- 1Key Laboratory of Bio-Resource and Eco-Environment of Ministry of Education, College of Life Sciences, Sichuan University, Chengdu, China
- 2Chengdu Institute of Biology, Chinese Academy of Sciences, Chengdu, China
- 3Nanjing Institute of Environmental Sciences, Ministry of Environmental Protection, Nanjing, China
- 4State Key Laboratory of Systematic and Evolutionary Botany, Institute of Botany, Chinese Academy of Sciences, Beijing, China
Despite the numerous studies on the large-scale patterns of species richness, the spatial variation and determinants of species richness for the alpine plants are still an outstanding question and critical to future biodiversity conservation. The genus Saxifraga is a typical alpine plant group with high species richness in the Himalaya-Hengduan Mountain (HHM) regions, China. We performed simple regression models and variance partitioning to assess the importance of different factors, especially soil-related ones, in driving Saxifraga richness patterns. The results showed that environmental energy, habitat heterogeneity, and soil heterogeneity together dominated the spatial variation of species richness. The coarse fragment volume (CRFVOL) of soil, elevation range, and soil heterogeneity are positively related to Saxifraga richness. Soil slightly outperforms habitat heterogeneity in predicting the spatial variation of Saxifraga species richness with an explanatory power of 39.3% and 36.6%, respectively. Environmental energy, such as the maximum temperature of the warmest quarter, is negatively correlated with species richness and explains 44.8% of spatial variation of Saxifraga richness. Multiple regression models, including three variables, each representing energy, soil, and habitat heterogeneity, can only explain 53.1% variation of species richness. Variance partitioning outscored 26% of the shared effects of the three variables, while the independent effect of each variable is less than 10%. These results indicated that the energy, soil, and habitat heterogeneity together are primary determinants of the spatial variation of Saxifraga species richness. However, there are probably other hidden factors predicting species richness variation due to the low explanatory power of the multiple regression models. Our study emphasizes the significance of soil properties in determining species richness patterns in China, especially for alpine plant groups. The negative association of species richness with temperature suggests a potential threat of alpine biodiversity loss in HHM from future warming.
Introduction
The mechanisms of large-scale patterns of species richness are one of the central and controversial issues in macroecology and biogeography (Gaston et al., 1995; Brown et al., 2004). Previous studies in large-scale species richness patterns focus on the effects of contemporary climate, habitat heterogeneity, and evolutionary history and have proposed productivity hypothesis, water–energy dynamic hypothesis, and niche conservatism hypothesis (Rosenzweig, 1995; O’Brien et al., 2000; Currie and Francis, 2004; Wiens and Donoghue, 2004; Wiens and Graham, 2005). China, spanning from tropical rainforest to boreal coniferous forest, harbors over 30,000 vascular plants. Southwestern China, especially the Himalaya-Hengduan Mountain (HHM) regions, holds the highest species richness and an extraordinary diversity in alpine plants. It was regarded as one of the global biodiversity hotspots (Myers et al., 2000). Despite the numerous studies on the patterns and determinants of species richness in China (Wang et al., 2009, 2011a,b; Shrestha et al., 2018a), the large-scale patterns, and determinants of alpine plant richness are still poorly understood.
The evolution of mountains can influence species richness through speciation, immigration, and extinction (Antonelli et al., 2018), especially in the HHM regions (Sun et al., 2017). First of all, the orogenic process in HHM regions since the Neogene increased the topography complexity and habitat heterogeneity; henceforth, it encouraged allopatric speciation and provided different climatic niches to promote the coexistence of more species (Du et al., 2017; Rahbek et al., 2019b). Shrestha et al. (2018b) found that habitat heterogeneity will increase species diversification and then increase species richness for a plant genus Rhododendron. Secondly, climate heterogeneity increased with topography complexity, and the uplift of HHM facilitated species movement along the altitudinal gradient. Therefore, HHM can play a role in refugium when climate change events happened. Previous studies found that habitat heterogeneity is also the primary determinant of spatial variation of plant species richness in drylands (Liu et al., 2019), Tibetan Plateau (Mao et al., 2013), and European mountains (Svenning and Skov, 2004; Svenning et al., 2006). These results are probably caused by the refugia effects, subsequent speciation events, and limited dispersals for species in the mountains. However, contemporary climate (e.g., climate seasonality) and climate change since last glacial maximum (LGM) are the primary determinants for the spatial variation of species richness for the herbaceous family Gesneriaceae in China (Liu et al., 2017) and palms in Madagascar (Rakotoarinivo et al., 2013). Similar results were found for other biological groups, e.g., tetrapods in Asia mountain regions (Antonelli et al., 2018). Other studies also found that habitat heterogeneity has negative or insignificant effects on species richness (Cramer and Willig, 2005; Lundholm, 2009; Laanisto et al., 2013; Coyle and Hurlbert, 2016). Thus, the relative importance of habitat heterogeneity and other environmental factors on the spatial variation of species richness is still controversial and has rarely been studied for herbaceous plants at a large scale.
Recently, Rahbek et al. (2019a) suggested that orogeny can promote soil formation and then fodder the species richness by affecting plant physiology, vegetation composition, and primary productivity. Soil attributes, e.g., soil heterogeneity, soil texture and structure, and soil fertility, varied with the uplift of mountains and the erosion of bedrocks (Antonelli et al., 2018). Soil heterogeneity usually positively correlates with the elevation range in mountain regions suggesting a high niche diversity (Stein et al., 2014; Antonelli et al., 2018). In mountain regions of Asia and Europe, soil heterogeneity even explains more on species richness than elevation range (Antonelli et al., 2018). The high content of cliff, shallow depth, and relative infertile are unique characters of mountain soil due to the limited biological activities, soil genesis, and evolution under low temperatures (Romeo et al., 2015). Where the slope becomes steeper, the soil becomes thinner and infertile because precipitation or surface runoff washes away topsoil easily in the mountains. Rising temperatures in alpine regions will promote soil moisture transpiration, result in soil water stress, and thus affect species survival (Zhu et al., 2016). Despite the harsh and diversified environment, alpine plant species have adapted well in the mountain soils and even diversified faster than other regions (Hughes and Atchison, 2015). However, soil effect on the spatial variation of species richness in mountain regions, especially the HHM, remains an outstanding question.
Saxifraga L. (Saxifragaceae) is a smallish herb, most of which are typical alpine plants and endemic to the HHM and adjacent regions (Yang et al., 2013; Ebersbach et al., 2017a; Rawat et al., 2019). Phylogenetic studies suggested that Saxifraga radiated rapidly in montane and alpine habitats. Along with the persistent uplift of mountains, Saxifraga species evolved two critical innovations of cushion life form and lime-secreting hydathodes to adapt to the colder and colder environment and exhibited a high diversification rate in the habitat of rock cliffs (de Casas et al., 2016; Ebersbach et al., 2017a). Meanwhile, sky islands and climate oscillation during the Quaternary period probably contributed to the high species diversity of Saxifraga through the allopatric speciation process and refugium effects in HHM as well (Ebersbach et al., 2017b). Therefore, the diversification of Saxifraga is connected tightly with the uplift of HHM, and the effects of the topographic factors and soil properties (e.g., the rock cliff content of the soil) are expected to be the primary determinants on the present species richness patterns.
Here, we evaluated the relative importance of habitat heterogeneity, soil factors, climate change since the LGM, and contemporary climate on the spatial variation of Saxifraga species richness based on county-level species distribution data in China. We specifically intend to comprehensively assess the effects of different soil properties on the species richness patterns for alpine plants. We performed an evaluation quantitatively to partition the effects of habitat heterogeneity, soil properties, climate change, and contemporary climate on Saxifraga richness patterns across China.
Materials and Methods
Species Distribution Data
The distribution of Saxifraga at county level in China was compiled from published flora and regional and local plant checklists, peer-reviewed articles, online databases, and herbarium specimens (Supplementary Appendix S1). Due to the broad area of several counties in northwest China, we divided the 12 large counties into 24 subcounties to reduce the effect of area on species richness (Wang et al., 2011a). The final geographic database based on county boundaries classified the whole of China into 2411 geographic units. We standardized and georeferenced the recorded geographical names from different literature following Xue (2014) and the global geographical names database (GeoNames1). Then, we assigned species distribution records to these subcounties according to data availability. All species names were standardized according to the Flora of China2. We merged intraspecific taxa to species level and excluded hybrids. In total, our database included 3532 distribution records for 224 species from 994 geographic units with an average area of 5648 km2 ± 12,250 km2.
