- 1CAS Key Laboratory of Coastal Environmental Processes and Ecological Remediation, Yantai Institute of Coastal Zone Research (YIC), Chinese Academy of Sciences (CAS), Yantai, China
- 2State Key Laboratory of Vegetation and Environmental Change, Institute of Botany, Chinese Academy of Sciences, Beijing, China
- 3World Agroforestry Centre (ICRAF), Eastern and Southern Africa Regional Office, Lusaka, Zambia
- 4Key Laboratory of Plant Resources and Biodiversity of Jiangxi Province, Jingdezhen University, Jingdezhen, China
There is still considerable debate about the relative importance of resource heterogeneity and resource quantity in the maintenance of species diversity in a community. The resource heterogeneity hypothesis proposes that spatial heterogeneity of limiting resources and inter-specific differences in resource requirements will determine species richness. In contrast, the resource quantity hypothesis predicts that average resource supply rates contribute to species richness by their effects on plant density and stochastic population dynamics. However, the evaluation of the two hypotheses in observational studies is associated with a major methodological challenge as average resource supply rate often covaries with resource heterogeneity. Using a novel approach derived from the relationships between average resource supply rate, resource heterogeneity (calculated as the standard deviation of environmental factors) and plant density in the resource hypotheses, we evaluated the relative importance of resource quantity and resource heterogeneity with a variation partitioning model in Gutianshan (GTS) forest plot, China, and Barro Colorado Island (BCI) forest plot, Central Panama. We found that resource quantity explained much less of the variation in species richness than resource heterogeneity in both GTS and BCI (44.5% vs. 4.9% in GTS and 20.4% vs. 0.8% in BCI at the 20 × 20 m scale, 57.5% vs. 3.4% in GTS at the 40 × 40 m scale, and 34.5% vs. 2.6% in BCI at the 50 × 50 m scale). We also found that resource heterogeneity governed species richness in GTS, whereas spatial processes dominated species diversity in BCI. Moreover, most of the effect of resource heterogeneity and resource quantity on species richness overlapped with that of spatial processes. This result indicates that most effects of resources could also be explained by spatial processes, such as dispersal limitation. Therefore, resource heterogeneity and spatial processes, but not resource quantity, played an important role in determining species diversity in these two old-growth forests. This is in contrast to the results of several manipulative studies, which found that resource quantity governed species diversity when one or a limited number of resources were considered. This suggests that the processes determining species richness along ecological gradients are complicated and determined by the interaction of various processes.
Introduction
Disentangling the importance of different processes in determining the species diversity of biotic communities has been an enduring challenge for ecologists (Ricklefs and Schluter, 1993; Abrams, 1995; Barot, 2004). For example, at opposite ends of a spectrum, the resource quantity hypothesis proposes that the species richness is determined by the average supply rates of limiting resources, through stochastic population dynamics (Wright, 1983; Stevens and Carson, 2002), while the resource heterogeneity hypothesis suggests that species richness is a function of the spatial heterogeneity in resource supply and inter-specific differences in resource requirements (Ricklefs, 1977; Huston, 1979; Stein et al., 2014). The importance of these two hypotheses for regulating plant species richness has been evaluated experimentally in grasslands (Stevens and Carson, 2002; Bakker et al., 2003; Baer et al., 2004; Eilts et al., 2011), where it was found that patterns of resource quantity (Stevens and Carson, 2002; Bakker et al., 2003) or resource heterogeneity (Eilts et al., 2011; Yang et al., 2015) or both (Questad and Foster, 2008; Cardinale et al., 2009) played crucial roles in shaping species richness. However, observational studies have rarely examined the relative contributions of resource quantity and resource heterogeneity to the maintenance of species richness in natural communities.
Testing the two resource hypotheses in observational studies is associated with a major methodological obstacle. Variation in resource supply rates among sites not only includes variation in the imbalance in resource supply rates among sites with different limiting resources (i.e., resource heterogeneity) but also contains the variation of resource quantity among sites with the same limiting resources. Hence, assessment of resource heterogeneity using solely within-site heterogeneity will lead to underestimation, whereas assessment of resource heterogeneity using both within-site heterogeneity and among-site resource supply rate will result in an inflated estimation (Lundholm, 2009). The close spatial covariation of resource quantity and resource heterogeneity has presented an enduring challenge in measuring their separate effects on species diversity (Stevens and Carson, 2002) and has hampered our ability to draw general conclusions regarding the mechanisms of diversity maintenance.
The resolution to the challenge in separating the effects of resource quantity and resource heterogeneity appears to lie with how resource supply rate covaries with plant density and how plant density influences species richness. It is widely appreciated that resource quantity and resource heterogeneity have a qualitatively different impact on species richness. The resource quantity hypothesis predicts that as the resource supply rates increase, the productivity and the density of plants in a community are either a monotonically increasing function (but may level off, more individuals hypothesis) (Srivastava and Lawton, 1998) or a unimodal function of resource supply rate (assemblage-level thinning hypothesis) (Stevens and Carson, 1999). Thus, species richness should also appear to be a monotonically increasing or a unimodal function of average resource supply rate, because increased plant density leads to a reduced risk of stochastic extinction for rare species (Stevens and Carson, 1999; Cardinale et al., 2009). Thus, the variation of species richness in natural communities explained by resource quantity should largely overlap with that explained by plant density. In contrast, the resource heterogeneity hypothesis predicts that spatial turnover in species composition will increase when resource heterogeneity (spatial niche dimensionality) increases within the same species pool and species differ in resource utilization (Questad and Foster, 2008; Eilts et al., 2011). The resource heterogeneity hypothesis does not necessarily rely on assumptions about the relationship among resource supply rate, plant density, and species richness. Therefore, it is possible to separate the effects of resource quantity and resource heterogeneity among sites using the joint effect of plant density and resource supply rate to estimate the effect of resource quantity.
