- 1Biology Department, Woods Hole Oceanographic Institution, Woods Hole, MA, United States
- 2National Oceanic and Atmospheric Administration (NOAA), Northeast Fisheries Science Center, Woods Hole, MA, United States
- 3Applied Ocean Physics and Engineering Department, Woods Hole Oceanographic Institution, Woods Hole, MA, United States
Animal biodiversity in the ocean’s vast mesopelagic zone is relatively poorly studied due to technological and logistical challenges. Environmental DNA (eDNA) analyses show great promise for efficiently characterizing biodiversity and could provide new insight into the presence of mesopelagic species, including those that are missed by traditional net sampling. Here, we explore the utility of eDNA for identifying animal taxa. We describe the results from an August 2018 cruise in Slope Water off the northeast United States. Samples for eDNA analysis were collected using Niskin bottles during five CTD casts. Sampling depths along each cast were selected based on the presence of biomass as indicated by the shipboard Simrad EK60 echosounder. Metabarcoding of the 18S V9 gene region was used to assess taxonomic diversity. eDNA metabarcoding results were compared with those from net-collected (MOCNESS) plankton samples. We found that the MOCNESS sampling recovered more animal taxa, but the number of taxa detected per liter of water sampled was significantly higher in the eDNA samples. eDNA was especially useful for detecting delicate gelatinous animals which are undersampled by nets. We also detected eDNA changes in community composition with depth, but not with sample collection time (day vs. night). We provide recommendations for applying eDNA-based methods in the mesopelagic including the need for studies enabling interpretation of eDNA signals and improvement of barcode reference databases.
Introduction
The ocean’s mesopelagic zone is poorly explored, in large part due to the vastness of the habitat and the technological and logistical challenges in accessing it. Recently, it has been discovered that mesopelagic biomass is significantly greater than previously thought (Irigoien et al., 2014; St. John et al., 2016); however, there is significant uncertainty about the composition of mesopelagic biomass. Deep pelagic waters, including the mesopelagic, likely contain numerous undescribed species (Robison, 2004, 2009). Traditional sampling nets may miss important taxa such as delicate gelatinous species that fall apart when collected, or fish that avoid capture altogether. A recent genetic study suggested that species diversity is significantly underestimated (Sommer et al., 2017), and genetic analysis has shown that many nominal species may consist of multiple cryptic species, sometimes even belonging to different families (Lindsay et al., 2017).
Mesopelagic species are under immediate risk for exploitation as it is a potential source of fish meal and nutraceuticals (St. John et al., 2016; Hidalgo and Browman, 2019), but our lack of knowledge about their distribution, life history, and ecology impedes sustainable management (Webb et al., 2010; St. John et al., 2016; Glover et al., 2018; Hidalgo and Browman, 2019). The consequences of overharvesting are potentially severe and global in nature. Mesopelagic fish and invertebrates play a key role in the biological carbon pump, which transfers carbon from surface production to the deep sea where it is sequestered (Giering et al., 2014). Anthropogenic changes to mesopelagic biodiversity and biomass could alter this process. Thus, it is urgent to improve the understanding of the composition, distribution, and abundance of mesopelagic fauna before irreparable anthropogenically induced changes occur (St. John et al., 2016; Glover et al., 2018; Hidalgo and Browman, 2019).
New tools are emerging that will facilitate scientific study of this region. One of these is the analysis of environmental DNA (eDNA), which is DNA that is present in the environment that, for metazoans, is no longer associated with the organisms from which it originated (reviewed in Ruppert et al., 2019). In eDNA analyses, water is collected and filtered, the eDNA is extracted from the filter, and one or more DNA markers are analyzed, typically through qPCR, or metabarcoding. There are many potential benefits of eDNA animal biodiversity assessments relative to traditional assessments, including cost effectiveness (Sigsgaard et al., 2015; Thomsen and Willerslev, 2015; Evans et al., 2017) and improved detection of species that are rare or are difficult to sample by traditional means (Dejean et al., 2012; Piaggio et al., 2014; Kelly et al., 2017; Closek et al., 2019; Stat et al., 2019).
The use of eDNA analyses is still in its infancy and there is no standard protocol that is universally applicable to any given system (Deiner et al., 2017; Ruppert et al., 2019). eDNA sampling at meaningful spatial and temporal scales in mesopelagic waters, as opposed to coastal and freshwater systems where most eDNA work has been done, may be especially challenging due to the enormity of the environment and the difficulty in accessing it. In marine coastal regions, samples are taken using Niskin bottles, often mounted on a CTD rosette, and sample volumes are typically around one liter (Andruszkiewicz et al., 2017b; Djurhuus et al., 2017). For the mesopelagic, an adaptive sampling approach that targets sampling locations based on remotely sensed information from shipboard acoustics could be beneficial for targeting concentrations of mesopelagic organisms such as in deep scattering layers (Kinzer, 1969; Orlowski, 1990; Hazen and Johnston, 2010; D’Elia et al., 2016). Multi-frequency and broadband acoustic approaches would detect the greatest variety of organisms over a range of depths (Lavery et al., 2007; Davison et al., 2015; Bassett et al., 2020), as acoustic scattering by organisms is frequency-dependent and frequencies vary in the depth that they penetrate.
The goal of this work was to explore the collection and utility of eDNA for surveying and characterizing metazoan biodiversity in the mesopelagic zone, in order to better understand the composition of this region’s biomass. We used an adaptive sampling approach based on acoustic backscatter (18, 38, 120, and 200 kHz frequencies) to target layers of organisms in daytime and nighttime depth-stratified sampling of eDNA (via Niskin bottles) and zooplankton (via MOCNESS nets). We conducted DNA metabarcoding on all samples using the 18S V9 barcode gene (Amaral-Zettler et al., 2009) to enable detection of a broad range of taxa above the species-level (i.e., genus or family), shedding light on the efficiency of both approaches for detecting animal taxa.
Materials and Methods
Environmental DNA Sample Collection and Shipboard Processing
Seawater samples were collected during a cruise on the NOAA Ship Henry B. Bigelow between the 2,000 and 3,000 m isobaths off the shelf break south of the island of Martha’s Vineyard in Massachusetts, United States (Figure 1). Samples were collected during casts of a Seabird 911 plus CTD mounted on a rosette frame with eight 5 l Niskin bottles. Niskin bottles were triggered to sample based on the layers identified in real time with the shipboard Simrad EK60 echosounder whose split-beam transducers (11° beam width for the 18 kHz and 7° beam width for the 38, 120, and 200 kHz) were mounted on a retractable keel on the vessel.
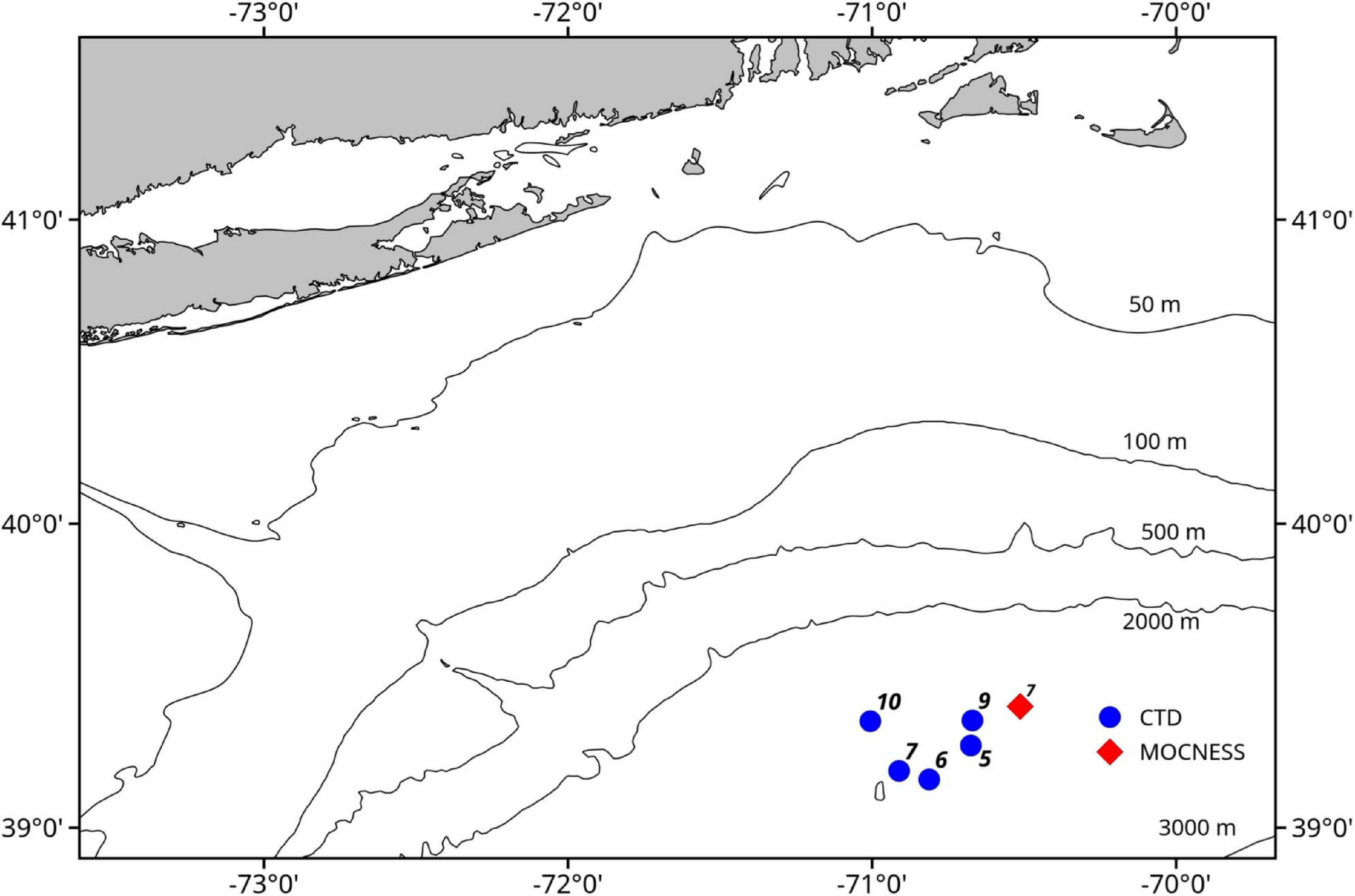
Figure 1. Map of study area. Blue circles indicate locations of the CTD casts that sampled eDNA and the red diamond indicates the location of the MOCNESS tow.
Three daytime and two nighttime CTD casts were completed (avoiding crepuscular periods when animals may be vertically migrating), yielding a total of 40 eDNA samples (Table 1) and consisting of 8 samples per cast. Niskin bottles were not cleaned between casts as the bottles were deployed open and thus exposed to the entire water column during the downcast, until they were triggered to close at the target depth on the upcast. In the first four casts, one sample was taken at each of 8 depths. On the final cast (Cast 10), two replicate samples were taken at each of 4 depths. Diel vertical migration was observed as the vertical shift in the position of the layers on the echosounder, and our water sampling for each cast coincided with these layers (Figure 2 and Supplementary Figures S1–S4). In all of the CTD casts, the water column was stratified with clines in density, temperature, and salinity between approximately 25 and 50 m below the surface (Figure 3 and Supplementary Figures S5, S6). Dissolved oxygen peaked in this region, before declining at the depth around 50–70 m. Oxygen minimums were found around approximately 250 m below surface in all casts (Supplementary Figures S5, S6).
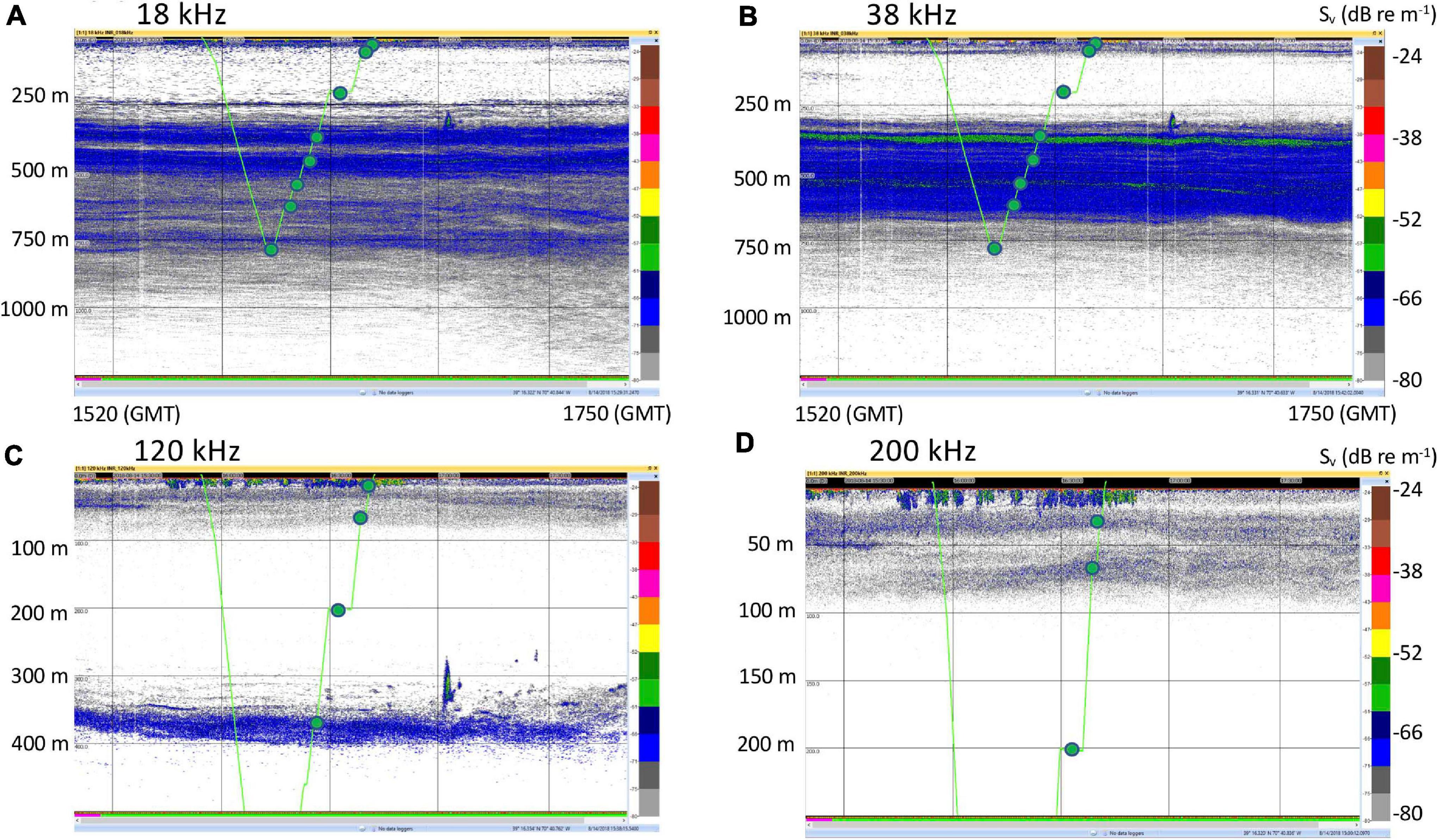
Figure 2. Representative echosounder images with noise reduction applied, and the CTD track and sampling locations overlain in green. (A) 18 kHz. (B) 38 kHz. (C) 120 kHz. (D) 200 kHz. These images are for Cast 5 on 14 August 2018. Images for the rest of the casts are in Supplementary Figures S1–S4. Time stamps (GMT) indicate the start and end of the echograms and vertical bars are at 30 min intervals. Note change of vertical scale for the 120 kHz (500 m) and 200 kHz (250 m) echograms.
