- 1Centre for Biodiversity Monitoring, Zoological Research Museum Alexander Koenig, Bonn, Germany
- 2Centre for Environment Fisheries and Aquaculture Science (Cefas), Weymouth, United Kingdom
Metabarcoding has proven to be a powerful tool to assess ecological patterns and diversity from different habitats. Terrestrial invertebrate diversity is frequently based on bulk samples, which require comparatively high sampling effort. With environmental DNA (eDNA) metabarcoding, field sampling effort can be reduced while increasing the number of recovered organism groups. However, a proof of concept is missing for several invertebrate groups, hampering the development of best-practice advice for these groups. This study aims to provide recommendations on key aspects for the processing of soil samples, from sampling effort to choice of DNA extraction method and marker genes. This study uses eDNA metabarcoding as a tool for assessing invertebrate biodiversity in soil samples, specifically comparing two DNA extraction methods (with and without a lysis step) and two genes, 18S and COI markers. The results show that the choice of marker and DNA extraction method (including a lysis step) significantly affect species detection rates and concomitantly observed invertebrate community composition. Combining methods, by using larger amounts of starting material and including a lysis step resulted in an increase of invertebrate species numbers. Together, these methods improved the detection of species with known lower population densities and allowed the assessment of temporary mesofauna. Furthermore, the choice of marker significantly influenced the diversity levels found. The 18S marker allowed the detection of a higher number of annelid and nematode OTUs, while the COI marker was more suitable for detecting changes in arthropod community structure, especially at the species level. This study makes significant advances to the field of invertebrate biodiversity assessment, particularly using metabarcoding tools by addressing several methodological considerations that are key for accurate ecological appraisals.
Introduction
Despite the indisputable fact that the fertility of soil is directly linked to existing fauna and flora (Delgado-Baquerizo et al., 2017) little is known about soil biodiversity. To prevent the ongoing loss of biodiversity leading to soil degradation processes, entailing annual costs of several billion dollars (Kuhlman et al., 2010), it is of uttermost importance to develop timely and cost-efficient assessment strategies.
In particular, environmental DNA (eDNA) metabarcoding appears to be a promising tool for filling-in the knowledge gap on soil biodiversity (Oliverio et al., 2018). As it is unnecessary to collect species to detect their presence, eDNA metabarcoding is a non-invasive approach, which limits the sampling effort to a minimum while retrieving unparalleled diversity information from any habitat with reduced costs (Deiner et al., 2017). Several studies have already shown the applicability of eDNA metabarcoding for the assessment of soil invertebrate diversity (Bienert et al., 2012; Zinger et al., 2019) even to study past ecosystems (Epp et al., 2012). Few methodological studies exist to date for soil arthropod detection despite in particular soil arthropods can be used as key indicators of faunal community structure (Neher et al., 2012).
The choice of method and protocol often has a direct influence on the assessed community composition (Alberdi et al., 2018; Dopheide et al., 2019). For microbial community studies it has been observed that DNA extraction methods (Delmont et al., 2011) and sample size (Kang and Mills, 2006) have an effect on the community composition found. Invertebrates, which have heterogenous morphologies, sizes and abundances (Taberlet et al., 2012; Dopheide et al., 2019), will likely require tailored DNA extraction steps as most available commercial kits are optimized for microbial diversity assessment (Zinger et al., 2016). Furthermore, many invertebrates undergo several life stages incorporating inactive phases (e.g., pupal or dormant stage) which might only be detected through a lysis step (Pietramellara et al., 2009). The amount of source material (e.g., grams of soil) used for DNA extraction and the inclusion of biological replicates can be crucial for maximum detection of soil arthropod species richness (Porter et al., 2019) but also marker choice can significantly influence the composition of the recovered community (Giebner et al., 2020). For the phylogenetically diverse soil invertebrates, it remains unclear which marker is most suitable or if a one-fits all marker exists. Previously, the 16S and 18S markers have been used to assess soil arthropod communities (Epp et al., 2012; Yang et al., 2014), but more recent studies also utilized the CO1 marker (Oliverio et al., 2018; Porter et al., 2019).
This study aims to provide recommendations on key aspects for processing soil samples, from sampling effort to choice of DNA extraction methods and marker genes. Here two well-known soil DNA extraction methods (with and without a lysis step) are compared to evaluate their suitability for invertebrate mesofauna community diversity assessment from forest soil samples. To our knowledge this is the first study investigating the direct effect of the application of a lysis step on soil eDNA metabarcoding targeting invertebrate taxa.
Materials and Methods
Sample Collection and Processing
To monitor changes in soil biodiversity over a period of 12 months, we sampled each season between summer 2016 and spring 2017 (Supplementary Table 2). In summer 2016, sample collection was conducted at 12 sites located in the Eifel National Park, in south-western Germany (Supplementary Figure 1 and Supplementary Table 1). In autumn, winter and spring, sample collection was conducted at 14 sites comprising the 12 sampling sites being sampled in summer (Supplementary Table 2 and Supplementary Figure 2). At each sampling site, three soil samples were collected approximately 4–5 m apart from each other from the top 10 cm of soil, using a hand-held metal corer of 4.4 cm diameter × 10 cm length. A total of 162 soil samples were collected and kept in individual 250 ml containers which were transported to the laboratory shortly after sampling and stored at −20°C until further processing.
For this study, a forest conversion gradient from a Norway spruce (Picea abies) monoculture to a european beech forest (Fagus sylvatica) was sampled. The four forest types sampled differed in tree species composition, degree of anthropogenic influence and in the approximate ages of the trees. The pure beech (120 years old) and pure spruce (Picea abies) (60 years old) sampling sites were located in monoculture stands. At the young beech sampling sites 60 year old spruce stands had only recently been underplanted with young beeches which had not yet reached 3 m in size at the time of sampling. At the so-called old beech sampling sites, 60 year old spruces were underplanted with beeches several years ago. At the time of sampling the beeches had already reached a height of more than 3 m and actions to remove spruces from the forest had already been undertaken.
DNA Extraction
Soil samples were removed from the −20°C chamber approximately 12 h before DNA extraction and stored at + 4°C overnight. The next morning, each sample was thoroughly homogenized by gently swirling the 250 ml container. Two different DNA extraction methods were used, one using the silica membrane based NucleoSpin Soil kit (Macherey-Nagel) (MN kit herein) with a lysis step and the other using the phosphate buffer protocol (PB herein) from Taberlet et al. (2012). For the first method, 0.5 g of soil was used per sample to extract DNA from the 162 soil samples using the MN kit, following the manufacturer’s protocol. The Taberlet et al. (2012) method consists of using a saturated phosphate buffer to desorb DNA fragments from sediment particles, whereby extracellular DNA is recovered using also the NucleoSpin Soil kit (Macherey-Nagel) but skipping the lysis step and following manufacturer’s instructions. Briefly, in the PB method, DNA was extracted from ca. 100 g of soil using a phosphate buffer-based solution (Na2HPO4; 0.12 M; pH 8) following the Taberlet et al. (2012) protocol. For the latter, soil samples were saturated in the phosphate buffer solution and placed in an orbital shaker at 120 rpm for 15 min. Subsequently, two 2 ml Eppendorf safe lock tubes were filled with 1.7 ml of the resulting mixture and centrifuged for 10 min at 10,000 g. Afterward, 400 μl of the resulting supernatant were transferred to a new 2 ml collection tube to which 200 μl of SB binding buffer of the NucleoSpin® Soil kit (Macherey-Nagel) was added. Supernatants from duplicate samples were loaded onto NucleoSpin® Soil Column and centrifuged at 10,000 g for 1 min. The remaining extraction steps followed the standard manufacturer’s protocol from the NucleoSpin® Soil kit starting from step 8 (excluding the lysis step). All DNA extracts were eluted with 50 μl of SE Buffer. Ten microliters of the resulting elution step were combined with 90 μl pure H2O (Sigma), followed by DNA purification using the PowerClean® Pro DNA Clean-Up Kit (MO Bio Laboratories, Inc.) following the manufacturer’s protocol. DNA extracts obtained with either of the two methods were subsequently quantified using the Quantus Fluorometer (Promega).
Choice of Primers and Library Preparation
Amplicon library preparation was conducted following a two-step PCR approach (Fonseca and Lallias, 2016). For library preparation of soil samples, two primer pairs targeting the COI and 18S markers respectively were used. A 380 bp fragment of the V4 region of the nuclear 18S rRNA was amplified using the following forward primer TAReuk454FWD1 (5′- ACACTCTTTCCCTACACGACGCTCTTCCGATCT CCAGCASCYGCGGTAATTCC-3′) combined with the reverse primer TAReukREV3r (5′-GTGACTGGAGTTCAGAC GTGTGCTCTTCCGATCT ACTTTCGTTCTTGATYRA-3′) (Stoeck et al., 2010). The mitochondrial COI primer pair consisted of the forward primer mlCOIintF (5′-ACACTCTTTCCCTACACGACGCTCTTCCGATCT GGWA CWGGWTGAACWGTWTAYCCYCC-3′) and the reverse primer dgHCO2198 (5′- GTGACTGGAGTTCAGACGTGTGC TCTTCCGATCT TAAACTTCAGGGTGACCAAARAAYCA-3′) (Leray et al., 2013), targeting a 313 bp region of the 658 bp long barcoding COI gene.
Approximately 10 ng of template DNA was used for all PCR reactions. For PCR1 the mastermix consisted of 7.5 μl Q5 Hot Start High-Fidelity 2X Master Mix (New England BioLabs), 5 μl Sigma H2O, 0.5 μl of each primer (10 μM), 0.5 μl Bovine Serum Albumin (Thermoscientific) and 1 μl template DNA, making up a final volume of 15 μl. The first PCR (PCR1) consisted in an initial denaturation for 2 min at 98°C, followed by 20 cycles with 40 s at 98°C, 40 s at 45°C, 30 s at 72°C (COI), or 20 cycles with 40 s at 98°C, 40 s at 55°C, 30 s at 72°C (18S), and a final extension of 3 min at 72°C. PCR1 products were then purified using 4 μl HT ExoSAP-ITTM (Applied Biosystems) per 10 μl of PCR1 product, following the manufacturer’s protocol. For PCR2, the purified PCR1 products were split into two PCR tubes. Each tube contained 12.5 μl Q5 Hot Start High-Fidelity 2X MasterMix (New England BioLabs), 3 μl Sigma H2O, 1.2 μl of forward index primer (10 μM) (AATGATACGGCGACCACCGAGATCTACAC NNNNNNNN ACACTCTTTCCCTACACGACGCTC) and 1.2 μl of reverse index primer (10 μM) (CAAGCAGAAGACGGCATACGAGAT NNNNNNNN GTGACTGGAGTTCAGACGTGTGCTC), and 8 μl purified PCR1 product. The PCR2 conditions consisted of an initial denaturation of 2 min at 98°C, followed by 20 cycles with 40 s at 98°C, 30 s at 55°C, 30 s at 72°C, and a final extension of 3 min at 72°C. PCR2 products were visualized by gel electrophoresis and purified using the QIAquick Gel Extraction Kit (Qiagen), according to manufacturer’s instructions. All final purified amplicons (PCR2) were quantified using the Quantus Fluorometer (Promega) and normalized to the same concentration (3 ng/μl) before being pooled together to create two amplicon libraries (18s and COI). The resulting purified amplicon library pools were sequenced on two runs on the Illumina Miseq (2 × 300 bp) sequencing platform at the Centre for Genomic Research (CGR, Liverpool University).
