- 1Department of Geography and the Environment, Villanova University, Villanova, PA, United States
- 2Department of Ecological Science, Vrije Universiteit Amsterdam, Amsterdam, Netherlands
Microbes are abundant inhabitants of the near-surface atmosphere in urban areas. The distribution of microbial communities may benefit or hinder human wellbeing and ecosystem function. Surveys of airborne microbial diversity are uncommon in both natural and built environments and those that investigate diversity are stationary in the city, thus missing continuous exposure to microbes that covary with three-dimensional urban structure. Individuals in cities are generally mobile and would be exposed to diverse urban structures outdoors and within indoor-transit systems in a day. We used mobile monitoring of microbial diversity and geographic information system spatial analysis, across Philadelphia, Pennsylvania, USA in outdoor and indoor-transit (subways and train cars) environments. This study identifies to the role of the three-dimensional urban landscape in structuring atmospheric microbiomes and employs mobile monitoring over ~1,920 kilometers to measure continuous biodiversity. We found more diverse communities outdoors that significantly differ from indoor-transit air in microbial community structure, function, likely source environment, and potentially pathogenic fraction of the community. Variation in the structure of the urban landscape was associated with diversity and function of the near-surface atmospheric microbiome in outdoor samples.
Importance
Global nutrient cycling and human health depend on the rich biodiversity of microorganisms. The influence of the urban environment on microbiomes remains poorly described despite cities being the fastest growing ecosystems. All life is exposed to the atmosphere and thus discerning what microbes are present and what their functions may be is critical to create resistant and resilient cities under climate change. This study combines a spatially explicit analysis of urban structure with mobile monitoring of the atmospheric microbiome.
Introduction
Microbes are abundant inhabitants of the near-surface atmosphere (Burrows et al., 2009) whose presence and distributions in urban areas have implications on public health and ecosystem function. The atmosphere serves as both a source and a sink for microorganisms that may return to the surface through dry or wet deposition (Chate et al., 2003; Wu et al., 2018). Furthermore, urban areas are the fastest growing ecosystems (Grimm et al., 2008) and are composed of heterogeneous landscapes (Cadenasso et al., 2007) with diverse land covers combined in various configurations and proportions. Many studies of urban structure rely on remotely sensed landcover datasets that distinguish between the built and natural elements of the landscape; however, they ignore the vertical dimension. Building height influences urban ecosystems by altering wind currents and casting shadows which alter physical properties such as air temperature and evapotranspiration potential.
Structure of Urban Landscape (STURLA) spatial analysis studies have demonstrated that cities are composed of a discrete number of three-dimensional composite land classes where a small number of classes (5–10) explain the urban landscape and host distinct physical properties of the environment (Larondelle et al., 2014; Hamstead et al., 2016) and is useful for studies of urban ecosystem services (Haase et al., 2014). The urban landscape is classified by applying a fishnet grid of 120 m2 sized pixels over a city and calculating the relative proportions of land cover elements within a pixel as derived from remotely sensed satellite imagery. STURLA classes represent combinations of the natural and built aspects of urban structure while incorporating the vertical dimension (lowrise, midrise, and highrise buildings) which gives it an explanatory advantage over most land-cover datasets. For example, class tgplm is a STURLA class heterogeneously composed of trees (t), grass (grass), pavement (p), low-rise (l), and mid-rise buildings (m). This framework allows for scalable and reproducible tracing of urban spatio-temporal patterns that can be applied across cities to identify structure function relationships. Likewise, it could easily be incorporated into models that forecast near-term changes (Dietze et al., 2018) in urban ecosystem services including and their resilience to change (Folke et al., 2002; Gunderson et al., 2012).
Urban areas host diverse microbiomes with unique selective pressures (e.g., pollution and chronic disturbance) that vary along spatial gradients (Reese et al., 2016; Mhuireach et al., 2019); however, relationships between urban landscape heterogeneity and atmospheric microbes remain largely unexplored. Aside from outdoor air, public transit systems also host unique microbiomes. These systems are taxonomically diverse (Dybwad et al., 2012; Leung et al., 2014; Triadó-Margarit et al., 2017; Gohli et al., 2019) and represent unique ecological niches due to their high usage rate by humans and rapid turnover in occupancy. Likewise, studies of outdoor atmospheric microbiomes have until now been mostly stationary (Bowers et al., 2009; Lee et al., 2009; Mazar et al., 2016; Cáliz et al., 2018; Stewart et al., 2020b) and miss the continuous biodiversity of the urban landscape. Microbiomes of both outdoor and indoor-transit air are assembled from diverse environments including soils (Bowers et al., 2009, 2011b), water (Methé et al., 1998; Linz et al., 2017), vegetation (Lymperopoulou et al., 2016), and animals (Täubel et al., 2009; Hospodsky et al., 2012; Leung et al., 2014). These communities host diverse bacterial phyla largely composed of three phyla: Proteobacteria, Actinobacteria, Firmicutes, and Bacteroidetes (Li et al., 2019; Mhuireach et al., 2019) that vary seasonally (Cáliz et al., 2018; Du et al., 2018) and by the environment surrounding the air (Bowers et al., 2011b, 2013). Mobile monitoring (sampling while moving) of the atmosphere is increasingly used to understand patterns of particles in air (Wallace et al., 2009; Shakya et al., 2019; Deshmukh et al., 2020) and microbial diversity in a subway (Leung et al., 2014), until now it has not been applied to identifying patterns of microbial biodiversity in outdoor air relevant to the populations living in cities.
Recently, the health effects of microbial exposure in addition to pathogen identification are now being explored (Ege et al., 2011; Hanski et al., 2012; Ruokolainen et al., 2015). Low-diversity microbiomes in animals are associated with chronic-diseases including: asthma, irritable bowel syndrome (Frank et al., 2007; Round and Mazmanian, 2009), and obesity (Turnbaugh et al., 2008). Research has identified exposure to microbially diverse outdoor and built environments during childhood offers protection against asthma and immune diseases (Rook, 2009; von Hertzen et al., 2011; Mills et al., 2017). Niche occupation of the respiratory tract by diverse taxa would limit the surface area where bacteria suggested to exacerbate asthma (e.g., Haemophilus spp. or Streptococcus spp.) could settle (Ege et al., 2011). Likewise, diverse microbial exposure has been suggested to activate downstream signaling pathways of toll-like receptors that induce regulatory T-cells (Schaub et al., 2009). Given these associations, discerning patterns of diversity in urban airs holds implications for human health.
Understanding how structural elements relate to atmospheric microbial diversity and function is necessary for understanding urban ecology (Jansson, 2013), and can guide urban development in ways that utilize ecological functions to enhance environmental performance and human wellbeing. In this study we combine mobile monitoring of the atmospheric microbiome from 07/01/19 to 07/25/19 using marker gene sequencing (16s rRNA) and genome prediction to characterize the diversity and inferred functional potential in outdoor and indoor-transit air. Samples were taken outdoors by driving across 240 kilometers with eight repetitions and commuting through three train lines four times in Philadelphia PA. This is complemented with three-dimensional spatial analysis of how subtle variation in urban landscape composition influences microbial communities.
Materials and Methods
Site Description and Sample Collection
Philadelphia, Pennsylvania, USA is a city flanked by two bodies of water located in the Mid-Atlantic region of the United States of America with a 2018 population estimate of 1,584,138 (Lynch et al., 2014). Samples were collected on sterilized (500°C for 3 h) quartz fiber filters (as done similarly in Stewart et al., 2020b) using a PDR1500 Air Quality Dust Monitor (Thermo-Fisher Scientific, United States of America) where the inlet tube and mesh filter holder was cleaned with 70% ethanol before sampling. Only particles smaller than 2.5 micrometers in diameter (PM2.5) were collected using a particulate matter sampler with a flow rate of 1.5 liters per minute. Particulate matter was impacted on quartz fiber filters, as done in similar studies (Bowers et al., 2013; Stewart et al., 2020b), where after collection the filter was removed using sterilized tweezers and stored in aluminum foil at−20°C until DNA extraction. DNA was then stored at−80°C until downstream analyses.
Urban Structure and Route Generation
Urban structure was characterized in 120 m2 blocks using the STructure of Urban LAndscapes (STURLA) system, a multi-dimensional spatial classification system that incorporates land cover with urban building/structure height as previously described (Hamstead et al., 2016). Relative frequencies of STURLA classes were calculated and those with an abundance below 0.1% were grouped into the category “other.” STURLA across Philadelphia can be found in Figure 1. Routes were generated to include at least 50 points in each of the composite classes represented in the city STURLA classification to maximize variation in urban structure. Points were randomly selected based on a stratified random sample to ensure adequate representation of the most common composite classes. In addition, points of interest were added to the random sample including industrial sites, EPA TRI sites, and EPA air pollution monitoring station sites. Using ESRI ArcGIS 10.7.1 Network Analyst, an optimal route of approximately 480 kilometers was generated, passing through the sample points. The route was then divided into two routes—R1 and R2 for feasibility of driving within one day. The routes were loaded to a professional-grade GPS unit (Thimble Juno 3b, USA), and the van was driven along each route. Each route was replicated 4 times for a total of 8 samples; however, variation of each route exists day to day to test the role of subtle variation in the urban landscape.