For each geographic unit, the number of species was counted, and the area was calculated in ArcGIS (version 10.4.1) using the Albers equal-area conic projection. The area has low explanatory power (adjusted-R2 = 0.09, modified t-test p = 0.013) on the spatial variation of Saxifraga species richness (Supplementary Figure S1 in Supplementary Appendix S2). Therefore, we exclude the area from subsequent analysis.
Environmental Variables
To estimate the relative importance of habitat heterogeneity, soil, contemporary climate, and change since LGM on Saxifraga species richness, we first included 54 potential environmental variables in our preliminary analysis (Supplementary Table S1 in Supplementary Appendix S3).
The contemporary climate factors include all 19 bioclimatic variables derived from monthly temperature and precipitation, moisture index (Im), aridity index (AI), potential evapotranspiration (PET), annual actual evapotranspiration (AET), and water deficit (WD) in our study. We classified all variables into environmental energy including temperature-related variables, water availability including precipitation-related variables, Im and AET, climate seasonality including temperature seasonality (TSN), and precipitation seasonality (PSN). The 19 bioclimatic variables were downloaded from CHELSA3 at a spatial resolution of 30 arc seconds. Im was calculated following Fang and Yoda (1990) using monthly temperature and monthly precipitation obtained from WorldClim4 at a spatial resolution of 2.5 arc minutes. AI, PET, and AET with the resolution of 30 arc seconds were downloaded from the Consortium of International Agricultural Research Centers consortium for spatial information5. WD was calculated as the difference between PET and AET. For each climatic variable, we estimated the value of each geographic unit by averaging all grid cells within it.
Habitat heterogeneity was measured by elevation range (ELER), mean annual temperature range (MATR), and mean annual precipitation range (MAPR) within each geographic unit. ELER, MATR, and MAPR were calculated as the difference between the maximum and minimum values across all grids within each geographic unit, respectively. ELER was used to represent the topographic relief while MATR and MAPR were used to represent the climate heterogeneity. Elevation with 2.5 arc minutes of resolution was also downloaded from WorldClim4.
We included 25 soil variables in our preliminary analysis and classified them into soil texture, soil fertility, and soil heterogeneity (Supplementary Table S1 in Supplementary Appendix S3). Soil texture included six variables and represented the fractions of each soil separate, e.g., the coarse fragments volume, silt, sand, and clay content of the soil. Bulk density and depth to the bedrock were also classified into soil texture. Soil fertility included six variables related to nutrient availability of soil, including available water capacity (AWCh3), cation exchange capacity (CECSOL), organic carbon density (OCDENS), organic carbon stock (OCSTHA), organic carbon content (ORCDRC), and pH of the soil. Soil heterogeneity was calculated as the standard deviation of all grid cells in each geographic unit for each of all 12 soil variables. The number of soil types within each geographic unit was counted as one factor of soil heterogeneity.
We downloaded soil variables at four depths (0,5,15, and 30 cm) from the global soil geographic database (SoilGrids6) at a spatial resolution of 1 km (Hengl et al., 2014, 2017). The mean value of four soil layers was estimated (Soil_mean) and applied in our study. Depth to bedrock (BDRICM), soil organic carbon stock (OCSTHA), and soil types variables (TAXNWRB) have no soil layer data.
Climate change since the LGM was represented by temperature anomaly (ANOM MAT, °C) and precipitation anomaly (ANOM MAP, mm). ANOM MAT and ANOM MAP were calculated as the absolute values of the contemporary minus the LGM mean annual temperature and mean annual precipitation, respectively. To account for the uncertainty in past climate simulations, the mean annual temperature and precipitation of LGM reconstructed based on the Community Earth System Model (CCSM4) and the Model for Interdisciplinary Research on Climate Earth System Model (MIROC-ESM) were used in our analysis. These data were downloaded from WorldClim4 at 2.5 arc minutes of spatial resolution.
We used zonal statistics in ArcGIS (version 10.4.1) to calculate the mean, range, and standard deviation values of each variable within each geographical unit. The spatial variation of each soil factor was mapped using ArcMap in ArcGIS (version 10.4.1). The latitudinal and longitudinal trends of each soil factor were plotted and estimated by “lowess” function, and Spearman’s correlations among each two variables were calculated by “cor” function in R with the “stats” package.
Statistical Analyses
We first used linear regression models (LM) to assess the effect of each potential factor on spatial variation of Saxifraga richness. Species richness was natural logarithm transformed [log (species richness)] in all statistical analyses. Owing to the high spatial autocorrelation of the spatial patterns of species richness, which can inflate the type I error, we performed a modified t-test (Dutilleul et al., 1993) to estimate the significance level of all regression models. Then, for each environmental group, we only selected one best variable explaining the highest explanatory power in LM and significant in the modified t-test (Table 1). Due to the low explanatory power and insignificant effects (modified t-test p > 0.1) of the climate change since LGM and climate seasonality on spatial variation of Saxifraga species richness, we removed variables from these two groups in the following analysis (Supplementary Table S2 in Supplementary Appendix S3).
To compare the relative importance of each environmental group on the spatial variation of species richness, we first built multiple regression models of species richness against the selected six variables. Because of the collinearity among these six factors (Supplementary Table S3 in Supplementary Appendix S3), we applied a hierarchical partitioning method to decompose the variation in species richness into the independent effect for each variable and conjoint effects with all other variables on species richness patterns (Chevan and Sutherland, 1991; Nally, 1996). Hierarchical multivariate regression was set in hierarchical partitioning considering all possible models for the response variable and predictors. By the incorporation of a given variable or combination of variables, hierarchical partitioning involves the calculation of the increased goodness of fit in models, and these are averaged over all combinations to provide measures of the effects of independent variables and their combinations (Chevan and Sutherland, 1991; Mac Nally and Walsh, 2004). For the three variables with the highest independent effect identified by hierarchical partitioning, we performed multiple regression and variance partition to estimate the independent effect of each variable and shared effects among variables.
We performed LM analysis using the “lm” function in the R package “stats” (Chambers and Hastie, 1992), modified t-tests using the R package “SpatialPack” (Dutilleul et al., 1993), hierarchical partitioning using the R package “hier.part” (Chevan and Sutherland, 1991; Mac Nally and Walsh, 2004), and variance partitioning using the R package “vegan” (Peres-Neto et al., 2006).
Results
The species richness patterns of Saxifraga were highly consistent with the topographical structure of China with a high striking species richness in the HHM regions (Figure 1). The Qinghai-Tibetan Plateau also harbors high species richness, whereas Saxifraga species were rarely distributed in the eastern part of China. Soil properties also showed clear spatial patterns and highly correlated with climate and elevation (Supplementary Table S3 in Supplementary Appendix S3).
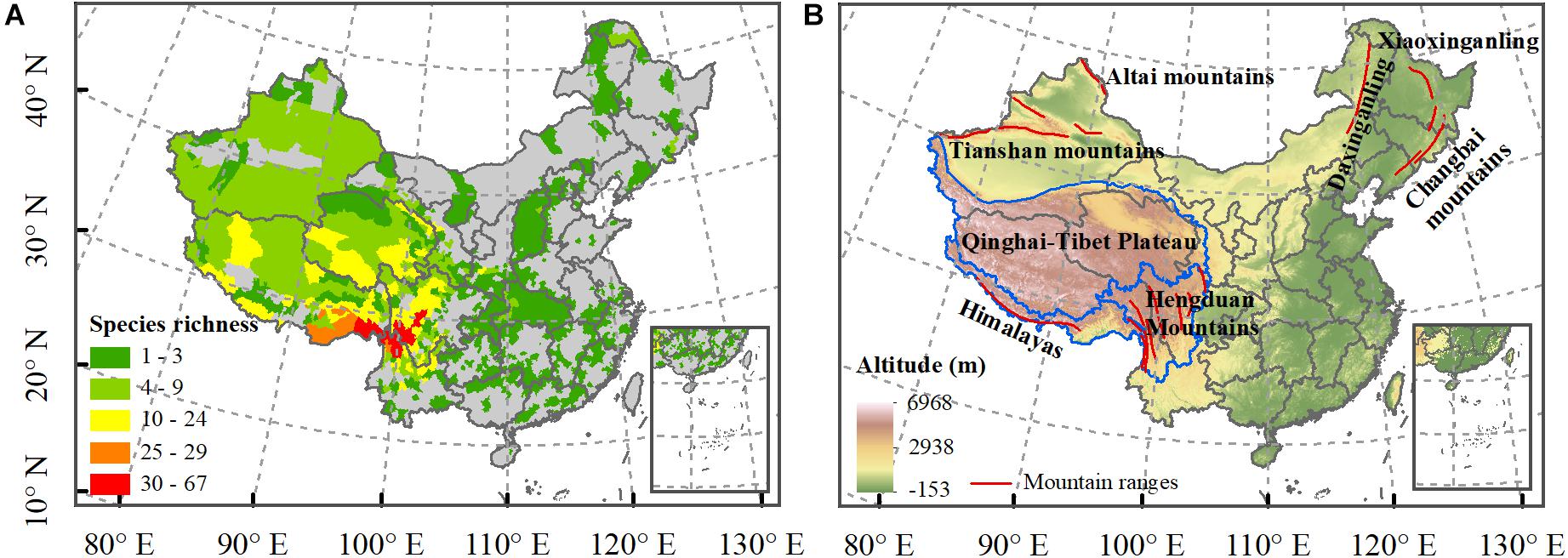
Figure 1. Spatial patterns of Saxifraga L. species richness in China (A). The maps of the Hengduan Mountains region, Himalayas region, QTP, and main mountain ranges (B).