Bioinformatics and Data Analysis
Initial quality check of raw sequences at CGR comprised trimming of fastq sequences for the presence of Illumina adapter sequences using Cutadapt version 1.2.1. Afterward, sequences were trimmed using Sickle version 1.200 with a minimum window quality score of 20. Only reads longer than 19 bp were kept for further analysis.
The fastq sequences were checked for the presence of the COI and 18S primers with Cutadapt version 1.18 (Martin, 2011) using the following settings: maximum error rate (−e): 0.1, minimum overlap (−O): 20, minimum sequence length (−m): 50. Sequences lacking either forward or reverse primers were removed from the dataset. Subsequently, paired-end reads were merged with vsearch version 2.7.0 (Rognes et al., 2016). Merged sequences with a length of 360–400 bp for the 18S and 293–333 bp for the COI dataset respectively were retained for further analysis and filtered with a maxEE threshold of 1.0 using vsearch (version 2.7.0) (Rognes et al., 2016). Afterward, fastq sequences were demultiplexed using the script split_libraries_fastq.py with a phred quality threshold of 19 implemented in QIIME1 (Caporaso et al., 2010). Dereplicating, size sorting, de novo chimera detection and Operational Taxonomic Units (OTUs) clustering at 97% cutoff was conducted with vsearch 2.7.0 (Rognes et al., 2016). An OTU table was built using the –usearch_global function in vsearch 2.7.0 (Rognes et al., 2016) and the python script “uc2otutab.py” written by Robert Edgar1. Resulting OTU tables for both markers were further curated using the LULU algorithm, known to decrease erroneous OTUs (Frøslev et al., 2017). Curation started with an initial blasting of OTU representative sequences against each other using blastn (version 2.9.0) with “query coverage high-scoring sequence pair percent” (-qcov_hsp_perc) set to 80 and minimum percent identity (-perc_identity) set to 84 and a customized output format defined by the –outfmt setting “6 qseqid sseqid pident.” Subsequently, the resulting filtered OTU match list was uploaded into R (version 3.5) (R Core Team, 2013), where the R-package “lulu” (version 0.1.0) (Frøslev et al., 2017) was used to perform post clustering curation using standard settings.
For taxonomy assignment, the COI dataset was blasted against the BOLD database (downloaded on May 5th 2019) using blastn 2.9.0 + (Altschul et al., 1990). As the BOLD database is strongly limited in number of bacterial sequences and barcodes of many eukaryotic species outside Metazoa, a second database was downloaded on February 27th 2020 from GenBank using the following search criteria: [COI(All Fields) OR COX1(All Fields)] OR CO1(All Fields) AND [fungi(filter) OR protists(filter) OR bacteria(filter) OR archaea(filter)]. All sequences not assigned to Metazoa when blasted against the downloaded BOLD database were compared to the above GenBank reference database. For taxonomy assignment of the 18S dataset all sequences were blasted against a customized reference database downloaded on February 27th 2020 from GenBank according to the following criteria: [(18S) OR V4 AND animals(filter) OR fungi(filter) OR plants(filter)]. Sequences without assignment were in a second step blasted against the newly released SILVA132 release2. Raw sequence data for this project are deposited in NCBI’s SRA database under accession number PRJNA681091.
Statistical and Ecological Analysis
The resulting OTU tables (Supplementary Tables 3, 4) were loaded into Excel and formatted for upload into R studio v3.5 (R Core Team, 2013). For statistical analysis, several R packages were used. For data visualization we used ggplot2 (Wickham, 2016) and for data manipulation dplyr version 0.8.3 (Wickham et al., 2015). To further visualize shared and unique OTU numbers per marker, phylum and season between the different methods we used VennDiagram version 1.6.20 (Chen and Boutros, 2011). Pairwise dissimilarities between the two methods on OTU presence-absence matrices based on Jaccard similarity index were performed for incidence data of detected OTUs with a 90% BlastID to Eukaryota, using the R-package betapart version 1.5.1 (Baselga and Orme, 2012). Sample completeness curves and sample-size-based R/E curve with extrapolations of Hill numbers for incidence data based on a combined dataset of both markers, encompassing all OTUs assigned to Arthropoda with a blastID of at least 99% (removed duplicate assignments) were prepared using the R-package iNEXT (Hsieh et al., 2016) at default settings (40 knots, 95% confidence intervals generated by the bootstrap procedure (50 bootstraps).
To visualize and analyze community dissimilarities between methods, PCoAs and statistical tests based on Jaccard similarity index for incidence data of detected eukaryote OTUs with a 90% BlastID were performed using betapart v1.5.1. The betadisper test was performed to test for homogeneity between samples followed by PERMANOVA (adonis) to further test for differences in community composition depending on the DNA extraction method and marker used.
To identify the insect species primarily contributing to community dissimilarities between extraction methods depending on season a SIMPER analysis (Gibert and Escarguel, 2019) was performed in R. The SIMPER analysis was done using COI OTUs assigned to Insecta at the species level with a blastID of at least 99%.
Results
Amplification of the COI marker resulted in the detection of 25,036,251 high quality-filtered reads, which were subsequently clustered into 31,781 OTUs. When amplifying the V4 region of the 18S marker a total of 22,036,784 high quality filtered reads were obtained, which were clustered into 33953 OTUs. After Lulu curation, the total number of OTUs was 23,004 OTUs for the COI dataset (72.4%) and 15,650 OTUs for the 18S dataset (46%).
The complete COI dataset showed a lower assignment rate compared to the 18S dataset (Figure 1). Based on a blast sequence identity cutoff (blastID) of at least 97%, 13.48% of all retrieved 18S OTUs matched an entry in the reference databases, whereas for the COI it was 10.08% (Figure 1A). At the kingdom level, 31.48% of the taxonomically identified 18S OTUs (664 OTUs) and 25.72% of the COI OTUs (635 OTUs) were assigned to Metazoa, respectively (Figure 1A). For both marker datasets it was found that with 68.42% (1443 OTUs) and 74.20% (1832 OTUs) the lion share of assigned OTUs accounted for eukaryotes outside of Metazoa. Additionally, two OTUs of each marker dataset were assigned to Bacteria. Within the Metazoa, the 18S marker identified ten phyla: Annelida, Arthropoda, Chordata, Cnidaria, Gastrotricha, Mollusca, Nematoda, Platyhelminthes, Rotifera, Tardigrada (Figure 1B), while the COI marker, identified six phyla (Annelida, Arthropoda, Chordata, Mollusca, Nematoda, Tardigrada). Collapsing all OTUs with the same taxonomic annotation with a blastID of at least 99% a total of 12 annelid species were identified. Out of them, eight were exclusively detected with the 18S rRNA gene, while the remaining four species were only found with the mitochondrial marker (Figure 2A). Out of the 208 detected arthropod species, 146 (70%) were exclusively found by the COI marker, whereas the 18S retrieved additional 57 species. The two marker datasets shared five arthropod species (Figure 2B). For the Insecta, 96 species were identified using the COI with a BlastID of at least 99%, and six species using the 18S marker (Figure 2C). No insect species was detected with both markers.
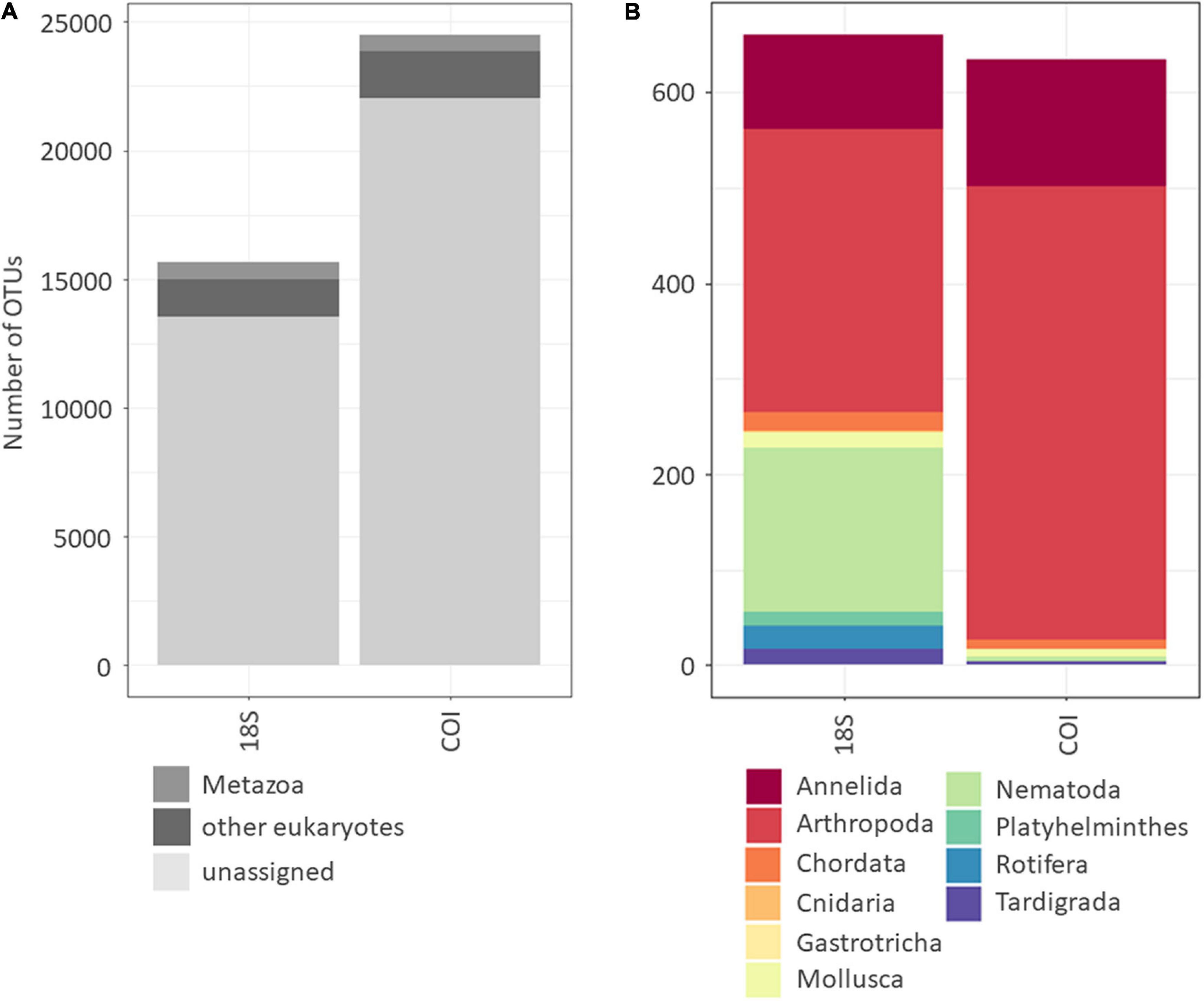
Figure 1. Number of OTUs per gene marker used (18S and COI) assigned to Bacteria, Metazoa and remaining Eukaryotes consisting of Fungi, Chromista, Protozoa, Plantae (blastID ≥97%). The number of unassigned OTUs is indicated in light gray (A). Number of OTUs assigned to Metazoa on phylum level (blastID ≥97%) (B).
