- 1Faculty of Interdisciplinary Studies, GEMA Center for Genomics, Ecology & Environment, Universidad Mayor, Santiago, Chile
- 2ETHICS—Estudios Transversales en Humanidades para las Ingenierías y Ciencias, Facultad de Ciencias Físicas y Matemáticas Universidad de Chile, Santiago, Chile
Social policies are commonly defined from scattered knowledge. It is hard to find examples of social policy design based on a systemic perspective, and even harder with formal modeling support. To advance in filling this gap, we studied the case of the tourism development on Barú Island. In this place, tourism is a critical economic activity based on local ecosystem services, but it is being developed without planning and outside of a sustainability focus. We build a qualitative model of the social structure of tourism development that is taking place in the locality. Model building was based on experiences and opinions of local stakeholders, gathered through participatory procedures. Using our model, we projected the future states of the system, assuming several hypothetical public policy scenarios. More specifically, we evaluated the system responses to: (a) a multivariate business–as–usual (BAU) scenario, and (b) two multivariate aprioristic scenarios, pointed to improve expected outcomes. These scenarios were based on the opinions of both stakeholders and experts. We also tested: (c) simple (univariate) aposterioristic scenarios, built from the structural analysis of the system using the sensitivity matrix. Finally, we included: (d) a trivial (and unattainable) scenario, in which every model variable was increased or decreased at convenience. The outcomes of our scenario analysis were evaluated using four different weight sets applied to the projected states of system variables, representing different strategic priorities: social-economic, environmental, mixed, and plain. Our results show that the projected outcomes differ among the tested scenarios and the strategic priority to be adopted. However, in all cases the BAU scenario was markedly the worst. For every strategic priority, some simple aposterioristic scenarios performed slightly better, as compared to the more complex aprioristic ones, and even better than the trivial scenario. This result was more noticeable under the plain strategy. We conclude that aposterioristic scenarios derived from the sensitivity matrix of the qualitative model, particularly decreasing migration risk and increasing governance strength, are the best candidates for tourism policy-making in Barú among the evaluated alternatives. These results should be put in its context and adapted before they can be transferred to other study-cases. Our findings suggest that social policy-making can benefit from structural qualitative modeling. In particular, this approach may give analytical support to the selection of intervention measures from a structural perspective, based on defined strategic priorities. For this purpose, however, the model itself and the outcomes obtained from its analysis should be updated steadily.
1. Introduction
Tourism is basically a non-extractive industry, and thus it has the potential to develop local economies on a sustainable basis (Anup, 2016). Tourism has experienced uninterrupted growth worldwide. In 2018, global tourism grew around 4.4%, whereas the world GDP grew by 3.6% (UNWTO, 2019). Note that 56% of travels are for leisure, recreation, or vacation purposes (UNWTO, 2019). In emerging economies, international tourism receipts grew 7.6/5.7% in 2017/2018, which contrasts with the 4.0/3.7% exhibited by advanced economies. In the Americas, South America presents by far the highest growth, with Colombia maintaining a leading place together with Brazil and, incidentally, Argentina. However, there are recognized social and ecological issues to the growth of the tourism industry (Higgins-Desbiolles, 2018; Chakraborty, 2020), and they should be considered for the design of strategic public policies directed to enhance a tourism–based economy (Hall, 2019).
In several South American countries, the healthy state of their ecosystems allows the development of a vigorous ecotourism industry. In places where tourism economies are still in their early stages, the proximity to well-established tourism centers may boost their development. However, the closeness to popular tourist centers often leads to increases in both the prices of services and the density of visitors, factors that could ultimately depress the value of the ecotourism offer. This is the case of Barú Island, located in northern Colombia on the Caribbean coast. In 2018, the tourism activity in Colombia increased 9% relative to 2018, and Cartagena de Indias, located close to Barú Island, was the second most visited destination after the Capital City Bogotá (MCIT, 2019). The tourist activities that take place in the study site include the use of the beach, snorkeling, diving, sailing, and hiking. The sustainability of these recreational activities, available all year round, are highly dependent on the health of marine and terrestrial ecosystems. Remarkably, water quality, air quality, marine biodiversity, terrestrial vegetation and fauna, among other ecosystem features, support ecotourism—and economic—development in Barú. The country, but particularly the inhabitants of Barú, deserve strategic public policies that promote the systemic development of sustainable local tourism. However, social policies, including tourism ones, are commonly defined inorganically, from scattered knowledge and following decision-making procedures with a weak participatory basis. It is hard to find examples of social policy design based on a systemic perspective, and even harder with formal modeling support.
In this study, we present an approach to support strategic policy decision-making based on scientific modeling. We apply our tools to contribute to the socio–ecological development of Barú Island, taken as a case study. To this end, we first develop a qualitative model of the structure of the tourism development in Barú, on the grounds of participatory methods. Then, we use our model to comparatively assess the performance of alternative public policy scenarios by analyzing the mathematical projections of the system states. As detailed below, we construct the competing scenarios from two contrasting standpoints, aprioristic and aposterioristic ones. For reference, we included a business-as-usual scenario that represent the absence of any active policy on tourism development. The predicted outcomes of policy scenarios are evaluated in reference to predefined strategic priorities. We adopted a structural qualitative modeling strategy, employing the formalism of sidigraphs. This choice allows us to obtain a picture of the system based on the direct causal influences among its main elements. Furthermore, sidigraph-based models are amenable to various useful forms of structural analysis (Hage and Harary, 1983; Dambacher et al., 2003; Dambacher and Ramos-Jiliberto, 2007) and we consider this approach as especially suitable for the modeling and analysis of a wide spectrum of socio-ecological systems. The novel aspect of our contribution rests on the interplay between sidigraph-based qualitative modeling, participatory model building and scenario analysis, applied to the development of strategic public policies.