Using a novel approach, we examined the relative importance of resource heterogeneity and resource quantity in explaining species diversity in two large-scale species-rich forest plots in Gutianshan (GTS) Nature Reserve, Southeast China, and Barro Colorado Island (BCI), Central Panama. The two plots are ideal for testing the relative importance of the two hypotheses, because the rugged terrain of GTS and relatively uniform topography of BCI represent two extreme ends of resource heterogeneity (Harms et al., 2001; Legendre et al., 2009), and the soil resources and species distribution in the two plots have been mapped at high resolution (John et al., 2007; Zhang et al., 2011). To test the two hypotheses, we first partitioned the variation of species richness into components explained by resource quantity, resource heterogeneity, and plant density. We further evaluated the relative importance of resource quantity and resource heterogeneity by accounting for the effect of space.
Materials and Methods
Study Site
We conducted research at two species-rich sites: (i) the 24-ha subtropical forest plot in GTS (29.25°N, 118.12°E, alt. 446–715 m), Zhejiang Province, East China; (ii) the 50-ha forest plot on BCI (09.15°N, 79.84°E, alt. 120–160 m), Central Panama. GTS is a subtropical evergreen broad-leaved forest supporting 159 species and 140,700 individuals (DBH ≥ 1cm). Castanopsis eyrei (Fagaceae), Schima superba (Theaceae), and Pinus massoniana (Pinaceae) are dominant species in this forest (Zhu et al., 2008). The soil is a subtropical red soil (equivalent to Ultisols in United States soil taxonomy).
Barro Colorado Island forest is a lowland semi-deciduous moist forest sustaining 299 species and 208, 400 individuals in the sixth census carried out in 2005. The soils in BCI are mostly well-weathered Kaolintic Oxisols (John et al., 2007).
Soil Sampling and Analysis
Soils were sampled and analyzed at GTS and BCI following the same protocol of soil survey but with different grid sizes (John et al., 2007; Zhang et al., 2011). The soils of the BCI plot were sampled in a previous study (John et al., 2007) using a grid of 50 × 50 m, and one additional point was added to each intersection point at 2 m, 8 m, or 20 m in a random compass direction from the grid. Soils in GTS were sampled with a regular grid of 30 × 30 m in an area of 390 × 600 m. Each regular point was associated with two additional sampling points at 2 m, 5 m, or 15 m in a random compass direction. The remaining area was divided into 10 × 30 m grid and sampled the cross points. Thus, 893 points in GTS and 300 points in BCI were sampled. The soil sampling and analysis methods are available in John et al. (2007) and Zhang et al. (2011).
We applied Ordinary Kriging to obtain average values of soil variables for 10 × 10 m, 20 × 20 m, 40 × 40 m quadrats (in GTS), and 50 × 50 m quadrats (in BCI). We chose 40 × 40 m quadrats in GTS instead of 50 × 50 m quadrats at larger scale due to sufficient sample size at 40 × 40 m (150 quadrats at 40 × 40 m vs. 96 quadrats at 50 × 50 m).
Data Analysis
Plant Richness and Density
Species richness was the number of species in a sampled quadrat. We used the number of individuals (plant density) in each quadrat as an explanatory factor due to the relationship between species richness and plant density predicted by the resource quantity hypothesis (Srivastava and Lawton, 1998; Stevens and Carson, 1999).
Environmental Factors
Environmental factors used in the analysis included soil nutrients and topographic variables. Available ions (Al, B, Ca, Cu, Fe, K, Mg, Mn, N, Na, Si, Zn), N mineralization rate (Nmin), pH, moisture, Total C (TC), Total N (TN), Total P (TP) for GTS, and available ions (Al, B, Ca, Cu, Fe, K, Mg, Mn, P, Zn, N), Nmin, pH for BCI were included in the analysis. Topographic variables (mean elevation, convexity, slope, and aspect) of both GTS and BCI were also included in the analysis. Topographic variables were calculated from elevation data following methods in Harms et al. (2001) and Legendre et al. (2009). Before the analysis, the soil nutrient except pH value was standardized by dividing the maximum value of each soil nutrient in the plot; pH value, mean elevation, convexity, and slope were centralized, while aspect was sine and cosine transformed to express east and north (Harms et al., 2001; John et al., 2007; Legendre et al., 2009). To assess the effects of resource heterogeneity and resource quantity on species diversity patterns, we combined soil nutrients and topographic variables as resource variables, because topographic factors can partly represent the light availability, soil temperature, or soil moisture in local communities.
Spatial Factors
Spatial structure of species richness among quadrats was represented by dbMEM (distance-based Moran’s eigenvector mapping) (Borcard and Legendre, 2002; Dray et al., 2006), which has also been called PCNM (principal coordinate of neighbor matrices). A truncated geographic distance matrix among quadrats was computed using the coordinates of quadrats, then principal coordinate analysis (PCoA) was employed to transform this matrix to dbMEMs (Borcard and Legendre, 2002). The dbMEMs were employed as explanatory variables to model spatial structures of species richness. Details of dbMEMs have been demonstrated in Legendre et al. (2009). Using function forward.sel() in R package packfor (Dray et al., 2012), we perform forward selection to choose significant dbMEMs representing spatial factors.
Statistical Analysis
We used a variation partitioning method (Borcard et al., 1992; Peres-Neto et al., 2006; Cao et al., 2019) to partition the variation of species richness into fractions that were explained by resource heterogeneity, resource quantity (mean resource level), and spatial structure. We used function varpart() in R package “vegan” to calculate the variation fractions (Oksanen et al., 2007).
We used the standard deviation of environmental factors in 4 and 16 sub-quadrats of 10 × 10 m to represent resource heterogeneity within a 20 × 20 m or a 40 × 40 m quadrat (within-quadrat resource heterogeneity) for GTS, respectively. Similarly, we used the standard deviation of environmental factors in 4 and 25 sub-quadrats of 10 × 10 m to represent within-quadrat resource heterogeneity within a 20 × 20 m or a 50 × 50 m quadrat for BCI (Tilman, 1982; Stevens and Carson, 2002). To estimate average resource supply rate, we used the mean values of environmental factors at the same scales (Stevens and Carson, 2002).