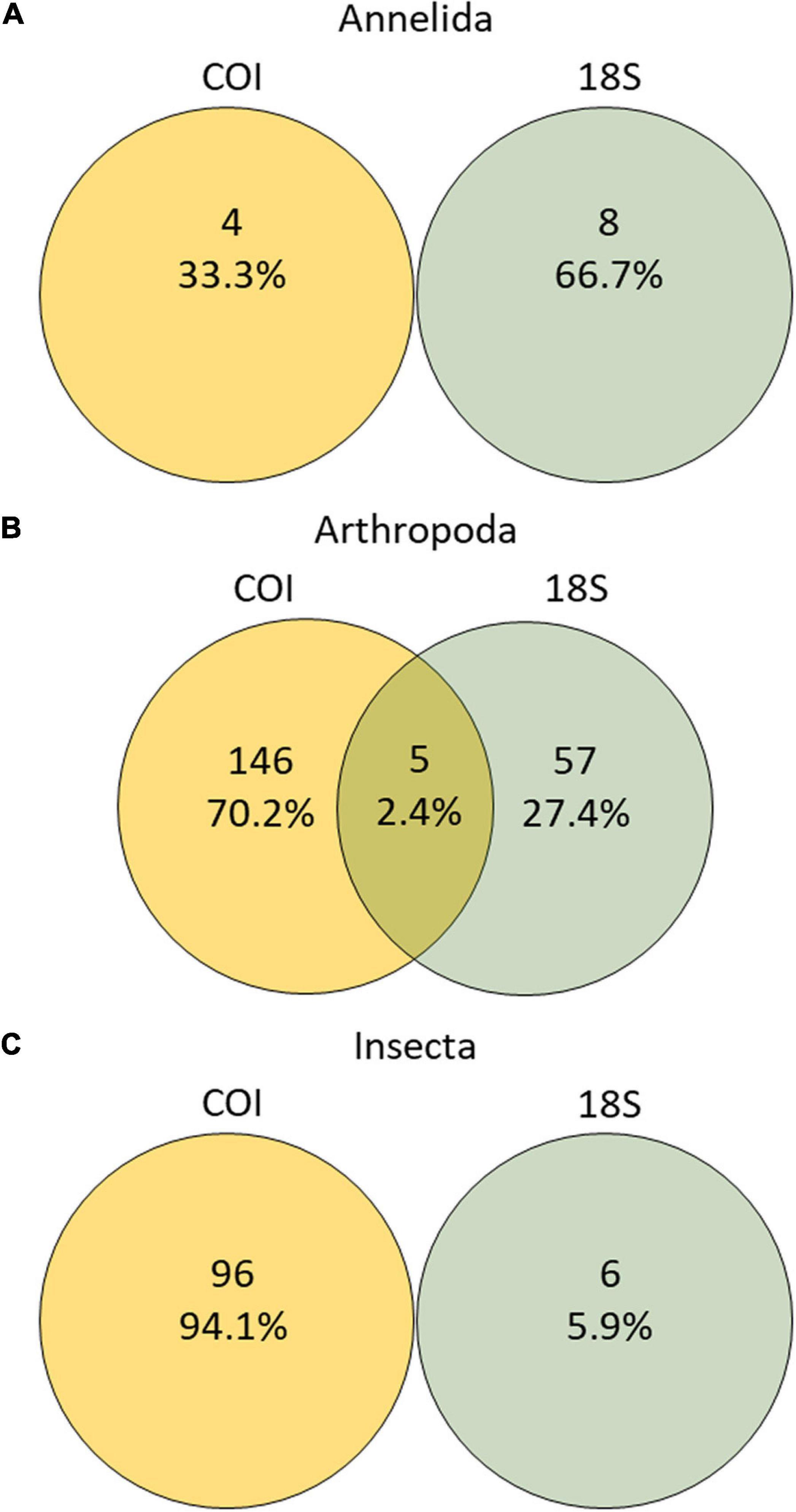
Figure 2. Number of OTUs with unique species assignment (blastID ≥99%) recovered with either or both markers for the main soil metazoan phyla (A) Annelida, (B) Arthropoda, (C) Insecta.
The number of OTUs did not vary substantially between extraction methods but more so between markers within each dataset representing either of the two extraction methods (Figure 3). For the Machery Nagel kit (MN kit) a total of 18,439 COI OTUs and 13,164 18S OTUs were found, while a total of 17,329 COI OTUs and 13,034 18S OTUs were identified with the Phosphate buffer (PB) (Figure 3). Followed by Metazoa, several OTUs were assigned to other eukaryotic taxa (for simplification herein referred to as “other Eukaryota,” mainly dominated by Fungi and protists). The 18S marker retrieved ca. seven times more OTUs assigned to “other eukaryotes” than COI, with a slightly higher number of 18S OTU numbers when using the MN kit (+1,173 OTUs) than the PB (+1,093 OTUs). For the Metazoa, the amplification of the 18S marker led to a slightly higher OTU yield when the PB was used for DNA extraction. The opposite was the case for the COI dataset, where an increase in the number of OTUs was associated with the use of the MN kit (Figure 3).
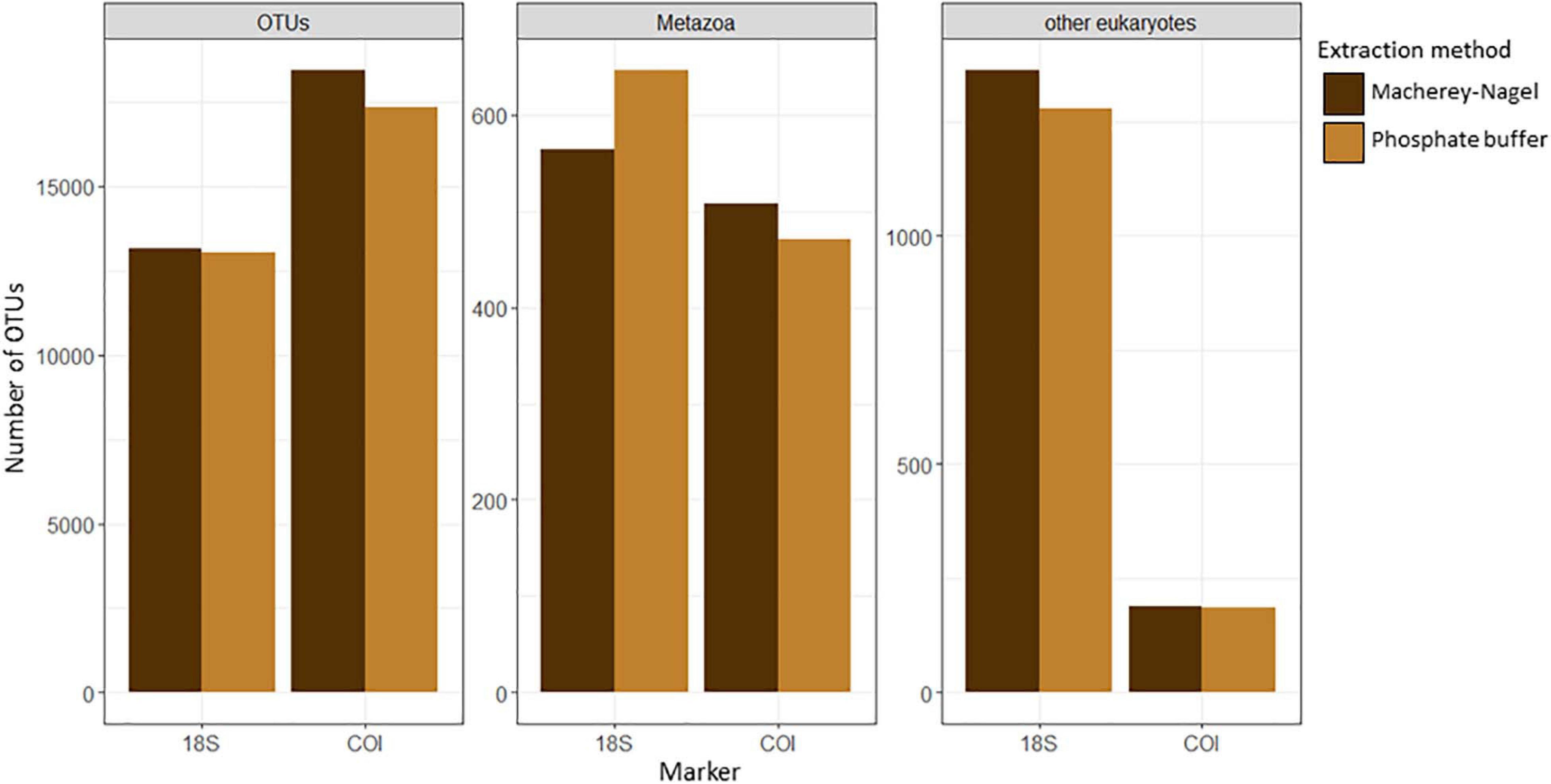
Figure 3. Total number of OTUs and number of OTUs assigned (blastID ≥97%) to Metazoa and other eukaryotes (consisting of Fungi, Chromista, Protozoa, Plantae) for the two extraction methods and markers used (18S and COI).
Accumulation (Figure 4A) and sampling effort curves (Figure 4B) from a total of 162 soil samples did not reach a plateau. An extrapolation indicated that at least 400 samples must be processed with each of the two extraction methods to cover total existing diversity in our sampled environments (Figure 4A).
