- 1Department of Animal Ecology, Netherlands Institute of Ecology (NIOO-KNAW), Wageningen, Netherlands
- 2Behavioural Ecology Group, Wageningen University & Research (WUR), Wageningen, Netherlands
- 3Bioinformatics Unit, Netherlands Institute of Ecology (NIOO-KNAW), Wageningen, Netherlands
- 4Finnish Museum of Natural History, University of Helsinki, Helsinki, Finland
Early developmental conditions are known to have life-long effects on an individual’s behavior, physiology and fitness. In altricial birds, a majority of these conditions, such as the number of siblings and the amount of food provisioned, are controlled by the parents. This opens up the potential for parents to adjust the behavior and physiology of their offspring according to local post-natal circumstances. However, the mechanisms underlying such intergenerational regulation remain largely unknown. A mechanism often proposed to possibly explain how parental effects mediate consistent phenotypic change is DNA methylation. To investigate whether early life effects on offspring phenotypes are mediated by DNA methylation, we cross-fostered great tit (Parus major) nestlings and manipulated their brood size in a natural study population. We assessed genome-wide DNA methylation levels of CpG sites in erythrocyte DNA, using Reduced Representation Bisulfite Sequencing (RRBS). By comparing DNA methylation levels between biological siblings raised in enlarged and reduced broods and between biological siblings of control broods, we assessed which CpG sites were differentially methylated due to brood size. We found 32 differentially methylated sites (DMS) between siblings from enlarged and reduced broods, a larger number than in the comparison between siblings from control broods. A considerable number of these DMS were located in or near genes involved in development, growth, metabolism, behavior and cognition. Since the biological functions of these genes line up with previously found effects of brood size and food availability, it is likely that the nestlings in the enlarged broods suffered from nutritional stress. We therefore conclude that early life stress might directly affect epigenetic regulation of genes related to early life conditions. Future studies should link such experimentally induced DNA methylation changes to expression of phenotypic traits and assess whether these effects affect parental fitness to determine if such changes are also adaptive.
Introduction
Developmental phenotypic plasticity can be defined as irreversible changes in the phenotype resulting from environmentally introduced alterations in development (Forsman, 2015). These changes can occur through parental effects, which occur when the parental environment or phenotype affects that of their offspring. Parents with extended brood care are known to affect their offspring via both prenatal and postnatal effects. Well known prenatal effects are those that occurred in humans after prenatal exposure to the Dutch famine, leading to for example lower glucose tolerance (de Rooij et al., 2006), obesity (Roseboom et al., 2006), diabetes (Kahn et al., 2009) and impaired selective attention (de Rooij et al., 2010). A classic example of a postnatal parental effect is that of maternal nursing and grooming on anxiety and stress response of rat pups (Weaver et al., 2004). A likely reason why parents adjust their offspring’s phenotype is to maximize parental fitness (Reddon, 2012), by transferring information about the current environment to their offspring and subsequently shape their offspring’s phenotype to match the environmental conditions. If these conditions remain stable, this might increase their offspring’s reproduction or survival (Champagne et al., 2003; Dantzer et al., 2013).
Parents can passively pass on information about current environmental conditions via prenatal hormone secretion (Dloniak et al., 2006; Dantzer et al., 2013) and resource allocation (de Rooij et al., 2006; Roseboom et al., 2006; Kahn et al., 2009) as has been observed in mammals or by yolk hormone deposition as observed in various bird species (Schwabl, 1993; Bentz et al., 2016). For example, yolk testosterone in wild Eastern Bluebirds (Sialia sialis) is positively correlated with breeding density and nestling growth (Bentz et al., 2016). Parents can also transfer information about current environmental conditions in an active way, by for example grooming behavior (Champagne et al., 2003; Weaver et al., 2004), thereby shaping early environmental conditions of the offspring after birth. Such early developmental conditions provided by the parents are known to have long-term influences on their offspring’s behavior (Carere et al., 2005; van Oers et al., 2015), physiology (Keller and van Noordwijk, 1994; DeKogel, 1997; Naef-Daenzer and Keller, 1999) and may also have fitness consequences (DeKogel, 1997; Naguib et al., 2006). Intergenerational parental effects indicate an information transfer from parent to offspring (Jablonka and Raz, 2009; Bošković and Rando, 2018) but this does not imply that the patterns will be stably inherited via parental germ cells (Heard and Martienssen, 2014; Guerrero-Bosagna et al., 2018).
Epigenetic mechanisms have repeatedly been suggested to mediate the parental regulation of offspring phenotype (Kappeler and Meaney, 2010; Groothuis and Trillmich, 2011; Kilvitis et al., 2014). These biochemical mechanisms stably alter gene expression by affecting either transcription or translation without a change in the primary nucleotide sequence of the genome. Since epigenetic mechanisms can be induced in response to the local environment (Weaver et al., 2004; Pertille et al., 2017; Zimmer et al., 2017; Liu et al., 2018) they are good candidates to facilitate early developmental effects on offspring phenotype. The best-studied epigenetic mark is DNA methylation, which is the addition of a methyl group to a nucleotide. In vertebrates, this nucleotide is usually a cytosine in a CpG context, which is a CG dinucleotide (5′-cytosine guanine-3′), separated by a phosphate (p) group. Methylation can affect gene expression by interfering with the binding of proteins necessary for transcription initiation (Bird, 2002; Moore et al., 2013). Pre- and postnatal parental effects on offspring DNA methylation have been found in vertebrates like humans (Tobi et al., 2009, 2014), fish (McGhee and Bell, 2014), rats (Weaver et al., 2004) and mice (St-Cyr and McGowan, 2015), but this is hardly studied in altricial birds, even though there are some very suitable model systems.
In altricial birds in natural conditions, a major part of the early developmental conditions are largely determined by the parents, because the nestlings completely rely on their parents for nutrition. The parents are able to affect the quality and quantity of food per nestling by adjusting the egg laying date and brood size (Pettifor et al., 1988, 2001; Perrins and McCleery, 1989), prey choice, food selectivity (Wright et al., 1998; García-Navas and Sanz, 2010; Mathot et al., 2017), provisioning frequency and food allocation (Christe et al., 1996; Naef-Daenzer and Keller, 1999; Wilkin et al., 2009; Mutzel et al., 2013; van Oers et al., 2015; Caro et al., 2016). Early environmental conditions have been extensively studied in altricial birds, since offspring may experience variable natural environmental conditions that are easily manipulated experimentally, such as brood size. The effects of brood size are likely due to nutritional stress, but the direct causes of nutritional stress may depend on the provisioning tactics of the parents (Mathot et al., 2017). Parents may be unable to compensate for an increased food demand (Gow and Wiebe, 2014; Mathot et al., 2017) or may increase the feeding frequency (Hinde and Kilner, 2007; Baldan et al., 2019) but reduce prey selectivity in enlarged broods (Wright et al., 1998; García-Navas and Sanz, 2010; Mathot et al., 2017). Another cause of nutritional stress could be an increase in nestling begging costs in enlarged broods (Neuenschwander et al., 2003) due to increased social stress and competition. Brood size most prominently modifies offspring growth (Tinbergen and Boerlijst, 1990; Naguib et al., 2004; Nettle et al., 2013) and development (Naguib et al., 2004), in turn affecting fledging age (Naguib et al., 2004) and fledging size/condition (Tinbergen and Boerlijst, 1990; Sanz and Tinbergen, 1999). However, brood size has also an effect on offspring physiology, where larger brood sizes cause changes in energy metabolism (Mertens, 1969), immunocompetence (Brinkhof et al., 1999; Saino et al., 2003; Naguib et al., 2004), testosterone levels (Naguib et al., 2004), the stress response (Naguib et al., 2011) and corticosterone levels (Saino et al., 2003). Ultimately, these changes have consequences for the cognitive ability (Nettle et al., 2015) and the behavior (Carere et al., 2005; Krause et al., 2009) of offspring. However, not much is known about how these early developmental conditions shape development, physiology and behavior of the offspring in a stable manner. Changes in DNA methylation due to these early developmental conditions are a good candidate for explaining parental induced phenotypic plasticity, but only few studies examined early environmental effects on DNA methylation in wild avian populations (Bentz et al., 2016; Rubenstein et al., 2016; Sheldon et al., 2018; Jimeno et al., 2019; Sepers et al., 2019). Only two of these studies made use of a brood size experiment. In a study on captive zebra finches (Taeniopygia guttata) individuals reared in large broods showed higher DNA methylation of the glucocorticoid receptor gene (Nr3c1) compared to individuals raised in small broods (Jimeno et al., 2019). Since only one candidate gene was targeted, it remains to be elucidated if more genes are affected. In another study on the same species, however, no difference in DNA methylation was detected when comparing experimentally reduced and enlarged broods using MS-AFLP (Sheldon et al., 2018). The chosen method (MS-AFLP; Reyna-López et al., 1997) has some drawbacks, it for example only screens anonymous loci and since no annotation is possible, no clear expectations can be formulated (Schrey et al., 2013). In the study of Sheldon et al. (2018), only the enlarged and reduced broods were manipulated, leaving the control broods unmanipulated. Manipulated individuals showed more hypomethylation compared to unmanipulated individuals, suggesting an effect of manipulation on DNA methylation (Sheldon et al., 2018). Thus, early developmental conditions might induce changes in nestling DNA methylation via post-natal effects such as brood size, however, to what extend early life conditions causally affect DNA methylation in functionally relevant genes is largely unknown.
Here, we experimentally manipulated brood size and assessed its effect on DNA methylation in a wild songbird species, the great tit (Parus major). The great tit has been a model system for ecological and evolutionary studies, with long-term studies in both wild and captive populations (Laine et al., 2016; Bosse et al., 2017; Spurgin et al., 2019). We cross-fostered 3-day old nestlings between pairs of matched broods creating enlarged broods with three nestlings extra and reduced broods with three nestlings less. In the two broods within a control pair, the original brood size of both broods remained the same, but half of the nest was cross-fostered. This classical approach has been shown to be an effective way to affect offspring behavior, physiology and body size (Sanz and Tinbergen, 1999; Neuenschwander et al., 2003; van Oers et al., 2015) and allows us to disentangle prehatching from rearing effects (Sepers et al., 2019). In birds, like most vertebrates, almost all methylation occurs at CpG sites (Derks et al., 2016; Laine et al., 2016). DNA methylation variation in the great tit has been associated with phenotypic traits such as exploratory behavior (Riyahi et al., 2015; Verhulst et al., 2016) and the onset of reproduction (Viitaniemi et al., 2019; Lindner et al., 2021a). Low levels of CpG promoter region methylation, and more specifically of sites in the transcription start site (TSS), are associated with increased gene expression in the great tit (Laine et al., 2016). We therefore used Reduced Representation Bisulfite Sequencing (RRBS) to compare CpG site-specific DNA methylation levels between siblings from enlarged and reduced broods, and between siblings from control broods. Furthermore, we used the existing annotation of the great tit reference genome to assess the functional importance of differentially methylated sites.
Materials and Methods
Subjects, Study Site, and General Procedures
This study was conducted in April, May and June 2016 in the Boslust study population, near Arnhem, Netherlands (5°850 E, 52°010 N), a 70 ha field site consisting of mixed pine-deciduous woodlands and grassy meadows. The study site contained about 150 nest boxes, which were predominantly used by great tits. From the first week of April onward, we checked nest boxes weekly to determine initiation of breeding activity. We inspected occupied nest boxes every other day to determine the date of first egg-laying, clutch size and start of incubation, allowing us to estimate hatch dates. By visiting nests daily around the expected hatch date, we determined the exact date at which the majority of the eggs within a clutch hatched (hereafter: hatch date).