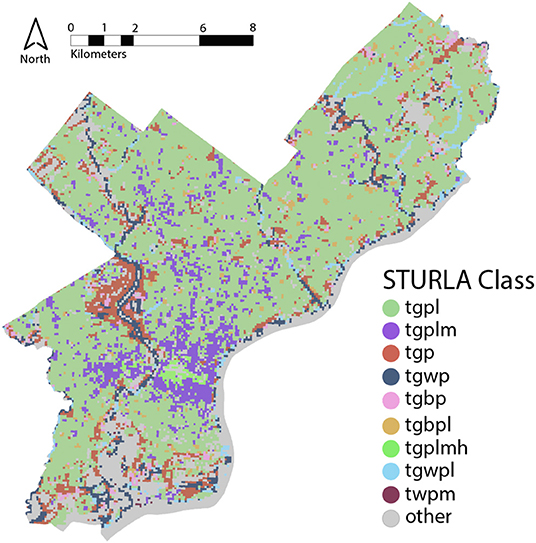
Figure 1. STURLA map of Philadelphia, Pennsylvania, USA where each color corresponds to a different STURLA code. T, trees; G, grass; B, bare; W, water; P, pavement; L, low-rise; M, midrise; H, highrise. The combination of these individual letters creates a STURLA code; for example, class tgpl is composed of various proportions of trees (T), grass (G), pavement (P), and low-rise buildings (L).
Outdoor Sampling
Outdoor air samples (n = 8) were collected using the same instrument (PDR1500) strapped to the roof of a van (~1.5 meters in height) with the inlet facing out to the side of the roof. Samples were collected from two routes (R1: Route 1, R2: Route 2) with R1 being more diverse in urban structure. The van was driven at a variety of speeds ranging from 0 km/h—~100 km/h (e.g., stoplight vs. highway). Average sampling period for each of the routes was 601 ± 48 min for each of the outdoor air sample days, collecting an average of 913.3 L ± 73.4 L of air. Variation exists within routes to address the roles of both large (between route) and small (within-route) variation in structuring the atmospheric microbiome (Figure 2). The distribution of STURLA classes throughout the city can be found in Figure 3B.
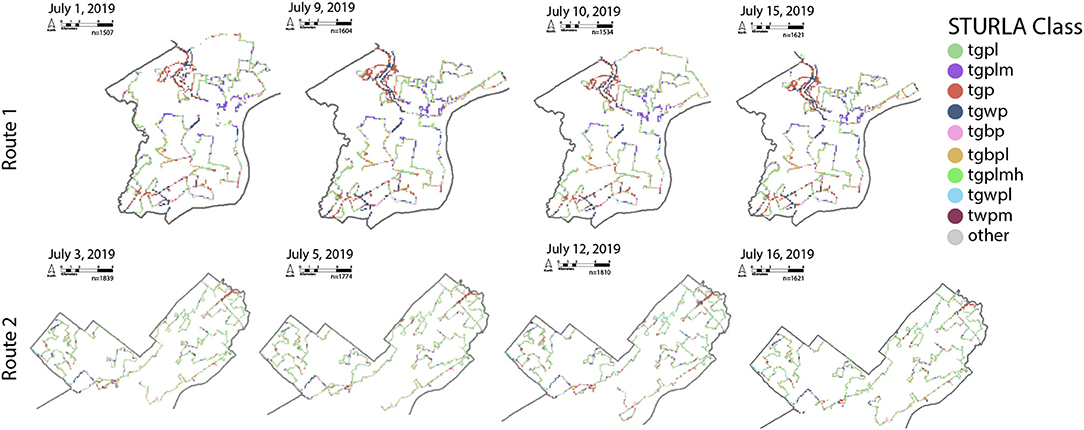
Figure 2. Sampling routes for each day of collection, colors correspond with STURLA class. The reader is encouraged to zoom in on each map to examine the spatial distribution of sampling and the corresponding STURLA class. T, trees; G, grass; B, bare; W, water; P, pavement; L, low-rise; M, midrise; H, highrise. The combination of these individual letters creates a STURLA code; for example, class tgpl is composed of various proportions of trees (T), grass (G), pavement (P), and low-rise buildings (L).
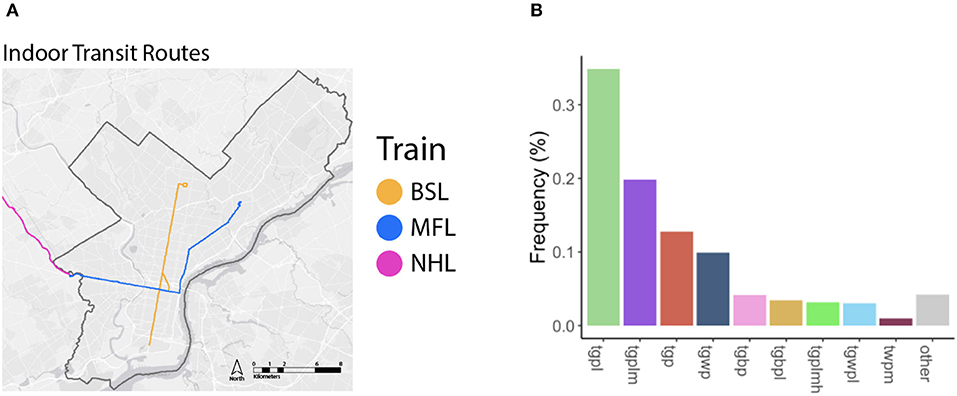
Figure 3. (A) Routes where indoor transit system air was collected (BSL, Broad Street Line; MFL, Market-Frankford Line; NHL, Norristown Highspeed Line). (B) Barplot of mean class frequency encountered while driving, colors correspond with STURLA class.
Indoor-Transit Sampling
Three indoor-transit lines (Figure 3A), the Market-Frankford Line (above/below ground), Norristown Line (above ground), and BSL (below ground) of air were collected to comprise one sample. Collection (n = 4) took place inside train cars for an average 288.3 ± 21.1 min impacting 438.1 ± 32.4 L of air. On average ~30 people were counted in each train car during sampling.
DNA Extraction, Sequencing, Processing, and Genome Prediction
DNA was extracted using the MoBio PowerSoil™ DNA Isolation Kit following the manufacturer's suggested protocol. The 16S rRNA gene HV4 region for bacterial and archaeal microorganisms (515F: 5′-GTGCCAGCMGCCGCGGTAA, 806R: 5′-GGACTACHVHHHTWTCTAAT, Thermo-Fisher, Waltham, MA, USA) was then amplified by polymerase chain reaction (PCR) using the Earth Microbiome Project's protocol (Earth Microbiome Project, 2016). A positive control of E. coli and a negative control of deionized water were used and no contamination was found. Sequencing was performed at MR DNA (www.mrdnalab.com, Shallowater, TX, USA) using the Illumina MiSeq platform. DNA sequences were analyzed and statistical analysis was conducted using QIIME 2 (Bolyen et al., 2019) following the Moving Pictures tutorial code and R (3.6). Briefly, raw reads were demultiplexed, demised using the DADA2 algorithm (Callahan et al., 2016), and truncated at 180 basepairs.
Sequences were rarefied to 1,55,547 sequences aligned to the SILVA 132 99% reference taxonomy database (Quast et al., 2013) using a Naive Bayes classifier to amplicon sequence variants (ASVs). Sequences were aligned using mafft (Katoh and Standley, 2013) followed by construction of an unrooted phylogenetic tree using FastTree (Price et al., 2009). Alpha diversity metrics including observed number of ASVs, Pielou's evenness, and Faith's Phylogenetic Diversity (Faith, 1992) were calculated. Beta diversity was calculated using unweighted UniFrac for phylogenetic analysis (Lozupone and Knight, 2005). Unweighted UniFrac was chosen as it is qualitative and thus makes our analyses less sensitive to differences in sampling volume and allows comparability to qualitative functional inference (e.g., functional Bray-Curtis PcoA). PICRUSt 2 (Douglas et al., 2019) was used to infer functional genes using the maximum parsimony hidden-state prediction methodology (Louca and Doebeli, 2018) as done in other studies of the atmospheric microbiome (Pan et al., 2019; Stewart et al., 2020b). Functional gene predictions were rarefied to the minimum number of features found between all samples. Beta diversity for functional gene predictions was conducted using Bray-Curtis dissimilarity and Principal Coordinates Analysis (PcoA).
Data Analysis
Identification of potentially pathogenic taxa was conducted comparing the average 40 most relatively abundant ASVs with a reference database of bacterial strains that are known human pathogens (UK Health and Safety Executive Advisory Committee on Dangerous Pathogens). Taxa representing <0.01% of the pathogenic subset of taxa were grouped into the category “Other.” Likely source environment for the top 40 ASVs was determined through a literature review (Stewart et al., 2020b) (supplemental references). A taxon found in more than one environment was categorized as a “generalist” as done in previous research (Stewart et al., 2020b). For this the relative abundance of each taxon was coded based on their likely source environment. Coded environment was then used for statistical analysis as seen below.
Bray-Curtis dissimilarity for functional gene predictions, source environment, and potentially pathogenic taxa was calculated. Kruskal-Wallis tests were used to test for significant differences indoor-transit, outdoor air, and variation between R1 and R2 in diversity. PERMANOVA tests identified if community structure (phylogenetic, by source environment, predicted functional genes, and potentially pathogenic taxa) varied between air types and route driven. Taxon reoccurrence was measured by taking the sum of the presence of a taxon in outdoor and indoor-transit samples and breaking them into equal thirds ranked by number of occurrences across all samples.