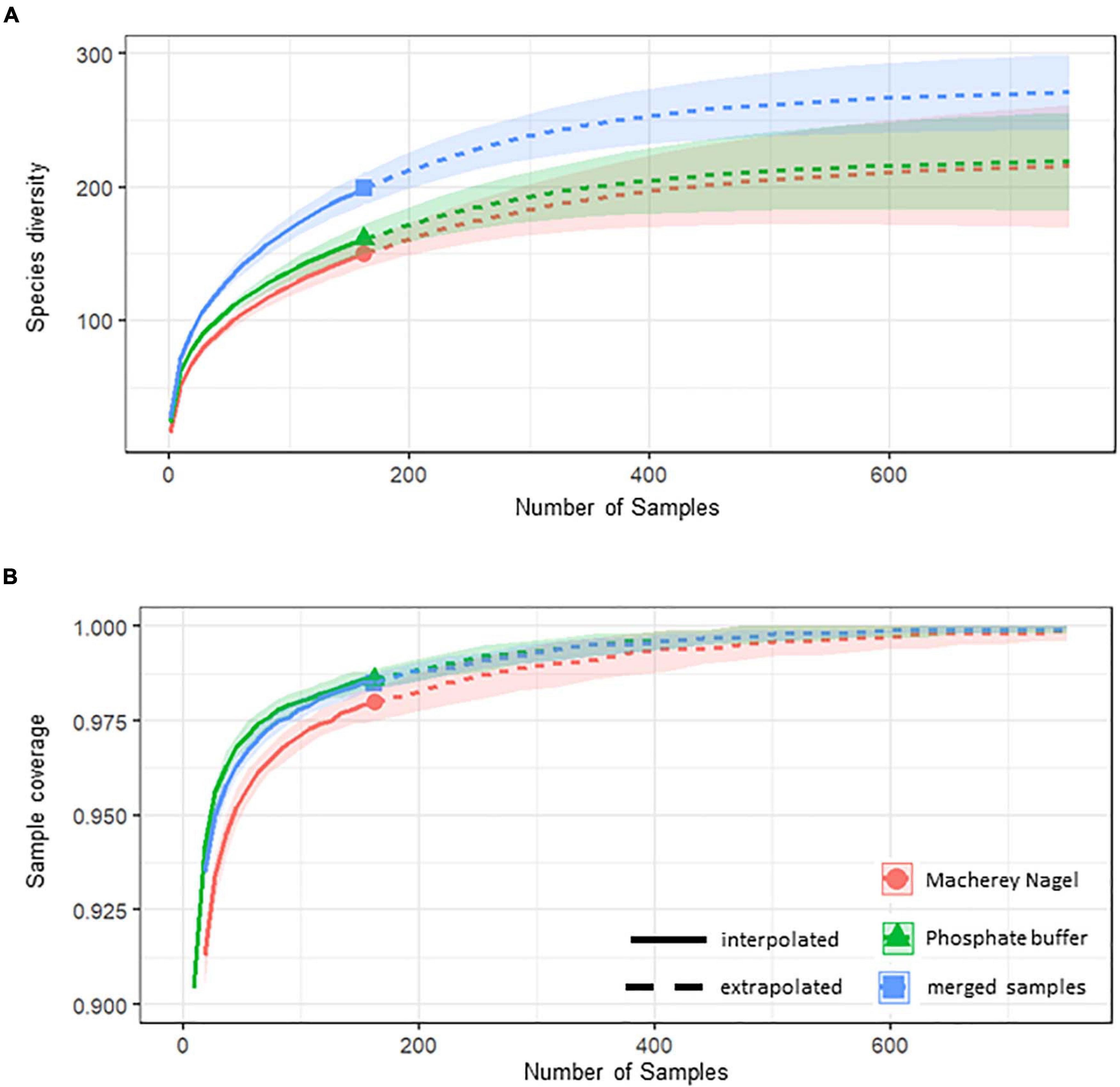
Figure 4. Sample completeness curves (solid line) with extrapolation (dashed line) showing number of detected arthropod species (blastID ≥99%) with both markers per number of soil samples taken for each extraction method (A). Additionally, sample coverage curves were calculated based on sample coverage per number of soil samples (B).
A Principal Coordinate Analysis (PCoAs) indicated major differences in the eukaryotic communities between the different extraction methods, although there was more overlap between the methods in the COI dataset. These differences between DNA extraction methods (beta diversity) were subsequently statistically confirmed (PERMANOVA, COI: F323 = 11.26, p < 0.001; 18S: F323 = 43.92, p < 0.001) (Figures 5A,B). However, for the COI as well as for the 18S dataset, a betadisper-test indicated a very heterogeneous dispersion within samples of each extraction and marker group (COI: F1 = 31.12, p = 0.001; 18S: F1 = 3.65, p < 0.05), highlighting the importance of replicates (Figures 5C,D).
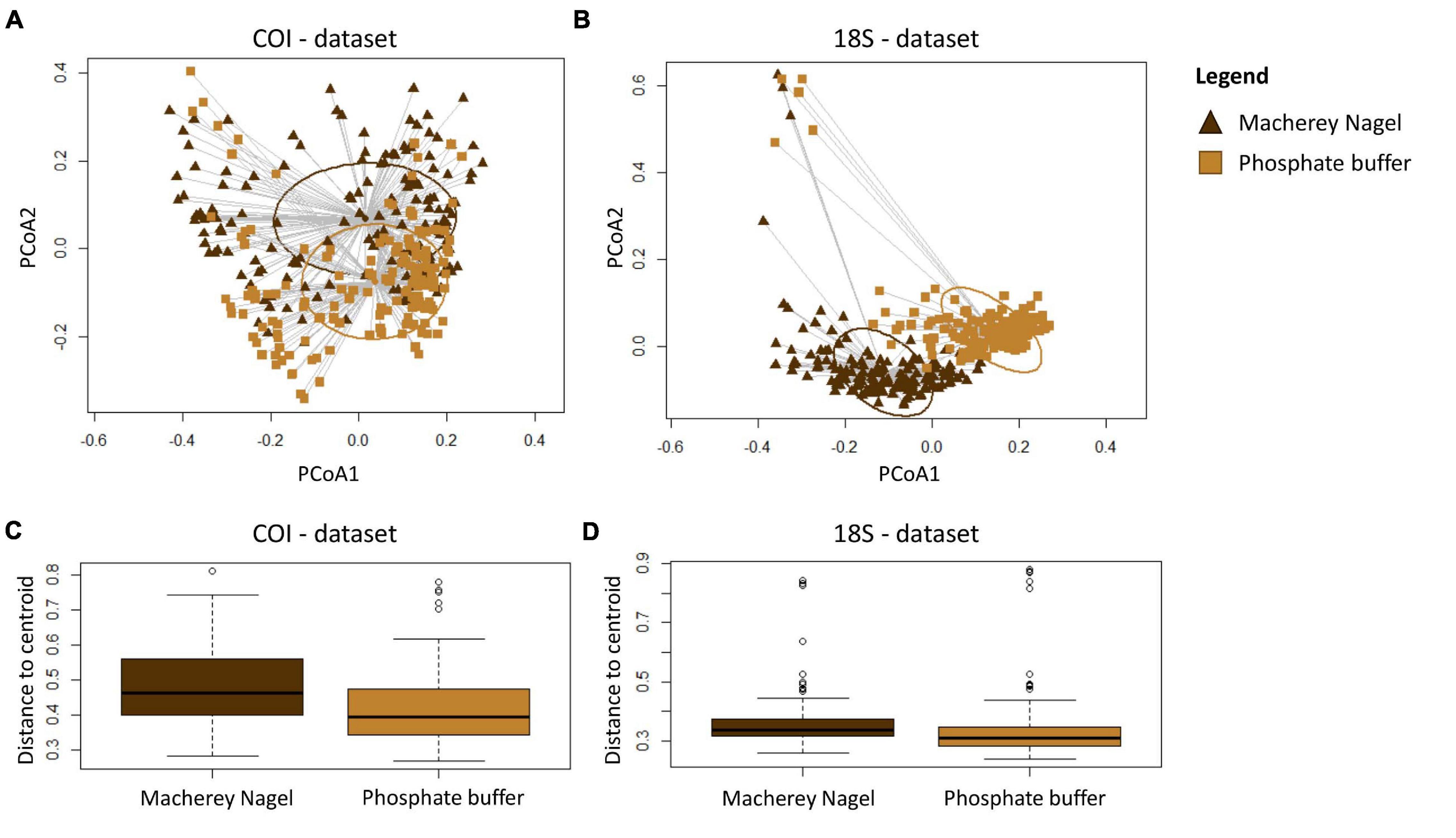
Figure 5. Eukaryotic community composition using Principal Coordinate Analysis (PCoA) based on a “jaccard” dissimilarity index for the (A) COI and (B) 18S dataset for incidence data of detected OTUs with a 90% BlastID to Eukaryota. Group dispersion plots indicate homogeneity of community composition within all soil samples using the same DNA extraction method for the (C) COI dataset and (D) 18S dataset. Light brown indicates community composition assessed using the phosphate buffer; Dark brown indicates community composition assessed with the Macherey Nagel kit.
When using the 18S marker, both extraction methods shared 8 and 47 species of annelids and arthropods, respectively. No arthropod and annelid species (blastID ≥99%) were exclusively identified with the MN kit, while one annelid and 10 arthropod species were unique to the PB method (Figure 6). When using the COI marker no differences were observed in the number of annelid and arthropod species between the two methods. The same four annelid species were identified with both extraction methods (Figure 6). From a total of 107 arthropod species, 68 were uncovered by both extraction methods and 39 species were unique to each method (Figure 6).
