- 1Department of Botany, School of Natural Sciences, Trinity College Dublin, Dublin, Ireland
- 2School of Biology, Faculty of Biological Sciences, University of Leeds, Leeds, United Kingdom
- 3UK Centre for Ecology & Hydrology, Wallingford, United Kingdom
Debate rages as to whether habitat fragmentation leads to the decline of biodiversity once habitat loss is accounted for. Previous studies have defined fragmentation variously, but research needs to address “fragmentation per se,” which excludes confounding effects of habitat loss. Our study controls for habitat area and employs a mechanistic multi-species simulation to explore processes that may lead some species groups to be more or less sensitive to fragmentation per se. Our multi-land-cover, landscape-scale, individual-based model incorporates the movement of generic species, each with different land cover preferences. We investigate how fragmentation per se changes diversity patterns; within (alpha), between (beta) and across (gamma) patches of a focal-land-cover, and if this differs among species groups according to their specialism and dependency on this focal-land-cover. We defined specialism as the increased competitive ability of specialists in suitable habitat and decreased ability in less suitable land covers compared to generalist species. We found fragmentation per se caused an increase in gamma diversity in the focal-land-cover if we considered all species regardless of focal-land-cover preference. However, critically for conservation, the gamma diversity of species for whom the focal land cover is suitable habitat declined under fragmentation per se. An exception to this finding occurred when these species were specialists, who were unaffected by fragmentation per se. In general, focal-land-cover species were under pressure from the influx of other species, with fragmentation per se leading to a loss of alpha diversity not compensated for by increases in beta diversity and, therefore, gamma diversity fell. The specialist species, which were more competitive, were less affected by the influx of species and therefore alpha diversity decreased less with fragmentation per se and beta diversity compensated for this loss, meaning gamma diversity did not decrease. Our findings help to inform the fragmentation per se debate, showing that effects on biodiversity can be negative or positive, depending on species’ competitive abilities and dependency on the fragmented land cover. Such differences in the effect of fragmentation per se would have important consequences for conservation. Focusing conservation efforts on reducing or preventing fragmentation in areas with species vulnerable to fragmentation.
Introduction
Humans have modified over 75% of the global land area, and the resulting habitat loss and degradation are recognized as the principal drivers of biodiversity declines (IPBES, 2018). A major consequence of landscape modification is that, aside from declines in habitat area, previously large blocks of natural habitats have become fragmented into smaller patches within a matrix of human-modified land-use such as farms and cities (Haddad et al., 2015). It is clear that habitat loss reduces species diversity, simply by shrinking the areas in which species using that habitat can live (MacArthur and Wilson, 1967; Hodgson et al., 2011; Keil et al., 2015). However, the effect of fragmenting habitats is less clear. “Fragmentation per se” (FPS) refers to the effects of fragmentation after taking account of, or in the absence of, habitat loss (Fahrig, 2003). Put another way, if there is no habitat loss, FPS comprises the altered spatial configuration of habitat, such that remaining patches are smaller but more numerous. The conservation literature has been focused on fragmentation in general being detrimental to biodiversity (Lawton et al., 2010; Eigenbrod et al., 2017). However, debate continues as to whether the effect of FPS on biodiversity is always negative (Fletcher et al., 2018a), or whether it is insignificant or positive (Fahrig, 2017; Fahrig et al., 2019). In reality, FPS and loss of habitat are intrinsically linked (Fletcher et al., 2018a). Nonetheless, separating the effects of FPS from those of area loss and assessing under what circumstances FPS leads to higher or lower species diversity are important for conservation decisions, such as restoration of habitat networks (Isaac et al., 2018) and the choices made by land managers about where to focus conservation efforts. Decisions include whether to conserve multiple small or fewer large habitat patches (Tulloch et al., 2016) or to allow activities that may limit loss of habitat, but increase fragmentation (Miller-Rushing et al., 2019). While there has been speculation about the mechanisms by which FPS may have positive or negative effects on biodiversity, these mechanisms require theoretical development and testing.
For example, understanding how positive vs. negative effects of FPS on diversity is driven by species’ characteristics such as ecological specializations and habitat associations will aid decisions about how to manage specific landscapes. It is often assumed that specialist species and those that are positively associated with the habitat that is becoming fragmented should be negatively affected by FPS (Kosydar et al., 2014; Halstead et al., 2019). If studies report a positive effect of FPS on biodiversity, one explanation given is that species richness and abundance of generalists increases with habitat fragmentation, leading to this rise in diversity (Hu et al., 2012). But in 97% of the studies considered in a review by Fahrig (2017), FPS had a positive effect on the landscape-level (gamma) diversity of apparently specialist, rare, or threatened species. This could be because FPS allows for separation of otherwise competing species among habitat patches within the landscape (Ramiadantsoa et al., 2018). However, this mechanism requires more clarity as to the distinction between specialists and generalists. Specialists and generalists are often defined by an association with a particular habitat or with many, respectively, but this association is open to interpretation (Da Silveira et al., 2016). Being a generalist does not mean the species has no habitat preferences (Townsend et al., 2008; Da Silveira et al., 2016). Chetcuti et al. (2019) analyzed the habitat associations of hundreds of beetle species, showing most species had a positive association with several habitats, and only a few species showed a strong restriction to only a narrow range of habitats. It is also often assumed that specialists are more competitive when in a preferred or more suitable habitat compared to generalists, but the generalists are more competitive on average across multiple habitats (Marvier et al., 2004). Here we assess responses to FPS using clear definitions: specialists are more competitive in their suitable habitats than are generalists, but less competitive than the generalists elsewhere, even where the species may share the same habitat preferences within a landscape.
A further issue concerning mechanisms is relating patch-scale effects to landscape-scale impacts of fragmentation. Long-term manipulation experiments usually show that patch attributes typically associated with fragmentation (e.g., reduced patch size) reduce biodiversity at the scale of an individual patch, i.e., alpha-diversity (Haddad et al., 2015; Fletcher et al., 2018a; Damschen et al., 2019). However, it has been suggested that mechanisms identified in patch-scale studies may not extrapolate to negative effects on biodiversity at the landscape scale (Fahrig, 2017). Indeed, at the landscape scale, containing multiple patches of a habitat, Fahrig (2017) reported that different studies find either a neutral or a positive response of biodiversity (gamma-diversity) to FPS (Fahrig, 2017). By contrast, the species-fragmented area relationship suggests that negative effects of FPS should reduce gamma-diversity compared to that predicted by the species-area relationship alone (Hanski et al., 2013). However, patch-scale studies and the modeling that describes the species-fragmented area relationship do not take into consideration mechanisms that can lead to positive effects of fragmentation, such as increased beta-diversity caused by competitive release and higher habitat diversity (Fahrig et al., 2019; Rybicki et al., 2019). These mechanisms may increase beta-diversity and thus lead to overall increases in gamma-diversity with FPS.
It can be difficult to separate the effects of habitat area loss from those of FPS, as highly fragmented habitats are often in smaller patches (Fahrig, 2003). In general, conducting manipulative landscape-scale studies is difficult and it is often impossible to control for habitat area, which results in a confounding of FPS with habitat loss (Fahrig, 2003; Betts et al., 2019). The effects of area can be isolated statistically (De Camargo et al., 2018; Watling et al., 2020), but in these cases, the change in area can swamp any FPS signal (Fahrig, 2003). Theoretical modeling is a useful way to address contested issues where field data are difficult to collect and are subject to confounding variables. To this end, simulation models have been used to study FPS, which represent individual organisms moving across simulated landscapes (Gunton et al., 2017; Rybicki et al., 2019; Thompson et al., 2019). However, these studies are conducted on binary landscapes with the space between the focal-habitat patches (the habitat type of interest), the matrix, being a single, usually highly unsuitable, habitat type. Obviously, binary landscapes are rarely found in nature, and so using binary habitats likely reduces the relevance and applicability of these simulation studies (Fardila et al., 2017). With only a single matrix habitat, one possible mechanism of FPS benefits is lost, that of increased habitat diversity. FPS can increase the diversity of other habitats adjacent to focal-habitat patches, and that in turn can increase the beta diversity of inhabitants of the focal-habitat (Fahrig et al., 2019; Rybicki et al., 2019). By having multiple matrix habitats, edge effects, which are typically considered a negative mechanism of FPS due to modification of edge due to changes in microclimate and increased predation from species outside of the habitat, can have a positive effect (Betts et al., 2019; Fahrig et al., 2019). In this study, we model FPS in terms of a single focal-habitat type, as is standard, but represent the matrix as a mix of different habitat types with randomized fragmentation and area (Bender and Fahrig, 2005). The multiple matrix habitats allow landscape-level effects to arise from species differences in their habitat preferences, thus better reflecting species’ differences in nature (Bollmann et al., 2005; Betts et al., 2014; Brodie and Newmark, 2019; Chetcuti et al., 2019).