Cross-Fostering and Brood Size Manipulation
Clutches with the same hatching date (D0) and similar brood sizes were assigned to a cross-foster pair (N = 30 broods; 15 cross-foster pairs). A cross-foster pair was randomly assigned to become either a control pair or a treatment pair, independently of original brood size. When nestlings were three days old (D3), a partial cross-foster design was employed. We used the method according to van Oers et al. (2015) for cross-fostering. For this, nestlings within broods were ranked based on their weight (using a digital scale, ±0.01 g) and then randomly either the even or the odd ranked nestlings were swapped between the two broods (Supplementary Figure 1). In this way, differences in weight between cross-fostered nestlings and nestlings that stayed in the brood of origin were minimized (van Oers et al., 2015). For control pairs (twelve control broods; six cross-foster pairs), half of the nestlings were swapped (cross-fostered) between the two broods, while the other half stayed in the brood of origin (unmoved), without changing the original brood size. For treatment pairs (nine reduced and nine enlarged broods; nine cross-foster pairs), one brood received three nestlings more than the original brood size (+3, enlarged) and the other brood received three nestlings less than the original brood size (−3, reduced) (Supplementary Figure 1). We aimed for similar numbers of unmoved compared to cross-fostered nestlings in a brood and minimal weight differences between unmoved and cross-fostered siblings.
To be able to identify individuals and their brood of origin, the down tufts on the head, wings and back of the nestlings were selectively plucked right before weighing and cross-fostering (van Oers et al., 2015). This enabled us to identify the nestlings up until day six, the day at which nestlings were fitted with uniquely numbered aluminum bands (Vogeltrekstation, Netherlands).
Fourteen days after hatching (D14), a blood sample (approximately 10 μL) was taken from the brachial vein and stored in Eppendorf tubes containing one ml cell lysis buffer. The tubes were stored at the NIOO-KNAW at room temperature until further analysis. Since some broods were deserted or nestlings were missing or found dead, we were able to take blood samples from 153 nestlings from 25 broods. Of these 25 broods nine were control broods (three complete control pairs and three single control broods) and seven were enlarged broods and nine were reduced broods (seven complete treatment pairs and two single treatment broods).
Sample Selection and Processing
From the seven treatment pairs (reduced and enlarged), matched samples from two biological siblings (N = 14; seven reduced and seven enlarged) were chosen for further analysis. To disentangle treatment effects on DNA methylation from biological variation in DNA methylation between the enlarged and reduced pool, we decided to compare two control pools as well. From the three control pairs, four samples (N = 12) per cross-foster pair were chosen for further analysis (biological sibling pairs being raised in different control broods). Since siblings are more similar to each other in their methylation profile than to nestlings from other broods (Viitaniemi et al., 2019; van Oers et al., 2020), this approach allowed us to control for prehatching differences in DNA methylation by only comparing DNA methylation levels between siblings raised in enlarged and reduced broods and between siblings raised in control broods. This approach resulted in a total sample size of 26 samples from 26 individuals (Supplementary Table 1).
Reduced Representation Bisulfite Sequencing Library Preparation and Sequencing
For DNA isolation, red blood cells were separated from the plasma by spinning the samples in a centrifuge at 14,000 rpm for twelve minutes Subsequently, the plasma was removed using Hamilton syringes (Merck KGaA) and the DNA was extracted from the red blood cells using FavorPrepT M 95-well Genomic DNA Kit. After DNA extraction, the DNA concentration of each individual sample was checked on a NanoDrop 2000 spectrophotometer (Thermo Scientific, Waltham, MA, United States). If needed, a sample was diluted to equalize the concentrations of all the samples. In addition, DNA quality was checked on agarose gels. Subsequently, the samples were pooled per treatment, resulting in a Reduced pool and an Enlarged pool (both consisting of seven individuals), and a Control1 pool and a Control2 pool (both consisting of six individuals) (Supplementary Table 1). The samples were pooled based on concentration, in order to minimize variation in the amount of starting DNA between individuals in one pool. Earlier research showed that pooling individuals is a reliable way to assess average group DNA methylation (Docherty et al., 2009). Within one pool all samples were, to our knowledge, unrelated. DNA isolation was finalized within one day to prevent batch effects between pools. We assessed genome-wide DNA methylation levels using Reduced Representation Bisulfite Sequencing (RRBS; Meissner, 2005). RRBS was done as mentioned in Derks et al. (2016). The genomic DNA was digested the with the enzyme Msp1. This enzyme cuts the genomic DNA at CCGG motif sites. The restriction fragments were size selected to a range of 20–200 bp, by cutting from gel after preparative gel electrophoresis. Secondly, the fragmented DNA was treated with the chemical sodium bisulfite, which turns unmethylated cytosines (C’s) into uracils (U’s) which will later be read as thymines (T’s). Bisulfite-PCR amplification was conducted using PfuTurboCx Hotstart DNA polymerase (Stratagene) and 18 PCR cycles. The final amplified Enlarged and Reduced pools were sequenced in 2016 on an Illumina HiSeq 2500 (100 bp from single end reads), and the final amplified control pools were sequenced in 2018 on an Illumina HiSeq 4000 (100 bp from single end reads). Bisulfite sequencing was done by the Roy J. Carver Biotechnology Centre (University of Illinois at Urbana-Champaign, United States).
DNA Methylation Analysis
Quality Control and Trimming
Raw reads from the RRBS data were quality checked and checked for adapter content using FastQC v0.11.8 (Andrews, 2010). FastQ screen v0.11.1 (Wingett and Andrews, 2018) in bisulfite mode was used to detect possible contaminations with pre-existing databases and indexed genomes. The databases and genomes were Phix (Coliphage phi-X174), vectors (UniVec Core), Arabidopsis thaliana (A. thaliana (thale cress), TAIR10), Escherichia coli (E. coli str. K-12 substr. MG1655) and Homo sapiens (Genome Reference Consortium Human Build 38). After assessment of the quality checks, measures were taken to improve the quality of the reads. For this, the reads were trimmed for quality (≥20 PHRED quality score), length (≥20 bp for the control pools and ≥36 bp for the treatment pools because of high per base N content) and adapter sequences using Trim Galore v0.4.1 (Krueger, 2012) with the –rrbs option. The results were summarized using Multiqc 1.7 (Ewels et al., 2016). Quality improvement of the reads was verified by FastQC, FastQ screen and Multiqc. Raw reads were submitted to NCBI under the BioProject PRJNA208335 with accession numbers SRR11078101 (Enlarged pool), SRR11078100 (Reduced pool), SRR11078099 (Control1) and SRR11078098 (Control2).
Alignment and Methylation Calling
Trimmed reads were aligned to the Parus major reference genome v1.11 (Laine et al., 2016) using BS-Seeker22 v2.0.6 (Guo et al., 2013) with Bowtie23 v2.1.0 (Langmead et al., 2009) using the end-to-end alignment mode. Of the aligned reads, the methylation levels for each site were determined by dividing methylated C’s of a site by the total coverage of that site (C/C + T) which was done with the methylation call script bs_seeker2-call_methylation.py from BS-Seeker2.
Filtering of Methylation Calls
Before the data was filtered it was transformed to fit the format of methylKit. This was done using a custom bash script and only for CpG sites. Filtering was done with R version 3.6.1 and the R package methylKit4 v1.15.3 (Akalin et al., 2012). First, a principle component analysis (PCA) was conducted on all CpG sites that were present in all pools to check clustering of the four pools. The default settings of the PCASamples function in methylKit were used, which means that the percent methylation matrix was transposed (this is equivalent to doing PCA on variables that are sites) and that sites with low variation in DNA methylation or low coverage (<10) were discarded prior to the PCA. To test whether average CpG methylation percentage differed significantly between the four pools, a one-way analysis of variance (ANOVA) and subsequent Tukey post hoc analyses were conducted. Next, sites that were not present in both pools (both treatment pools or both control pools), sites with low coverage (<10) and sites with methylation levels of 0 or 100% in all pools were excluded. The treatment pools were sequenced deeper (i.e., higher coverage) than the control pools and to avoid a PCR bias in the statistical tests, we applied percentile filtering (99.9) and the coverage of the samples was normalized. After filtering of the control pools, 213,764 out of the 247,979 CpG sites that were present in both control pools could be used for further analysis. After filtering of the treatment pools, 235,618 out of the 252,698 CpG sites that were present in both treatment pools could be used for further analysis. Correlation matrixes were made to check for abnormalities.
Statistical Analysis
Differentially methylated sites (DMS) between Reduced and Enlarged and between Control1 and Control2 were also assessed using the R package methylKit v1.15.3 and R version 3.6.1 (Akalin et al., 2012). MethylKit reads the data and creates a data frame where it calculates the percentage of methylated C’s at a given site from the methylation ratio created by BSSeeker2. Complementary CpG dinucleotides were not merged. Next, differential methylation per site was assessed by comparing the fraction of methylated C’s between two pools using a Fisher’s exact test, since there was only one pool per group for both comparisons. To minimize the chance of getting false positives, we decided to use a stringent threshold of 25% instead of 10% differential methylation. We used a Bonferroni corrected α-threshold [−log10(0.05/213,764) = 6.63 for Control1 vs. Control2 and −log10(0.05/235,618) = 6.67 for Reduced vs. Enlarged] for a site to be considered a DMS.
Gene Annotation
DMS were annotated using the Parus major reference genome build v1.15, annotation version 1026, custom R scripts and R packages GenomicFeatures v1.30.0 (Lawrence et al., 2013) and rtracklayer v1.42.2 (Lawrence et al., 2009). Genomic regions were TSS, promoter, intron, exon, five prime untranslated region (5’UTR), three prime untranslated region (3’UTR), upstream and downstream. TSS regions were defined as 300 bp upstream to 50 bp downstream of the annotated transcription starting position of each gene (Laine et al., 2016; Viitaniemi et al., 2019). Since TSS regions overlap with promoter regions, DMS associated to a TSS region were also associated to a promoter region. In such cases, only the TSS region was reported. We defined the TSS region as in Laine et al., 2016 since in this study, low levels of CpG methylation in specifically this region were associated with increased gene expression in the great tit. Promoter regions were defined as 2000 bp upstream to 200 bp downstream of the annotated gene start site (Lindner et al., 2021a). Upstream and downstream regions were defined as 10 K bp up- and downstream regions adjacent to the gene body, respectively (Laine et al., 2016; Lindner et al., 2021b). Since DNA methylation is reciprocal on both strands, annotation was not directional (i.e., each DMS could overlap with the Watson and Crick strands). Please note that one site can be associated to multiple genes or regions, because genes can be located on opposite strands, regions within a gene can have overlapping regions depending on the transcript and genes can have opposite transcription directions. If this was the case, we checked the site in NCBI Genome Data Viewer and prioritized DMS in TSS and promoter regions, because CpG methylation within the regulatory region is known to affect gene expression in great tits (Laine et al., 2016).
Gene Ontology Analysis
The function and significance of the genes that were associated to a DMS were investigated by looking up GO terms and descriptions of the genes of chicken at uniprot7, NCBI8, Ensembl9 and genecards10. We focused on molecular functions and biological processes. If there were no GO terms and descriptions of chicken (Gallus gallus) genes available, we used zebra finch (T. guttata) or human genes (Homo sapiens). Uncharacterized LOC genes were checked using NCBI and Ensembl. A LOC gene was included if its biological function could be predicted and was excluded if it was likely to be a duplication of (part of) the gene it was predicted to be, ncRNA or truly uncharacterized. We focused on DMS within regulatory regions (promoter and TSS regions) of genes and DMS that occurred in the same gene. Information about other DMS can be found in Supplementary Tables 4–9.
Additionally, GOrilla was used to identify enriched GO terms (Eden et al., 2009). Since there was not enough power to provide both a background and a target list, all genes in which a CpG site was found, so all genes that were covered by both pools in one comparison, were given as input. This was done for the Enlarged pool versus the Reduced pool comparison and the Control1 pool versus Control2 pool comparison separately. The genes were ranked according to how well the associated CpG site differentiated between the two pools that were compared using the p-value from the Fisher’s exact test as described above. LOC genes were excluded by Gorilla. GOrilla was run with default running parameters (species used: H. sapiens; single ranked list of genes, p-value <0.001, GO database last updated on December 12, 2020). The FDR method was used to correct enrichment tests for multiple testing of the GO terms. GO categories were considered significantly enriched if the FDR corrected p-value was <0.05.
All scripts used for the bioinformatics and biostatistics steps can be found in the Supplementary Material.
