- 1Instituto de Ecología A.C., Xalapa, Mexico
- 2Facultad de Biología, Universidad Veracruzana, Xalapa, Mexico
- 3Instituto de Biotecnología y Ecología Aplicada, Universidad Veracruzana, Xalapa, México
Frugivory interactions between birds and fruit-bearing plants are shaped by the abundance of its interacting species, their temporal overlap, the matching of their morphologies, as well as fruit and seed characteristics. Our study evaluates the role of seven factors of fruits and plants in determining the frequency of whole-fruit consumption by birds. We studied the frugivory network of a Neotropical periurban park in Xalapa, Veracruz, Mexico, and quantified relative abundance and phenology of birds and fruit, as well as fruit morphology, chromatic and achromatic contrast, and nutritional content. Using a maximum likelihood approach, we compared the observed interaction network with 62 single- and multiple-variable probabilistic models. Our network is composed of 11 plants and 17 birds involved in 81 frugivory interactions. This network is nested, modular, and relatively specialized. However, the frequency of pairwise interactions is not explained by the variables examined in our probabilistic models and found the null model has the best performance. This indicates that no single predictor or combination of them is better at explaining the observed frequency of pairwise interactions than the null model. The subsequent four top-ranking models, with ΔAIC values < 100, are single-variable ones: carbohydrate content, lipid content, chromatic contrast, and morphology. Two- and three-variable models show the poorest fit to observed data. The lack of a deterministic pattern does not support any of our predictions nor neutral- or niche-based processes shaping the observed pattern of fruit consumption in our interaction network. It may also mean that fruit consumption by birds in this periurban park is a random process. Although our study failed to find a pattern, our work exemplifies how investigations done in urban settings, poor in species and interactions, can help us understand the role of disturbance in the organization of frugivory networks and the processes governing their structure.
Introduction
In bird–plant frugivory interactions, certain fruit characteristics influence how they are selected, determine their consumption rate by birds, and ultimately rule how their networks are structured. Examples of such characteristics are nutritional content (Gautier-Hion et al., 1985; Jordano, 2000; Cazetta et al., 2012; Blendinger et al., 2015), fruit size, seed quantity, and seed size (Wheelwright, 1985), and detectability as determined by their color (Van der Pijl, 1982; Schaefer and Schmidt, 2004; Schmidt et al., 2004; Cazetta et al., 2012; Duan et al., 2014; Ordano et al., 2017). In addition to characteristics of fruit availability, the organization of these bird–plant frugivory interactions can be affected by the relationship between a bird’s bill and fruit morphology (González-Castro et al., 2015; Bender et al., 2018), the spatiotemporal overlap of species (Ramos-Robles et al., 2016), and the relative abundance of fruits and birds (González-Castro et al., 2015). These variables represent neutral- and niche-based processes that may operate in structuring frugivory networks (Machado-de-Souza et al., 2019).
Human activities, however, also have consequences in the way these ecological interactions are organized. At small spatial scales, for example, a local rise in temperature and variation in water availability can cause the phenological mismatch between plant flowering and pollinator presence (Gordo and Sanz, 2005; Hegland et al., 2009). These consequences can be much broader, and a recent meta-analysis demonstrated climate-induced global shifts are responsible for a substantial number of species decouplings (Kharouba et al., 2018).
Other factors, such as the introduction of exotic species in cities’ greenspaces, city noise, and distance to water bodies also affect the way these organisms interact (Tylianakis and Morris, 2017; Barbosa et al., 2020). Exotic plants with large quantities of fruit are frequently selected over native species (Gosper et al., 2005). This is more evident in species with asynchronous fruiting pulses, since these plants’ high fruiting output during periods of low local productivity may result in their fruits selected over natives simply for their availability in highly disturbed environments (Montaldo, 2000; Greenberg and Walter, 2010). One related factor with known effects in fruit consumption by birds is their morphologic matching: altered environments generally do not have fruits of large sizes and those present therein have a lower dispersal probability because their large-sized frugivores are more likely to be absent in these habitats (Galetti et al., 2013; Emer et al., 2018). Urban environments tend to have a low number of species and interactions, resulting in simplified plant-bird frugivory and pollination networks dominated by generalists (Maruyama et al., 2019; Salazar-Rivera et al., 2020; Schneiberg et al., 2020). Conversely, forest cover and connectivity are key in the maintenance of interaction diversity in seed dispersal networks (Monteiro et al., 2021).
The literature highlights the role of habitat fragmentation as another element that alters the nature of these interactions. Fragmentation is known to result in a random pattern of fruit detectability (not based in color) in contrast with better-preserved habitats where certain fruit colors are selected over others (Galetti et al., 2003).
It is reasonable to expect that some of the factors determining the structure and frequency of frugivory interactions between plants and birds in a conserved area would differ from those in highly disturbed places such as cities and their periphery, whereas other factors would not. It is likewise tenable to expect the role and services these networks play in topical urban areas is different than those of “natural” habitats. Our investigation sought to determine the role of different characteristics of available fruit in a Neotropical periurban park on the frequency of whole-fruit consumption by frugivorous birds. As a consequence of the low floristic diversity but heterogenous vegetation structure in our study site, we expected fruit abundance and phenology would be the factors that better explain the observed frequency of pairwise interactions, as we expected a network dominated by generalists (Laliberté and Tylianakis, 2012). We also expected that nutritional content and chromatic contrast would have a considerable predictive value since birds actively seek to fulfill certain dietary needs through fruit consumption (Petchey et al., 2008). In the case of chromatic contrast, our prediction was that fruits that have a higher background contrast would also have a higher detectability (Schmidt et al., 2004; Ordano et al., 2017). Last, we expected morphological matching would have the lowest predictive value since disturbed areas have a higher trait similarity (e.g., fruit and bird bill sizes), and less network-wide morphological limitations due to fruits of large size and frugivores capable of consuming them (Corlett, 2005; Galetti et al., 2013).
Our work is centered on a frugivory network composed of a few plant and bird species. We consider that, in contrast with better preserved habitats with a larger number of interacting species, the characteristics of our study system can help us better understand the functional role of disturbance in frugivory networks. The objective of this paper is to evaluate the role of seven factors (relative abundance, phenology, morphology, chromatic and achromatic contrasts, and carbohydrate and lipid content) as determinants of the frequency of interactions between fruit-bearing plants and frugivorous birds in a Neotropical periurban park.
Materials and Methods
Study Site
Our field work took place at the Universidad Veracruzana’s Campus para la Cultura, las Artes y el Deporte (hereon UV-CCAD), located in the southern outskirts of the city of Xalapa, Veracruz, Mexico (19° 30′ 31.90″ N, 96° 54′ 56.05″ W, elevation 1,417 m).
The UV-CCAD is a small size (33-ha) periurban park with a successionally recent vegetation history and under active management (Figure 1). It was established in 1997 in an area formerly occupied with soccer fields and cattle pastures. Twenty-four years ago, its compacted clay soils hosted mainly forage grasses for horses, mules, and cows, and scattered huizache trees (Acacia spp.). It was until the founding of the first facilities that the greenspaces of this campus received some management. Roughly one-half of the area was left to continue its process of natural secondary succession while the other half was converted into sports facilities, concert halls, a large library, administrative buildings, artificial impoundments, ornamental lakes, and managed gardens with native and exotic ornamental species.
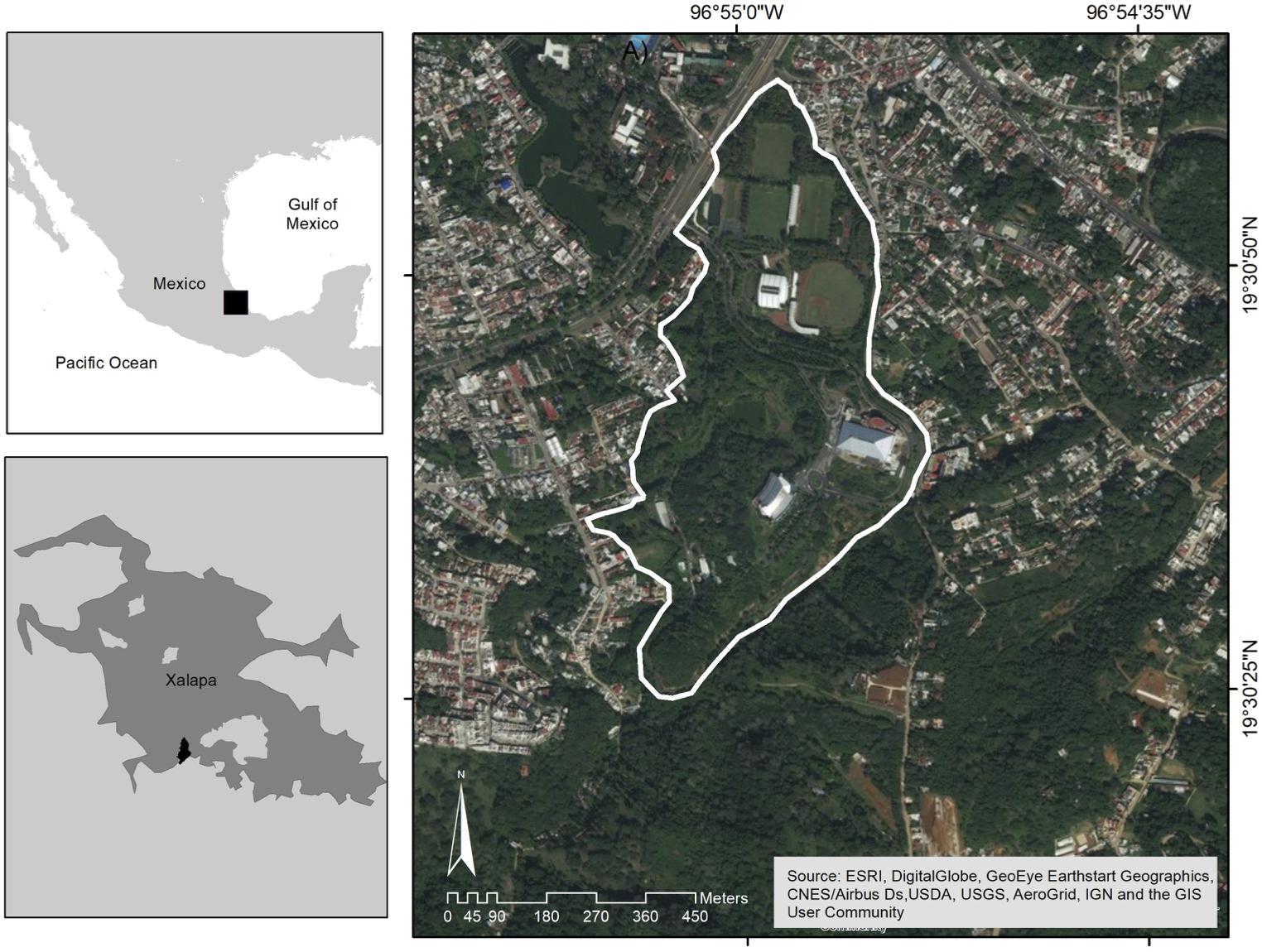
Figure 1. The Campus para la Cultura, las Artes y el Deporte de la Universidad Veracruzana in Xalapa, Veracruz, Mexico, a 33-ha Neotropical periurban park. Courtesy of Sara P. Ibarra-Zavaleta.