We use an individual based model (IBM) to provide a mechanistic assessment of FPS effects on alpha-, beta- and gamma-diversity, by simulating FPS at the landscape scale. We did this with multiple matrix habitats, and for species with differing specializations for, and dependencies on, the different habitats in the landscape. Dependencies are different from specialization as they relate to the habitats the species would do best in and species preference. Specialization on the other hand is if a species is a specialist or generalist. Even if two species had the same preference for habitats, the specialist would be more competitive than the generalist in the preferred habitat, but less so in less preferred habitats. Having created a complex simulation with multiple species and habitats, we keep the individual species simple, to allow clear conclusions to be drawn. We opt to focus the simulation on the interaction of these different species types with the landscape to assess if these would lead to differing effects of FPS on alpha-, beta- and gamma-diversity. We expect a loss in patch-scale alpha-diversity with FPS, at least for species favoring the focal-habitat, both because the area of patches is lower, and because this smaller area should reduce population viability. Conversely, we predict that FPS will cause beta-diversity to increase because FPS allows the persistence of more species among different focal-habitat patches. Furthermore, FPS will increase the edge-to-area ratio and so the degree to which the focal-habitat interfaces with matrix habitats. This will lead to higher beta-diversity of species for whom the focal-habitat has high suitability due to these species having access to a greater variety of secondary habitat, reflecting the different species-specific habitat preferences. This increased edge will also lead to an influx of species for whom the focal-habitat is less suitable. These tourist species, also called vagrants (Magurran, 2004; Rickert et al., 2012), could potentially counter some of the overall loss in alpha-diversity, but lead to higher competition for species dependent on focal-habitat. Gamma-diversity is a product of alpha- and beta-diversity. So, depending on the rate of decline of alpha-diversity compared to the rate of increase in beta-diversity with FPS, gamma-diversity can increase, decrease or stay the same.
Therefore, we hypothesize that, (1) Increasing fragmentation (FPS) of a habitat causes steeper declines in alpha-diversity of species associated with that habitat due to increased competition from an influx of “tourist” species from the matrix, and therefore the gamma-diversity will decrease with FPS. Focal-habitat dependent specialist species, which are more competitive in the focal-habitat they find most suitable, will be better able to hold out against the influx of tourist species. Generalists will be able to utilize more of the landscape and will coexist with the specialists, but will decline with fragmentation. Therefore, we hypothesize that, (2) Declines in alpha-diversity with fragmentation of a habitat (FPS) will be less steep where the species using that habitat divide into specialists and generalists, due to decreased competition, and so gamma-diversity will be either unaffected or increase with FPS. Additionally, we assess whether results are consistent at high (40%) vs. low (10%) levels of focal-habitat cover, testing the fragmentation threshold hypothesis that FPS should only have a negative effect when habitat amount is low (Fahrig, 2017; De Camargo et al., 2018). We include some of the possible factors influencing the impact of FPS, i.e., reduced competition and higher local habitat diversity surrounding fragmented patches. We also partially include edge effects, through the inherent increase in edge with fragmentation, although we do not include edge effects on the micro-climate.
Materials and Methods
We created a multi-species and landscapes IBM simulation to assess the emergent properties arising from multiple individuals and species moving around a landscape containing a high number of patches and habitats (Figure 1). Our IBM was built using NetLogo (v6.0.4) (Wilensky, 1999). The model is a discrete-time, discrete-space model with the landscape being a grid of habitat cells. The simulation parameters were set up, run and the outputs analyzed using R version 3.5 (R Core Team, 2018). We describe the model in the Supplementary Information following the Overview, Design concepts, Details (ODD), protocol for describing individual-based models (Grimm et al., 2006, 2010), but summarize it here.
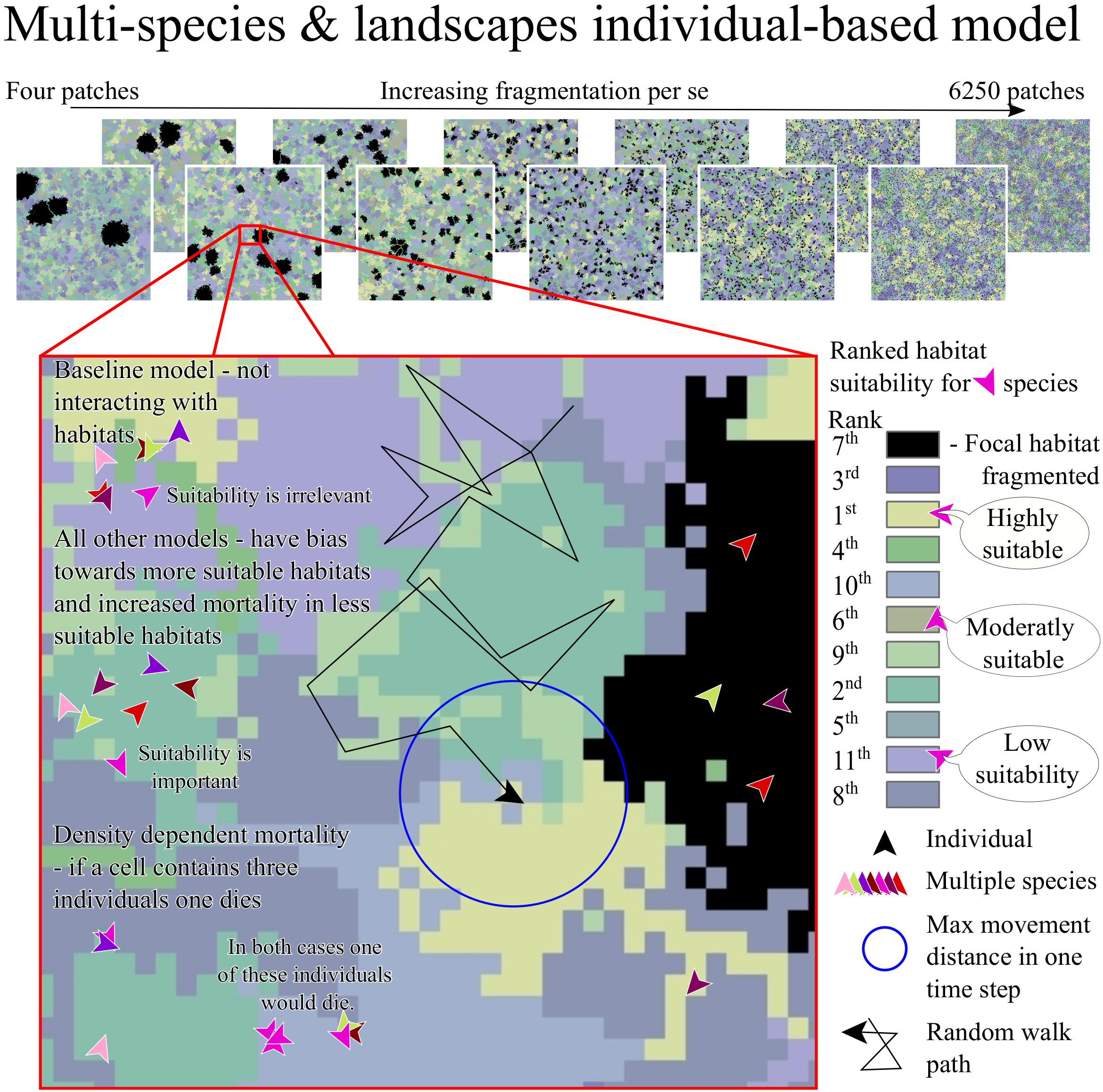
Figure 1. A figurative description of the individual-based model, showing how we represented FPS by increasing the number of patches of the focal-habitat (in black) while keeping its total area the same. We give an example of the ranked suitability for habitats on the right for one species and an example of a random walk in the middle. The simulation used a baseline model, in which the individuals did not have differing mortality or movement bias for different habitats. We simulated two other scenarios in which the individuals interact with the habitats according to their assigned suitability. In the first scenario, the habitat modified mortality and individuals showed biased movement. The second scenario was the same, with the addition that half of the species were specialists and half generalists. We defined specialists and generalists as the former being more competitive in preferred habitats and less competitive in non-preferred habitats compared to generalists.
Generating Habitats and Landscapes
We generated landscapes containing patches of a focal-habitat and other matrix habitats to allow for exploration of FPS without confounding variables such as focal-habitat area loss that is often present in empirical data. We did this by writing an R script (Supplementary Material 2). The simulated landscapes were 1000 × 1000 (=10^6) cells in size and contained habitat patches that were a range of shapes (Figure 1). We generated eleven habitat types: the focal-habitat, and ten others comprising the matrix. In keeping with the known complexity of species habitat associations, we allowed species to have a diversity of associations with and use of habitats within the landscape (Betts et al., 2014; Chetcuti et al., 2019). We generated a new landscape for every model run.