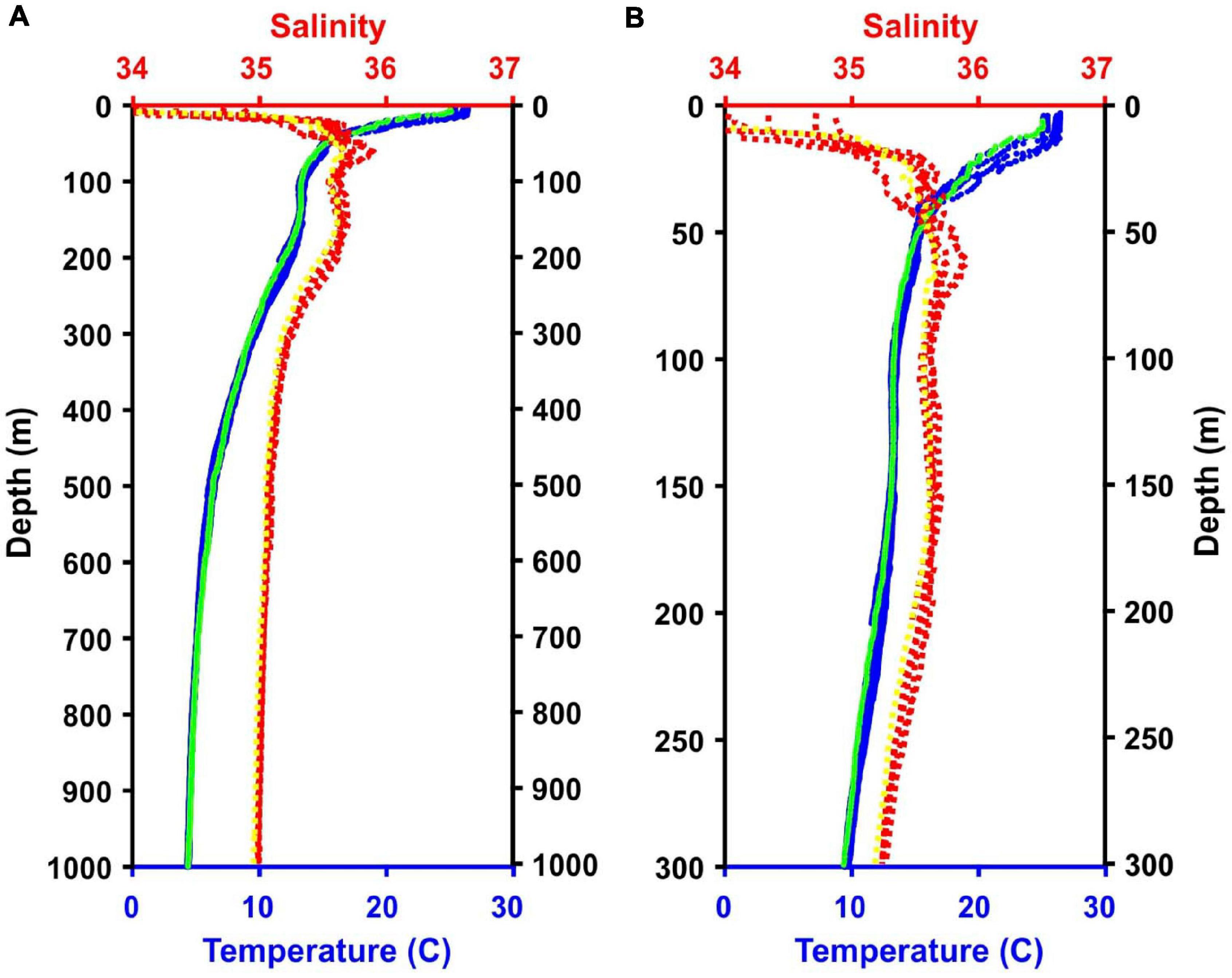
Figure 3. Temperature and salinity data from the five CTD casts and the MOCNESS tow. Note, lines for all casts and the tow are present although they are not always visible because they overlap. (A) Data from 0 to 1,000 m. (B) Data from the top 300 m of the water column. Blue = CTD temperature data; Green = MOCNESS temperature data; Red = CTD salinity data; Yellow = MOCNESS salinity data.
Water from the Niskin bottles was filtered in a temperature-controlled cold room (maintained at 13–15°C) on the ship and began immediately upon retrieval of the CTD-rosette. The work area in the cold room was wiped down daily with bleach, followed by several rinsing wipes with Milli-Q water. Nitrile gloves were worn throughout the filtering protocol and were changed frequently. Three samples were processed at a time. Bottles were removed from the rosette, brought into the processing room, and secured on custom-built stands. The remaining bottles were wrapped in ice packs until they could be processed.
Three peristaltic pumps were used to pump water from the Niskin bottles through sterile encapsulated, single-use 0.2 μm PES Sterivex filters. The tubing was first cleaned by pumping with a minimum of 200 ml of Milli-Q water and then flushed with a minimum of 200 ml of sample water. The Sterivex filters were attached after cleaning. The remaining Niskin bottle water was filtered through the Sterivex and the volume of the flow-through was collected and measured (Table 1). Once filtration was complete, the Sterivex filter was removed, the ends of the filter were sealed with parafilm, the filter was placed in a sterile 50 ml Falcon tube, and the tube was stored at −80°C. A control sample was filtered along with each cast. The control samples consisted of approximately 4.8 l of Milli-Q water dispensed into pre-sterilized, single-use Whirlpak sample bags and pumped through Sterivex filters.
Zooplankton Sample Collection and Shipboard Processing
A 1 m2 MOCNESS (Multiple opening and closing net and environmental sensing system; Wiebe et al., 1985) zooplankton tow was taken for comparison with the eDNA data from the CTD casts. The MOCNESS was equipped with nine nets (333 μm mesh), SeaBird temperature and conductivity probes, a pressure sensor, and a flowmeter. Eight of the MOCNESS nets sampled at discrete depth intervals ranging from the base of the mesopelagic zone (1,000 m) to the surface, and an additional net sampled the integrated water column (0–1,000 m). The MOCNESS tow was taken in the vicinity of the CTD casts (Figure 1). Temperature and salinity data were consistent with those from the CTD casts, indicating that MOCNESS and CTD sampled the same water mass (Figure 3). The nine nets sampled the following depth intervals: 0–1,000, 1,000–800, 800–600, 600–400, 400–200, 200–100, 100–50, 50–24, and 24–0 m, and tow sample volumes ranged from approximately 149 to 5,208 m3 (Table 2). Upon retrieval, each net was washed and the cod end buckets were removed. The zooplankton from each net were split into four equal parts using a Folsom plankton splitter (McEwen et al., 1954). One of these splits was preserved in 95% ethanol for metabarcoding analysis. The ethanol preservative was replaced with fresh 95% ethanol approximately 24 h after collection.
Environmental DNA Extraction and Sequencing
Upon return to the laboratory, genomic DNA and controls were extracted using DNeasy Power Water Sterivex Kits (Qiagen). DNA extractions were performed in a dedicated, pre-amplification DNA work area. The work area was cleaned before use with 10% bleach followed by several rinses with Milli-Q water. Next Generation library preparation and sequencing were conducted at the Center for Genome Innovation at the University of Connecticut (Storrs, CT). Each extract was normalized to 5 ng/μl and amplified in duplicate using 2× KAPA HiFi HotStart ReadyMix (Kapa Biosciences, Wilmington, MA) following the 16S Metagenomic Sequencing Library Preparation guide (Illumina, San Diego, CA). The PCR was set up in a PCR hood and the surface area was first cleaned with 70% bleach and additionally decontaminated using UV light, and only sterile pipette tips with embedded aerosol filters were used for pipetting. 2.5 μl of sample was added to each PCR. Per sample and filtration control two PCR replicates were run and pooled after the first PCR. The primers used were the 1380F and 1510R V9 primers (Amaral-Zettler et al., 2009) with Illumina adapters. The primer sequences with their adapters (in bold) were: 1380F 5′-TCGTCGGCAGCGTCAGATGTGTATAAGAGACAGCCCTGC CHTTTGTACACAC-3′ and 1510R 5′-GTCTCGTGGGCTCGG AGATGTGTATAAGAGACAGCCTTCYGCAGGTTCACCTAC-3′. The PCR conditions for the first PCR were: 95°C for 3 min; 25 cycles of: 95°C for 30 s, 55°C for 30 s, 72°C for 30 s; 72°C for 5 min. Amplification success was assessed on the pooled PCRs using an Agilent 4200 TapeStation electrophoresis system using the High Sensitivity DNA D1000 assay (Agilent Technologies, Santa Clara, CA) and the products were purified using Agencourt AMPure XP Beads (Beckman Coulter, Brea, CA).
Index barcode sequences were incorporated into the purified amplicons by a second PCR using NexteraXT Index Kit v2 Sets C and D (Illumina, San Diego, CA) and 2× KAPA HiFi HotStart ReadyMix (Kapa Biosciences, Wilmington, MA). The second PCR protocol was: 95°C for 3 min; 8 cycles of: 95°C for 30 s, 55°C for 30 s, 72°C for 30 s; and 1 cycle of 72°C for 5 min. The indexed PCR products were purified using AMPure XP Beads (Agencourt) and assessed for quality and adapter removal using an Agilent 4200 TapeStation electrophoresis system (High Sensitivity DNA D1000 assay). Libraries were quantified using a Qubit 3.0 fluorometer (Life Technologies, Carlsbad, CA), normalized, pooled, and denatured according to Illumina MiSeq sample preparation for sequencing. 20–30% PhiX (Illumina, San Diego, CA) was added to our amplicons before running on the Illumina MiSeq using the 500 cycle v2 reagent kit. Our targeted sequencing depth was 200,000 reads per sample. The negative control samples were also sequenced. For pooling the negative controls (which did not contain detectable amounts of DNA), we added the maximum volume that was used for the samples.
Zooplankton DNA Extraction and Sequencing
Zooplankton samples were sorted into small (333–1,000 μm) and large (>1,000 μm) size fractions before extraction in order to maximize the detection of low-biomass organisms and the wet weight of each was recorded (Supplementary Table S1). The samples were poured through stacked 150 and 1,000 μm sieves and the samples were rinsed with Milli-Q water, which also washed away any remaining ethanol. When present, exceptionally large animals or fragments were picked out of the 1,000 μm sieve and preserved for later individual (Sanger) sequencing. Sieve contents were rinsed into pre-weighed, sterile 50 ml Falcon tubes using as little water as possible and concentrated by spinning 1 min at 1,000 rpm. Residual water overlying the zooplankton was pipetted away.
Zooplankton samples were homogenized using a Benchmark D1000 homogenizer with a 10 mm sawtooth probe until the Falcon tube contents were visibly smooth and well-mixed. The homogenized tubes were centrifuged for 2 min at 5,000g and excess water overlying the pellets was decanted off. All tubes were weighed. The homogenizer was cleaned between samples by running it for 20 s in a 10% bleach solution followed by 3 additional runs in separate tubes of Milli Q water.
Two hundred mg of zooplankton biomass was transferred from each tube to a sterile 15 ml Falcon tube and DNA was extracted using DNeasy Blood and Tissue DNA extraction kits, using a slight modification of the manufacturer’s protocol to accommodate the relatively large tissue biomass in the samples. Specifically, 1,800 μl of Buffer ATL and 200 μl of proteinase K were added to each tube, and tubes were incubated for 3 h at 56°C and vortexed periodically. After incubation, 200 μl of lysate was transferred to a new, sterile 1.5 ml tube. At that point, the Qiagen manufacturer’s protocol was followed exactly, with a final elution into 200 μl Buffer AE. Aliquots of the extracted DNA were sent to the Center for Genomic Innovation at the University of Connecticut for library preparation and sequencing, following the same protocol as for the eDNA samples.
Genomic DNA of the large individuals that were set aside was extracted using the DNeasy Blood and Tissue Kit (Qiagen) following the manufacturer’s protocol. The 18S V9 gene was amplified using the V9 primers (Amaral-Zettler et al., 2009) under the following conditions: 95°C for 3 min; 35 cycles of: 95°C for 30 s, 55°C for 30 s, 72°C for 30 s; and 1 cycle of 72°C for 5 min. PCR products were amplified on a 1.2% agarose gel and visualized with GelRed. No additional attempts were made to amplify samples that failed the first time, in order to be consistent with the metabarcoding protocol. Amplicons were purified with QiaQuick PCR Purification kits (Qiagen) according to the manufacturer’s protocol, quantified using a Nanodrop, and sequenced in both directions at Eurofins Genomics1.