The original vegetation, certainly well over 100 years ago, was tropical montane cloud forest (Castillo-Campos, 1991). Tropical montane cloud forests are a comparatively species-rich habitat, with a tree stratum dominated by species of Nearctic affinity and Neotropical origin understory plants. These forests are also known for rich in vascular plant endemics. Its narrow elevation range, locally from 1,250 to 1,995 m, together with its naturally limited distributional range under intense anthropogenic pressure, make of these forests it one of the most at-risk vegetation types in Mexico (Williams-Linera, 2007). This park has very few arboreal elements of its original vegetation such as a handful of oaks (Quercus spp.), sweetgums (Liquidambar styraciflua), sycamores (Platanus mexicana), and marangolas (Clethra mexicana). However, the current condition of natural growth areas is well-developed secondary vegetation (locally called “acahual”) with a canopy of about 12–15 m, younger age clearings, interspersed coffee and citrus trees, banana plants, a growing number of advanced second growth vegetation patches, and some elements of mature cloud forest. Currently, the UV-CCAD maintains connectivity with a mosaic of more than 500 ha of shade-grown coffee and second growth vegetation in its southern fringes.
Bird and Plant Frequency of Interactions
We observed interactions between birds and plants in three 1-ha plots within the UV-CCAD. The three plots differed in vegetation cover. There, we collected focal observations of feeding birds from 07:00 to 10:00 during two sampling periods (see below).
For the purpose of this study, one individual bird feeding on one fruit counted as a single interaction, including only events where the whole fruit was entirely consumed (including its seeds). Fruit pecking observations (e.g., birds not eating the entire fruit) were excluded from our analyses. We collected these focal observations haphazardly: on sampling days, we walked our three study plots using a non-fixed route and recorded all confirmed, non-pseudoreplicate frugivory interactions. We identified the bird (with 8 × 42 binoculars, using Howell and Webb, 1995; and Sibley, 2003) as well as the plant species involved (from a photographic catalogue we developed for this purpose or by collecting a specimen for later identification in a herbarium). Our observations took place during two sampling periods in 2017: one during the dry season (April and May, n = 15 sampling days) and one during the rainy season (August and September, n = 16 sampling days; the sum of both sampling effort equals about 90 h). Our sampling periods included the known fruiting seasons of both native cloud forest and exotic species (Williams-Linera, 2007). With those data, we made a matrix in which rows were the plant species and columns bird species, and we added the frequency of interactions in the intersecting cells.
Early in the collection of our frugivory data, we discovered that the number of species that we could find participating in this frugivory network would be quite limited, possibly including only a few tens of species in each level. In order to assess the completeness of our dataset, we estimated accumulation curves via a R package for Hill numbers (an estimate of the effective number of species as a function of sampling effort) called iNEXT (Hsieh et al., 2016). This package can generate seamless rarefied (interpolated) species accumulation curves based on observed data and is also able to project a rarefied extrapolation of the predicted number of species or species interactions given a larger sampling effort. Those calculations are accompanied by 95% confidence intervals.
We applied the method of Hsieh et al. (2016) to both, the bird and the plant groups, as well as to the observed and predicted number of pairwise interactions between them. The code of Hsieh et al. (2016) has a function to extrapolate its values given a hypothetical sample with two or three times as many focal records, enabling its users to estimate a predicted number of species and determine whether its actual (interpolated) or simulated (extrapolated) curve reaches an asymptote. In assessing the completeness of our sampling, we extrapolated the number of focal observations to twice the number of focal observations (n = 162 data points).
We modeled the interaction network based on observed data, and estimated network metrics such as weighted NODF (WNODF; Almeida-Neto et al., 2007). In a nested network, specialists tend to interact with generalists, and provide WNODF range values from 0–100 where values close to 100 mean a nested structure. Complementary specialization (H2′) calculates the proportion of interaction frequencies contrasted with the expected, based on species abundances, and range from a low of 0 for a non-specialized network, to 1 for a totally specialized network (Blüthgen et al., 2006). We also estimated the network’s modularity, the tendency of subsets of species (modules) to strongly interact with another subset of species (Thébault, 2013) and calculated Beckett’s modularity (QB; Beckett, 2016) a metric with values 0–100, where higher values mean a modular structure. We contrasted the observed values with randomly generated networks with 1,000 iterations, using a Patefield algorithm with given marginals. All metrics and random networks were estimated using R (R Core Team, 2019) with the package bipartite (Dormann et al., 2009).
Factors Affecting the Frequency of Interactions
We recorded several characteristics of fruits as factors that could explain the frequency of interactions with birds: relative abundance, phenology, fruit characteristics (such as size, color, and weight), nutritional content (carbohydrates and lipids; we made no attempt to quantify proteins due to logistical and financial limitations), as well as characteristics of birds that could help us eliminate forbidden interactions (i.e., impossible combinations due to the physical impediment of a bird to consume a given fruit, such as bill gape and body weight, or bird and plant seasonality mismatches).
Bird Phenology and Abundance
We assessed bird phenology and abundance similarly. We obtained presence-absence and abundance data from two different 800-m long by 40-m wide transects part of a long-term study at the UV-CCAD (ERI, unpublished data). Although transects are much longer than the sampling plots of this frugivory study, they cover two out of the three plots of this study and we consider them an appropriate measure of bird presence and abundance. We surveyed these transects biweekly during the same months of our frugivory study and recorded bird species present as well as the number of individuals per species. Transect surveys were done approximately from 7:00 to 9:00 h.
Bird Body Mass and Gape Width
Body size and gape opening are two key bird features that may allow or forbid fruit consumption. We collected field measurements of body mass (with an electronic scale) and gape width (with a caliper, at the bill’s commissure) from wild birds by capturing them with mist nets. We placed arrays of 10 12 × 2.5 m nets (30 mm mesh opening) in each plot. The mist netting effort per season/plot was the same (ca. 105 mist net hours), for a total of 210 mist net hours. We used mean mass and gape measurements from either five males or five females (when sex could be determined based on external characters), or simply from 10 individuals trapped for species with similar sexes. In cases where we could not obtain the desired number of measurements, we supplemented those with specimen data from the bird collection of the Museo de Zoología at the Facultad de Ciencias of the Universidad Nacional Autónoma de México or mass data from published literature (Dunning, 2007).
Fruit Phenology and Abundance
We assessed the phenology of fruiting plants by recording the months in which a plant has ripe fruits. Total fruit abundance per plot was estimated as the approximate number of fruits per plant species. These estimations consisted of direct counts of fruits per branch, or in racemes of different sizes, that were later extrapolated to the total number of plants per species found on each plot. We consider that our estimates of fruit abundance were an appropriate proxy of fruit availability, as the fruits we found had a low interspecific variation in size (weighted arithmetic mean fruit diameter of 11 species of plants/15 fruits per species: 8.90 mm; SD: 4.07 mm).
Fruit Size
For each species, we collected 15 ripe fruits from different individual plants, obtained its mean weight, equatorial diameter (in some particular cases, we used the minimum diameter because of the way those fruits are ingested by birds, e.g., the oval fruits of Phoenix canariensis). Because we were concerned with the morphologic fit between bird’s bills and fruit dimensions, we only analyzed fruit diameter rather than weight as a proxy for size.
Nutritional Content
Carbohydrates and lipids have been documented as the nutrients that play the central role in fruit consumption by birds (Jordano, 2000). We estimated the nutritional content of all plant species recorded in this frugivory network by collecting approximately 100 g of ripe, undamaged fruits following the AOAC (1984) protocol. We dried whole fruits (pulp and seeds included) in a stove at 60°C for 3 days to get them ready for analysis. We determined the quantity of lipids using a Soxhlet extractor using petroleum ether as a solvent following the method of the AOAC. To estimate the quantity of carbohydrates, we used the formula to determine nitrogen-free extracts of the AOAC (1984).