Results
General
The percentage of fully bisulfite converted reads was >99.99% in all pools (Supplementary Table 2). A Principle Component Analysis (PCA) revealed that 51.95% was explained by PC1, 39.07% by PC2, 8.98% by PC3 and 2.20 × 10–29% by PC4 (Supplementary Figure 2). The Reduced pool and the Enlarged pool cluster together very closely along both PC1 and PC2 (Supplementary Figure 3). The two control pools are relatively close to one another along PC2 but vary along PC1.
Average CpG methylation percentage differed significantly between the four pools (one-way ANOVA: F3,836192 = 96.8, p < 0.02 × 10–14). A Tukey post hoc test showed that all pools differed significantly in average CpG methylation percentage: the methylation percentage was significantly lower in the Reduced pool compared to the Enlarged pool (mean ± SE; Reduced: 27.48 ± 0.0007; Enlarged: 27.76 ± 0.0007; p = 0.04). The methylation percentage was significantly higher in Control1 compared to Control2 (Control1: 29.13 ± 0.0008; Control2: 28.41 ± 0.0007; p = 0.07 × 10–9). The methylation percentages in the treatment pools were significantly lower compared to the control pools (all p ≤ 0.04 × 10–7).
An overview of the number of reads and CpG sites before and after filtering and the mapping and calling success is given in Supplementary Table 3. 235,618 CpG sites were present in both treatment pools and 213,764 CpG sites were present in both control pools (after filtering). 209,049 CpG sites were shared by all four pools (Supplementary Figure 4).
Of the 213,764 CpG sites that were present in both control pools, 17 sites were significantly differentially methylated (Figure 1A), of which 14 were located on autosomes, two on the sex chromosome (chrZ) and one on a scaffold (Figure 2A). Of the 17 DMS, 14 sites could be annotated. Of these 14 DMS, four were located in a promoter region, of which two were located in a TSS region. Furthermore, one DMS was located in an exonic region, five in an intronic region, two in a downstream region, one in an upstream region and one DMS in both an upstream and downstream region (Supplementary Table 4). The 14 DMS were located in or nearby 15 genes (Supplementary Table 5).
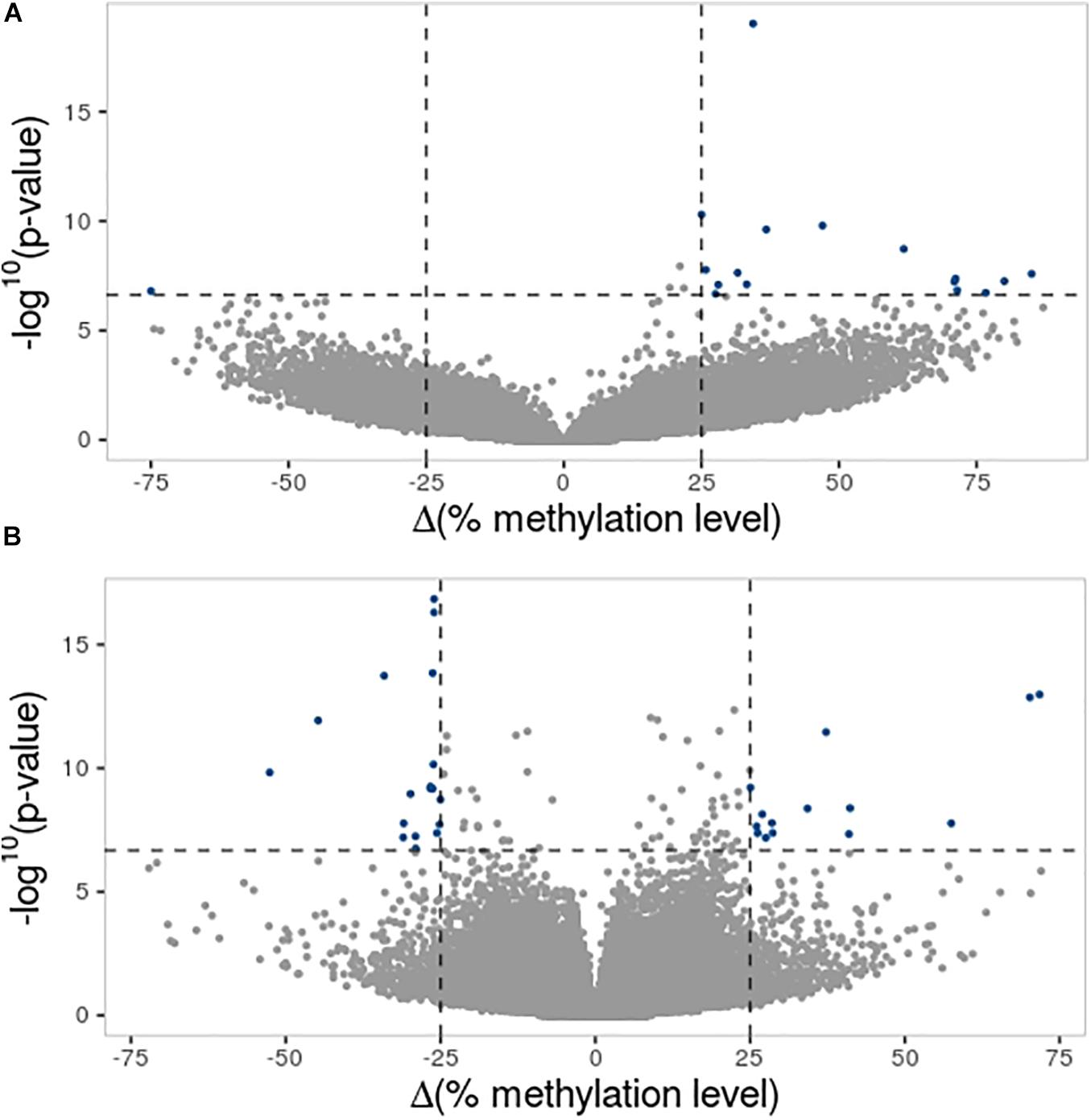
Figure 1. Volcano plot of p-values (in −log10 scale) corresponding to the significance of the variation in DNA methylation between two pools. Each dot represents a CpG site tested for differential methylation. Dark blue dots represent CpG sites that differ significantly between the pools with a minimal difference of 25% differential methylation. Dotted horizontal lines marks the genome wide significance threshold. Dotted vertical lines represent 25% differential methylation. (A) Control1 versus Control2, (n = 213,764). (B) Reduced versus Enlarged, (n = 235,618).
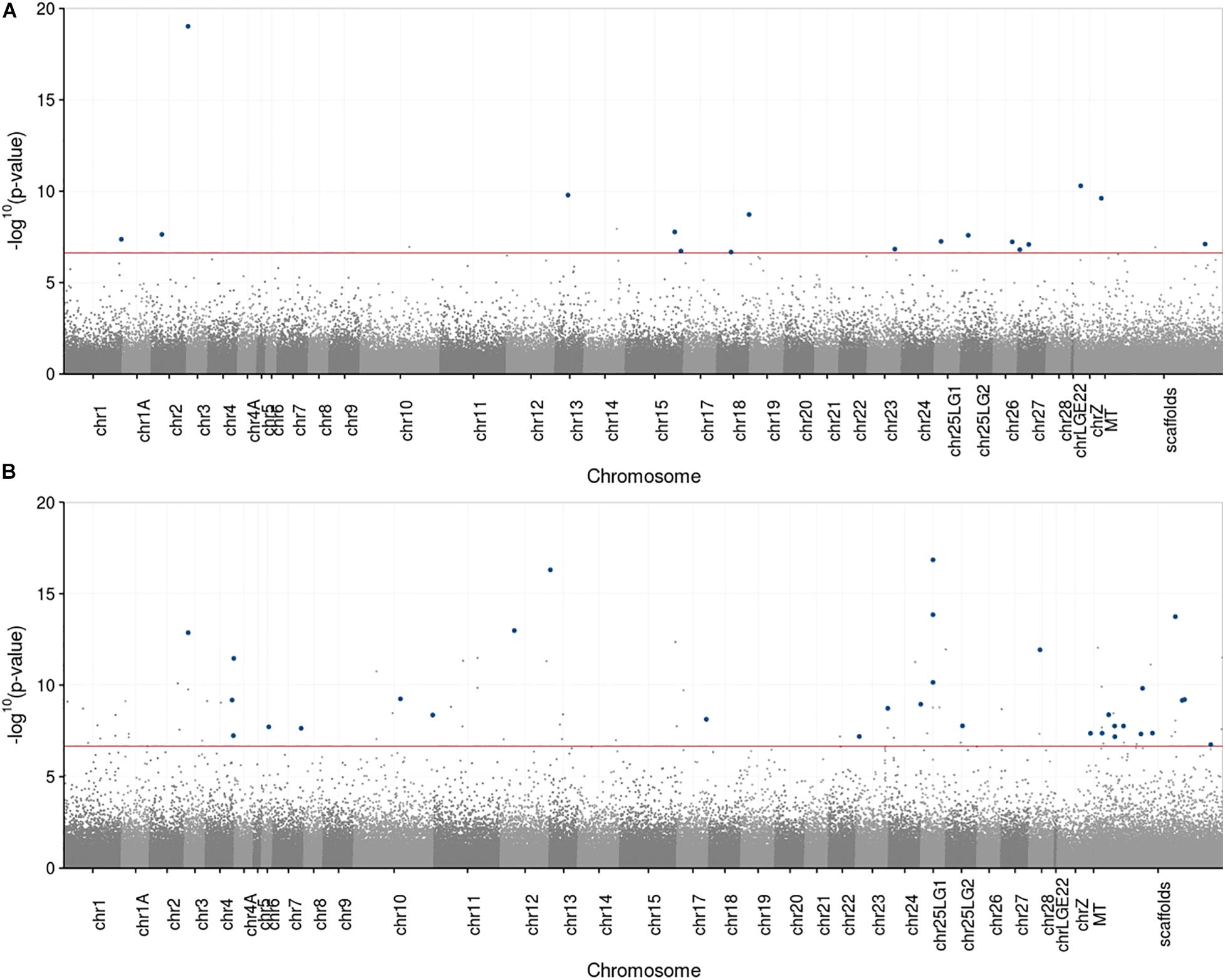
Figure 2. Manhattan plot of p-values (in −log10 scale) corresponding to the significance of the variation in DNA methylation between the pools. Each dot represents a CpG site tested for differential methylation. Dark blue dots represent CpG sites that differ significantly between the pools with a minimal difference of 25% differential methylation. The dotted red line marks the genome wide significance threshold. The sites are plotted against the location of the associated site within the genome. Alternating colors help to differentiate adjacently displayed chromosomes. ChrZ is a sex chromosome, all the other chromosomes are autosomes. All unplaced scaffolds are merged into the category “scaffolds”. (A) Control1 versus Control2, (n = 213,764). (B) Reduced versus Enlarged, (n = 235,618).
Of the 235,618 CpG sites that were present in both treatment pools, 32 sites were significantly differentially methylated (Figure 1B), of which 20 were located on autosomes, one on the sex chromosome and 12 on scaffolds (Figure 2B). Of the 32 DMS, 23 could be annotated. Of these 23 DMS, nine were located in a promoter region, of which three in a TSS region. Furthermore, three DMS were located in an exonic regions, eight DMS were located in an intronic region, two in a downstream region and one in an upstream region (Supplementary Table 4). The 23 DMS were located in or nearby 21 genes (Supplementary Table 6).
None of the 17 DMS between Control1 pool and Control2 pool were significantly differentially methylated between the Reduced pool and the Enlarged pool (Supplementary Figure 5 and Supplementary Table 7). Out of the 17 DMS, 11 were covered in the Reduced pool and the Enlarged pool. None of the 32 DMS between the Reduced pool and the Enlarged pool were significantly differentially methylated between Control1 pool and Control2 pool (Supplementary Figure 5 and Supplementary Table 7). Out of the 32 DMS, 26 were covered in both Control pools.