Bioinformatics
The same bioinformatics workflow was applied to both the eDNA and the MOCNESS zooplankton samples, although each group was processed separately as they were sequenced on separate runs and thus differed in their sequencing error profiles. Demultiplexed paired-end sequence reads were processed using Quantitative Insights Into Microbial Ecology 2 (QIIME2) version 2019.1 (Sigsgaard et al., 2015; Thomsen and Willerslev, 2015; Bolyen et al., 2018). Forward primer sequences were trimmed, and forward and reverse reads were truncated based on quality plots of the data after base pair 120. Reverse primers were removed (if present) using the Cutadapt (Martin, 2011) Qiime2 plugin (v. 2020.11.1). DADA2 (Callahan et al., 2016) implemented through QIIME2 performed sequence quality control including error correction and chimera removal, and paired the reads to generate unique (i.e., 100% similarity) amplicon sequence variants (ASVs). The control samples contained very few reads (e.g., 0.3% of the metazoan-only dataset). For each ASV in the dataset that was present in both the samples and controls, the maximum number of reads found in any control was subtracted from every sample. ASVs that had a frequency of less than 10 (summed across all 40 samples) were deleted from the dataset.
Taxonomy was assigned using a naïve Bayesian classifier (Bokulich et al., 2018) that was trained on the Silva v. 132 99% database (Quast et al., 2013) using 18S sequences only for the 18S V9 gene region. The resulting dataset was filtered to generate a metazoan-only dataset that provided a high-level taxonomic classification for a broad range of animal species (Level 5 on the Silva database classification). We further resolved the most abundant categories based on Level 6 of the Silva database. Taxa comparisons between eDNA and MOCNESS were based on Level 7 (the deepest level) of the Silva database.
We also searched all ASVs against the GenBank nr database. Blast search parameters were set to exclude uncultured, unfiltered, and unclassified entries. We reported top hits when the percent identity between our ASV and the Genbank entry was 97% or greater. This threshold has been used in other studies (Pearman et al., 2014; Casas et al., 2017) and is effective in distinguishing genera and families in copepods (Wu et al., 2015). Note that even a perfect (100%) match does not necessarily indicate a species-level identification (Wu et al., 2015; Blanco-Bercial, 2020) and that, while better populated than the Silva database, representative V9 sequences for many mesopelagic animal species are not available on Genbank.
Rarefaction curves were generated on the eDNA and MOCNESS all-data and metazoan-only datasets to evaluate whether the obtained sequencing depths were sufficient to recover all taxa. Using datasets where sequencing depth in all samples was truncated to the lowest observed sequencing depth, we generated Jaccard (presence-absence) and Bray-Curtis indices in QIIME2, and used these to create non-metric multidimensional scaling (nMDS) plots with vegan 2.3_5 in the statistical package R (Oksanen et al., 2016). Data points were visualized relative to time of sampling (day or night) and sampling depth categories (0–100, 100–200, 200–500, 500–800 m). Functional regressions of the data points against each nMDS dimension were performed in Matlab to assess the significance of observed patterns (Ricker, 1973).
Results
Environmental DNA Sequence Data Summary
A total of 13,561,867 sequences were obtained from 40 samples and 5 negative controls, with an average of 301,375 ± 22,404 (SE) reads per sample. The number of reads in the negative controls ranged from 550 to 6,899. A sixth negative control (from the PCRs) had no reads. In the DADA2 analysis, a total of 12,181,342 sequences (approximately 90%) remained after the filtering, denoising, merging, and chimera removal steps, with an average of 270,696 ± 20,331 (SE) sequences per sample (Supplementary Table S2). These sequences were classified into 10,543 unique amplicon sequence variants (ASVs). After removing rare ASVs (total frequency across samples < 10), 8,417 ASVs comprising 12,170,626 sequences remained. Of these, 351 ASVs comprising 1,421,650 sequences were metazoans. There were 65 ASVs that were present in both the negative controls and the samples (Supplementary Table S3). For each of these, we took the maximum number of reads found in any of the controls and subtracted that number from the corresponding ASV read count from every sample. Four additional ASVs that were discovered to be either misclassified by Silva as metazoans (based on our Blast results, below) or were unlikely mesopelagic inhabitants were also removed from our analyses.
The percentage of ASVs that were classified as metazoan taxa varied considerably between samples from different depths. The proportion of metazoan sequences in the results generally declined with depth beginning at 30 m (approximately coinciding with the pycnocline; Supplementary Figure S6), and in all cases except one, metazoans comprised 21% or less of the total number of obtained reads in samples collected below 200 m (Figure 4). Non-metazoan taxa (Supplementary Table S4) were not analyzed further.
A broad range of metazoan taxa were recovered in both daytime and nighttime casts (Figure 5). In Cast 10, where duplicate samples were taken at each depth, we observed considerable differences in the presence and abundance of taxa in the reads we obtained (Figure 5C). Overall, crustaceans (Copepoda, Eumalacostraca, Myodocopa) and medusozoans (Scyphozoa, Hydroidolina, Trachylina) were particularly well-represented. Crustacean ASVs comprised 53.7 ± 28.9% of the reads in mesopelagic samples (200–800 m) and 73.0 ± 24.2% of the reads in epipelagic samples (0–200 m). Conversely, medusozoan ASVs were generally a more prominent component of the reads (35.2 ± 24.8%) in mesopelagic depths than in epipelagic depths (16.6 ± 25.4%). The Silva database Level 6 analysis revealed that the major components of crustacean reads were classified as calanoid and cyclopoid copepods (Figure 6), and the major components of the obtained medusozoan reads were siphonopohores (especially at mesopelagic depths) and anthoathecate medusae (Figure 7). We also found that 150 of the 275 eDNA ASVs matched GenBank entries with 97% identity or greater (Supplementary Table S5). Consistent with the Silva classification, taxa with Genbank matches included many calanoid and cyclopoid copepods, siphonophores, and anthoathecate medusae, as well as other taxa such as trachymedusae, scyphomedusae, ctenophores, and other less commonly recovered taxa.
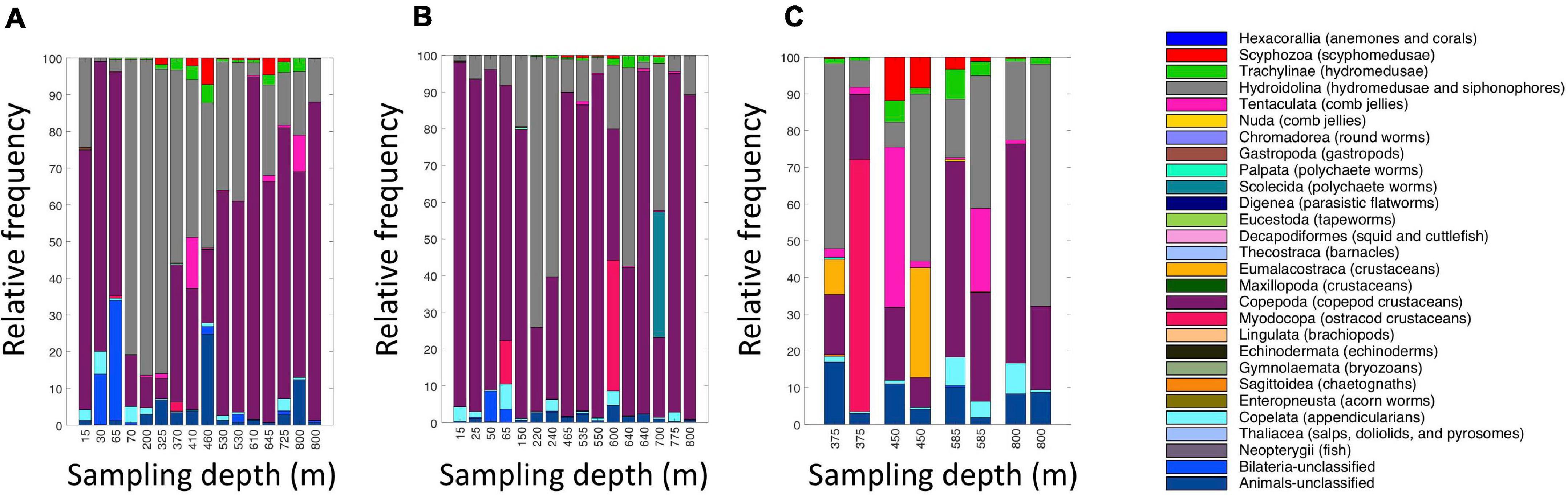
Figure 5. Relative read abundances of metazoan taxa in the eDNA samples from (A) daytime casts (casts 5 and 7); (B) nighttime casts (casts 6 and 9); and (C) daytime cast with duplicates (cast 10). Taxon categories are based on the Silva database, Level 5. Different depths were sampled in each cast.
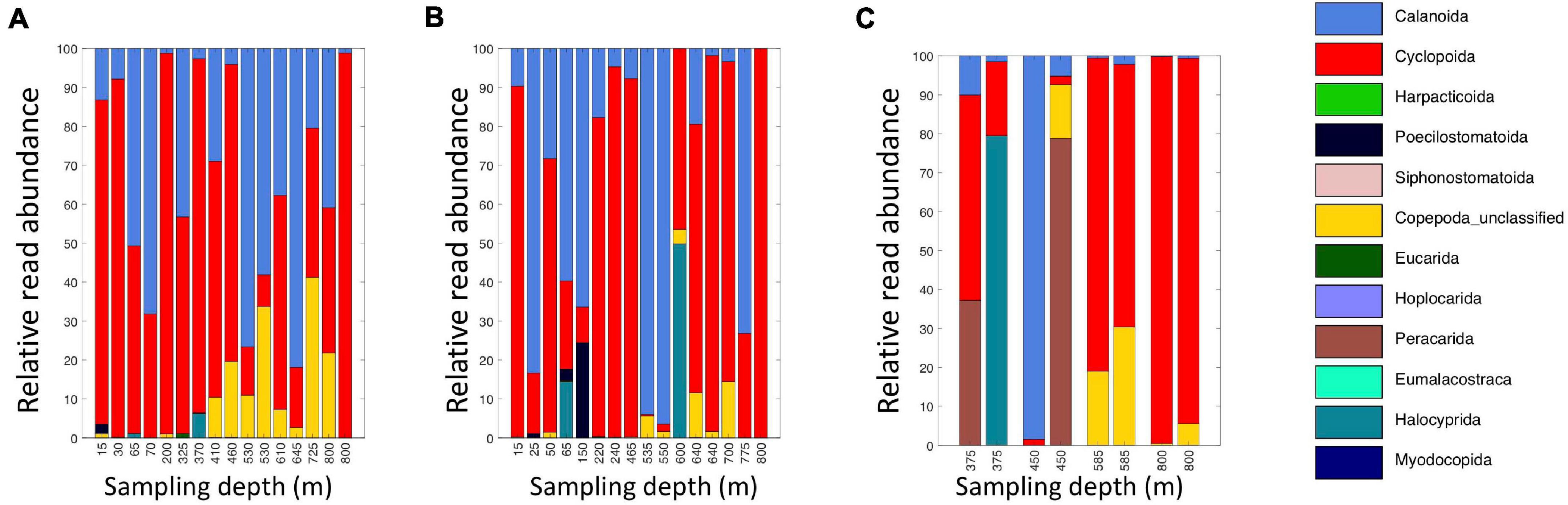
Figure 6. Relative read abundances of classifications of eDNA Level 6 taxa identified as crustaceans (Copepoda, Eumalacostraca, Myodocopa, Thecostraca). (A) Daytime casts 5 and 8. (B) Nighttime casts 6 and 9. (C) Daytime cast 10 with duplicate sampling.
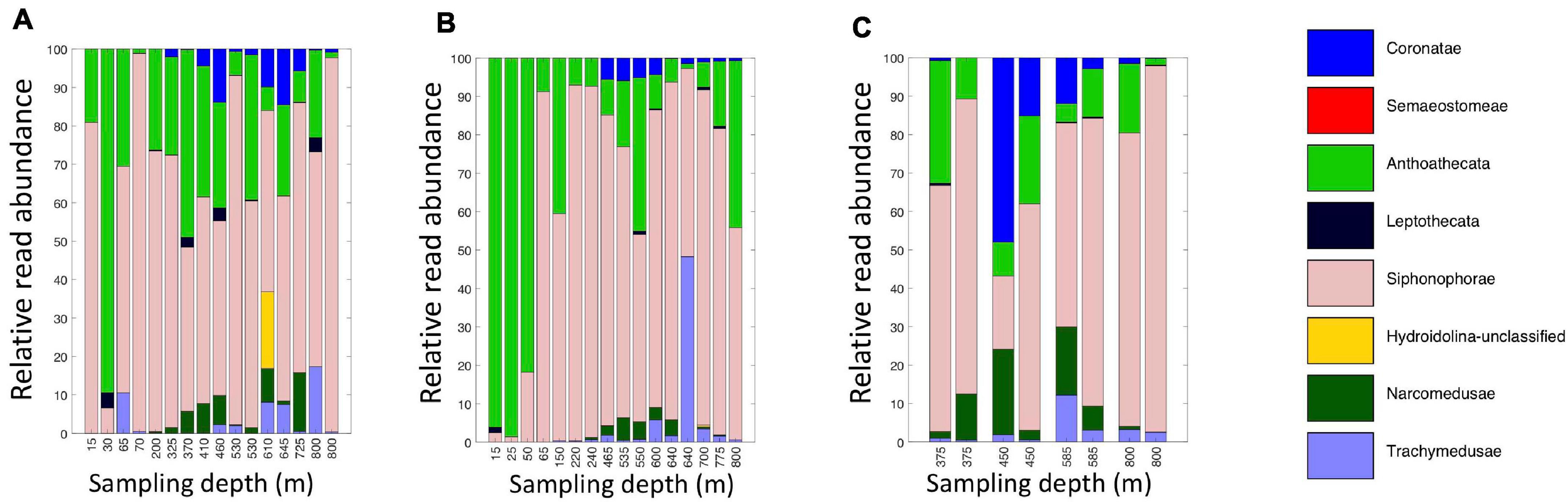
Figure 7. Relative read abundances of eDNA Level 6 taxa identified as Medusozoan (Scyphozoa, Hydroidolina, Anthoatheca, Leptotheca, and Trachylinae). (A) Daytime casts 5 and 8. (B) Nighttime casts 6 and 9. (C) Daytime cast 10 with duplicate sampling.
