Available online at: https://www.alterfor-project.eu/deliverables-and-milestones.html (accessed January 23 2019) Milestone 6 of the ALTERFOR project (WP2).
Forsell, N., Korosuo, A., Havlík, P., Valin, H., Lauri, P., and Gusti, M. (2016). Study on Impacts on Resource Efficiency of Future EU Demand for Bioenergy (ReceBio). Final Report. Luxembourg: Publications Office of the European Union. Available online at: http://ec.europa.eu/environment/enveco/resource_efficiency/pdf/bioenergy/KH-02-16-505-EN-N%20-%20final%20report.pdf (accessed January 02 2019) Project: ENV.F.1/ETU/2013/0033.
Fricko, O., Havlik, P., Rogelj, J., Klimont, Z., Gusti, M., Johnson, N., et al. (2017). The marker quantification of the Shared Socioeconomic Pathway 2: a middle-of-the-road scenario for the 21st century. Global Environm. Change 42, 251–267. doi: 10.1016/j.gloenvcha.2016.06.004
FSC (2012). FSC Irish Forest Stewardship Standard. FSC National Initiative in Ireland New Ross, Co. Wexford, Ireland. Available online at: https://ic.fsc.org/file-download.fsc-forest-stewardship-standard-for-ireland.a-1686.pdf (accessed March 06 2019).
Gamfeldt, L., Hillebrand, H., and Jonsson, P. R. (2008). Multiple functions increase the importance of biodiversity for overall ecosystem functioning. Ecology 89, 1223–1231. doi: 10.1890/06-2091.1
Gamfeldt, L., Snäll, T., Bagchi, R., Jonsson, M., Gustafsson, L., Kjellander, P., et al. (2013). Higher levels of multiple ecosystem services are found in forests with more tree species. Nat. Commun. 4, 1–8. doi: 10.1038/ncomms2328
Giergiczny, M., Czajkowski, M., Zylicz, T., and Angelstam, P. (2015). Choice experiment assessment of public preferences for forest structural attributes. Ecol. Econom. 119, 8–23. doi: 10.1016/j.ecolecon.2015.07.032
Gómez-Baggethun, E., de Groot, R., Lomas, P. L., and Montes, C. (2010). The history of ecosystem services in economic theory and practice: from early notions to markets and payment schemes. Ecol. Econom. 69, 1209–1218. doi: 10.1016/j.ecolecon.2009.11.007
Green, C., Avitabile, V., Farrell, E. P., and Byrne, K. A. (2006). Reporting harvested wood products in national greenhouse gas inventories: implications for Ireland. Biomass Bioen. 30, 105–114. doi: 10.1016/j.biombioe.2005.11.001
Havlík, P., Valin, H., Herrero, M., Obersteiner, M., Schmid, E., Rufino, M. C., et al. (2014). Climate change mitigation through livestock system transitions. Proc. Natl. Acad. Sci. U.S.A. 111, 3709–3714. doi: 10.1073/pnas.1308044111
Hector, A., and Bagchi, R. (2007). Biodiversity and ecosystem multifunctionality. Nature 448, 188–190. doi: 10.1038/nature05947
Hennigar, C., Erdle, T., Gullison, J., and MacLean, D. (2013). Re-examining wood supply in light of future spruce budworm outbreaks: a case study in New Brunswick. Forestry Chron. 89, 42–53. doi: 10.5558/tfc2013-010
Hofer, R. (2009). “History of the sustainability concept – renaissance of renewable resources,” in Sustainable Solutions for Modern Economies, ed R. Hofer (Cambridge: The Royal Society of Chemistry), 1–11. doi: 10.1039/9781847552686-00001
Houghton, J. T., Ding, Y., Griggs, D. J., Noguer, M., van der Linden, P. J., Dai, X., et al. (2001). Climate Change 2001: The Scientific Basis. Cambridge: The Press Syndicate of the University of Cambridge.
IPCC (2006). 2006 IPCC Guidelines for National Greenhouse Gas Inventories, Prepared by the National Greenhouse Gas Inventories Programme. IPCC. IGES, Japan, Vol. 4: Agriculture, Forestryand Other Land Use. Chapter 4: Forest Land.