Soil properties showed distinct geographical patterns and are closely related to temperature and elevation range (Figure 2, Supplementary Figure S2 in Supplementary Appendix S2, and Supplementary Table S3 in Supplementary Appendix S3). CRFVOL and a standard deviation of depth to bedrock (STD_BDRICM) increase from east to west and are high in the Hengduan mountains and Qinghai-Tibet Plateau. Cation exchange capacity (CECSOL) is high in Hengduan Mountains; Daxinganling, Xiaoxinganling, and Changbai Mountains; Altai Mountains, and Tianshan Mountains and low in lowland regions (Figure 2). All these three variables were positively correlated with elevation range and negatively correlated with mean temperature in the warmest quarter (Figure 2 and Supplementary Table S3 in Supplementary Appendix S3). Mean temperature in the warmest quarter showed the highest association with CRFVOL, followed by the STD_BDRICM and CECSOL with Spearman’s ρ of −0.645, −0.6, and −0.57, respectively. The elevation range showed the highest correlation with a STD_BDRICM, followed by CRFVOL and CECSOL with Spearman’s ρ of 0.809, 0.688, and 0.39, respectively.
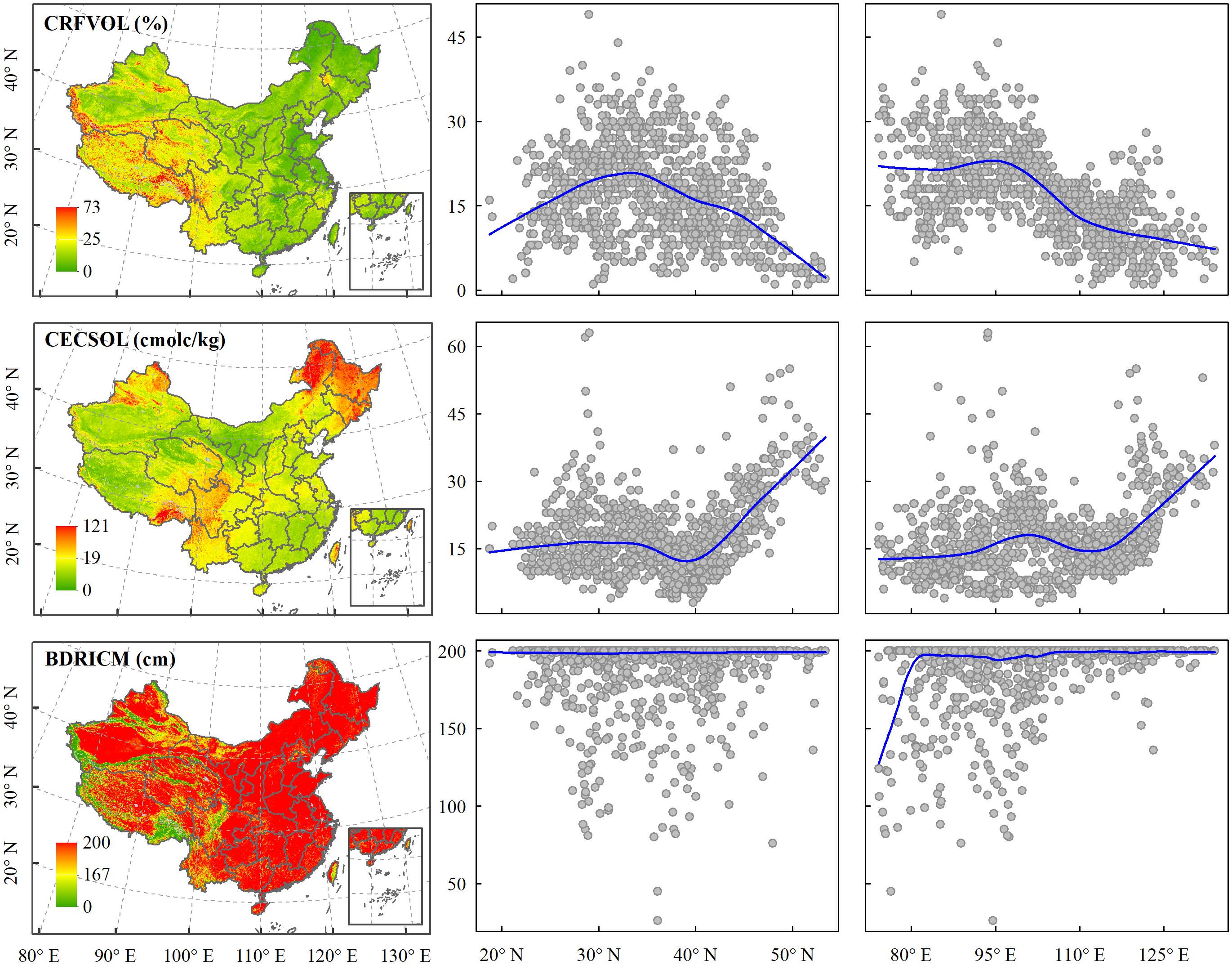
Figure 2. Spatial patterns, latitudinal and longitudinal gradients of the coarse fragment volume (CRFVOL), cation exchange capacity (CECSOL), and depth to the bedrock (BDRICM) at the resolution of 1 km. The scatter plot was drawn based on 992 randomly sampled points.
As expected, results of simple linear regression analysis showed that CRFVOL representing soil texture and elevation range representing habitat heterogeneity are positively related and explained 39.3% and 36.6% of the spatial variation of species richness, respectively. The soil heterogeneity, represented by the standardized deviation of depth to bedrock, and the soil fertility, represented by CECSOL, are positively related to the spatial variations of the species richness with the explanatory power of 31.7% and 23.9%, respectively (Figure 2 and Supplementary Table S2 in Appendix S3). Out of expectation, the environmental energy represented by the mean temperature of the warmest quarter showed the highest but negative effect (adjusted-R2 = 44.75%, modified t-test p < 0.001) on the spatial variation of species richness among all examined variables. Environmental water availability is also negatively correlated with species richness but with a relatively low explanatory power (the best variable PDM adjusted-R2 = 18%, modified t-test p < 0.1).
The multiple regression model, including six variables, can explain 56.7% of species richness variation (Table 2). Hierarchical partitioning analyses showed consistent results with simple LM analyses. MTWQ had the highest independent and conjoint effects on species richness followed by CRFVOL and ELER, representing soil texture and habitat heterogeneity, respectively (Figure 3). Despite relatively low independent effects (<5% of the total R2), we found a high conjoint effect of soil heterogeneity (>10% of the total R2) on species richness (Figure 4).