We generated fragmented landscapes by increasing the number of focal-habitat patches – being contiguous cells of the focal-habitat – while keeping the overall, landscape-level area of focal-habitat constant. We increased the number of focal-habitat patches following a geometric series, starting with four patches (allowing calculation of beta-diversity), up to a maximum number of patches. Patches were spatially separated by at least two cells. The maximum number of patches was defined as when the patches were a minimum size of four cells. We considered fragmentation in landscapes where the focal-habitat covered 10% or 40% of the landscape. When the focal-habitat covered 10% the maximum number of patches was 6250. For 40% cover, we used a maximum number of patches of 8192, less than the theoretical maximum, but ensuring computational feasibility. For the focal-habitat, we imposed the number of patches and percentage habitat cover. The patches were located in the landscape by generating random coordinates (seeds) for starting locations using the R package “mobsim” (May et al., 2018). Our program then grew patches around the seeds by selecting adjacent cells at random. Patches grew until the area of the focal-habitat (“habitat one”) reached the required amount (10% or 40% of the total cells in the habitat). The size of each patch was determined by using a uniform distribution allowing for a range of patch sizes. The program repeated the procedure for habitats two to eleven (in a random order) one at a time to fill remaining space. Each matrix habitat had between one and 200 patches and each covered a uniform random proportion of the matrix. This process was sequential; once patches of a habitat could grow no more, then that habitat was considered complete and the next randomly chosen habitat was grown. The last habitat was distributed differently, so that it filled all the remaining matrix.
Multi-Species Landscape Model Description
Our simulation had much in common with other IBMs, such as random-walking species, density-dependence at a cell scale, random distance of movement up to a maximum distance and random starting locations (Fahrig, 2001). To test our hypotheses, the simulation modeled different species types; which were not based on real species but designed to vary realistically in key characteristics while having the same dispersal ability. In the baseline model (which could be considered a type of neutral model) these species were identical, and were defined only by unique identifiers. In the two more complex models, we varied species in terms of their habitat-biased movement and habitat-modified mortality. To do this, we assigned each species a different set of associations with the eleven habitats. We did this by randomly ranking each of the eleven habitats from one (most suitable) to eleven (least suitable), and doing this anew for each species. Preference, dependence, association or ranked suitability, as used in this paper, are simply the rank value a habitat had for a species.
Individuals of each species (see “Modeled scenarios” concerning assignment of individuals to species) moved with a random walk in the baseline scenario and a habitat-biased random walk in the other scenarios (see “Modeled scenarios”). At each time-step the simulation iterated through individuals in random order so that the simulation did not always assess the same individuals first within each time-step. This random order was important when the population was over the carrying capacity and when assessing density-dependent mortality. To simulate density-dependent mortality, if a cell had more than two individuals after the movement phase in each time step, all but two individuals, chosen at random, died. Where movement and mortality were habitat-biased we used a logistic function defined by a midpoint and slope to determine a multiplier between zero and one for each habitat (Figure 2). The multiplier for habitat-modified mortality increased the probability of dying in a time-step for individuals in less suitable habitats. The habitat-biased movement multiplier modified the probability of moving into a cell of a habitat, giving bias toward preferred habitat, but still allowing individuals to move into other habitats. Each individual did this by counting the cells of each habitat in the circle around it defined by the maximum movement distance (Figure 1) and multiplying these by the bias multiplier. Each habitat was then assigned a proportion of values between zero and one and a random number generated between zero and one selected a habitat (Figure 3). The probability of individuals moving to any point in the circle was equal in the baseline model. In the other scenarios with habitat-biased movement, individuals were more likely to choose to move into the habitat more preferred (those with a higher ranking) by their species within the circle around it.
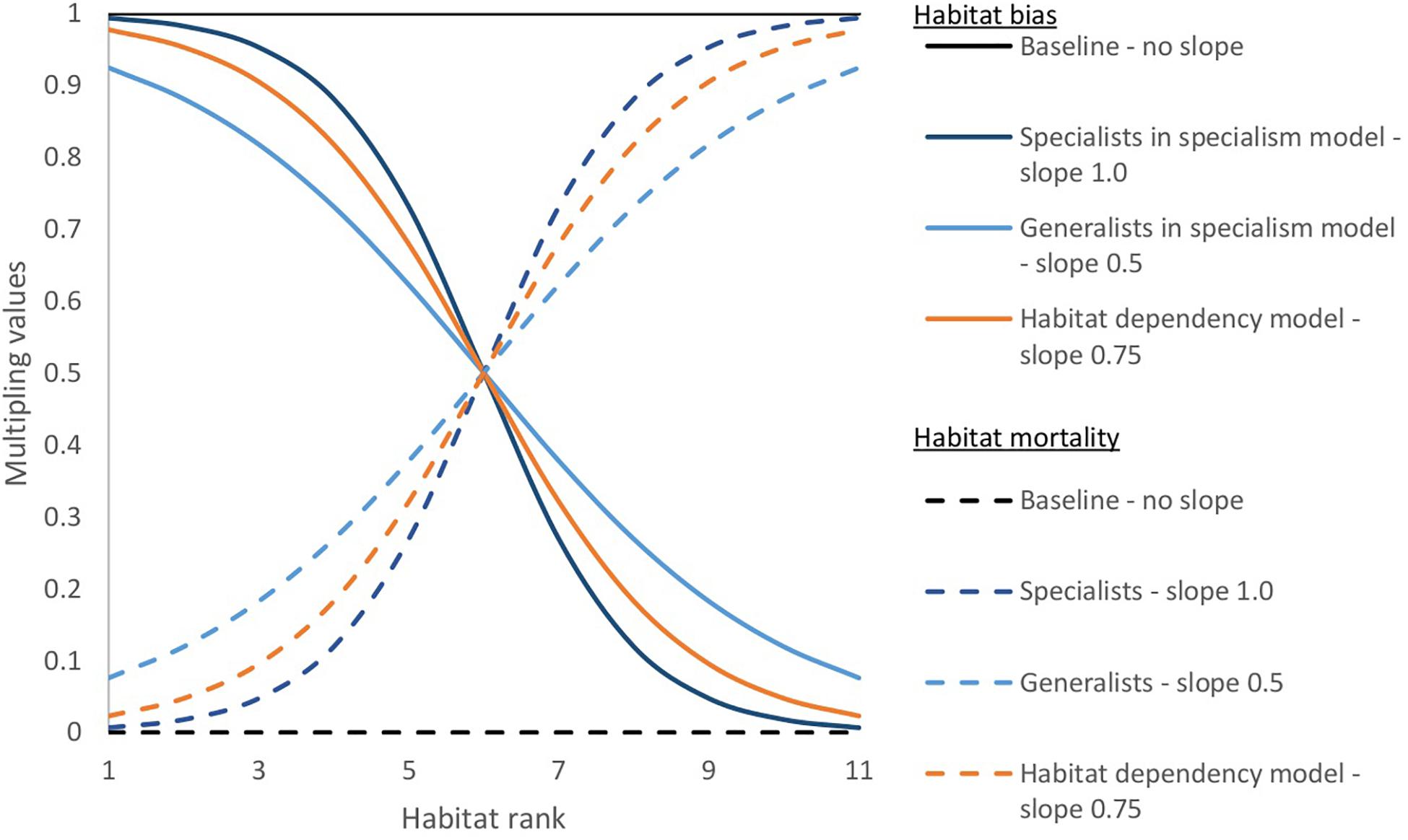
Figure 2. Values used for the logistic slope within each scenario for habitat-biased movement and mortality. The graph shows the effect the slopes have on the multiplying values used to bias the movement toward more suitable habitat and to increase mortality in less suitable habitat. There is mortality due density-dependence and from being over the carrying capacity of the whole simulation. The habitat-modified mortality is additional mortality above the normal levels. To link levels of additional mortality to that of the reproductive rate, the habitat mortality is multiplied by the reproduction rate 5 × 10– 4 to give the additional amount of mortality. We used the same scenarios and values for 10 and 40% cover simulations. The specialist species were more competitive in more suitable habitats than the species in the habitat-dependency model and those more so than the generalists. Competitiveness was reversed in less suitable habitat.
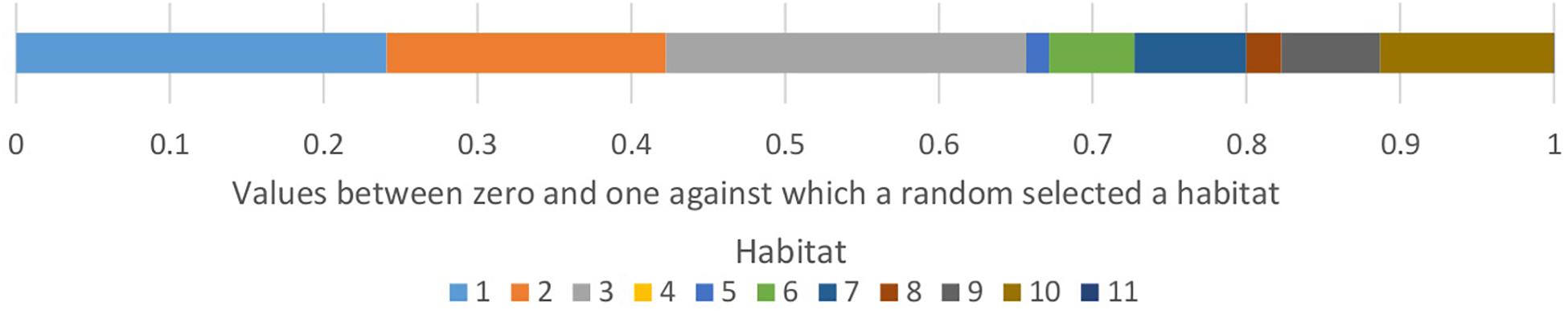
Figure 3. A representation of how each individual chose where to move to in a time-step. It did this by multiplying the proportion of each habitat in a circle around it up to the maximum movement distance, by the bias multiplier. The values were normalized and stacked and then a random number between zero and one was drawn which selected the habitat. The individual then moved to a random cell of that habitat within the maximum movement distance.
