- 1Department of Botany and Biodiversity Research, University of Vienna, Vienna, Austria
- 2Institute for Ecology, Evolution and Diversity, Goethe University of Frankfurt, Frankfurt, Germany
Land use change has led to large-scale insect decline, threatening ecosystem resilience through reduced functional diversity. Even in nature reserves, losses in insect diversity have been detected. Hereby, changes in local habitat quality and landscape-scale habitat quantity can play a role driving functional diversity toward erosion. Our aim was to analyze how local and landscape-scale factors simultaneously affect functional insect diversity. Therefore, we sampled moths in two Italian coastal forest reserves at 60 sites. Our focus was on functional richness, redundancy and niche occupation, being important for ecosystem resilience, following the insurance framework. Ecological information about 387 species and 14 traits was used to analyze functional diversity. Twenty-five functional groups were recognized and used to estimate niche occupation and redundancy. Fourteen local and 12 landscape-scale factors were measured and condensed by using Principal Components Analysis. The resulting PC-axes served as predictors in linear mixed effects models. Functional richness, redundancy and niche occupation of moths were lower at sites with low habitat quality and quantity, indicating reduced ecosystem resilience. Especially landscape diversity and habitat structure, viz. a humidity-nutrient gradient, but also plant diversity, were promoting functional richness. Landscape fragmentation, indicating increased impermeability for insects, reduced local functional richness, redundancy and niche occupation. Local habitat quality and landscape-wide habitat quantity are both important for maintaining functional insect diversity inside reserves. Therefore, small and isolated nature reserves might fail in preserving biodiversity and ecosystem functions through adverse effects acting from the surrounding landscape structure and configuration.
Introduction
Changing environmental conditions due to human activities can be ever more challenging for the communities inhabiting the few remaining near-natural areas. Land use change, especially intensified management practices and habitat fragmentation, in fact are currently the most important drivers of biodiversity decline (Newbold et al., 2015). However, maintaining species-rich communities is crucial for ecosystem functioning as biodiversity enhances ecosystem multifunctionality (Diaz et al., 2007; Allan et al., 2015), is linked to ecosystem productivity (Duffy et al., 2017) and to ecosystem resilience (Mori et al., 2013; Oliver et al., 2015). Yachi and Loreau (1999) theoretically investigated the relationship between species richness and ecosystem function by formulating the ‘insurance hypothesis’. This hypothesis was repeatedly supported (Oliver et al., 2015; Isbell et al., 2018), indicating the importance of species richness and functional redundancy for the resilience of ecosystem function. Basically, functional redundancy ensures that even if some species vanish because of disturbance or stochastic effects, other ones might occupy sufficiently similar ecological niches and so maintain the function of the whole ecosystem. As species richness alone cannot inform about which functional niches are occupied by how many species (Lewis et al., 2014), measures of functional diversity have become an important tool for community ecology research (Mason and De Bello, 2013; Gagic et al., 2015). Different functional aspects of species here can give important insight into how species react to disturbance (response traits), and how they affect ecosystem processes (effect traits). This response-effect trait framework has been described by Suding et al. (2008) and was, similar to other functional diversity approaches, first introduced in plant community research (Diaz et al., 2007; Allan et al., 2015).
However recently, functional diversity has also become more common in insect studies (Greenop et al., 2018; Woodcock et al., 2019; Guariento et al., 2020). Insects as a very species-rich group occupy multiple important niches that guarantee ecosystem functions and services, such as pollination, decomposition, herbivory and predation, as well as food supply for higher trophic levels (Greenop et al., 2018; Woodcock et al., 2019; Beck and McCain, 2020). At the same time, insect decline over the last decades can be observed at multiple scales (Habel et al., 2019a, b; Seibold et al., 2019) and has become a topic of public interest (Leather, 2018; Saunders, 2019). The drastic decline in insect abundances and diversity is an alarming sign of overexploiting our natural environment at the cost of biodiversity and ecosystem function (Woodcock et al., 2014, 2019). Insect diversity decreases due to intensive local management (Chisté et al., 2016; Mangels et al., 2017) and large-scale landscape simplification (Merckx et al., 2012b; Bates et al., 2014; Gámez-Virués et al., 2015), and is accompanied by reduced species turnover across landscapes (Merckx and Van Dyck, 2019). This finally leads to decreasing landscape-wide gamma diversity and an all-over homogenized species pool mainly consisting of generalists (Clavel et al., 2011; Seibold et al., 2019; Piano et al., 2020). So, when dividing land use factors into different spatial scales of effect, insect decline seems to be driven by loss in local habitat quality (e.g., driven by management intensification) as well as landscape-wide habitat quantity (e.g., landscape simplification).
Habitat quantity describes the amount of habitable area available for subsets of species in a certain range of effect. The definition of a ‘habitat’ always depends on the focal species and its demands on the environment. For example, the amount of forested area in a 1,000 m range around a focal site might influence the number of forest species to be found there (Fahrig, 2013). Taking this paradigm to the next spatial level, higher landscape-level habitat diversity might positively affect taxonomic and functional diversity of local assemblages. With more different habitat types around a focal site, more functional niches are available which sustain a larger regional species pool (Woodcock et al., 2014; Merckx et al., 2019). This determines how many species on the local level, as a subset of the regional pool, can be found (Tscharntke et al., 2012). Further proxies of habitat quantity might be the total amount of semi-natural habitats in the vicinity of the focal site, or – in reverse – how much area around has been modified by land use (e.g., agriculture or buildings), and might therefore no longer be habitable to most biota.