2. Materials and Methods
2.1. Study System
Barú Island in Colombia is a peninsula converted into an artificial island by a navigation channel, the Dique Channel. In a land area of near 7,200 ha, Barú Island is populated by about 7,800 inhabitants and it is located 33 km away from the renowned touristic city Cartagena de Indias. Barú Island presents a rich and integrated set of priority ecosystems (coral reefs, meadows, mangroves, tropical dry forests, temporary channels, coastal lagoons), rich birdlife, and high marine biodiversity, besides archaeological and cultural heritage areas. The marine areas of Barú are part of a protected site that includes one of the largest barrier reefs in the Colombian Caribbean. These areas, having a high ecological value, are under strong pressure and threat from current disorderly tourism development and future large-scale tourism projects (Barrios, 2000; D́ıaz et al., 2003; Andrade, 2011). However, Barú is a socially fragile rural system, with an impoverished population that exhibits low income levels, underemployment, and deficient standards in education and health services. It also has a weak territorial structure and a fragile, largely informal economy, which stands on agriculture and fishing, and progressively on tourism. Many of these economic activities are of artisanal nature (Ordosgoitia, 2011). People of Barú Island are critically dependent on the services provided by local ecosystems. Therefore, environmental disturbances may profoundly impact the social structure of the region and threaten the subsistence of its original inhabitants. Today, Barú is under increasing pressure on tourism development, as a result of the proximity to Cartagena. Particularly, its beaches constitute a daily destination for many foreigners. Furthermore, the Island is under increasing—and largely unplanned—urban growth and real estate development. These processes are giving rise to environmental and territorial affections that are already easily perceptible (Pineda et al., 2006; Herrera Arango, 2014).
2.2. Model Building
2.2.1. Participatory Modeling Process
We employed a participatory approach (Martínez López, 2007; Montañés Serrano, 2007) for modeling the system of the tourism development of Barú Island. Our modeling procedure comprised four main steps: (i) selection of stakeholders, (ii) selection of key variables, (iii) validation of variables, and (iv) a 7-session modeling workshop. The information was originally gathered for a public socioeconomic study (TAU Consultora Ambiental–ACON, 2010) in which one of us (RJH) was in charge. Stakeholders, including decision-makers, were incorporated into the modeling process, which was carried out through the following phases and activities. First, stakeholders of the tourism development system of Barú Island were identified. This was carried out through a search of official records and interviews with qualified experts. The database included actors from different social areas; institutional actors with environmental, social, economic, and territorial competences in Barú Island; private organizations with interests on Barú Island; social organizations and NGOs. In total, this preliminary list comprised more than 60 stakeholders. From this data, we used a stakeholder analysis (Ballejos and Montagna, 2008) to select a subset of 42 actors that were finally invited to the modeling workshop. In Supplementary Table S1, we provide additional details about the selected stakeholders. In a second step, we conducted a preliminary identification of key variables as candidates for being the state variables of our qualitative model of the tourist development system of Barú Island. This was carried out through a literature review and interviews with local experts. Our identification procedure considered the demographic, environmental, economic, social, urban-territorial, political-institutional, and public policy dimensions of the tourism development in the site of study. As a result, 20 key variables were defined employing the rapid rural diagnosis methodology (Schönhuth et al., 1994; Townsley, 1996). As a third step, we conducted a validation survey among the interest groups, who had the option of either accepting the proposed variables, eliminating variables, or including new variables to the list. As a result of this phase, two variables were eliminated, and two new variables were added. The fourth step was the organization of a modeling workshop with the purpose of building the system model to be studied, on the grounds of the participatory modeling approach (Prell et al., 2007; Voinov et al., 2018). The central purposes of the workshop were, first, to review the validated list of key variables to generate the definitive set of state variables of the model and, second, to establish the links (i.e., direct causal influences, with direction and sign) among the defined state variables. Before the workshop, informative documents were provided to the stakeholders, explaining the objective of the modeling workshop, the detailed agenda, the synthesis of the characterization of the tourism development system of Barú Island, and the validated list of key variables for the model building procedure. The workshop comprised seven sessions of 1.5 h, during 2 consecutive days (November 23–24, 2009, Cartagena de Indias). In the first session we introduced the aims and goals of the model building. In the second session, we discussed the meaning, scope, and relevance of the 20 key variables formerly selected, as well as the formal requirements for being included in the structural model. At this point, three variables were deleted from the list and 10 variables were redefined to fulfill the modeling formalism. This session ended with a final list of 17 key variables that were used as the elements (state variables) of our model system. In Supplementary Table S2, we list the key variables selected at each step of our procedure. We assigned a “normative value” (−1 or 1) to each system variable. A normative value of −1/1 indicates that, to promote a sustainable tourism development, it is desirable for this variable to decrease/increase its level. During the third and fourth sessions, each of four working groups, with the help of a technical chair, elaborated a preliminary model of the system of sustainable tourism of Barú Island. For this purpose, the participants had at their disposal the informative documents previously delivered, the definitions of each of the 17 system variables, and a brief modeling guide. The core task was to define collaboratively the set of relations among system variables. These relations represented direct (and directed) causal influences between a source and a target variable. Therefore, the resulting models expressed agreements about what stakeholders consider are appropriate representations of the focal system. The fifth was a plenary session in which the four preliminary model proposals were presented and discussed. The discussion focused on the explanation and justification of the influence relations defined. In the sixth session, a new group composed of a member of each of the four original working groups developed the final model proposal, which integrated the four initial ones and considered the discussion outcomes of the previous session. In the seventh session, the final version of the model system was presented, discussed, and validated by all participants.
2.2.2. Sidigraphs
Signed directed graphs, or sidigraphs in short, represent the relations between elements of a network. Elements are displayed as vertices and relations between pairs of vertices are represented as arcs that have direction and sign. Elements are the state variables of the system, that must represent continuous quantitative variables. An arc from element vi to element vj is positive if vi exerts a direct positive influence on vj, i.e., ∂vj/∂vi > 0, negative if ∂vj/∂vi < 0 and zero if vi does not exert any direct influence on vj. Due to the possible nonlinearities of relations, we assume these derivatives are evaluated at a stable system equilibrium point (Puccia and Levins, 1985). The corresponding adjacency matrix A of a sidigraph with N vertices is an N × N matrix, in which the entry aij can contain “1” or “−1” if there is a positive or a negative arc from vertex j to vertex i. A “0” in the entry aij indicates that there is no arc from vertex j to vertex i.
2.3. Public Policy Scenarios
As a first step, we split the system variables into two groups. One group contained variables that we consider unfeasible or impractical to intervene directly. Therefore, they are not subject to management and should not be included in policy scenarios. The second group is composed of variables that can be managed with a reasonable investment and thus could be included in the intervention scenarios we defined. Variables assigned to each of the two groups are listed in Table 1 and graphically shown in Figure 1. In our setting, intervention scenarios are sustained pressures applied to the system. Specifically, they consist of varying (increasing or decreasing) steadily the level of a non-empty set of system variables.