Shannon diversity (H) was used to calculate the diversity of STURLA classifications encountered (Shannon and Weaver, 1963). Richness of land cover was measured by taking the sum of the number of unique STURLA classes passed through on each route. These two metrics are used as proxies for how many STURLA classes (e.g., as one walks throughout a city) and which classes in a city are to be associated with microbial diversity. Dissimilarity in routes was calculated using the Bray-Curtis dissimilarity metric (Beals, 1984). Permutational correlations (to account for spatial and temporal autocorrelation) with Bonferroni correction were used to test for relationships between STURLA diversity and microbial structure/function using relative proportions of STURLA classes (as this accounts for differences in total driving time). A mantel test was employed to test for correlation between microbial beta diversity and STURLA dissimilarity.
Data Availability
DNA sequences have been deposited to the National Institute of Health Sequence Read Archive under accession number: SRR11612565. Code can be found at https://github.com/thecrobe/STURLAMicrobiome.
Results
Microbial Community Structure and Function
Molecular analysis from mobile monitoring revealed diverse communities of 337 amplicon sequence variants (ASVs, a proxy for species). Twenty two phyla were observed that varied significantly in community structure (Figure 4A, p = 0.001) and inferred functional potential (Figure 4B, p = 0.002) between outdoor and indoor-transit air as well as between outdoor route driven. Outdoor communities were seen to host reoccurring taxa less frequently than indoors (Supplementary Figure 1). A diverse set of metabolic genes were identified including carbon fixation, sulfate reduction/assimilation/oxidation, nitrate reduction, and aromatic compound degradation. No virulence genes were observed. Despite differences in composition and inferred functional potential, both indoor-transit, and outside air hosted reoccurring taxa similarly (p = 0.9631, Supplementary Figure 1) where the majority of ASVs in outdoor and indoor-transit were found in all samples. No phylum was unique to a single sample, air type, or route (Figure 4C) thus suggesting that the atmospheric microbiome in general is panmictic; however may vary between indoor and outdoor airs.
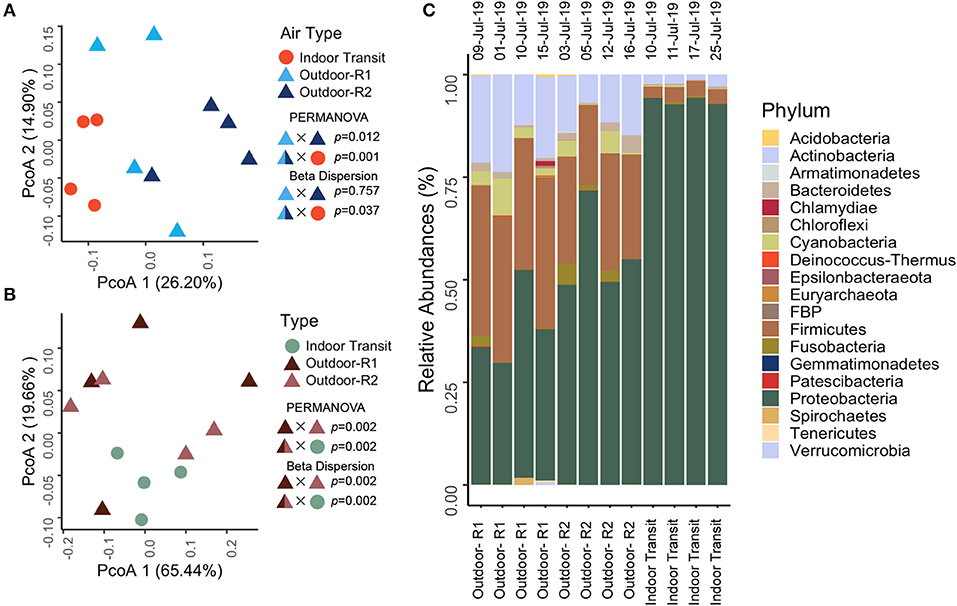
Figure 4. (A) Principal Coordinates Analysis (PcoA) of phylogenetic beta-diversity (between sites) using unweighted UnifFrac distances with air type colored by site and shaped by indoor (circle) and outdoor (triangle) air. Shape/Color X Shape/Color indicates the factors used in hypothesis testing with the test above and p-value to the right. (B) Principal Coordinates Analysis (PcoA) of inferred functional potential beta-diversity (between sites) using Bray-Curtis dissimilarity with air type colored by site (green: indoor transit, dark brown: outdoor route 1, light brown: outdoor route 2) and shaped by indoor (circle) and outdoor (triangle) air. Shape/Color X Shape/Color indicates the factors used in hypothesis testing with the test above and p-value to the right. It should be noted the split color is for visualization and statistical tests were conducted on outdoor ~ indoor samples. (C) Relative abundances of phyla identified in samples separated by air type (outdoor route 1 vs. outdoor route 2 vs. indoor transit) with collection date above each column.
Indoor air hosted almost double the number of rare taxa (49 ASVs), an ASV found in just one sample, than outdoor air (26 ASVs). Outdoor air hosted significantly more phylogenetic (p = 0.006, Figure 5A) and functionally diverse (p = 0.002, Figure 5B) communities as compared to indoor-transit air. Furthermore, outdoor communities were more rich (p = 0.045, Figure 5C), and evenly distributed (p = 0.007, Figure 5D). The 40 most abundant ASVs accounted for an average 86.01% (coefficient of variance: 11.06%) of all taxa across all samples were identified as likely originating from animals, soil, or were generalists (Figure 6A). Enterobacteriaceae (likely from mammalian gastro-intestinal systems) were on average the most abundant animal-associated microorganisms and were more variable outdoors (16.71 ± 15.01%) than indoors (90.5 ± 1.7%).
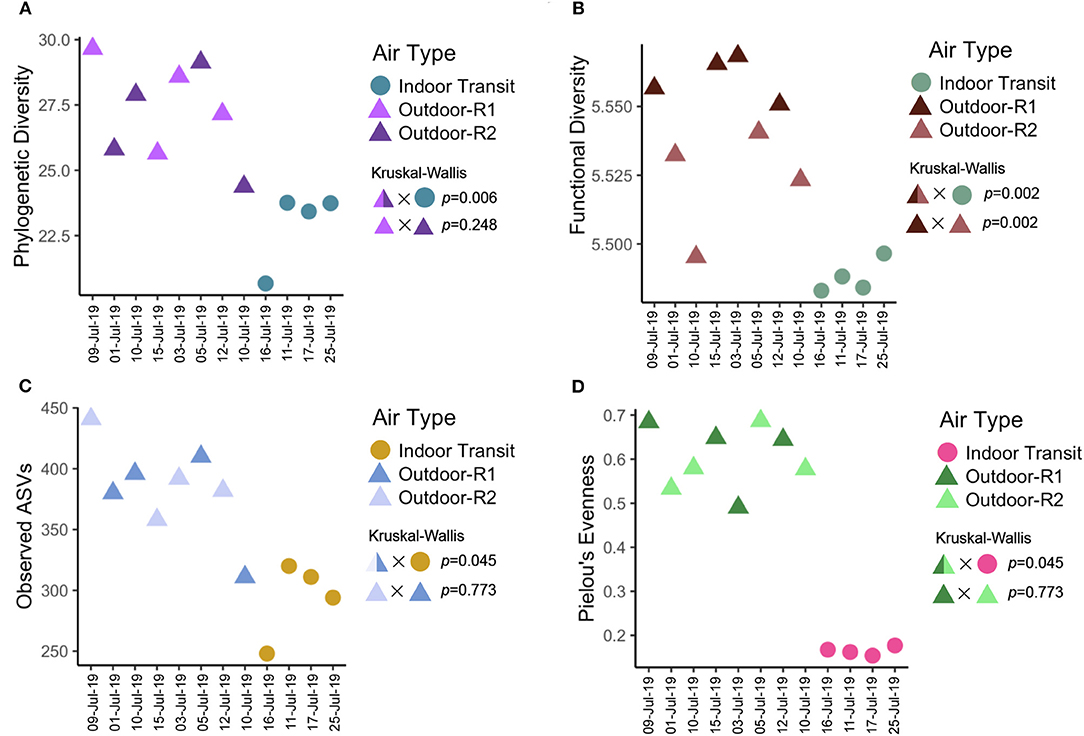
Figure 5. Alpha Diversity Metrics separated by air type (circle: indoor transit, triangle: outdoor) and route driven for outdoor samples (color) with date of collection on the x axis. Shape/Color X Shape/Color indicates the factors used in hypothesis testing with the test above and p-value to the right. It should be noted the split color is for visualization and statistical tests were conducted on outdoor ~ indoor samples. (A) Faith's phylogenetic diversity (PD). (B) Inferred functional diversity calculated using the Shannon index on PICRUSt functional pathways. (C) Observed number of ASVs. (D) Pielou's evenness metric.