Fruit Chromatic and Achromatic Contrast
Because fruit detectability, the ease to stand out against its background, has been demonstrated to influence fruit consumption (Schaefer et al., 2007, 2014; Ordano et al., 2017), we used chromatic contrast as a detectability proxy. We determined the reflectance of each plant by collecting spectrophotometry values of five different fruits and five different leaves per species. We used an Ocean Optics USB2000 spectrophotometer with the SpectraSuite software (Ocean Optics Inc., Largo, FL, United States), and calculated both values using the R package pavo (Maia et al., 2013). The birds we found in our study belong to two slightly different spectral sensitivities. With the exception of one non-passerine species, we used the visual model of the European starling (Sturnus vulgaris). For the single galliform species of this study, the plain chachalaca (Ortalis vetula), we used the reference values of the Indian peafowl (Pavo cristatus). All the chromatic and achromatic values are represented in Just Noticeable Difference (JND) units (a JND is the minimum threshold value in a bird’s ability to sense differences in visual stimuli).
Probability Matrices
We evaluated the role of seven individual factors [abundance (Ab), phenology (Ph), morphology (Mo), carbohydrate availability (Ca), lipid availability (La), chromatic contrast (Cc), and achromatic contrast (Ac)] and all the possible combinations of these factors in predicting the observed frequency of interactions between birds and plants. To do this, we generated probability matrices for each of the factors examined and compared them to the observed interaction matrix. The conceptual and analytical reference we used for these comparisons is that of Vázquez et al. (2009a) and subsequent modifications by Maruyama et al. (2014) and Vizentin-Bugoni et al. (2014). Probability matrices were built as follows:
Observed Matrix
This is the observed, quantitative matrix of interactions between plants and birds in which plants are placed in rows (j) and birds in columns (i). The value in the intersecting cells (aij) is the cumulative number of interactions between those two species during both field sampling periods (April–May and August–September).
Abundance
The values of each intersecting cell (aij) were a product of the relative abundance of each bird species multiplied by the abundance of each plant. The resulting matrix can be considered as a neutral statistical model because it considers that species interact in proportion to their abundances (Gotelli and McGill, 2006; Vázquez et al., 2007; Vizentin-Bugoni et al., 2014).
Phenology
We determined the role of onsite bird seasonal presence and fruiting plant phenological overlap by building a matrix where the entries of each intersecting cell (aij) are expressed as the number of months when a particular bird and plant species co-occurred. For months in which we actually recorded an interaction, but the phenology data did not match such pair of species, we assigned an arbitrary value of 1 × 10–8. This would allow us to run the analyses avoiding biases in our results (González-Castro et al., 2015; Gonzalez and Loiselle, 2016).
Morphology
To consider the importance of the morphological matching between bird gape width (g) and fruit diameter (f) we built three matrices. In the first one, we placed on each intersecting cell (aij) the quotient of f/g. When the diameter of the fruit was larger than the mean gape width, the divisor was placed in the position of the dividend, g/f. When f > g, quotient values ≤ 1 reveal a better gape width-fruit diameter matching and hence a greater interaction probability and the opposite when f < g. The second matrix was a binary one, where a value of 1 reflects possible interactions when gape width is larger than fruit diameter and a value of 0 reflects unlikely or forbidden interactions because there is no possible morphological matching. The third and last matrix is the quotient of f/g placed on each cell, so birds with larger gape width had a higher probability of consuming fruits and birds with gape widths smaller than fruit diameter were given a value of 0 (as in the second matrix). Last, we compared the three versions in order to select the single matrix that better explained the observed frequency of interactions using a likelihood approach and calculating values of the Akaike Information Criteria (AIC). The result was the use of the first matrix because it had the highest explanatory value.
Carbohydrate Availability
We created two matrices: in the first one, we placed in the intersecting cell (aij) the mean percentage of carbohydrate content for each fruit species. That way, any bird species had the same probability of interaction with a given plant species, and fruits with higher proportion of carbohydrates would have a higher probability of interaction. In the second matrix, we used carbohydrate percentage and bird body mass. We consider that birds of larger size require more total energy, and have larger home range, that allows them to forage areas that are larger than those of smaller birds, and this could have an effect in fruit consumption. To account for this, we multiplied the percentage of carbohydrates times the mass of birds to fill the intersecting matrix cells. Upon comparing both matrices, we chose the matrix that uses the percentage of carbohydrates since it performed better in explaining the observed frequency of interactions.
Lipid Availability
We generated a lipid availability matrix following the same rationale than generating those of carbohydrates. We placed on each intersecting cell (aij) the mean percentage of lipid content for each fruit species.
Chromatic and Achromatic Contrasts
We built a matrix by placing in the intersecting cells (aij) the chromatic contrast value obtained from fruits of each plant species compared with that of its own leaves. We used two visual models, as described for the fruit chromatic contrast and achromatic contrast (section “Fruit Chromatic and Achromatic Contrasts”). Our assumption is that plants with a higher chromatic contrast between fruits and leaves would have a higher probability of detection and therefore being consumed by birds. In the second matrix we placed achromatic contrast values in the same fashion, resulting in the former matrix performing better in explaining fruit consumption patterns than the latter (because of this, Ac was excluded from further analyses).
Contrasting the Observed Interaction Network With Probabilistic Matrices
In order to evaluate the influence of multiple factors in the observed patterns of fruit consumption (i.e., in the frequency interaction matrix) by birds, the six-resulting single-factor matrices (Ab, Mo, Ph, Ca, La, and Cc) were combined in all possible ways (62 alternatives). The matrices that combined factors are a result of direct multiplications of two cells (i.e., the Hadamard or Schur product, Davis, 1962) containing the same pair of interacting species found in the observed matrix. We also created a null model (Nu) in which all species had the same interaction probability. This matrix was taken as a reference point for the remaining comparisons with probability matrices (Vázquez et al., 2009b). Each matrix was normalized by dividing the value of each cell by the grand sum of all values tabulated in such matrix, so the final additive value of each probability matrix equals 1.
In order to evaluate the potential of each of the 62 probability models in predicting the observed frequency of pairwise interactions matrix, we used a maximum likelihood approach through the calculation of AIC (Burnham and Anderson, 2002). We assumed that the probabilities of interaction between birds and plants follow a multinomial distribution (Vázquez et al., 2009b) and used the function dmultinom to calculate its likelihood in the built-in stats package of the R software (The R Core Team, 2019).
The number of parameters we used to weigh the analyses was the sum of species of each level included in the model under evaluation, whereas the null matrix consisted of a single parameter (fide Maruyama et al., 2014; Saldaña-Vázquez, 2014). Last, we made the comparisons among probability matrices by calculating the AIC differential (ΔAIC) by deducting the model’s AIC with the lowest AIC value of the remaining models. By definition, the model with the best predicting fit had an ΔAIC = 0.
Results
Network Features
We documented 81 bird–plant interactions in the frugivory network of the UV-CCAD. On the plant level, we found 11 species of plants, from which the exotic palm P. canariensis had the highest degree, with most of its interactions with the plain chachalaca. The plant species with the highest degree distribution was the nightshade Witheringia stramoniifolia with seven species of birds feeding on its fruits (Figure 2).
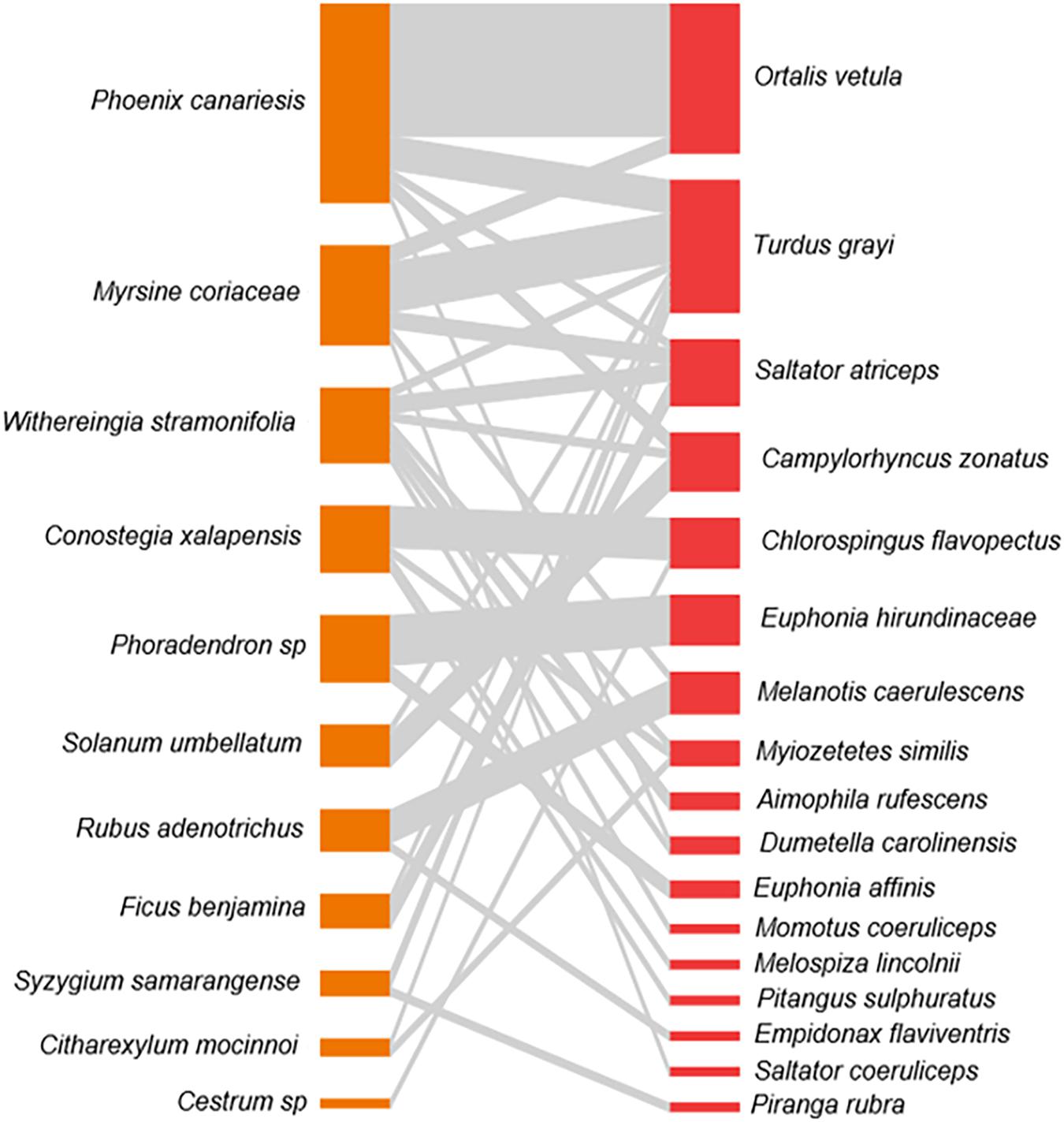
Figure 2. The plant (orange, left column) and bird (red, right column) frugivory network at the Campus para la Cultura, las Artes y el Deporte in Xalapa, Veracruz, Mexico. Lines between species represent species interactions and thickness indicates frequency of interactions.