IPCC (2014). 2013 Revised Supplementary Methods and Good Practice Guidance Arising from the Kyoto Protocol. Intergovernmental Panel on Climate Change.
Keenan, P., Carroll, P., Conway, H., and Lee, S. (2017). “Spatial modelling of climate change in Irish forestry,” in Twenty-Third Americas Conference on Information Systems (Boston, MA), 1–9.
Kelly-Quinn, M., Bruen, M., Harrison, S., Healy, M., Clarke, J., Drinan, T., et al. (2016). HYDROFOR: Assessment of the Impacts of Forest Operations on the Ecological Quality of Water. EPA Research Report 169, 1–64. doi: 10.13140/RG.2.1.3141.0161
Koca, D., Smith, B., and Sykes, M. T. (2006). Modelling regional climate change effects on potential natural ecosystems in Sweden. Clim. Change 78, 381–406. doi: 10.1007/s10584-005-9030-1
La Porta, N., Capretti, P., Thomsen, I. M., Kasanen, R., Hietala, A. M., and Von Weissenberg, K. (2008). Forest pathogens with higher damage potential due to climate change in Europe. Can. J. Plant Pathol. 30, 177–195. doi: 10.1080/07060661.2008.10540534
Lassauce, A., Lieutier, F., and Bouget, C. (2012). Woodfuel harvesting and biodiversity conservation in temperate forests: effects of logging residue characteristics on saproxylic beetle assemblages. Biol. Conserv. 147, 204–212. doi: 10.1016/j.biocon.2012.01.001
Lefcheck, J. S., Byrnes, J. E., Isbell, F., Gamfeldt, L., Griffin, J. N., Eisenhauer, N., et al. (2015). Biodiversity enhances ecosystem multifunctionality across trophic levels and habitats. Nat. Commun. 6, 1–7. doi: 10.1038/ncomms7936
Lindner, M., Maroschek, M., Netherer, S., Kremer, A., Barbati, A., Garcia-Gonzalo, J., et al. (2010). Climate change impacts, adaptive capacity, and vulnerability of European forest ecosystems. For. Ecol. Manage 259, 698–709. doi: 10.1016/j.foreco.2009.09.023
Lundholm, A., Corrigan, E., and Nieuwenhuis, M. (2019). Implementing climate change and associated future timber price trends in a decision support system designed for irish forest management and applied to Ireland's Western peatland forests. Forests 10, 270–295. doi: 10.3390/f10030270
Lundmark, T., Bergh, J., Nordin, A., Fahlvik, N., and Poudel, B. C. (2016). Comparison of carbon balances between continuous-cover and clear-cut forestry in Sweden. Ambio 45, 203–213. doi: 10.1007/s13280-015-0756-3
Lynch, J. A., Corbett, E. S., and Mussallem, K. (1985). Best management practices for controlling nonpoint-source pollution on forested watersheds. J. Soil Water Conserv. 40, 164–167.
Lynch, T. (1985). “Windthrow damage in research plots,” in Proceedings IUFRO Symposium on The Influence of Spacing and Selectivity in Thinning on Stand Development, Operations and Economy (Dublin: Project Group), 4.
Marques, S., Marto, M., Bushenkov, V., McDill, M., and Borges, J. (2017). Addressing wildfire risk in forest management planning with multiple criteria decision making methods. Sustainability 9, 298–315. doi: 10.3390/su9020298
Marto, M., Reynolds, K., Borges, J., Bushenkov, V., and Marques, S. (2018). Combining decision support approaches for optimizing the selection of bundles of ecosystem services. Forests 9, 438–452. doi: 10.3390/f9070438
Micales, J. A., and Skog, K. E. (1997). The decomposition of forest products in landfills. Intern. Biodeter. Biodegrad. 39, 145–158. doi: 10.1016/S0964-8305(97)83389-6
Mockler, E. M., Deakin, J., Archbold, M., Gill, L., Daly, D., and Bruen, M. (2017). Sources of nitrogen and phosphorus emissions to Irish rivers and coastal waters: Estimates from a nutrient load apportionment framework. Sci. Total Environm. 601, 326–339. doi: 10.1016/j.scitotenv.2017.05.186
Montoro Girona, M., Morin, H., Lussier, J.-M., and Ruel, J.-C. (2019). Post-cutting mortality following experimental silvicultural treatments in unmanaged boreal forest stands. Front. Forests Global Change 2:4. doi: 10.3389/ffgc.2019.00004
Moorkens, E., Purser, P., Wilson, F., and Allott, N. (2013). Forestry Management for the Freshwater Pearl Mussel Margaritifera Final Report - FORMMAR (University of Dublin, Trinity College), 21. Available online at: https://www.mdpi.com/1999-4907/7/7/128 (accessed January 02 2019).