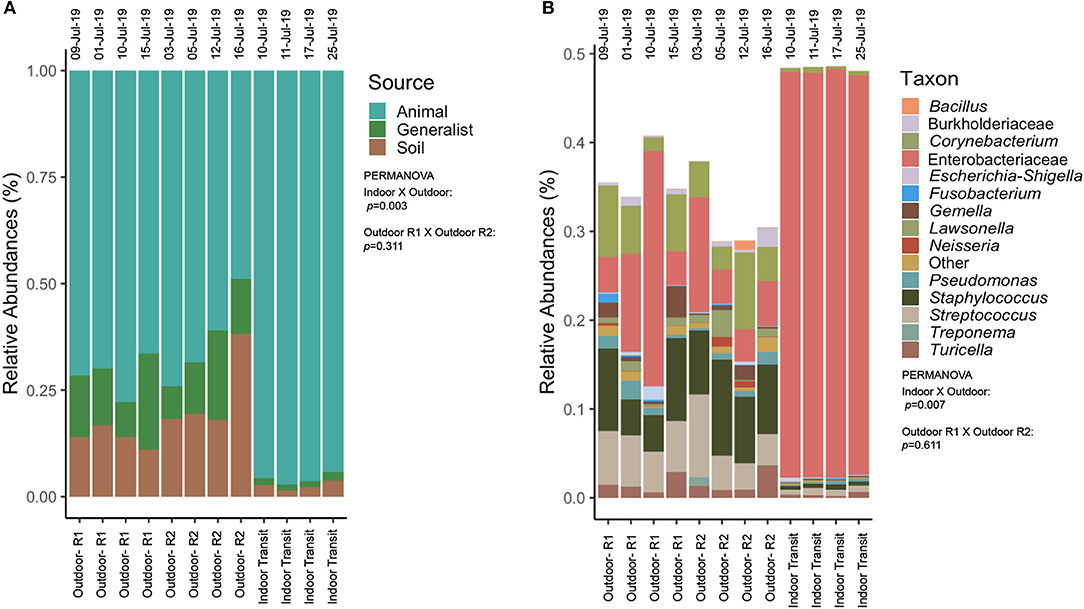
Figure 6. (A) Relative abundances of sources identified in samples separated by air type on the bottom (outdoor route 1 vs. outdoor route 2 vs. indoor transit) with collection date above each column. Hypothesis test results and factors are under the legend. (B) Relative abundances of potentially pathogenic taxa identified in samples separated by air type on the bottom (outdoor route 1 vs. outdoor route 2 vs. indoor transit) with collection date above each column. Taxa in abundance smaller than 0.01% were grouped in the category “other” for visualization only. Hypothesis test results and factors are under the legend.
While indoor transit air was dominated by Enterobacteriaceae, the skin associated genus Staphylococcus (mean: 14.22%, coefficient of variance: 30.62%) made notable contributions to the potentially pathogenic fraction of outdoor air communities and was more variable in abundance across samples as compared to indoors (mean: 0.01%, coefficient of variance: 18.90%). Plant pathogen containing genus Ralstonia represented the most abundant taxa sourced to soil and were in greater abundance and less variable outdoors (mean: 17.56%, coefficient of variance: 9.48%) than indoors (mean: 2.49%, coefficient of variance 39.15%). Lastly, the most abundant generalist ASVs were identified as the Oxyphotobacteria, the only group of bacteria that can carry out oxygenic photosynthesis, were also more abundant and variable outdoors (mean: 4.16%, coefficient of variance: 82.73% than indoors (mean: 0.24%, coefficient of variance: 70.87%). Taxonomic composition by source environment significantly differed from outdoor and indoor-transit air (p = 0.003) but not by route driven (p = 0.311) Proportions of source environments were not uniform between outdoor and indoor-transit air as the most abundant ASVs in outdoor air were attributed to more evenly distributed source environments.
A total of 30 Amplicon Sequence Variants (ASVs) were identified as being potentially pathogenic where, on average, these taxa composed (mean: 33.89%, coefficient of variance: 19.0%) of outdoor air and (mean: 48.41%, coefficient of variance 21.1%) of indoor-transit microbial communities (Figure 6B). The majority of these taxa are commensal human bacteria that are also opportunistic pathogens. Corynebacterium spp., and Pseudomonas spp., were absent in indoor-transit air. Structure and taxonomic composition of potentially pathogenic taxa was significantly different between indoor-transit and outdoor air (p = 0.007) but not between route driven outdoors (p = 0.661).
The Three-Dimensional Landscape and Biodiversity
Classification of the urban landscape identified 42 STURLA classes encountered while driving with tgpl (trees, grass, pavement, lowrise buildings) being most frequent (Figure 2, larger maps found in Supplementary Figures 5–12). The majority of the urban landscape from routes driven was homogenous in class composition where 92.16 ± 1.43% was explained by 9 STURLA classes (Figure 3B). Despite this homogeneity, routes significantly differed (p = 0.0364) in STURLA classes encountered each day. Diversity of STURLA classes encountered was significantly correlated with phylogenetic diversity (Rho: 0.74, p = 0.045) and observed number of ASVs (Rho: 0.83, p = 0.011) but not with community evenness (Rho: 0.21, p = 0.620). We found that variation of the STURLA classes was significantly associated with beta diversity of microbial communities (Mantel Test, r: 0.341, p = 0.04) At the individual STURLA class level, inferred functional diversity was significantly correlated with tgpl, tgplmh, other (all p < 0.05) and tgp, tgwp, tgbp, (all p < 0.1) (Figure 7) as was the number of observed ASVs and phylogenetic diversity with tgpl and tgplmh. Furthermore, diversity of STURLA classes by day was strongly, albeit insignificantly, correlated with 14 metabolic pathways (Supplementary Figure 2), 25 biosynthesis pathways (Supplementary Figure 3), and 10 degradation pathways (Supplementary Figure 4). Class richness was also correlated with 3 metabolic pathways, 9 biosynthesis pathways, and 4 degradation pathways.
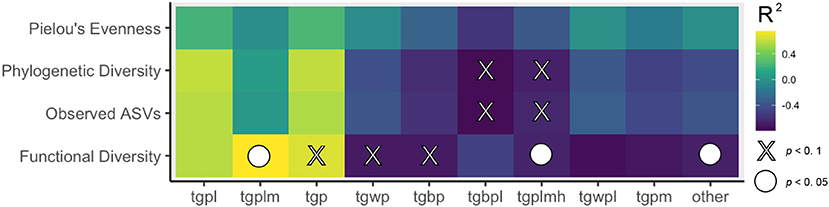
Figure 7. A. Heatmap of Permutational correlation coefficients where lighter colors indicate magnitude of the correlation. X indicates a statistically significant p-value after Bonferroni correction. STURLA Code elements: t, trees; g, grass; p, pavement; b, bare; w, water; l, lowrise; m, midrise; h, highrise.
Discussion
The high level of diversity observed in outdoor particulate matter is likely attributed to the unrestricted dispersal by wind at both the local (within-city) (Pankhurst et al., 2012) and intercontinental scales (Smith et al., 2013). This diversity may also be a function of high temporal variation at short time scales (i.e., days) of microbial biodiversity previously found in the atmospheric microbiome (Stewart et al., 2020b) and should be considered when interpreting these results. This is attributed to sampling across diverse landscapes that included water features, greenspace, unpaved land as well as a dense urban core consisting of the built environment where humans and animal companions primarily walk and drive outside. For atmospheric communities it is expected that dispersal is the dominating process affecting community assembly as opposed to diversification, selection, and drift (Vellend, 2010; Nemergut et al., 2013). We also found a relatively small number of ASVs as compared to other environments (e.g., soil); however, this is consistent with estimates of atmospheric microbial biodiversity that has found 29–690 phylotypes pers sample (Bowers et al., 2013). Our finding that communities host reoccurring taxa over time in both outdoor and indoor-transit air suggests that atmospheric microorganisms are not endemic and have cosmopolitan distributions in their environment, likely a function of the large populations of microorganisms combined with their small size (Wilkinson et al., 2012). Despite this, the significant differences in community composition by air type also suggests that the mixing is dispersal limited (e.g., greater mixing outside as opposed to a largely enclosed built environment). When outdoors, aerosolized microbes are blocked by buildings, trees, and everything in the urban landscape; however they have a wider possible geographic range (a function of wind dispersal) than indoors. For example, a microbe aerosolized from a plant leaf can move in all directions, including vertically where it could be lifted and transported long distances (Smith et al., 2013). Being able to across wide geographic ranges allows endemic microbes to escape their native geographic ranges. While inside a building such as a subway or train station wind speeds do not reach levels found outdoors. Likewise, indoor microbes have an obstacle, exits/entrances. The microorganisms would have to exit the system before it could enter outdoor air.
Furthermore, in semi-closed environments such as indoor-transit systems, physical dispersal limitation (e.g., a pillar or turnstile) should increase residence time for microbes (Adams et al., 2013; Kembel et al., 2014) and influence microbial diversity. The closed environment (aside from exits) of the indoor-transit system combined with high human occupancy seems to enrich the taxa brought into the system by its riders as it does in other structures of the built environment (Meadow et al., 2014). It is expected to find bacteria disperse into and throughout the indoor-transit environment by three primary mechanisms: (1) contact with indoor surfaces; (2) particle emission from breath, clothes, skin and hair; and (3) resuspension of dust containing other microbial-loaded particles (Meadow et al., 2015). Given that humans carry 1012 microorganisms on the epidermis and 1014 microorganisms in the alimentary tract (Luckey, 1972) and shed ~ 106 particles (>0.5 μm in diameter) an hour (You et al., 2013; Bhangar et al., 2016) we likely serve as the dominant source of microorganisms in indoor-transit air.