Similar to habitat quantity, also the definition of quality always depends on the requirements of the target organisms. Therefore, quality is nearly impossible to define for a whole community consisting of different species with different needs (Dennis et al., 2014). Nevertheless, for terrestrial insects – especially pollinators and herbivores, which have a long history of co-evolution with plants (Macior, 1971) – a high quality site might be generally marked by high plant species richness and high structural complexity of its vegetation (Chisté et al., 2016; Alison et al., 2017; Knuff et al., 2020). Looking for example at forest habitats, a near-natural forest structure with unevenly aged trees, a substantial understory layer, and a certain amount of deadwood might for many species be of higher quality than evenly aged, dense, young forest stands which are planted for a maximum gain in timber production (Thorn et al., 2015).
As insect decline is not only known from agricultural areas with intensive management (Fiedler et al., 2017; Mangels et al., 2017), but also in conservation areas (Seibold et al., 2019), the focus of our study was to simultaneously unravel the roles of small-scaled differences in local habitat quality and landscape-wide habitat quantity for functional moth diversity inside nature reserves. We here define how these two scales might be circumscribed, and correlate them to the functional diversity of moths as a species-rich insect group, which often has been used as indicators of ecological integrity of biota (Merckx et al., 2012b; Uhl et al., 2016). We emphasize that our focus here lies on small-scaled functional diversity patterns within nature reserves, rather than on overall changes in gamma diversity between different areas under study embedded in a larger landscape unit. Detecting these small-scale changes along habitat quality and quantity gradients within conservation areas might be crucial for ameliorating conservation management practices, aiming to preserve intact and species-rich ecosystems.
For analyzing functional diversity, different indices have been developed. Functional diversity measures can be separated into three main categories: Functional richness (hereafter FRic), evenness (hereafter FEve) and divergence (hereafter FDiv; Mouchet et al., 2010). All three facets of functional diversity have to be considered as no single index of functional diversity gives full insight into all aspects (Mouchet et al., 2010). However, as the focus of our study lies on the importance of functional richness for ecosystems, mainly measures related to this aspect of functional diversity will be considered. As FRic is sensitive to outliers (Laliberté and Legendre, 2010) and only takes into account the most extreme trait values (Mouchet et al., 2010), we further explore how species in local communities are distributed across the available trait space by investigating trait space occupation and functional redundancy. Results for FEve, FDiv and functional dispersion (hereafter FDis) will also be presented, but will not be discussed in detail.
The main research question of our study was: How far are small-scaled moth functional diversity patterns influenced by local habitat quality or rather by landscape-scale habitat quantity? For plant communities, FRic and functional redundancy have been shown to depend on local management (Laliberté et al., 2010; Niu et al., 2016) and also landscape-scale parameters (Bruno et al., 2016; Feit et al., 2019). However, the effect of local habitat quality and landscape wide quantity has to date not been assessed for insect communities inside conservation areas, especially when it comes to multivariate analyses. In particular, we want to address the following hypotheses:
• Both local habitat quality and landscape-scale quantity determine FRic and functional redundancy of local insect communities.
• Niche occupancy is related to landscape diversity and the structural complexity of the habitats, as with more heterogeneity, more niche options are available.
Methods
Study Areas
Our study sites were situated in two isolated forest reserves in north-eastern Italy, near the city of Ravenna. Both reserves – Pineta san Vitale (hereafter PsV) and Pineta di Classe (hereafter PdC) – are today of high legal conservation concern, as they are part of the regional park Po Delta, listed as UNESCO biosphere reserves, are protected as Natura 2,000 sites and as important bird area.
The two forests – which were connected until the 18th century – were planted on paleodunes and used for pine nut harvest, cattle grazing and wood production (Andreatta, 2010). With the abandonment of these extensive management practices the pinewoods developed, due to natural succession, toward a semi-natural forest structure (Wölfling et al., 2019). In parallel, agricultural intensification and urbanization in the surroundings lead to ongoing fragmentation and isolation, such that of the initial 6,000 ha forest area less than 2,000 ha, split up between PsV (950 ha) and PdC (900ha), remain to the present (Malfitano, 2002; Andreatta, 2010). Today, the main habitat type inside the reserves is a mix of oak and pine forest, but also other vegetation types like riparian forests, reed areas and open grasslands can be found (Uhl et al., 2020a).
The areas around the two reserves are dominated by intensive agriculture (in the case of PdC), the industrial harbor of Ravenna to the south of PsV, and some other protected natural areas in the north of PsV. These different landscape surroundings as well as small-scaled variation in the local vegetation form the basis of our present investigations on correlations between local functional diversity of insects, habitat quality and quantity.
Data Collection
We chose 60 sites (30 in each reserve) equally distributed throughout the study area (Figure 1). Distances between sites were rather low with about 500m between neighboring light trap sites. All sites were situated in mixed forest and were accessible by one of the numerous forest paths. Sampling took place from 2015 to 2017, with 20 randomly chosen sites visited per year.