We found 17 species of birds in this network, with the aforementioned plain chachalaca as the species with the highest degree, whereas the clay-colored thrush (Turdus grayi) was recorded as the bird feeding on the highest number of plants, a total of seven (Figure 2). The frugivory network of this periurban park is nested (WNODF = 9.26, P < 0.01), modular (QB = 0.59, P < 0.01), and its specialization value is relatively high (H2′ = 0.6, P < 0.01). We consider our dataset is small, but sufficient to represent the most common and abundant species (Figure 3A–C).
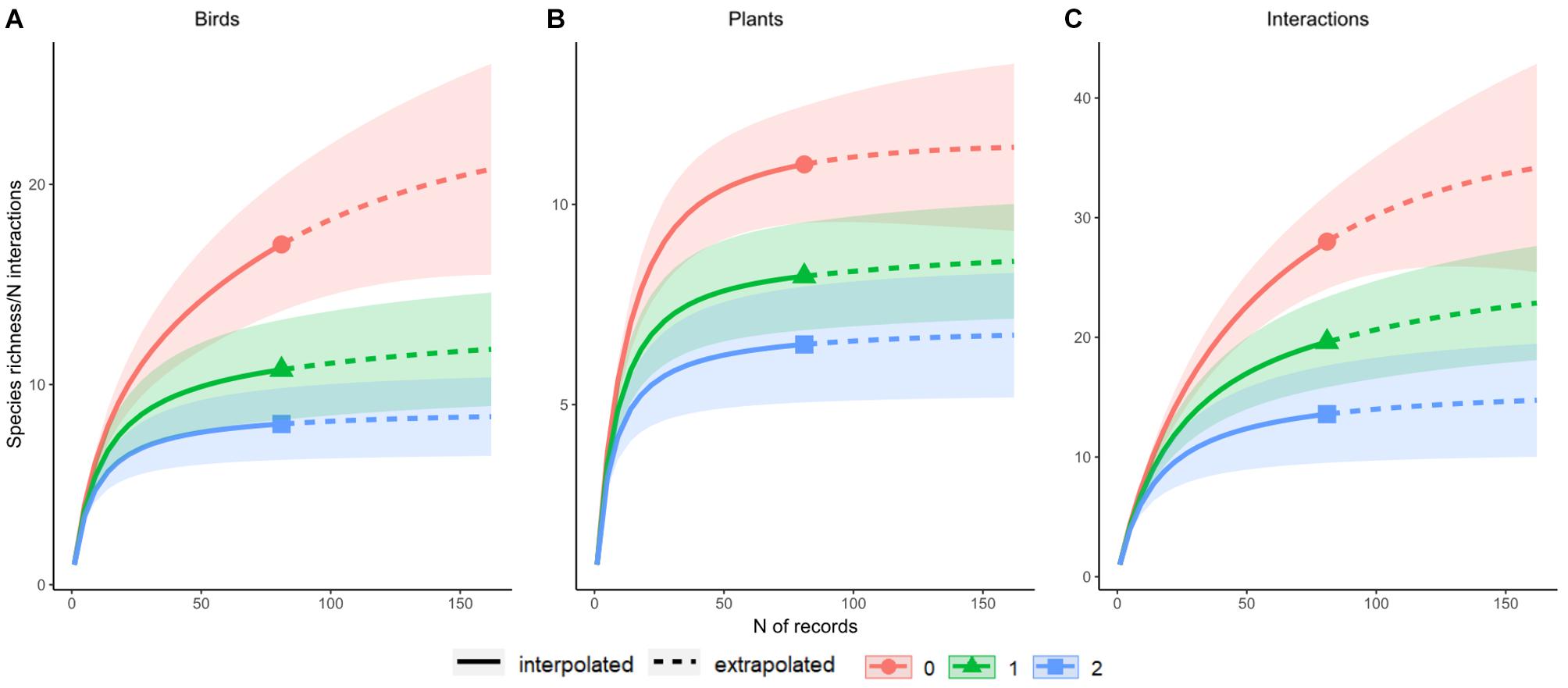
Figure 3. Sampling completeness of the frugivory network of the Campus para la Cultura, las Artes y el Deporte in Xalapa, Veracruz, Mexico. These completeness estimates are based on observed (interpolated, solid line) and simulated (extrapolated, dotted line) data for (A) birds, (B) plants, and (C) pairwise interactions. Abundant (blue), common (green), and total (red) species are well-represented in our sample.
Plant Species Characteristics
Fruit abundance was highly heterogeneous among species – ranging from plants with a mean of 118 fruits per plant (e.g., the berry Rubus adenotrichus) to species like the canalito (Conostegia xalapensis) with 12,330 fruits per plant. The mean fruit abundance estimate was 3,255 fruits per plant (Table 1). All the fruit species recorded interacting with birds had a diameter of <20 mm. They ranged from 4 to 18 mm, with a mean equatorial diameter of 8.8 mm. Its nutritional content was primarily carbohydrates followed by lipids (Table 1). Carbohydrate content ranged from 6 to 53%, with a mean value of 22.3%, whereas lipid content ranged from 1 to 8% with a mean value of 2.8%. Lastly, the chromatic contrast values between a plant’s fruits and its own leaves had low values, ranging from 2 JND in W. stramoniifolia to 11.0 JND in R. adenotrichus (with an overall mean value of 7.9 JND).
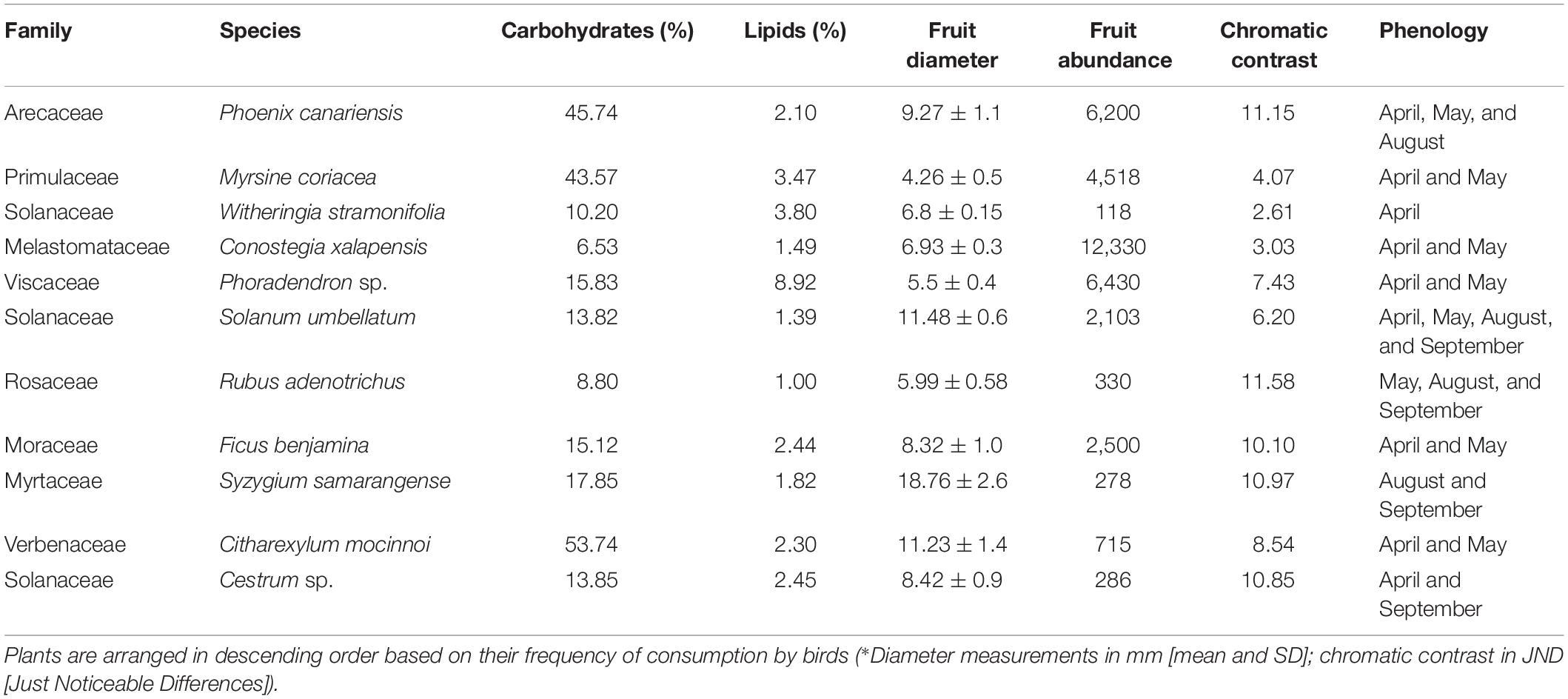
Table 1. Nutritional content, morphology, abundance, chromatic contrast, and phenology of fruits consumed by birds in a Neotropical periurban park in Xalapa, Veracruz, Mexico.