Differentially Methylated Sites in Genes and in Regulatory Regions
Control Pools
When comparing the two control pools, none of the DMS occurred within the same genes. All of these DMS were hypermethylated, which translates to a higher methylation percentage in Control1 compared to Control2. We found four DMS to be situated in regulatory regions (Table 1). These DMS were located in the promoter region of heat shock protein family A member 2 (HSPA2), in the promoter region of methyltransferase like 8 (METTL8), around the TSS region of the predicted gene zinc finger SWIM domain-containing protein 1 (ZSWIM1-like; LOC107213098) and around the TSS region of the predicted gene oncostatin-M-specific receptor subunit beta-like (OSMR-like; LOC107198170).
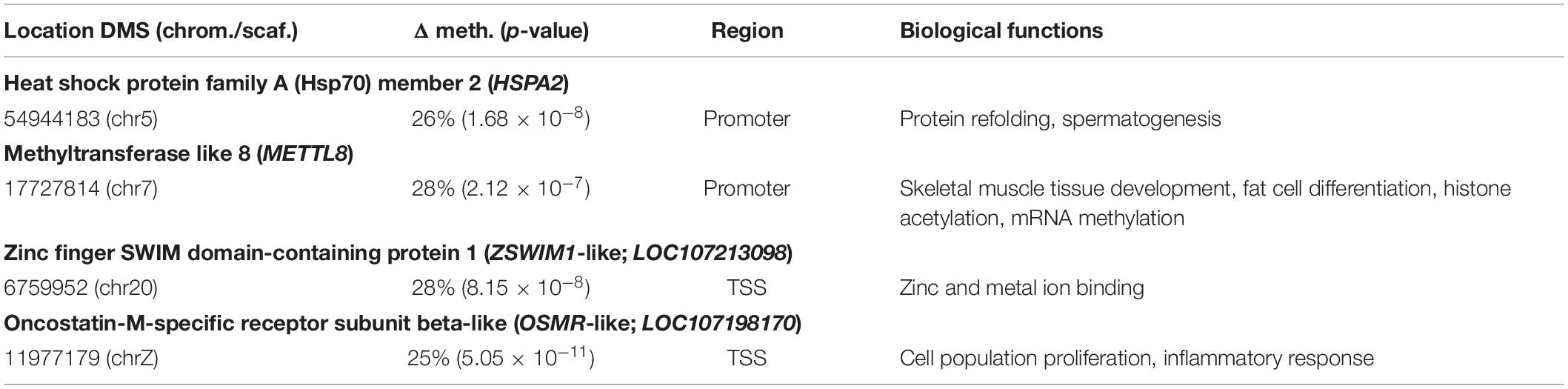
Table 1. Full name, gene name, location, chromosome number or unplaced scaffold, Δ of % methylation level, p-value, region and biological functions associated with DMS between Control1 pool and Control2 pool.
Out of all 15,603 covered genes, 11,182 were recognized by GOrilla. We detected 98 enriched GO terms for the ontology biological process, 28 for the molecular functions and 16 for cellular component (p-value <0.001). After FDR correction, 47 of the biological function GO terms were significantly enriched, 19 of the molecular function and eight of the cellular component (FDR q-value <0.05) (Supplementary Table 10). The most significant GO terms were anatomical structure development (GO:0048856, FDR = 1.95 × 10–8), developmental process (GO:0032502, FDR = 7.28 × 10–6) and system development (GO:0048731, FDR = 5.09 × 10–5). Of all genes (excluding the LOC-genes) in which a DMS was found, only HSPA2 was associated with the significantly enriched GO terms.
Reduced Versus Enlarged
In the treatment comparison, three DMS were found within the same gene (Table 2). These three DMS were hypomethylated, which translated to a lower methylation percentage in the Enlarged pool than in the Reduced pool. Of these three DMS, one was situated in the exonic region and two in the intronic region of laminin subunit gamma 3 (LAMC3). Nine DMS were situated in the regulatory regions of genes and none of these DMS occurred in the same gene (Table 2). Of these nine DMS, five were hypermethylated, which translated to a higher methylation percentage in the Enlarged pool than in the Reduced pool. These DMS were situated around the TSS region of tissue specific transplantation antigen P35B (TSTA3), around the TSS region of the predicted gene ketosamine-3-kinase-like (FN3KRP-like; LOC107198385), in the promoter region of prominin 2 (PROM2), around the TSS region of zinc finger protein 664-like (ZNF664-like; LOC107199222) and in the promoter region of plectin-like (PLEC-like, LOC107199333). The four remaining DMS in regulatory regions of genes were hypomethylated, which translated to a lower methylation percentage in the Enlarged pool than in the Reduced pool. These DMS were situated in the promoter region of complement C1q subcomponent subunit C-like (C1QC-like; LOC107213704), in the promoter region of the gene activating transcription factor 6 (ATF6), in the promoter region of prolactin regulatory element binding (PREB) and in the promoter region of WD repeat domain 83 opposite strand (WDR83OS).
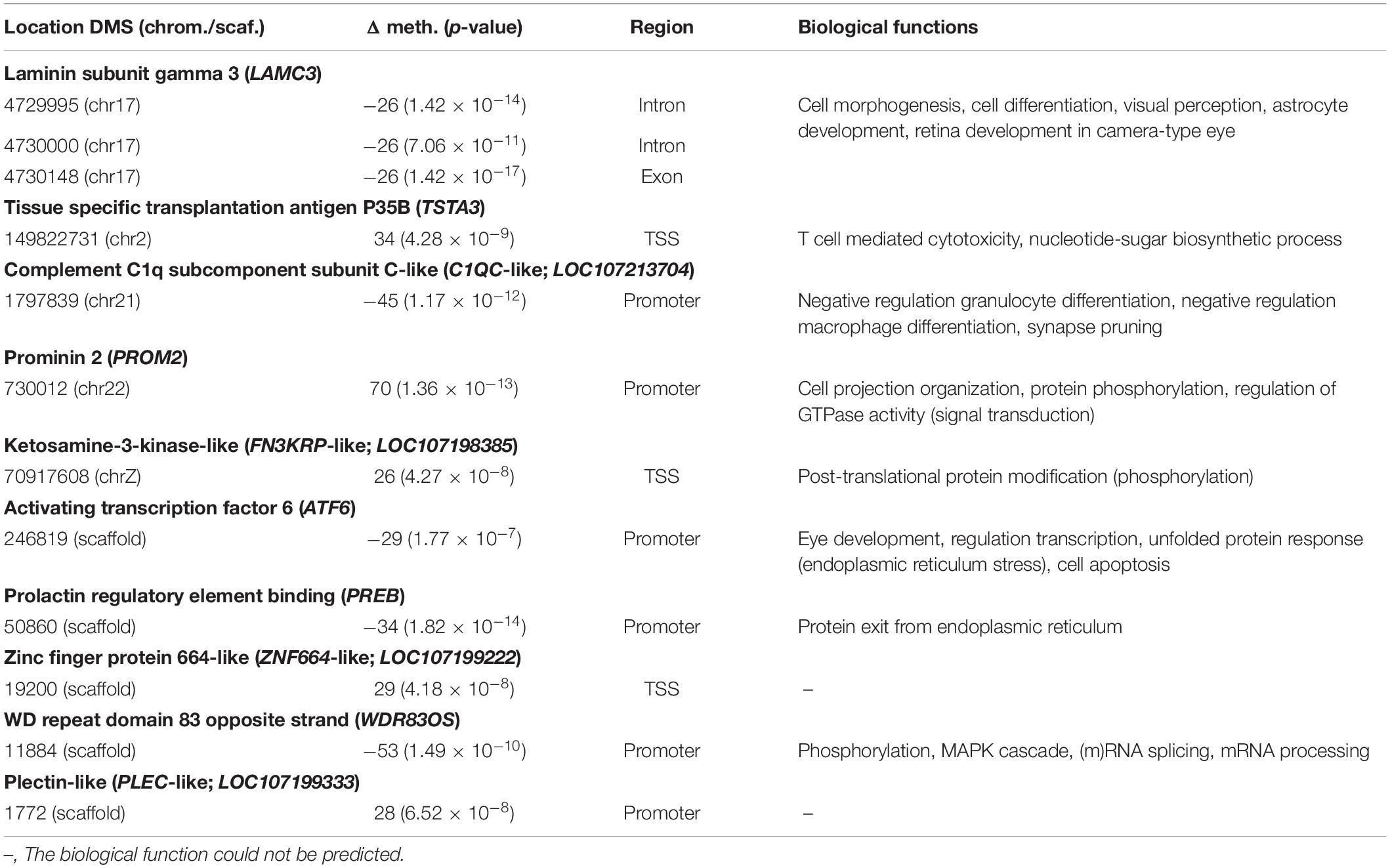
Table 2. Full name, gene name, location, chromosome number or unplaced scaffold, Δ of % methylation level, p-value, region and biological functions associated with DMS between the Reduced pool and the Enlarged pool.
Out of all 15,718 covered genes, 11,210 were recognized by GOrilla. We detected 82 enriched GO terms for the ontology biological process, 23 for the molecular functions and seven for cellular component (p-value <0.001). After FDR correction, 42 of the biological function GO terms were significantly enriched, eleven of the molecular function and one of the cellular component (FDR q-value <0.05) (Supplementary Table 11). The most significant GO terms were developmental process (GO:0032502, FDR = 1.64 × 10–5), regulation of multicellular organismal process (GO:0051239, FDR = 6.72 × 10–5), anatomical structure morphogenesis (GO:0009653, FDR = 4,71 × 10–5), anatomical structure development (GO:0048856, FRD = 1.09 × 10–4) and regulation of cell differentiation (GO:0045595, FDR = 9.59 × 10–5). All genes (excluding the LOC-genes) in which a DMS was found were associated with the significantly enriched GO terms.
The results that were obtained with a less stringent threshold of 10% differential methylation are reported in Supplementary Tables 12–16.
Discussion
The mechanisms underlying intergenerational regulation of developmental phenotypic plasticity in birds remain largely unknown, but recent studies indicate a role for DNA methylation (Bentz et al., 2016; Sheldon et al., 2018). Here, we explored this further by experimentally manipulating brood size in a partial cross-foster experiment and assessing the effect of experimental brood size on DNA methylation in a wild songbird species, the great tit. We found more CpG sites in red blood cells to be differentially methylated between biological sibling-pairs raised in experimentally enlarged and reduced broods, than between siblings raised in partially cross-fostered control broods with unchanged brood size. Since differential DNA methylation is more apparent between nestlings from enlarged and reduced broods than between nestlings from control broods, this indicates that experimental variation in brood size affects DNA methylation. Furthermore, we found for the enlarged versus reduced comparison more differentially methylated CpG sites to be situated in regulatory regions (promoter and TSS regions) than for the control brood comparison. Since CpG methylation within the regulatory region is known to affect gene expression in great tits (Laine et al., 2016), we expect more functional differences in gene expression between nestlings from enlarged and reduced broods than between nestlings from control broods.
The average CpG methylation percentage differed between pools of nestlings from the enlarged and the reduced broods and between the control broods. Nestlings from experimentally enlarged and reduced broods were hypomethylated compared to nestlings from control broods. This indicates that any manipulation of brood size affected CpG methylation. A similar result was found in the study of Sheldon et al. (2018). Here, zebra finch nestlings from reduced and enlarged broods showed more hypomethylation compared to control nestlings. However, in this study, the control broods were completely unmanipulated, whereas in our study nestlings from control broods were also cross-fostered. Moreover, we found that nestlings from experimentally enlarged broods were hypermethylated compared to nestlings from reduced broods. This result supports the hypermethylation of Nr3c1 in zebra finches reared in large broods (Jimeno et al., 2019) and the positive correlation between natal brood size and the percentage of DNA methylation in Sheldon et al. (2018). However, this result does not match the lack of difference in methylation between nestlings from experimentally reduced and enlarged broods (Sheldon et al., 2018). This might be caused by the targeted approach we used in this study, compared to the MS-AFLP approach. In spite of our targeted approach, slight differences in methylation remain undetected when average DNA methylation levels are compared. Since DNA methylation is very gene- and region specific, it is important to assess site specific differences in possibly functionally relevant genes as well. Furthermore, the difference in methylation percentage between nestlings from reduced and enlarged broods was only 0.28% and the difference between nestlings that experienced a manipulation in brood size and nestlings from control broods ranged from 0.65 to 1.65%, also minimal differences. It has to be elucidated if such small differences are large enough to result in differential gene expression. Hence, it is unknown if such small differences hold any biological significance or are just caused by a statistical artifact.