We first partitioned the variation of species richness into fractions explained by resource quantity, resource heterogeneity, and plant density as shown in Figure 1. The fraction explained by within-quadrat resource heterogeneity is represented by fractions [1] + [5] + [6] in Figure 1. To disentangle the effect of resource heterogeneity and resource quantity, we used the joint effect of average resource supply rate and plant density to estimate the effect of resource quantity on species richness (fractions [4] + [7] in Figure 1) and used the other parts of the fraction explained by average resource supply rate to estimate resource heterogeneity among quadrats (among-quadrat resource heterogeneity) (fractions [2] + [5] in Figure 1). Thus, the effect of resource heterogeneity can be estimated as the sum of within-quadrat resource heterogeneity and among-quadrat resource heterogeneity (fractions [1] + [2] + [5] + [6] in Figure 1).
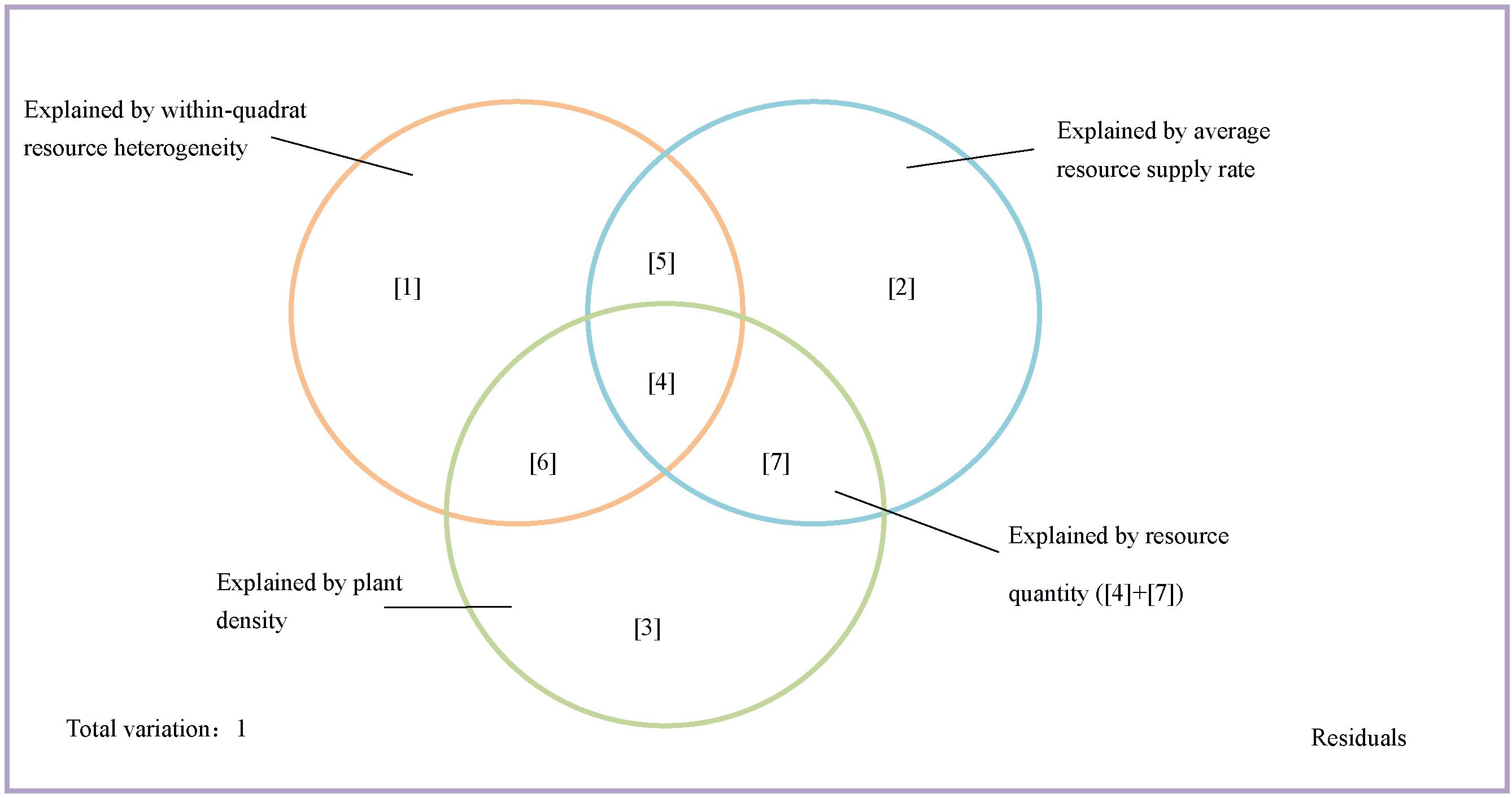
Figure 1. Variation partitioning model for testing predictions of the resource quantity hypothesis and the resource heterogeneity hypothesis. The box encompasses the total variation in species richness of the studied plot. Circles stands for the fractions of variation that within-quadrat resource heterogeneity, average resource supply rate, and plant density could explain. [1]∼[7] represent the fractions of variation in species richness that could be explained by different variables. [1], [2], and [3] are the fraction of variation that could be explained by one factor controlling other factors, respectively. [4]∼[7] represent the intersection of variation that could be explained by joint effect of two or three variables.
Next, we further evaluated the effect of resource quantity and resource heterogeneity on species richness by accounting for the effect of space. We partitioned the variation in species richness into fractions explained by resource heterogeneity, average resource supply rate, plant density, and dbMEMs as shown in Supplementary Figure S1. Similarly, the variation in species richness explained by resource quantity is the sum of fractions [7], [10], [12], and [15], and the fractions explained by resource heterogeneity is the sum of fractions [1], [2], [5], [8], [9], and [14]. The joint effect of resource quantity and space is the sum of fractions [12] and [15], and the joint effect of resource heterogeneity and space is the sum of fractions [8], [9], and [14]. The pure effect of space is [4].
To model non-linear relationships between species richness and resource heterogeneity, average resource supply rate, and plant density, a third-order polynomial equation was applied to these factors to obtain monomials (Legendre et al., 2009). We used forward selection (with permutation tests, at the 5% significance level, of the increase in the adjusted R2) to eliminate high collinearity among these variables and select a parsimonious subset of variables that significantly affect the distribution of species richness.