Complementing our findings of higher proportions of animal-associated taxa in indoor-transit air, it has been seen that human occupancy increases the load of animal-associated bacteria in the built environment. This includes subways (Leung et al., 2014; Hsu et al., 2016) university classrooms (Hospodsky et al., 2012; Meadow et al., 2014), public restrooms (Flores et al., 2011), dust (Täubel et al., 2009), and kitchens (Flores et al., 2013). Outdoor air microbes also seem to enter into the transit system, albeit to a lesser extent, given there were no unique phyla identified outside that were not encountered in transit air. Furthermore, architectural design (Kembel et al., 2012, 2014) has been seen to influence microbial community structure and acts as a physical barrier differentiating outdoor vs. indoor-transit air which may account for observed variation in diversity in this study.
High human occupancy in small spaces such as train cars combined with our finding of almost half of the microbiome being potentially pathogenic may alter the health state of the lung and respiratory tract microbiome. Despite the lack of specific inferred virulence genes, shifts in microbial composition can have negative health effects as an emergent property. A decrease in microbial diversity, richness, and evenness, as we found in indoor-transit air compared to outdoor air, are frequent features of chronic lung inflammation (Hilty et al., 2010; You et al., 2013). The presence of microbial derived molecules influences immune systems through Toll-like receptor recognition even when a microbe is dead. These molecules include bacterial lipopolysaccharide (Faure et al., 2000; Ibeagha-Awemu et al., 2008), flagellin (Hayashi et al., 2001), and lipoproteins (Takeuchi et al., 2001). Thus, dead microbes that lack pathogenicity genes may still have negative health outcomes upon inhalation by activating the immune system. Previous research has demonstrated that ~80% of particulate matter (PM2.5) in subway systems deposits in human respiratory tract and biologically stresses the immune system and cultured lung cells (Karlsson et al., 2005; Bachoual et al., 2007). This combined with the high percentages of potentially pathogenic taxa in indoor-transit air may have negative health effects on those with unstable lung microbial communities such as individuals who have a recurring cough, mechanically disrupting microbes, or have decreased diversity due to antibiotic use (Klepac-Ceraj et al., 2010; Zhao et al., 2012). In contrast, the lower proportions of potentially pathogenic taxa and relatively higher diversity of outdoor air (Ege et al., 2011; Lynch et al., 2014) has been linked to decreased rates of asthma. The influence of outdoor air may be less than that of the indoor-transit system as 90% of urban individuals spend their time indoors (Klepeis et al., 2001).
One possible benefit of spending time outdoors aside from aesthetics and recreation (Soga and Gaston, 2016) may be improving respiratory health. It should be noted that these are suggestive findings that would benefit from empirical measurements from qPCR or other functional analyses. Effects of microbial presence in the atmosphere on respiratory tract colonization is currently unknown; however, it is suggested to be largely determined by processes including birth, death, immigration, and emigration (Whiteson et al., 2014; Willis et al., 2020). Recent studies have also observed relationships between post-mortem microbial biodiversity on the human body (e.g., nose, rectum, and eyes) that suggest urban inhabitants are influenced by their local atmospheric microbiome (Pearson et al., 2019, 2020) and population demographics (Pechal et al., 2018). Likewise, black carbon (Janssen et al., 2011), particulate matter (Dockery et al., 1993), and other pollutants may play a role in counteracting the benefits of microbial exposure.
While it is known that urban and rural/agricultural air host distinct microbial communities (Shaffer and Lighthart, 1997; Bowers et al., 2011a; Leung et al., 2014; Stewart et al., 2020b) the role of within city variation where there are less obvious contrasts in landscape structure is largely unstudied.
Likewise, understanding the biogeography of microbial communities in cities offers a way to investigate colonization of urban residents by microbes. Recent studies investigating these relationships have shown that microbial diversity of deceased individuals is significantly and positively correlated with greenness (Pearson et al., 2019, 2020). While this study does not investigate individual human microbiomes, we do find the opposite relationships with classes that host greenness, specifically tgbpl and tgplmh. Both of these STURLA classes host greenness (t) and the interaction between greenspace and other urban elements (l, m, and h) suggest that relationships between urban structure and atmospheric microbial biodiversity are an emergent property of the built and natural environment. Likewise as trees across the majority of Philadelphia's urban landscape (tgpl) measuring greenness alone does not account for the true variation in the 3D landscape.
More associations between biodiversity and urban structure were seen with STURLA diversity as opposed to STURLA richness, suggesting the composition of heterogeneous 3D landscapes has greater influence on microbiome composition than the number of times the same landscape is encountered throughout a day. Likewise more correlations with urban structure were observed with inferred function genes which suggests that STURLA classes may host similar taxa phylogenetically and taxonomically but be tailored to specific functions. This may be a result of environmental filtering of microbial communities on the land (Lauber et al., 2013) below the atmosphere that are then aerosolized. Further investigations of microbial biodiversity in the atmosphere above and on the land within STURLA classes could provide urban planners with buildable units of land to increase functional diversity (Allison and Martiny, 2008) and urban resilience (Gómez-Baggethun and Barton, 2013; Jansson, 2013; McPhearson et al., 2016). For example, significant positive correlations between inferred functional diversity and STURLA class tgplm were observed while a negative relationship was found with tgplmh. This research provides further evidence that biodiversity is influenced by urban structure composed of the built and natural environment (Pearson et al., 2019). It should be noted that the sampling was heterogenous as samples were collected while stationary (e.g., stoplight) as well as when moving (e.g., city streets and highways) as well as the density of cars and people on the streets. These findings suggest that different structures of the urban landscape host taxonomically and functionally distinct microbiomes in their aerosols.
In a rapidly urbanizing global community, humans spend the majority of their time in urban landscapes of varying landscape structure, density and configuration. While both outdoors and in transit systems, we constantly emit and interact with microbes sourced from diverse environments that may influence our well-being. In this study we found that microbial communities vary between outdoor and indoor-transit air with the former being more diverse and associated with the structure of the urban landscape. Likewise indoor-transit systems hosted largely homogenous communities with higher proportions of potentially pathogenic taxa likely emitted from animals. Limitations include not analyzing the fungal and viral fractions of atmospheric particles, use of inferred functional pathways instead of directly measuring gene expression or metagenomes (Steele and Streit, 2005), and testing the PM2.5 subset of the atmospheric particles as opposed to total suspended particles (TSP). Likewise, sampling efficiency/length/speed, local pollutant conditions inhibiting molecular analyses and seasonality may be latent factors influencing our results. Future studies may consider exploring the variations of relationships to microbial diversity within a STURLA class as test the influence of within-season and between-season variation.
Data Availability Statement
The datasets presented in this study can be found in online repositories. The names of the repository/repositories and accession number(s) can be found in the article/ Supplementary Material.
Author Contributions
JS and PK: conceptualization, sample collection, data analysis, and writing. AS and MC: sample collection, writing. KS and PK: sample collection, data analysis, writing, funding. All authors contributed to the article and approved the submitted version.
Funding
This research was supported by NSF Grant 1832407—Funding for urban ecosystem service research.
Conflict of Interest
The authors declare that the research was conducted in the absence of any commercial or financial relationships that could be construed as a potential conflict of interest.
Acknowledgments
This manuscript has been released as a preprint at Biorxiv under doi: 10.1101/2020.06.17.157651 (Stewart et al., 2020a). We appreciate the help from Gerardo Bandera and Jennifer Santoro for analyses and Radley Reist for sample collection. Collina Strada's sustainable fashion line is credited with colors used in graphics. We thank the editor and two reviewers for making this a stronger manuscript.