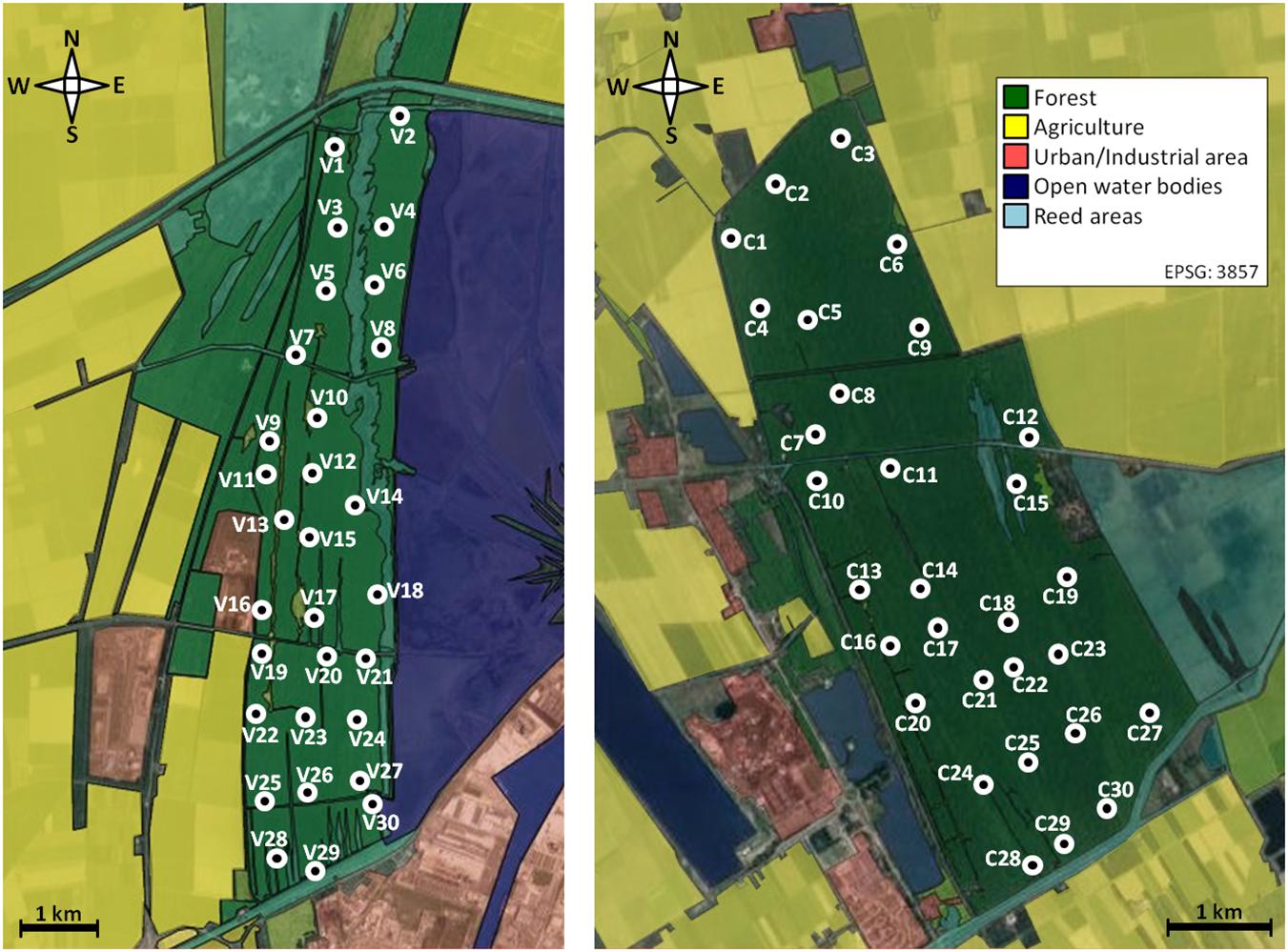
Figure 1. Map showing the 60 study sites (white circles) within the two investigation areas in NE Italy (left: Pineta san Vitale, right: Pineta di Classe). The map is based on Google MapsTM satellite images and modified via QGIS. The coordinates of the two reserves are: 44°27′48.09″ to 44°31′39.15″ N; 12°13′01.08″to 12°14′16.97″ E (Pineta san Vitale), 44°19′35.00″ to 44°22′36.35″ N; 12°15′35.51″ to 12°18′04.46″ E (Pineta di Classe).
Moth sampling took place in June and in August. So, two samples of each site were available, representing the early summer and late summer moth aspect. For the following analysis, we pooled these two samples to get one species abundance list per site. We used automated light traps as described in Axmacher and Fiedler (2004), equipped with two 18W tubes (Sylvania Blacklight and White Blacklight) powered by 12V dry battery packs. Start of moth sampling was at dusk, with a sampling duration of about 5-8 h per night. As moths normally are on the wing until midnight and only few individuals are active after the dew point is reached (personal observations), we assume that minor differences in sampling time per night did not affect the outcome. All Lepidopterans found in the traps were subsequently mounted and identified to species level using faunal monographs. Where necessary, we dissected genitalia for identification.
For functional diversity analysis, 50 ecological characters were collated, scoring 14 different physiological, behavioral and ecological traits. Traits to describe the physiological and phenological characteristics of species were: mean forewing length, the presence of a proboscis, voltinism, and the overwintering stage. Behavioral traits comprised larval sociality, activity time of day of adults, and migratory behavior. Ecological traits were: the degree of larval food specialization, identity of larval food plant families (taking into account 16 frequently used plant families), development in beehives, food plant type (deciduous trees, conifers, grasses, herbs, inside fruits, on lichens/algae, fungi, mosses, detritus, water plants, in wood, or root feeding), larval feeding mode (endophagous, ectophagous, or semi-concealed between folded leaves), preferred habitat type (forest, shrub, grass, reed), and the northern limit of the distribution area in Europe. Details on scoring of the traits and sources of data can be found in Supplementary Table 2.
Using this broad array of traits, we primarily aimed to achieve an integrative description of the ecological needs of all sampled moth species. Most information can be considered as response traits, yet the segregation of effect from response traits for moths remains controversial. Some characteristics like body size or the number of generations per year must be considered both, effect and response trait. Indeed both concepts may overlap (Suding et al., 2008). Many unequivocal effect traits like pollination efficiency or nutritional value for birds and bats remain unknown for practically all species. As a consequence, we decided not to partition our analyses arbitrarily between putative response and effect traits.