Spatial structure variables (dbMEMs) were produced by “PCNM” package (Legendre et al., 2012), forward selection was computed by “packfor” package (Dray et al., 2012), and variation partitioning method was performed using the “vegan” package (Oksanen et al., 2007). All the analysis was conducted in the open source software R 2.15.2 (R Development Core Team, 2012), and the R code was shown in the supporting information (Supplementary Text S1).
Results
The present work asked a central question: what is the relative contribution of resource quantity and resource heterogeneity to species diversity maintenance? We first addressed this question by partitioning the variation in species richness at GTS and BCI into fractions explained by resource heterogeneity, resource quantity, and plant density (Figures 2A,B and Table 1). In GTS plot, 60.0% of the variation in species richness could be explained by all variables at the 20 × 20 m scale, but only 21.6% at the same scale in BCI. However, we found consistent results across both plots, in terms of the relative importance of resource quantity and resource heterogeneity. Resource quantity could explain very little of variation in species richness. In GTS only 4.9% of the variation in species richness was explained by resource quantity, which contrasted markedly with 44.5% of the variation explained by resource heterogeneity (including both within-quadrat and among-quadrat resource heterogeneity) at the 20 × 20 m scale (Figure 2A and Table 1). Similarly, in BCI only 0.8% of the variation in species richness could be explained by resource quantity compared with 20.4% of the variation explained by resource heterogeneity at the 20 × 20 m scale (Figure 3A and Table 1). We found the similar results at the 40 × 40 m scale in GTS and at the 50 × 50 m scale in BCI (Figures 2B, 3B and Table 1).
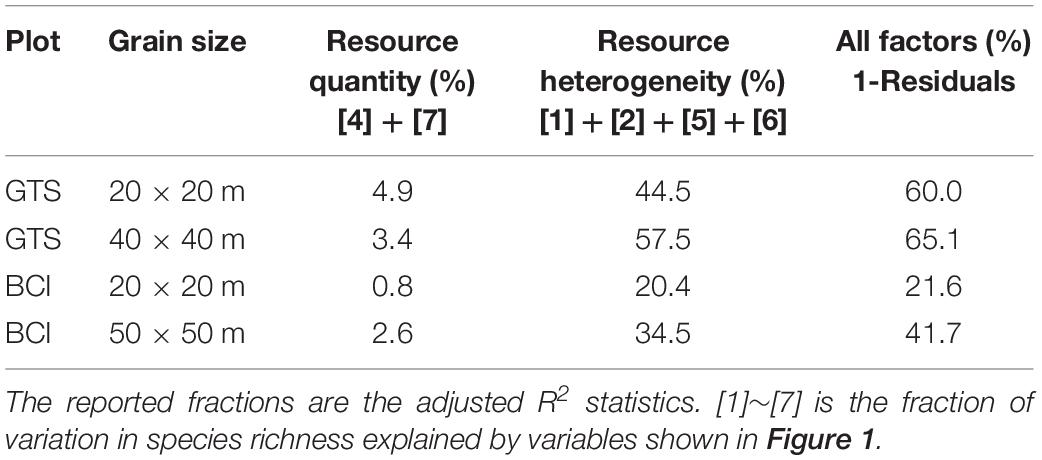
Table 1. Results of partitioning the variation of species richness explained by resource heterogeneity and resource quantity in Gutianshan (GTS) and Barro Colorado Island (BCI).
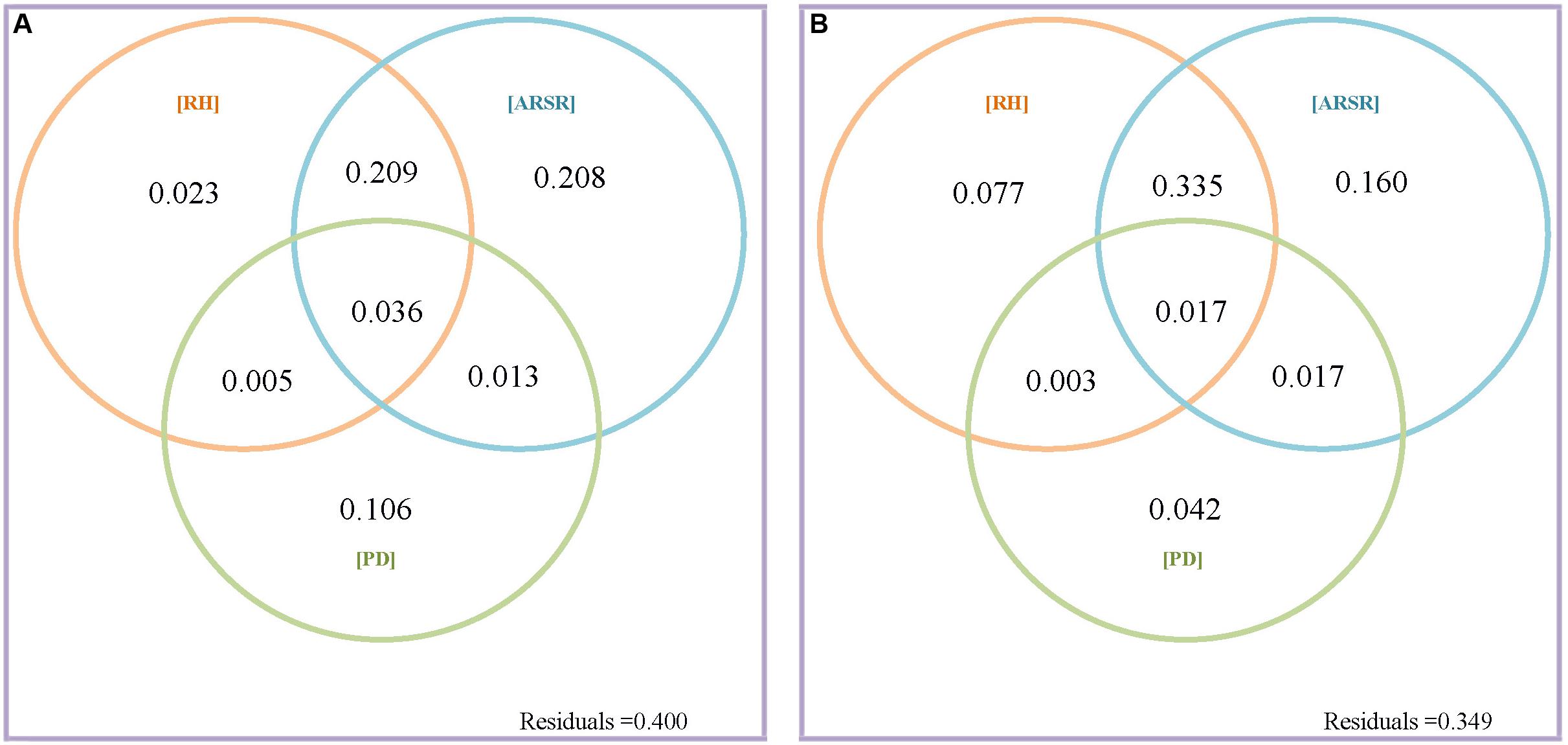
Figure 2. Venn diagrams of variation partitioning results of variation in species richness explained by resource heterogeneity, average resource supply rate, and plant density at grain size 20 × 20 m (A) and 40 × 40 (B) for GTS; “RH” is the fraction explained by within-quadrat resource heterogeneity; “ARSR” is the fraction explained by average resource supply rate including the effect of resource quantity and among-quadrat resource heterogeneity; and “PD” is the fraction explained by plant density.