Bird Species Characteristics
The most abundant species were the social flycatcher (Myiozetetes similis) with 16 individuals and band-backed wren (Campylorhynchus zonatus) with 15 individuals, whereas the least abundant two species, the blue-capped motmot (Momotus coeruliceps) and the summer tanager (Piranga rubra), were recorded only once. The UV-CCAD lacks large avian frugivores. The mass of fruit-eating birds was highly variable and ranges from 10–584 g; all but two species weigh < 75 g (plain chachalaca and blue-capped motmot, Table 2). In a similar fashion, the gape width of these birds ranges from 6 mm (yellow-throated euphonia, Euphonia affinis) to 28 mm (plain chachalaca); the mean gape width is 12.3 mm, larger than the mean fruit size (Table 2).
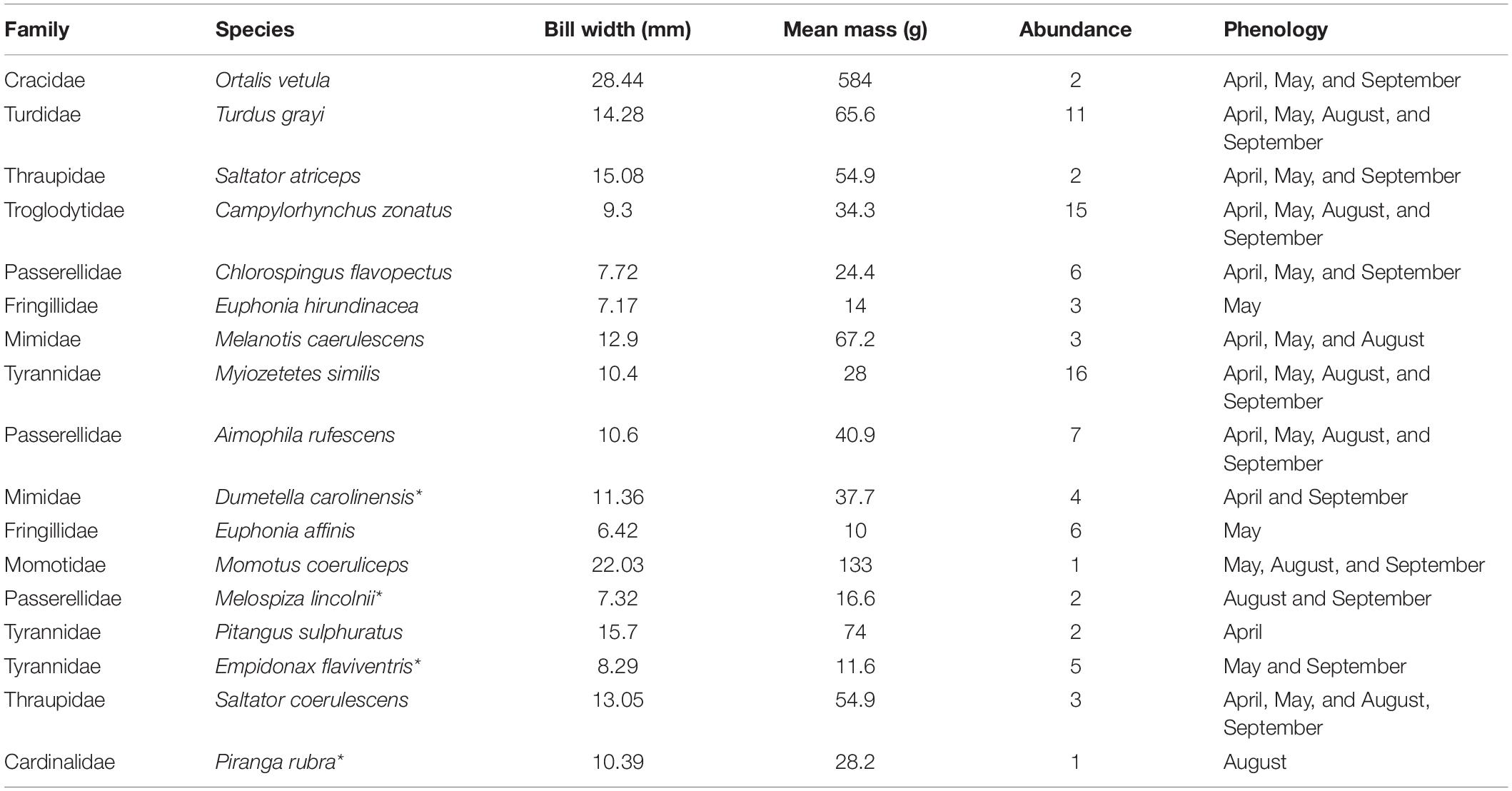
Table 2. Bill width, mass, abundance, and seasonal presence of frugivorous birds in a Neotropical periurban park in Xalapa, Veracruz, Mexico. Species are listed in descending order by interaction frequency (*Neotropical migratory birds).
Bird and Plant Phenology
We found the months of the largest number of phenological matches in the months of April and May, in which we recorded 10 species of plant and 15 bird species, whereas in August and September both had five species of plants fruiting and 14 species of birds. Thirteen species of birds (76%) were present in both field sampling periods; however, only four species of plants (36%) beared fruits in both periods.
Performance of Null, Single-, and Multiple-Variable Probabilistic Models
The top performing model was the null model. This indicates that no single predictor or combination of them is better at explaining the observed frequency of pairwise interactions than randomness (Figure 4). Upon ranking the top-20 best performing models, we found the ΔAIC values of all single-variable models exceeded 50 (except abundance which exceeded the ΔAIC of 100). Carbohydrate and La models were the closest to the null one, followed by chromatic contrast (Figure 4). In general, single-variable models performed better (have lower ΔAIC values) than two- and three-variable models, with the latter showing the poorest fit to observed data. The top-ranking multiple-variable models all contained lipid availability and chromatic contrast (Figure 4).
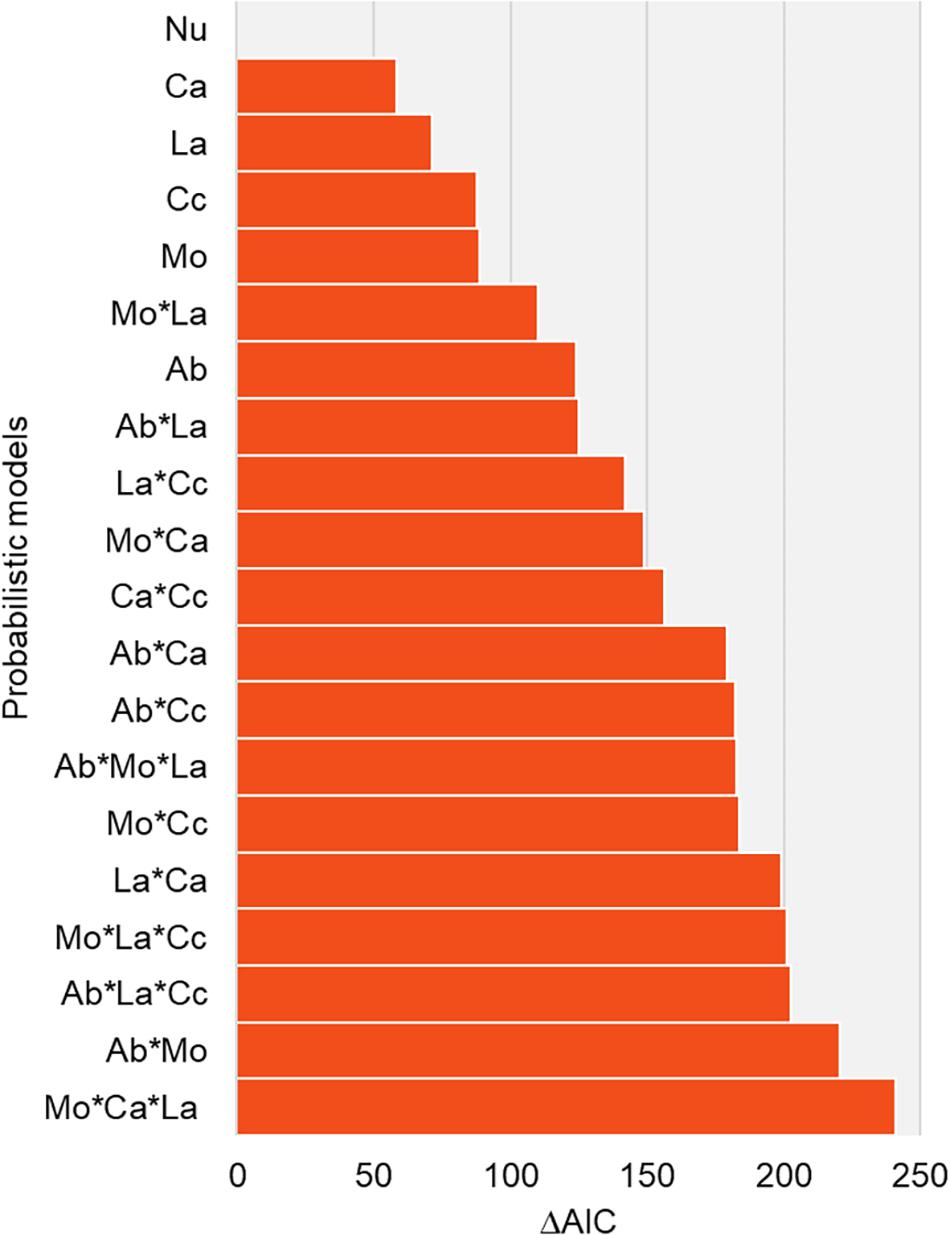
Figure 4. Factors that may influence fruit consumption by birds in a Neotropical periurban park. Twenty top-ranking probabilistic models ranked according to ΔAIC values. Single- and multiple-variable models included here contain null (Nu), carbohydrate availability (Ca), lipid availability (La), chromatic contrast (Cc), morphology (Mo), and abundance (Ab) models.