Mouillot, D., and Leprêtre, A. (1999). A comparison of species diversity estimators. Res. Popul. Ecol. (Kyoto) 41, 203–215. doi: 10.1007/s101440050024
Mozgeris, G., Brukas, V., Pivoriunas, N., Cinga, G., Makrickiene, E., Byčenkiene, S., et al. (2019). Spatial pattern of climate change effects on lithuanian forestry. Forests 10, 809. doi: 10.3390/f10090809
Ní Dhubháin, Á., Bulfin, M., Keane, M., Mills, P., and Walshe, J. (2009). The development and validation of a windthrow probability model for Sitka spruce in Ireland. Irish Forestry 66, 74–84. Available online at: https://journal.societyofirishforesters.ie/index.php/forestry/article/view/10024
Nieuwenhuis, M., and Nordström, E.-M. (2017). Milestone 9 - Quality Standards to be Met by the Up-Graded DSSs. ALTERFOR Milestone Report. Available online at: https://alterfor-project.eu/files/alterfor/download/Deliverables/D1.1/D1.1_FMM_I_II_Introduction.pdf (accessed September 30 2019).
Nieuwenhuis, M., and Williamson, G. P. (1993). Harvesting coillte's forests: the right tree at the right time. Irish Forestry 50, 122–133.
Nobre, S., Eriksson, L.-O., and Trubins, R. (2016). The use of decision support systems in forest management: analysis of FORSYS Country reports. Forests 7, 72–90. doi: 10.3390/f7030072
Nordström, E.-M., Nieuwenhuis, M., Başkent, E. Z., Biber, P., Black, K., Borges, J. G., et al. (2019). Forest decision support systems for the analysis of ecosystem services provisioning at the landscape scale under global climate and market change scenarios. Eur. J. For. Res 138, 1–21. doi: 10.1007/s10342-019-01189-z
Ó hUallacháin, D. (2014). Wider riparian buffer strips: a cost-effective conservation measure for freshwater pearl mussels in Ireland? Biol. Environ. 114B, 101–107. doi: 10.3318/bioe.2014.12
OCarroll, N. (2004). Forestry in Ireland: A Concise History. Arena House, Arena Road, Sandyford, Dublin 18, Ireland: National Council for Forest Research and Development (COFORD).
Ode, Å., Tveit, M. S., and Fry, G. (2008). Capturing landscape visual character using indicators: touching base with landscape aesthetic theory. Lands. Res. 33, 89–117. doi: 10.1080/01426390701773854
Öhman, K., and Wikström, P. (2008). Incorporating aspects of habitat fragmentation into long-term forest planning using mixed integer programming. Forest Ecol. Managem. 255, 440–446. doi: 10.1016/j.foreco.2007.09.033
Olajuyigbe, S. O., Tobin, B., Gardiner, P., and Nieuwenhuis, M. (2011). Stocks and decay dynamics of above and belowground coarse woody debris in managed sitka spruce forests in Ireland. For. Ecol. Manage 262, 1109–1118. doi: 10.1016/j.foreco.2011.06.010
Raudsepp-Hearne, C., Peterson, G. D., and Bennett, E. M. (2010). Ecosystem service bundles for analyzing tradeoffs in diverse landscapes. Proc. Natl. Acad. Sci. U.S.A. 107, 5242–5247. doi: 10.1073/pnas.0907284107
Ray, D., Xenakis, G., Semmler, T., and Black, K. (2008). The Impact of Climate Change on Forests in Ireland and Some Options for Adaptation. Dublin: COFORD.