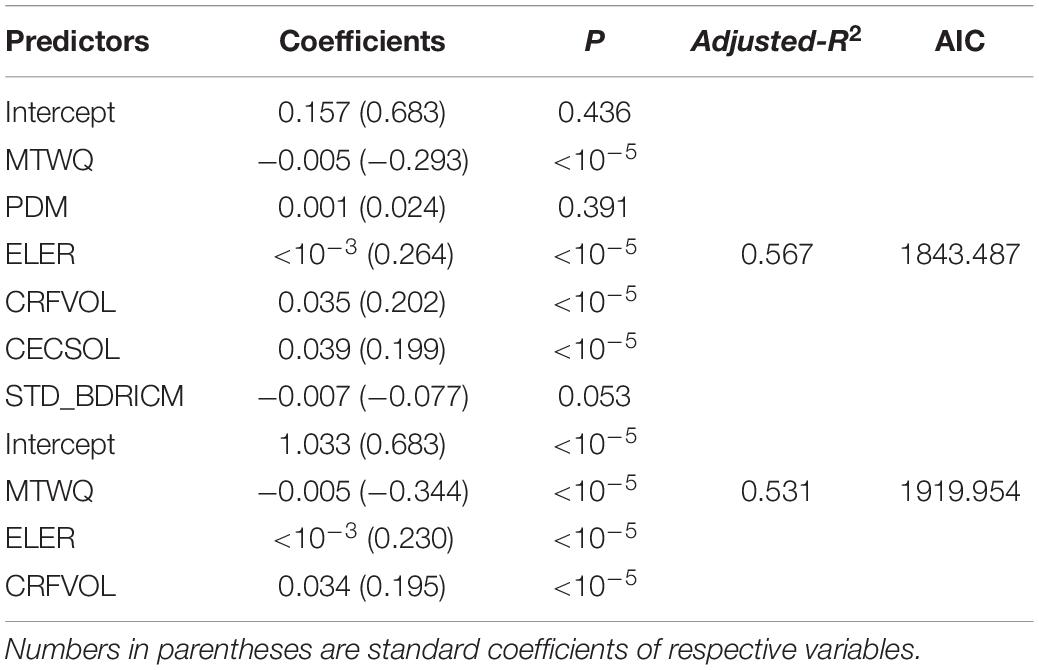
Table 2. The regression coefficients, p-values, and adjusted-R2 of two regression models based on the variables which were used by the hierarchical partitioning analyses and the variance partition analyses, respectively.
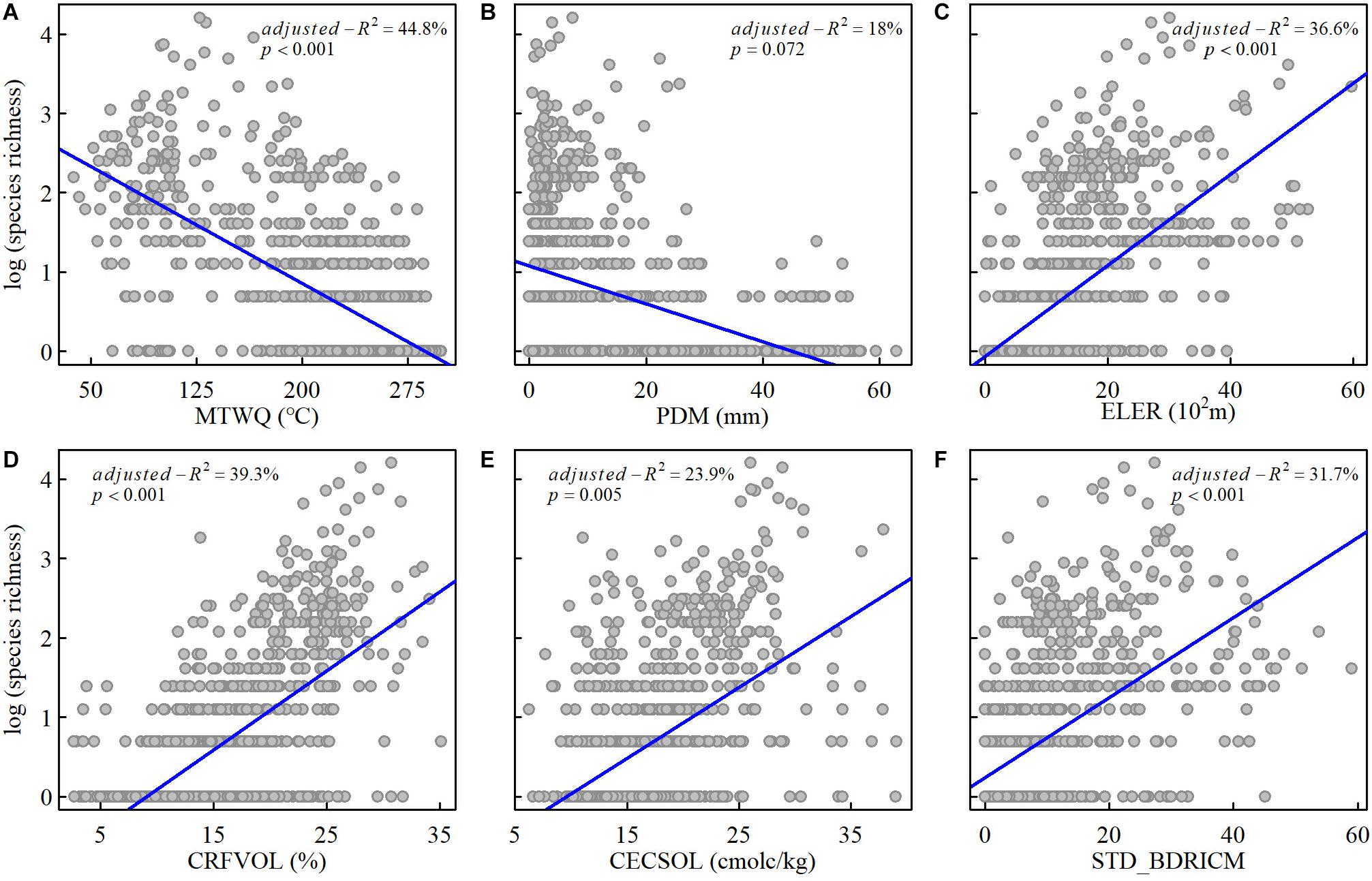
Figure 3. Relationships between the log-transformed species richness of Saxifraga L. in China and mean temperature of warmest quarter (MTWQ) (A), precipitation of driest month (PDM) (B), elevation range (ELER) (C), coarse fragment volume (CRFVOL) (D), cation exchange capacity (CECSOL) (E), and standard deviation of depth to the bedrock (STD_BDRICM) (F), respectively.
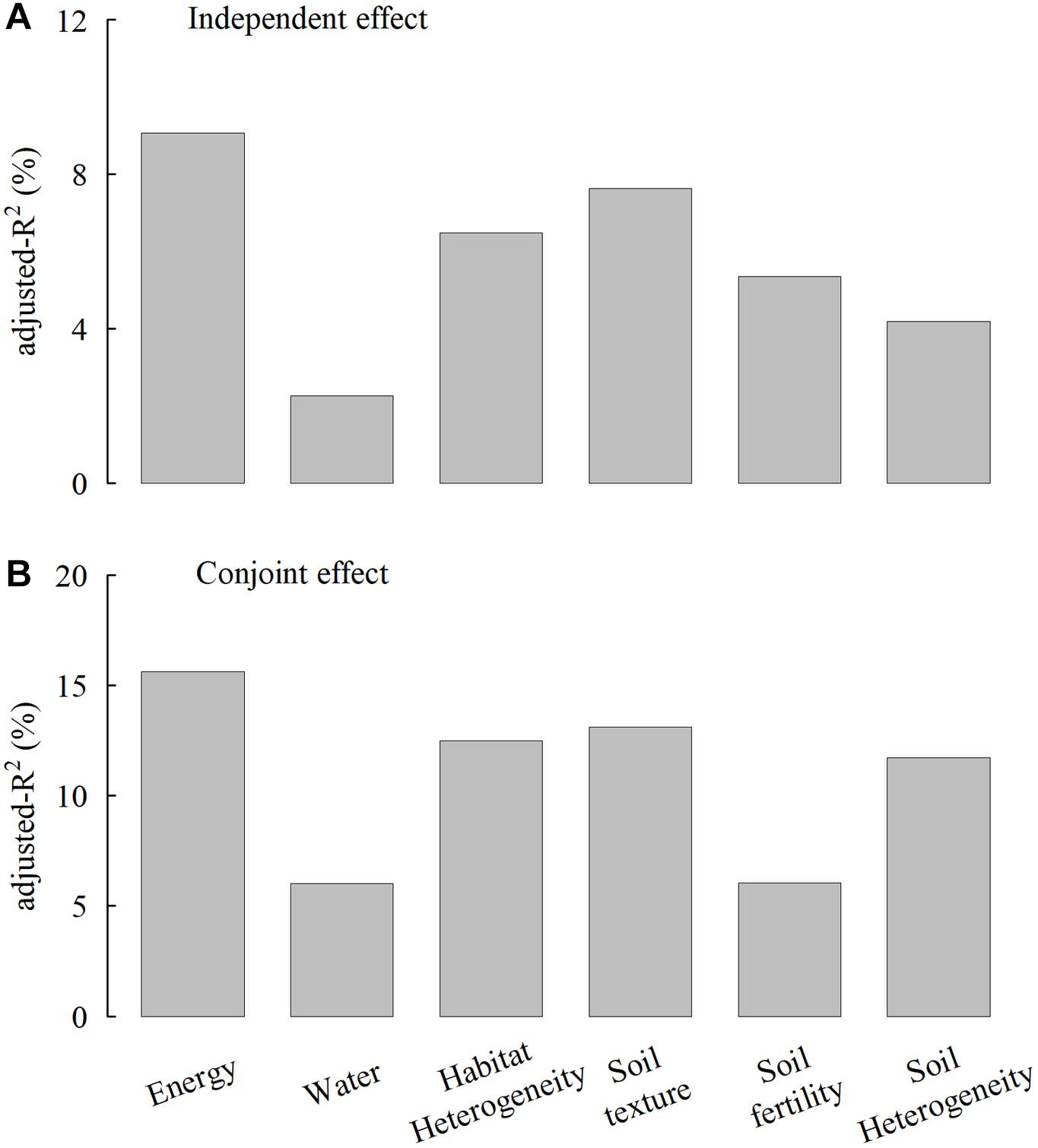
Figure 4. Comparisons of the independent effects (A) and conjunct effects (B) (adjusted-R2, %, given as the percentage of the total explained variance) of six environmental factor groups on the species richness. The adjusted-R2 was estimated based on the hierarchical partitioning method. Energy, water, habitat heterogeneity, soil texture, soil fertility, and soil heterogeneity were represented by the mean temperature of the warmest quarter (MTWQ), precipitation of the driest month (PDM), elevation range (ELER), coarse fragment volume (CRFVOL), cation exchange capacity (CECSOL), and the standard deviation of depth to bedrock (STD_BDRICM).