Rarefaction plots of all ASVs and the filtered metazoan-only dataset showed an initial increase in the number of ASVs with sequence subsampling depth and then leveled off, indicating that the sequencing depth was sufficient to capture ASV diversity in our samples (Supplementary Figure S7). Using the metazoan datasets truncated at the lowest sequence depth, nMDS plots revealed clustering was related to depth but not time of sampling (Figure 8). The structuring was more evident with the Jaccard indices (stress = 0.128) than with the Bray-Curtis indices (stress = 0.221). However when the data were log-transformed, the stress index for the Bray-Curtis analysis improved (stress = 0.154). Note also, unlike Jaccard similarity which is based on presence/absence of taxa, Bray-Curtis indices take into account read abundances, which in metabarcode data, are influenced by PCR biases (Kelly et al., 2018) and eDNA dynamics (Allan et al., 2020). A regression of the Jaccard data points against the nMDS axes revealed a significant relationship with dimension 1 (R2 = 0.728, p < 0.001) but less so with dimension 2 (R2 = 0.108, p = 0.0.38). Samples corresponding to the shallowest depth bin (0–100 m) appeared to be driving this relationship (Figure 8), so to assess the relationship with depth for the deeper samples, we repeated the regression analysis without the 0–100 m samples. We found significant correlations with both dimension 1 (R2 = 0.34, p < 0.001) and dimension 2 (R2 = 0.755, p = 0.001). There was no significant relationship with sampling time (day vs. night) for either dimension (dimension 1: R2 = 0.025, p = 0.333; dimension 2: R2 = 0.003, p = 0.744). The results were similar for the Bray-Curtis analysis based on the log-transformed data.
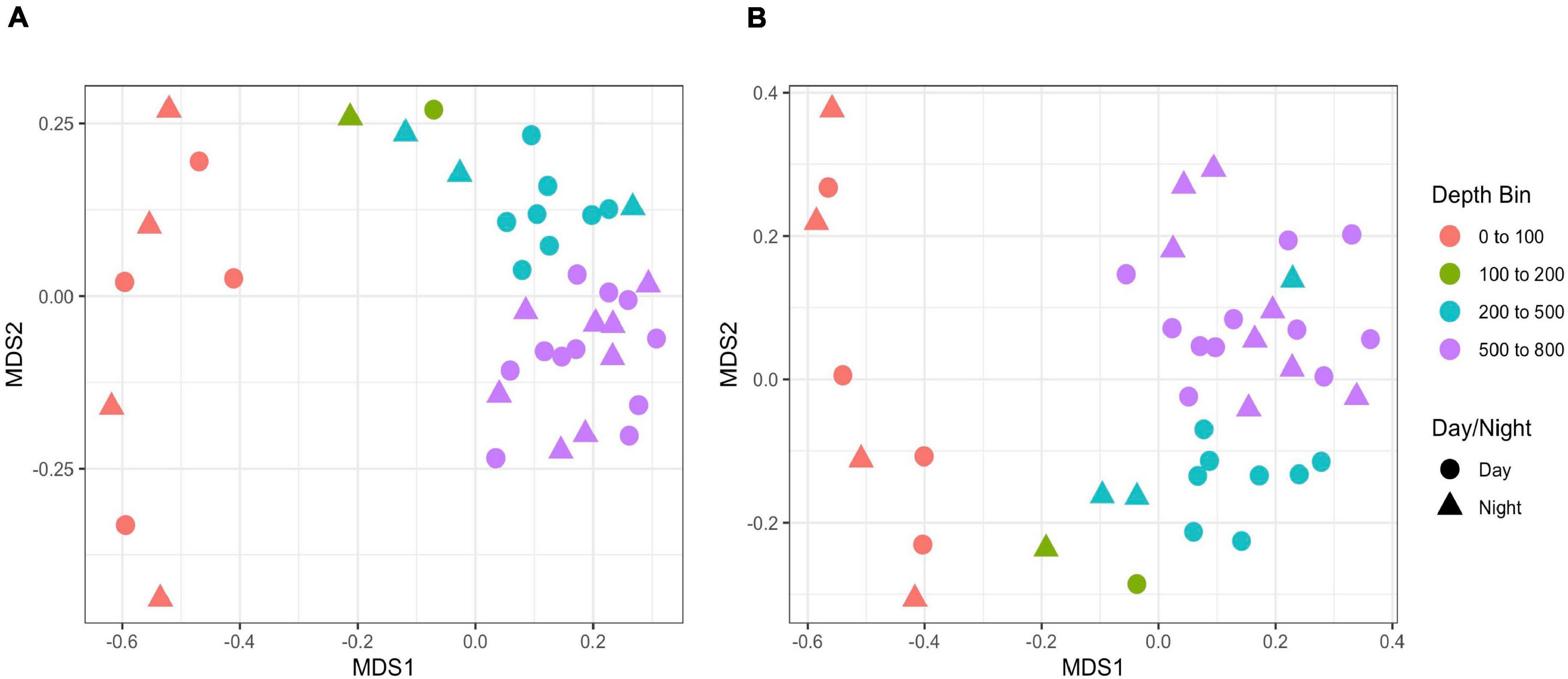
Figure 8. NMDS plots of eDNA data based on (A) Jaccard and (B) Bray-Curtis indices. Bray-Curtis indices are based on log-transformed data. Shapes indicate day vs. night sampling and color indicates depth bins.
MOCNESS Zooplankton Sequence Data Summary and Comparison With eDNA
Altogether, the 9 MOCNESS nets sampled a combined volume of 12,193.7 m3 (=12,193,700 l). From this, a total of 4,129,604 sequences were obtained from the large and small size fraction samples combined, with an average of 229,422 ± 8,025 (SE) reads per sample. A total of 3,629,395 sequences (approximately 87%) remained after the filtering, denoising, merging, and chimera removal steps, with an average of 201,633 ± 7,352 (SE) sequences per sample (Supplementary Table S6). These sequences were classified into 866 unique amplicon sequence variants (ASVs). After sequences with a frequency less than 10 were removed, 622 ASVs (3,628,313 sequences) were retained, 470 of which were metazoans that, like the eDNA results, comprised a diverse array of animal taxa (Figure 9 and Supplementary Table S7). However, the number of ASV’s obtained from the zooplankton dataset (455) was approximately two-thirds greater than that obtained from the eDNA dataset (275). Overall, medusozoan taxa were less abundant in the MOCNESS tow than in the eDNA samples (3.11 ± 3.35% in the large and 7.86 ± 10.34% in the small size fractions), but crustaceans were generally highly abundant (91.10 ± 4.06% in the large and 88.32 ± 11.93% in the small size fractions). Also in contrast to the eDNA results, the major components of the crustacea were calanoid copepods (but few cyclopoid copepods) and eumalacostracans (Figure 10). The medusozoan groups were comprised of primarily siphonophores, but other groups including narcomedusae, trachymedusae, and others were also detected (Figure 11). The Blast results included many 97% or greater matches with numerous calanoid copepods, and eumalacostracans including euphausiids, amphipods, and decapods. Other matches included a greater variety of non-crustacean taxa as compared to the eDNA results, and these included siphonophores and other medusazoan taxa as well as chaetognaths, polychaetes, fishes, salps, and others (Supplementary Table S7). As with the eDNA dataset, rarefaction curves showed that sequencing depth was sufficient to capture the ASV diversity in both the full data set and the metazoan-only dataset (Supplementary Figure S8).
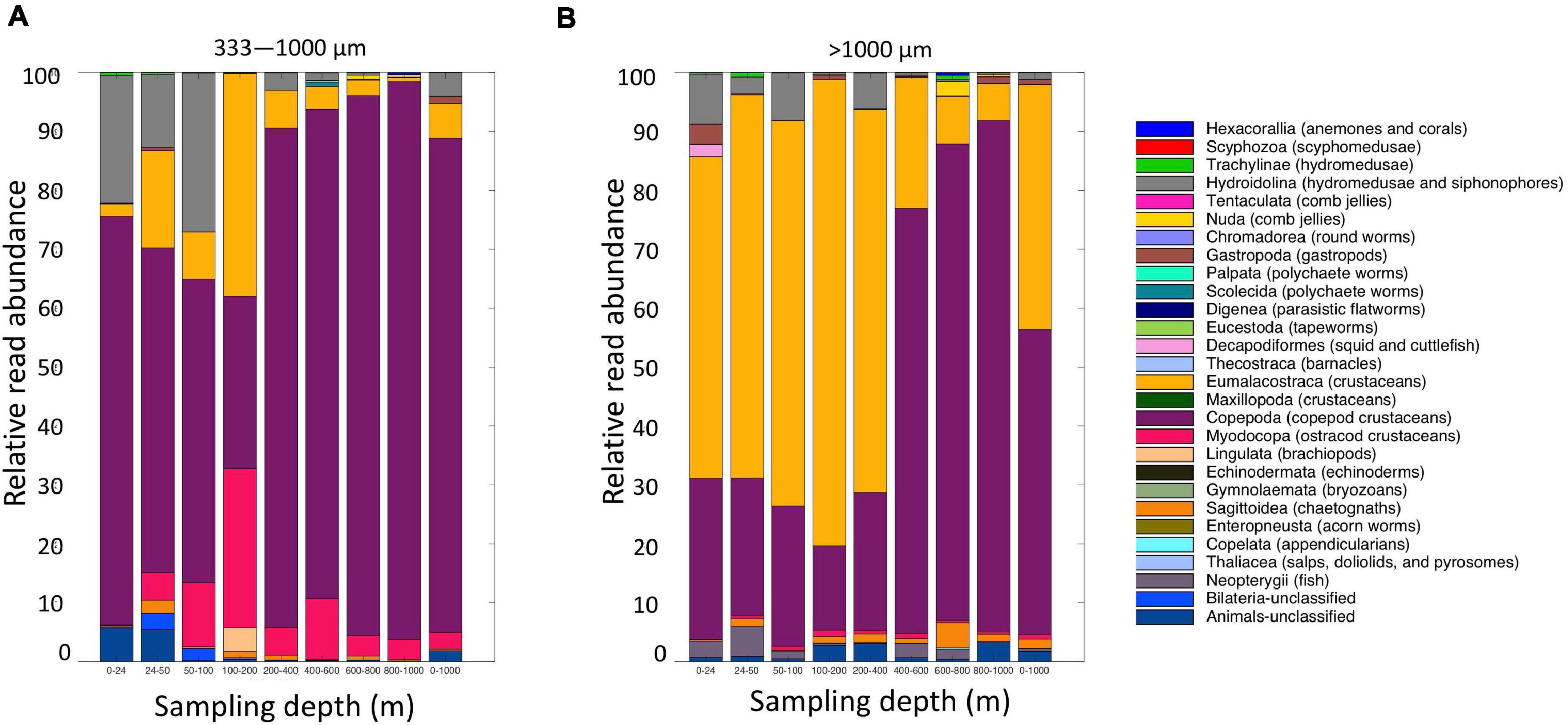
Figure 9. Relative read abundances of metazoan taxa from the (A) small (333–1,000 μm); and (B) large (>1,000 μm) fractions of the MOCNESS tow. Taxon categories are based on the Silva database Level 5.
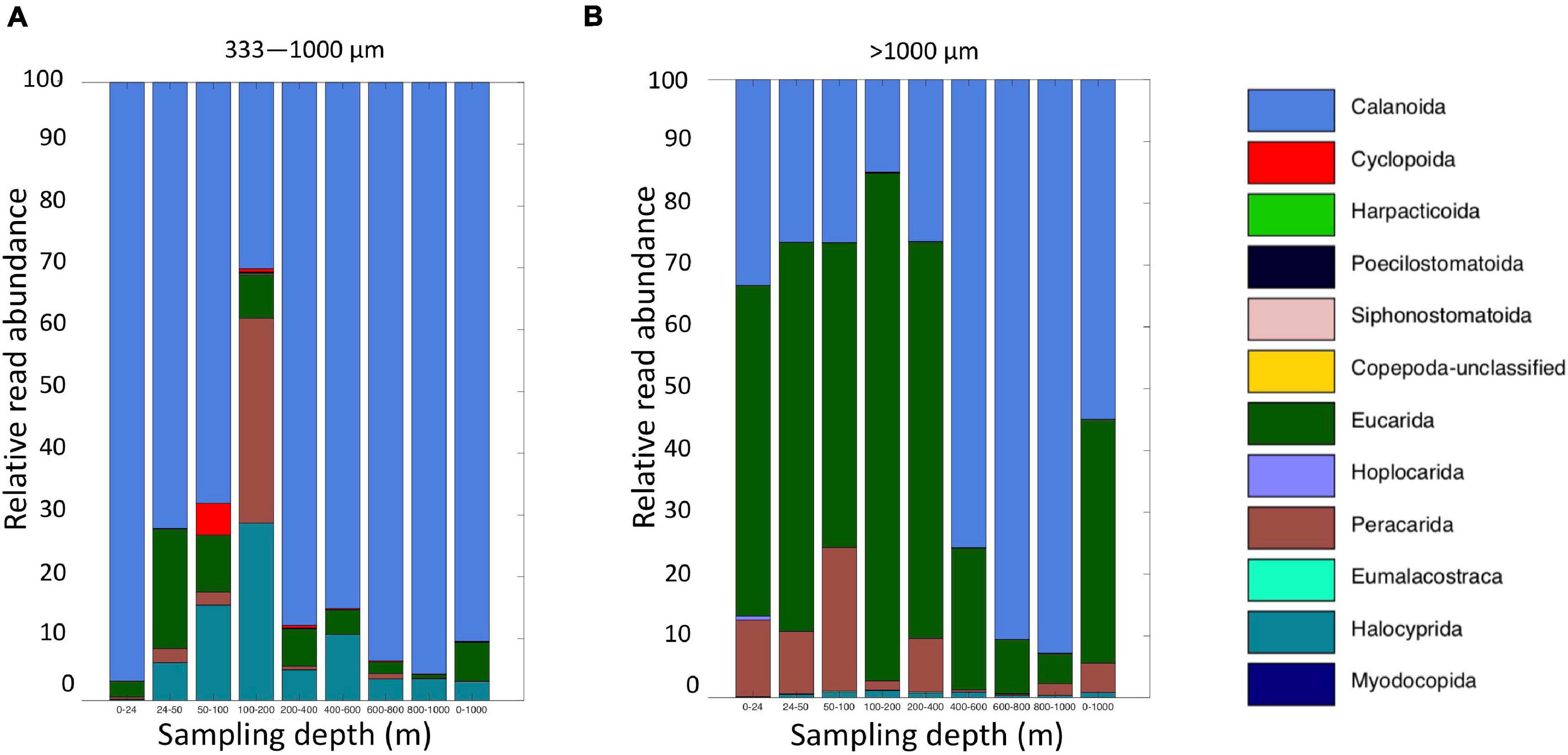
Figure 10. Relative read abundances of classifications of Level 6 taxa identified as crustaceans (Copepoda, Eumalacostraca, Myodocopa, Thecostraca) (A) MOCNESS small size fraction and (B) MOCNESS large size fraction.