We chose a maximum movement distance of individuals of five cells per time-step and 5 × 10–4 chance of reproducing during a time-step. These arbitrary values are realistic for different species. For example, based on allometric equations (Sibly et al., 2013) this could relate to: invertebrates if a cell was a meter and the time-step a minute, resulting in ∼5 m per minute and ∼260 offspring a year (525,600 min in a year × 5 × 10–4 = 260);, or birds or mammals if a cell was a kilometer and the time-step an hour, resulting in ∼5 km per hour and four offspring a year. To stop our simulation from running longer than the 24-h time-limit of the JASMIN HPC cluster LOTUS (Lawrence et al., 2013) we used, we chose a carrying capacity of 4000 individuals in the landscape. We implemented the carrying capacity by increasing the chance of dying for all individuals when numbers were higher than the carrying capacity. We added a bounding area around the edge of the landscape of 10 cells wide with each cell in the area being randomly assigned a different habitat, to prevent species with biased movement from being influenced by the edge of the simulation. This created an invisible edge with individuals remaining or leaving the landscape. Individuals that left the landscape died.
Modeled Scenarios
We generated 400 species per simulation run, starting with ten individuals of each species. In the baseline scenario, all had identical mortalities, fecundities and movement abilities, and no habitat preferences. In the other two models, species’ were given ranked habitat suitabilities as described above, and these were generated anew for each simulation run using the R packages “gtools” (Warnes et al., 2018) to permute the order of the vector 1:11 to give a rank for each habitat and “prodlim” (Gerds, 2018) to avoid repeating a particular ranking for >1 species within a simulation run. For the habitat-dependency model, each species had movement and mortality modified by their habitat suitability (Figure 2). In the specialization scenario, we compared the effect of FPS on specialists and generalists. In this case, we created 200 of each type of species, using the values in Figure 2 for the logistic slope for habitat bias and mortality. Specialist species had a slope value of one, and a higher bias toward more suitable habitats but higher mortality in less suitable habitats than the generalists, which were species with a slope of 0.5.
We carried out preliminary simulation runs using four patches of the focal-habitat. We used these runs to calibrate the model, choosing values for habitat movement bias and modified mortality that allowed the simulation to run for 200,000 model time-steps and from these runs we realized the need to include a carrying capacity to limit the population. This number of time-steps allowed the number of species to reduce to close to the equilibrium number of species (i.e., if the model ran until no more species were lost), and allowed time-efficiency in running multiple models. Each scenario and FPS level combination was repeated 50 times. Seventy-one runs failed due to java issues on the clusters, resulting in the minimum number of replicates being 45.
Alpha-, Beta- and Gamma-Diversity
We calculated diversity scores for the focal-habitat only, habitat one, reflecting our focus on impacts of fragmentation of a single habitat type. At the end of the simulation, we counted species within each patch of the focal-habitat. We then calculated the focal-habitat gamma-diversity, mean alpha-diversity per patch and mean pairwise (between pairs of patches) beta-sim-diversity (Barwell et al., 2015) using the R package “vegan” (Oksanen et al., 2019). We used beta-sim-diversity as it is considered the best metric for presence-absence data and is unaffected by sample size, which could be an issue here as our patches got smaller with FPS and therefore included fewer individuals (Koleff et al., 2003; Barwell et al., 2015). For the habitat-dependency and specialization models, we classified species into three groups: high suitability, those for whom the focal-habitat was highly suitable (rank one to three); low suitability (rank nine to eleven); and moderate suitability (all other species). The moderate suitability was therefore a bigger group and could contain more species.
Analysis of Results
We analyzed the effect of FPS on species diversity using generalized linear models for gamma-diversity (with a Poisson distribution) and alpha-diversity (with a gamma distribution), and beta regression for beta-diversity (“betareg”) (values between zero and one) (Cribari-Neto and Zeileis, 2010). FPS was represented by the number of patches (on a log scale in the case of the beta-diversity, see Supplementary Information). Differences between pairs of scenarios were tested by including both scenarios and creating interaction terms. Due to the simulation nature of our study, using p-values is not advisable as significance can be forced simply running more replicates (White et al., 2014). We instead focus on effect size and 95% confidence intervals. The effect size is usually calculated over an increase of a unit of the independent variable. In our study this would be a single patch but this slight increase is not very informative. It is more appropriate to consider the effect size over the range of FPS simulated. We calculated the effects over the range of FPS using the R package “effects” (Fox, 2003; Fox and Weisberg, 2019).
Results
Considering all species found across the focal-habitat patches, gamma-diversity increased with higher FPS in all models. In the baseline (neutral) model, where habitat did not influence movement or mortality, we observed that individuals became scattered randomly across the landscape. As a result, individual species became more concentrated by chance in different locations through random movement combined with reproduction, and conversely, became vacant from other parts; this resulted in increasing beta-diversity with higher FPS (Supplementary Figure 1). Because the species were equivalent in the baseline model, individual species only went extinct through stochasticity. The gamma-diversity, therefore, remained high after the 200,000-time-steps of the simulation. Although there was a positive effect of FPS on gamma-diversity in the baseline model, the 95% confidence interval of the slope included negative values and the effect size was low (Figure 4). By contrast, the FPS showed an increasing positive effect on gamma-diversity in the habitat dependency and specialization models; increasing gamma-diversity by 2.7 and 4.5 species, respectively, over the full range of fragmentation. In these habitat dependency and specialization models, the mean pairwise beta-diversity between patches increased faster than mean patch alpha-diversity declined with increasing FPS. When the focal-habitat had low FPS, beta-diversity was low, as the few large patches contained similar sets of species. As FPS increased, beta-diversity increased because there were more patches, and these were possibly in different landscape settings that suited different sets of species. By contrast, in the baseline model, the movement and mortality of species did not differ among the habitats, and so the species distributed across the landscape through stochastic processes only. Therefore, more patches in different landscape settings made no difference to the beta-diversity in the baseline model and gamma-diversity only increased slightly due to the increased number of patches sampling more of the landscape (+ 0.69 species). All results were qualitatively the same for high (40%) and low (10%) overall focal-habitat cover (Supplementary Tables 1–3). So, we present results for 10% cover results here, while those for 40% cover are in Supplementary Tables 4–6.
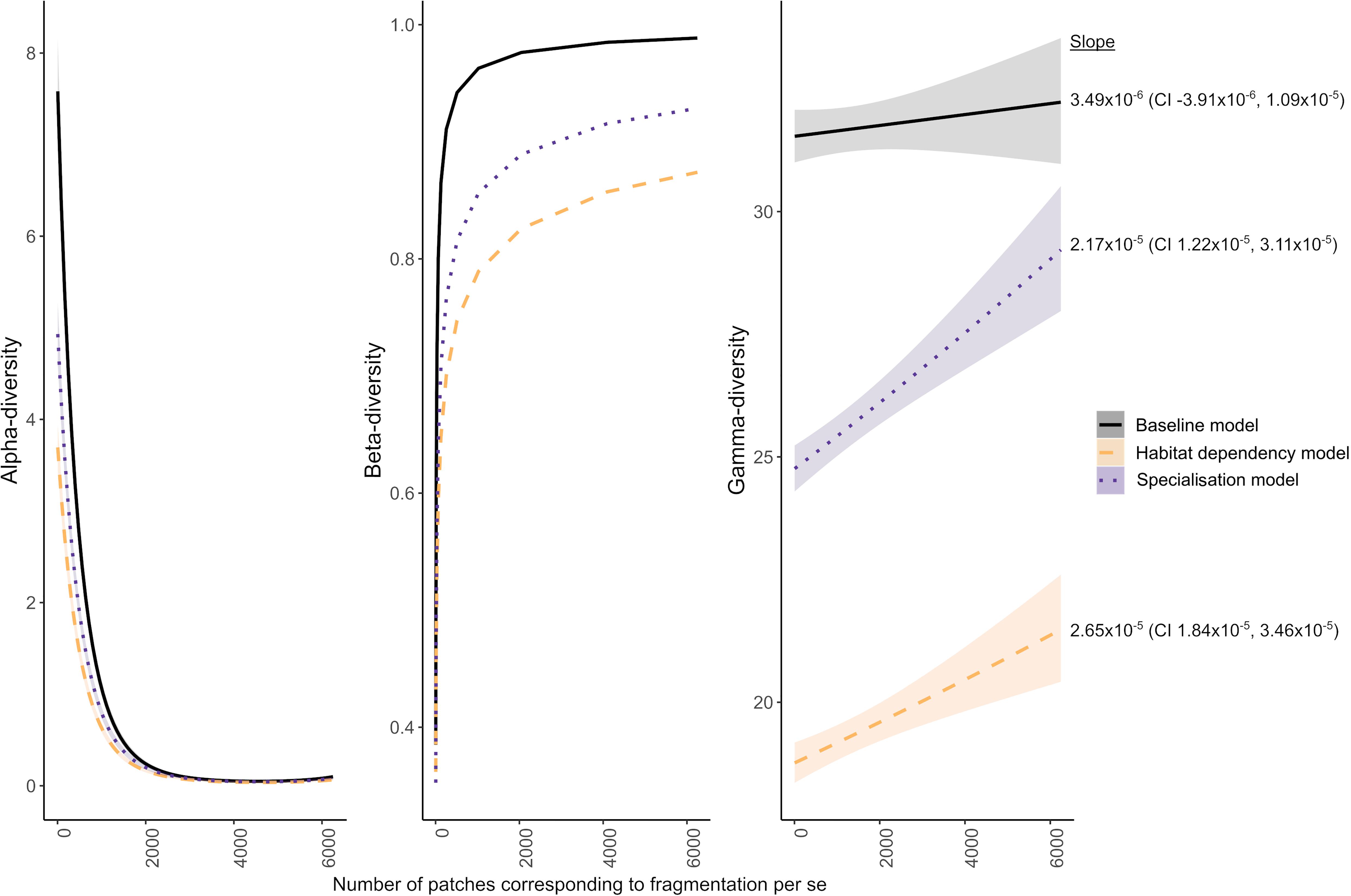
Figure 4. Mean patch scale alpha-diversity, mean pairwise beta-diversity and gamma-diversity for all species in the focal-habitat at 10% cover, with fitted lines and standard errors. Gamma-diversity increased with the number of patches (albeit not greatly for the baseline model), which represents FPS. In all cases, alpha-diversity declined, and beta-diversity increased.