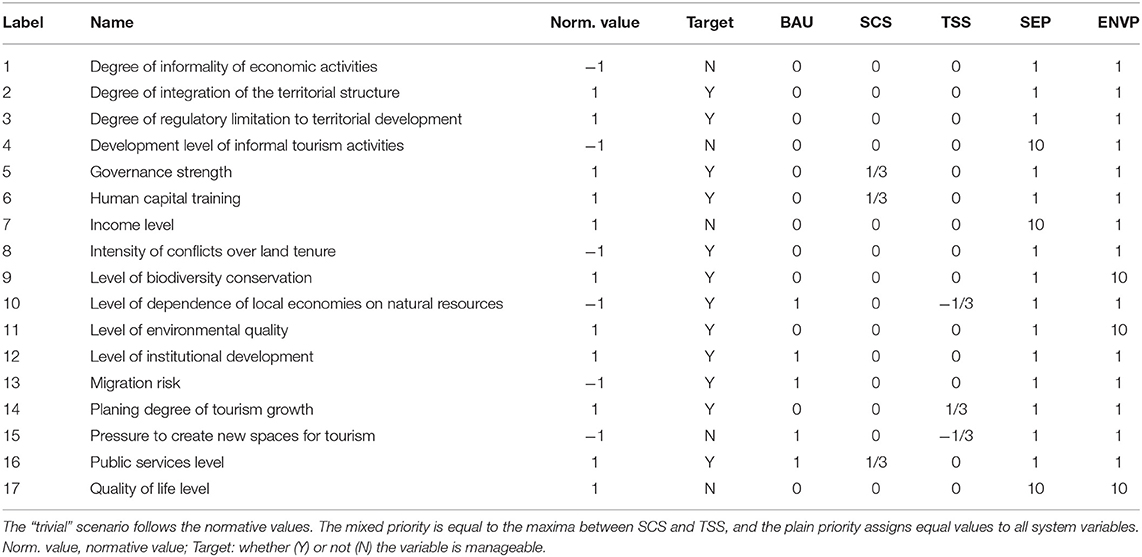
Table 1. Description of system variables, aprioristic scenarios (BAU, business–as–usual; SCS, social containment; TSS, tourism sustainability), and strategic priorities (SEP, social-economic; ENVP, environmental).
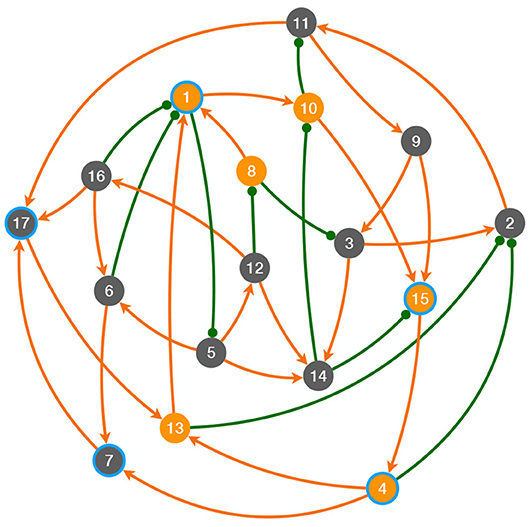
Figure 1. Sidigraph displaying the model system. The descriptions of variables are shown in Table 1. Gray/orange nodes represent system variables with positive/negative normative values. Nodes surrounded by cyan rings indicate variables that are not targets for direct intervention. Orange links ended in arrowheads represent positive influences. Green links ended in circles represent negative influences.
We designed two types of intervention scenarios as potential candidates for public policy. For the first type, we generated three “aprioristic” scenarios: the business-as-usual scenario (BAU), the social containment scenario (SCS), and the tourism sustainability scenario (TSS). They were built from previous knowledge of the tourism development in Barú Island and the main issues that threaten its progress toward a sustainable eco-tourism economy. This information was gathered during the modeling workshops mentioned above. Since for aprioristic scenarios we exerted simultaneous pressures on three system variables, we took care that SCS and TSS scenarios, following a Saaty's scale (Harker and Vargas, 1987), were roughly equivalent in implementation costs. The BAU scenario represents the major identified pressures that steadily affect the tourism system of Barú from the surrounding. This scenario can be considered as a control treatment, where no intervention takes place. Perceived BAU pressures are increasing the level of dependence of local economies on natural resources, increasing the level of institutional development, increasing the migration risk, increasing the pressures to create new spaces for tourism, and increasing the public services level. Then, we built two contrasting alternatives for public policy intervention. The SCS illustrates a reactive policy that attempts to contain the major conflicts manifested within the tourism development system, although it does not address the structural aspects that generate them. The pressures that compose this scenario are increasing governance strength, increasing human capital training, and increasing public services level. Last, the TSS illustrates a proactive public policy that attempts to drive structural improvements to the system. The pressures are decreasing level of dependence of local economies on natural resources, increasing the planning degree of tourism growth, and decreasing pressure to create new spaces for tourism.
Scenarios of the second type were “aposterioristic” ones. They constitute agnostic intervention alternatives built from the outcomes of the structural analysis of the model system. We defined these agnostic scenarios as the simulated pressures that resulted in a larger number of “successes,” relative to the number of “failures.” We define a “success” as a projected increase/decrease in the level of a variable with positive/negative normative value, after applying a pressure on the system. Conversely, a “failure” is a projected increase/decrease in the level of a variable with negative/positive normative value, after applying a pressure. We measured the goodness of a pressure—as a potential intervention measure—by the “success ratio” r, defined as
where s is the number of successes, and f the number of failures. The normalized version of this index, SR, is
with N being the number of system variables. Thus, SR assumes values between zero (only failures) and one (only successes). Here we test aposterioristic scenarios only composed of pressures on a single system variable. Therefore, they constitute cost-efficient alternatives in terms of effort and resources needed to implement them. Finally, we also included a “trivial” (and unattainable) scenario as a reference one, in which every positively valued model variable was increased, and every negatively valued model variable was decreased.
2.4. Strategic Priorities
The projected performance of each social policy scenario was assessed via assigning weights to the predicted shifts in each system variable. In particular, we defined four sets of weights that represent different strategic priorities of public policy: social-economic, environmental, mixed (i.e., social-economic and environmental), and plain (i.e., all variables having the same weight). Social-economic strategic priority (SEP) gives a weight equal to 10 to shifts in the following variables: decreasing development level of informal tourism activities, increasing income level, and increasing the quality of life level, and a weight equal to 1 to shifts in all other variables, respecting the sign of their normative value. Environmental strategic priority (ENVP) gives a weight equal to 10 to increasing the level of biodiversity conservation, increasing the level of environmental quality, and increasing the quality of life level. Shifts in other system variables have a weight of 1, respecting the sign of their normative value. Mixed strategic priority utilizes the maxima between SEP and ENVP for each system variable. All weights within each strategic priority are normalized to sum to one. Table 1 shows the definitions and normative values of system variables, together with the aprioristic scenarios and strategic priorities defined.