Ray, D., Xenakis, G., Tene, A., and Black, K. (2009). Developing a site classification system to assess the impact of climate change on species selection in Ireland. Irish Forestry 66, 101–122. Available online at: https://journal.societyofirishforesters.ie/index.php/forestry/article/view/10026
Reid, W. V., Mooney, H. A., Cropper, A., Capistrano, D., Carpenter, S. R., Chopra, K., et al. (2005). Ecosystems and Human Well-Being-Synthesis: A Report of the Millennium Ecosystem Assessment. Tullamore: Island Press.
Renou, F., and Farrell, E. P. (2005). “Reclaiming peatlands for forestry: the Irish experience,” in Restoration of Boreal and Temperate Forests, ed J. A. Stanturf. (Boca Raton, FL: CRC Press), 541–557. doi: 10.1201/9780203497784.ch34
Renou-Wilson, F., and Byrne, K. A. (2015). “Irish peatland forests: lessons from the past and pathways to a sustainable future,” in Restoration of Boreal and Temperate Forests, ed J. A. Stanturf (Boca Raton, FL: CRC Press), 321–335.
Reynolds, K. M. (2005). Integrated decision support for sustainable forest management in the United States: fact or fiction? Comp. Electron. Agric. 49, 6–23. doi: 10.1016/j.compag.2005.02.002
Reynolds, M. K., Twery, M., Lexer, J. M., Vacik, H., Ray, D., Shao, G., et al. (2008). “Decision support systems in forest management,” in Handbook on Decision Support Systems 2: Variations. (Heidelberg: Springer Berlin Heidelberg), 499–533. doi: 10.1007/978-3-540-48716-6_24
Rodgers, M., O'Connor, M., O'Driscoll, C., Asam, Z.-,u,.-Z., Muller, M., and Xiao, L. (2012). Phosphorus Release from Forest Harvesting on an Upland Blanket Peat. For. Ecol. Manage 260, 2241–2248. doi: 10.1016/j.foreco.2010.09.037
Saad, C., Boulanger, Y., Beaudet, M., Gachon, P., Ruel, J.-C., and Gauthier, S. (2017). Potential impact of climate change on the risk of windthrow in eastern Canada's forests. Clim. Change 143, 487–501. doi: 10.1007/s10584-017-1995-z
Sathre, R., and O'Connor, J. (2010). Meta-analysis of greenhouse gas displacement factors of wood product substitution. Environm. Sci. Policy 13, 104–114. doi: 10.1016/j.envsci.2009.12.005
Schwaiger, F., Poschenrieder, W., Biber, P., and Pretzsch, H. (2018). Species mixing regulation with respect to forest ecosystem service provision. Forests 9:632. doi: 10.3390/f9100632
Schwaiger, F., Poschenrieder, W., Biber, P., and Pretzsch, H. (2019). Ecosystem service trade-offs for adaptive forest management. Ecosyst. Serv. 39:100993. doi: 10.1016/j.ecoser.2019.100993
Scott, R. E., and Mitchell, S. J. (2005). Empirical modelling of windthrow risk in partially harvested stands using tree, neighbourhood, and stand attributes. For. Ecol. Manage 218, 193–209. doi: 10.1016/j.foreco.2005.07.012
Seidl, R., Schelhaas, M.-J., Lindner, M., and Lexer, M. J. (2009). Modelling bark beetle disturbances in a large scale forest scenario model to assess climate change impacts and evaluate adaptive management strategies. Reg. Environm. Change 9, 101–119. doi: 10.1007/s10113-008-0068-2
Seidl, R., Schelhaas, M.-J., Rammer, W., and Verkerk, P. J. (2014). Increasing forest disturbances in Europe and their impact on carbon storage. Nat. Clim. Change 4, 806–810. doi: 10.1038/nclimate2318
Senf, C., and Seidl, R. (2020). Mapping the coupled human and natural disturbance regimes of Europe's forests. bioRxiv [Preprint]. doi: 10.1101/2020.03.30.015875
Shannon, C. E. (1948). A mathematical theory of communication. Bell System Techn. J. 27, 379–423. doi: 10.1002/j.1538-7305.1948.tb01338.x
Smyth, C., Rampley, G., Lemprière, T. C., Schwab, O., and Kurz, W. A. (2017). Estimating product and energy substitution benefits in national-scale mitigation analyses for Canada. Gcb Bioenergy 9, 1071–1084. doi: 10.1111/gcbb.12389
Söderberg, C., and Eckerberg, K. (2013). Rising policy conflicts in Europe over bioenergy and forestry. Forest Policy Econ. 33, 112–119. doi: 10.1016/j.forpol.2012.09.015
Teagasc (2019). Farm Forestry: Land Availability, Take-Up Rates and Economics [Online]. Carlow, Ireland: Teagasc. Available online at: https://www.teagasc.ie/crops/forestry/research/land-availability-take-up-rates-and-economics/ (accessed January 22 2019).