As mentioned above, the biological functions of the genes and the possible consequences of methylation for gene expression will be discussed below. Since low levels of CpG promoter region methylation, and more specifically of sites in the TSS region, are associated with increased gene expression in the great tit (Laine et al., 2016), hypermethylated DMS are expected to be associated with lower gene expression and hypomethylated DMS are expected to be associated with higher gene expression. The limitations of such a generalization will also be discussed.
Sites that were differentially methylated in the enlarged versus reduced comparison, were mainly found to be related to development, metabolism and behavior and cognition. This involved sites in the genes LAMC3, PREB, PROM2, TSTA3, ATF6, FN3KRP-like and WDR83OS. The biological functions of ZNF664-like and PLEC-like could not be predicted because these LOC genes were likely to be a duplication of (part of) the genes they were predicted to be or ncRNA. In the gene LAMC3, three different DMS were found, although none of them occurred in the regulatory region of the gene, indicating that we have no proof for a possible change in gene expression in the great tit (Laine et al., 2016). The DNA methylation levels in all three sites were higher in the reduced pool in comparison with the enlarged pool, cautiously indicating lower expression of LAMC3 in nestlings from reduced broods. Since LAMC3 expression is relatively high during human development (Barak et al., 2011) and low to moderate in adulthood (Hawrylycz et al., 2012; Zeng et al., 2012), this might mean that the nestlings from the reduced broods were further developed than the nestlings from the enlarged broods, which is in the expected direction based on previously found effects of brood size and food availability on development (Nettle et al., 2013) and condition (Tinbergen and Boerlijst, 1990; DeKogel, 1997; Sanz and Tinbergen, 1999). In addition, we found one DMS in the genes PREB, PROM2, TSTA3, ATF6, FN3KRP-like and WDR83OS. The DMS in both PROM2 and TSTA3 were hypermethylated in the enlarged pool compared to the reduced pool, suggesting lower gene expression in nestling from the enlarged broods. In the case of PROM2, this might indicate lower levels of cholesterol (Singh et al., 2013) in the nestlings from enlarged broods. In the case of TSTA3, this might indicate a lower growth potential (Willson et al., 2018) of nestlings in the enlarged broods and an effect on (bone) metabolism (Johnsson et al., 2015). The DMS in PREB was hypomethylated in the enlarged group, suggesting higher expression of these genes in nestlings from the enlarged group. Higher PREB expression might lead to lower prolactin (PRL) expression (Hiyama et al., 2015), which might indicate later sexual maturity and lower body weights (Bhattacharya et al., 2011) in nestlings from the enlarged broods. Overall, the DMS in PREB, PROM2 and TSTA3 indicate that the nestlings from the enlarged broods weighed less, developed slower and adjusted their metabolism. These effects are in the expected direction based on previously found effects of brood size on nestling condition (DeKogel, 1997; Sanz and Tinbergen, 1999), weight (Tinbergen and Boerlijst, 1990; DeKogel, 1997) and resting metabolic rate (Verhulst et al., 2006).
The genes ATF6, PREB, FN3KRP-like and WDR83OS suggest an effect of brood size on insulin-glucose metabolism specifically. The DMS in PREB, ATF6 and WDR83OS were hypomethylated in the enlarged pool compared to the reduced pool, suggesting higher gene expression in nestlings from the enlarged broods. This indicates in the case of ATF6 glucose intolerance (Barbosa et al., 2016), in the case of WDR83OS increased levels of insulin (Kesherwani et al., 2017) and in the case of PREB higher insulin sensitivity (Park et al., 2018) in nestlings from the enlarged broods. The function of FN3KRP-like function is not fully understood (Szwergold et al., 2011), although the hypermethylated DMS in the enlarged pool suggests lower gene expression in nestlings from the enlarged broods, which indicates differences in glucose metabolism (Sajuthi et al., 2016) between nestlings from the reduced and enlarged broods. The effects are in the expected direction based on previously found effects of brood size on energy metabolism (Mertens, 1969) and the results indicate food scarcity in the enlarged broods. The specific effect of food scarcity on insulin-glucose metabolism has to be elucidated yet, because the effect is dependent on the developmental stage of an individual (Gardner et al., 2005; Tobi et al., 2009). Nevertheless, insulin-glucose metabolism might be a way of dealing with nutritional constraint in the enlarged broods, allowing for growth under poor food conditions (Gardner et al., 2004, 2005). Similar results have been found before. For example, women exposed to the Dutch famine during gestation gave birth to individuals with lower glucose tolerance during adulthood, probably caused by impaired insulin secretion (de Rooij et al., 2006). This is thought to be the result of fetal adaptations to scarcity i.e., the thrifty phenotype (Hales and Barker, 1992), which becomes maladaptive when an individual is exposed to an abundance of food later in life (Stanner and Yudkin, 2001; Schulz, 2010). In humans prenatally exposed to famine, but exposed to an abundance of food later in life, this has led to higher rates of obesity (Roseboom et al., 2006) and diabetes (Kahn et al., 2009). Furthermore, differentially methylated regions were found in whole blood when compared to their siblings and these regions were associated to prenatal malnutrition, early development, metabolism and growth (Tobi et al., 2009, 2014). Thus, the consequences of early life conditions might be mediated by adjusting metabolic efficiency and DNA methylation might be one of the mechanisms behind this.
One gene, C1QC, is a regulator of the immune response and synapse development and was previously associated to cognition and behavior. C1QC expression has been associated to Alzheimer’s disease and alterations in learning behavior (Khoonsari et al., 2016; Haure-Mirande et al., 2019), ADHD and autism (Corbett et al., 2007; Trent et al., 2014). However, the direction of the effect is not completely understood and might be dependent on the developmental stage (Davies et al., 2009; Trent et al., 2014). The DMS in C1QC-like was hypermethylated in the reduced pool compared to the enlarged pool, suggesting lower C1QC-like expression in nestlings from the reduced broods. Although the role of C1QC in development of Alzheimer’s disease, ADHD and autism is not fully understood yet, this might indicate a difference in brain development, synaptic structure, behavior and cognition between the two treatment groups. This is expected based on previously found effects of food availability, diet quality and brood size on behavior (Carere et al., 2005; Krause et al., 2009; van Oers et al., 2015) and cognition (Nettle et al., 2015) in birds. Food availability is known to affect nestling stress response (van Oers et al., 2015) and exploratory behavior (Carere et al., 2005) and diet quality affects the latency to approach food and feed later in life (Krause et al., 2009). Furthermore, small natal brood size has been associated to slow conditioning to a stimulus and slow reversal learning (Nettle et al., 2015).
The genes and their functions described above are supported by the significantly enriched GO terms developmental process, regulation of multicellular organismal process, anatomical structure morphogenesis, anatomical structure development and regulation of cell differentiation, which all indicate a difference in development between nestlings from enlarged and reduced broods. Although more GO terms were significantly enriched in the comparison of the two control pools and these were similar to those in the comparison of the two treatment pools, more GO terms in the comparison of the treatment pools were highly significantly enriched. This means that more GO terms were highly enriched when comparing reduced with enlarged nestlings than when comparing nestlings from control broods.
Overall, we show that experimental brood size variation leads to more differential DNA methylation in more regulatory regions of genes than when performing a control experiment. This indicates that DNA methylation in response to experimental variation in brood size has the potential to alter gene expression. Most of the genes were functional in tissues other than blood and this may affect how gene expression is related to a trait, due to tissue differentiation. However, multiple studies have shown that gene expression levels in the blood were related to the processes associated with that gene (Roulin et al., 2011; Zhu et al., 2017; Lindner et al., 2021b), suggesting that gene expression levels in blood in our study could explain plasticity in phenotypic traits related to brood size variation. Although these results demonstrate that early life stress affects epigenetic regulation of genes related to brood size, namely genes that are known to affect development, growth, metabolism, behavior and cognition, future work is needed. Further work should assess the causal effects of changes in DNA methylation on gene expression at these loci and related phenotypic traits, and specifically to find repeatable results in similar experiments. Furthermore, it should be assessed whether a single DMS can affect gene expression.
The expectation was that we would not find any differentially methylated sites when comparing pools of control siblings raised in different broods. Still, we found several DMS in the regulatory regions of the genes HSPA2, METTL8, ZSWIM1-like (LOC107213098) and OSMR-like (LOC107198170). These results show that our control pools were not identical in terms of DNA methylation or that these results are false positives. However, we expect the chance of these DMS to be false positives to be very slim because of our stringent approach during data analysis; we applied coverage filtering, a threshold of 25% differential methylation and a Bonferroni corrected α-threshold. Furthermore, we cannot link these differences to our experimental approach. A previous study suggested an effect of manipulation (i.e., being moved to a different nest) on DNA methylation in zebra finches (Sheldon et al., 2018), which was controlled for in the current experimental approach. This means that this cannot explain the DMS and the direction of methylation in the control comparison. One likely explanation may be the existence of large individual variation in DNA methylation (Viitaniemi et al., 2019). By pooling individuals we tried to focus on average group DNA methylation (Docherty et al., 2009) instead of individual variation. However, the number of individuals in our pools might have been too small to completely discard such individual differences. This suggests that some of our DMS in the treatment comparisons might have also been caused by individual differences. Nevertheless, PCA revealed that the reduced pool and the enlarged pool clustered together very closely, which indicates that our experimental setup, matching sibling pairs that were raised in differently sized broods, worked, since these pools were very comparable.
The fact that our control pools were not as similar as thought was supported by the finding that the hyper/hypo distribution between the control broods is non-equally divided with all differentially methylated sites being hypermethylated in Control1. This indicates that by chance some factor that induces DNA methylation in a certain direction was present in one pool. Furthermore, the two control pools did not perfectly cluster together in the PCA plot, unlike the reduced and enlarged pools. This is surprising, since we would expect control pools to cluster in the same way, since siblings were paired there as well. We therefore conclude that the controls pools were not balanced, causing the number of DMS to be higher than if the control pools would have been balanced. One reason could be a biased sex ratio (Natt et al., 2014). However, only one DMS in a regulatory region was situated on the sex chromosome, which was equal to the number found in the treatment comparison. One DMS was found in HSPA2, a gene involved in protein refolding and in spermatogenesis. Given the importance of this gene for male fertility (Dix et al., 1996; Son et al., 1999), this might indicate a difference in expression between the pools, which might be caused by a biased sex ratio in the individuals included in the pools, although this methylation change does not have to be sex dependent. In great tits, visual determination of the sex before the first molt is unreliable, and most birds from this study were not recaptured after the first molt and have therefore not been molecularly sexed, making it impossible to balance the number of males and females in the pools. Since a DMS in HSPA2 might also indicate a bias in brood temperature, because HSPA2 expression is affected by temperature in chicken testes (Wang et al., 2013, 2015), we do not expect our main findings to be affected by a potential sex-bias. Another possible, but unlikely explanation could be that the differences were caused by genetic variation between the pools, since a large fraction of erythrocyte DNA methylation is similar between relatives (Viitaniemi et al., 2019; van Oers et al., 2020). However, since the samples in one pool were, to our knowledge, unrelated and the samples in Control1 originated from siblings of the samples in Control2, we expect the genetic diversity to be larger within than between the pools, although we did not check for extra-pair paternity, which is estimated at about 10% for this population (van Oers et al., 2008). Therefore, it could be that nestlings of one sibling pair were half-siblings, because they were sired by different males. However, since we do not have individual DNA methylation information, we can only speculate on these causes and functional validation of the candidate loci is needed to assess the causal relationship between our experiment and the change in methylation, highlighting the need for studies that assess individual variation in DNA methylation levels. Although, with our design, we cannot disentangle these possible alternative explanations explaining the high number of DMS and the direction of methylation in the control comparison. However, since the two treatment pools were, unlike the two control pools, very similar, we do think that the difference between the number of DMS in the control comparison and the treatment comparison is conservative rather than exaggerated. Therefore, we conclude that most of the DMS in the treatment comparison are likely related to the treatment and the DMS between the control pools may also be caused by some non-explained bias in the control pools.