2.5. Model Analysis
2.5.1. Sensitivity Matrix
The change in equilibrium level of each state variable after a sustained perturbation exerted on the system is captured by the sensitivity matrix S,
where each entry of S, sij, shows the net effect of a sustained increase pj in the value of element vj on the steady-state value of element vi, i.e., (Nakajima, 1992; Dambacher et al., 2003; Novak et al., 2016). This calculation integrates all direct and indirect influences among system elements.
2.5.2. Matrix Randomization and Computer Simulations
To analyze the system responses to alternative intervention scenarios (see next section), we conducted the following steps. First, we assigned random values to the entries of matrix A. To do this, we multiplied each aij value (with i ≠ j) by a random number drawn from a uniform distribution in the range [1/2, 2]. This step allowed us to partially remove the potential dependence of system responses to specific parameter values. For the diagonal entries aii, we set a fixed negative value sufficiently small as to obtain stable matrices according to the Lyapunov criterion (i.e., the largest eigenvalue being negative). In our study we set aii = −3.5. For each random matrix , with k = 1, 2, …, m and m = 1, 000 we calculated its sensitivity matrix using Equation (1). Then, we obtain the matrix , whose entries . Then, we rescaled the entries of to fall between 0 and 1. The entries of the rescaled matrix were finally categorized to form S through
where threshold values were set as UL = 0.01 and UH = 0.7. In this way, matrix S has entries representing potential net effects between system variables, which are categorized as null (, weakly enhancing (), strongly enhancing (), weakly inhibitory (), and strongly inhibitory ().
2.5.3. Intervention Analysis
We define a public policy scenario as a set of sustained pressures exerted on a set of system variables. Each pressure can be either positive or negative and it is applied on a single variable vi with intensity Ii. Here we incorporate a public policy scenario as an additional variable in random matrices . The pressures are added as nonzero values in entries âijk of the column j that represents the scenario.
As a simple measure of the potential performance of a public policy scenario, we computed the “total benefit” projected by a given scenario. This measure is simply the sum of the successes (i.e., increases in positively valued variables and decreases in negatively valued ones) minus failures (i.e., decreases in positively valued variables and increases in negatively valued ones), weighted by the values assigned to the potential shifts in state variables, defined in a “strategic priority.”
in which column j of S contains the pressures that define the public policy being tested, is the normative value (1 or −1) of variable vi, and is the value (1 or 10) of changing the equilibrium value of vi in the direction of its normative value, defined in the strategic priority.
3. Results
The obtained model for the system of tourism development in Barú Island is displayed as a sidigraph in Figure 1. The model system has 17 variables and 34 directed links (i.e., arcs) between variables. Relations are composed of 24 positive arcs and 10 negative arcs. System connectance, that is the fraction of all possible links that are realized, is 0.125. System connectance C is calculated as C = L/[N(N−1)], where L in the number of links in the system and N is the number of system variables. The maximum of possible unidirectional links in the system is N(N−1), which does not consider self-links. The sidigraph is strongly connected, that is, every vertex is reachable from every other vertex, respecting the direction of the system links.
The sensitivity of system variables to a sustained increase in every single variable is shown in Figure 2. Through reversing the sign of the external pressure, we obtain projections with opposite sign. The largest normalized success ratio SR after applying pressures on single system variables were obtained by decreasing variable 13 (SR = 1), decreasing variable 1 (SR = 0.94), and decreasing variable 8 (SR = 0.89). Next, increases in variables 5 and 12 produced an SR value of 0.47, while increasing variable 6 gives SR = 0.44. These single-variable pressures, which produced the higher SR values, were set as our aposterioristic scenarios, with the exception of increasing variable 1, since this variable was defined as non-target (see Table 1).
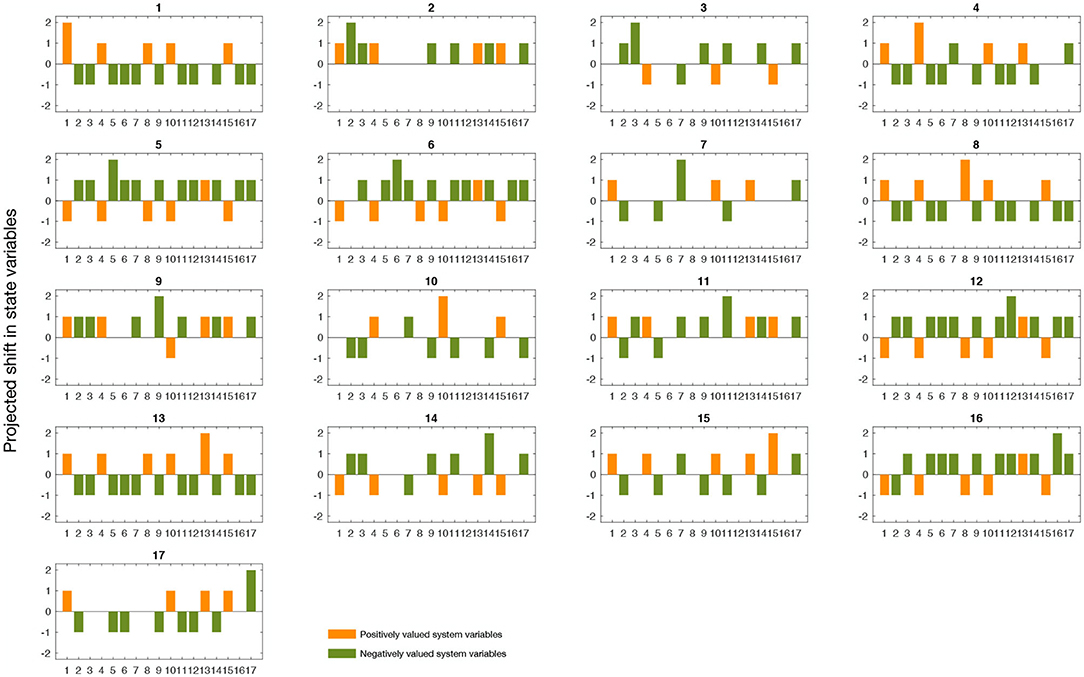
Figure 2. Projected responses of state variables to sustained pressures on each single variable, which is numbered at the top of each plot. For example, the top left plot shows the projected categorical shift (1: weak, 2: strong) in the equilibrium level of each variable (1–17 on x-axis) after externally increasing the level of variable 1. Green/orange bars represent variables with positive/negative normative values.