Habitat Quality Metrics (Local Factors)
For surveying vegetation composition and structure, we sampled each site with five 1 × 1 m2 plots in the herb layer, and five 5 × 5 m2 plots in the shrub layer. This was done in spring and early summer (April-June), when many species were in flower and so more easy to identify. Within these plots, every vascular plant species was identified and recorded. Forest structure was analyzed by doing 10 point-centered-quarter analyses per site, following Mitchell (2010). The point-centered-quarter analysis is a non-plot-based sampling technique for analyzing forest structure, where the distance to the four nearest trees is measured. Additionally, for each of the recorded trees its diameter at breast height, height and species identity were noted. With these data, we were able to calculate proxies for forest density (in trees/ha), forest cover of deciduous and conifer trees (in m2/ha), mean basal areas of trees (as a proxy for forest age) and the standard deviation of basal areas (as a proxy for age heterogeneity). As an additional component of forest structure, we took four tree crown density measurements per season (16 measurements per site in total) using a forest densiometer, and estimated the amount of deadwood around the light trap location by sight. Across all plant species found at a location, mean Ellenberg indicator values for humidity, soil nutrients, temperature and light were calculated. For the functional analysis of plants, we established a trait matrix containing 46 different functional aspects like family affiliation, mode of seed dispersal, root type or leaf structure (Supplementary Table 1). We then calculated functional dispersion among plant species per site using the packages FD (Laliberté and Legendre, 2010) and vegan (Oksanen et al., 2018) in the statistical R environment (R Core Team, 2018). Additionally, we quantified compositional herb and shrub heterogeneity (beta diversity) using the betadisper function for multivariate dispersion (Anderson et al., 2006). Further details on the vegetation sampling can be looked up in Uhl et al. (2020a). All habitat quality metrics are listed in Table 1.
Habitat Quantity Metrics (Landscape-Scale Factors)
At the landscape level, we analyzed two different scales of effect: the close vicinity around the sampling locations (200 m buffer) and the larger-scale landscape context (1000 m buffer) around each light trap location. The 200 m buffer hereby was mainly describing habitat quantity inside the conservation areas, while the 1,000 m scale was strongly influenced by landscape elements outside the reserves. Therefore, the two scales – which are not correlated – were considered as revealing complementary aspects of the landscape context of our study sites. For the analysis, we used the program QGIS (QGISDevelopmentTeam, 2018) and satellite images taken from the two forest areas in 2017, as provided by Google MapsTM. We worked with a scale of 1:9083 (tiles resolution 256 px × 256 px).
Based on the satellite images, we defined in total six different landscape elements: forested area, open grassland, reed vegetation, open waterbodies, urban/industrial areas, and arable fields. We created semi-transparent polygons for each, setting the area covered by the referring habitat type (Figure 1). From the area fractions of the first three landscape elements, we calculated the Shannon diversity of natural habitats, while the last two landscape elements were summed up as ‘proportion of modified areas’. At the small-scale (viz. 200 m), only the proportion of open grassland and reed areas, as well as the diversity of natural habitats was used for further analyses, as other structural elements like open water and human-modified land were mostly missing within this radius. The proportion of forest area was also discarded, as it was inversely proportional to the other measured structures. For the wider landscape level (1,000 m), we included the proportion of forest, open and reed areas as well as the proportion of modified areas around the moth collection sites. Additionally, landscape Shannon diversity of natural habitats and edge density (as a proxy for landscape fragmentation and permeability, in m/ha) within 1,000 m radius were considered. For the edge density, the length of all borders between different habitat types within the 1,000 m radius were measured and extrapolated to the unit m/ha. Edge density serves as proxy for landscape impermeability, as every border between two habitat types can pose a barrier for dispersing insects. Finally, we also measured the distance from each light trap site to the nearest forest edge (to address possible edge effects), water canal (as a proxy for water availability) and industrial plant (as potential pollution source). All habitat quantity metrics are listed in Table 2.
Data Analysis
Where appropriate, environmental data were transformed to approximate Gaussian distributions. Proportions like tree crown density and landscape element quantity were logit transformed (Warton and Hui, 2011). After that, we performed a PCA with all 14 local variables to avoid collinearity. The resulting first five PC-axes served as environmental predictors in subsequent linear mixed effects models (LMMs). The same procedure was applied to the 12 landscape descriptors (see also Uhl et al., 2020b; Supplementary Table 3). For both PCAs varimax rotation was applied to capture as much environmental information as possible in the first eigenvalues.
For the functional diversity analysis, a dendrogram of species according to their trait scores, based on Gower dissimilarities and Ward clustering, was calculated. Using this dendrogram, we defined and named different functional groups, to better understand the occupancy of functional trait space in the moth communities. We defined the critical threshold for separating groups after visual inspection of the species clusters following their ecological characteristics (Supplementary Figure 1). With a distance threshold of 0.3 we assessed whether these so defined groups differed significantly from another by performing a Permanova test with 999 permutations, as implemented in the ‘vegan’ package in R (Oksanen et al., 2018). Trait space occupancy was calculated for each sampling site by dividing the number of locally represented functional groups of moths through the total number of functional groups that was found throughout PsV and PdC together. It is therefore a proportional value and was logit transformed for inclusion in LMMs. As a measure of functional redundancy, we calculated the mean number of species present in each functional group per site. The remaining standard functional diversity measures were calculated using the package ‘FD’ (Laliberté and Legendre, 2010). Contrary to trait space occupation and functional redundancy measurement, the calculation of FRic, FEve, FDiv and FDis was done using a convex hull volume approach with the ‘dbFD’ function.