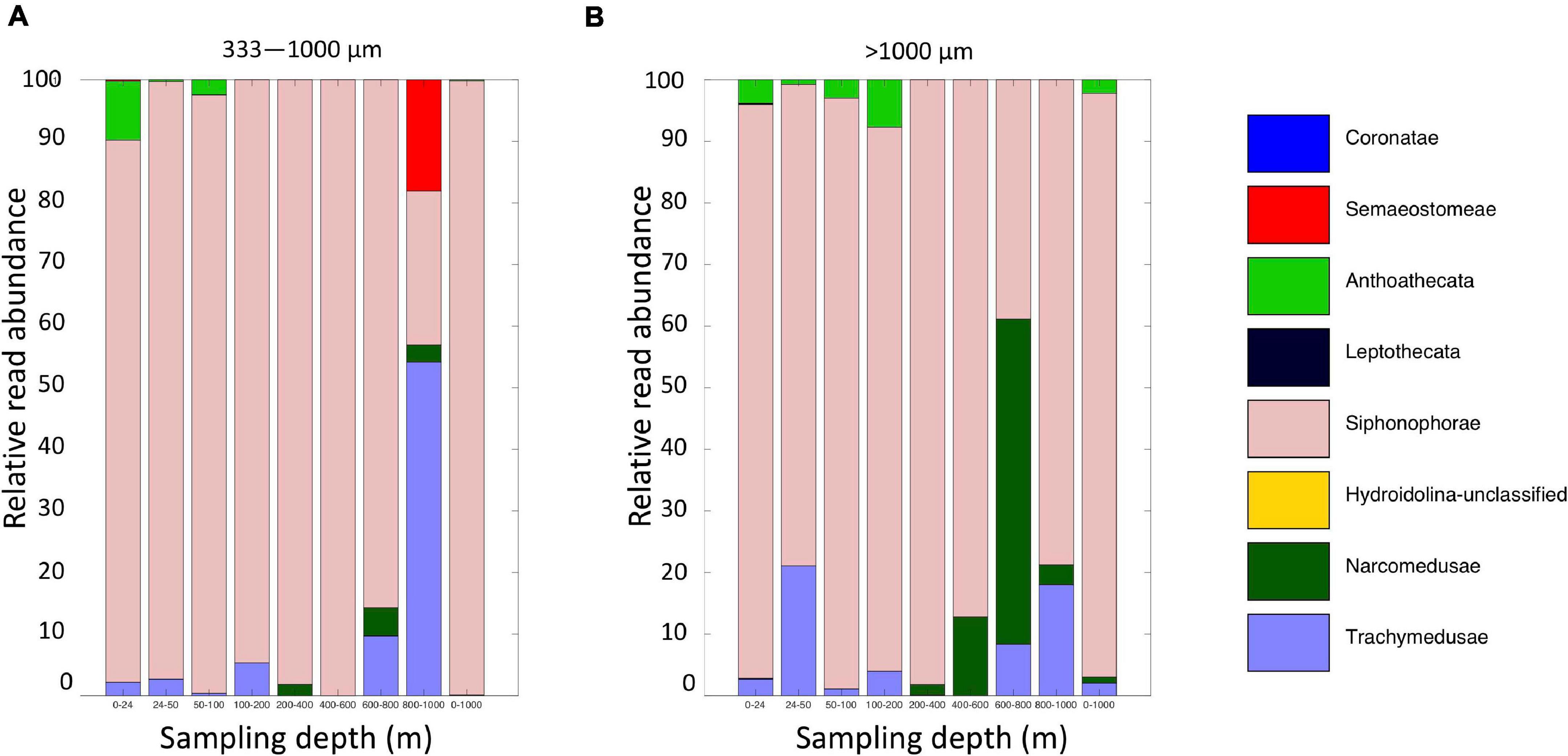
Figure 11. Relative read abundances of Level 6 taxa identified as Medusozoan (Scyphozoa, Hydroidolina, Anthoatheca, Leptotheca, and Trachylinae). (A) MOCNESS small size fraction. (B) MOCNESS large size fraction.
Additionally, a total of 10 large individuals and organism fragments were removed from the MOCNESS samples before homogenizing. Six of these produced PCR bands, and 5 were sequenced successfully (Supplementary Table S8). Three of these were fish, one was a scyphozoan, and one was likely a eumalacostracan. All were identical to MOCNESS ASVs. The specimens that failed to be sequenced included 3 fish, one shrimp, and one unidentified gelatinous animal.
The MOCNESS sampling recovered a greater number of both metazoan ASVs and metazoan taxa than did sampling with the Niskin bottles (eDNA samples). Overall, we found 455 metazoan ASVs in the MOCNESS samples (metabarcoding ASVs from small and large fractions combined plus the one unique large-individual sequence), and 275 in the eDNA samples (all casts and samples combined). We compared the number of high-level animal taxa (as classified to Level 7 in the Silva database) and found that the MOCNESS sampling (all nets and fractions combined, including large individuals) detected 23 more taxa than the eDNA sampling. Thirty-nine taxa were detected in both approaches, 17 were found in eDNA only, and 40 were found in the MOCNESS only (Supplementary Table S9). Despite the greater number of metazoan taxa sampled by the MOCNESS, sampling efficiency, defined here as number of metazoan ASVs detected per liter of water sampled, was far greater for eDNA (275 ASVs/206 l = 1.335 ASVs/l; volume filtered from Table 1) than for the MOCNESS (455 ASVs/12,193,700 l = 0.00003731 ASVs/l).
Discussion
Biodiversity Detection
Our results suggest that eDNA analysis is an informative and highly efficient approach for invertebrate biodiversity detection in the mesopelagic that complements net sampling. Our sequence results were based on the 18S V9 marker, which detects a comprehensive and phylogenetically diverse range of animals (Wu et al., 2015; Bucklin et al., 2019; Blanco-Bercial, 2020). The 18S V9 marker is well-suited for broadly focused eDNA surveys due to its relatively short length, as eDNA is likely degraded and thus comprised of relatively small fragments (Jo et al., 2017).
The animal component of our eDNA results declined with depth, and this result is consistent with Stefanoudis et al. (2019), who, in a survey extending to 800 m depth based on specimen identifications from net tows, showed that zooplankton abundance decreased substantially below 200 m. In our study, the observed decline was approximately coincident with the pycnocline (which was located in the 0–200 m depth interval in Stefanoudis et al. (2019)). While the pycnocline can be a barrier for animal dispersal (Suzuki et al., 2018), Closek et al. (2019) found no significant difference in fish taxa identified from eDNA above and below the pycnocline off of the central California coast. It will be interesting for future studies to examine whether or not the pycnocline presents a barrier to eDNA dispersal and how that impacts interpretation of eDNA signals.
The eDNA sequences originated primarily from calanoid and cyclopoid copepods, siphonophores, and other medusozoans. In contrast, the crustacean component of the MOCNESS sequences comprised primarily calanoid copepods (and relatively few cyclopoids) and eumalacostracans (e.g., euphausiids and amphipods). The medusozoan component of the MOCNESS sequences also included siphonophores (but with fewer ASVs), and the relative proportions of other medusuzoans were different than in the eDNA dataset.
Can Environmental DNA Can Add Substantially to Plankton Metabarcoding Analyses?
While the number and types of metazoan taxa detected in the genetic material collected by MOCNESS sampling were greater than those detected by the eDNA sampling, we detected 17 taxa via eDNA that were not detected by the MOCNESS. The volume of water sampled by Niskin bottles for eDNA analysis, however, was a tiny fraction of that sampled by the MOCNESS. Thus, in terms of the number of metazoan ASVs and taxa detected per volume of water sampled, eDNA from Niskin bottles outperformed MOCNESS sampling, although each approach detected taxa that the other did not. Given the close proximity of the CTD and MOCNESS sampling locations and times, and the temperature and salinity data that showed that the sampling locations belonged to the same water mass, we assume that the pool of biodiversity was similar for each sampling event and that the observed differences are due to sampling biases associated with each method. Our findings that many of the same taxa were recovered in both sampling approaches and that other taxa that were recovered in only one or the other approach, are also consistent with other studies that compare eDNA with other survey methods (Thomsen et al., 2016; Kelly et al., 2017; Sigsgaard et al., 2017; Closek et al., 2019; Liu et al., 2019).
The relative frequencies of medusozoan ASVs in our eDNA reads compared to our MOCNESS reads could indicate a relatively high eDNA shedding rate or a relatively high biomass—or both. While there are few studies on eDNA shedding rates, the available data show that they are variable between taxa, between individuals within a species, and between different stages of an organism’s life cycle (Sansom and Sassoubre, 2017). Most studies on eDNA shedding focus on coastal and freshwater fish (Takahara et al., 2012; Klymus et al., 2015; Sassoubre et al., 2016; Nevers et al., 2018). However, Minamoto et al. (2017) found that medusae shed eDNA at a significantly greater rate than fish (by 1–3 orders of magnitude). Allan et al. (2020) also found that medusae may shed eDNA at higher rates than other animal types. Animals with hard external surfaces (e.g., bivalves and crustaceans) may have lower shedding rates (Sansom and Sassoubre, 2017; Allan et al., 2020). Interestingly our eDNA data lacked eumalacostracan ASVs, which were common in the MOCNESS data. Eumalacostracans include euphausiids, which are a well-known component of mesopelagic biomass and so their paucity in the eDNA is notable. Additional research on shedding rates in mesopelagic organisms is critical for interpreting eDNA signals.
The biomass of medusozoans and other gelatinous animals in the mesopelagic is likely substantially underestimated due to difficulties in sampling them (Madin and Harbison, 1978; Larson et al., 1991; Robison, 2004, 2009). These animals are destroyed by traditional plankton net sampling, and many new forms were discovered only when human-occupied underwater vehicles (HOVs) and remotely operated vehicles (ROVs) came into use (Madin and Harbison, 1978; Larson et al., 1991; Robison, 2004, 2009). Our eDNA and MOCNESS results showing a greater presence of gelatinous animals in eDNA are consistent with the hypothesis that gelatinous animal biomass is under-sampled using net sampling and also demonstrate that analysis of eDNA provides another, complementary approach to detecting these fragile animals.
Biomass and eDNA Sampling Strategy
Our sampling strategy focused on acoustic scattering layers identified by the 18 and 38 kHz (deep scattering layers) and the 120 and 200 kHz (near-surface scattering layers) Simrad EK60 echosounders. These frequencies are typically used to detect biomass in fisheries surveys (Jech and Sullivan, 2014; Proud et al., 2019). We chose this approach as these layers likely had relatively high concentrations of organisms, although the types of taxa detected by acoustic backscatter is frequency-dependent (Lavery et al., 2007). The lower frequencies that we used (18 and 38 kHz) were more informative for our sampling as they penetrate to deeper depths. These frequencies also tend to detect gas-bearing organisms such as siphonophores and fish with swim bladders (Lavery et al., 2007) although deep scattering layers may contain a wide range of animal taxa (Stefanoudis et al., 2019). While the relationship between eDNA concentration and abundance or biomass is often not direct (Andruszkiewicz et al., 2017b), biomass indicated by acoustic backscatter has been correlated with eDNA signals. Yamamoto et al. (2016) found that in general, fish eDNA signals were correlated with biomass as determined from a 120 kHz echosounder in Maizuru Bay, a shallow semi-enclosed water body in the Sea of Japan. They noted, though, that confounding factors, such as the presence of exogenous eDNA sources, could disrupt this correlation.
The fact that we sampled in the deep-scattering layers where acoustic backscatter from ship-borne echosounders presumably indicates the presence of fish, yet did not detect fish eDNA, suggests more effort is needed for understanding how eDNA relates to biomass in the mesopelagic region. While 18S V9 is known to pick up a broad range of animals including fish (Blanco-Bercial, 2020), other taxa are potentially preferentially amplified (Sawaya et al., 2019). Fish were also only a very small component of the MOCNESS DNA results; however the failure to sequence three of our fish specimens from the MOCNESS sampling also suggests that some species may be missed with this marker. Analysis of eDNA samples with a marker such as 12S that targets vertebrates may be needed to uncover fish biodiversity (Andruszkiewicz et al., 2017b; Kelly et al., 2017). Additionally, the frequency-dependent scattering by organisms can bias our interpretation of the types of organisms present using acoustic echograms. This is especially true using ship-borne systems where the frequency content of the acoustic data decreases with range from the transducer—i.e., “higher” frequencies attenuate with depth so only “lower” frequency data are available for interpretation. Notably, our mesopelagic sampling was based on the two lower frequencies (18 and 38 kHz) and while we did not detect fish, we did observe a significant eDNA signal from siphonophores. Furthermore, our Blast search found that several siphonophore ASVs matched families in the suborder Physonectae, which contain gas-filled pneumatophores (Dunn et al., 2005), suggesting that they could be the source of those acoustic signals. eDNA studies that focus solely on 12S may miss these potentially important taxa.