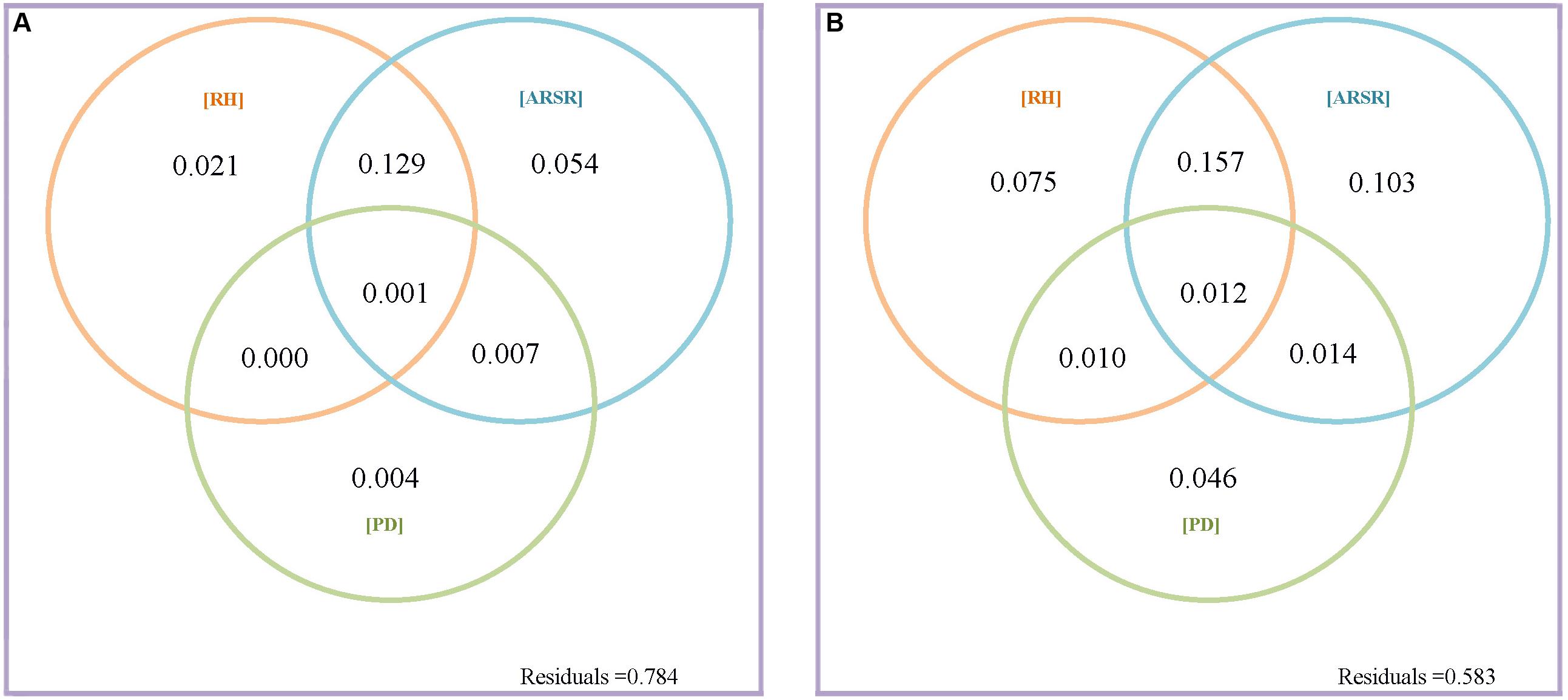
Figure 3. Venn diagrams of variation partitioning results of variation in species richness explained by resource heterogeneity, average resource supply rate, and plant density at grain size 20 × 20 m (A) and 50 × 50 m (B) for BCI; “RH” is the fraction explained by within-quadrat resource heterogeneity; “ARSR” is the fraction explained by average resource supply rate including the effect of resource quantity and among-quadrat resource heterogeneity; and “PD” is the fraction explained by plant density.
We further evaluated the relative importance of resource quantity and resource heterogeneity on species richness while accounting for the effect of space (Supplementary Table S1 and Supplementary Figure S2). We found that most of the effect of resource quantity and resource heterogeneity on species richness overlapped with the effect of space (Supplementary Table S1 and Supplementary Figure S2). In GTS, we found that 61.2% of the effect of resource quantity and 99.8% of the effect of resource heterogeneity on species richness could be explained by space at the 20 × 20 m scale (Supplementary Table S1 and Supplementary Figures S2A,B). Likewise, in BCI almost 100% of effect of both resource quantity and resource heterogeneity could be explained by space at the 20 × 20 m scale (Supplementary Table S1 and Supplementary Figures S2C,D). We found the similar results at larger scales in both plots (Supplementary Table S1 and Supplementary Figure S2). Moreover, we found that the effect of resource heterogeneity (44.5% for the effect of resource heterogeneity vs. 9.2% for the pure effect of space and 3.4% for the effect of resource quantity) governed species richness in GTS at the 20 × 20 m scale, whereas the pure effect of space (31.4% for the pure effect of space vs. 20.4% for the effect of resource heterogeneity and 0.8% for the effect of resource quantity) dominated the species diversity in BCI at the same scale (Supplementary Figure S2 and Supplementary Table S1). Again, results at the larger scales were similar (Supplementary Figure S2 and Supplementary Table S1).