In Figure 3, we show the projected outcomes of the competing public policy scenario, both aprioristic and aposterioristic ones, considering the four alternative strategic priorities. An analysis of Figure 3 reveals that the total benefit derived from intervening in the system differs among public policy scenarios. Besides, the magnitude of the differences among scenarios depend on the adopted strategic priority. In all cases, the BAU scenario was noticeably the worst, leading to the smallest total benefit (between −0.14 and 0.27). Under the environmental strategic priority, the total benefit from BAU (−0.14) was markedly lower than the rest of the scenarios (≥0.91). However, under the socio-economic scenario the difference in total benefit between BAU (0.27) the other scenarios (≥0.5) was smaller. At low intervention intensity, the trivial and the +5 scenario (i.e., increasing variable 5) rendered the highest total benefit (around 1.0), followed by +12 and SCS (≥0.8). Most curves approach an asymptote at intervention intensities between 3 and 4. At higher intervention intensities, the aposterioristic scenario −13 (decreasing variable 13) led to the highest total benefit (≥1.02), followed by the unattainable trivial scenario (1.0) and then by scenario +5 (between 0.98 and 1.0). The rest of scenarios, ordered from the highest to the lowest total benefit rendered, were SCS, +12, −8, +6, TSS, and BAU. Not considering BAU, the differences in total benefit among the tested scenarios were wider under the socio-economic priority (standard deviation of asymptotic values = 0.18) and narrower under the environmental priority (standard deviation = 0.04). Finally, when scenarios −13 and +5 are combined into a single one by pressuring the implied system variables simultaneously, the obtained total benefit is the highest at every level of intervention intensity (see Supplementary Figure S1).
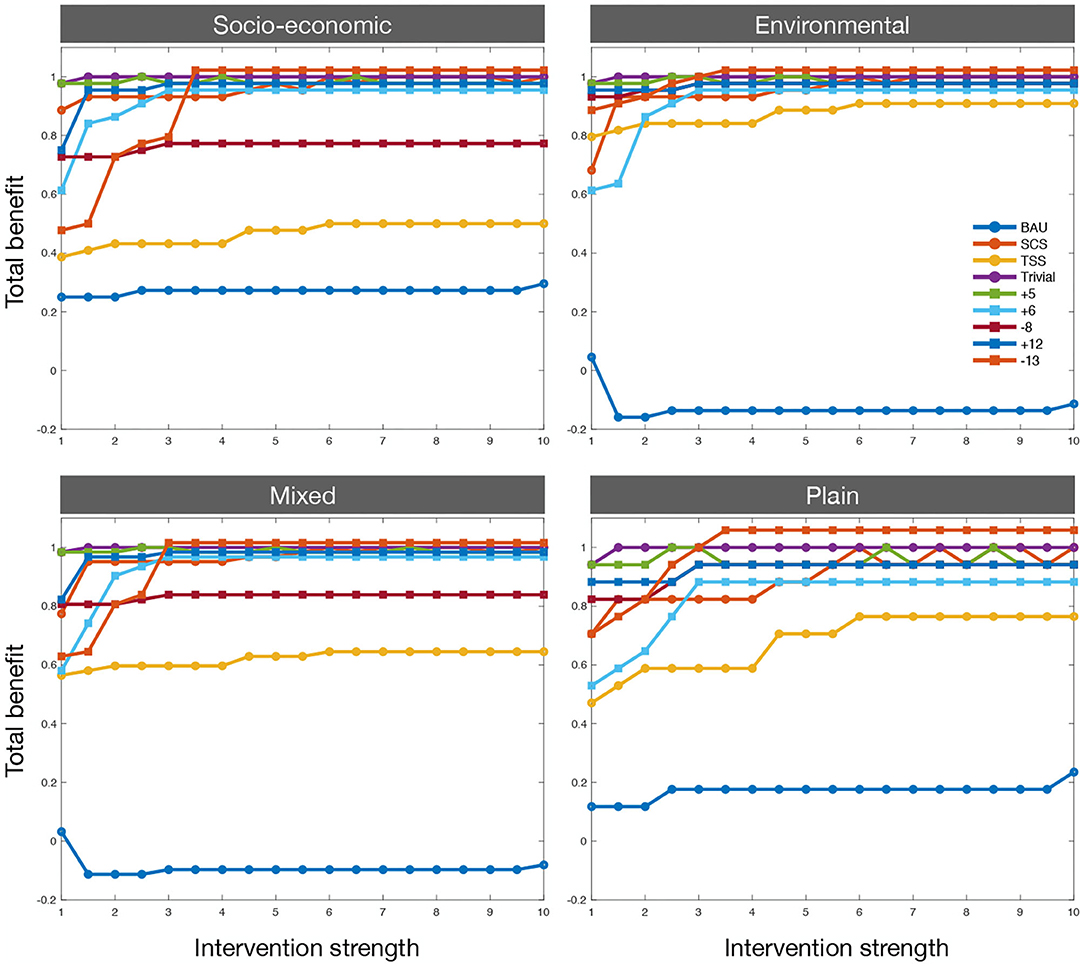
Figure 3. Total benefit projected for the tested public policy scenarios, at various levels of intervention strength. Circles show aprioristic scenarios: BAU, SCS, TSS, trivial. Squares show aposterioristic scenarios: increasing (+) or decreasing (−) variables 5, 6, 8, 12, and 13. Each plot shows the results for a defined strategic priority: socio–economic, environmental, mixed, and plain.
4. Discussion
Our results suggest that an active public policy is needed for the sustainable development of an ecotourism-based economy in Barú Island. The business-as-usual scenario, which synthesizes the major pressures that are taking place in the study site, resulted to be the worst case among the analyzed scenarios, which was most noticeable under the environmental strategic priority. This finding underlines the need for proper intervention actions whenever the ecological dimension plays an important role for political decisions on tourism development.
In our study, tested aprioristic policies were composed of a set of sustained pressures applied simultaneously on several system variables. These policy scenarios emerged as a natural outcome of stakeholder experiences, and guided by common sense. The performance of the social containment scenario (SCS) was higher than the tourism sustainability one (TSS). However, the differences in total benefit projected from these policies were the widest under the socio-economic strategic priority, the narrowest under the environmental priority, and intermediate under the mixed and plain priorities. Aposterioristic policy scenarios were build as simpler policy scenarios in which a single sustained pressure is exerted on a unique system variable. They were chosen based on the sensitivity matrix of the model sidigraph, as those single pressures that maximize projected successes; that is, increases of positively valued variables and decreases of negatively valued ones, without considering any definition on strategic priority. At any given value of intervention strength, there was at least one aposterioristic policy scenario that outcompeted aprioristic ones. For intervention strengths over 4, the univariate scenario −13, that is, reduction of migration risk, was the best scenario under the four tested strategic priorities. This policy scenario performed even better than the trivial and unattainable scenario in which all variables are pressured simultaneously according to their normative value. On the other hand, at low intervention strength (roughly below 4), the best policy scenario was increasing governance strength (+5). Although the total benefit projected from this scenario was slightly below that of the trivial policy, it was higher than any aprioristic one. The simultaneous pressure on variables 13 and 5 showed an additive effect and gave the maximum total benefit at every intervention strength, outcompeting all other tested policy alternatives.