FRic is defined as the convex hull volume on trait space (Villéger et al., 2008). It therefore is only dependent on species trait values and not abundance-weighted. FEve describes the distribution of species in trait space. It normally is calculated as the equalness of distributions of the species among the minimum spanning tree in trait space (Villéger et al., 2008). When weighted by abundance, FEve describes two different components, the regularity of species distribution in trait space and the homogeneity of species abundances (Legras and Gaertner, 2018). Without weighting, only species distribution is considered. So, as FEve is composed of these two components this index sometimes fails in reflecting real functional evenness (Legras and Gaertner, 2018). Hence, we followed the suggestion of Legras and Gaertner (2018) and computed abundance-weighted as well as unweighted versions of FEve to better understand the contribution of its two components. FDiv captures the deviance of individual species to the trait space center, weighted by abundance (Villéger et al., 2008). It therefore represents niche differentiation and indicates the degree of competition among abundant species (Mason et al., 2005; Mouchet et al., 2010). Independently from these three aspects, FDis represents a functional pendant to the taxonomic species diversity indices, as it reflects the abundance-weighted dispersion of species in trait space (Laliberté and Legendre, 2010). Following Bellwood et al. (2005), who defined ‘functional specialization’ as ‘the relative distance of a species from the centroid’, FDis – as it is calculated through the abundance-weighted mean distance between the species and the functional trait space centroid (Laliberté and Legendre, 2010) – might also be interpreted as “mean functional specialization” of a community.
We used the seven different functional diversity indices as response variables in linear mixed effects models, done with the ‘nlme’ package (Pinheiro et al., 2018). Reserve affiliation served as random factor. We first constructed full models without interactions between predictors and then selected the best model for each functional diversity index by using the Akaike information criterion (AIC) with the stepAIC function in the MASS R package (Venables and Ripley, 2002). The statistical routine of stepAIC selects the best model by stepwise adding and removing predictors to the initial model and checking for the resulting AIC values. The residuals of the resulting best models were then checked for spatial autocorrelation using Moran’s I test. As spatial autocorrelation never was an issue, no further correction approaches were needed. Additionally, bivariate models for each response variable with every predictor separately were performed to check for any relationships that might be hidden within the model selection approaches.
Results
We analyzed 23,375 moth individuals, representing 387 species in 27 families. The first five PC-axes of each of the two PCAs explained 71% (local factors) and 84% (landscape factors) of the total measured variation, respectively. To facilitate understanding, we named these PC-axes following their major factor loadings (Supplementary Table 3). For the local factors, the first ‘Humidity-nutrient gradient’-Axis describes a gradient from drier and nutrient poor locations with open canopy, to sites with denser foliage, where soil humidity and nutrient availability is higher. The second axis was mainly loaded by forest age and density and therefore is called ‘Old, open forest’. The third axis (‘Plant diversity’) mirrors increasing plant species richness and plant functional diversity. The fourth ‘Conifer cover’ axis shows the gradient from sites with low to high amount of conifer biomass contributing to the forest stands. Additionally, conifer sites were characterized by a more heterogeneous herb layer. The fifth axis (‘Tree health’) reflects the decreasing number of dead trees at a location.
For the landscape PCA, the first axis ‘Habitat diversity’ refers to the diversity of near-natural habitats close to the sampling sites (radius of 200 m). The vicinity to industrial plants is also represented by this axis. The second axis ‘Anthropogenic influence’ shows the gradient from reserve centers with many natural areas around to the forest edges, where increasingly modified areas in a 1,000 m range around the sampling site can be found. The third axis ‘Landscape diversity’ reflects an increasing diversity of natural habitats in a 1,000 m radius. ‘Landscape fragmentation’ mainly represents the landscape-wide edge density, while the fifth axis ‘Open habitats’ describes the proportion of open habitats within 200 m around the sampling locations.
The functional dendrogram of moths shows two main sections, with one containing mainly forest dwelling species dependent on trees (e.g., larvae feeding on foliage of trees or developing in wood), and the other mostly consisting of open habitat species with other larval feeding habits (most of them feeding on herbs, but also including grass, moss and lichen feeders). These sections further split up into 25 functional groups (8 in the first forest species section, 17 in the second section) which were significantly different from each other (R2 = 0.71, p = 0.001). The functional groups and the whole dendrogram are presented Supplementary Figure 1. Each functional group comprised 6 to 49 species (mean: 15.5 ± 9.7), indicating substantial functional redundancy in the regional moth fauna. The largest group (49 species) comprises forest species which develop as concealed feeders on deciduous trees, while the smallest group (6 species) contains all conifer-feeding species. Based on the functional groups, trait space occupancy and mean functional redundancy per site were calculated. Highest mean functional redundancy was found at site V14 (trait space occupancy: 100%, mean functional redundancy: 5.12 species per functional group), while C22 had lowest values (trait space occupancy: 60%, mean functional redundancy: 1.32 species; Figure 2). On average, we found 2.96 species per functional group at each site.