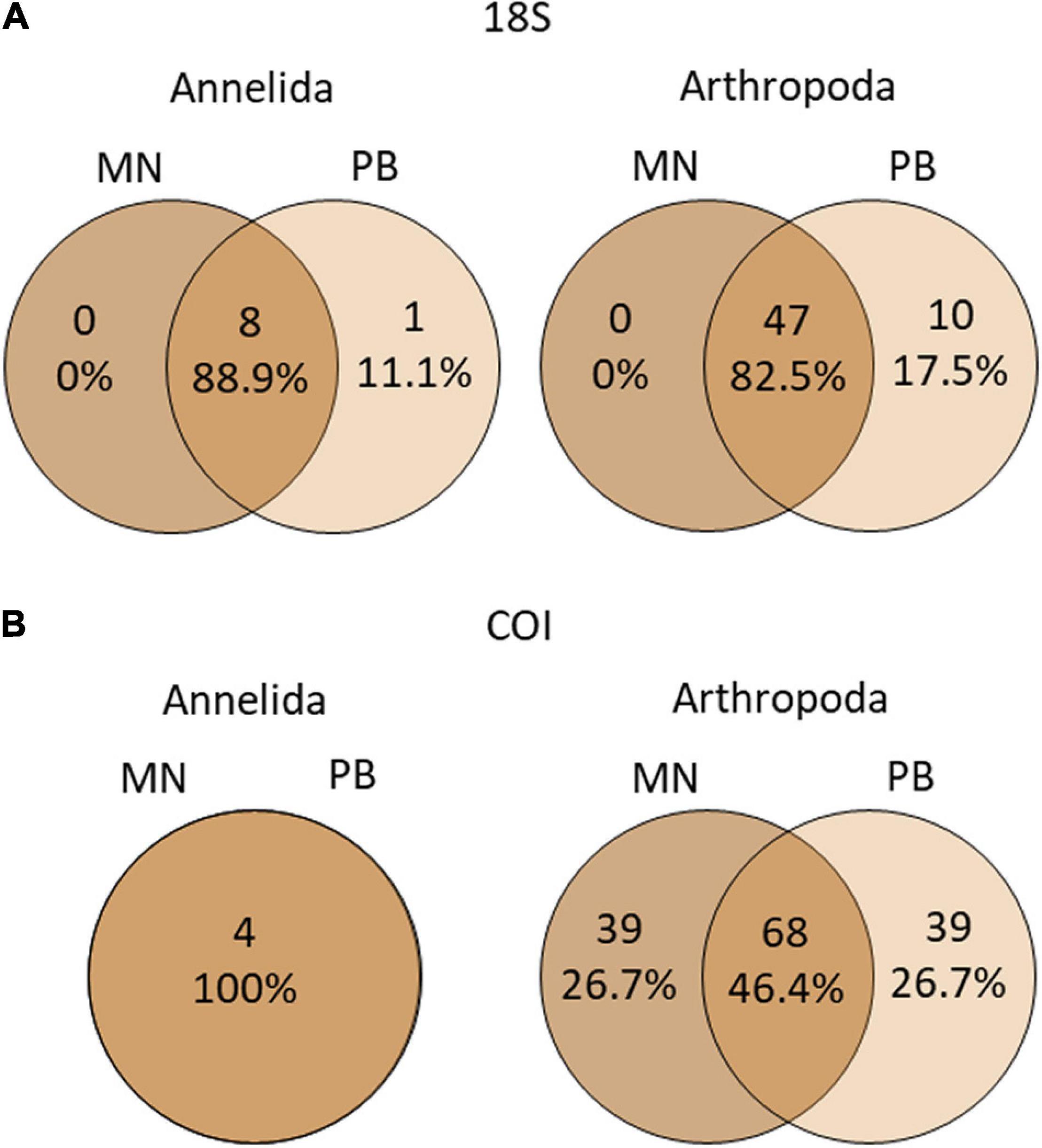
Figure 6. Venn diagram showing number of shared and unique Annelida and Arthropoda species (blastID ≥99%) per extraction method for the (A) 18S and (B) COI marker.
Based on the complete dataset, seasonal differences were observed between the two DNA extraction methods. The summer season retrieved the highest number of arthropod species when using the MN kit (36) as opposed to the PB (23). The MN kit also showed a peak in Diptera diversity during summer (20) as opposed to the autumn (6), winter (3), and spring (4) seasons (Figure 7). The number of insect species identified during autumn was 29 for the MN kit and 31 for the PB, respectively, with 19 species uncovered by both methods. For winter and spring seasons the PB uncovered more insect species, with 36 species in each season, while using the MN kit 26 and 27 species were found, respectively (Figure 7). While the MN kit resulted in the detection of a higher number of dipteran species in summer, in each season more coleopteran species were identified by the PB (summer: + 2; autumn: + 5; winter: + 7; spring: + 8). When considering data from all seasons and forest types 17 coleopteran species were exclusively detected with the phosphate buffer, while the MN kit exclusively revealed the presence of five coleopteran species. For the dipterans, extraction with the MN kit resulted in the exclusive detection of 18 species but the same method left seven species undetected which were found by the PB. Depending on the dipteran family, differences in relative species count were observed between the two extraction methods. Based on the COI marker more species for the families Sciaridae (+2) Mycetophilidae (+3), Limoniidae (+3), and Phoridae (+4) were retrieved with the MN kit (Supplementary Figure 4).
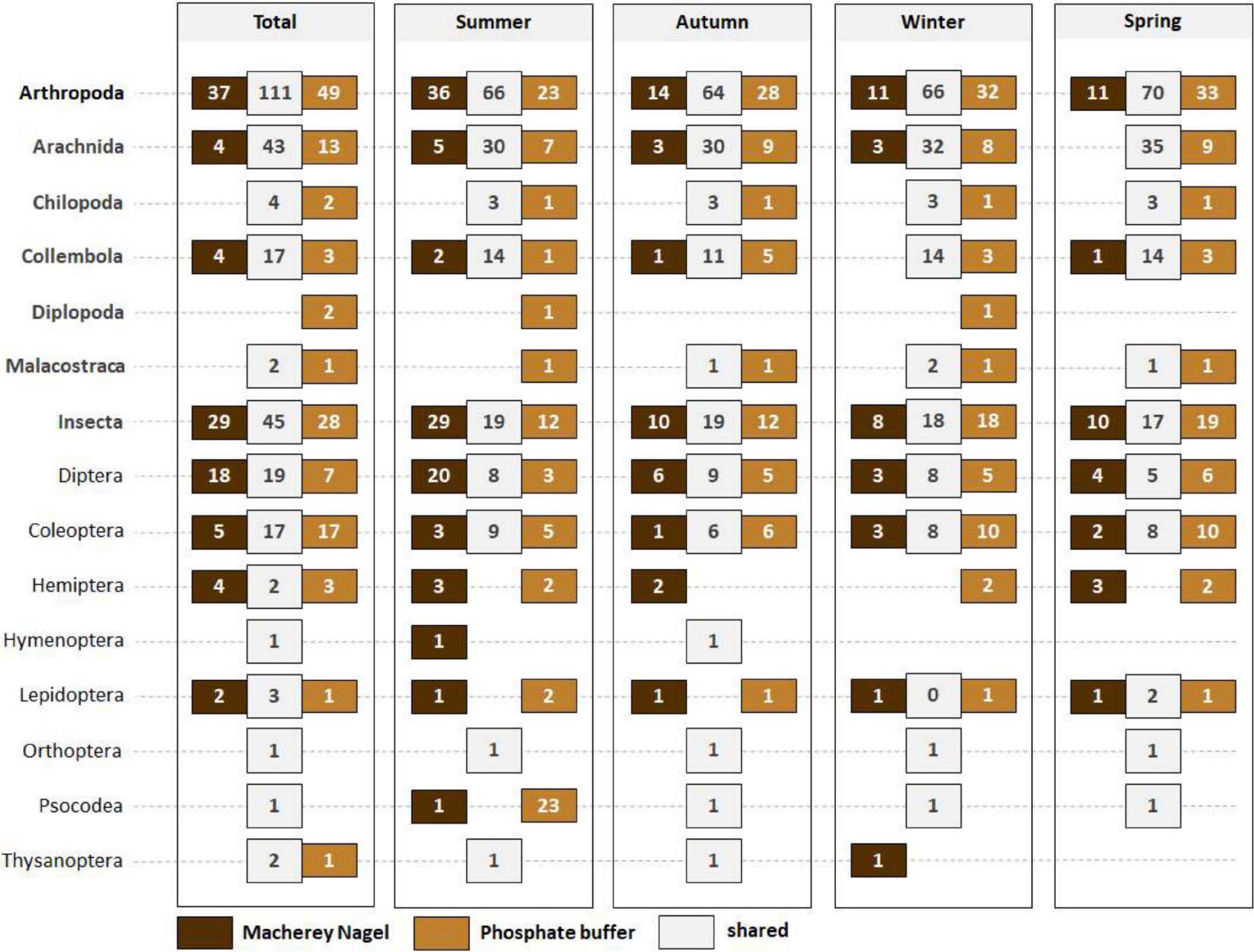
Figure 7. Number of shared and unique arthropod species (blastID ≥99%) found between extraction methods for each season using both markers. The number of species per arthropod class and insect order recovered with either one or both of the two extraction methods is shown. Dark brown: OTUs from Macherey Nagel kit; Light brown: OTUs from phosphate buffer; White box: shared OTUs between Macherey Nagel kit and phosphate buffer.
SIMPER analysis revealed that Athous subfuscus (10.64%), Ctenosciara lutea (6.42%), Corynoptera globiformis (4.30%), Cratyna perplexa (4.00%), and Strophosoma melanogrammum (3.82%) had a key influence on the assessed community differences between extraction methods in the summer season. In autumn, next to C. globiformis (7.10%) and Athous subfuscus (9.75%) also Tipula limbata (10.23%), Othius myrmecophilus (8.52%), and Barypeithes araneiformis (5.97%) were significantly contributing to the observed differences. In winter, again A. subfuscus (12.19%), C. globiformis (7.75%), O. myrmecophilus (6.36%), and T. limbata (6.01%) were under the five species responsible for observed differences. Additionally, a high contribution (%) was also observed for Corynoptera minima (6.83%). In winter season, A. subfuscus (12.73%), T. limbata (8.64%), C. globiformis, S. melanogrammum, and Berypeithes pellucidus were the five species identified as most relevant for observed differences between extraction methods (Table 1).
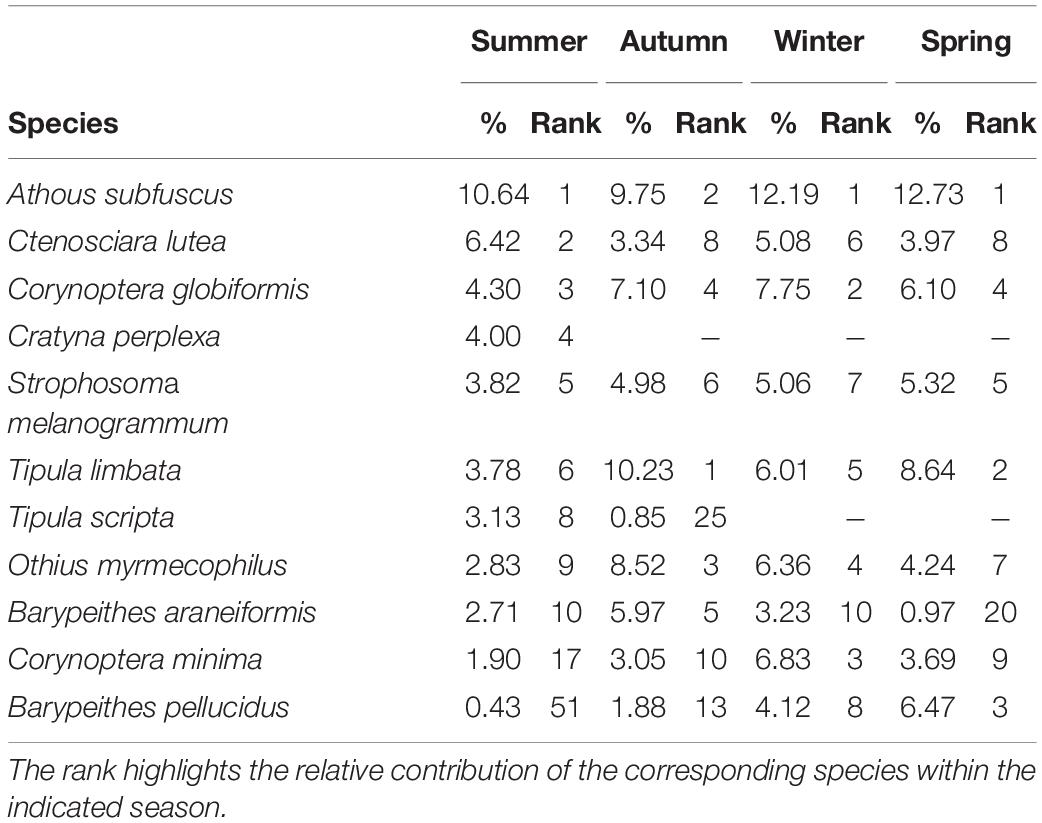
Table 1. SIMPER analysis showing the contribution (%) of Insect OTUs assigned on species level with a blastID of at least 99% to the differences between extraction methods depending on season.