Tiernan, D. (2007). Environmental and social enhancement of forest plantations on western peatlands-a case study. Irish Forestry 64, 5–16. Available online at: https://journal.societyofirishforesters.ie/index.php/forestry/article/view/9993
Tiernan, D. (2008). Redesigning Afforested Western Peatlands in Ireland: (published in): After Wise Use - The Future of Peatlands. Irish Peatland Society.
Turner, R., and Daily, G. (2008). The ecosystem services framework and natural capital conservation. Environ. Res. Econ. 39, 25–35. doi: 10.1007/s10640-007-9176-6
Tveit, M., Ode, Å., and Fry, G. (2006). Key concepts in a framework for analysing visual landscape character. Landscape Res. 31, 229–255. doi: 10.1080/01426390600783269
Vacik, H., and Lexer, J. M. (2014). Past, current and future drivers for the development of decision support systems in forest management. Scand. J. Forest Res. 29, 2–19. doi: 10.1080/02827581.2013.830768
Van Der Plas, F., Manning, P., Allan, E., Scherer-Lorenzen, M., Verheyen, K., Wirth, C., et al. (2016). Jack-of-all-trades effects drive biodiversity–ecosystem multifunctionality relationships in European forests. Nat. Commun. 7, 1–11. doi: 10.1038/ncomms11109
van Kooten, G. C. (2018). The challenge of mitigating climate change through forestry activities: What are the rules of the game? Ecol. Econ. 146, 35–43. doi: 10.1016/j.ecolecon.2017.10.002
Verkerk, P., Lindner, M., Zanchi, G., and Zudin, S. (2011). Assessing impacts of intensified biomass removal on deadwood in European forests. Ecol. Indic 11, 27–35. doi: 10.1016/j.ecolind.2009.04.004
Walters, K. R. (1993). “Design and development of a generalised forest management system: Woodstock,” in International Symposium on Systems Analysis and Management Decisions in Forestry (Valdivia: Remsoft Inc.).
Weslien, J., and Schroeder, M. L. (1999). Population levels of bark beetles and associated insects in managed and unmanaged spruce stands. For. Ecol. Manage 115, 267–275. doi: 10.1016/S0378-1127(98)00405-8
Whittaker, R. H. (1972). Evolution and Measurement of Species Diversity. Taxon 21(2/3), 213–251. doi: 10.2307/1218190
Keywords: forest planning, Remsoft Woodstock, sustainable forest management, blanket peat, linear programming, afforested western peatlands
Citation: Lundholm A, Black K, Corrigan E and Nieuwenhuis M (2020) Evaluating the Impact of Future Global Climate Change and Bioeconomy Scenarios on Ecosystem Services Using a Strategic Forest Management Decision Support System. Front. Ecol. Evol. 8:200. doi: 10.3389/fevo.2020.00200
Received: 01 March 2020; Accepted: 02 June 2020;
Published: 08 July 2020.
Edited by:
Miguel Montoro Girona, Université du Québec en Abitibi Témiscamingue, CanadaReviewed by:
Maxence Martin, Université du Québec à Chicoutimi, CanadaNúria Aquilué Junyent, Centre Tecnològic Forestal de Catalunya (CTFC), Spain
Copyright © 2020 Lundholm, Black, Corrigan and Nieuwenhuis. This is an open-access article distributed under the terms of the Creative Commons Attribution License (CC BY). The use, distribution or reproduction in other forums is permitted, provided the original author(s) and the copyright owner(s) are credited and that the original publication in this journal is cited, in accordance with accepted academic practice. No use, distribution or reproduction is permitted which does not comply with these terms.
*Correspondence: Anders Lundholm, YW5kZXJzLmx1bmRob2xtQHVjZGNvbm5lY3QuaWU=