In the near-surface scattering layers, the combination of all frequencies (18, 38, 120, and 200 kHz) provided additional information on a greater variety of animals and presumably a less biased interpretation (Davison et al., 2015; Bassett et al., 2020). Vertically migrating mesopelagic animals, including fish, crustacea, and gelatinous animals, comprise these near-surface scattering layers at night, and surface data collected at night may provide useful information on these mesopelagic species. Focusing on surface sampling during the night could thus be an efficient strategy; however, deep water sampling is necessary to identify non-migrating mesopelagic species.
Because our sampling depths were chosen based on real time echosounder data, sampling depths differed between casts and were not consistent with the MOCNESS intervals. To look for patterns associated with depth, we therefore combined our data from all casts into four 100–300 m depth bins and found that composition of our eDNA reads differed significantly among these depth bins. Thus, eDNA appears to be a suitable tool for examining biodiversity patterns at that vertical scale. Finer scale resolution of potential vertical patterns, such as those associated with acoustically detected layers, was limited by the number of Niskin bottles (and thus samples) available to us (8) given our goal to explore variation in the water column, but could be a direction for future research. Other studies have found that eDNA can resolve community differences between marine habitats, despite the biological and physical interconnectedness of those habitats (Jeunen et al., 2019; Laroche et al., 2020; West et al., 2020).
Interpreting Mesopelagic eDNA Signals
Many biological and physical factors could have influenced our observed eDNA signals, including eDNA shedding rates, decay rates, and transport (Sansom and Sassoubre, 2017). As described above, eDNA shedding estimates are derived primarily from fish, but there is some evidence that medusae may shed eDNA at a substantially higher rate (Minamoto et al., 2017; Allan et al., 2020). This could potentially result in a disproportionately greater observed read abundance for medusozoan taxa. Shedding rates are also influenced by temperature (Lacoursière-Roussel et al., 2016), and constraining shedding rates of vertically migrating mesopelagic animals that experience a range of temperatures on a daily basis may be especially complex. eDNA decay estimates, which are also based primarily on fish studies, suggest that eDNA can be detected about 1–7 days after shedding (Thomsen et al., 2012; Sansom and Sassoubre, 2017). However, like shedding, the decay rate depends on a variety of environmental factors such as temperature. Cowart et al. (2018) reported a slower decay rate (with a half-life of 37.2 h) potentially allowing detection after 25 days from fish in subzero Antarctic waters. As physical and biogeochemical conditions in the mesopelagic zone are dramatically different from the surface layer of the ocean, eDNA decay rate derived for surface conditions might not be applicable to the mesopelagic zone. Interestingly, one seemingly relevant factor that varies with depth is sunlight, which does not appear to impact eDNA decay (Andruszkiewicz et al., 2017a).
Our eDNA samples may include signals from animals both in our sampling location as well as eDNA transported to that location from elsewhere. Water movement can transport eDNA downstream of its source. Thus, eDNA signals from flowing environments may actually indicate the presence of species in an upstream location (Wacker et al., 2019). Additionally, water mixing can cause the signal of eDNA released over a small spatial scale to quickly become diluted and undetectable (Pilliod et al., 2014; Stoeckle et al., 2017). Most studies on eDNA dispersal to date are from river and stream environments, where the flow is primarily unidirectional. Based on modeling in a coastal system (Monterey Bay, California), Andruszkiewicz et al. (2019) found that eDNA can be transported several 10 s of km horizontally by regional currents over the course of a few days. Open ocean environments are much more complex and subject to the influences of different flows, including mesoscale, sub-mesoscale, tidal, upwelling, and downwelling currents. In the Slope Water off the Northeast U.S. coast where this study occurred, flow can be impacted by the Gulf Stream, warm-core rings, subduction and upwelling along the ring periphery, slope current, tides, and internal tides (Joyce, 1984; Flagg et al., 2006; Kelly et al., 2016; Zhang and Partida, 2018). These flows could redistribute eDNA in the three-dimensional space.
Despite the potential for eDNA dispersal by ocean currents, some studies in coastal systems suggest that the eDNA signal can remain localized. In their survey of Maizuru Bay, Japan, Minamoto et al. (2017) found spatial and temporal correlation between eDNA concentrations of jellyfish from surface and subsurface samples and simultaneously performed visual surface-water observations. Kelly et al. (2018) found that tidal flow had minimal effect on characterizing local communities in tidally dynamic nearshore habitats. Similarly, Jeunen et al. (2019) found unique localized eDNA signatures from four coastal distinct, yet interconnected habitats that were within a few km of each other. Weak spatial dispersal of eDNA signal could result from either long residence time of the water in the eDNA source region or rapid decay of the eDNA materials. The relative importance of physical transport vs. biological degradation in eDNA signal dispersal is a question that calls for further study.
In the mesopelagic ecosystem, there is an additional complicating process not found in other aquatic habitats that may influence our interpretation of our eDNA signals. Diel vertical migration (DVM), or the movement of many mesopelagic animals to surface waters at night to feed and back down to deeper depths during the day, has been referred to as the largest migration on the planet (Hays, 2003; Sutton, 2013). Animals can travel vertical distances of hundreds of meters in each direction on a daily basis. However, we did not find a significant pattern associated with the time of sampling (day vs. night) in any depth bin as might be expected from vertically migrating animals. The lack of day/night signal suggests that the eDNA shedding rate might be very low or the time scale of diel vertical migration might be too short relative to eDNA transport and decay processes, both of which may limit our ability to detect vertical migration (Allan et al., 2020). Furthermore, the animals themselves may expedite the downward transport of eDNA originating from surface organisms through their feeding activities. For example, a migrating animal may feed at the surface at night, and release traces of eDNA from its surface prey through defecation at depth. Thus, while it is tempting to try to infer DVM-related (and other) changes in taxa with depth using eDNA, it is first necessary to understand the spatiotemporal scales of the relevant biological and physical processes in the surface through mesopelagic depths.
Lastly, we note that on our final cast where duplicate samples were taken at four depths, we observed considerable variation in read abundance between those duplicates. Other studies also show variation between replicates (Andruszkiewicz et al., 2017b). We do not currently understand the scale of eDNA distributions, which may be different for different species, and emphasize that the volume of our eDNA samples (5 l) is minimal relative to the scale of the habitat. More work should be done to optimize the scope and scale of eDNA sampling and replication to improve the accuracy of eDNA biodiversity estimates. Additionally, technological advances that allow for multiple, large-volume sample acquisition (Govindarajan et al., 2015; McQuillan and Robidart, 2017) may be especially useful for mesopelagic eDNA studies.
Taxonomy, Reference Libraries, and Marker Resolution
In our results, most of our ASVs did not have exact matches on GenBank. Possible explanations for lack of exact matches are bioinformatics error and lack of available reference sequences. Metabarcoding studies typically uncover vastly more putative taxa than traditional morphological studies (Lindeque et al., 2013; Kelly et al., 2017; Sommer et al., 2017). While to some extent, this greater diversity may be real, it is also possible that the observed diversity is artifactual (Caron and Hu, 2019; Santoferrara, 2019). We feel this is unlikely in our case, as we employed an approach that assesses and corrects likely sequencing errors to produce “amplicon sequencing variants” (ASVs), which is thought to be more accurate than traditional clustering or “operational taxonomic unit” (OTU) approaches (Callahan et al., 2017; Macheriotou et al., 2019), especially for characterizing community biodiversity (Pauvert et al., 2019). We furthermore conservatively removed rare ASVs from our data analysis.
Identifying taxa in metabarcoding studies relies on accurate, well-populated reference libraries (Cristescu, 2014; Bucklin et al., 2016; Elbrecht et al., 2016; Porter and Hajibabaei, 2018). DNA sequences are matched against a reference database for taxon assignment; however, existing databases, including GenBank, are notoriously incomplete or contain errors (Leray and Knowlton, 2016; Lindsay et al., 2017; Santoferrara, 2019). Given the relative inaccessibility of deep oceanic waters, reference libraries may be particularly depauperate for animals in the mesopelagic. Our Genbank results showed matches for a variety of crustaceans and cnidarians; however we also noted an absence of V9 reference sequences on Genbank for many species of mesopelagic fishes (Govindarajan, pers. obs.). For some taxa, there is a mismatch between traditional barcode efforts that focus on the COI and 16S genes (Cristescu, 2014; Lindsay et al., 2015; Elbrecht et al., 2016; Leray and Knowlton, 2016), and metabarcoding analyses which often instead utilize 18S markers to capture a broad range of higher level taxa (de Vargas et al., 2015; Kelly et al., 2017; Djurhuus et al., 2018; Bucklin et al., 2019; Sawaya et al., 2019; Blanco-Bercial, 2020). Additionally, nominal species on Genbank may be misidentified, either due to mistakes in identification due to lack of taxonomic expertise or to the existence of cryptic species (Lindsay et al., 2015, 2017; Abad et al., 2017). Development of reference barcode libraries, grounded in taxonomic expertise (Wheeler, 2018; Pinheiro et al., 2019), for mesopelagic animals are essential for future applications of eDNA analyses in the mesopelagic.
Our goal, however, was primarily to identify higher level taxa rather than species, and V9 is better suited toward this task due to its relatively conserved nature compared to markers such as COI and 16S. Thus, an exact match to a reference V9 sequence might not necessarily indicate a species identification, because multiple closely related species could potentially share that sequence (Abad et al., 2017; Blanco-Bercial, 2020). On the other hand, because evolutionary rates are variable between lineages, a given percent similarity (e.g., 97%) might indicate different degrees of taxonomic classification depending on the lineage. In our Blast searches, we selected a 97% identity as our threshold for reporting Genbank results based on calibration of this marker in copepods (Wu et al., 2015). Similar calibration analyses should be conducted for other animal groups and markers as reference barcode libraries are expanded in order to better understand the taxonomic makeup of the sampled biodiversity.
Conclusion
More information on mesopelagic biodiversity is urgently needed as interest in harvesting these resources increases. eDNA analysis has tremendous potential to contribute to our understanding of this environment and fill important knowledge gaps, such as those related to the detection of animals that are poorly sampled by other methods. eDNA analysis is also highly efficient in terms of the number of taxa recovered per unit effort and can complement other traditional sampling methods. However, eDNA analysis is a new approach that to date has been primarily used in freshwater and coastal marine environments. Based on our results, we suggest the following future research directions for facilitating the application of eDNA analyses to the mesopelagic environment: (1) Experiments to determine eDNA shedding, decay, transport, and dispersal rates under mesopelagic physical and biological conditions to improve our interpretation of eDNA signals; (2) Assess relationships between eDNA and biomass; and (3) Populate genetic reference databases with sequences from mesopelagic species that have been morphologically identified by taxonomic experts for improved taxonomic assignments.
Data Availability Statement
The datasets presented in this study can be found in online repositories. The names of the repository/repositories and accession number(s) can be found below: Dryad repository (doi: 10.5061/dryad.4xgxd255j.
Author Contributions
AG conceived the study and conducted the DNA sequence analyses and drafted the manuscript. AG, AL, JMJ, WZ, JL, and PW planned, prepared, and conducted the shipboard sampling. RF conducted the lab work and assisted with the acoustics plots. JMJ conducted the acoustics analyses. WZ and PW conducted the CTD analyses. All authors contributed to data interpretation, structuring of the manuscript, reviewed and edited the manuscript, and approved the final version for submission.
Funding
This research was part of the Woods Hole Oceanographic Institution’s Ocean Twilight Zone Project, funded as part of The Audacious Project housed at TED. Funding for the NOAA Ship Henry B Bigelow was provided by NOAA’s Office of Marine and Aviation Operations (OMAO).
Conflict of Interest
The authors declare that the research was conducted in the absence of any commercial or financial relationships that could be construed as a potential conflict of interest.
Acknowledgments
We thank the crew and scientists of the NOAA Ship Henry B Bigelow, Glenn McDonald (WHOI) and Helena McMonagle (WHOI) for assistance with eDNA sampling, Bo Reese (UConn Center for Genomic Innovation) for assistance with library preparation and DNA sequencing, Dana Yoerger (WHOI) for assistance with plots, and Elizabeth Allan (WHOI) for helpful comments on the manuscript.