Discussion
This study demonstrates that extraction methods can greatly influence the levels of diversity and species uncovered in a specific location and this is even more significant depending on the targeted taxa and gene used. Many factors can influence eDNA yields from soil samples, namely organic content and humic substances, choice of buffer and purification steps utilized (Frostegård et al., 1999), and thereby the completeness of species lists retrieved. Dopheide et al. (2019) found a correlation between the amount of source material and the number of species retrieved, a finding which is partly contradicting the results of this study. Here we found that the amount of starting material did not significantly influence the number of species retrieved, but more so the taxonomic composition and representativeness of the sampled area. However, it cannot be excluded that the detection of several species exclusively found by the phosphate buffer were also associated with the larger amount of source material used (Taberlet et al., 2012; Dopheide et al., 2019). However, we argue that the taxonomic differences found between the DNA extraction methods are partly inherent to the specificities of the protocols. Up to 44% of species identified were unique to each method showing that half of the species would not have been identified if using one extraction method only, disproving a positive effect of sample size on completeness of community composition.
Both markers recovered a high number of OTUs assigned to groups outside the Metazoa, which supports the understanding of significant non-targeted amplification (Yu et al., 2012; Yang et al., 2014; Giebner et al., 2020). The COI barcode is especially limited when working with eDNA due to the vast diversity of the DNA mixture (Deagle et al., 2014). This marker is known to fail to amplify some groups of arthropods (Marquina et al., 2019a), especially in eDNA samples where primers are rarely universal and have different amplification efficiencies. In this study a high proportion of the COI OTUs found could not be taxonomically assigned, probably because the COI marker is less well-used outside Metazoa (Kress and Ericksoneds, 2012) leading to incomplete databases. Additionally, the use of lower blast thresholds (sequence% ID ≤97%) and the presence of a consensus blast could have allowed more assignments and greater confidence in the assigned taxonomies, but such parameters were not tested in this study. While the phosphate buffer exclusively allowed the extraction of extracellular DNA, the Macherey Nagel kit included a lysis step, additionally enabling the extraction of intracellular DNA. As the highest amount of intracellular DNA in soil usually originates from microbial organisms (Taberlet et al., 2012) the application of a lysis step is expected to lead to an accumulation of microbial DNA in the DNA extract. Nonetheless, we observed that lysis also allowed the detection of specific invertebrate groups, namely temporary mesofauna (e.g., transient life stages). In the summer, extraction with the Macherey Nagel kit indicated a peak in dipteran diversity, in particular for the families Sciaridae, Mycetophilidiae, Liimonidae, and Phoridae (Supplementary Figure 5) which are known to have larval stages developing in the soils (Barnard, 2011; Disney, 2012; Jakovlev, 2012). From the ten species identified as primarily contributing to the observed community dissimilarities between extraction methods in summer three were members of the dipteran family Sciaridae. This highlights the direct effect of choice of extraction method on the composition of the dipteran diversity found.
As the proportion with which a species contributes to the DNA mixture directly influences its detection probability (Elbrecht et al., 2017, 2019), lysis can facilitate the detection of transient species, but for the costs of a lower detection probability of DNA traces. Although little is known about natural eDNA release processes in soil and how they might vary between species it can be expected that detection rate is affected by population density, whereby highly abundant species together with high primer affinities will likely be PCR amplified more efficiently, with concomitantly higher amplification success and more reads (Hajibabaei et al., 2011; Brandon-Mong et al., 2015). Former studies indicated that annelids can reach abundances of up to 134,000 specimens per m2 (Coleman et al., 2004), with fecal pellets up to 29% of the volume of the higher soil A- horizon (Davidson et al., 2002). Here, with only 0.5 g of soil using the Macherey Nagel kit, we captured exceptionally high levels of oligochaete Enchytraeid DNA, but both extraction methods captured the same species, probably due to their high abundance and biomass in soils. Although the number of dipteran species exclusively recovered with the Macherey-Nagel kit exceeded the number detected with the phosphate buffer, a high number of small sized dipteran Sciaridae species was also recovered with both extraction methods. In dipterans up to 14,500 larvae can accumulate on very narrow areas (Altmüller, 1977), which can result in an accumulation of DNA traces detectable with both methods.
Soils are heterogenous and stratified, either horizontally or vertically thus sampling larger quantities of soil will allow a better representativeness and homogeneity between replicates. Consequently, size and replication will be key when targeting larger organisms, such as meso- and macrofauna. Here, we observed that soil communities were indeed taxonomically more similar between sample replicates when using the phosphate buffer (Supplementary Figures 3A,B). Such findings corroborate the idea that using larger amounts of soil for DNA extraction will increase the chances to assess a more complete picture of the existing invertebrate diversity. Similarly, the rarefaction curves evidenced the need to increase the sampling effort and combine different methods whenever possible, since a total of 162 soil samples did not reach a plateau and at least 400 samples would be needed from each extraction method to cover the existing diversity in our sampled environments. By doing so, we would have been able to assess the arthropod diversity at a given area, as shown by the leveled sampling and species efforts curves. The relatively high percentage of species exclusively recovered from one sampling site using either extraction method substantiates the fact that even at small scales there is a large variation in soil community composition. A more extensive sampling and the combination of different extraction methods can therefore lead to higher local-diversity levels (alpha-diversity) which are commonly found in soils (Nielsen et al., 2010).
Both COI and 18S markers showed non-targeted amplification, but for Metazoa, the 18S gene identified three times more phyla than the COI from the forest soil eDNA samples. This is mainly due to the highly conserved priming sites in 18S, that allow amplification across broader taxonomic groups (Hebert et al., 2003; Zhang et al., 2018). Due to COI marker having a higher taxonomic resolution for Metazoa and especially Arthropoda, more OTUs had an assignment to these phyla relative to the 18S marker. Whereas, the lower number of 18S OTUs assigned to Metazoa were likely because some sequences originating from different species/genus are merged into the same OTU due to the limited species-level resolution in the 18S marker (Potter et al., 2017). However, it must be noted that many COI OTUs did not get a taxonomic assignment mainly because available COI databases can still be fragmentary for some taxonomic groups (Clarke et al., 2017). When focusing on the two main metazoan phyla Annelida and Arthropoda, we observed that it was mainly the marker used that influenced the number OTUs retrieved per phyla. While with the 18S marker more arthropod and annelid species (blastID ≥99%) were detected when the extraction was conducted with the phosphate buffer. Conversely, when using the COI marker the extraction method did not influence the number of arthropod and annelid species identified. As previously mentioned, the 18S marker at a 99% nucleotide divergence threshold is prone to underestimate the real diversity of several metazoans at lower taxonomic levels, namely the Arthropoda (Tang et al., 2012; Drummond et al., 2015). While the primer binding sites of the 18S marker are more conserved (Clarke et al., 2017), its species-level resolution is strongly hampered by the lack of variability within the discriminative region (Tang et al., 2012; Yang et al., 2013). However, due to its low variability in primer sites, amplification success of the 18S marker might be less influenced by the complexity and composition of the DNA mixture, as opposed to COI, since primer affinities are substantially more similar for the majority of taxa.
So far, no primer or single gene region has been identified that will amplify all taxa in eDNA samples and assessments of complete biodiversity are nearly impossible. The combination of several genetic markers can allow better estimates of biodiversity in a given habitat (Drummond et al., 2015; Zhang et al., 2018; Marquina et al., 2019a, b), especially when looking at different phyla or samples with high taxonomic diversity. For example the COI marker is not suitable to identify nematodes and the 18S marker alone would not be suitable to target specific arthropods, due to the specificities of the markers (impairing higher taxon delineation) and available databases. In fact, a recent study found that the combination of at least two markers can improve taxonomic resolution by up to 10% (Marquina et al., 2019a) and can significantly increase the number of target invertebrate taxa. Notwithstanding some studies targeting arthropods using multiple COI primers suggest that when targeting taxonomic groups with limited diversity the use of multiple primer sets could represent unnecessary costs with no substantial improvement in taxon detection (Elbrecht et al., 2019), allowing maximum richness but not affecting beta diversity (Hajibabaei et al., 2019). Despite the fact that the COI barcode covers up to 95% of several groups of organisms (Hajibabaei et al., 2007), it is not an all-purpose answer as its taxonomic resolution and coverage is limited for many invertebrate taxa (Kvist, 2014; Creer et al., 2016). Due to the absence of a COI barcoding gap for earthworms (Bienert et al., 2012; Kvist, 2014) and the low taxonomic resolution of the 18S marker (Tang et al., 2012), none of the 12 annelid species identified were simultaneously retrieved by both markers. This demonstrates how complementary nuclear and mitochondrial markers can be (Drummond et al., 2015; Giebner et al., 2020) and how incorporating these strategies can impact further biodiversity and ecological assessment on a given habitat.
The results presented here highlight that prior knowledge about the target group and an understanding of the methodological trade-offs is required to allow for decisions that can significantly improve taxon detection. Based on our results, we suggest the following recommendations for invertebrate biodiversity assessment from forest soil samples: (1) Choice of marker should be carefully considered based on target groups (e.g., CO1 for arthropods, 18S for nematodes, platyhelminthes, rotifers, and tardigrades); (2) The use of a phosphate buffer is suitable for the detection of eDNA traces from macro invertebrates which actively interact with their habitat; (3) The use of a lysis based extraction method is more suitable for the detection of micro-invertebrates as well as other life stages of macro invertebrates such as eggs and larvae; (4) Sampling effort can be maximized by combining several DNA extraction methods, but this will add to cost (5) the use of a multi-marker approach (markers or primer pairs, depending on the study objectives) will improve taxon recovery in environmental samples with high taxonomic diversity and concomitantly better reflect biodiversity levels, but this will add to cost; (7) Sampling effort to cover mesofaunal diversity in the forest ecosystem under study should be high (ca. 500 forest soil samples using both extraction methods).