Supplementary Material
The Supplementary Material for this article can be found online at: https://www.frontiersin.org/articles/10.3389/fevo.2021.620461/full#supplementary-material
References
Adams, R. I., Miletto, M., Taylor, J. W., and Bruns, T. D. (2013). Dispersal in microbes: fungi in indoor air are dominated by outdoor air and show dispersal limitation at short distances. ISME J. 7, 1262–1273. doi: 10.1038/ismej.2013.28
Allison, S. D., and Martiny, J. B. H. (2008). Resistance, resilience, and redundancy in microbial communities. Proc. Natl. Acad. Sci. U.S.A. 105, 11512–11519. doi: 10.1073/pnas.0801925105
Bachoual, R., Boczkowski, J., Goven, D., Amara, N., Tabet, L., On, D., et al. (2007). Biological effects of particles from the paris subway system. Chem. Res. Toxicol. 20:1426–1433. doi: 10.1021/tx700093j
Beals, E. W. (1984). “Bray-curtis ordination: an effective strategy for analysis of multivariate ecological data,” in Advances in Ecological Research, eds. A. MacFadyen and E. D. Ford (London: Academic Press), 1-55. doi: 10.1016/S0065-2504(08)60168-3
Bhangar, S., Adams, R. I., Pasut, W., Huffman, J. A. (2016). Chamber bioaerosol study: human emissions of size-resolved fluorescent biological aerosol particles. Indoor. 26:193–206. doi: 10.1111/ina.12195
Bolyen, E., Rideout, J. R., Dillon, M. R., Bokulich, N. A., Abnet, C. C., Al-Ghalith, G. A., et al. (2019). Author correction: reproducible, interactive, scalable and extensible microbiome data science using QIIME 2. Nat. Biotechnol. 37:1091. doi: 10.1038/s41587-019-0252-6
Bowers, R. M., Clements, N., Emerson, J. B., Wiedinmyer, C., Hannigan, M. P., and Fierer, N. (2013). Seasonal variability in bacterial and fungal diversity of the near-surface atmosphere. Environ. Sci. Technol. 47, 12097–12106. doi: 10.1021/es402970s
Bowers, R. M., Lauber, C. L., Wiedinmyer, C., Hamady, M., Hallar, A. G., Fall, R., et al. (2009). Characterization of airborne microbial communities at a high-elevation site and their potential to act as atmospheric ice nuclei. Appl. Environ. Microbiol. 75, 5121–5130. doi: 10.1128/AEM.00447-09
Bowers, R. M., McLetchie, S., Knight, R., and Fierer, N. (2011a). Spatial variability in airborne bacterial communities across land-use types and their relationship to the bacterial communities of potential source environments. ISME J. 5, 601–612. doi: 10.1038/ismej.2010.167
Bowers, R. M., Sullivan, A. P., Costello, E. K., Collett Jr, J. L., Knight, R., and Fierer, N. (2011b). Sources of bacteria in outdoor air across cities in the midwestern United States. Appl. Environ. Microbiol. 77, 6350–6356. doi: 10.1128/AEM.05498-11
Burrows, S. M., Elbert, W., Lawrence, M. G., and Pöschl, U. (2009). Bacteria in the global atmosphere - Part 1: Review and synthesis of literature data for different ecosystems. Atmos. Chem. Phys. 9, 9263–9280. doi: 10.5194/acp-9-9263-2009
Cadenasso, M. L., Pickett, S. T. A., and Schwarz, K. (2007). Spatial heterogeneity in urban ecosystems: reconceptualizing land cover and a framework for classification. Front. Ecol. Environ. 5, 80–88. doi: 10.1890/1540-9295(2007)5[80:SHIUER]2.0.CO;2
Cáliz, J., Triadó-Margarit, X., Camarero, L., and Casamayor, E. O. (2018). A long-term survey unveils strong seasonal patterns in the airborne microbiome coupled to general and regional atmospheric circulations. Proc. Natl. Acad. Sci. U.S.A. 115, 12229–12234. doi: 10.1073/pnas.1812826115
Callahan, B. J., McMurdie, P. J., Rosen, M. J., Han, A. W., Johnson, A. J. A., and Holmes, S. P. (2016). DADA2: High-resolution sample inference from Illumina amplicon data. Nat. Methods 13, 581–583. doi: 10.1038/nmeth.3869
Chate, D. M., Rao, P. S. P., Naik, M. S., Momin, G. A., Safai, P. D., and Ali, K. (2003). Scavenging of aerosols and their chemical species by rain. Atmos. Environ. 37, 2477–2484. doi: 10.1016/S1352-2310(03)00162-6
Deshmukh, P., Kimbrough, S., Krabbe, S., Logan, R., Isakov, V., and Baldauf, R. (2020). Identifying air pollution source impacts in urban communities using mobile monitoring. Sci. Total Environ. 715:136979. doi: 10.1016/j.scitotenv.2020.136979
Dietze, M. C., Fox, A., Beck-Johnson, L. M., Betancourt, J. L., Hooten, M. B., Jarnevich, C. S., et al. (2018). Iterative near-term ecological forecasting: Needs, opportunities, and challenges. Proc. Natl. Acad. Sci. U.S.A. 115:1424–1432. doi: 10.1073/pnas.1710231115
Dockery, D. W., Pope, C. A., Xu, X., Spengler, J. D., Ware, J. H., Fay, M. E., et al. (1993). An association between air pollution and mortality in six U.S. cities. N. Engl. J. Med. 329, 1753–1759. doi: 10.1056/NEJM199312093292401
Douglas, G. M., Maffei, V. J., Zaneveld, J., Yurgel, S. N., Brown, J. R., Taylor, C. M., et al. (2019). PICRUSt2: An improved and extensible approach for metagenome inference. bioRxiv. doi: 10.1101/672295
Du, P., Du, R., Lu, Z., Ren, W., and Fu, P. (2018). Variation of bacterial and fungal community structures in PM2.5 collected during the 2014 APEC Summit Periods. Aerosol Air Qual. Res. 18, 444–455. doi: 10.4209/aaqr.2017.07.0238
Dybwad, M., Granum, P. E., Bruheim, P., and Blatny, J. M. (2012). Characterization of airborne bacteria at an underground subway station. Appl. Environ. Microbiol. 78, 1917–1929. doi: 10.1128/AEM.07212-11
Earth Microbiome Project (2016). 16S PCR Protocol. Available online at: https://phylogenomics.me/protocols/16s-pcr-protocol/ [Accessed November 19, 2018].
Ege, M. J., Mayer, M., Normand, A.-C., Genuneit, J., Cookson, W. O. C. M., Braun-Fahrländer, C., et al. (2011). Exposure to environmental microorganisms and childhood asthma. N. Engl. J. Med. 364, 701–709. doi: 10.1056/NEJMoa1007302
Faith, D. P. (1992). Conservation evaluation and phylogenetic diversity. Biol. Conserv. 61, 1–10. doi: 10.1016/0006-3207(92)91201-3
Faure, E., Equils, O., Sieling, P. A., Thomas, L., Zhang, F. X., Kirschning, C. J., et al. (2000). Bacterial lipopolysaccharide activates NF-κB through toll-like receptor 4 (TLR-4) in cultured human dermal endothelial cells. Differential expression of TLR-4 and TLR-2 in endothelial cells. J. Biol. Chem. 275:11058–11063. doi: 10.1074/jbc.275.15.11058
Flores, G. E., Bates, S. T., Caporaso, J. G., Lauber, C. L., Leff, J. W., Knight, R., et al. (2013). Diversity, distribution and sources of bacteria in residential kitchens. Environ. Microbiol. 15, 588–596. doi: 10.1111/1462-2920.12036
Flores, G. E., Bates, S. T., Knights, D., Lauber, C. L., Stombaugh, J., Knight, R., et al. (2011). Microbial biogeography of public restroom surfaces. PLoS One 6, e28132. doi: 10.1371/journal.pone.0028132
Folke, C., Carpenter, S., Elmqvist, T., Gunderson, L., Holling, C. S., and Walker, B. (2002). Resilience and sustainable development: building adaptive capacity in a world of transformations. Ambio 31, 437–440. doi: 10.1579/0044-7447-31.5.437
Frank, D. N., St. Amand, A. L., Feldman, R. A., Boedeker, E. C., Harpaz, N., and Pace, N. R. (2007). Molecular-phylogenetic characterization of microbial community imbalances in human inflammatory bowel diseases. Proc. Natl. Acad. Sci. U.S.A. 104, 13780–13785. doi: 10.1073/pnas.0706625104
Gohli, J., Bøifot, K. O., Moen, L. V., Pastuszek, P., Skogan, G., Udekwu, K. I., et al. (2019). The subway microbiome: seasonal dynamics and direct comparison of air and surface bacterial communities. Microbiome 7, 160. doi: 10.1186/s40168-019-0772-9
Gómez-Baggethun, E., and Barton, D. N. (2013). Classifying and valuing ecosystem services for urban planning. Ecol. Econ. 86, 235–245. doi: 10.1016/j.ecolecon.2012.08.019
Grimm, N. B., Faeth, S. H., Golubiewski, N. E., Redman, C. L., Wu, J., Bai, X., et al. (2008). Global change and the ecology of cities. Science. 319, 756–760. doi: 10.1126/science.1150195
Gunderson, L. H., Allen, C. R., and Holling, C. S. (2012). Foundations of Ecological Resilience. London; Washington, DC; Covelo, CA: Island Press. Available online at: https://books.google.nl/books?hl=en&lr=&id=C0q7hXOGuY8C&oi=fnd&pg=PR5&dq=foundations+of+ecological+resilience&ots=6VyyXpD98C&sig=dlUCBuRmqEpY61KUfi_JAZIOUHY#v=onepage&q=foundations%20of%20ecological%20resilience&f=false
Haase, D., Larondelle, N., Andersson, E., Artmann, M., Borgström, S., Breuste, J., et al. (2014). A quantitative review of urban ecosystem service assessments: concepts, models, and implementation. Ambio 43, 413–433. doi: 10.1007/s13280-014-0504-0