Supplementary Material
The Supplementary Material for this article can be found online at: https://www.frontiersin.org/articles/10.3389/fevo.2021.574877/full#supplementary-material
Footnotes
References
Abad, D., Albaina, A., Aguirre, M., and Estonba, A. (2017). 18S V9 metabarcoding correctly depicts plankton estuarine community drivers. Mar. Ecol. Prog. Ser. 584, 31–43. doi: 10.3354/meps12373
Allan, E. A., Zhang, W. G., Lavery, A. C., and Govindarajan, A. F. (2020). Environmental DNA shedding and decay rates from diverse animal forms and thermal regimes. Environ. DNA 2020:141. doi: 10.1002/edn3.141
Amaral-Zettler, L. A., McCliment, E. A., Ducklow, H. W., and Huse, S. M. (2009). A Method for Studying Protistan Diversity Using Massively Parallel Sequencing of V9 Hypervariable Regions of Small-Subunit Ribosomal RNA Genes. PLoS One 4:e6372. doi: 10.1371/journal.pone.0006372
Andruszkiewicz, E. A., Koseff, J. R., Fringer, O. B., Ouellette, N. T., Lowe, A. B., Edwards, C. A., et al. (2019). Modeling Environmental DNA Transport in the Coastal Ocean Using Lagrangian Particle Tracking. Front. Mar. Sci. 6:.477. doi: 10.3389/fmars.2019.00477
Andruszkiewicz, E. A., Sassoubre, L. M., and Boehm, A. B. (2017a). Persistence of marine fish environmental DNA and the influence of sunlight. PLoS One 12:e0185043. doi: 10.1371/journal.pone.0185043
Andruszkiewicz, E. A., Starks, H. A., Chavez, F. P., Sassoubre, L. M., Block, B. A., and Boehm, A. B. (2017b). Biomonitoring of marine vertebrates in Monterey Bay using eDNA metabarcoding. PLoS One 12:e0176343. doi: 10.1371/journal.pone.0176343
Bassett, C., Lavery, A. C., Stanton, T. K., and Cotter, E. D. (2020). Frequency- and depth-dependent target strength measurements of individual mesopelagic scatterers. J. Acoust. Soc. Am. 148, EL153–EL158. doi: 10.1121/10.0001745
Blanco-Bercial, L. (2020). Metabarcoding Analyses and Seasonality of the Zooplankton Community at BATS. Front. Mar. Sci. 7:173. doi: 10.3389/fmars.2020.00173
Bokulich, N. A., Kaehler, B. D., Rideout, J. R., Dillon, M., Bolyen, E., Knight, R., et al. (2018). Optimizing taxonomic classification of marker-gene amplicon sequences with QIIME 2’s q2-feature-classifier plugin. Microbiome 6:90. doi: 10.1186/s40168-018-0470-z
Bolyen, E., Rideout, J. R., Dillon, M. R., Bokulich, N. A., Abnet, C., Al-Ghalith, G. A., et al. (2018). QIIME 2: Reproducible, interactive, scalable, and extensible microbiome data science. PeerJ 2018:27295v2. doi: 10.7287/peerj.preprints.27295v2
Bucklin, A., Lindeque, P. K., Rodriguez-Ezpeleta, N., Albaina, A., and Lehtiniemi, M. (2016). Metabarcoding of marine zooplankton: prospects, progress and pitfalls. J. Plankton Res. 38, 393–400. doi: 10.1093/plankt/fbw023
Bucklin, A., Yeh, H. D., Questel, J. M., Richardson, D. E., Reese, B., Copley, N. J., et al. (2019). Time-series metabarcoding analysis of zooplankton diversity of the NW Atlantic continental shelf. ICES J. Mar. Sci. 76, 1162–1176. doi: 10.1093/icesjms/fsz021
Callahan, B. J., McMurdie, P. J., and Holmes, S. P. (2017). Exact sequence variants should replace operational taxonomic units in marker-gene data analysis. ISME J. 11, 2639–2643. doi: 10.1038/ismej.2017.119
Callahan, B. J., McMurdie, P. J., Rosen, M. J., Han, A. W., Johnson, A. J. A., and Holmes, S. P. (2016). DADA2: High-resolution sample inference from Illumina amplicon data. Nat. Methods 13, 581–583. doi: 10.1038/nmeth.3869
Caron, D. A., and Hu, S. K. (2019). Are We Overestimating Protistan Diversity in Nature? Trends Microbiol. 27, 197–205. doi: 10.1016/j.tim.2018.10.009
Casas, L., Pearman, J. K., and Irigoien, X. (2017). Metabarcoding Reveals Seasonal and Temperature-Dependent Succession of Zooplankton Communities in the Red Sea. Front. Mar. Sci. 4:241. doi: 10.3389/fmars.2017.00241
Closek, C. J., Santora, J. A., Starks, H. A., Schroeder, I. D., Andruszkiewicz, E. A., Sakuma, K. M., et al. (2019). Marine Vertebrate Biodiversity and Distribution Within the Central California Current Using Environmental DNA (eDNA) Metabarcoding and Ecosystem Surveys. Front. Mar. Sci. 6:732. doi: 10.3389/fmars.2019.00732
Cowart, D. A., Murphy, K. R., and Cheng, C.-H. C. (2018). Metagenomic sequencing of environmental DNA reveals marine faunal assemblages from the West Antarctic Peninsula. Mar. Genomics 37, 148–160. doi: 10.1016/j.margen.2017.11.003
Cristescu, M. E. (2014). From barcoding single individuals to metabarcoding biological communities: towards an integrative approach to the study of global biodiversity. Trends Ecol. Evol. 29, 566–571. doi: 10.1016/j.tree.2014.08.001
D’Elia, M., Warren, J. D., Rodriguez-Pinto, I., Sutton, T. T., Cook, A., and Boswell, K. M. (2016). Diel variation in the vertical distribution of deep-water scattering layers in the Gulf of Mexico. Deep Sea Res. Part I Oceanogr. Res. Papers 115, 91–102. doi: 10.1016/j.dsr.2016.05.014
Davison, P. C., Koslow, J. A., and Kloser, R. J. (2015). Acoustic biomass estimation of mesopelagic fish: backscattering from individuals, populations, and communities. ICES J. Mar. Sci. 72, 1413–1424. doi: 10.1093/icesjms/fsv023
de Vargas, C., de, Audic, S., Henry, N., Decelle, J., Mahé, F., et al. (2015). Eukaryotic plankton diversity in the sunlit ocean. Science 348:1261605. doi: 10.1126/science.1261605
Deiner, K., Bik, H. M., Mächler, E., Seymour, M., Lacoursière−Roussel, A., Altermatt, F., et al. (2017). Environmental DNA metabarcoding: Transforming how we survey animal and plant communities. Mol. Ecol. 26, 5872–5895. doi: 10.1111/mec.14350
Dejean, T., Valentini, A., Miquel, C., Taberlet, P., Bellemain, E., and Miaud, C. (2012). Improved detection of an alien invasive species through environmental DNA barcoding: the example of the American bullfrog Lithobates catesbeianus. J. Appl. Ecol. 49, 953–959. doi: 10.1111/j.1365-2664.2012.02171.x
Djurhuus, A., Pitz, K., Sawaya, N. A., Rojas−Márquez, J., Michaud, B., Montes, E., et al. (2018). Evaluation of marine zooplankton community structure through environmental DNA metabarcoding. Limnol. Oceanogr. Methods 16, 209–221. doi: 10.1002/lom3.10237
Djurhuus, A., Port, J., Closek, C. J., Yamahara, K. M., Romero-Maraccini, O., Walz, K. R., et al. (2017). Evaluation of Filtration and DNA Extraction Methods for Environmental DNA Biodiversity Assessments across Multiple Trophic Levels. Front. Mar. Sci. 4:314. doi: 10.3389/fmars.2017.00314
Dunn, C. W., Pugh, P. R., and Haddock, S. H. D. (2005). Molecular Phylogenetics of the Siphonophora (Cnidaria), with Implications for the Evolution of Functional Specialization. Syst. Biol. 54, 916–935. doi: 10.1080/10635150500354837
Elbrecht, V., Taberlet, P., Dejean, T., Valentini, A., Usseglio-Polatera, P., Beisel, J.-N., et al. (2016). Testing the potential of a ribosomal 16S marker for DNA metabarcoding of insects. PeerJ 4:e1966. doi: 10.7717/peerj.1966
Evans, N. T., Li, Y., Renshaw, M. A., Olds, B. P., Deiner, K., Turner, C. R., et al. (2017). Fish community assessment with eDNA metabarcoding: effects of sampling design and bioinformatic filtering. Can. J. Fish. Aquat. Sci. 74, 1362–1374. doi: 10.1139/cjfas-2016-0306
Flagg, C. N., Dunn, M., Wang, D.-P., Rossby, H. T., and Benway, R. L. (2006). A study of the currents of the outer shelf and upper slope from a decade of shipboard ADCP observations in the Middle Atlantic Bight. J. Geophys. Res. Oceans 111:2005JC003116. doi: 10.1029/2005JC003116
Giering, S. L. C., Sanders, R., Lampitt, R. S., Anderson, T. R., Tamburini, C., Boutrif, M., et al. (2014). Reconciliation of the carbon budget in the ocean’s twilight zone. Nature 507, 480–483. doi: 10.1038/nature13123
Glover, A. G., Wiklund, H., Chen, C., and Dahlgren, T. G. (2018). Managing a sustainable deep-sea ‘blue economy’ requires knowledge of what actually lives there. eLife 7:e41319. doi: 10.7554/eLife.41319
Govindarajan, A. F., Pineda, J., Purcell, M., and Breier, J. A. (2015). Species- and stage-specific barnacle larval distributions obtained from AUV sampling and genetic analysis in Buzzards Bay, Massachusetts, USA. J. Exp. Mar. Biol. Ecol. 472, 158–165. doi: 10.1016/j.jembe.2015.07.012
Hays, G. C. (2003). A review of the adaptive significance and ecosystem consequences of zooplankton diel vertical migrations. Hydrobiologia 503, 163–170. doi: 10.1023/B:HYDR.0000008476.23617.b0
Hazen, E. L., and Johnston, D. W. (2010). Meridional patterns in the deep scattering layers and top predator distribution in the central equatorial Pacific. Fisher. Oceanogr. 19, 427–433. doi: 10.1111/j.1365-2419.2010.00561.x
Hidalgo, M., and Browman, H. I. (2019). Developing the knowledge base needed to sustainably manage mesopelagic resources. ICES J. Mar. Sci. 76, 609–615. doi: 10.1093/icesjms/fsz067
Irigoien, X., Klevjer, T. A., Røstad, A., Martinez, U., Boyra, G., Acuña, J. L., et al. (2014). Large mesopelagic fishes biomass and trophic efficiency in the open ocean. Nat. Commun. 5:3271. doi: 10.1038/ncomms4271
Jech, J. M., and Sullivan, P. J. (2014). Distribution of Atlantic herring (Clupea harengus) in the Gulf of Maine from 1998 to 2012. Fisher. Res. 156, 26–33. doi: 10.1016/j.fishres.2014.04.016
Jeunen, G.-J., Knapp, M., Spencer, H. G., Lamare, M. D., Taylor, H. R., Stat, M., et al. (2019). Environmental DNA (eDNA) metabarcoding reveals strong discrimination among diverse marine habitats connected by water movement. Mol. Ecol. Resour. 19, 426–438. doi: 10.1111/1755-0998.12982
Jo, T., Murakami, H., Masuda, R., Sakata, M. K., Yamamoto, S., and Minamoto, T. (2017). Rapid degradation of longer DNA fragments enables the improved estimation of distribution and biomass using environmental DNA. Mol. Ecol. Resour. 17, 25–33e. doi: 10.1111/1755-0998.12685
Joyce, T. M. (1984). Velocity and Hydrographic Structure of a Gulf Stream Warm-Core Ring. J. Phys. Oceanogr. 14, 936–947. doi: 10.1175/1520-04851984014<0936:VAHSOA<2.0.CO;2
Kelly, R. P., Closek, C. J., O’Donnell, J. L., Kralj, J. E., Shelton, A. O., and Samhouri, J. F. (2017). Genetic and Manual Survey Methods Yield Different and Complementary Views of an Ecosystem. Front. Mar. Sci. 3:283. doi: 10.3389/fmars.2016.00283
Kelly, R. P., Gallego, R., and Jacobs-Palmer, E. (2018). The effect of tides on nearshore environmental DNA. PeerJ 6:e4521. doi: 10.7717/peerj.4521
Kelly, S. M., Lermusiaux, P. F. J., Duda, T. F., and Haley, P. J. (2016). A Coupled-Mode Shallow-Water Model for Tidal Analysis: Internal Tide Reflection and Refraction by the Gulf Stream. J. Phys. Oceanogr. 46, 3661–3679. doi: 10.1175/JPO-D-16-0018.1
Kinzer, J. (1969). On the quantitative distribution of zooplankton in deep scattering layers. Deep Sea Res. Oceanogr. Abstracts 16, 117–125. doi: 10.1016/0011-7471(69)90068-0
Klymus, K. E., Richter, C. A., Chapman, D. C., and Paukert, C. (2015). Quantification of eDNA shedding rates from invasive bighead carp Hypophthalmichthys nobilis and silver carp Hypophthalmichthys molitrix. Biol. Conserv. 183, 77–84. doi: 10.1016/j.biocon.2014.11.020
Lacoursière-Roussel, A., Rosabal, M., and Bernatchez, L. (2016). Estimating fish abundance and biomass from eDNA concentrations: variability among capture methods and environmental conditions. Mol. Ecol. Resour. 16, 1401–1414. doi: 10.1111/1755-0998.12522
Laroche, O., Kersten, O., Smith, C. R., and Goetze, E. (2020). Environmental DNA surveys detect distinct metazoan communities across abyssal plains and seamounts in the western Clarion Clipperton Zone. Mol. Ecol. 2020:15484. doi: 10.1111/mec.15484
Larson, R. J., Mills, C. E., and Harbison, G. R. (1991). Western Atlantic midwater hydrozoan and scyphozoan medusae: in situ studies using manned submersibles. Hydrobiologia 216, 311–317. doi: 10.1007/BF00026480
Lavery, A. C., Wiebe, P. H., Stanton, T. K., Lawson, G. L., Benfield, M. C., and Copley, N. (2007). Determining dominant scatterers of sound in mixed zooplankton populations. J. Acoust. Soc. Am. 122, 3304–3326. doi: 10.1121/1.2793613
Leray, M., and Knowlton, N. (2016). Censusing marine eukaryotic diversity in the twenty-first century. Philosop. Trans. R. Soc. B Biol. Sci. 371:20150331. doi: 10.1098/rstb.2015.0331
Lindeque, P. K., Parry, H. E., Harmer, R. A., Somerfield, P. J., and Atkinson, A. (2013). Next Generation Sequencing Reveals the Hidden Diversity of Zooplankton Assemblages. PLoS One 8:e81327. doi: 10.1371/journal.pone.0081327
Lindsay, D. J., Grossmann, M. M., Bentlage, B., Collins, A. G., Minemizu, R., Hopcroft, R. R., et al. (2017). The perils of online biogeographic databases: a case study with the ‘monospecific’ genus Aegina (Cnidaria, Hydrozoa, Narcomedusae). Mar. Biol. Res. 13, 494–512. doi: 10.1080/17451000.2016.1268261
Lindsay, D. J., Grossmann, M. M., Nishikawa, J., Bentlage, B., and Collins, A. G. (2015). DNA barcoding of pelagic cnidarians: current status and future prospects. Bull. Plankton Soc. Japan 62, 39–43. doi: 10.24763/bpsj.62.1_39
Liu, Y., Wikfors, G. H., Rose, J. M., McBride, R. S., Milke, L. M., and Mercaldo-Allen, R. (2019). Application of Environmental DNA Metabarcoding to Spatiotemporal Finfish Community Assessment in a Temperate Embayment. Front. Mar. Sci. 6:674. doi: 10.3389/fmars.2019.00674
Macheriotou, L., Guilini, K., Bezerra, T. N., Tytgat, B., Nguyen, D. T., Nguyen, T. X. P., et al. (2019). Metabarcoding free-living marine nematodes using curated 18S and CO1 reference sequence databases for species-level taxonomic assignments. Ecol. Evol. 9, 1211–1226. doi: 10.1002/ece3.4814
Madin, L. P., and Harbison, G. R. (1978). Bathocyroe fosteri gen.nov., sp.nov.: a mesopelagic ctenophore observed and collected from a submersible. J. Mar. Biol. Associat. U K. 58, 559–564. doi: 10.1017/S0025315400041217
Martin, M. (2011). Cutadapt removes adapter sequences from high-throughput sequencing reads. EMBnet J. 17, 10–12. doi: 10.14806/ej.17.1.200
McEwen, G. F., Johnson, M. W., and Folsom, T. R. (1954). A statistical analysis of the performance of the folsom plankton sample splitter, based upon test observations. Arch. Met. Geoph. Biokl. A 7, 502–527. doi: 10.1007/BF02277939
McQuillan, J. S., and Robidart, J. C. (2017). Molecular-biological sensing in aquatic environments: recent developments and emerging capabilities. Curr. Opin. Biotechnol. 45, 43–50. doi: 10.1016/j.copbio.2016.11.022
Minamoto, T., Fukuda, M., Katsuhara, K. R., Fujiwara, A., Hidaka, S., Yamamoto, S., et al. (2017). Environmental DNA reflects spatial and temporal jellyfish distribution. PLoS One 12:e0173073. doi: 10.1371/journal.pone.0173073
Nevers, M. B., Byappanahalli, M. N., Morris, C. C., Shively, D., Przybyla-Kelly, K., Spoljaric, A. M., et al. (2018). Environmental DNA (eDNA): A tool for quantifying the abundant but elusive round goby (Neogobius melanostomus). PLoS One 13:e0191720. doi: 10.1371/journal.pone.0191720
Oksanen, J., Blanchet, F. G., Friendly, M., Kindt, R., Legendre, P., McGlinn, D., et al. (2016). vegan: Community Ecology Package. R package version 2.4-3. Vienna: R Foundation for Statistical Computing.