The habitat-dependency and specialization models had differences in habitat-dependent mortality and movement bias among species. This led to lower gamma-diversity values than in the baseline model as the species were more rapidly sorted in space and species less suited overall to the specific landscape of a simulation run died out. In these models, we observed that particular species became concentrated in areas of the landscape through their habitat associations (Supplementary Figure 1). In many cases, a few species dominated single habitat patches. In the specialization model, the gamma-diversity of the specialists and generalists together summed to give a higher overall gamma-diversity than in the simpler habitat-dependency model (Figure 4).
Considering the different species groups in the habitat-dependency model, the focal-habitat gamma-diversity of the species for whom the focal-habitat had low or moderate suitability increased with FPS (Figure 5). This was as expected, as the increased edge-to-area ratio under FPS would mean more of these species moved into focal-habitat patches by chance. Interestingly the gamma-diversity of species for whom the focal-habitat had high suitability declined with FPS. The reduction in gamma-diversity over the whole range of FPS was, 2 species, amounting to a 25% reduction. This reduction was also due to a greater amount of edge with higher FPS. In this case this greater edge meant these species were more likely to leave focal-habitat patches and also to be excluded from these patches by the influx of those species for whom the focal-habitat had low or moderate suitability.
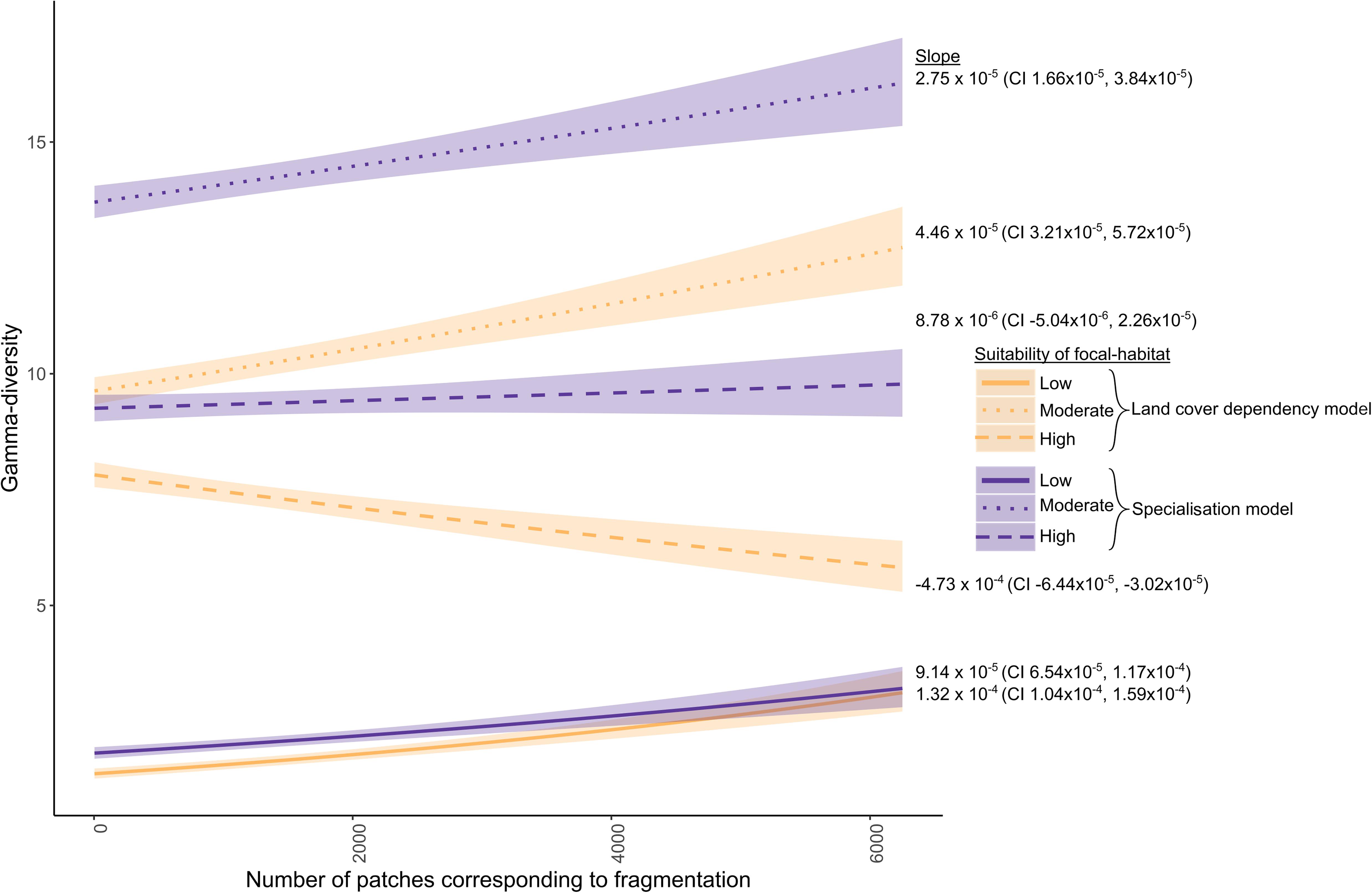
Figure 5. Gamma-diversity for three groups of species – those for whom the focal-habitat had high, moderate, or low suitability – for the habitat dependency model (habitat bias and mortality slope 0.75) and specialization model (habitat bias and mortality slope 1 and 0.5, respectively). Gamma-diversity increased with FPS in both models for the species who for whom the focal-habitat had low or moderate suitability, and those for whom the focal-habitat had high suitability in the specialization model. By contrast, in the habitat dependency model, gamma-diversity declined with increasing FPS for the species for whom the focal-habitat had high suitability.
In the specialization model, the gamma-diversity of the species for whom the focal-habitat had high suitability did not change with FPS. In contrast to the simpler habitat-dependency model, the specialist species were more competitive in habitats to which they were suited, so they were better able to resist species that found the habitat less suitable and their beta-diversity increased at a rate similar or slightly greater than the decline in alpha-diversity, so gamma-diversity did not decline (Figure 6). The generalist species for whom the focal-habitat had high suitability also did better under high FPS than the species in the habitat-dependency model (which had neither specialists nor generalists), as they were able to use more of the wider landscape.