This study adds recommendations on key aspects for processing soil samples, from sampling effort to the importance of the DNA extraction method chosen and the use of a multiplex marker approach, which will allow a better assessment of diversity levels in one of the most species-rich habitats, the soils. We show that eDNA is an effective tool that allows diversity assessments of soil invertebrate communities, but its efficacy relies (but not only) on a combined effect of the method used, the development of specific primer pairs or multiplex approach and the completeness of public databases.
Data Availability Statement
The datasets presented in this study can be found in online repositories. The names of the repository/repositories and accession number(s) can be found below: NCBI BioProject, accession no: PRJNA681091.
Author Contributions
VF conceived the original idea. VF and SB supervised the project. AK and KL carried out the field and lab work. AK performed the analysis and wrote the manuscript with support from SB and VF. All authors provided critical feedback and helped shape the research, analysis and manuscript.
Funding
This study was partly funded by the German Federal Ministry of Education and Research, through the project German Barcode of Life (GBOL1, FKZ01LI1101 and GBOL2, FKZ01LI1501).
Conflict of Interest
The authors declare that the research was conducted in the absence of any commercial or financial relationships that could be construed as a potential conflict of interest.
Acknowledgments
We thank Sönke Twietmeyer from the Eifel Nationalpark for his support during the field work phase. We would also like to thank all helpers who went with us to the field and helped us to collect soil samples. This study contains material that has previously formed part of my Ph.D. thesis which will be published according to the requirements of the institution awarding the qualification (University of Bonn).
Supplementary Material
The Supplementary Material for this article can be found online at: https://www.frontiersin.org/articles/10.3389/fevo.2021.630560/full#supplementary-material
Supplementary Figure 1 | Location of the sampling sites. The area highlighted in purple corresponds to the Eifel National Park. Maps were downloaded from https://www.geoportal.nrw/ on 20th of November 2019 and modified according to our purposes using Microsoft Power Point 2016.
Supplementary Figure 2 | Number of collected soil samples per forest type and season. In summer three sampling sites per forest type were sampled. At each sampling site three replicates were taken. In the remaining four seasons triplicates were taken at 14 sampling sites. While three sampling sites were located at the pure beech and young beech sites, respectively, (9 samples per forest type and season), at the pure spruce and old beech forests triplicates were taken at four sampling sites (12 samples were forest type and season). In total 162 samples were collected.
Supplementary Figure 3 | (A) Number of unique and shared species between sampling sites (cardinals) depending on forest type (columns) and season (rows) using the Macherey-Nagel kit. The data shown here comprises all arthropod species detected with either one or both of the two used markers (18S and COI). Only species detected with a BlastID of at least 99% to the reference databases are considered. (B) Number of unique and shared species between sampling sites (cardinals) depending on forest type (columns) and season (rows) based on the extraction with the phosphate buffer. The data shown here comprises all arthropod species detected with either one or both of the two used markers (18S and COI). Only species detected with a blastID of at least 99% to the reference databases are considered.
Supplementary Figure 4 | Number of detected dipteran species (blastID ≥ 99%) per family depending on extraction method.
Supplementary Figure 5 | Number of detected dipteran species (blastID 99%) per family in summer season depending on extraction method.
Supplementary Table 1 | Geographical location and ecological characteristics of the 14 sampling sites. For each sampling site, the coordinates (altitude N and latitude E) and the associated forest type are specified.
Supplementary Table 2 | Time of sampling. For each sampling period the season, associated time of the year, number of samples taken and sampling dates (d,m,y) are specified.
Supplementary Table 3 | Presence/absence OTU Table for the COI marker. The table contains information on OTU occurrence at each sampling site. Each column (2–324) represents one sample. The name of each sample (XXYYrZSX) indicates extraction method (XX), sampling site (YY), replicate number (rZ) and collection season (SX). Taxonomic assignment (Blast ID%) for assigned OTUs is indicated.
Supplementary Table 4 | Presence/absence OTU Table for the COI marker. The table contains information on OTU occurrence at each sampling site. Each column (2–324) represents one sample. The name of each sample (XXYYrZSX) indicates extraction method (XX), sampling site (YY), replicate number (rZ) and collection season (SX). Taxonomic assignment (Blast ID%) for assigned OTUs is indicated.
Supplementary Table 5 | presence/absence list of OTUs assigned at species level (blastID ≥ 90%) with either of the two extraction methods and marker. The table contains information on species occurrence at each sampling site. Each column (2–324) represents one sample. The name of each sample (XXYYrZSX) indicates extraction method (XX), sampling site (YY), replicate number (rZ), and collection season (SX).
Footnotes
References
Alberdi, A., Aizpurua, O., Gilbert, M. T. P., and Bohmann, K. (2018). Scrutinizing key steps for reliable metabarcoding of environmental samples. Methods Ecol. Evol. 9, 134–147. doi: 10.1111/2041-210x.12849
Altmüller, R. (1977). “Ökoenergetische untersuchungen an dipterenpopulationen im buchenwald,” in Verhandlungen der Gesellschaft für Ökologie, Göttingen 1976: 6. Jahresversammlung vom 20. bis 24. September 1976 in Göttingen, ed. P. Müller, (Dordrecht: Springer Netherlands), 133–138. doi: 10.1007/978-94-011-5957-9_13
Altschul, S. F., Gish, W., Miller, W., Myers, E. W., and Lipman, D. J. (1990). Basic local alignment search tool. J. Mol. Biol. 215, 403–410.
Barnard, P. C. (2011). The Royal Entomological Society Book of British Insects. New York, NY: John Wiley & Sons.
Baselga, A., and Orme, C. D. L. (2012). betapart: an R package for the study of beta diversity. Methods Ecol. Evol. 3, 808–812. doi: 10.1111/j.2041-210x.2012.00224.x
Bienert, F., De Danieli, S., Miquel, C., Coissac, E., Poillot, C., Brun, J., et al. (2012). Tracking earthworm communities from soil DNA. Mol. Ecol. 21, 2017–2030. doi: 10.1111/j.1365-294x.2011.05407.x
Brandon-Mong, G.-J., Gan, H.-M., Sing, K.-W., Lee, P.-S., Lim, P.-E., and Wilson, J.-J. (2015). DNA metabarcoding of insects and allies: an evaluation of primers and pipelines. Bull. Entomol. Res. 105, 717–727. doi: 10.1017/s0007485315000681
Caporaso, J. G., Kuczynski, J., Stombaugh, J., Bittinger, K., Bushman, F. D., Costello, E. K., et al. (2010). QIIME allows analysis of high-throughput community sequencing data. Nat. Methods 7, 335–336. doi: 10.1038/nmeth.f.303
Chen, H., and Boutros, P. C. (2011). VennDiagram: a package for the generation of highly-customizable Venn and Euler diagrams in R. BMC Bioinform. 12:35. doi: 10.1186/1471-2105-12-35
Clarke, L. J., Beard, J. M., Swadling, K. M., and Deagle, B. E. (2017). Effect of marker choice and thermal cycling protocol on zooplankton DNA metabarcoding studies. Ecol. Evol. 7, 873–883. doi: 10.1002/ece3.2667
Coleman, D. C., Crossley, D. A. Jr., and Hendrix, P. F. (2004). Fundamentals of Soil Ecology. London: Academic Press.
Creer, S., Deiner, K., Frey, S., Porazinska, D., Taberlet, P., Thomas, W. K., et al. (2016). The ecologist’s field guide to sequence-based identification of biodiversity. Methods Ecol. Evol. 7, 1008–1018. doi: 10.1111/2041-210x.12574
Davidson, D., Grieve, I., and Young, I. (2002). Impacts of fauna on an upland grassland soil as determined by micromorphological analysis. Appl. Soil Ecol. 20, 133–143. doi: 10.1016/s0929-1393(02)00017-3
Deagle, B. E., Jarman, S. N., Coissac, E., Pompanon, F., and Taberlet, P. (2014). DNA metabarcoding and the cytochrome c oxidase subunit I marker: not a perfect match. Biol. Lett. 10:20140562. doi: 10.1098/rsbl.2014.0562
Deiner, K., Bik, H. M., Mächler, E., Seymour, M., Lacoursière-Roussel, A., Altermatt, F., et al. (2017). Environmental DNA metabarcoding: transforming how we survey animal and plant communities. Mol. Ecol. 26, 5872–5895. doi: 10.1111/mec.14350
Delgado-Baquerizo, M., Powell, J. R., Hamonts, K., Reith, F., Mele, P., Brown, M. V., et al. (2017). Circular linkages between soil biodiversity, fertility and plant productivity are limited to topsoil at the continental scale. New Phytol. 215, 1186–1196. doi: 10.1111/nph.14634
Delmont, T. O., Robe, P., Cecillon, S., Clark, I. M., Constancias, F., Simonet, P., et al. (2011). Accessing the soil metagenome for studies of microbial diversity. Appl. Environ. Microbiol. 77, 1315–1324. doi: 10.1128/aem.01526-10
Dopheide, A., Xie, D., Buckley, T. R., Drummond, A. J., and Newcomb, R. D. (2019). Impacts of DNA extraction and PCR on DNA metabarcoding estimates of soil biodiversity. Methods Ecol. Evol. 10, 120–133. doi: 10.1111/2041-210x.13086
Drummond, A. J., Newcomb, R. D., Buckley, T. R., Xie, D., Dopheide, A., Potter, B. C., et al. (2015). Evaluating a multigene environmental DNA approach for biodiversity assessment. GigaScience 4:s13742-15.
Elbrecht, V., Braukmann, T. W., Ivanova, N. V., Prosser, S. W., Hajibabaei, M., Wright, M., et al. (2019). Validation of COI metabarcoding primers for terrestrial arthropods. PeerJ 7:e27801v1.