Discussion
The interaction network between fruiting plants and frugivorous birds in this Neotropical periurban park had a low number of interacting species, a nested and modular pattern, and a relatively high specialization. It also seemed to have a random (e.g., non-deterministic) frequency of pairwise interactions (May, 1972; Thébault and Fontaine, 2010; Serván et al., 2018). Factors such as abundance, phenology, morphology, nutritional content, chromatic contrast, or combinations of them underperform a null model in explaining these pairwise interactions.
Upon reviewing the literature, we found that similar results had not been reported either in frugivory (e.g., Almeida and de Mikich, 2018) or pollination networks (Gonzalez and Loiselle, 2016) in “non-disturbed” habitats. In these investigations, the most frequent outcome is the correspondence between morphology, food abundance, and phenology to explain the organization of interactions (Vázquez et al., 2007; González-Castro et al., 2015; Ramos-Robles et al., 2016).
None of these studies, however, took place in urban or periurban settings, of which we found very few previously published works. Research done in plant-hummingbird pollination networks in urban and periurban settings showed lower modularity and nestedness values, denoting unstructured networks (Maruyama et al., 2019; Schneiberg et al., 2020). Our specialization value, however, was high compared to these works, possibly because of the habitat heterogeneity of our study site and influence from adjacent habitats, since we found birds that aren’t frequent in urban parks. The sample size of our study prevented us from exploring the role that individual factors may play in it (Figure 3a–c, Chacoff et al., 2011).
Null Versus Single- and Multiple-Variable Models
Our study showed the null model as the best performing one. We suspect that the interference of variables relative to one another plays an important role in this result, given that in certain fruit-bearing plant species the frequency of interactions could be determined by its abundance, nutritional content, or morphology, but such single-factor determined patterns are not repeated enough to outweigh the one of the remaining species. When variables are analyzed in combination with others, the addition ends up affecting the performance of multiple-variable models (Burnham and Anderson, 2002).
The Role of Abundance
Contrary to our hypotheses, and to proponents of neutral-based processes as mechanisms structuring networks (Vázquez et al., 2007), fruit and bird abundance were variables of little importance in explaining the observed pattern of pairwise interactions. Plants in our network contain examples like C. xalapensis, whose plants provide the most abundant fruit, but one that is low in carbohydrate content. Species like P. canariensis, one of the most abundant plants with a large quantity of fruits rich in carbohydrates, had fruits that are large (relative to mean bill size, Table 1), but fibrous and hard for birds to feed on. These two examples show how nutritional content or morphology can forestall the importance of abundance to explain frequency of interactions.
The low predictive value of fruit abundance reported elsewhere may also be related to how abundance is estimated. Previous studies have reported abundance as a good predictor of pairwise interaction frequency, but those works made their abundance estimations in a way that is dependent from the network under study (Vázquez et al., 2009b; Schleuning et al., 2014). In contrast, other authors have demonstrated that independent estimates of fruit abundance (as it is our case) place this factor as a lower ranking variable explaining the observed frequency of interactions (Maruyama et al., 2014; Vizentin-Bugoni et al., 2014; González-Castro et al., 2015).
One last possible reason why fruit and bird abundance are poor predictors of interaction frequency is the fact that virtually all bird species found in our study system include other items in their diet besides fruit, notably insects. The extent to which the relative content of fruit in the diet of omnivore or frugivore-insectivore birds influence the observed interaction frequencies remains to be explored.
Morphological Overlap Doesn’t Explain the Frequency of Pairwise Interactions
In our study, the morphological overlap model was a poor predictor of frequency of pairwise interactions, a result that also disagrees with proponents of niche-based network organization mechanisms (Jordano et al., 2003). This is particularly evident when exploring our field data: bill gape and fruit diameter have a substantial overlap. Previous studies have found that interactions between birds and plants are constrained by the morphological overlap between bird bills and fruit diameters and becomes the main determinant of interaction frequency, but this doesn’t seem to be the case here (González-Castro et al., 2015; Bender et al., 2018). It seems, however, that this morphological relationship is of lesser importance when compared with fruit abundance (Saavedra et al., 2014). Disturbed environments reportedly have fewer quantities of large-sized fruits because their large fruit sizes limit easy dispersal and augment their local extinction proneness (Cramer et al., 2007; Galetti et al., 2013). The interactions between birds and plants with small fruit sizes and broad size overlaps, like the one we analyze here, must have less morphological limitations than those of better-preserved habitats where large-sized fruits can be found (Corlett, 2005). In fact, our observations provide little support to the central importance of size alone as an attribute that can define frugivory network interactions since some fruits aren’t consumed whole (e.g., Solanum umbellatum and Syzygium samarangense) but in parts by some birds with small bill size.
Nutritional Content
Nutritional content is an attribute that has been identified as a strong influence in fruit consumption, primarily carbohydrates (Gautier-Hion et al., 1985; Jordano, 2000) and secondarily lipids (Howe, 1993; Lepzcyk et al., 2000). In our study, the two most consumed fruits are those with the highest carbohydrate content; as explained above, we did not estimate protein content.
The two models of nutritional content (carbohydrates and lipids) are the closest to the null model, highlighting its importance as factors that play a role in structuring bird–plant frequency of pairwise interactions. Curiously, few studies have used nutritional content as an influential factor in defining network interactions: in the study of González-Castro et al. (2015), in a Mediterranean scrub frugivory network, the use of carbohydrate content as a predictive variable was outweighed by morphology and relative abundance as the best explanatory variables in spite of the apparent importance of the former.
Chromatic Contrast Performed Better Than Morphology
The chromatic contrast model was better at explaining fruit consumption than morphology. Although some reports have suggested fruit consumption is influenced by the chromatic contrast of fruits and its background (Schmidt et al., 2004; Schaefer et al., 2007), ours is one of the few studies that we are aware that uses chromatic contrast values to assess its role as a determinant of interaction frequency in a frugivory network (e.g., Ordano et al., 2017). Although our results do not demonstrate that fruit consumption is determined by fruit chromatic contrast, we report the fruit detectability model performs better than morphological matching in this periurban park. The outcome may well be different in better-preserved habitats, since the vegetation cover in our study site is less dense, fruits are more readily exposed, and receive more light – characteristics that could favor its detection.
Unforeseen Extrinsic Factors and Caveats
Although we found no pattern in fruit consumption by birds in our study site, our results do not unequivocally demonstrate a random process of fruit consumption. The most important factors to consider are our limited sample size, the limited number of sites and habitat types, and the annual cycle coverage of this study, limited to a 2-month period during the rainy season and a 2-month period during the dry season.
We also think that other, unforeseen factors may also play a role in these interactions but were not evaluated here. Examples of these are trait similarity between birds and fruit and the unknown degree of fruit consumption in the total diet of participating birds. Other possible explanations may be the heterogeneity of vegetation in our study site, a variable that may favor a network arrangement different than the one we might expect in habitats undergoing secondary succession (Palacio et al., 2016). The periurban nature of our study site, embedded in a landscape matrix with abundant gray (e.g., buildings, roads, impervious surfaces, and other human-made) infrastructure that can affect the movements of birds (MacGregor-Fors and Escobar-Ibáñez, 2017), could induce changes in foraging behavior. Other factors, such as macroecological and historical vegetation patterns, the robustness of our dataset, the inclusion of detailed bird behavioral data (e.g., pulp-pecking vs. whole-fruit swallowing and the proportion of fruit on each bird’s diet), may also be at play (e.g., Machado-de-Souza et al., 2019).
Urban and periurban greenspaces preserve fundamental ecological interactions, many of them deeply shaped by human management and activity (Gasperin and Pizo, 2009). While we know some characteristics of these networks, e.g., urban greenspaces as environments that favor generalist species, capable of exploiting available resources broadly and effectively (Clavel et al., 2011; Machado-de-Souza et al., 2019; Palacio, 2019; Salazar-Rivera et al., 2020; Schneiberg et al., 2020), we also know little about many other features, such as the ones explored in this paper. We consider our understanding of how bird–plant frugivory interactions are structured and organized in cities will benefit from including extrinsic factors such as those considered in spatial analyses. We also consider the combined contribution of single site-based studies like ours will help to solve theoretical approaches, such as neutral- and niche-based processes governing ecosystem services in tropical urban areas.
Data Availability Statement
The original contributions presented in the study are included in the article/Supplementary Material, further inquiries can be directed to the corresponding author.
Ethics Statement
Ethical review and approval was not required for the animal study because observational study, no need for permissions.
Author Contributions
IAT-F, EC-G, and ERI: conceptualization, methodology, software, formal analysis, visualization, and writing (review and editing). ERI: translated and edited the manuscript. All authors contributed to the article and approved the submitted version.
Funding
IAT-F and EC-G thank Mexico’s Consejo Nacional de Ciencia y Tecnología (CONACYT) for fellowships 1003381 and 609146, respectively. ERI thanks CONACYT for fellowship 47135 of the Sistema Nacional de Investigadores and the Secretaría de Educación Pública’s PRODEP Program for grant UV-PTC-868. At the Universidad Veracruzana, Domingo Canales Espinosa (Dean of the DGAA Ciencias Biológicas y Agropecuarias) and Raciel D. Martínez Gómez (Dean of the DG Comunicación Universitaria) were instrumental in securing funding to cover publication costs.