Our results suggest that, for our study case, an effective intervention strategy should be based on strengthening local governance and alleviating migration risk. Strengthening local governance could imply increasing the presence and activity of the local municipal administration on the island, and generating new instances of local management, particularly those responsible for sustainable tourism development. Besides, this line of intervention policy should include strengthening social organizations and increasing their participation in institutional decision-making instances, in agreement with current inclusive models of local governance (Welch et al., 2004). Furthermore, a successful strategy should be based on proactive local migration management, which could produce important benefits for the local economy (Solimano, 2008; Migración Colombia, 2017). In this vein, institutions and society should prepare for effective social integration of the migrant population. This can be fostered by the identification of productive vocations, business opportunities, and local human capital needs. Such measures would promote novel productive developments that could arise from the entry of talents associated with migration. These actions should be enforced by the implementation of effective mechanisms for managing the negative residual effects related to security, and by migration control procedures based on strict respect for human rights.
Besides identifying a small set of candidate variables for, through their intervention, promoting sustainable tourism in the study site, our study serves as a template for implementing a recursive procedure of modeling, analysis, policy proposal, evaluation, back to modeling, and so forth. Our structural approach is appropriate for supporting strategic decisions, considering tourism development as a system (Mai and Smith, 2015; Roxas et al., 2020), instead a mere collection of services. The design and monitoring of a sustainable strategic policy for tourism development are especially important for the study site and for the whole region of South America. From the assessment of the state of biodiversity in Latin America and the Caribbean, the UN Environment Programme World Conservation Monitoring Centre explicitly advocates linking tourism to development planning in coastal nations (UNEP-WCMC, 2016). This region has some of the most diverse ecosystems in the world. However, they are seriously threatened by the inorganic impulses of the countries toward their economic development. According to the National Biodiversity Index (NBI) adopted by the Convention of Biological Diversity (Convention of Biological Diversity, 2001), Colombia exhibits the highest levels of biodiversity (corrected by surface) in South America, with an NBI of 0.935, only outstripped globally by Indonesia (NBI = 1.0). In South America, NBI of Colombia is followed by those of Brazil (NBI = 0.877), Ecuador (NBI = 0.873), Venezuela (NBI = 0.850), and Perú (NBI = 0.843). The rest of the countries are below the regional mean of 0.722. Nevertheless, South America exhibited the highest rate of net forest loss in the world during the past decades. Between 1990 and 2020, this region lost 4.3 million ha/year on average, followed by Africa with 3.5 million ha/year (FAO, 2020). Furthermore, only 17% of forests have long-term management plans in South America, compared to 96% in Europe. To foster biodiversity conservation in the Colombian Caribbean, Aldana-Doḿınguez et al. (2017) stress the need to develop, in the middle and the long term, an integrated territorial planning and to promote the social-ecological systems perspective, highlighting the still limited contribution of social sciences and interdisciplinary studies to the biodiversity conservation knowledge.
Concerning the socio-ecological dimension of our study, the sustainable development of the tourism system on Barú Island faces great challenges that may become critical in the near future. These challenges arise mainly from the interplay between climate change, with uncertain effects on Caribbean ecosystems, the risk of over-tourism (Peterson, 2020), derived from the ongoing increase in visitors to the region, and the financial and political decisions made by the governments. These decisions determine the strategic priorities and the public policy adopted for reconciling socio-economic development and the care of local ecosystems. Ecotourism, as a sustainable economic activity, depend on availability of infrastructure and trained staff in varied sectors, such as accommodation, transportation, food, and entertainment. However, the basis and distinction of ecotourism lies in the quality of services provided by local ecosystems (Phelan et al., 2020). The so-called cultural ecosystem services (Milcu et al., 2013) were defined as “the nonmaterial benefits people obtain from ecosystems through spiritual enrichment, cognitive development, reflection, recreation, and aesthetic experiences” (Reid et al., 2005). Therefore, safeguarding the future of ecotourism is intrinsically linked to the preservation of ecosystem services and the biodiversity that generates them (Nahuelhual et al., 2014).
From a methodological point of view, we conclude that aposterioristic scenarios derived from the sensitivity matrix of a qualitative model can generate good and cost-effective plan proposals for policy formulation. In particular, structural qualitative modeling may give analytical support to the selection of intervention measures from a structural perspective, based on defined strategic priorities. We do not intend to give an all-in-one solution for adopting complex strategic decisions. Our study constitutes a partial guidance on how to approach a socio-ecological goal from a systemic perspective. However, in this study we only considered a set of local variables related to tourism development. There are other relevant socio-ecological aspects of this industry, such as the global consumption of natural resources, carbon emissions, natural habitat loss, among others (Chakraborty, 2020). Tourism system researchers should include these variables when constructing models of tourism development planned on broader geographical and temporal scales.
There are also two limitations of our methodological approach that are important to consider. First, there is an inherent difficulty of building reliable models of socio-ecological systems. Second, our analysis of the model, based on the sensitivity matrix, is strictly valid only in the neighborhood of a stable equilibrium of the system. The first caveat rests in that there is a wide variety of potential variables composing social-ecological systems, from which it is difficult to select a subset that represents the essence of the system for the planned purposes. Besides, the causal links between system variables are also difficult to determine, since often all variables are related, but there is no unambiguous procedure to discern between direct (those that define the model) and indirect (those that emerge) causal relations. Thus, we rested on participatory procedures to build a consensus model which posses credibility for stakeholders. This choice also implies to recognize and consider that other models could also be valid and, more important, that the model should be revised over time as new information becomes available. The second problem is related to the equilibrium assumption. The calculation of the sensitivity matrix rests on assuming that before and some time after applying the intervention, the system is at a stable equilibrium point. However, there is evidence that for natural systems that hardly present a strict stable equilibrium, this methodology passes empirical testing (Dambacher and Ramos-Jiliberto, 2007). Recent advances in the theory of non-autonomous dynamical systems (Kloeden and Pötzsche, 2013), present “entire bounded solutions” as a generalization for fixed-point equilibria, which appears as a promising avenue to extend the structural qualitative analysis of social-ecological systems beyond the limitations of local perturbations. In a broader perspective, systems may exhibit noticeable changes on a short timescale, alternating with long periods of either stasis or slow gradual changes. This is consistent with the emerging quasi-stationarity perspective in ecology (Coulson, 2020), potentially applicable to socio-ecological systems. In fact, we observe abrupt shifts in a wide variety of ecosystems (van den Elsen et al., 2020). However, and perhaps more interesting, is that natural and social catastrophes are often closely linked (Nel and Righarts, 2008), with natural disasters triggering social ones, including wars (Lee, 2018) and revolutions (Manning et al., 2017). Under the quasi-stationarity view, the equilibrium assumption is valid for the static periods, with one model characterizing each period. However, during the phases of abrupt shifts, the equilibrium assumption seems to be no longer valid. In this vein, a great advance would be to develop a theory that allows describing a sequence of structural qualitative systems, their rules of transformation, and tools for describing and analyzing their transitional dynamics. In the meantime, we have to deal with the equilibrium assumption and consider our models as contingent representations of the study system, that need to be updated over time.