Orlowski, A. (1990). Hydroacoustic characteristics of scattering layers in the Northeastern Atlantic Ocean. J. Acoust. Soc. Am. 88, 298–309. doi: 10.1121/1.399952
Pauvert, C., Buée, M., Laval, V., Edel-Hermann, V., Fauchery, L., Gautier, A., et al. (2019). Bioinformatics matters: The accuracy of plant and soil fungal community data is highly dependent on the metabarcoding pipeline. Fungal Ecol. 41, 23–33. doi: 10.1016/j.funeco.2019.03.005
Pearman, J. K., El-Sherbiny, M. M., Lanzén, A., Al-Aidaroos, A. M., and Irigoien, X. (2014). Zooplankton diversity across three Red Sea reefs using pyrosequencing. Front. Mar. Sci. 1:27. doi: 10.3389/fmars.2014.00027
Piaggio, A. J., Engeman, R. M., Hopken, M. W., Humphrey, J. S., Keacher, K. L., Bruce, W. E., et al. (2014). Detecting an elusive invasive species: a diagnostic PCR to detect Burmese python in Florida waters and an assessment of persistence of environmental DNA. Mol. Ecol. Resour. 14, 374–380. doi: 10.1111/1755-0998.12180
Pilliod, D. S., Goldberg, C. S., Arkle, R. S., and Waits, L. P. (2014). Factors influencing detection of eDNA from a stream-dwelling amphibian. Mol. Ecol. Resour. 14, 109–116. doi: 10.1111/1755-0998.12159
Pinheiro, H. T., Moreau, C. S., Daly, M., and Rocha, L. A. (2019). Will DNA barcoding meet taxonomic needs? Science 365, 873–874. doi: 10.1126/science.aay7174
Porter, T. M., and Hajibabaei, M. (2018). Automated high throughput animal CO1 metabarcode classification. Sci. Rep. 8, 1–10. doi: 10.1038/s41598-018-22505-4
Proud, R., Kloser, R. J., Handegard, N. O., Cox, M. J., and Brierley, A. S. (2019). Mapping the global prey-field: Combining acoustics, optics and net samples to reduce uncertainty in estimates of mesopelagic biomass. J. Acoust. Soc. Am. 146, 2898–2898. doi: 10.1121/1.5137055
Quast, C., Pruesse, E., Yilmaz, P., Gerken, J., Schweer, T., Yarza, P., et al. (2013). The SILVA ribosomal RNA gene database project: improved data processing and web-based tools. Nucleic Acids Res. 41, D590–D596. doi: 10.1093/nar/gks1219
Ricker, W. E. (1973). Linear Regressions in Fishery Research. J. Fisher. Board Can. 30, 409–434. doi: 10.1139/f73-072
Robison, B. H. (2004). Deep pelagic biology. J. Exp. Mar. Biol. Ecol. 300, 253–272. doi: 10.1016/j.jembe.2004.01.012
Robison, B. H. (2009). Conservation of Deep Pelagic Biodiversity. Conserv. Biol. 23, 847–858. doi: 10.1111/j.1523-1739.2009.01219.x
Ruppert, K. M., Kline, R. J., and Rahman, M. S. (2019). Past, present, and future perspectives of environmental DNA (eDNA) metabarcoding: A systematic review in methods, monitoring, and applications of global eDNA. Glob. Ecol. Conserv. 17:e00547. doi: 10.1016/j.gecco.2019.e00547
Sansom, B. J., and Sassoubre, L. M. (2017). Environmental DNA (eDNA) Shedding and Decay Rates to Model Freshwater Mussel eDNA Transport in a River. Environ. Sci. Technol. 51, 14244–14253. doi: 10.1021/acs.est.7b05199
Santoferrara, L. F. (2019). Current practice in plankton metabarcoding: optimization and error management. J. Plankton Res. 41, 571–582. doi: 10.1093/plankt/fbz041
Sassoubre, L. M., Yamahara, K. M., Gardner, L. D., Block, B. A., and Boehm, A. B. (2016). Quantification of Environmental DNA (eDNA) Shedding and Decay Rates for Three Marine Fish. Environ. Sci. Technol. 50, 10456–10464. doi: 10.1021/acs.est.6b03114
Sawaya, N. A., Djurhuus, A., Closek, C. J., Hepner, M., Olesin, E., Visser, L., et al. (2019). Assessing eukaryotic biodiversity in the Florida Keys National Marine Sanctuary through environmental DNA metabarcoding. Ecol. Evol. 9, 1029–1040. doi: 10.1002/ece3.4742
Sigsgaard, E. E., Carl, H., Møller, P. R., and Thomsen, P. F. (2015). Monitoring the near-extinct European weather loach in Denmark based on environmental DNA from water samples. Biol. Conserv. 183, 46–52. doi: 10.1016/j.biocon.2014.11.023
Sigsgaard, E. E., Nielsen, I. B., Carl, H., Krag, M. A., Knudsen, S. W., Xing, Y., et al. (2017). Seawater environmental DNA reflects seasonality of a coastal fish community. Mar. Biol. 164:128. doi: 10.1007/s00227-017-3147-4
Sommer, S. A., Woudenberg, L. V., Lenz, P. H., Cepeda, G., and Goetze, E. (2017). Vertical gradients in species richness and community composition across the twilight zone in the North Pacific Subtropical Gyre. Mol. Ecol. 26, 6136–6156. doi: 10.1111/mec.14286
St. John, M. A., Borja, A., Chust, G., Heath, M., Grigorov, I., Mariani, P., et al. (2016). A Dark Hole in Our Understanding of Marine Ecosystems and Their Services: Perspectives from the Mesopelagic Community. Front. Mar. Sci. 3:31. doi: 10.3389/fmars.2016.00031
Stat, M., John, J., DiBattista, J. D., Newman, S. J., Bunce, M., and Harvey, E. S. (2019). Combined use of eDNA metabarcoding and video surveillance for the assessment of fish biodiversity. Conserv. Biol. 33, 196–205. doi: 10.1111/cobi.13183
Stefanoudis, P. V., Rivers, M., Ford, H., Yashayaev, I. M., Rogers, A. D., and Woodall, L. C. (2019). Changes in zooplankton communities from epipelagic to lower mesopelagic waters. Mar. Environ. Res. 146, 1–11. doi: 10.1016/j.marenvres.2019.02.014
Stoeckle, M. Y., Soboleva, L., and Charlop-Powers, Z. (2017). Aquatic environmental DNA detects seasonal fish abundance and habitat preference in an urban estuary. PLoS One 12:175186. doi: 10.1371/journal.pone.0175186
Sutton, T. T. (2013). Vertical ecology of the pelagic ocean: classical patterns and new perspectives. J. Fish Biol. 83, 1508–1527. doi: 10.1111/jfb.12263
Suzuki, K. S., Niida, Y., Tsubono, T., Takimoto, H., Kumakura, E., Ishii, H., et al. (2018). Mechanisms underlying heterogeneous distribution of moon jellyfish Aurelia aurita s.l. across a sharp pycnocline. Mar. Ecol. Prog. Ser. 591, 229–239. doi: 10.3354/meps12357
Takahara, T., Minamoto, T., Yamanaka, H., Doi, H., and Kawabata, Z. (2012). Estimation of Fish Biomass Using Environmental DNA. PLoS One 7:e35868. doi: 10.1371/journal.pone.0035868
Thomsen, P. F., and Willerslev, E. (2015). Environmental DNA – An emerging tool in conservation for monitoring past and present biodiversity. Biol. Conserv. 183, 4–18. doi: 10.1016/j.biocon.2014.11.019
Thomsen, P. F., Kielgast, J., Iversen, L. L., Wiuf, C., Rasmussen, M., Gilbert, M. T. P., et al. (2012). Monitoring endangered freshwater biodiversity using environmental DNA. Mol. Ecol. 21, 2565–2573. doi: 10.1111/j.1365-294X.2011.05418.x
Thomsen, P. F., Møller, P. R., Sigsgaard, E. E., Knudsen, S. W., Jørgensen, O. A., and Willerslev, E. (2016). Environmental DNA from Seawater Samples Correlate with Trawl Catches of Subarctic, Deepwater Fishes. PLoS One 11:165252. doi: 10.1371/journal.pone.0165252
Wacker, S., Fossøy, F., Larsen, B. M., Brandsegg, H., Sivertsgård, R., and Karlsson, S. (2019). Downstream transport and seasonal variation in freshwater pearl mussel (Margaritifera margaritifera) eDNA concentration. Environ. DNA 1, 64–73. doi: 10.1002/edn3.10
Webb, T. J., Berghe, E. V., and O’Dor, R. (2010). Biodiversity’s Big Wet Secret: The Global Distribution of Marine Biological Records Reveals Chronic Under-Exploration of the Deep Pelagic Ocean. PLoS One 5:e10223. doi: 10.1371/journal.pone.0010223
West, K. M., Stat, M., Harvey, E. S., Skepper, C. L., DiBattista, J. D., Richards, Z. T., et al. (2020). eDNA metabarcoding survey reveals fine-scale coral reef community variation across a remote, tropical island ecosystem. Mol. Ecol. 29, 1069–1086. doi: 10.1111/mec.15382
Wheeler, Q. (2018). Blank Canvas: The Case for Descriptive Taxonomy. Integr. Comp. Biol. 58, 1118–1121. doi: 10.1093/icb/icy067
Wiebe, P. H., Morton, A. W., Bradley, A. M., Backus, R. H., Craddock, J. E., Barber, V., et al. (1985). New development in the MOCNESS, an apparatus for sampling zooplankton and micronekton. Mar. Biol. 87, 313–323. doi: 10.1007/BF00397811
Wu, S., Xiong, J., and Yu, Y. (2015). Taxonomic Resolutions Based on 18S rRNA Genes: A Case Study of Subclass Copepoda. PLoS One 10:131498. doi: 10.1371/journal.pone.0131498
Yamamoto, S., Minami, K., Fukaya, K., Takahashi, K., Sawada, H., Murakami, H., et al. (2016). Environmental DNA as a ‘Snapshot’ of Fish Distribution: A Case Study of Japanese Jack Mackerel in Maizuru Bay, Sea of Japan. PLoS One 11:e0149786. doi: 10.1371/journal.pone.0149786
Keywords: environmental DNA, mesopelagic, biodiversity, metabarcoding, zooplankton
Citation: Govindarajan AF, Francolini RD, Jech JM, Lavery AC, Llopiz JK, Wiebe PH and Zhang W (2021) Exploring the Use of Environmental DNA (eDNA) to Detect Animal Taxa in the Mesopelagic Zone. Front. Ecol. Evol. 9:574877. doi: 10.3389/fevo.2021.574877
Received: 23 June 2020; Accepted: 22 February 2021;
Published: 15 March 2021.
Edited by:
Hiroki Yamanaka, Ryukoku University, JapanReviewed by:
Bettina Thalinger, University of Guelph, CanadaJudith Van Bleijswijk, Royal Netherlands Institute for Sea Research (NIOZ), Netherlands
Copyright © 2021 Govindarajan, Francolini, Jech, Lavery, Llopiz, Wiebe and Zhang. This is an open-access article distributed under the terms of the Creative Commons Attribution License (CC BY). The use, distribution or reproduction in other forums is permitted, provided the original author(s) and the copyright owner(s) are credited and that the original publication in this journal is cited, in accordance with accepted academic practice. No use, distribution or reproduction is permitted which does not comply with these terms.
*Correspondence: Annette F. Govindarajan, YWZyZXNlQHdob2kuZWR1