Hamstead, Z. A., Kremer, P., Larondelle, N., McPhearson, T., and Haase, D. (2016). Classification of the heterogeneous structure of urban landscapes (STURLA) as an indicator of landscape function applied to surface temperature in New York City. Ecol. Indic. 70, 574–585. doi: 10.1016/j.ecolind.2015.10.014
Hanski, I., von Hertzen, L., Fyhrquist, N., Koskinen, K., Torppa, K., Laatikainen, T., et al. (2012). Environmental biodiversity, human microbiota, and allergy are interrelated. Proc. Natl. Acad. Sci. U.S.A. 109, 8334–8339. doi: 10.1073/pnas.1205624109
Hayashi, F., Smith, K. D., Ozinsky, A., Hawn, T. R., Yi, E. C., Goodlett, D. R., et al. (2001). The innate immune response to bacterial flagellin is mediated by Toll-like receptor 5. Nature. 410:1099–1103. doi: 10.1038/35074106
Hilty, M., Burke, C., Pedro, H., Cardenas, P., Bush, A., Bossley, C., et al. (2010). Disordered microbial communities in asthmatic airways. PLoS ONE 5:e8578. doi: 10.1371/journal.pone.0008578
Hospodsky, D., Qian, J., Nazaroff, W. W., Yamamoto, N., Bibby, K., Rismani-Yazdi, H., et al. (2012). Human occupancy as a source of indoor airborne bacteria. PLoS ONE 7:e34867. doi: 10.1371/journal.pone.0034867
Hsu, T., Joice, R., Vallarino, J., Abu-Ali, G., Hartmann, E. M., Shafquat, A., et al. (2016). Urban transit system microbial communities differ by surface type and interaction with humans and the environment. mSystems 1:16. doi: 10.1128/mSystems.00018-16
Ibeagha-Awemu, E. M., Lee, J. W., Ibeagha, A. E., Bannerman, D. D., Paape, M. J., and Zhao, X. (2008). Bacterial lipopolysaccharide induces increased expression of toll-like receptor (TLR) 4 and downstream TLR signaling molecules in bovine mammary epithelial cells. Vet. Res. 39:11. doi: 10.1051/vetres:2007047
Janssen, N. A. H., Hoek, G., Simic-Lawson, M., Fischer, P., van Bree, L., ten Brink, H., et al. (2011). Black carbon as an additional indicator of the adverse health effects of airborne particles compared with PM10 and PM2.5. Environ. Heal. Perspect. 119, 1691–1699. doi: 10.1289/ehp.1003369
Jansson, Å. (2013). Reaching for a sustainable, resilient urban future using the lens of ecosystem services. Ecol. Econ. 86, 285–291. doi: 10.1016/j.ecolecon.2012.06.013
Karlsson, H. L., Nilsson, L., and Möller, L. (2005). Subway particles are more genotoxic than street particles and induce oxidative stress in cultured human lung cells. Chem. Res. Toxicol. 18:19–23. doi: 10.1021/tx049723c
Katoh, K., and Standley, D. M. (2013). MAFFT multiple sequence alignment software version 7: improvements in performance and usability. Mol. Biol. Evol. 30, 772–780. doi: 10.1093/molbev/mst010
Kembel, S. W., Jones, E., Kline, J., Northcutt, D., Stenson, J., Womack, A. M., et al. (2012). Architectural design influences the diversity and structure of the built environment microbiome. ISME J. 6, 1469–1479. doi: 10.1038/ismej.2011.211
Kembel, S. W., Meadow, J. F., O'Connor, T. K., Mhuireach, G., Northcutt, D., Kline, J., et al. (2014). Architectural design drives the biogeography of indoor bacterial communities. PLoS ONE 9:e87093. doi: 10.1371/journal.pone.0087093
Klepac-Ceraj, V., Lemon, K. P., Martin, T. R., Allgaier, M., Kembel, S. W., Knapp, A. A., et al. (2010). Relationship between cystic fibrosis respiratory tract bacterial communities and age, genotype, antibiotics and Pseudomonas aeruginosa. Environ. Microbiol. 12, 1293–1303. doi: 10.1111/j.1462-2920.2010.02173.x
Klepeis, N. E., Nelson, W. C., Ott, W. R., Robinson, J. P., Tsang, A. M., Switzer, P., et al. (2001). The National human activity pattern survey (NHAPS): a resource for assessing exposure to environmental pollutants. J. Expo. Anal. Environ. Epidemiol. 11, 231–252. doi: 10.1038/sj.jea.7500165
Larondelle, N., Hamstead, Z. A., Kremer, P., Haase, D., and McPhearson, T. (2014). Applying a novel urban structure classification to compare the relationships of urban structure and surface temperature in Berlin and New York City. Appl. Geogr. 53, 427–437. doi: 10.1016/j.apgeog.2014.07.004
Lauber, C. L., Ramirez, K. S., Aanderud, Z., Lennon, J., and Fierer, N. (2013). Temporal variability in soil microbial communities across land-use types. ISME J. 7, 1641–1650. doi: 10.1038/ismej.2013.50
Lee, S., Choi, B., Yi, S. M., and Ko, G. P. (2009). Characterization of microbial community during Asian dust events in Korea. Sci. Total Environ. 407, 5308–5314. doi: 10.1016/j.scitotenv.2009.06.052
Leung, M. H. Y., Wilkins, D., Li, E. K. T., Kong, F. K. F., and Lee, P. K. H. (2014). Indoor-air microbiome in an urban subway network: diversity and dynamics. Appl. Environ. Microbiol. 80, 6760–6770. doi: 10.1128/AEM.02244-14
Li, H., Zhou, X.-Y., Yang, X.-R., Zhu, Y.-G., Hong, Y.-W., and Su, J.-Q. (2019). Spatial and seasonal variation of the airborne microbiome in a rapidly developing city of China. Sci. Total Environ. 665, 61–68. doi: 10.1016/j.scitotenv.2019.01.367
Linz, A. M., Crary, B. C., Shade, A., Owens, S., Gilbert, J. A., Knight, R., et al. (2017). Bacterial community composition and dynamics spanning five years in freshwater bog lakes. mSphere 2:17. doi: 10.1128/mSphere.00169-17
Louca, S., and Doebeli, M. (2018). Efficient comparative phylogenetics on large trees. Bioinformatics 34, 1053–1055. doi: 10.1093/bioinformatics/btx701
Lozupone, C., and Knight, R. (2005). UniFrac: a new phylogenetic method for comparing microbial communities. Appl. Environ. Microbiol. 71, 8228–8235. doi: 10.1128/AEM.71.12.8228-8235.2005
Luckey, T. D. (1972). Introduction to intestinal microecology. Am. J. Clin. Nutr. 25, 1292–1294. doi: 10.1093/ajcn/25.12.1292
Lymperopoulou, D. S., Adams, R. I., and Lindow, S. E. (2016). Contribution of vegetation to the microbial composition of nearby outdoor air. Appl. Environ. Microbiol. 82, 3822–3833. doi: 10.1128/AEM.00610-16
Lynch, S. V., Wood, R. A., Boushey, H., Bacharier, L. B., Bloomberg, G. R., Kattan, M., et al. (2014). Effects of early-life exposure to allergens and bacteria on recurrent wheeze and atopy in urban children. J. Allergy Clin. Immunol. 134, 593-601.e12. doi: 10.1016/j.jaci.2014.04.018
Mazar, Y., Cytryn, E., Erel, Y., and Rudich, Y. (2016). Effect of dust storms on the atmospheric microbiome in the Eastern Mediterranean. Environ. Sci. Technol. 50, 4194–4202. doi: 10.1021/acs.est.5b06348
McPhearson, T., Pickett, S. T. A. A., Grimm, N. B., Niemelä, J., Alberti, M., Elmqvist, T., et al. (2016). Advancing urban ecology toward a science of cities. Bioscience 2016:biw002. doi: 10.1093/biosci/biw002
Meadow, J. F., Altrichter, A. E., Bateman, A. C., Stenson, J., Brown, G. Z., Green, J. L., et al. (2015). Humans differ in their personal microbial cloud. PeerJ. 3:e1258. doi: 10.7717/peerj.1258
Meadow, J. F., Altrichter, A. E., Kembel, S. W., Kline, J., Mhuireach, G., Moriyama, M., et al. (2014). Indoor airborne bacterial communities are influenced by ventilation, occupancy, and outdoor air source. Indoor Air 24, 41–48. doi: 10.1111/ina.12047
Methé, B. A., Hiorns, W. D., and Zehr, J. P. (1998). Contrasts between marine and freshwater bacterial community composition: Analyses of communities in Lake George and six other Adirondack lakes. Limnol. Ocean. 43, 368–374. doi: 10.4319/lo.1998.43.2.0368
Mhuireach, G. Á., Betancourt-Román, C. M., Green, J. L., and Johnson, B. R. (2019). Spatiotemporal controls on the urban aerobiome. Front. Ecol. Evol. 7:43. doi: 10.3389/fevo.2019.00043
Mills, J. G., Weinstein, P., Gellie, N. J. C., Weyrich, L. S., Lowe, A. J., and Breed, M. F. (2017). Urban habitat restoration provides a human health benefit through microbiome rewilding: the Microbiome Rewilding Hypothesis. Restor. Ecol. 25, 866–872. doi: 10.1111/rec.12610
Nemergut, D. R., Schmidt, S. K., Fukami, T., O'Neill, S. P., Bilinski, T. M., Stanish, L. F., et al. (2013). Patterns and processes of microbial community assembly. Microbiol. Mol. Biol. Rev. 77, 342–356. doi: 10.1128/MMBR.00051-12
Pan, Y., Pan, X., Xiao, H., and Xiao, H. (2019). Structural characteristics and functional implications of PM2.5 bacterial communities during fall in Beijing and Shanghai, China. Front. Microbiol. 10:2369. doi: 10.3389/fmicb.2019.02369