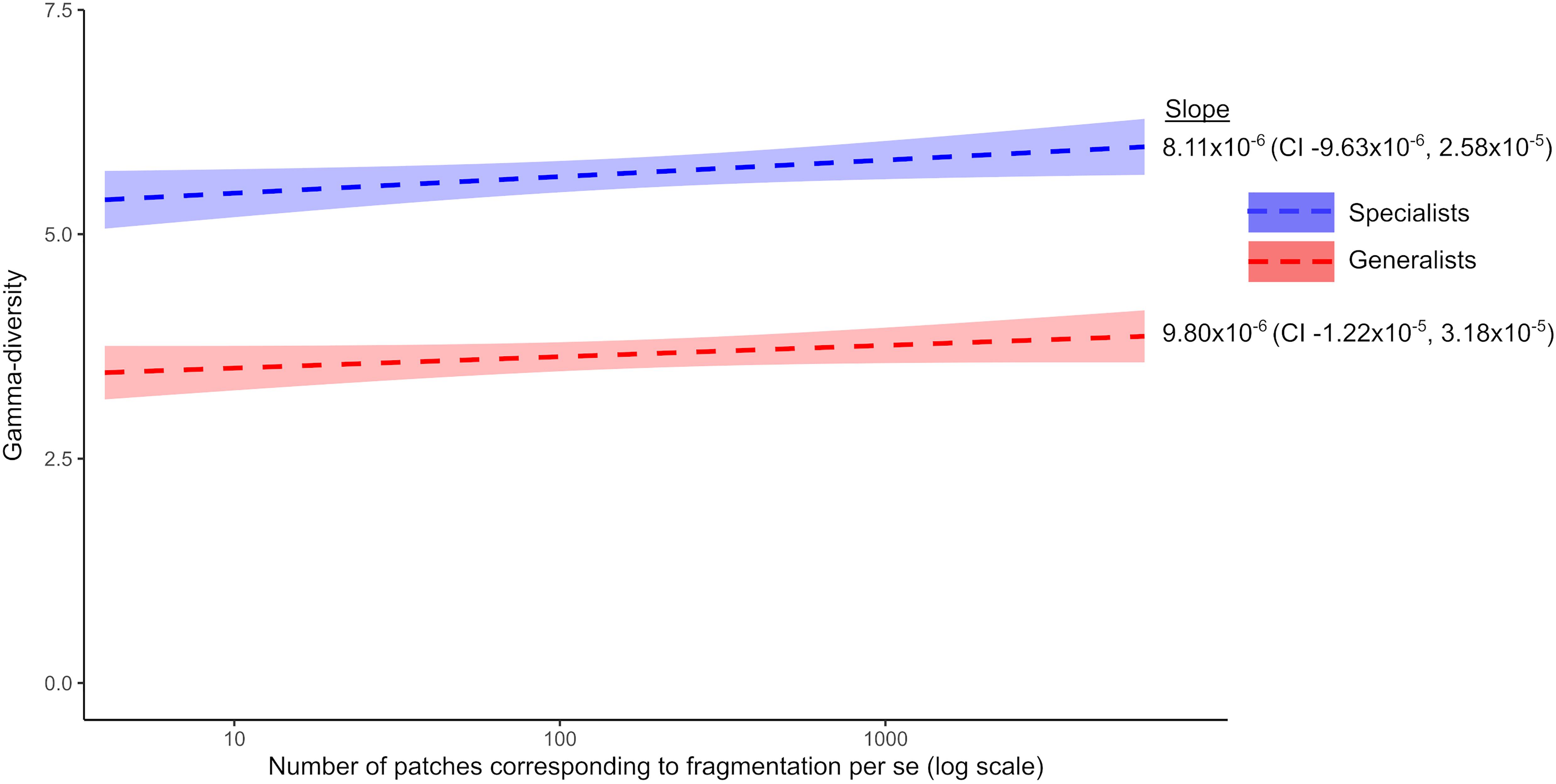
Figure 6. Gamma-diversity for those species for whom the focal-habitat had high suitability for the specialization model (habitat bias and mortality slope 1 and 0.5, respectively) showing specialists and generalists separately. Gamma-diversity increases with FPS for both specialists and generalists in all cases.
Discussion
This study helps to inform the debate on the effects of FPS on biodiversity (Fahrig, 2017; Fletcher et al., 2018a; Fahrig et al., 2019; Thompson et al., 2019). FPS had no effect or a positive effect on overall gamma-diversity of the focal-habitat across the patches in a landscape, but the gamma-diversity of species for which the habitat had high suitability could decline with FPS depending on species characteristics with respect to specialization and competitive ability. Our results were consistent when contrasting landscapes with relatively low (10%) to relatively high (40%) cover by the focal-habitat, suggesting some generality.
We found that beta-diversity and gamma-diversity increased overall even without differences among species in habitat specializations. In the baseline the increase in gamma-diversity was negligible, with a possible small increase caused by patches covering more of the landscape with FPS and increasing sampling of species which were aggregated through limited dispersal as predicted by neutral theory (Hubbell, 2011). Despite overall increases, in the habitat-dependency model, the gamma-diversity of species for whom the focal-habitat was highly suitable declined with FPS, while these species did not decline in the specialization model. In the habitat-dependency model, the species for whom the focal-habitat was highly suitable were likely under pressure due to the influx into the more fragmented patches by species for whom the habitat was less suitable, and the beta-diversity increase of these species suited to the focal-habitat did not outweigh the loss in alpha-diversity, so gamma-diversity declined.
In the specialization model, the specialists were more competitive against other species in the focal-habitat and therefore their beta-diversity increased at a similar rate to the decline in alpha-diversity decline with higher FPS, resulting in no change in the specialist species gamma-diversity with FPS. The gamma-diversity of the generalists did not decline, probably due to competitive release, or as they were better able to use multiple habitats outside of the focal-habitat. These findings indicate that the effect of FPS is context dependent. More competitive (specialist) species did not decline with FPS. But, the gamma-diversity of those species suited to the focal-habitat, but which were not more competitive there (in our habitat-dependency model) did decline with FPS. Such patterns might arise in nature where environmental change could cause species to become less competitive in their preferred habitat and therefore become more affected by FPS. For example, increased nutrients or climate change can change competitive abilities (Staley et al., 2011; Lancaster et al., 2017). Future studies might conduct simulations considering FPS more explicitly with such environmental change. It is often assumed that less competitive species have increased dispersal efficiency (Bonte et al., 2012). For simplicity, we did not include such differences in dispersal ability. If we had, we suggest a negative effect of FPS would be less likely as the generalists with increased dispersal would be able to better utilize other areas of the landscape. This could be explored in future studies.
Habitat dependency and the degree of specialization of species were very important in changing the direction of the relationship of gamma-diversity to FPS, suggesting information on species’ habitat relationships are critical to planning landscape-scale conservation. In terms of conservation, it is often the less competitive species, with high dependencies on specific habitats that are of highest concern and that are the targets for conservation (Carrete et al., 2010; Fletcher et al., 2018b). The effect fragmentation has on these species should, therefore, be assessed and this might determine how the landscape should be managed to conserve these species. Doing so will have consequences for species in other (“matrix”) habitats, however, and the resulting trade-offs should be analyzed and considered. Fragmentation per se creates smaller patches, which have lower mean alpha-diversity as shown in our modeling. Lower alpha-diversity has a negative effect on ecosystem functioning at the patch scale, but beta-diversity has been suggested as important at a larger scale in supporting multiple ecosystem functions (Mori et al., 2018). Our model used a series of static landscapes. Dynamic landscapes could be simulated in different ways, but if we had simulated dynamic landscapes that changed over time, without species arriving at the edge of the model domain, species diversity could not have increased. As patches are removed, moved or shrunk, some species would be extirpated, possibly with a lag (extinction debt). Without new species, the resulting colonization credit (Jackson and Sax, 2010) would go unfulfilled. A further implication of this, is that if fragmentation is happening over a large enough scale or in an isolated landscape, colonization may not be able to counter the debt. If our simulation provided for colonization, we would expect the result of dynamic landscapes to be similar to that of our static landscapes.
We generated species and habitats at random, meaning our results are general and not specific to any real landscape or communities. Our simulations do not show whether any particular species would be retained or, conversely, lost with increasing FPS. We used a large species pool of potential diversity, providing each simulation run with 400 randomly generated species. We also randomly generated the habitat matrix between the patches of the focal-habitat, always having ten other habitats. Given the importance of the intervening habitat matrix in determining what species are in the landscape and how species move between patches (Brodie and Newmark, 2019; Chetcuti et al., 2019), future studies might look at the matrix specifically, non-randomly generating habitat matrices and including different mixes of anthropogenic and semi-natural habitats (Fletcher et al., 2018a). Our baseline model represented movement as a random walk, and we introduced bias based on habitat suitability in the more complex models. In reality, many organisms show complex movement behavior (Gurarie et al., 2016), which is likely to be important in modeling how FPS affects biodiversity and could be a focus of future research. If our results are representative of the ways in which introducing greater ecological complexity (movement, multiple habitat types, habitat dependency and specialization) can affect conclusions about FPS effects on species diversity, then they have important consequences for practical conservation. The habitat dependency model shows fragmentation can have strongly negative effects on (gamma) diversity. But where the biota shows strong division of species into specialists or generalists, gamma-diversity may be unaffected by fragmentation as long as the landscape contains a diversity of habitats. Considering how we define species as specialist or generalist and using analysis of habitat association, conservation efforts could be focused on mitigating fragmentation for those species groups that may be negatively affected by a fragmented landscape. Further, specialization could be looked at from the emergent perspective of species appearing specialist because of the other species in the landscape or the structure of the landscape. In the absence of more competitive species, such as on islands or isolated parts of the landscape, specialist species may utilize a broader range of habitats and benefit further from a heterogeneous landscape of resources and habitats.