Data Availability Statement
The original contributions presented in the study are included in the article/Supplementary Material, further inquiries can be directed to the corresponding author/s.
Author Contributions
RR-J and RJH conceived the study, made revisions to the draft, and approved the final version. RJH conducted participatory modeling procedures. RR-J analyzed the model system. RR-J wrote the first draft of the paper. All authors contributed to the article and approved the submitted version.
Funding
This work was partially supported by the Chilean National Agency for Research and Development (ANID), through projects FONDECYT 1190173 and Fondef ID20I10147.
Conflict of Interest
The authors declare that the research was conducted in the absence of any commercial or financial relationships that could be construed as a potential conflict of interest.
Acknowledgments
We thank the professional staff of the TAU and ACON organizations and the public officials of the state of Colombia who collaborated in the early participatory modeling process.
Supplementary Material
The Supplementary Material for this article can be found online at: https://www.frontiersin.org/articles/10.3389/fevo.2021.632067/full#supplementary-material
References
Aldana-Domínguez, J., Montes, C., Martínez, M., Medina, N., Hahn, J., and Duque, M. (2017). Biodiversity and ecosystem services knowledge in the colombian caribbean: progress and challenges. Trop. Conserv. Sci. 10:1940082917714229. doi: 10.1177/1940082917714229
Andrade, M. G. (2011). Estado del conocimiento de la biodiversidad en colombia y sus amenazas. consideraciones para fortalecer la interacción ciencia-política. Rev. Acad. Colomb. Ciencias Exact. Físicas y Nat. 35, 491-508.
Anup, K. (2016). “Ecotourism and its role in sustainable development of Nepal,” in INTECH Open Science, Rijeka, 31–59.
Ballejos, L. C., and Montagna, J. M. (2008). Method for stakeholder identification in interorganizational environments. Requir. Eng. 13, 281–297. doi: 10.1007/s00766-008-0069-1
Barrios, L. (2000). Evaluación de las Principales Condiciones de Deterioro de los Corales pétreos en el Caribe Colombiano. Santa Marta: Biol. Mar. Univ. Nal. de Colombia.
Chakraborty, A. (2020). Can tourism contribute to environmentally sustainable development? Arguments from an ecological limits perspective. Environ. Dev. Sustain. 1–17. doi: 10.1007/s10668-020-00987-5
Convention of Biological Diversity (2001). Global Biodiversity Outlook 1. Annex 1. Convention of Biological Diversity.
Coulson, T. (2020). We live in a changing world, but that shouldn't mean we abandon the concept of equilibrium. Ecol. Lett. 24, 3?-5. doi: 10.1111/ele.13629
Dambacher, J. M., Li, H. W., and Rossignol, P. A. (2003). Qualitative predictions in model ecosystems. Ecol. Model. 161, 79–93. doi: 10.1016/S0304-3800(02)00295-8
Dambacher, J. M., and Ramos-Jiliberto, R. (2007). Understanding and predicting effects of modified interactions through a qualitative analysis of community structure. Q. Rev. Biol. 82, 227–250. doi: 10.1086/519966
Díaz, J. M., Barrios, L. M., and Gómez-López, D. I. (2003). Las Praderas de Pastos Marinos en Colombia: Estructura y Distribución de un Ecosistema Estratégico. INVEMAR, Serie Publicaciones Especiales, 160.
Hage, P., and Harary, F. (1983). Structural Models in Anthropology: Cambridge Studies in Social Anthropology. Cambridge: Cambridge University Press.
Hall, C. M. (2019). Constructing sustainable tourism development: the 2030 agenda and the managerial ecology of sustainable tourism. J. Sustain. Tour. 27, 1044–1060. doi: 10.1080/09669582.2018.1560456
Harker, P. T., and Vargas, L. G. (1987). The theory of ratio scale estimation: Saaty's analytic hierarchy process. Manage. Sci. 33, 1383–1403. doi: 10.1287/mnsc.33.11.1383
Herrera Arango, J. (2014). Del turismo caribe no y otras disputas territoriales. Observ. Territ. Etnic. Campes. 2.
Higgins-Desbiolles, F. (2018). Sustainable tourism: sustaining tourism or something more? Tour. Manage. Perspect. 25, 157–160. doi: 10.1016/j.tmp.2017.11.017
Kloeden, P. E., and Pötzsche, C. (2013). Nonautonomous dynamical systems in the life sciences. Cham: Springer.
Lee, H. F. (2018). Internal wars in history: triggered by natural disasters or socio-ecological catastrophes? Holocene 28, 1071–1081. doi: 10.1177/0959683618761549
Mai, T., and Smith, C. (2015). Addressing the threats to tourism sustainability using systems thinking: a case study of cat BA Island, Vietnam. J. Sustain. Tour. 23, 1504–1528. doi: 10.1080/09669582.2015.1045514
Manning, J. G., Ludlow, F., Stine, A. R., Boos, W. R., Sigl, M., and Marlon, J. R. (2017). Volcanic suppression of Nile summer flooding triggers revolt and constrains interstate conflict in ancient Egypt. Nat. Commun. 8, 1–9. doi: 10.1038/s41467-017-00957-y
Martínez López, M. (2007). Complejidad y participación: la senda de la invención estratégica. Polít. Soc. 44, 31–53.
MCIT, C. (2019). Ministry of Commerce, Industry and Tourism, Colombia. Resultados para el turismo para el año 2018, MCIT.
Migración Colombia (2017). Oportunidades de la Migración Internacional en un Contexto de Paz en Colombia. Organización Internacional para las Migraciones (OIM-Misión Colombia).