In conclusion, to our knowledge, this is the first study that investigates the effects of experimentally altered brood size on genome-wide DNA methylation in a wild bird population and that disentangles prehatching from rearing effects with a partial cross-foster design, controls for possible effects of manipulation and assesses the functionality of annotated differentially methylated sites. Our work demonstrates that early life stress due to variation in brood size directly affects epigenetic regulation of genes that are known to affect brood-size dependent phenotypes, such as development, growth, metabolism, behavior and cognition. Although future studies are needed to validate our findings, this study underlines the potential role for DNA methylation in the intergenerational regulation of developmental phenotypic plasticity in altricial birds.
Data Availability Statement
The datasets presented in this study can be found in online repositories. The names of the repository/repositories and accession number(s) can be found below: https://www.ncbi.nlm.nih.gov/biosample/14093678; https://www.ncbi.nlm.nih.gov/biosample/14093679; https://www.ncbi.nlm.nih.gov/biosample/14093680; and https://www.ncbi.nlm.nih.gov/biosample/14093681.
Ethics Statement
The animal study was reviewed and approved by the Institutional Animal Care and Use Committee (NIOO-IvD) and the Koninklijke Nederlandse Akademie van Wetenschappen – Dier Experimenten Commissie (KNAW-DEC licenses NIOO 10.07 and 14.11 to KO).
Author Contributions
BS and KO designed the study and obtained funding. BS conducted the experiment. BS and JE conducted the data analysis with help of FG and VL. BS, JE, and KO drafted the manuscript. KO supervised the study. All authors contributed to editing the manuscript.
Funding
This research was supported by two grants from the Lucie Burgers Foundation for Comparative Behaviour Research, Arnhem, Netherlands to BS and an NWO-ALW open competition grant (ALWOP.314) to KO.
Conflict of Interest
The authors declare that the research was conducted in the absence of any commercial or financial relationships that could be construed as a potential conflict of interest.
Acknowledgments
We gratefully acknowledge Piet de Goede and Lies Zandberg for fieldwork assistance and Christa Mateman for laboratory work. We thank Koen Verhoeven, Melanie Lindner, and Melissah Rowe for helpful discussions and comments on the manuscript. We also thank two reviewers for helpful comments on previous versions of this work. This study was approved by the Institutional Animal Care and Use Committee (NIOO-IvD) and the Koninklijke Nederlandse Akademie van Wetenschappen – Dier Experimenten Commissie (KNAW-DEC licenses NIOO 10.07 and 14.11 to KO).
Supplementary Material
The Supplementary Material for this article can be found online at: https://www.frontiersin.org/articles/10.3389/fevo.2021.609061/full#supplementary-material
Footnotes
- ^ https://www.ncbi.nlm.nih.gov/assembly/GCF_001522545.3
- ^ https://github.com/BSSeeker/BSseeker2
- ^ http://bowtie-bio.sf.net/bowtie2
- ^ https://github.com/al2na/methylKit
- ^ https://www.ncbi.nlm.nih.gov/assembly/GCF_001522545.3
- ^ https://ftp.ncbi.nlm.nih.gov/genomes/all/annotation_releases/9157/102/
- ^ www.uniprot.org
- ^ www.ncbi.nlm.nih.gov
- ^ www.ensembl.org
- ^ http://www.genecards.org
References
Akalin, A., Kormaksson, M., Li, S., Garrett-Bakelman, F. E., Figueroa, M. E., Melnick, A., et al. (2012). methylKit: a comprehensive R package for the analysis of genome-wide DNA methylation profiles. Genome Biol. 13:9. doi: 10.1186/gb-2012-13-10-R87
Andrews, S. (2010). FastQC: A Quality Control Tool for High Throughput Sequence Data. Available http://www.bioinformatics.babraham.ac.uk/projects/fastqc/ (accessed October 2, 2019).
Baldan, D., Curk, T., Hinde, C. A., and Lessells, C. M. (2019). Alternation of nest visits varies with experimentally manipulated workload in brood-provisioning great tits. Anim. Behav. 156, 139–146. doi: 10.1016/j.anbehav.2019.08.004
Barak, T., Kwan, K. Y., Louvi, A., Demirbilek, V., Saygi, S., Tuysuz, B., et al. (2011). Recessive LAMC3 mutations cause malformations of occipital cortical development. Nat. Genet. 43, 590–U132. doi: 10.1038/ng.836
Barbosa, T. D., Ingerslev, L. R., Alm, P. S., Versteyhe, S., Massart, J., Rasmussen, M., et al. (2016). High-fat diet reprograms the epigenome of rat spermatozoa and transgenerationally affects metabolism of the offspring. Mol. Metab. 5, 184–197. doi: 10.1016/j.molmet.2015.12.002
Bentz, A. B., Sirman, A. E., Wada, H., Navara, K. J., and Hood, W. R. (2016). Relationship between maternal environment and DNA methylation patterns of estrogen receptor alpha in wild Eastern Bluebird (Sialia sialis) nestlings: a pilot study. Ecol. Evol. 6, 4741–4752. doi: 10.1002/ece3.2162
Bhattacharya, T. K., Chatterjee, R. N., Sharma, R. P., Niranjan, M., Rajkumar, U., and Reddy, B. L. N. (2011). Polymorphism in the prolactin promoter and its association with growth traits in chickens. Biochem. Genet. 49, 385–394. doi: 10.1007/s10528-010-9415-3
Bird, A. (2002). DNA methylation patterns and epigenetic memory. Genes Dev. 16, 6–21. doi: 10.1101/gad.947102
Bošković, A., and Rando, O. J. (2018). Transgenerational epigenetic inheritance. Annu. Rev. Genet. 52, 21–41. doi: 10.1146/annurev-genet-120417-031404
Bosse, M., Spurgin, L. G., Laine, V. N., Cole, E. F., Firth, J. A., Gienapp, P., et al. (2017). Recent natural selection causes adaptive evolution of an avian polygenic trait. Science 358, 365–368. doi: 10.1126/science.aal3298
Brinkhof, M. W. G., Heeb, P., Kölliker, M., and Richner, H. (1999). Immunocompetence of nestling great tits in relation to rearing environment and parentage. Proc. R. Soc. Lond. B Biol. Sci. 266, 2315–2322. doi: 10.1098/rspb.1999.0925
Carere, C., Drent, P. J., Koolhaas, J. M., and Groothuis, T. G. G. (2005). Epigenetic effects on personality traits: early food provisioning and sibling competition. Behaviour 142, 1329–1355.
Caro, S. M., Griffin, A. S., Hinde, C. A., and West, S. A. (2016). Unpredictable environments lead to the evolution of parental neglect in birds. Nat. Commun. 7:10. doi: 10.1038/ncomms10985
Champagne, F. A., Francis, D. D., Mar, A., and Meaney, M. J. (2003). Variations in maternal care in the rat as a mediating influence for the effects of environment on development. Physiol. Behav. 79, 359–371. doi: 10.1016/S0031-9384(03)00149-5
Christe, P., Richner, H., and Oppliger, A. (1996). Begging, food provisioning, and nestling competition in great tit broods infested with ectoparasites. Behav. Ecol. 7, 127–131. doi: 10.1093/beheco/7.2.127
Corbett, B. A., Kantor, A. B., Schulman, H., Walker, W. L., Lit, L., Ashwood, P., et al. (2007). A proteomic study of serum from children with autism showing differential expression of apolipoproteins and complement proteins. Mol. Psychiatry 12, 292–306. doi: 10.1038/sj.mp.4001943
Dantzer, B., Newman, A. E. M., Boonstra, R., Palme, R., Boutin, S., Humphries, M. M., et al. (2013). Density triggers maternal hormones that increase adaptive offspring growth in a wild mammal. Science 340, 1215–1217. doi: 10.1126/science.1235765
Davies, W., Humby, T., Kong, W., Otter, T., Burgoyne, P. S., and Wilkinson, L. S. (2009). Converging pharmacological and genetic evidence indicates a role for steroid sulfatase in attention. Biol. Psychiatry 66, 360–367. doi: 10.1016/j.biopsych.2009.01.001
de Rooij, S. R., Painter, R. C., Phillips, D. I. W., Osmond, C., Michels, R. P. J., Godsland, I. F., et al. (2006). Impaired insulin secretion after prenatal exposure to the dutch famine. Diabetes Care 29, 1897–1901. doi: 10.2337/dc06-0460
de Rooij, S. R., Wouters, H., Yonker, J. E., Painter, R. C., and Roseboom, T. J. (2010). Prenatal undernutrition and cognitive function in late adulthood. Proc. Natl. Acad. Sci. U.S.A. 107, 16881–16886. doi: 10.1073/pnas.1009459107
DeKogel, C. H. (1997). Long-term effects of brood size manipulation on morphological development and sex-specific mortality of offspring. J. Anim. Ecol. 66, 167–178.
Derks, M. F. L., Schachtschneider, K. M., Madsen, O., Schijlen, E., Verhoeven, K. J. F., and van Oers, K. (2016). Gene and transposable element methylation in great tit (Parus major) brain and blood. BMC Genomics 17:13. doi: 10.1186/s12864-016-2653-y
Dix, D. J., Allen, J. W., Collins, B. W., Mori, C., Nakamura, N., PoormanAllen, P., et al. (1996). Targeted gene disruption of Hsp70-2 results in failed meiosis, germ cell apoptosis, and male infertility. Proc. Natl. Acad. Sci. U.S.A. 93, 3264–3268. doi: 10.1073/pnas.93.8.3264
Dloniak, S. M., French, J. A., and Holekamp, K. E. (2006). Rank-related maternal effects of androgens on behaviour in wild spotted hyaenas. Nature 440, 1190–1193. doi: 10.1038/nature04540
Docherty, S. J., Davis, O. S. P., Haworth, C. M. A., Plomin, R., and Mill, J. (2009). Bisulfite-based epityping on pooled genomic DNA provides an accurate estimate of average group DNA methylation. Epigenet. Chromatin 2:3. doi: 10.1186/1756-8935-2-3
Eden, E., Navon, R., Steinfeld, I., Lipson, D., and Yakhini, Z. (2009). GOrilla: a tool for discovery and visualization of enriched GO terms in ranked gene lists. BMC Bioinformatics 10:48. doi: 10.1186/1471-2105-10-48
Ewels, P., Magnusson, M., Lundin, S., and Käller, M. (2016). MultiQC: summarize analysis results for multiple tools and samples in a single report. Bioinformatics 32, 3047–3048. doi: 10.1093/bioinformatics/btw354
Forsman, A. (2015). Rethinking phenotypic plasticity and its consequences for individuals, populations and species. Heredity 115, 276–284. doi: 10.1038/hdy.2014.92
García-Navas, V., and Sanz, J. J. (2010). Flexibility in the foraging behavior of blue tits in response to short-term manipulations of brood size. Ethology 116, 744–754. doi: 10.1111/j.1439-0310.2010.01788.x
Gardner, D. S., Pearce, S., Dandrea, J., Walker, R., Ramsay, M. M., Stephenson, T., et al. (2004). Peri-implantation undernutrition programs blunted angiotensin ii evoked baroreflex responses in young adult sheep. Hypertension 43, 1290–1296. doi: 10.1161/01.HYP.0000126991.67203.7b
Gardner, D. S., Tingey, K., Van Bon, B. W. M., Ozanne, S. E., Wilson, V., Dandrea, J., et al. (2005). Programming of glucose-insulin metabolism in adult sheep after maternal undernutrition. Am. J. Physiol. Regul. Integr. Comp. Physiol. 289, R947–R954. doi: 10.1152/ajpregu.00120.2005
Gow, E. A., and Wiebe, K. L. (2014). Responses by central-place foragers to manipulations of brood size: parent flickers respond to proximate cues but do not increase work rate. Ethology 120, 881–892. doi: 10.1111/eth.12259
Groothuis, T. G. G., and Trillmich, F. (2011). Unfolding personalities: the importance of studying ontogeny. Dev. Psychobiol. 53, 641–655. doi: 10.1002/dev.20574
Guerrero-Bosagna, C., Morisson, M., Liaubet, L., Rodenburg, T. B., de Haas, E. N., Kostal, L., et al. (2018). Transgenerational epigenetic inheritance in birds. Env. Epigenet. 4:dvy008. doi: 10.1093/eep/dvy008
Guo, W. L., Fiziev, P., Yan, W. H., Cokus, S., Sun, X. G., Zhang, M. Q., et al. (2013). BS-Seeker2: a versatile aligning pipeline for bisulfite sequencing data. BMC Genomics 14:8. doi: 10.1186/1471-2164-14-774
Hales, C. N., and Barker, D. J. P. (1992). Type 2 (non-insulin-dependent) diabetes mellitus: the thrifty phenotype hypothesis. Diabetologia 35, 595–601. doi: 10.1007/BF00400248
Haure-Mirande, J.-V., Wang, M., Audrain, M., Fanutza, T., Kim, S. H., Heja, S., et al. (2019). Integrative approach to sporadic Alzheimer’s disease: deficiency of TYROBP in cerebral Aβ amyloidosis mouse normalizes clinical phenotype and complement subnetwork molecular pathology without reducing Aβ burden. Mol. Psychiatry 24, 431–446. doi: 10.1038/s41380-018-0255-6
Hawrylycz, M. J., Lein, E. S., Guillozet-Bongaarts, A. L., Shen, E. H., Ng, L., Miller, J. A., et al. (2012). An anatomically comprehensive atlas of the adult human brain transcriptome. Nature 489, 391–399. doi: 10.1038/nature11405
Heard, E., and Martienssen, R. A. (2014). Transgenerational epigenetic inheritance: myths and mechanisms. Cell 157, 95–109. doi: 10.1016/j.cell.2014.02.045
Hinde, C. A., and Kilner, R. M. (2007). Negotiations within the family over the supply of parental care. Proc. R. Soc. B Biol. Sci. 274, 53–60. doi: 10.1098/rspb.2006.3692
Hiyama, G., Kansaku, N., Tanaka, T., Wakui, S., and Zadworny, D. (2015). Characterization of chicken prolactin regulatory element binding protein and its expression in the anterior pituitary gland during embryogenesis and different reproductive stages. J. Poult. Sci. 52, 42–51. doi: 10.2141/jpsa.0140036
Jablonka, E., and Raz, G. (2009). Transgenerational epigenetic inheritance: prevalence, mechanisms, and implications for the study of heredity and evolution. Q. Rev. Biol. 84, 131–176.