The multiple regression model, including three variables with the highest independent effect, has almost equal predictive power with the six variable multiple regression model (adjusted-R2 = 53.1%) (Table 2). The results of variance partition analysis showed that the conjoint effects of energy, habitat heterogeneity, and soil texture on Saxifraga species richness were 26% and higher than the independent explanatory power of each variable. The independent effect of MTWQ is the highest but only explains 6% of the species richness variation. The independent effects of elevation range and soil texture were almost equally low to 3% and 2%, respectively. The conjoint effects of energy and soil texture were 8% and higher than that of soil texture and elevation range and that of energy and elevation range (Figure 5).
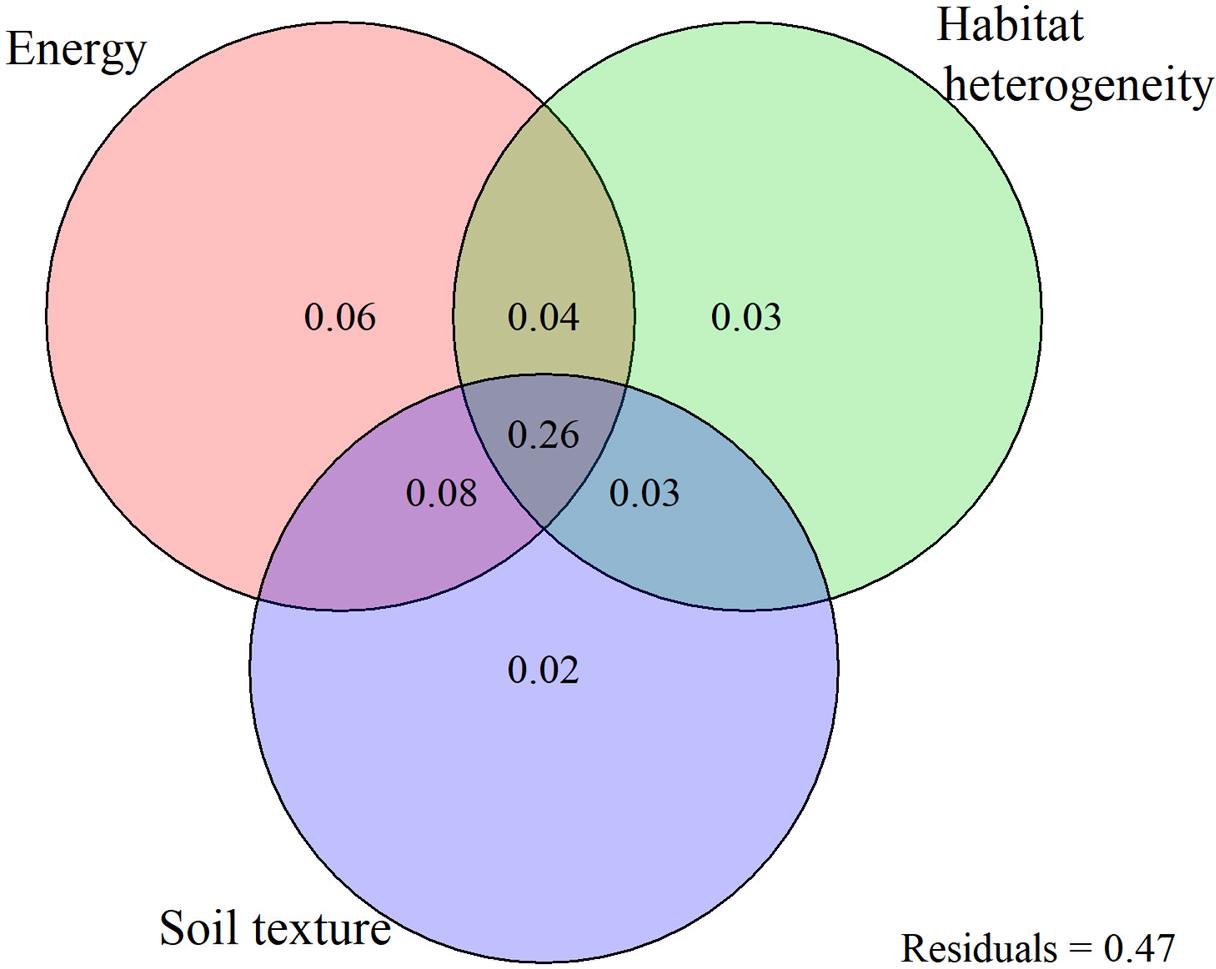
Figure 5. The unique and shared variance of environmental energy, habitat heterogeneity, and soil texture to Saxifraga species richness using the variance partitioning analysis. The proportions of explained variances and the unexplained variance add up to one. Energy, habitat heterogeneity, and soil texture were represented by the mean temperature of the warmest quarter (WTWQ), elevation range (ELER), and coarse fragment volume (CRFVOL), respectively.
Discussion
Our results supported the role of environmental energy, soil texture, and habitat heterogeneity in determining the species richness patterns of the alpine genus Saxifraga in China. With a particular high species richness in HHM regions, the habitat heterogeneity accounted for 36.6% of the species richness variation, which is lower than the explanatory power of the mean temperature of warmest quarter (MTWQ, adjusted-R2 = 44.75%) and the coarse fragments volume of soil (CRFVOL, adjusted-R2 = 39.3%). Results of variance partitioning confirm that environmental energy, soil, and habitat heterogeneity are the primary determinants of the Saxifraga species richness patterns. We further established a negative relationship of energy–alpine species richness and an insignificant relationship of water–species richness, which suggested considerable discrepancy of climate impacts on species richness between alpine herbs and woody plants/lowland regions at large scale (Wang et al., 2009, 2018; Liu et al., 2017). In comparison with other landforms, orogenic dynamics promoted habitat heterogeneity and fostered the distinctive soil properties and novel habitats in mountain regions by surface uplift, runoff, and erosions, thereby resulting in the increase in diversification rate and exceptionally high species richness (Rahbek et al., 2019a).