Discussion
The resource quantity hypothesis and the resource heterogeneity hypothesis are two contrasting views developed to explain the maintenance of species richness in the forest ecosystems (Tilman, 1982; Wright, 1983). However, the close spatial covariation in average resource supply rate and resource heterogeneity in natural communities make it difficult to isolate the effect of resource quantity and resource heterogeneity (Stevens and Carson, 2002). Using an approach derived from the relationship among resource quantity, resource heterogeneity, and plant density in the resource hypotheses, we found that resource quantity played a limited role in maintaining species diversity in two species-rich forests.
We found that resource quantity could explain much less of the variation in species richness than resource heterogeneity in both of GTS and BCI. Thus, our results confirmed the resource heterogeneity hypothesis, but failed to support the resource quantity hypothesis. This suggests that resource heterogeneity may play a more important role in maintaining plant species diversity than resource quantity, at least in these two old-growth forests. This result is consistent with the findings that confirmed resource heterogeneity hypothesis in heterogeneous habitats (Bell et al., 2000; Balvanera and Aguirre, 2006; Douda et al., 2012; Ortega et al., 2018; Liu et al., 2019). In experimental studies, Stevens and Carson (2002) and Baer et al. (2004) showed that resource quantity contributes to species richness in artificially created uniform niches, such as light availability. Kumar et al. (2018) also found that mean light level was more important than light heterogeneity in explaining the understory species richness. Our results and above-mentioned studies may collectively support Bartels and Chen (2010)’s hypothesis that resource quantity determines species richness along a particular niche, whereas resource heterogeneity dominates species diversity across niches.
When spatial factors were included into the analysis, we found that most of the effect of resource heterogeneity and resource quantity on species richness overlapped with the effect of space, and the pure effects of resource quantity and resource heterogeneity were small (Supplementary Figure S2 and Supplementary Table S1). This result indicates that most effects of resources could also be explained by spatial processes, such as dispersal limitation, invalidating the default assumption in both hypotheses that all appropriate species can reach all suitable habitats (Lundholm, 2009). On the other hand, the spatial arrange of habitats such as mountain ridge, hillside, or valley which related to the spatial distribution of resources also played an important role in regulating species richness pattern of these two forests (Harms et al., 2001; Lai et al., 2009; Legendre et al., 2009). This paralleled findings from experimental studies that propagule supply and resource heterogeneity jointly determine the species diversity of a community (Questad and Foster, 2008; Eilts et al., 2011; Myers and Harms, 2011). We also found that the effect of resource heterogeneity governed species richness in GTS, whereas the pure effect of space dominated the species diversity in BCI (Supplementary Figure S2 and Supplementary Table S1). As we included soil nutrients and within-quadrat resource heterogeneity into analysis in GTS, we found that the effect of resource heterogeneity on species richness (44.5 and 57.5% at the 20 × 20 m and 40 × 40 m scales) was larger than that found by Legendre et al. (2009) (25.8 and 36.2% at the 20 × 20 m and 40 × 40 m scales, Table 1). It also suggested that the positive effect of the resource heterogeneity on species richness was more important at the larger scale. This may be because larger units encompassed more variability and species turnover (Stein et al., 2014).
In studies, the sum of pure effect of resource heterogeneity and the joint of resource heterogeneity and space was interpreted as the outcome of resource heterogeneity, while the pure effect of space was interpreted as the effect of spatial processes such as dispersal limitation (Jones et al., 2008; Legendre et al., 2009). However, caution should be excised when attributing fractions of variation partitioning to the consequences of these processes even though soil nutrients were measured to a high resolution in both plots. The effect of resource quantity and resource heterogeneity may be underestimated, because the effect space may include the effects of unmeasured habitat factors, such as light availability; while the effect of space may be underestimated, because limited dispersal often correlates with spatial arrangement of habitats (Gilbert and Bennett, 2010; Smith and Lundholm, 2010).
Additionally, it is difficult to manipulate the resource heterogeneity and average resource supply rate to test the related hypotheses in the forest ecosystem. However, the method used in our study is novel, which could address this problem. Above all, the variation partitioning was used to separate the impacts of resources heterogeneity and resource quantity with in situ observational data, which could be a more appropriate way to reveal the assembly rule of the forests. Secondly, the variation partitioning could account for the spatial effect which is one crucial factor contributed importantly to the community assembly of the forests, and it makes the analysis more robust (Hu et al., 2018).
In summary, we evaluated the relative importance of resource quantity and resource heterogeneity in determining species richness in a subtropical forest plot in China. Using a novel approach to partition the effects of resource quantity and resource heterogeneity, we found that resource quantity played a limited role in structuring species diversity in two species-rich forests. To the best our knowledge, our study is the first evaluation of the relative contribution of resource quantity and resource heterogeneity in natural forest communities. We also found that resource heterogeneity governed species richness in GTS, whereas spatial processes such as dispersal limitation dominated species diversity in BCI. Our results, together with experimental and observational studies, suggest that the processes determining variation in species richness along ecological gradients in natural communities may be more complicated than can be represented by a single hypothesis. In the future, other types of forest ecosystem such as temperate forests could be tested using this method to gain more general results in the forest ecosystem. Moreover, the temporal pattern of the relative importance of resource heterogeneity and resource quantity also needs to be examined and compared, and it may help to deepen the understanding of community assembly rules in the forests.
Data Availability Statement
The datasets generated for this study are available on request to the corresponding author.
Author Contributions
LZ, XMi, and RH contributed to the experiment performance, data collection, data analysis and interpretation, and manuscript writing. BY and XMan contributed to the experiment performance and data collection. HR and KM contributed to the experimental design and interpretation. All authors contributed to the article and approved the submitted version.