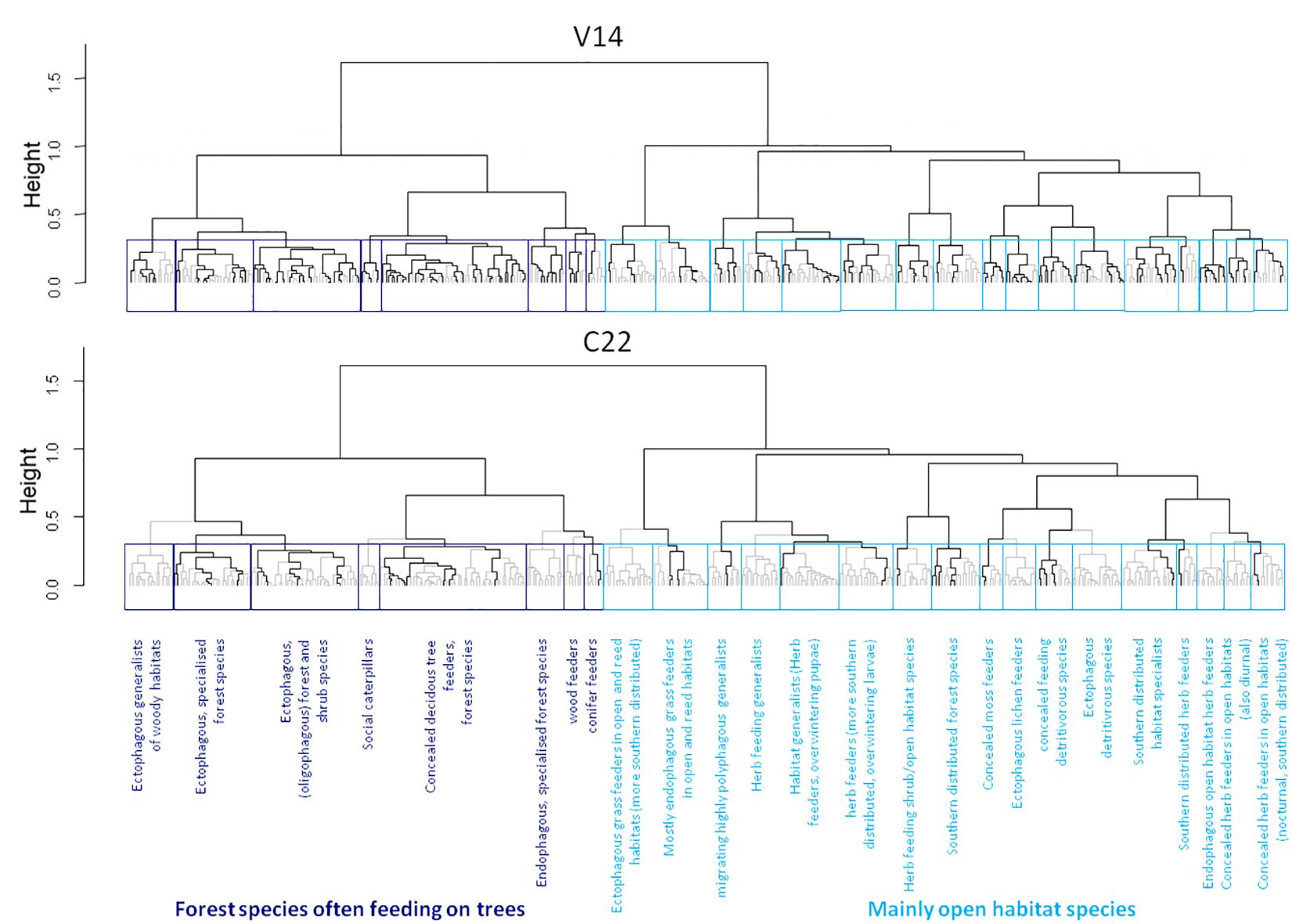
Figure 2. Functional dendrogram of the whole observed moth species pool (387 species) in the two pine forest reserves in NE Italy. The two most extreme local moth communities are depicted exemplarily. Upper panel: a species-rich ‘high quality’ plot (V14) with multiple species represented in most functional groups. Lower panel: a species poor plot (C22) with few or even no species representing most functional groups. Species present at each site are indicated by black lines. Statistically significant sub-clusters after a PERMANOVA (accepted as functional groups in this study) are shown as blue boxes. Different colors indicate the two main sections of species that generally partition the functional dendrogram into moths of woodland (dark blue) and open habitats (light blue), respectively.
Detailed results of the bivariate linear mixed effects models can be found in Supplementary Table 4. We observed significant positive relationships between FRic and ‘Plant diversity’, the ‘Humidity-nutrient gradient’ and ‘Landscape diversity’ (Figures 3A,B,D), which were – together with ‘Landscape fragmentation’ (Figure 3C) – combined in the best model (Table 3). Altogether, these factors explained roughly 50% of the total variation. The best model for trait space occupancy comprised six predictors, of which ‘Plant diversity’, the ‘Humidity-nutrient gradient’, ‘Landscape diversity’ (Figures 4A,B,D) and the ‘Old, open forest’-axis were positively correlated, while ‘Tree health’ (Figure 4C) and ‘Landscape fragmentation’ were negatively correlated with the response variable (Table 3). Here, 43% of the total variation could be explained. Looking at functional redundancy, four factors, viz. ‘Plant diversity’, the ‘Humidity-nutrient gradient’, ‘Landscape diversity’ (all three positively correlated, Figures 5A,B,D) and ‘Landscape fragmentation’ (negatively correlated, Figure 5C) were included in the best model (Table 3). The model explained 47% of the total variation.