This theoretical modeling considered FPS in heterogeneous landscapes, unpicking some of the mechanisms that can cause gamma-diversity to increase or decrease with FPS. Interestingly, we found that FPS could have a positive, negative or no effect on gamma-diversity, suggesting there is no simple answer to the question; is habitat fragmentation good or bad for biodiversity (Fletcher et al., 2018a; Fahrig et al., 2019). Species that were highly suited to the focal-habitat showed declining gamma-diversity with fragmentation, but where we added characteristics separating species into specialists and generalist, both did better under FPS. A key process was species’ movement; for example species suited to the focal-habitat declined with FPS in the habitat-dependency model, as they were unable to hold out against increasing influxes of species for whom the focal-habitat had lower suitability. Our research opens new avenues for research into how species demography and movement in relation to the focal-habitat affect biodiversity responses to FPS. Species’ specializations, habitat preferences and demography in different habitats (Chetcuti et al., 2019) should be taken into consideration when planning conservation as well as considering that under some circumstances FPS may lead to the conservation objectives of increased beta-diversity.
Data Availability Statement
The NetLogo simulation can be found at: https://zenodo.org/badge/latestdoi/289454188 and the R package LcvGen at: https://zenodo.org/badge/latestdoi/205820444. All other supporting data supporting the conclusions of this article will be made available by the authors, without undue reservation.
Author Contributions
JC did the analysis and wrote the first draft of the manuscript. JB wrote parts of the text. JB and WK provided guidance on the building and parameterization of the simulation and provided significant guidance and editing of the manuscript. All authors contributed to the article and approved the submitted version.
Funding
JC was funded by a studentship from the NERC SPHERES Doctoral Training Partnership (NE/L002574/1). This work used the JASMIN at RAL STFC (http://jasmin.ac.uk), operated jointly by the centre of environmental data analysis and the scientific computing department. This facility was funded by NERC.
Conflict of Interest
The authors declare that the research was conducted in the absence of any commercial or financial relationships that could be construed as a potential conflict of interest.
Supplementary Material
The Supplementary Material for this article can be found online at: https://www.frontiersin.org/articles/10.3389/fevo.2020.607619/full#supplementary-material
References
Barwell, L. J., Isaac, N. J. B., and Kunin, W. E. (2015). Measuring β - diversity with species abundance data. J. Anim. Ecol. 84, 1112–1122. doi: 10.1111/1365-2656.12362
Bender, D. J., and Fahrig, L. (2005). Matrix structure obscures the relationship between interpatch movement and patch size and isolation. Ecology 86, 1023–1033. doi: 10.1890/03-0769
Betts, M. G., Fahrig, L., Hadley, A. S., Halstead, K. E., Bowman, J., Robinson, W. D., et al. (2014). A species-centered approach for uncovering generalities in organism responses to habitat loss and fragmentation. Ecography 37, 517–527. doi: 10.1111/ecog.00740
Betts, M. G., Wolf, C., Pfeifer, M., Banks-Leite, C., Arroyo-Rodríguez, V., Ribeiro, D. B., et al. (2019). Extinction filters mediate the global effects of habitat fragmentation on animals. Science 366, 1236–1239. doi: 10.1126/science.aax9387
Bollmann, K., Weibel, P., and Graf, R. F. (2005). An analysis of central Alpine capercaillie spring habitat at the forest stand scale. For. Ecol. Manage. 215, 307–318. doi: 10.1016/j.foreco.2005.05.019
Bonte, D., Van Dyck, H., Bullock, J. M., Coulon, A., Delgado, M., Gibbs, M., et al. (2012). Costs of dispersal. Biol. Rev. 87, 290–312. doi: 10.1111/j.1469-185X.2011.00201.x
Brodie, J. F., and Newmark, W. D. (2019). Heterogeneous matrix habitat drives species occurrences in complex, fragmented landscapes. Am. Nat. 193, 748–754. doi: 10.1086/702589
Carrete, M., Lambertucci, S. A., Speziale, K., Ceballos, O., Travaini, A., Delibes, M., et al. (2010). Winners and losers in human-made habitats: Interspecific competition outcomes in two Neotropical vultures. Anim. Conserv. 13, 390–398. doi: 10.1111/j.1469-1795.2010.00352.x
Chetcuti, J., Kunin, W. E., and Bullock, J. M. (2019). A weighting method to improve habitat association analysis: tested on British carabids. Ecography 42, 1395–1404. doi: 10.1111/ecog.04295
Da Silveira, N. S., Niebuhr, B. B. S., Muylaert, R. D. L., Ribeiro, M. C., and Pizo, M. A. (2016). Effects of land cover on the movement of frugivorous birds in a heterogeneous landscape. PLoS One 11:e0156688. doi: 10.1371/journal.pone.0156688
Damschen, E. I., Brudvig, L. A., Burt, M. A., Fletcher, R. J., Haddad, N. M., Levey, D. J., et al. (2019). Ongoing accumulation of plant diversity through habitat connectivity in an 18-year experiment. Science 365, 1478–1480. doi: 10.1126/science.aax8992
De Camargo, R. X., Boucher-Lalonde, V. V. V., and Currie, D. J. (2018). At the landscape level, birds respond strongly to habitat amount but weakly to fragmentation. Divers. Distrib. 24, 629–639. doi: 10.1111/ddi.12706
Eigenbrod, F., Adams, W., Hill, C., Macgregor, N., Osborne, P., Clarke, D., et al. (2017). A review of large-scale conservation in England, Scotland and Wales. Nat. Engl. Joint Publ. JP019:227.
Fahrig, L. (2001). How much habitat is enough? Biol. Conserv. 100, 65–74. doi: 10.1016/S0006-3207(00)00208-1
Fahrig, L. (2003). Effects of Habitat Fragmentation on Biodiversity. Annu. Rev. Ecol. Evol. Syst. 34, 487–515. doi: 10.1146/annurev.ecolsys.34.011802.132419
Fahrig, L. (2017). Ecological Responses to Habitat Fragmentation Per Se. Annu. Rev. Ecol. Evol. Syst. 48:annurev-ecolsys-110316-122612. doi: 10.1146/annurev-ecolsys-110316-122612
Fahrig, L., Arroyo-Rodríguez, V., Bennett, J. R., Boucher-Lalonde, V., Cazetta, E., Currie, D. J., et al. (2019). Is habitat fragmentation bad for biodiversity? Biol. Conserv. 230, 179–186. doi: 10.1016/j.biocon.2018.12.026
Fardila, D., Kelly, L. T., Moore, J. L., and McCarthy, M. A. (2017). A systematic review reveals changes in where and how we have studied habitat loss and fragmentation over 20 years. Biol. Conserv. 212, 130–138. doi: 10.1016/j.biocon.2017.04.031
Fletcher, R. J., Didham, R. K., Banks-leite, C., Barlow, J., Ewers, R. M., Rosindell, J., et al. (2018a). Is habitat fragmentation good for biodiversity? Biol. Conserv. 226, 9–15. doi: 10.1016/j.biocon.2018.07.022
Fletcher, R. J., Reichert, B. E., and Holmes, K. (2018b). The negative effects of habitat fragmentation operate at the scale of dispersal. Ecology 99, 2176–2186. doi: 10.1002/ecy.2467
Fox, J., and Weisberg, S. (2019). An R Companion to Applied Regression, 3rd Edn. Thousand Oaks CA: Sage.
Gerds, T. A. (2018). prodlim: Product-Limit Estimation for Censored Event History Analysis. Available online at: https://cran.r-project.org/package=prodlim.
Grimm, V., Berger, U., Bastiansen, F., Eliassen, S., Ginot, V., Giske, J., et al. (2006). A standard protocol for describing individual-based and agent-based models. Ecol. Modell. 198, 115–126. doi: 10.1016/j.ecolmodel.2006.04.023
Grimm, V., Berger, U., DeAngelis, D. L., Polhill, J. G., Giske, J., and Railsback, S. F. (2010). The ODD protocol: a review and first update. Ecol. Modell. 221, 2760–2768. doi: 10.1016/j.ecolmodel.2010.08.019
Gunton, R. M., Marsh, C. J., Moulherat, S., Malchow, A.-K. K., Bocedi, G., Klenke, R. A., et al. (2017). Multicriterion trade-offs and synergies for spatial conservation planning. J. Appl. Ecol. 54, 903–913. doi: 10.1111/1365-2664.12803
Gurarie, E., Bracis, C., Delgado, M., Meckley, T. D., Kojola, I., and Wagner, C. M. (2016). What is the animal doing? Tools for exploring behavioural structure in animal movements. J. Anim. Ecol. 85, 69–84. doi: 10.1111/1365-2656.12379
Haddad, N. M., Brudvig, L. A., Clobert, J., Davies, K. F., Gonzalez, A., Holt, R. D., et al. (2015). Habitat fragmentation and its lasting impact on Earth’s ecosystems. Sci. Adv. 1, 1–9. doi: 10.1126/sciadv.1500052
Halstead, K. E., Alexander, J. D., Hadley, A. S., Stephens, J. L., Yang, Z., and Betts, M. G. (2019). Using a species-centered approach to predict bird community responses to habitat fragmentation. Landsc. Ecol 34, 1919–1935. doi: 10.1007/s10980-019-00860-5
Hanski, I., Zurita, G. A., Bellocq, M. I., and Rybicki, J. (2013). Species-fragmented area relationship. Proc. Natl. Acad. Sci. U.S.A. 110, 12715–12720. doi: 10.1073/pnas.1311491110
Hodgson, J. A., Moilanen, A., Wintle, B. A., and Thomas, C. D. (2011). Habitat area, quality and connectivity: striking the balance for efficient conservation. J. Appl. Ecol. 48, 148–152. doi: 10.1111/j.1365-2664.2010.01919.x
Hu, G., Wu, J., Feeley, K. J., Xu, G., and Yu, M. (2012). The effects of landscape variables on the species-area relationship during late-stage habitat fragmentation. PLoS One 7:e0043894. doi: 10.1371/journal.pone.0043894
Hubbell, S. P. (2011). The Unified Neutral Theory of Biodiversity and Biogeography (MPB-32). Princeton: Princeton University Press.