Jimeno, B., Hau, M., Gomez-Diaz, E., and Verhulst, S. (2019). Developmental conditions modulate DNA methylation at the glucocorticoid receptor gene with cascading effects on expression and corticosterone levels in zebra finches. Sci. Rep. 9:11. doi: 10.1038/s41598-019-52203-8
Johnsson, M., Jonsson, K. B., Andersson, L., Jensen, P., and Wright, D. (2015). Genetic regulation of bone metabolism in the chicken: similarities and differences to mammalian systems. PLoS Genet. 11:e1005250. doi: 10.1371/journal.pgen.1005250
Kahn, H. S., Graff, M., Stein, A. D., and Lumey, L. H. (2009). A fingerprint marker from early gestation associated with diabetes in middle age: the dutch hunger winter families study. Int. J. Epidemiol. 38, 101–109. doi: 10.1093/ije/dyn158
Kappeler, L., and Meaney, M. J. (2010). Epigenetics and parental effects. BioEssays 32, 818–827. doi: 10.1002/bies.201000015
Keller, L. F., and van Noordwijk, A. J. (1994). Effects of local environmental conditions on nestling growth in the great tit Parus major L. Ardea 82, 349–362.
Kesherwani, V., Shahshahan, H. R., and Mishra, P. K. (2017). Cardiac transcriptome profiling of diabetic Akita mice using microarray and next generation sequencing. PLoS One 12:e0182828. doi: 10.1371/journal.pone.0182828
Khoonsari, P. E., Haggmark, A., Lonnberg, M., Mikus, M., Kilander, L., Lannfelt, L., et al. (2016). Analysis of the cerebrospinal fluid proteome in Alzheimer’s disease. PLoS One 11:e0150672. doi: 10.1371/journal.pone.0150672
Kilvitis, H. J., Alvarez, M., Foust, C. M., Schrey, A. W., Robertson, M., and Richards, C. L. (2014). “Ecological epigenetics,” in Ecological Genomics: Ecology and the Evolution of Genes and Genomes Advances in Experimental Medicine and Biology, eds C. R. Landry and N. AubinHorth (New York: Springer), 191–210.
Krause, E. T., Honarmand, M., Wetzel, J., and Naguib, M. (2009). Early fasting is long lasting: differences in early nutritional conditions reappear under stressful conditions in adult female zebra finches. PLoS One 4:e5015. doi: 10.1371/journal.pone.0005015
Krueger, F. (2012). Trim Galore: A Wrapper Tool Around Cutadapt and FastQC to Consistently Apply Quality and Adapter Trimming to FastQ files, with Some Extra Functionality for MspI-digested RRBS-type (Reduced Representation Bisufite-Seq) Libraries. Available online at: https://www.bioinformatics.babraham.ac.uk/projects/trim_galore/ (accessed October 7, 2019).
Laine, V. N., Gossmann, T. I., Schachtschneider, K. M., Garroway, C. J., Madsen, O., Verhoeven, K. J. F., et al. (2016). Evolutionary signals of selection on cognition from the great tit genome and methylome. Nat. Commun. 7:9. doi: 10.1038/ncomms10474
Langmead, B., Trapnell, C., Pop, M., and Salzberg, S. L. (2009). Ultrafast and memory-efficient alignment of short DNA sequences to the human genome. Genome Biol. 10:10. doi: 10.1186/gb-2009-10-3-r25
Lawrence, M., Gentleman, R., and Carey, V. (2009). rtracklayer: an R package for interfacing with genome browsers. Bioinformatics 25, 1841–1842. doi: 10.1093/bioinformatics/btp328
Lawrence, M., Huber, W., Pagès, H., Aboyoun, P., Carlson, M., Gentleman, R., et al. (2013). Software for computing and annotating genomic ranges. PLoS Comput. Biol. 9:e1003118. doi: 10.1371/journal.pcbi.1003118
Lindner, M., Laine, V. N., Verhagen, I., Viitaniemi, H. M., Visser, M. E., van Oers, K., et al. (2021a). Rapid changes in DNA methylation associated with the initiation of reproduction in a small songbird. Mol. Ecol. doi: 10.1111/mec.15803 [Epub ahead of print].
Lindner, M., Verhagen, I., Viitaniemi, H. M., Laine, V. N., Visser, M. E., van Oers, K., et al. (2021b). Temporal changes in DNA methylation and RNA expression in a small song bird: within- and between-tissue comparisons. BMC Genomics 22:36. doi: 10.1186/s12864-020-07329-9
Liu, L., Yang, N., Xu, G. Y., Liu, S. L., Wang, D., Song, J. Z., et al. (2018). Transgenerational transmission of maternal stimulatory experience in domesticated birds. FASEB J. 32, 7002–7017. doi: 10.1096/fj.201800762RR
Mathot, K. J., Olsen, A.-L., Mutzel, A., Araya-Ajoy, Y. G., Nicolaus, M., Westneat, D. F., et al. (2017). Provisioning tactics of great tits (Parus major) in response to long-term brood size manipulations differ across years. Behav. Ecol. 28, 1402–1413. doi: 10.1093/beheco/arx083
McGhee, K. E., and Bell, A. M. (2014). Paternal care in a fish: epigenetics and fitness enhancing effects on offspring anxiety. Proc. R. Soc. B Biol. Sci. 281;6. doi: 10.1098/rspb.2014.1146
Meissner, A. (2005). Reduced representation bisulfite sequencing for comparative high-resolution DNA methylation analysis. Nucleic Acids Res. 33, 5868–5877. doi: 10.1093/nar/gki901
Mertens, J. A. L. (1969). Influence of brood size on energy metabolism and water loss of nestling great tits Parus major major. IBIS 111, 11–18. doi: 10.1111/j.1474-919X.1969.tb01599.x
Moore, L. D., Le, T., and Fan, G. (2013). DNA methylation and its basic function. Neuropsychopharmacology 38, 23–38. doi: 10.1038/npp.2012.112
Mutzel, A., Dingemanse, N. J., Araya-Ajoy, Y. G., and Kempenaers, B. (2013). Parental provisioning behaviour plays a key role in linking personality with reproductive success. Proc. R. Soc. B Biol. Sci. 280:9. doi: 10.1098/rspb.2013.1019
Naef-Daenzer, B., and Keller, L. F. (1999). The foraging performance of great and blue tits (Parus major and P-caerulens) in relation to caterpillar development, and its consequences for nestling growth and fledging weight. J. Anim. Ecol. 68, 708–718. doi: 10.1046/j.1365-2656.1999.00318.x
Naguib, M., Florcke, C., and van Oers, K. (2011). Effects of social conditions during early development on stress response and personality traits in great tits (Parus major). Dev. Psychobiol. 53, 592–600. doi: 10.1002/dev.20533
Naguib, M., Nemitz, A., and Gil, D. (2006). Maternal developmental stress reduces reproductive success of female offspring in zebra finches. Proc. R. Soc. B Biol. Sci. 273, 1901–1905. doi: 10.1098/rspb.2006.3526
Naguib, M., Riebel, K., Marzal, A., and Gil, D. (2004). Nestling immunocompetence and testosterone covary with brood size in a songbird. Proc. R. Soc. Lond. B Biol. Sci. 271, 833–838. doi: 10.1098/rspb.2003.2673
Natt, D., Agnvall, B., and Jensen, P. (2014). Large sex differences in chicken behavior and brain gene expression coincide with few differences in promoter DNA-methylation. PLoS One 9:e0096376. doi: 10.1371/journal.pone.0096376
Nettle, D., Andrews, C. P., Monaghan, P., Brilot, B. O., Bedford, T., Gillespie, R., et al. (2015). Developmental and familial predictors of adult cognitive traits in the European starling. Anim. Behav. 107, 239–248. doi: 10.1016/j.anbehav.2015.07.002
Nettle, D., Monaghan, P., Boner, W., Gillespie, R., and Bateson, M. (2013). Bottom of the heap: having heavier competitors accelerates early-life telomere loss in the European starling, Sturnus vulgaris. PLoS One 8:e0083617. doi: 10.1371/journal.pone.0083617
Neuenschwander, S., Brinkhof, M. W. G., Kolliker, M., and Richner, H. (2003). Brood size, sibling competition, and the cost of begging in great tits (Parus major). Behav. Ecol. 14, 457–462. doi: 10.1093/beheco/arg025
Park, J. M., Kim, M. Y., Kim, T. H., Min, D. K., Yang, G. E., and Ahn, Y. H. (2018). Prolactin regulatory element-binding (PREB) protein regulates hepatic glucose homeostasis. Biochim. Biophys. Acta Mol. Basis Dis. 1864, 2097–2107. doi: 10.1016/j.bbadis.2018.03.024
Perrins, C. M., and McCleery, R. H. (1989). Laying dates and clutch size in the great tit. Wilson Bull. 101, 236–253.