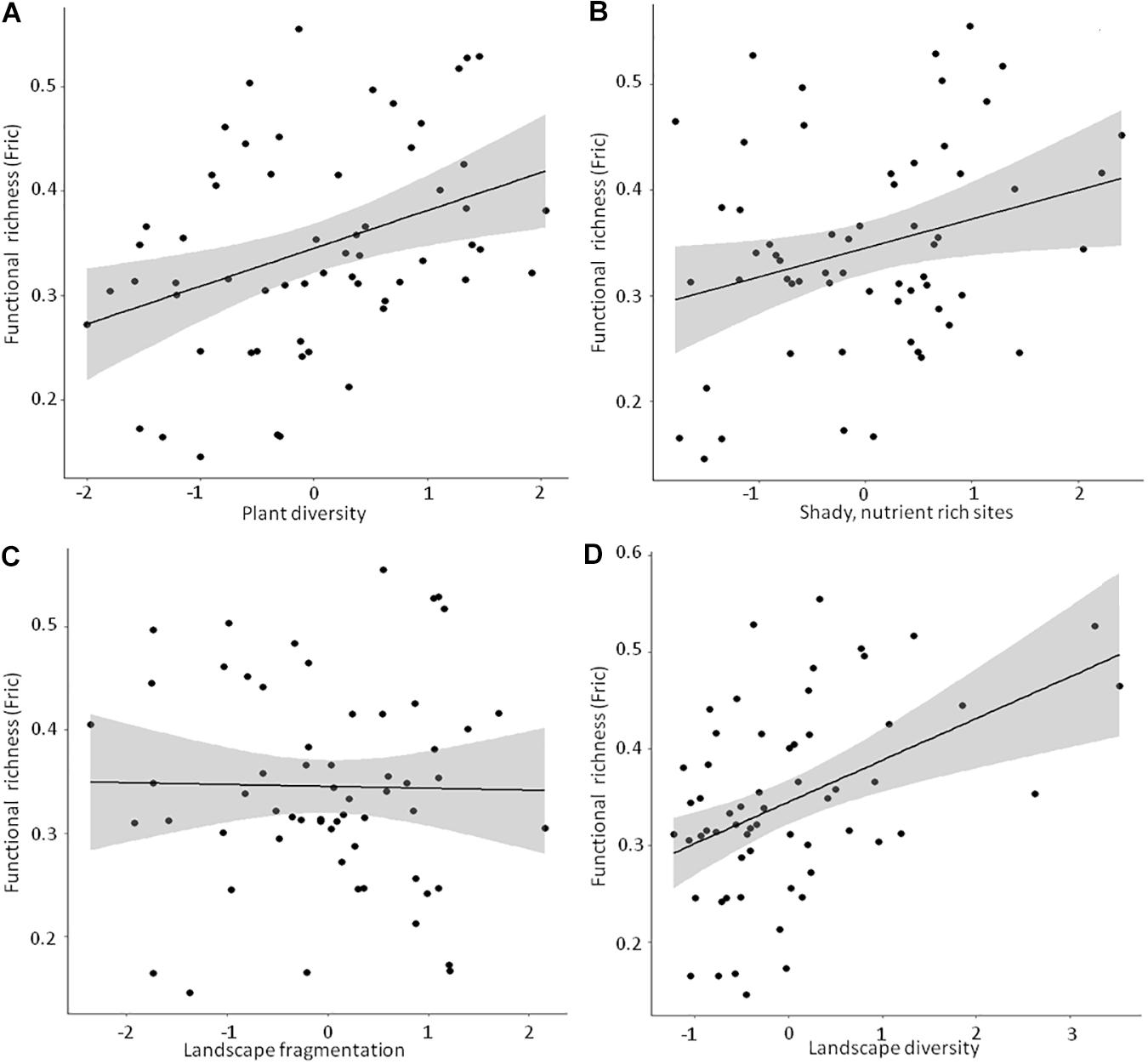
Figure 3. Linear bivariate regressions showing the relationships between moth functional richness (FRic) and (A) Plant diversity, (B) Shady, humid, nutrient rich sites, (C) Landscape fragmentation and (D) Landscape diversity. The detailed results of the linear mixed effects models (shown as black line and shaded area) can be looked up in Supplementary Table 4.
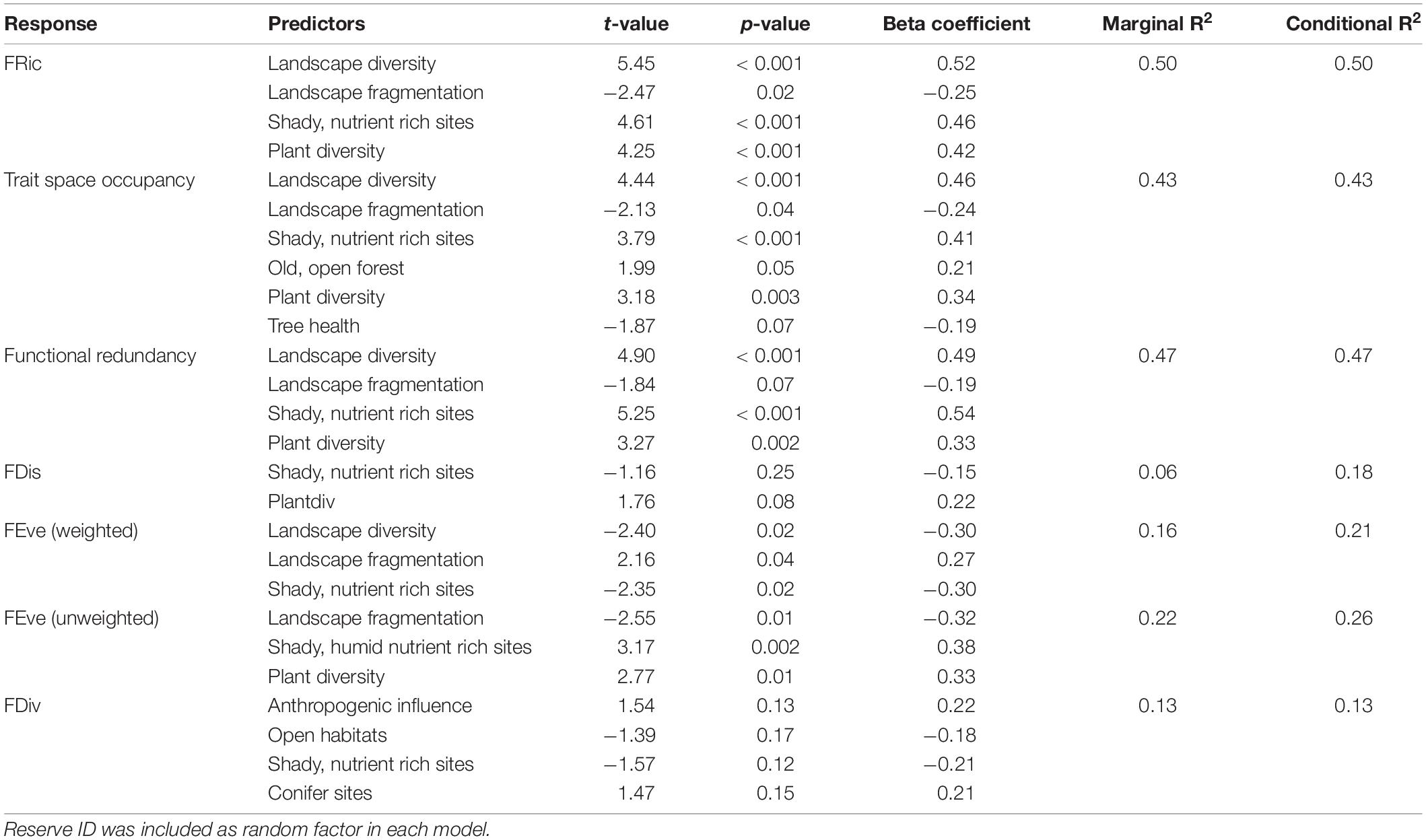
Table 3. Best models for seven different functional diversity indices (as response variables) and multiple predictors tested simultaneously, selected via AIC.