IPBES (2018). “The IPBES assessment report on land degradation and restoration,” in Secretariat of the Intergovernmental Science-Policy Platform on Biodiversity and Ecosystem Services, eds L. Montanarella, R. Scholes, and A. Brainich (Bonn: IPBES).
Isaac, N. J. B., Brotherton, P. N. M., Bullock, J. M., Gregory, R. D., Boehning-Gaese, K., Connor, B., et al. (2018). Defining and delivering resilient ecological networks: Nature conservation in England. J. Appl. Ecol. 55, 2537–2543. doi: 10.1111/1365-2664.13196
Jackson, S. T., and Sax, D. F. (2010). Balancing biodiversity in a changing environment: extinction debt, immigration credit and species turnover. Trends Ecol. Evol. 25, 153–160. doi: 10.1016/j.tree.2009.10.001
Keil, P., Storch, D., and Jetz, W. (2015). On the decline of biodiversity due to area loss. Nat. Commun. 6:8837. doi: 10.1038/ncomms9837
Koleff, P., Gaston, K. J., and Lennon, J. J. (2003). Measuring beta diversity for presence-absence data. J. Anim. Ecol. 72, 367–382. doi: 10.1046/j.1365-2656.2003.00710.x
Kosydar, A. J., Conquest, L. L., and Tewksbury, J. J. (2014). Can life histories predict the effects of habitat fragmentation? a meta-analysis with terrestrial mammals. Appl. Ecol. Environ. Res. 12, 505–521. doi: 10.15666/aeer/1202_505521
Lancaster, L. T., Morrison, G., and Fitt, R. N. (2017). Life history trade-offs, the intensity of competition, and coexistence in novel and evolving communities under climate change. Philos. Trans. R. Soc. B Biol. Sci. 372:20160046. doi: 10.1098/rstb.2016.0046
Lawrence, B. N., Bennett, V. L., Churchill, J., Juckes, M., Kershaw, P., Pascoe, S., et al. (2013). “Storing and manipulating environmental big data with JASMIN,” in Proceedings of the 2013 IEEE International Conference on Big Data, Big Data 6-9 2013, San Francisco, CA, 68–75. doi: 10.1109/BigData.2013.6691556
Lawton, J. H., Brotherton, P. N. M., Brown, V. K., Elphick, C., Fitter, A. H., Forshaw, J., et al. (2010). Making space for nature: a review of England’s wildlife Sites and ecological network. Rep. to Defra 107.
MacArthur, R. H., and Wilson, E. O. (1967). The Theory of Island Biogeography. Princeton: Princeton University Press.
Magurran, A. (2004). Measuring Biological Diversity. Hoboken, NJ: Wiley, doi: 10.1017/CBO9781107415324.004
Marvier, M., Kareiva, P., and Neubert, M. G. (2004). Habitat destruction, fragmentation, and disturbance promote invasion by habitat generalists in a multispecies metapopulation. Risk Anal. 24, 869–878. doi: 10.1111/j.0272-4332.2004.00485.x
May, F., Gerstner, K., and McGlinn, D. (2018). MoBiodiv/mobsim: first mobsim release (Version v0.1.1). bioRxiv[Preprint]. doi: 10.1101/209502
Miller-Rushing, A. J., Primack, R. B., Devictor, V., Corlett, R. T., Cumming, G. S., Loyola, R., et al. (2019). How does habitat fragmentation affect biodiversity? A controversial question at the core of conservation biology. Biol. Conserv. 232, 271–273. doi: 10.1016/j.biocon.2018.12.029
Mori, A. S., Isbell, F., and Seidl, R. (2018). β-Diversity, community assembly, and ecosystem functioning. Trends Ecol. Evol. 33, 549-564. doi: 10.1016/j.tree.2018.04.012
Oksanen, J., Blanchet, F. G., Friendly, M., Kindt, R., Legendre, P., McGlinn, D., et al. (2019). vegan: Community Ecology Package. Available online at: https://cran.r-project.org/package=vegan.
R Core Team. (2018). R: A Language and Environment for Statistical Computing. Vienna: R Foundation for Statistical Computing.
Ramiadantsoa, T., Hanski, I., and Ovaskainen, O. (2018). Responses of generalist and specialist species to fragmented landscapes. Theor. Popul. Biol. 124, 31–40. doi: 10.1016/j.tpb.2018.08.001
Rickert, C., Fichtner, A., van Klink, R., and Bakker, J. P. (2012). α- and β-Diversity in moth communities in salt marshes is driven by grazing management. Biol. Conserv. 146, 24–31. doi: 10.1016/j.biocon.2011.11.024
Rybicki, J., Abrego, N., and Ovaskainen, O. (2019). Habitat fragmentation and species diversity in competitive communities. Ecol. Lett. 23, 506-517. doi: 10.1111/ele.13450
Sibly, R. M., Grimm, V., Martin, B. T., Johnston, A. S. A., Kulakowska, K., Topping, C. J., et al. (2013). Representing the acquisition and use of energy by individuals in agent-based models of animal populations. Methods Ecol. Evol. 4, 151–161. doi: 10.1111/2041-210x.12002
Staley, J. T., Stafford, D. B., Green, E. R., Leather, S. R., Rossiter, J. T., Poppy, G. M., et al. (2011). Plant nutrient supply determines competition between phytophagous insects. Proc. R. Soc. B Biol. Sci. 278, 718–724. doi: 10.1098/rspb.2010.1593
Thompson, S. E. D., Chisholm, R. A., and Rosindell, J. (2019). Characterising extinction debt following habitat fragmentation using neutral theory. Ecol. Lett. 22, 2087–2096. doi: 10.1111/ele.13398
Townsend, C. R., Begon, M., and Harper, J. L. (2008). Essentials of Ecology, 3rd Edn. Hoboken, NJ: Blackwell Publishing.
Tulloch, A. I. T. T., Barnes, M. D., Ringma, J., Fuller, R. A., and Watson, J. E. M. M. (2016). Understanding the importance of small patches of habitat for conservation. J. Appl. Ecol. 53, 418–429. doi: 10.1111/1365-2664.12547
Warnes, G. R., Bolker, B., and Lumley, T. (2018). gtools: Various R Programming Tools. Available online at: https://cran.r-project.org/package=gtools.
Watling, J., Arroyo-Rodríguez, V., Pfeifer, M., Baeten, L., Banks-Leite, C., Cisneros, L., et al. (2020). Support for the habitat amount hypothesis from a global synthesis of species density studies. Ecol. Lett. 23, 674-681.
White, J. W., Rassweiler, A., Samhouri, J. F., Stier, A. C., and White, C. (2014). Ecologists should not use statistical significance tests to interpret simulation model results. Oikos 123, 385–388. doi: 10.1111/j.1600-0706.2013.01073.x
Wilensky, U. (1999). NetLogo. http://ccl.northwestern.edu/netlogo/. Evanston, IL: Center for Connected Learning and Computer-Based Modeling, Northwestern University.
Keywords: species diversity, fragmentation per se, individual-base model, specialisation, habitat association, habitat preference, movement ecology, simulation
Citation: Chetcuti J, Kunin WE and Bullock JM (2020) Habitat Fragmentation Increases Overall Richness, but Not of Habitat-Dependent Species. Front. Ecol. Evol. 8:607619. doi: 10.3389/fevo.2020.607619
Received: 17 September 2020; Accepted: 30 November 2020;
Published: 17 December 2020.
Edited by:
John Maxwell Halley, University of Ioannina, GreeceReviewed by:
Alessandro Chiarucci, University of Bologna, ItalyAthanasios Kallimanis, Aristotle University of Thessaloniki, Greece
Copyright © 2020 Chetcuti, Kunin and Bullock. This is an open-access article distributed under the terms of the Creative Commons Attribution License (CC BY). The use, distribution or reproduction in other forums is permitted, provided the original author(s) and the copyright owner(s) are credited and that the original publication in this journal is cited, in accordance with accepted academic practice. No use, distribution or reproduction is permitted which does not comply with these terms.
*Correspondence: Jordan Chetcuti, Y2hldGN1dGpAdGNkLmll