Elbrecht, V., Peinert, B., and Leese, F. (2017). Sorting things out: assessing effects of unequal specimen biomass on DNA metabarcoding. Ecol. Evol. 7, 6918–6926. doi: 10.1002/ece3.3192
Epp, L. S., Boessenkool, S., Bellemain, E. P., Haile, J., Esposito, A., Riaz, T., et al. (2012). New environmental metabarcodes for analysing soil DNA: potential for studying past and present ecosystems. Mol. Ecol. 21, 1821–1833. doi: 10.1111/j.1365-294x.2012.05537.x
Fonseca, V. G., and Lallias, D. (2016). Metabarcoding marine sediments: preparation of amplicon libraries. Methods Mol Biol. 1452, 183–196. doi: 10.1007/978-1-4939-3774-5_12
Frøslev, T. G., Kjøller, R., Bruun, H. H., Ejrnæs, R., Brunbjerg, A. K., Pietroni, C., et al. (2017). Algorithm for post-clustering curation of DNA amplicon data yields reliable biodiversity estimates. Nat. Commun. 8, 1–11. doi: 10.1038/s41467-017-01312-x
Frostegård, Å, Courtois, S., Ramisse, V., Clerc, S., Bernillon, D., Le Gall, F., et al. (1999). Quantification of bias related to the extraction of DNA directly from soils. Appl. Environ. Microbiol. 65, 5409–5420. doi: 10.1128/aem.65.12.5409-5420.1999
Gibert, C., and Escarguel, G. (2019). PER-SIMPER—a new tool for inferring community assembly processes from taxon occurrences. Glob. Ecol. Biogeogr. 28, 374–385. doi: 10.1111/geb.12859
Giebner, H., Langen, K., Bourlat, S., Kukowka, S., Mayer, C., Astrin, J., et al. (2020). Comparing diversity levels in environmental samples: DNA sequence capture and metabarcoding approaches using 18S and COI genes. Mol. Ecol. Resourc. 20, 1333–1345. doi: 10.1111/1755-0998.13201
Hajibabaei, M., Porter, T. M., Wright, M., and Rudar, J. (2019). COI metabarcoding primer choice affects richness and recovery of indicator taxa in freshwater systems. PLoS One 14:e0220953. doi: 10.1371/journal.pone.0220953
Hajibabaei, M., Shokralla, S., Zhou, X., Singer, G. A., and Baird, D. J. (2011). Environmental barcoding: a next-generation sequencing approach for biomonitoring applications using river benthos. PLoS One 6:e17497. doi: 10.1371/journal.pone.0017497
Hajibabaei, M., Singer, G. A., Clare, E. L. and Hebert, P. D. N. (2007). Design and applicability of DNA arrays and DNA barcodes in biodiversity monitoring. BMC Biol. 5:24. doi: 10.1186/1741-7007-5-24
Hebert, P. D. N., Cywinska, A., Ball, S. L., and deWaard, J. R. (2003). Biological identifications through DNA barcodes. Proc. R. Soc. Lond. Ser. B Biol. Sci. 270, 313–321. doi: 10.1098/rspb.2002.2218
Hsieh, T., Ma, K., and Chao, A. (2016). iNEXT: an R package for rarefaction and extrapolation of species diversity (H ill numbers). Methods Ecol. Evol. 7, 1451–1456. doi: 10.1111/2041-210x.12613
Jakovlev, J. (2012). Fungal hosts of mycetophilids (Diptera: Sciaroidea excluding Sciaridae): a review. Mycology 3, 11–23.
Kang, S., and Mills, A. L. (2006). The effect of sample size in studies of soil microbial community structure. J. Microbiol. Methods 66, 242–250. doi: 10.1016/j.mimet.2005.11.013
Kress, W. J., and Erickson, D. L. eds (2012). “DNA barcodes: methods and protocols,” in DNA Barcodes, (Berlin: Springer), 3–8. doi: 10.1007/978-1-61779-591-6_1
Kuhlman, T., Reinhard, S., and Gaaff, A. (2010). Estimating the costs and benefits of soil conservation in Europe. Land Use Policy 27, 22–32. doi: 10.1016/j.landusepol.2008.08.002
Kvist, S. (2014). Does a global DNA barcoding gap exist in Annelida? Mitochondr. DNA Part A 27, 2241–2252.
Leray, M., Yang, J. Y., Meyer, C. P., Mills, S. C., Agudelo, N., Ranwez, V., et al. (2013). A new versatile primer set targeting a short fragment of the mitochondrial COI region for metabarcoding metazoan diversity: application for characterizing coral reef fish gut contents. Front. Zool. 10:34. doi: 10.1186/1742-9994-10-34
Marquina, D., Andersson, A. F., and Ronquist, F. (2019a). New mitochondrial primers for metabarcoding of insects, designed and evaluated using in silico methods. Mol. Ecol. Resourc. 19, 90–104. doi: 10.1111/1755-0998.12942
Marquina, D., Esparza-Salas, R., Roslin, T., and Ronquist, F. (2019b). Establishing arthropod community composition using metabarcoding: surprising inconsistencies between soil samples and preservative ethanol and homogenate from Malaise trap catches. Mol. Ecol. Resourc. 19, 1516–1530. doi: 10.1111/1755-0998.13071
Martin, M. (2011). Cutadapt removes adapter sequences from high-throughput sequencing reads. EMBnet J. 17, 10–12. doi: 10.14806/ej.17.1.200
Neher, D., Weicht, T., and Barbercheck, M. E. (2012). Linking invertebrate communities to decomposition rate and nitrogen availability in pine forest soils. Appl. Soil Ecol. 54, 14–23. doi: 10.1016/j.apsoil.2011.12.001
Nielsen, U. N., Osler, G. H., Campbell, C. D., Neilson, R., Burslem, D. F., and Van der Wal, R. (2010). The enigma of soil animal species diversity revisited: the role of small-scale heterogeneity. PLoS One 5:e11567. doi: 10.1371/journal.pone.0011567
Oliverio, A. M., Gan, H., Wickings, K., and Fierer, N. (2018). A DNA metabarcoding approach to characterize soil arthropod communities. Soil Biol. Biochem. 125, 37–43. doi: 10.1016/j.soilbio.2018.06.026
Pietramellara, G., Ascher, J., Borgogni, F., Ceccherini, M., Guerri, G., and Nannipieri, P. (2009). Extracellular DNA in soil and sediment: fate and ecological relevance. Biol. Fertil. Soils 45, 219–235. doi: 10.1007/s00374-008-0345-8
Porter, T. M., Morris, D. M., Basiliko, N., Hajibabaei, M., Doucet, D., Bowman, S., et al. (2019). Variations in terrestrial arthropod DnA metabarcoding methods recovers robust beta diversity but variable richness and site indicators. Sci. Rep. 9, 1–11.
Potter, C., Tang, C. Q., Fonseca, V., Lallias, D., Gaspar, J. M., Thomas, K., et al. (2017). De novo species delimitation in metabarcoding datasets using ecology and phylogeny. PeerJ 5:e3121v1.
R Core Team, (2013). R: A Language and Environment for Statistical Computing. Vienna: R Foundation for Statistical Computing.
Rognes, T., Flouri, T., Nichols, B., Quince, C., and Mahé, F. (2016). VSEARCH: a versatile open source tool for metagenomics. PeerJ 4:e2584. doi: 10.7717/peerj.2584
Stoeck, T., Bass, D., Nebel, M., Christen, R., Jones, M. D., Breiner, H., et al. (2010). Multiple marker parallel tag environmental DNA sequencing reveals a highly complex eukaryotic community in marine anoxic water. Mol. Ecol. 19, 21–31. doi: 10.1111/j.1365-294x.2009.04480.x
Taberlet, P., PRUD’HOMME, S. M., Campione, E., Roy, J., Miquel, C., Shehzad, W., et al. (2012). Soil sampling and isolation of extracellular DNA from large amount of starting material suitable for metabarcoding studies. Mol. Ecol. 21, 1816–1820. doi: 10.1111/j.1365-294x.2011.05317.x
Tang, C. Q., Leasi, F., Obertegger, U., Kieneke, A., Barraclough, T. G., and Fontaneto, D. (2012). The widely used small subunit 18S rDNA molecule greatly underestimates true diversity in biodiversity surveys of the meiofauna. Proc. Natl. Acad. Sci. U.S.A. 109, 16208–16212. doi: 10.1073/pnas.1209160109
Wickham, H., Francois, R., Henry, L., and Müller, K. (2015). dplyr: A Grammar of Data Manipulation. R Package Version 0.8.3 3.
Yang, C., Ji, Y., Wang, X., Yang, C., and Douglas, W. Y. (2013). Testing three pipelines for 18S rDNA-based metabarcoding of soil faunal diversity. Sci. China Life Sci. 56, 73–81. doi: 10.1007/s11427-012-4423-7
Yang, C., Wang, X., Miller, J. A., de Blécourt, M., Ji, Y., Yang, C., et al. (2014). Using metabarcoding to ask if easily collected soil and leaf-litter samples can be used as a general biodiversity indicator. Ecol. Indicat. 46, 379–389. doi: 10.1016/j.ecolind.2014.06.028
Yu, D. W., Ji, Y., Emerson, B. C., Wang, X., Ye, C., Yang, C., et al. (2012). Biodiversity soup: metabarcoding of arthropods for rapid biodiversity assessment and biomonitoring. Methods Ecol. Evol. 3, 613–623. doi: 10.1111/j.2041-210X.2012.00198.x
Zhang, G. K., Chain, F. J., Abbott, C. L., and Cristescu, M. E. (2018). Metabarcoding using multiplexed markers increases species detection in complex zooplankton communities. Evol. Appl. 11, 1901–1914. doi: 10.1111/eva.12694
Zinger, L., Chave, J., Coissac, E., Iribar, A., Louisanna, E., Manzi, S., et al. (2016). Extracellular DNA extraction is a fast, cheap and reliable alternative for multi-taxa surveys based on soil DNA. Soil Biol. Biochem. 96, 16–19. doi: 10.1016/j.soilbio.2016.01.008
Keywords: eDNA extraction, extracellular DNA, intracellular DNA, invertebrates, metabarcoding, metazoa, soil biodiversity, eDNA metabarcoding
Citation: Kirse A, Bourlat SJ, Langen K and Fonseca VG (2021) Unearthing the Potential of Soil eDNA Metabarcoding—Towards Best Practice Advice for Invertebrate Biodiversity Assessment. Front. Ecol. Evol. 9:630560. doi: 10.3389/fevo.2021.630560
Received: 17 November 2020; Accepted: 05 May 2021;
Published: 28 May 2021.
Edited by:
Katy Klymus, United States Geological Survey (USGS), United StatesReviewed by:
Stefano Mariani, Liverpool John Moores University, United KingdomTobias Guldberg Frøslev, University of Copenhagen, Denmark
Copyright © 2021 Kirse, Bourlat, Langen and Fonseca. This is an open-access article distributed under the terms of the Creative Commons Attribution License (CC BY). The use, distribution or reproduction in other forums is permitted, provided the original author(s) and the copyright owner(s) are credited and that the original publication in this journal is cited, in accordance with accepted academic practice. No use, distribution or reproduction is permitted which does not comply with these terms.
*Correspondence: Ameli Kirse, QS5LaXJzZUBsZWlibml6LXpmbWsuZGU=; Vera G. Fonseca, dmVyYS5mb25zZWNhQGNlZmFzLmNvLnVr