Funding
Soil work in the BCI plot was supported by NSF DEB 021104, 021115, 0212284, 0212818, and OISE 0314581, and the STRI soils initiative. This research was funded by the National Science and Technology Support Program (2012BAC01B05), the NSFC Project (31170401), and the Youth Innovation Promotion Association CAS (2018247).
Conflict of Interest
The authors declare that the research was conducted in the absence of any commercial or financial relationships that could be construed as a potential conflict of interest.
Acknowledgments
The authors are grateful to Pierre Legendre and Kyle Harms for their valuable comments on data analysis and manuscript revision.
Supplementary Material
The Supplementary Material for this article can be found online at: https://www.frontiersin.org/articles/10.3389/fevo.2020.00224/full#supplementary-material
References
Abrams, P. A. (1995). Monotonic or unimodal diversity productivity gradients - what does competition theory predict. Ecology 76, 2019–2027. doi: 10.2307/1941677
Baer, S. G., Blair, J. M., Collins, S. L., and Knapp, A. K. (2004). Plant community responses to resource availability and heterogeneity during restoration. Oecologia 139, 617–629. doi: 10.1007/s00442-004-1541-3
Bakker, C., Blair, J. M., and Knapp, A. K. (2003). Does resource availability, resource heterogeneity or species turnover mediate changes in plant species richness in grazed grasslands? Oecologia 137, 385–391. doi: 10.1007/s00442-003-1360-y
Balvanera, P., and Aguirre, E. (2006). Tree diversity, environmental heterogeneity, and productivity in a Mexican tropical dry forest. Biotropica 38, 479–491. doi: 10.1111/j.1744-7429.2006.00161.x
Barot, S. (2004). Mechanisms promoting plant coexistence: can all the proposed processes be reconciled? Oikos 106, 185–192. doi: 10.1111/j.0030-1299.2004.13038.x
Bartels, S. F., and Chen, H. Y. H. (2010). Is understory plant species diversity driven by resource quantity or resource heterogeneity? Ecology 91, 1931–1938. doi: 10.1890/09-1376.1
Bell, G., Lechowicz, M. J., and Waterway, M. J. (2000). Environmental heterogeneity and species diversity of forest sedges. J. Ecol. 88, 67–87. doi: 10.1046/j.1365-2745.2000.00427.x
Borcard, D., and Legendre, P. (2002). All-scale spatial analysis of ecological data by means of principal coordinates of neighbour matrices. Ecol. Model. 153, 51–68. doi: 10.1016/s0304-3800(01)00501-4
Borcard, D., Legendre, P., and Drapeau, P. (1992). Partialling out the Spatial Component of Ecological Variation. Ecology 73, 1045–1055. doi: 10.2307/1940179
Cao, K., Mi, X. C., Zhang, L. W., Ren, H. B., Yu, M. J., Chen, J. H., et al. (2019). Examining residual spatial correlation in variation partitioning of beta diversity in a subtropical forest. J. Plant Ecol. 12, 636–644. doi: 10.1093/jpe/rty058
Cardinale, B. J., Bennett, D. M., Nelson, C. E., and Gross, K. (2009). Does productivity drive diversity or vice versa? A test of the multivariate productivity-diversity hypothesis in streams. Ecology 90, 1227–1241. doi: 10.1890/08-1038.1
Douda, J., Doudova-Kochankova, J., Boublik, K., and Drasnarova, A. (2012). Plant species coexistence at local scale in temperate swamp forest: test of habitat heterogeneity hypothesis. Oecologia 169, 523–534. doi: 10.1007/s00442-011-2211-x
Dray, S., Legendre, P., and Blanchet, G. (2012). packfor: R package for forward selection, version 0.0-8. Available online at: http://R-Forge.R-project.org (accessed July, 2013).
Dray, S., Legendre, P., and Peres-Neto, P. R. (2006). Spatial modelling: a comprehensive framework for principal coordinate analysis of neighbour matrices (PCNM). Ecol. Model. 196, 483–493. doi: 10.1016/j.ecolmodel.2006.02.015
Eilts, J. A., Mittelbach, G. G., Reynolds, H. L., and Gross, K. L. (2011). Resource Heterogeneity, Soil Fertility, and Species Diversity: Effects of Clonal Species on Plant Communities. Am. Nat. 177, 574–588. doi: 10.1086/659633
Gilbert, B., and Bennett, J. R. (2010). Partitioning variation in ecological communities: do the numbers add up? J. Appl. Ecol. 47, 1071–1082. doi: 10.1111/j.1365-2664.2010.01861.x
Harms, K. E., Condit, R., Hubbell, S. P., and Foster, R. B. (2001). Habitat associations of trees and shrubs in a 50-ha neotropical forest plot. J. Ecol. 89, 947–959. doi: 10.1111/j.1365-2745.2001.00615.x
Hu, Y. H., Johnson, D. J., Mi, X. C., Wang, X. G., Ye, W. H., Li, Y. D., et al. (2018). The relative importance of space compared to topography increases from rare to common tree species across latitude. J. Biogeogr. 45, 2520–2532. doi: 10.1111/jbi.13420
Huston, M. (1979). A general hypothesis of species diversity. Am. Nat. 113, 81–101. doi: 10.1086/283366
John, R., Dalling, J. W., Harms, K. E., Yavitt, J. B., Stallard, R. F., Mirabello, M., et al. (2007). Soil nutrients influence spatial distributions of tropical tree species. Proc. Natl. Acad. Sci. U.S.A. 104, 864–869. doi: 10.1073/pnas.0604666104
Jones, M. M., Tuomisto, H., Borcard, D., Legendre, P., Clark, D. B., and Olivas, P. C. (2008). Explaining variation in tropical plant community composition: influence of environmental and spatial data quality. Oecologia 155, 593–604. doi: 10.1007/s00442-007-0923-8
Kumar, P., Chen, H. Y. H., Thomas, S. C., and Shahi, C. (2018). Linking resource availability and heterogeneity to understorey species diversity through succession in boreal forest of Canada. J. Ecol. 106, 1266–1276. doi: 10.1111/1365-2745.12861
Lai, J. S., Mi, X. C., Ren, H. B., and Ma, K. P. (2009). Species-habitat associations change in a subtropical forest of China. J. Veget. Sci. 20, 415–423. doi: 10.1111/j.1654-1103.2009.01065.x
Legendre, P., Borcard, D., Blanchet, F. G., Caceres, M. D., and Dray, S. (2012). PCNM: MEM spatial eigenfunction and principal coordinate analyses, Version: 2.1-2. Available online at: http://r-forge.r-project.org/ (accessed July, 2013).