Habitat Heterogeneity Effects on Alpine Species Richness
Habitat heterogeneity is widely accepted as a primary driver of the high species richness in mountain regions by increasing speciation and decreasing extinction and niche space for species coexistence (Stein et al., 2014; Favre et al., 2016; Ebersbach et al., 2017b). During the uplift of the mountains, habitat heterogeneity increases with the increase in elevation, which raised a large number of sky islands and modified the species dispersal barriers (Hughes and Eastwood, 2006; Ebersbach et al., 2017b). The unique north–south orientation of the Hengduan Mountain and its parallel valleys form numerous and complex ecological niches, which promote the exchange of species in the north–south direction but also strengthen the isolation of species in the east–west direction (Sun et al., 2017). These processes together promoted the allopatric speciation rate in mountain regions. Meanwhile, the increasing elevation range and diversified microclimates and habitats during the mountain building process result in many refugia, which can buffer the species extinction rate when global climate oscillations happened (Qian and Ricklefs, 2000; Ebersbach et al., 2017b). The buffering effect of habitat heterogeneity probably is one reason for the insignificant impact of the historical climate change on species richness in Asia (Wang et al., 2011b; Xu et al., 2019). Furthermore, the increased habitat heterogeneity also offers numerous empty niches for species to occupy and increases the number of coexistence species (Stein et al., 2014). Albeit the previous findings of an insignificant topographic relief effect on species richness in High Asia (Antonelli et al., 2018), our study confirmed the importance of habitat heterogeneity in giving rise to the high diversity in the HHM regions in southwest China, as well as the previous researches for other plant groups (Shrestha et al., 2018a, b).
Soil Effects on Large-Scale Alpine Species Richness
However, in contrast to the well-acknowledged significance of habitat heterogeneity, the striking effects of soil on the high species richness mostly focused on restricted areas or at a local scale (Kutiel and Danin, 1987; Harrison and Rajakaruna, 2011; Escudero et al., 2015; Abdelaal et al., 2019; Kontopanou and Panitsa, 2020). Most studies suggested that soil can take effect on plant species richness at the local scale because of the direct impact on water and nutrient supplies (Huston, 1980; Kammer et al., 2013; Dingaan et al., 2017). The large-scale variation of soil properties might not be able to capture the real effects of soil on plants. However, our results showed that soil effects on species richness at the large scale are almost equal to climate and habitat heterogeneity.
On the one hand, soils derived from the mafic and ultramafic rocks harbor high plant diversity by the radiation of a lineage specialized for such edaphic niche (Rahbek et al., 2019a). For example, serpentine habitat specialists are remarkably rich in California and can adapt to low levels of phosphorus and high content of magnesium. Recent studies have found a high speciation rate and species richness of the Gesneriaceae in the karst limestone mountainous areas in southern China (Liu et al., 2017). Consistent with the above studies, the Saxifraga species are well adapted to rock crevices and showed a higher diversification rate in rock cliffs than in other habitats (de Casas et al., 2016; Ebersbach et al., 2017b). These probably are reasons for the high explanatory power of CRFVOL of soil on species richness in our study.
On the other hand, soil heterogeneity, as one part of habitat heterogeneity, showed consistent positive impacts on species richness in mountain regions by creating the novel habitat and ecological niche (Antonelli et al., 2018). Previous studies found that the physical structure and chemical composition of the cliffs, as well as their relation position, provide more habitats to promote plants’ coexistence (Cooper, 1997). Consistent with these studies, our result also showed that all soil heterogeneity factors are positively correlated with Saxifraga species richness. In our study, all soil heterogeneity factors are positively correlated with elevation range (Spearman’s p range from 0.34 to 0.8), suggesting that the uplift of mountains in the HHM regions increased both the elevation range and soil heterogeneity, thereby contributing to the increase of species richness.
Finally, the significant correlations between soil properties, climate, and elevation range and the high conjoint effect on species richness are probably due to the direct impact of climate and topographic structure on soil development. These also suggested that climate and elevation range can indirectly influence species richness through soils. Although Laliberte et al. (2013) proposed a framework by using the structural equation modeling to explore the soil effects on latitudinal species richness patterns, further study is still needed.
The Negative Effect of Temperature on Alpine Species Richness
Although climate shapes the main patterns of diversity in mountain regions all over the world (Rahbek et al., 2019b), the negative temperature–richness climate has been rarely established and may be the outcome of the evolutionary history of this alpine genus and available cold habitat with the uplift of Hengduan Mountains. Despite the climate-based hypothesis supporting a positive association between species richness with environmental energy (Currie, 1991), the temperature–richness relationship probably depends on the evolutionary history of the specific groups based on niche conservatism hypothesis (Wiens and Donoghue, 2004; Wiens and Graham, 2005; Xu et al., 2013, 2019). Niche conservatism suggested that the positive associations of energy and species richness for all flowering plants are the result of their warm ancestral niche. Due to the tendency of the descendants to occupy a similar niche with their ancestors, most flowering plants cannot adapt to the cold environment (Wiens and Donoghue, 2004). Research on Asian oaks also found that a striking difference in temperature impacts on species richness between subtropical and temperate lineage supported niche conservatism hypothesis (Xu et al., 2013). The phylogenetic study found that Saxifraga originated in a temperate habitat around 74 Mya, e.g., the ancestor of Saxifragaceae and Grossulariaceae had occupied temperate habitat since 81 Mya. With the continuous cooling since Late Paleocene, Saxifraga colonized Europe and the QTP region as global temperatures declines in the Late Eocene and diversified rapidly since Mid-Miocene when continuous climatic cooling and oscillation occurred (Ebersbach et al., 2017a). During these dispersal and colonization processes, niche conservatism probably promoted speciation of Saxifraga by geographic isolation and accumulated high species richness in a cold environment, which is also supported by theoretical simulations (Hua and Wiens, 2013).
Except for niche conservatism, niche divergence might also contribute to a negative richness–temperature relationship. Based on macroevolution analysis, niche lability of most clades in Saxifragales has increased since ca. 5 Mya when climatic cooling and oscillations occurred and showed to be greater in the clade originated in cooler environment. Consequently, the Saxifraga with a cold ancestral habitat developed cold-associated phenotypic traits and adapted to the freezing climate with global cooling through niche divergence. For example, the trichomes of Saxifraga may be helpful in tolerance to drought, high UV radiations, and low temperature at high altitudes (Rawat et al., 2019). Saxifraga cernua growing at 10°C has a higher dark respiration rate than 20°C (Mcnulty and Cummins, 1987). Thereby, the deep time niche conservatism and recent niche divergence to freezing climate together may contribute to the negative richness–temperature relationship.
Conclusion
In summary, our results suggest that environmental energy, soil, and habitat heterogeneity all acted as the essential determinants for the richness patterns of Saxifraga L. in China. Our results might be generalized to other alpine plant groups due to their similar evolutionary history and environmental adaptations. Our study emphasizes the significance of soil in determining the high alpine species richness, which even outperformed the habitat heterogeneity. The negative species richness–temperature relationship might be the result of the cold adaptation of alpine plants, which suggests that climate warming might threaten the species loss of alpine diversity.
Data Availability Statement
The datasets generated for this study are available on request to the corresponding author.
Author Contributions
XX conceived and designed the study. LL, WD, YT, LiZ, LeZ, JW, YX, and CS compiled the data. LL, YX, YT, and CS analyzed the data. XX and LL led the writing. All authors contributed to the writing and agreed to be accountable for all aspects of the work.
Funding
This study was supported by the National Natural Science Foundation of China (31770566), the National Key Research Development Program of China (2017YFC0505200), the Fundamental Research Funds for the Central Universities of China (YJ201721), and Biodiversity Survey, Observation and Assessment Program of Ministry of Ecology and Environment of China.
Conflict of Interest
The authors declare that the research was conducted in the absence of any commercial or financial relationships that could be construed as a potential conflict of interest.
Acknowledgments
We thank Professor Kangshan Mao from Sichuan University for valuable feedback and discussion.
Supplementary Material
The Supplementary Material for this article can be found online at: https://www.frontiersin.org/articles/10.3389/fevo.2020.00244/full#supplementary-material
APPENDIX S1 | Data sources for the compilation of Saxifraga L. distributions.
APPENDIX S2 | Supplementary figures.
APPENDIX S3 | Supplementary tables.
Footnotes
- ^ http://www.geonames.org/
- ^ http://www.efloras.org//
- ^ http://chelsa-climate.org/, version 1.2.
- ^ www.worldclim.org, version 1.4.
- ^ http://www.cgiar-csi.org/
- ^ https://soilgrids.org/, access date: April, 2018.