Milcu, A. I., Hanspach, J., Abson, D., and Fischer, J. (2013). Cultural ecosystem services: a literature review and prospects for future research. Ecol. Soc. 18:44. doi: 10.5751/ES-05790-180344
Montañés Serrano, M. (2007). Más allá del debate cuantitativo/cualitativo: la necesidad de aplicar metodologías participativas conversacionales. Polít. Soc. 44, 13–29.
Nahuelhual, L., Carmona, A., Aguayo, M., and Echeverria, C. (2014). Land use change and ecosystem services provision: a case study of recreation and ecotourism opportunities in southern Chile. Landsc. Ecol. 29, 329–344. doi: 10.1007/s10980-013-9958-x
Nakajima, H. (1992). Sensitivity and stability of flow networks. Ecol. Model. 62, 123–133. doi: 10.1016/0304-3800(92)90085-S
Nel, P., and Righarts, M. (2008). Natural disasters and the risk of violent civil conflict. Int. Stud. Q. 52, 159–185. doi: 10.1111/j.1468-2478.2007.00495.x
Novak, M., Yeakel, J. D., Noble, A. E., Doak, D. F., Emmerson, M., Estes, J. A., et al. (2016). Characterizing species interactions to understand press perturbations: what is the community matrix? Annu. Rev. Ecol. Evol. Syst. 47, 409–432. doi: 10.1146/annurev-ecolsys-032416-010215
Ordosgoitia, Y. (2011). “Procesos históricos de las comunidades asentadas en el archipiélago del rosario, isla barú, isla de tierra bomba y archipiélago de san bernardo,” in El entorno ambiental del Parque Nacional Natural Corales del Rosario y San Bernardo (PNNCRSB). Primera edición: Colombia: Parques Nacionales Naturales de Colombia, E. Zarza-González (Cartagena de Indias: Ministerio de Ambiente), 13–25.
Peterson, R. R. (2020). Over the Caribbean top: community well-being and over-tourism in small island tourism economies. Int. J. Commun. Well-Being 1–38. doi: 10.1007/s42413-020-00094-3
Phelan, A., Ruhanen, L., and Mair, J. (2020). Ecosystem services approach for community-based ecotourism: towards an equitable and sustainable blue economy. J. Sustain. Tour. 28, 1665–1685. doi: 10.1080/09669582.2020.1747475
Pineda, I., Martínez, L., Bedoya, D., Caparroso, P., and Rojas, J. (2006). Plan de Manejo del Parque Nacional Natural Corales del Rosario y San Bernardo. UAESPNN, Territorial Costa Caribe, Cartagena.
Prell, C., Hubacek, K., Reed, M., Quinn, C., Jin, N., Holden, J., et al. (2007). If you have a hammer everything looks like a nail: traditional versus participatory model building. Interdisc. Sci. Rev. 32, 263–282. doi: 10.1179/030801807X211720
Puccia, C. J., and Levins, R. (1985). Qualitative Modeling of Complex Systems. An Introduction to Loop Analysis and Time Averaging. Cambridge, MA: Harvard University Press. doi: 10.4159/harvard.9780674435070
Reid, W. V., Mooney, H. A., Cropper, A., Capistrano, D., Carpenter, S. R., Chopra, K., et al. (2005). Ecosystems and Human Well-Being-Synthesis: A Report of the Millennium Ecosystem Assessment. Washington, DC: Island Press.
Roxas, F. M. Y., Rivera, J. P. R., and Gutierrez, E. L. M. (2020). Framework for creating sustainable tourism using systems thinking. Curr. Issues Tour. 23, 280–296. doi: 10.1080/13683500.2018.1534805
Schönhuth, M., and Kievelitz, U. (1994). Participatory Learning Approaches: Rapid Rural Appraisal, Participatory Appraisal. An Introductory Guide. Rossdorf: TZ-Verlagsgesellschaft.
Solimano, A. (2008). International Migration, Risk Management and Social Policy: the Latin America Case. Geneva: United Nations Research Institute for Social Development.
TAU Consultora Ambiental-ACON (2010). Evaluación Ambiental Estratégica de Políticas, Planes y Programas de Turismo en la Zona de Barú, Distrito Turístico de Cartagena de Indias. Informe Final de Consultoría. Bogotá: Ministerio de Ministerio de Ambiente, Vivienda y Desarrollo Territorial, República de Colombia.
Townsley, P. (1996). Rapid Rural Appraisal, Participatory Rural Appraisal and Aquaculture. Food & Agriculture Org.
UNEP-WCMC (2016). The State of Biodiversity in Latin America and the Caribbean: A Mid-Term Review of Progress Towards the Aichi Biodiversity Targets. UNEP-WCMC.
UNWTO (2019). World Tourism Organization. International Tourism Highlights, 2019 Edition. Madrid: UNWTO. doi: 10.18111/9789284421152
van den Elsen, E., Stringer, L. C., De Ita, C., Hessel, R., Kéfi, S., Schneider, F. D., et al. (2020). Advances in understanding and managing catastrophic ecosystem shifts in mediterranean ecosystems. Front. Ecol. Evol. 8:323. doi: 10.3389/fevo.2020.561101
Voinov, A., Jenni, K., Gray, S., Kolagani, N., Glynn, P. D., Bommel, P., et al. (2018). Tools and methods in participatory modeling: selecting the right tool for the job. Environ. Model. Softw. 109, 232–255. doi: 10.1016/j.envsoft.2018.08.028
Keywords: signed directed graphs, community matrix, jacobian matrix, loop analysis, ecological models
Citation: Ramos-Jiliberto R and Jiliberto Herrera R (2021) Evaluating Social Policy Scenarios for Tourism Development of Barú Island (Colombia) Using Structural Qualitative Modeling. Front. Ecol. Evol. 9:632067. doi: 10.3389/fevo.2021.632067
Received: 22 November 2020; Accepted: 30 March 2021;
Published: 29 April 2021.
Edited by:
Jeffrey Mark Dambacher, CSIRO, AustraliaReviewed by:
Marcello Vitale, Sapienza University of Rome, ItalyGwendolyn Peyre, University of Los Andes, Colombia
Copyright © 2021 Ramos-Jiliberto and Jiliberto Herrera. This is an open-access article distributed under the terms of the Creative Commons Attribution License (CC BY). The use, distribution or reproduction in other forums is permitted, provided the original author(s) and the copyright owner(s) are credited and that the original publication in this journal is cited, in accordance with accepted academic practice. No use, distribution or reproduction is permitted which does not comply with these terms.
*Correspondence: Rodrigo Ramos-Jiliberto, cm9kcmlnby5yYW1vc0B1bWF5b3IuY2w=