Conflict of Interest
The authors declare that the research was conducted in the absence of any commercial or financial relationships that could be construed as a potential conflict of interest.
Publisher’s Note
All claims expressed in this article are solely those of the authors and do not necessarily represent those of their affiliated organizations, or those of the publisher, the editors and the reviewers. Any product that may be evaluated in this article, or claim that may be made by its manufacturer, is not guaranteed or endorsed by the publisher.
Acknowledgments
Dinesh Rao at the Universidad Veracruzana’s Instituto de Biotecnología y Ecología Aplicada kindly helped us with analyses of color, chromatic and achromatic contrast, as well as providing equipment and software for this part of our study. Sergio Martínez-Hernández allowed us to use his laboratory equipment for nutrient content analyses. We are also indebted to Gabriela I. Salazar Rivera for her assistance in the development of this investigation. Yolanda Cocotle Ronzón, and her laboratory assistant Maritza M. González Juárez at the Universidad Veracruzana’s Facultad de Ciencias Químicas, helped us with fruit nutritional content analyses. We also thank Alejandro Gordillo Martínez, Violeta Andrade, and Genaro Rodríguez Otero at the Museo de Zoología, Facultad de Ciencias, UNAM, for their help securing additional measurements of bird bills from their collection. Paulo Inácio Prado and Sergei Petrovskii provided useful criticisms to an earlier version of this manuscript. We thank Frontiers in Ecology and Evolution’s guest editor Franco Leandro Souza and three reviewers for their thoughtful criticisms.
Supplementary Material
The Supplementary Material for this article can be found online at: https://www.frontiersin.org/articles/10.3389/fevo.2021.630150/full#supplementary-material
References
Almeida, A., and de Mikich, S. B. (2018). Combining plant-frugivore networks for describing the structure of neotropical communities. Oikos 127, 184–197. doi: 10.1111/oik.04774
Almeida-Neto, M., Guimarães, P. R. Jr., and Lewinsohn, T. M. (2007). On nestedness analyses: rethinking matrix temperature and anti-nestedness. Oikos 116, 716–722. doi: 10.1111/j.0030-1299.2007.15803.x
AOAC (1984). Methods of analysis 14th ed. Washington, DC: Association of Official Analytical Chemists.
Barbosa, K. V. C., Rodewald, A. D., Ribeiro, M. C., and Jahn, A. E. (2020). Noise level and water distance drive resident and migratory bird species richness within a Neotropical megacity. Landscape Urb. Plan. 197:103769. doi: 10.1016/j.landurbplan.2020.103769
Beckett, S. J. (2016). Improved community detection in weighted bipartite networks. R. Soc. Open Sci. 3:140536. doi: 10.1098/rsos.140536
Bender, I. M. A., Kissling, W. D., Blendinger, P. G., Böhning-Gaese, K., Hensen, I., Kühn, I., et al. (2018). Morphological trait matching shapes plant–frugivore networks across the Andes. Ecography 41, 1910–1919. doi: 10.1111/ecog.03396
Blendinger, P. G., Giannini, N. P., Zampini, I. C., Ordoñez, R., Torres, S., Sayago, J. E., et al. (2015). Nutrients in fruits as determinants of resource tracking by birds. Ibis 157, 480–495. doi: 10.1111/ibi.12274
Blüthgen, N., Menzel, F., and Blüthgen, N. (2006). Measuring specialization in species interaction networks. BMC Ecol. 6:9. doi: 10.1186/1472-6785-6-9
Burnham, K. P., and Anderson, D. R. (2002). Model selection and multimodel inference: A practical information-theoretic approach, 2nd Edn. New York, NY: Springer.
Castillo-Campos, G. (1991). Vegetación y Flora del Municipio de Xalapa, Veracruz, Vol. 148., Xalapa: Instituto de Ecología.
Cazetta, E., Galetti, M., Rezende, E. L., and Schaefer, H. M. (2012). On the reliability of visual communication in vertebrate-dispersed fruits. J. Ecol. 100, 277–286. doi: 10.1111/j.1365-2745.2011.01901.x
Chacoff, N. P., Vázquez, D. P., Lomáscolo, S. B., Stevani, E. L., Dorado, J., and Padrón, B. (2011). Evaluating sampling completeness in a desert plantpollinator network. J. Anim. Ecol. 81, 190–200. doi: 10.1111/j.1365-2656.2011.01883.x
Clavel, J., Julliard, R., and Devictor, V. (2011). Worldwide decline of specialist species: toward a global functional homogenization? Front. Ecol. Env. 9:222–228. doi: 10.1890/080216
Corlett, R. T. (2005). Interactions between birds, fruit bats and exotic plants in urban Hong Kong. South China. Urb. Ecosyst. 8, 275–283. doi: 10.1007/s11252-005-3260-x
Cramer, J. M., Mesquita, R. C. G., and Williamson, G. B. (2007). Forest fragmentation differentially affects seed dispersal of large and small-seeded tropical trees. Biol. Conserv. 137, 415–423. doi: 10.1016/j.biocon.2007.02.019
Dormann, C. F., Fruend, J., and Gruber, B. (2009). Indices graphs and null models: analyzing bipartite ecological networks. Open Ecol. J. 2, 7–24. doi: 10.2174/1874213000902010007
Duan, Q., Goodale, E., and Quan, R. (2014). Bird fruit preferences match the frequency of colours in tropical Asia. Sci. Rep. 4:5627. doi: 10.1038/srep05627
Emer, C., Galetti, M., Pizo, M. A., Guimarães, P. R., Moraes, S., Piratelli, A., et al. (2018). Seed-dispersal interactions in fragmented landscapes—a metanetwork approach. Ecol. Lett. 21, 484–493. doi: 10.1111/ele.12909
Galetti, M., Alves-Costa, C. P., and Cazetta, E. (2003). Effects of forest fragmentation, anthropogenic edges and fruit colour on the consumption of ornithocoric fruits. Biol. Cons 111, 269–273. doi: 10.1016/S0006-3207(02)00299-9
Galetti, M., Guevara, R., Côrtes, M. C., Fadini, R., Von Matter, S., Leite, A. B., et al. (2013). Functional extinction of birds drives rapid evolutionary changes in seed size. Science 340, 1086–1090. doi: 10.1126/science.1233774
Gasperin, G., and Pizo, M. A. (2009). Frugivory and habitat use by thrushes (Turdus spp.) in a suburban area in south Brazil. Urb. Ecosys. 12, 425–436. doi: 10.1007/s11252-009-0090-2
Gautier-Hion, A., Duplantier, J. M., Quris, R., Feer, F., Sourd, C., Decoux, J. P., et al. (1985). Fruit characters as a basis of fruit choice and seed dispersal in a tropical forest vertebrate community. Oecologia 65, 324–337. doi: 10.1007/BF00378906
Gonzalez, O., and Loiselle, B. A. (2016). Species interactions in an Andean bird-flowering plant network: phenology is more important than abundance or morphology. PeerJ 4:e2789. doi: 10.7717/peerj.2789
González-Castro, A., Yang, S., Nogales, M., and Carlo, T. A. (2015). Relative importance of phenotypic trait matching and species abundances in determining plant-avian seed dispersal interactions in a small insular community. AoB Plants 7, 1–10. doi: 10.1093/aobpla/plv017
Gordo, O., and Sanz, J. J. (2005). Phenology and climate change: a long-term study in a Mediterranean locality. Oecologia 146:484. doi: 10.1007/s00442-005-0240-z
Gosper, C. R., Stansbury, C. D., and Vivian-Smith, G. (2005). Seed dispersal of fleshy-fruited invasive plants by birds: Contributing factors and management options. Divers. Distrib. 11, 549–558. doi: 10.1111/j.1366-9516.2005.00195.x
Gotelli, N. J., and McGill, B. J. (2006). Null versus neutral models: What’s the difference? Ecography 29, 780–793. doi: 10.1111/j.2006.0906-7590.04714.x
Greenberg, C. H., and Walter, S. T. (2010). Fleshy fruit removal and nutritional composition of winter-fruiting plants: A comparison of non-native invasive and native species. Nat. Areas J. 30, 312–321. doi: 10.3375/043.030.0306
Hegland, S. J., Nielsen, A., Lázaro, A., Bjerknes, A. L., and Totland, Ø (2009). How does cimate warming affect plant pollinator interactions? Ecol. Lett. 12, 184–195. doi: 10.1111/j.1461-0248.2008.01269.x
Howe, H. F. (1993). Specialized and generalized dispersal systems: Where does the paradigm stand? Vegetatio 107, 3–13. doi: 10.1007/BF00052208
Howell, S. N. G., and Webb, S. (1995). A Guide to the Birds of Mexico and Northern Central America. Oxford, England, United Kingdom. Oxford University Press.
Hsieh, T. C., Ma, K. H., Chao, A., and McInerny, G. (2016). iNEXT: an R package for rarefaction and extrapolation of species diversity (Hill numbers). Methods Ecol. Evol. 7, 1451–1456. doi: 10.1111/2041-210X.12613
Jordano, P. (2000). “Fruits and frugivory,” in Seeds, the ecology of regeneration in plant communities. 2nd edition, ed. M. Fenner (Wallingford, UK: CABI Publishers).