References
Abdelaal, M., Ahmed, D., Fois, M., Fenu, G., and Bacchetta, G. (2019). Floristic patterns and ecological drivers of sand dune ecosystem along the Mediterranean coast of Egypt. Arid Land Res. Manage. 33, 388–411. doi: 10.1080/15324982.2018.1564147
Antonelli, A., Kissling, W. D., Flantua, S. G., Bermúdez, M. A., Mulch, A., Muellner-Riehl, A. N., et al. (2018). Geological and climatic influences on mountain biodiversity. Nat. Geosci. 11, 718–725. doi: 10.1038/s41561-018-0236-z
Brown, J. H., Gillooly, J. F., Allen, A. P., Savage, V. M., and West, G. B. (2004). Toward a metabolic theory of ecology. Ecology 85, 1771–1789. doi: 10.1890/03-9000
Chambers, J., and Hastie, T. (1992). “Linear models,” in Statistical Models in S, (Pacific Grove, CA: Wadsworth & Brooks/Cole).
Cooper, A. (1997). Plant species coexistence in cliff habitats. J. Biogeogr. 24, 483–494. doi: 10.1111/j.1365-2699.1997.00128.x
Coyle, J. R., and Hurlbert, A. H. (2016). Environmental optimality, not heterogeneity, drives regional and local species richness in lichen epiphytes. Glob. Ecol. Biogeogr. 25, 406–417. doi: 10.1111/geb.12420
Cramer, M. J., and Willig, M. R. (2005). Habitat heterogeneity, species diversity and null models. Oikos 108, 209–218. doi: 10.1111/j.0030-1299.2005.12944.x
Currie, D. J. (1991). Energy and large-scale patterns of animal-and plant-species richness. Am. Nat. 137, 27–49. doi: 10.1086/285144
Currie, D. J., and Francis, A. P. (2004). Regional versus climatic effect on taxon richness in angiosperms: reply to Qian and Ricklefs. Am. Nat. 163, 780–785. doi: 10.1086/383596
de Casas, R. R., Mort, M. E., and Soltis, D. E. (2016). The influence of habitat on the evolution of plants: a case study across Saxifragales. Ann. Bot. 118, 1317–1328. doi: 10.1093/aob/mcw160
Dingaan, M. N. V., Tsubo, M., Walker, S., and Newby, T. (2017). Soil chemical properties and plant species diversity along a rainfall gradient in semi-arid grassland of South Africa. Plant Ecol. Evol. 150, 35–44. doi: 10.5091/plecevo.2017.1260
Du, F. K., Hou, M., Wang, W., Mao, K., and Hampe, A. (2017). Phylogeography of Quercus aquifolioides provides novel insights into the Neogene history of a major global hotspot of plant diversity in southwest China. J. Biogeogr. 44, 294–307. doi: 10.1111/jbi.12836
Dutilleul, P., Clifford, P., Richardson, S., and Hemon, D. (1993). Modifying the t test for assessing the correlation between two spatial processes. Biometrics 49, 305–314.
Ebersbach, J., Muellner-Riehl, A. N., Michalak, I., Tkach, N., Hoffmann, M. H., Röser, M., et al. (2017a). In and out of the Qinghai-Tibet Plateau: divergence time estimation and historical biogeography of the large arctic-alpine genus Saxifraga L. J. Biogeogr. 44, 900–910. doi: 10.1111/jbi.12899
Ebersbach, J., Schnitzler, J., Favre, A., and Muellner-Riehl, A. N. (2017b). Evolutionary radiations in the species-rich mountain genus Saxifraga L. Bmc. Evol. Biol. 17:119. doi: 10.1186/s12862-017-0967-2
Escudero, A., Palacio, S., Maestre, F. T., and Luzuriaga, A. L. (2015). Plant life on gypsum: a review of its multiple facets. Biol. Rev. 90, 1–18. doi: 10.1111/brv.12092
Fang, J., and Yoda, K. (1990). Climate and vegetation in China III water balance and distribution of vegetation. Ecol. Res. 5, 9–23. doi: 10.1007/bf02348460
Favre, A., Michalak, I., Chen, C.-H., Wang, J.-C., Pringle, J. S., Matuszak, S., et al. (2016). Out-of-Tibet: the spatio-temporal evolution of Gentiana (Gentianaceae). J. Biogeogr. 43, 1967–1978. doi: 10.1111/jbi.12840
Gaston, K. J., Williams, P. H., Eggleton, P., and Humphries, C. J. (1995). Large scale patterns of biodiversity: spatial variation in family richness. P. Roy. Soc. Lond. B. Bio. 260, 149–154. doi: 10.1098/rspb.1995.0072
Harrison, S., and Rajakaruna, N. (2011). Serpentine: The Evolution and Ecology of a Model System. Berkeley. Los Angeles, CA: University of California Press.
Hengl, T., de Jesus, J. M., MacMillan, R. A., Batjes, N. H., Heuvelink, G. B., Ribeiro, E., et al. (2014). SoilGrids1km-global soil information based on automated mapping. PLoS One 9:e0105992. doi: 10.1371/journal.pone.0105992
Hengl, T., Mendes de Jesus, J., Heuvelink, G. B., Ruiperez Gonzalez, M., Kilibarda, M., Blagotic, A., et al. (2017). SoilGrids250m: global gridded soil information based on machine learning. PLoS One 12:e0169748. doi: 10.1371/journal.pone.0169748
Hua, X., and Wiens, J. J. (2013). How does climate influence speciation? Am. Nat. 182, 1–12. doi: 10.1086/670690
Hughes, C., and Eastwood, R. (2006). Island radiation on a continental scale: exceptional rates of plant diversification after uplift of the Andes. Proc. Natl. Acad. Sci. U.S.A. 103, 10334–10339. doi: 10.1073/pnas.0601928103
Hughes, C. E., and Atchison, G. W. (2015). The ubiquity of alpine plant radiations: from the andes to the hengduan mountains. New Phytol. 207, 275–282. doi: 10.1111/nph.13230
Huston, M. (1980). Soil nutrients and tree species richness in costa rican forests. J. Biogeogr. 7, 147–157.
Kammer, P. M., Schöb, C., Eberhard, G., Gallina, R., Meyer, R., and Tschanz, C. (2013). The relationship between soil water storage capacity and plant species diversity in high alpine vegetation. Plant Ecol. Divers. 6, 457–466. doi: 10.1080/17550874.2013.783142
Kontopanou, A., and Panitsa, M. (2020). Habitat islands on the Aegean Islands (Greece): elevational gradient of chasmophytic diversity, endemism, phytogeographical patterns and need for monitoring and conservation. Diversity 12:33. doi: 10.3390/d12010033
Kutiel, P., and Danin, A. (1987). Annual-species diversity and aboveground phytomass in relation to some soil properties in the sand dunes of the northern Sharon Plains. Israel. Vegetatio. 70, 45–49.