Pertille, F., Brantsaeter, M., Nordgreen, J., Coutinho, L. L., Janczak, A. M., Jensen, P., et al. (2017). DNA methylation profiles in red blood cells of adult hens correlate with their rearing conditions. J. Exp. Biol. 220, 3579–3587. doi: 10.1242/jeb.157891
Pettifor, R. A., Perrins, C. M., and McCleery, R. H. (1988). Individual optimization of clutch size in Great Tits. Nature 336, 160–162. doi: 10.1038/336160a0
Pettifor, R. A., Perrins, C. M., and McCleery, R. H. (2001). The individual optimization of fitness: variation in reproductive output, including clutch size, mean nestling mass and offspring recruitment, in manipulated broods of great tits Parus major. J. Anim. Ecol. 70, 62–79. doi: 10.1046/j.1365-2656.2001.00465.x
Reddon, A. R. (2012). Parental effects on animal personality. Behav. Ecol. 23, 242–245. doi: 10.1093/beheco/arr210
Reyna-López, G. E., Simpson, J., and Ruiz-Herrera, J. (1997). Differences in DNA methylation patterns are detectable during the dimorphic transition of fungi by amplification of restriction polymorphisms. Mol. Gen. Genet. MGG 253, 703–710. doi: 10.1007/s004380050374
Riyahi, S., Sanchez-Delgado, M., Calafell, F., Monk, D., and Senar, J. C. (2015). Combined epigenetic and intraspecific variation of the DRD4 and SERT genes influence novelty seeking behavior in great tit Parus major. Epigenetics 10, 516–525. doi: 10.1080/15592294.2015.1046027
Roseboom, T., de Rooij, S., and Painter, R. (2006). The Dutch famine and its long-term consequences for adult health. Early Hum. Dev. 82, 485–491. doi: 10.1016/j.earlhumdev.2006.07.001
Roulin, A., Emaresi, G., Bize, P., Gasparini, J., Piault, R., and Ducrest, A.-L. (2011). Pale and dark reddish melanic tawny owls differentially regulate the level of blood circulating POMC prohormone in relation to environmental conditions. Oecologia 166, 913–921. doi: 10.1007/s00442-011-1955-7
Rubenstein, D. R., Skolnik, H., Berrio, A., Champagne, F. A., Phelps, S., and Solomon, J. (2016). Sex-specific fitness effects of unpredictable early life conditions are associated with DNA methylation in the avian glucocorticoid receptor. Mol. Ecol. 25, 1714–1728. doi: 10.1111/mec.13483
Saino, N., Suffritti, C., Martinelli, R., Rubolini, D., and Møller, A. P. (2003). Immune response covaries with corticosterone plasma levels under experimentally stressful conditions in nestling barn swallows (Hirundo rustica). Behav. Ecol. 14, 318–325. doi: 10.1093/beheco/14.3.318
Sajuthi, S. P., Sharma, N. K., Chou, J. W., Palmer, N. D., McWilliams, D. R., Beal, J., et al. (2016). Mapping adipose and muscle tissue expression quantitative trait loci in African Americans to identify genes for type 2 diabetes and obesity. Hum. Genet. 135, 869–880. doi: 10.1007/s00439-016-1680-8
Sanz, J. J., and Tinbergen, J. M. (1999). Energy expenditure, nestling age, and brood size: an experimental study of parental behavior in the great tit Parus major. Behav. Ecol. 10, 598–606. doi: 10.1093/beheco/10.5.598
Schrey, A. W., Alvarez, M., Foust, C. M., Kilvitis, H. J., Lee, J. D., Liebl, A. L., et al. (2013). Ecological epigenetics: beyond MS-AFLP. Integr. Comp. Biol. 53, 340–350. doi: 10.1093/icb/ict012
Schulz, L. C. (2010). The Dutch Hunger Winter and the developmental origins of health and disease. Proc. Natl. Acad. Sci. U.S.A. 107, 16757–16758. doi: 10.1073/pnas.1012911107
Schwabl, H. (1993). Yolk is a source of maternal testosterone for developing birds. Proc. Natl. Acad. Sci. U.S.A. 90, 11446–11450. doi: 10.1073/pnas.90.24.11446
Sepers, B., van den Heuvel, K., Lindner, M., Viitaniemi, H., Husby, A., and van Oers, K. (2019). Avian ecological epigenetics: pitfalls and promises. J. Ornithol. 160, 1183–1203. doi: 10.1007/s10336-019-01684-5
Sheldon, E. L., Schrey, A. W., Ragsdale, A. K., and Griffith, S. C. (2018). Brood size influences patterns of DNA methylation in wild Zebra Finches (Taeniopygia guttata). Auk 135, 1113–1122. doi: 10.1642/auk-18-61.1
Singh, R. D., Schroeder, A. S., Scheffer, L., Holicky, E. L., Wheatley, C. L., Marks, D. L., et al. (2013). Prominin-2 expression increases protrusions, decreases caveolae and inhibits Cdc42 dependent fluid phase endocytosis. Biochem. Biophys. Res. Commun. 434, 466–472. doi: 10.1016/j.bbrc.2013.03.097
Son, W. Y., Hwang, S. H., Han, C. T., Lee, J. H., Kim, S., and Kim, Y. C. (1999). Specific expression of heat shock protein HspA2 in human male germ cells. Mol. Hum. Reprod. 5, 1122–1126. doi: 10.1093/molehr/5.12.1122
Spurgin, L. G., Bosse, M., Adriaensen, F., Albayrak, T., Barboutis, C., Belda, E., et al. (2019). The great tit HapMap project: a continental-scale analysis of genomic variation in a songbird. bioRxiv [Preprint]. doi: 10.1101/561399
Stanner, S. A., and Yudkin, J. S. (2001). Fetal programming and the leningrad siege study. Twin Res. 4, 287–292. doi: 10.1375/twin.4.5.287
St-Cyr, S., and McGowan, P. O. (2015). Programming of stress-related behavior and epigenetic neural gene regulation in mice offspring through maternal exposure to predator odor. Front. Behav. Neurosci. 9:145. doi: 10.3389/fnbeh.2015.00145
Szwergold, B. S., Bunker, R. D., and Loomes, K. M. (2011). The physiological substrates of fructosamine-3-kinase-related-protein (FN3KRP) are intermediates of nonenzymatic reactions between biological amines and ketose sugars (fructation products). Med. Hypotheses 77, 739–744. doi: 10.1016/j.mehy.2011.07.027
Tinbergen, J. M., and Boerlijst, M. C. (1990). Nestling weight and survival in individual Great Tits (Parus major). J. Anim. Ecol. 59, 1113–1127. doi: 10.2307/5035
Tobi, E. W., Goeman, J. J., Monajemi, R., Gu, H., Putter, H., Zhang, Y., et al. (2014). DNA methylation signatures link prenatal famine exposure to growth and metabolism. Nat. Commun. 5:5592. doi: 10.1038/ncomms6592
Tobi, E. W., Lumey, L. H., Talens, R. P., Kremer, D., Putter, H., Stein, A. D., et al. (2009). DNA methylation differences after exposure to prenatal famine are common and timing- and sex-specific. Hum. Mol. Genet. 18, 4046–4053. doi: 10.1093/hmg/ddp353
Trent, S., Fry, J. P., Ojarikre, O. A., and Davies, W. (2014). Altered brain gene expression but not steroid biochemistry in a genetic mouse model of neurodevelopmental disorder. Mol. Autism 5:21. doi: 10.1186/2040-2392-5-21
van Oers, K., Drent, P. J., Dingemanse, N. J., and Kempenaers, B. (2008). Personality is associated with extrapair paternity in great tits, Parus major. Anim. Behav. 76, 555–563. doi: 10.1016/j.anbehav.2008.03.011
van Oers, K., Kohn, G. M., Hinde, C. A., and Naguib, M. (2015). Parental food provisioning is related to nestling stress response in wild great tit nestlings: implications for the development of personality. Front. Zool. 12:10. doi: 10.1186/1742-9994-12-s1-s10
van Oers, K., Sepers, B., Sies, W., Gawehns, F., Verhoeven, K. J. F., and Laine, V. N. (2020). Epigenetics of animal personality: DNA methylation cannot explain the heritability of exploratory behavior in a songbird. Integr. Comp. Biol. 60, 1517–1530. doi: 10.1093/icb/icaa138
Verhulst, E. C., Mateman, A. C., Zwier, M. V., Caro, S. P., Verhoeven, K. J. F., and Van Oers, K. (2016). Natural variation in animal personality is associated with transgenerationally consistent DNA methylation. Mol. Ecol. 25, 1801–1811. doi: 10.1111/mec.13519
Verhulst, S., Holveck, M.-J., and Riebel, K. (2006). Long-term effects of manipulated natal brood size on metabolic rate in zebra finches. Biol. Lett. 2, 478–480. doi: 10.1098/rsbl.2006.0496
Viitaniemi, H. M., Verhagen, I., Visser, M. E., Honkela, A., van Oers, K., and Husby, A. (2019). Seasonal variation in genome-wide DNA methylation patterns and the onset of seasonal timing of reproduction in great tits. Genome Biol. Evol. 11, 970–983. doi: 10.1093/gbe/evz044
Wang, S. H., Cheng, C. Y., Tang, P. C., Chen, C. F., Chen, H. H., Lee, Y. P., et al. (2013). Differential gene expressions in testes of L2 strain Taiwan country chicken in response to acute heat stress. Theriogenology 79, 374–382. doi: 10.1016/j.theriogenology.2012.10.010
Wang, S. H., Cheng, C. Y., Tang, P. C., Chen, C. F., Chen, H. H., Lee, Y. P., et al. (2015). Acute heat stress induces differential gene expressions in the testes of a broiler-type strain of taiwan country chickens. PLoS One 10:e0125816. doi: 10.1371/journal.pone.0125816
Weaver, I. C. G., Cervoni, N., Champagne, F. A., D’Alessio, A. C., Sharma, S., Seckl, J. R., et al. (2004). Epigenetic programming by maternal behavior. Nat. Neurosci. 7, 847–854. doi: 10.1038/nn1276
Wilkin, T. A., King, L. E., and Sheldon, B. C. (2009). Habitat quality, nestling diet, and provisioning behaviour in great tits Parus major. J. Avian Biol. 40, 135–145. doi: 10.1111/j.1600-048X.2009.04362.x
Willson, N. L., Forder, R. E. A., Tearle, R., Williams, J. L., Hughes, R. J., Nattrass, G. S., et al. (2018). Transcriptional analysis of liver from chickens with fast (meat bird), moderate (F1 layer x meat bird cross) and low (layer bird) growth potential. BMC Genomics 19:12. doi: 10.1186/s12864-018-4723-9
Wingett, S. W., and Andrews, S. (2018). FastQ screen: a tool for multi-genome mapping and quality control. F1000Research 7:1338. doi: 10.12688/f1000research.15931.2
Wright, J., Both, C., Cotton, P. A., and Bryant, D. (1998). Quality vs. quantity: energetic and nutritional trade-offs in parental provisioning strategies. J. Anim. Ecol. 67, 620–634.
Zeng, H. K., Shen, E. H., Hohmann, J. G., Oh, S. W., Bernard, A., Royall, J. J., et al. (2012). Large-scale cellular-resolution gene profiling in human neocortex reveals species-specific molecular signatures. Cell 149, 483–496. doi: 10.1016/j.cell.2012.02.052
Zhu, J., Wang, Y., Zhu, K., Gao, J., Wan, X., Pang, X., et al. (2017). Serum fibroblast growth factor 1 is associated with the decreased risk of obesity in human. Exp. Clin. Endocrinol. Diabetes 125, 322–326. doi: 10.1055/s-0043-104532
Keywords: parental effects, development, postnatal conditions, growth, physiology, behavior
Citation: Sepers B, Erven JAM, Gawehns F, Laine VN and van Oers K (2021) Epigenetics and Early Life Stress: Experimental Brood Size Affects DNA Methylation in Great Tits (Parus major). Front. Ecol. Evol. 9:609061. doi: 10.3389/fevo.2021.609061
Received: 22 September 2020; Accepted: 15 February 2021;
Published: 04 March 2021.
Edited by:
Olivia Roth, GEOMAR Helmholtz Center for Ocean Research Kiel, GermanyReviewed by:
Britta Meyer, Max Planck Institute for Evolutionary Biology, GermanyDafni Anastasiadi, The New Zealand Institute for Plant and Food Research Ltd., New Zealand
Copyright © 2021 Sepers, Erven, Gawehns, Laine and van Oers. This is an open-access article distributed under the terms of the Creative Commons Attribution License (CC BY). The use, distribution or reproduction in other forums is permitted, provided the original author(s) and the copyright owner(s) are credited and that the original publication in this journal is cited, in accordance with accepted academic practice. No use, distribution or reproduction is permitted which does not comply with these terms.
*Correspondence: Bernice Sepers, Yi5zZXBlcnNAbmlvby5rbmF3Lm5s; ay52YW5vZXJzQG5pb28ua25hdy5ubA==