Jordano, P., Bascompte, J., and Olesen, J. M. (2003). Invariant properties in coevolutionary networks of plant-animal interactions. Ecol. Lett. 6, 69–81. doi: 10.1046/j.1461-0248.2003.00403.x
Kharouba, H. M., Ehrlén, J., Gelman, A., Bolmgren, K., Allen, J. M., Travers, S. E., et al. (2018). Global shifts in the phenological synchrony of species interactions over recent decades. PNAS 115, 5211–5216. doi: 10.1073/pnas.1714511115
Laliberté, E., and Tylianakis, J. M. (2012). Cascading effects of longterm land-use changes on plant traits and ecosystem functioning. Ecology 93, 145–155. doi: 10.1890/11-0338.16
Lepzcyk, C. A., Murray, K. G., Winnet-Murray, K., Bartell, P., Geyer, E., and Work, T. (2000). Seasonal fruit preferences for lipids and sugars by American Robins. Auk 117, 709–717. doi: 10.1093/auk/117.3.709
Machado-de-Souza, T., Campos, R. P., Devoto, M., and Galarda Varassin, I. (2019). Local drivers of the structure of a tropical birdseed dispersal network. Oecologia 189, 421–433. doi: 10.1007/s00442-018-4322-0
MacGregor-Fors, I., and Escobar-Ibáñez, J. F. (2017). Avian ecology in Latin American cityscapes. Cham: Springer.
Maia, R., Eliason, C. M., Bitton, P. P., Doucet, S. M., and Shawkey, M. D. (2013). Pavo: An R package for the analysis, visualization and organization of spectral data Methods Ecol. Evol. 4, 609–613. doi: 10.1111/2041-210X.12069
Maruyama, P. K., Bonizário, C., Marcon, A. P., D’Angelo, G., da Silva, M. M., da Silva Neto, E., et al. (2019). Plant-hummingbird interaction networks in urban areas: generalization and the importance of trees with specialized flowers as a nectar resource for pollinator conservation. Biol. Cons. 230, 187–194. doi: 10.1016/j.biocon.2018.12.012
Maruyama, P. K., Vizentin-Bugoni, J., Oliveira, G. M., Oliveira, P. E., and Dalsgaard, B. (2014). Morphological and spatio-temporal mismatches shape a Neotropical savanna plant-hummingbird Network. Biotropica 46, 740–747. doi: 10.1111/btp.12170
May, R. M. (1972). Will a large complex system be stable? Nature 238, 413–414. doi: 10.1038/238413a0
Montaldo, N. H. (2000). Éxito reproductivo de plantas ornitócoras en un relicto de selva subtropical en Argentina. Rev. Chil. de Hist. Nat. 73, 511–524. doi: 10.4067/S0716-078X2000000300015
Monteiro, E. C. S., Pizo, M. A., Vancine, M. H., and Ribeiro, M. C. (2021). Forest cover and connectivity have pervasive effects on the maintenance of evolutionary distinct interactions in seed dispersal networks. Oikos 130:8240. doi: 10.1111/oik.08240
Ordano, M., Blendinger, P. G., Silvia Lomáscolo, B., Chacoff, N. P., Sánchez, M. S., Núñez Montellano, M. G., et al. (2017). The role of trait combination in the conspicuousness of fruit display among bird−dispersed plants. Functional Ecology 31, 1718–1727. doi: 10.1111/1365-2435.12899
Palacio, F. X. (2019). Urban exploiters have broader dietary niche than urban avoiders. Ibis 162, 42–49. doi: 10.1111/ibi.12732
Palacio, R. D., Valderrama-Ardilla, C., and Kattan, G. H. (2016). Generalist species have a central role in a highly diverse plant-frugivore network. Biotropica 48, 349–355. doi: 10.1111/btp.12290
Petchey, O. L., Beckerman, A. P., Riede, J. O., and Warren, P. H. (2008). Size, foraging, and food web structure. PNAS 105, 4191–4196. doi: 10.1073/pnas.0710672105
R Core Team (2019). R: A language and environment for statistical computing. Vienna: R Foundation for Statistical Computing.
Ramos-Robles, M., Andresen, E., and Díaz-Castelazo, C. (2016). Temporal changes in the structure of a plant-frugivore network are influenced by bird migration and fruit availability. PeerJ 4:e2048. doi: 10.7717/peerj.2048
Saavedra, F., Hensen, I., Beck, S. G., Böhning-Gaese, K., Lippok, D., Töpfer, T., et al. (2014). Functional importance of avian seed dispersers changes in response to human-induced forest edges in tropical seed-dispersal networks. Oecologia 176, 837–848. doi: 10.1007/s00442-014-3056-x
Salazar-Rivera, G. I., Dáttilo, W., Castillo-Campos, G., Flores-Estévez, N., Ramírez García, B., and Ruelas Inzunza, E. (2020). The frugivory network properties of a simplified ecosystem: birds and plants in a neotropical periurban park. Ecol. Evol. 10, 8579–8591. doi: 10.1002/ece3.6481
Saldaña-Vázquez, R. A. (2014). Intrinsic and extrinsic factors affecting dietary specialization in Neotropical frugivorous bats Mamm. Rev 44, 215–224. doi: 10.1111/mam.12024
Schaefer, H. M., Schaefer, V., and Vorobyev, M. (2007). Are fruit colors adapted to consumer vision and birds equally efficient in detecting colorful signals? Am. Nat. 169, (Suppl. 1), S159–S169. doi: 10.1086/510097
Schaefer, H. M., and Schmidt, V. (2004). Detectability and content as opposing signal characteristics in fruits. Proc. R. Soc. B: Biol. Sci. 271, 370–373. doi: 10.1098/rsbl.2004.0191
Schaefer, H. M., Valido, A., and Jordano, P. (2014). Birds see the true colours of fruits to live off the fat of the land. Proc. R. Soc. B: Biol. Sci. 281:20132516. doi: 10.1098/rspb.2013.2516
Schleuning, M., Ingmann, L., Strauß, R., Fritz, S. A., Dalsgaard, B., Matthias Dehling, D., et al. (2014). Ecological, historical and evolutionary determinants of modularity in weighted seed-dispersal networks. Ecol. Lett. 17, 454–463. doi: 10.1111/ele.12245
Schmidt, V., Schaefer, H. M., and Winkler, H. (2004). Conspicuousness, not colour as foraging cue in plant-animal signaling. Oikos 106, 551–557. doi: 10.1111/j.0030-1299.2004.12769.x
Schneiberg, I. M., Boscolo, D., Devoto, M., Mercilio-Silva, V., Dalmaso, C. A., Ribeiro, M. C., et al. (2020). Urbanization homogenizes the interactions of plant-frugivore bird networks. Urb. Ecosyst. 23, 457–470.
Serván, C. A., Capitán, J. A., Grilli, J., Morrison, K. E., and Allesina, S. (2018). Coexistence of many species in random ecosystems. Nat. Ecol. Evol. 2, 1237–1242. doi: 10.1038/s41559-018-0603-6
Sibley, D. A. (2003). The Sibley Field Guide to Birds of Western North America. U.S.A. New York, NY: Alfred A. Knopf.
Thébault, E. (2013). Identifying compartments in presence-absence matrices and bipartite networks: insights into modularity measures. J. Biogeogr. 40, 759–768. doi: 10.1111/jbi.12015
Thébault, E., and Fontaine, C. (2010). Stability of ecological communities and the architecture of mutualistic and trophic networks. Science 329, 853–856. doi: 10.1126/science.1188321
Tylianakis, J. M., and Morris, R. J. (2017). Ecological networks across environmental gradients. Annu Rev. Ecol. Syst. 48, 25–48. doi: 10.1146/annurev-ecolsys-110316-022821
Van der Pijl, L. (1982). Principles of dispersal in higher plants. Third edition. Verlag. Berlin: Springer.
Vázquez, D. P., Bluthgen, N., Cagnolo, L., and Chacoff, N. P. (2009a). Uniting pattern and process in plant-animal mutualistic networks: A review. Ann. Bot. 103, 1445–1457. doi: 10.1093/aob/mcp057
Vázquez, D. P., Chacoff, N. P., and Cagnolo, L. (2009b). Evaluating multiple determinants of the structure of plant-animal mutualistic networks. Ecology 90, 2039–2046. doi: 10.1890/08-1837.1
Vázquez, D. P., Melián, C. J., Williams, N. M., Blüthgen, N., Krasnov, B. R., and Poulin, R. (2007). Species abundance and asymmetric interaction strength in ecological networks. Oikos 116, 1120–1127. doi: 10.1111/j.0030-1299.2007.15828.x
Vizentin-Bugoni, J., Maruyama, P. K., and Sazima, M. (2014). Processes entangling interactions in communities: Forbidden links are more important than abundance in a hummingbird-plant network. Proc. R. Soc. B: Biol. Sci. 281:20132397. doi: 10.1098/rspb.2013.2397
Wheelwright, N. T. (1985). Fruit-size, gape width, and the diets of fruit-eating birds. Ecology 66, 808–818. doi: 10.2307/1940542
Keywords: chromatic contrast, forbidden links, frugivory interactions, interaction frequency, probabilistic models, neutrality, nutritional content, urban areas
Citation: Teodosio-Faustino IA, Chávez-González E and Ruelas Inzunza E (2021) In a Neotropical Periurban Park, Fruit Consumption by Birds Seems to Be a Random Process. Front. Ecol. Evol. 9:630150. doi: 10.3389/fevo.2021.630150
Received: 16 November 2020; Accepted: 06 August 2021;
Published: 21 September 2021.
Edited by:
Franco Leandro Souza, Federal University of Mato Grosso do Sul, BrazilReviewed by:
David Horák, Charles University, CzechiaMilton Cezar Ribeiro, São Paulo State University, Brazil
Copyright © 2021 Teodosio-Faustino, Chávez-González and Ruelas Inzunza. This is an open-access article distributed under the terms of the Creative Commons Attribution License (CC BY). The use, distribution or reproduction in other forums is permitted, provided the original author(s) and the copyright owner(s) are credited and that the original publication in this journal is cited, in accordance with accepted academic practice. No use, distribution or reproduction is permitted which does not comply with these terms.
*Correspondence: Ernesto Ruelas Inzunza, cnVlbGFzLnV2QGdtYWlsLmNvbQ==