Legendre, P., Mi, X. C., Ren, H. B., Ma, K. P., Yu, M. J., Sun, I. F., et al. (2009). Partitioning beta diversity in a subtropical broad-leaved forest of China. Ecology 90, 663–674. doi: 10.1890/07-1880.1
Liu, Y. J., De Boeck, H. J., Li, Z. Q., and Nijs, I. (2019). Unimodal relationship between three-dimensional soil heterogeneity and plant species diversity in experimental mesocosms. Plant Soil 436, 397–411. doi: 10.1007/s11104-019-03938-w
Lundholm, J. T. (2009). Plant species diversity and environmental heterogeneity: spatial scale and competing hypotheses. J. Veget. Sci. 20, 377–391. doi: 10.1111/j.1654-1103.2009.05577.x
Myers, J. A., and Harms, K. E. (2011). Seed arrival and ecological filters interact to assemble high-diversity plant communities. Ecology 92, 676–686. doi: 10.1890/10-1001.1
Oksanen, J., Kindt, R., Legendre, P., and O’hara, R. B. (2007). vegan: community ecology package. R package version 1.9-25. Available online at: http://cran.r-project.org/ (accessed July, 2013).
Ortega, J. C. G., Thomaz, S. M., and Bini, L. M. (2018). Experiments reveal that environmental heterogeneity increases species richness, but they are rarely designed to detect the underlying mechanisms. Oecologia 188, 11–22. doi: 10.1007/s00442-018-4150-2
Peres-Neto, P. R., Legendre, P., Dray, S., and Borcard, D. (2006). Variation partitioning of species data matrices: estimation and comparison of fractions. Ecology 87, 2614–2625. doi: 10.1890/0012-9658(2006)87[2614:vposdm]2.0.co;2
Questad, E. J., and Foster, B. L. (2008). Coexistence through spatio-temporal heterogeneity and species sorting in grassland plant communities. Ecol. Lett. 11, 717–726. doi: 10.1111/j.1461-0248.2008.01186.x
R Development Core Team (2012). R: A Language and Environment for Statistical Computing. Vienna: The R Foundation for Statistical Computing.
Ricklefs, R. E. (1977). Environmental heterogeneity and plant species diversity: a hypothesis. Am. Nat. 111, 376–381. doi: 10.1086/283169
Ricklefs, R. E., and Schluter, D. (1993). Species Diversity in Ecological Communities: Historical and Geographical Perspectives. Chicago, IL: University of Chicago Press.
Smith, T. W., and Lundholm, J. T. (2010). Variation partitioning as a tool to distinguish between niche and neutral processes. Ecography 33, 648–655. doi: 10.1111/j.1600-0587.2009.06105.x
Srivastava, D. S., and Lawton, J. H. (1998). Why more productive sites have more species: An experimental test of theory using tree-hole communities. Am. Nat. 152, 510–529. doi: 10.1086/286187
Stein, A., Gerstner, K., and Kreft, H. (2014). Environmental heterogeneity as a universal driver of species richness across taxa, biomes and spatial scales. Ecol. Lett. 17, 866–880. doi: 10.1111/ele.12277
Stevens, M. H. H., and Carson, W. P. (1999). The significance of assemblage-level thinning for species richness. J. Ecol. 87, 490–502. doi: 10.1046/j.1365-2745.1999.00374.x
Stevens, M. H. H., and Carson, W. P. (2002). Resource quantity, not resource heterogeneity, maintains plant diversity. Ecol. Lett. 5, 420–426. doi: 10.1046/j.1461-0248.2002.00333.x
Tilman, D. (1982). Resource Competition and Community Structure. Princeton: Princeton University Press.
Wright, D. H. (1983). Species energy theory: an extension of species-area theory. Oikos 41, 496–506.
Yang, Z. Y., Liu, X. Q., Zhou, M. H., Ai, D., Wang, G., Wang, Y. S., et al. (2015). The effect of environmental heterogeneity on species richness depends on community position along the environmental gradient. Sci. Rep. 5:15723.
Zhang, L. W., Mi, X. C., Shao, H. B., and Ma, K. P. (2011). Strong plant-soil associations in a heterogeneous subtropical broad-leaved forest. Plant Soil 347, 211–220. doi: 10.1007/s11104-011-0839-2
Keywords: resource quantity, resource heterogeneity, variation partitioning, species richness, ecological niche, plant density, spatial covariation
Citation: Zhang L, Mi X, Harrison RD, Yang B, Man X, Ren H and Ma K (2020) Resource Heterogeneity, Not Resource Quantity, Plays an Important Role in Determining Tree Species Diversity in Two Species-Rich Forests. Front. Ecol. Evol. 8:224. doi: 10.3389/fevo.2020.00224
Received: 21 January 2020; Accepted: 18 June 2020;
Published: 16 July 2020.
Edited by:
Zehao Shen, Peking University, ChinaReviewed by:
Tiago Vasconcelos, São Paulo State University, BrazilMingxi Jiang, Chinese Academy of Sciences, China
Copyright © 2020 Zhang, Mi, Harrison, Yang, Man, Ren and Ma. This is an open-access article distributed under the terms of the Creative Commons Attribution License (CC BY). The use, distribution or reproduction in other forums is permitted, provided the original author(s) and the copyright owner(s) are credited and that the original publication in this journal is cited, in accordance with accepted academic practice. No use, distribution or reproduction is permitted which does not comply with these terms.
*Correspondence: Xiangcheng Mi, bWl4aWFuZ2NoZW5nQGliY2FzLmFjLmNu