Laanisto, L., Tamme, R., Hiiesalu, I., Szava-Kovats, R., Gazol, A., and Pärtel, M. (2013). Microfragmentation concept explains non-positive environmental heterogeneity–diversity relationships. Oecologia 171, 217–226. doi: 10.1007/s00442-012-2398-5
Laliberte, E., Grace, J. B., Huston, M. A., Lambers, H., Teste, F. P., Turner, B. L., et al. (2013). How does pedogenesis drive plant diversity? Trends Ecol. Evol. 28, 331–340. doi: 10.1016/j.tree.2013.02.008
Liu, Y., Shen, Z., Wang, Q., Su, X., Zhang, W., Shrestha, N., et al. (2017). Determinants of richness patterns differ between rare and common species: implications for Gesneriaceae conservation in China. Divers. Distrib. 23, 235–246. doi: 10.1111/ddi.12523
Liu, Y., Su, X., Shrestha, N., Xu, X., Wang, S., Li, Y., et al. (2019). Effects of contemporary environment and Quaternary climate change on drylands plant diversity differ between growth forms. Ecography 42, 334–345. doi: 10.1111/ecog.03698
Lundholm, J. T. (2009). Plant species diversity and environmental heterogeneity: spatial scale and competing hypotheses. J. Veg. Sci. 20, 377–391. doi: 10.1111/j.1654-1103.2009.05577.x
Mac Nally, R., and Walsh, C. J. (2004). Hierarchical partitioning public-domain software. Biodivers. Conserv. 13, 659–660. doi: 10.1023/b:bioc.0000009515.11717.0b
Mao, L. F., Chen, S. B., Zhang, J. L., Hou, Y. H., Zhou, G. S., and Zhang, X. S. (2013). Vascular plant diversity on the roof of the world: spatial patterns and environmental determinants. J. Syst. Evol. 51, 371–381. doi: 10.1111/j.1759-6831.2012.00240.x
Mcnulty, A. K., and Cummins, W. R. (1987). The relationship between respiration and temperature in leaves of the arctic plant Saxifraga cernua. Plant Cell Environ. 10, 319–325. doi: 10.1111/j.1365-3040.1987.tb01612.x
Myers, N., Mittermeier, R. A., Mittermeier, C. G., da Fonseca, G. A. B., and Kent, J. (2000). Biodiversity hotspots for conservation priorities. Nature 403, 853–858. doi: 10.1038/35002501
Nally, R. M. (1996). Hierarchical partitioning as an interpretative tool in multivariate inference. Aust. J. Ecol. 21, 224–228. doi: 10.1111/j.1442-9993.1996.tb00602.x
O’Brien, E. M., Field, R., and Whittaker, R. J. (2000). Climatic gradients in woody plant (tree and shrub) diversity: water-energy dynamics, residual variation, and topography. Oikos 89, 588–600. doi: 10.1034/j.1600-0706.2000.890319.x
Peres-Neto, P. R., Legendre, P., Dray, S., and Borcard, D. (2006). Variation partitioning of species data matrices: estimation and comparison of fractions. Ecology 87, 2614–2625. doi: 10.1890/0012-9658(2006)87[2614:vposdm]2.0.co;2
Qian, H., and Ricklefs, R. E. (2000). Large-scale processes and the Asian bias in species diversity of temperate plants. Nature 407, 180–182. doi: 10.1038/35025052
Rahbek, C., Borregaard, M. K., Antonelli, A., Colwell, R. K., Holt, B. G., Nogues-Bravo, D., et al. (2019a). Building mountain biodiversity: geological and evolutionary processes. Science 365, 1114–1119. doi: 10.1126/science.aax0151
Rahbek, C., Borregaard, M. K., Colwell, R. K., Dalsgaard, B., Holt, B. G., Morueta-Holme, N., et al. (2019b). Humboldt’s enigma: what causes global patterns of mountain biodiversity? Science 365, 1108–1113. doi: 10.1126/science.aax0149
Rakotoarinivo, M., Blach-Overgaard, A., Baker, W. J., Dransfield, J., Moat, J., and Svenning, J.-C. (2013). Palaeo-precipitation is a major determinant of palm species richness patterns across Madagascar: a tropical biodiversity hotspot. P. Roy. Soc. B Biol. Sci. 280:20123048. doi: 10.1098/rspb.2012.3048
Rawat, D. S., Uniyal, P., and Chandra, S. (2019). Micromorphology and distribution of trichome in Saxifraga L. species from Western Indian Himalaya and its taxonomic implications. Taiwania 64, 13–22.
Romeo, R., Vita, A., Manuelli, S., Zanini, E., Freppaz, M., and Stanchi, S. (2015). Understanding Mountain soils: A Contribution From Mountain Areas to the International Year of Soils 2015. Rome: FAO.
Rosenzweig, M. L. (1995). Species Diversity in Space and Time. Cambridge, MA: Cambridge University Press.
Shrestha, N., Su, X., Xu, X., and Wang, Z. (2018a). The drivers of high Rhododendron diversity in southwest China: does seasonality matter? J. Biogeogr. 45, 438–447. doi: 10.1111/jbi.13136
Shrestha, N., Wang, Z., Su, X., Xu, X., Lyu, L., Liu, Y., et al. (2018b). Global patterns of Rhododendron diversity: the role of evolutionary time and diversification rates. Glob. Ecol. Biogeogr. 27, 913–924. doi: 10.1111/geb.12750
Stein, A., Gerstner, K., and Kreft, H. (2014). Environmental heterogeneity as a universal driver of species richness across taxa, biomes and spatial scales. Ecol. Lett. 17, 866–880. doi: 10.1111/ele.12277
Sun, H., Zhang, J., Deng, T., and Boufford, D. E. (2017). Origins and evolution of plant diversity in the Hengduan Mountains. China. Plant Divers. 39, 161–166. doi: 10.1016/j.pld.2017.09.004
Svenning, J.-C., Normand, S., and Skov, F. (2006). Range filling in European trees. J. Biogeogr. 33, 2018–2021. doi: 10.1111/j.1365-2699.2006.01630.x
Svenning, J. C., and Skov, F. (2004). Limited filling of the potential range in European tree species. Ecol Lett. 7, 565–573. doi: 10.1111/j.1461-0248.2004.00614.x
Wang, Q., Wu, S., Su, X., Zhang, L., Xu, X., Lyu, L., et al. (2018). Niche conservatism and elevated diversification shape species diversity in drylands: evidence from Zygophyllaceae. Proc. Biol. Sci. 285:20181742. doi: 10.1098/rspb.2018.1742
Wang, Z., Brown, J. H., Tang, Z., and Fang, J. (2009). Temperature dependence, spatial scale, and tree species diversity in eastern Asia and North America. Proc. Natl. Acad. Sci. U.S.A. 106, 13388–13392. doi: 10.1073/pnas.0905030106
Wang, Z., Fang, J., Tang, Z., and Lin, X. (2011a). Patterns, determinants and models of woody plant diversity in China. P. Roy. Soc. B Biol. Sci. 278, 2122–2132. doi: 10.1098/rspb.2010.1897
Wang, Z., Fang, J., Tang, Z., and Lin, X. (2011b). Relative role of contemporary environment versus history in shaping diversity patterns of China’s woody plants. Ecography 34, 1–10. doi: 10.1111/j.1600-0587.2011.06781.x
Wiens, J. J., and Donoghue, M. J. (2004). Historical biogeography, ecology and species richness. Trends Ecol. Evol. 19, 639–644. doi: 10.1016/j.tree.2004.09.011
Wiens, J. J., and Graham, C. H. (2005). Niche conservatism: integrating evolution, ecology, and conservation biology. Annu. Rev. Ecol. Evol. Syst. 36, 519–539. doi: 10.2307/30033815
Xu, X., Wang, Z., Rahbek, C., Lessard, J.-P., Fang, J., and Burns, K. C. (2013). Evolutionary history influences the effects of water-energy dynamics on oak diversity in Asia. J. Biogeogr. 40, 2146–2155. doi: 10.1111/jbi.12149
Xu, X., Dimitrov, D., Shrestha, N., Rahbek, C., Wang, Z., and Jordan, G. (2019). A consistent species richness–climate relationship for oaks across the northern hemisphere. Glob. Ecol. Biogeogr. 28, 1051–1066. doi: 10.1111/geb.12913
Xue, G. (2014). Ancient and Contemporary Geographical Names in China. Shanghai: Lexicographical Publishing House.
Yang, A. M., Wu, R., Li, J. Y., and Guo, W. J. (2013). Chemical constituents of Saxifraga umbellulata. Adv. Mater. Res. 781-784, 2289–2291. doi: 10.4028/www.scientific.net/AMR.781-784.2289
Keywords: alpine, habitat heterogeneity, rock cliff, Saxifraga, soil fertility, soil texture, species richness, temperature
Citation: Liu L, Xu Y, Tang Y, Du W, Shao C, Wu J, Zhao L, Zhang L, Liu J and Xu X (2020) The Importance of Including Soil Properties When Disentangling the Drivers of Species Richness: The Case of the Alpine Genus Saxifraga L. in China. Front. Ecol. Evol. 8:244. doi: 10.3389/fevo.2020.00244
Received: 04 February 2020; Accepted: 06 July 2020;
Published: 31 July 2020.
Edited by:
Meng Yao, Peking University, ChinaCopyright © 2020 Liu, Xu, Tang, Du, Shao, Wu, Zhao, Zhang, Liu and Xu. This is an open-access article distributed under the terms of the Creative Commons Attribution License (CC BY). The use, distribution or reproduction in other forums is permitted, provided the original author(s) and the copyright owner(s) are credited and that the original publication in this journal is cited, in accordance with accepted academic practice. No use, distribution or reproduction is permitted which does not comply with these terms.
*Correspondence: Xiaoting Xu, eGlhb3Rpbmd4dUBzY3UuZWR1LmNu; eGlhb3Rpbmd4dUBwa3UuZWR1LmNu