Pankhurst, L. J., Whitby, C., Pawlett, M., Larcombe, L. D., McKew, B., Deacon, L. J., et al. (2012). Temporal and spatial changes in the microbial bioaerosol communities in green-waste composting. FEMS Microbiol. Ecol. 79, 229–239. doi: 10.1111/j.1574-6941.2011.01210.x
Pearson, A. L., Pechal, J., Lin, Z., Benbow, M. E., Schmidt, C., and Mavoa, S. (2020). Associations detected between measures of neighborhood environmental conditions and human microbiome diversity. Sci. Total Environ. 745:141029. doi: 10.1016/j.scitotenv.2020.141029
Pearson, A. L., Rzotkiewicz, A., Pechal, J. L., Schmidt, C. J., Jordan, H. R., Zwickle, A., et al. (2019). Initial evidence of the relationships between the human postmortem microbiome and neighborhood blight and greening efforts. Ann. Am. Assoc. Geogr. 109, 958–978. doi: 10.1080/24694452.2018.1519407
Pechal, J. L., Schmidt, C. J., Jordan, H. R., and Benbow, M. E. (2018). A large-scale survey of the postmortem human microbiome, and its potential to provide insight into the living health condition. Sci. Rep. doi: 10.1038/s41598-018-23989-w
Price, M. N., Dehal, P. S., and Arkin, A. P. (2009). FastTree: computing large minimum evolution trees with profiles instead of a distance matrix. Mol. Biol. Evol. 26, 1641–1650. doi: 10.1093/molbev/msp077
Quast, C., Pruesse, E., Yilmaz, P., Gerken, J., Schweer, T., Yarza, P., et al. (2013). The SILVA ribosomal RNA gene database project: improved data processing and web-based tools. Nucleic Acids Res. 41, D590–D596. doi: 10.1093/nar/gks1219
Reese, A. T., Savage, A., Youngsteadt, E., McGuire, K. L., Koling, A., Watkins, O., et al. (2016). Urban stress is associated with variation in microbial species composition-but not richness-in Manhattan. ISME J. 10, 751–760. doi: 10.1038/ismej.2015.152
Rook, G. A. W. (2009). Review series on helminths, immune modulation and the hygiene hypothesis: the broader implications of the hygiene hypothesis. Immunology 126, 3–11. doi: 10.1111/j.1365-2567.2008.03007.x
Round, J. L., and Mazmanian, S. K. (2009). The gut microbiota shapes intestinal immune responses during health and disease. Nat. Rev. Immunol. 9, 313–323. doi: 10.1038/nri2515
Ruokolainen, L., von Hertzen, L., Fyhrquist, N., Laatikainen, T., Lehtomäki, J., Auvinen, P., et al. (2015). Green areas around homes reduce atopic sensitization in children. Allergy 70, 195–202. doi: 10.1111/all.12545
Schaub, B., Liu, J., Höppler, S., Schleich, I., Huehn, J., Olek, S., et al. (2009). Maternal farm exposure modulates neonatal immune mechanisms through regulatory T cells. J. Allergy Clin. Immunol. 123:774–882.e5. doi: 10.1016/j.jaci.2009.01.056
Shaffer, B. T., and Lighthart, B. (1997). Survey of culturable airborne bacteria at four diverse locations in Oregon: Urban, rural, forest, and coastal. Microb. Ecol. 34, 167–177. doi: 10.1007/s002489900046
Shakya, K. M., Kremer, P., Henderson, K., McMahon, M., Peltier, R. E., Bromberg, S., et al. (2019). Mobile monitoring of air and noise pollution in Philadelphia neighborhoods during summer 2017. Environ. Pollut. 255:113195. doi: 10.1016/j.envpol.2019.113195
Shannon, C. E., and Weaver, W. (1963). The Mathematical Theory of Communication. (Univ. Illinois Press), 1–131.
Smith, D. J., Timonen, H. J., Jaffe, D. A., Griffin, D. W., Birmele, M. N., Perry, K. D., et al. (2013). Intercontinental dispersal of bacteria and archaea by transpacific winds. Appl. Environ. Microbiol. 79, 1134–1139. doi: 10.1128/AEM.03029-12
Soga, M., and Gaston, K. J. (2016). Extinction of experience: the loss of human-nature interactions. Front. Ecol. Environ. 14, 94–101. doi: 10.1002/fee.1225
Steele, H. L., and Streit, W. R. (2005). Metagenomics: advances in ecology and biotechnology. FEMS Microbiol. Lett. 247, 105–111. doi: 10.1016/j.femsle.2005.05.011
Stewart, J., Shakya, K. M., Bilinski, T., Wilson, J., Ravi, S., and Choi, C. S. (2020b). Variation of near surface atmosphere microbial communities at an urban and a suburban site in Philadelphia, PA, USA. Sci. Total Environ. 13:8353. doi: 10.1016/j.scitotenv.2020.138353
Stewart, J. D., Kremer, P., Shakya, K. M., Conway, M., and Saad, A. (2020a). Outdoor atmospheric microbial diversity is associated with urban landscape structure and differs from indoor-transit systems as revealed by mobile monitoring and three-dimensional spatial analysis. bioRxiv. doi: 10.1101/2020.06.17.157651
Takeuchi, O., Kawai, T., Mühlradt, P. F., Morr, M., Radolf, J. D., Zychlinsky, A., et al. (2001). Discrimination of bacterial lipoproteins by Toll-like recepttor 6. Int. Immunol. 13:933–940. doi: 10.1093/intimm/13.7.933
Täubel, M., Rintala, H., Pitkäranta, M., Paulin, L., Laitinen, S., Pekkanen, J., et al. (2009). The occupant as a source of house dust bacteria. J. Allergy Clin. Immunol. 124, 834–40.e47. doi: 10.1016/j.jaci.2009.07.045
Triadó-Margarit, X., Veillette, M., Duchaine, C., Talbot, M., Amato, F., Minguillón, M. C., et al. (2017). Bioaerosols in the Barcelona subway system. Indoor Air 27, 564–575. doi: 10.1111/ina.12343
Turnbaugh, P. J., Bäckhed, F., Fulton, L., and Gordon, J. I. (2008). Diet-induced obesity is linked to marked but reversible alterations in the mouse distal gut microbiome. Cell Host Microbe 3, 213–223. doi: 10.1016/j.chom.2008.02.015
Vellend, M. (2010). Conceptual synthesis in community ecology. Q. Rev. Biol. 85, 183–206. doi: 10.1086/652373
von Hertzen, L., Hanski, I., and Haahtela, T. (2011). Natural immunity. EMBO Rep. 12, 1089–1093. doi: 10.1038/embor.2011.195
Wallace, J., Corr, D., Deluca, P., Kanaroglou, P., and McCarry, B. (2009). Mobile monitoring of air pollution in cities: the case of Hamilton, Ontario, Canada. J. Environ. Monit. 11, 998–1003. doi: 10.1039/b818477a
Whiteson, K. L., Bailey, B., Bergkessel, M., Conrad, D., Delhaes, L., Felts, B., et al. (2014). The upper respiratory tract as a microbial source for pulmonary infections in cystic fibrosis: Parallels from island biogeography. Am. J. Respir. Crit. Care Med. 189:1309–1315. doi: 10.1164/rccm.201312-2129PP
Wilkinson, D. M., Koumoutsaris, S., Mitchell, E. A. D., and Bey, I. (2012). Modelling the effect of size on the aerial dispersal of microorganisms. J. Biogeogr. 39, 89–97. doi: 10.1111/j.1365-2699.2011.02569.x
Willis, K. A., Stewart, J. D., and Ambalavanan, N. (2020). Recent advances in understanding the ecology of the lung microbiota and deciphering the gut-lung. Axis. 319:L710–L716. doi: 10.1152/ajplung.00360.2020
Wu, Y., Liu, J., Zhai, J., Cong, L., Wang, Y., Ma, W., et al. (2018). Comparison of dry and wet deposition of particulate matter in near-surface waters during summer. PLoS ONE 13:e0199241. doi: 10.1371/journal.pone.0199241
You, R., Cui, W., Chen, C., and Zhao, B. (2013). Measuring the short-term emission rates of particles in the “personal cloud” with different clothes and activity intensities in a sealed chamber. Aerosol Air Qual. Res. 13:911e921. doi: 10.4209/aaqr.2012.03.0061
Keywords: urban structure, 16S, STURLA, mobile monitoring, microbiome
Citation: Stewart JD, Kremer P, Shakya KM, Conway M and Saad A (2021) Outdoor Atmospheric Microbial Diversity Is Associated With Urban Landscape Structure and Differs From Indoor-Transit Systems as Revealed by Mobile Monitoring and Three-Dimensional Spatial Analysis. Front. Ecol. Evol. 9:620461. doi: 10.3389/fevo.2021.620461
Received: 22 October 2020; Accepted: 05 January 2021;
Published: 02 February 2021.
Edited by:
Stephanie Ann Yarwood, University of Maryland, College Park, United StatesReviewed by:
Patrick K. H. Lee, City University of Hong Kong, Hong KongM. Eric Benbow, Michigan State University, United States
Copyright © 2021 Stewart, Kremer, Shakya, Conway and Saad. This is an open-access article distributed under the terms of the Creative Commons Attribution License (CC BY). The use, distribution or reproduction in other forums is permitted, provided the original author(s) and the copyright owner(s) are credited and that the original publication in this journal is cited, in accordance with accepted academic practice. No use, distribution or reproduction is permitted which does not comply with these terms.
*Correspondence: Justin D. Stewart, ai5kLnN0ZXdhcnRAdnUubmw=