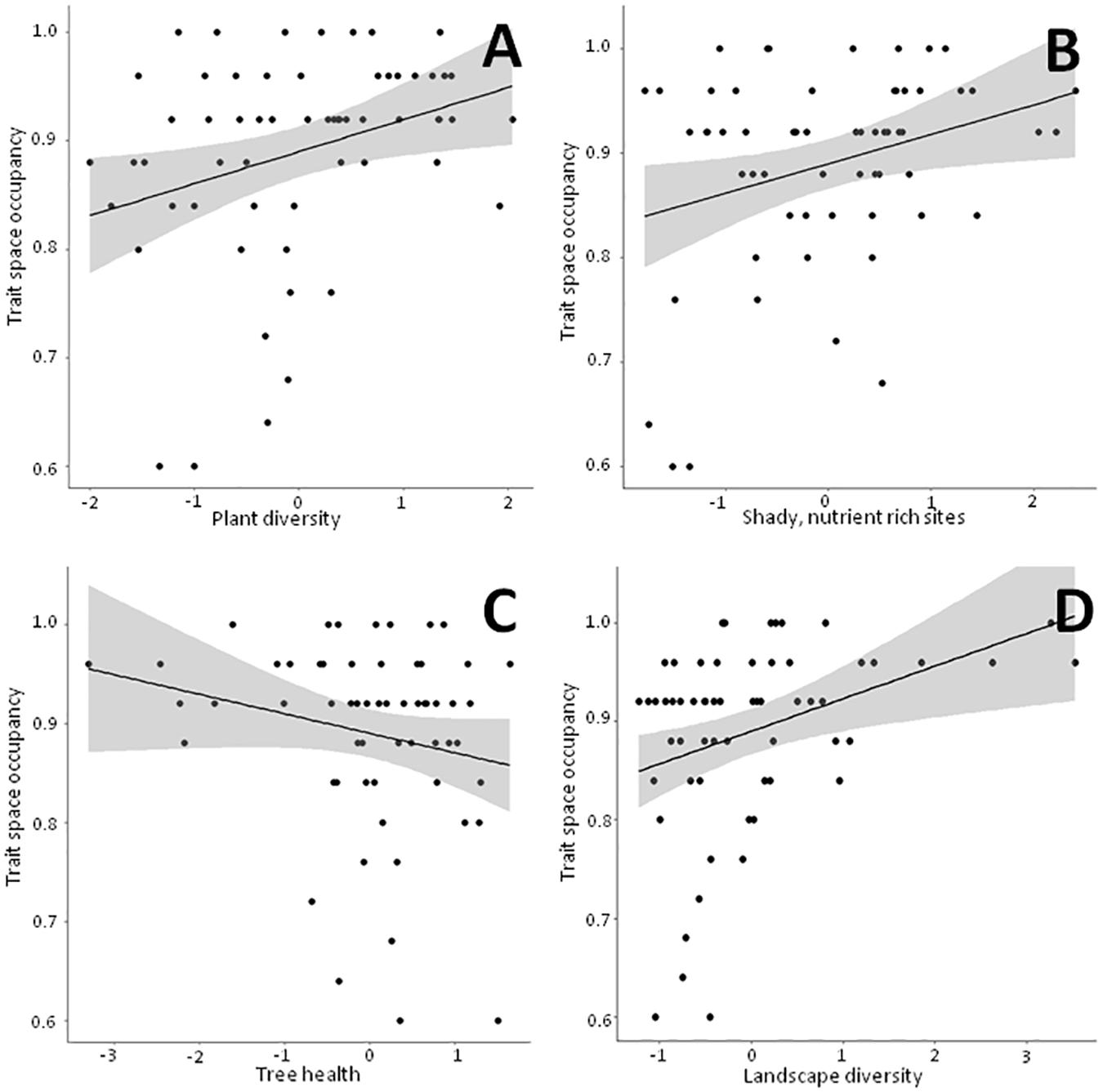
Figure 4. Linear bivariate regressions showing the relationships between trait space occupancy and the PC axes depicting (A) Plant diversity, (B) Shady, humid, nutrient rich sites, (C) Tree health and (D) Landscape diversity. The detailed results of the linear mixed effects models (shown as black line and shaded area) can be looked up in Supplementary Table 4.
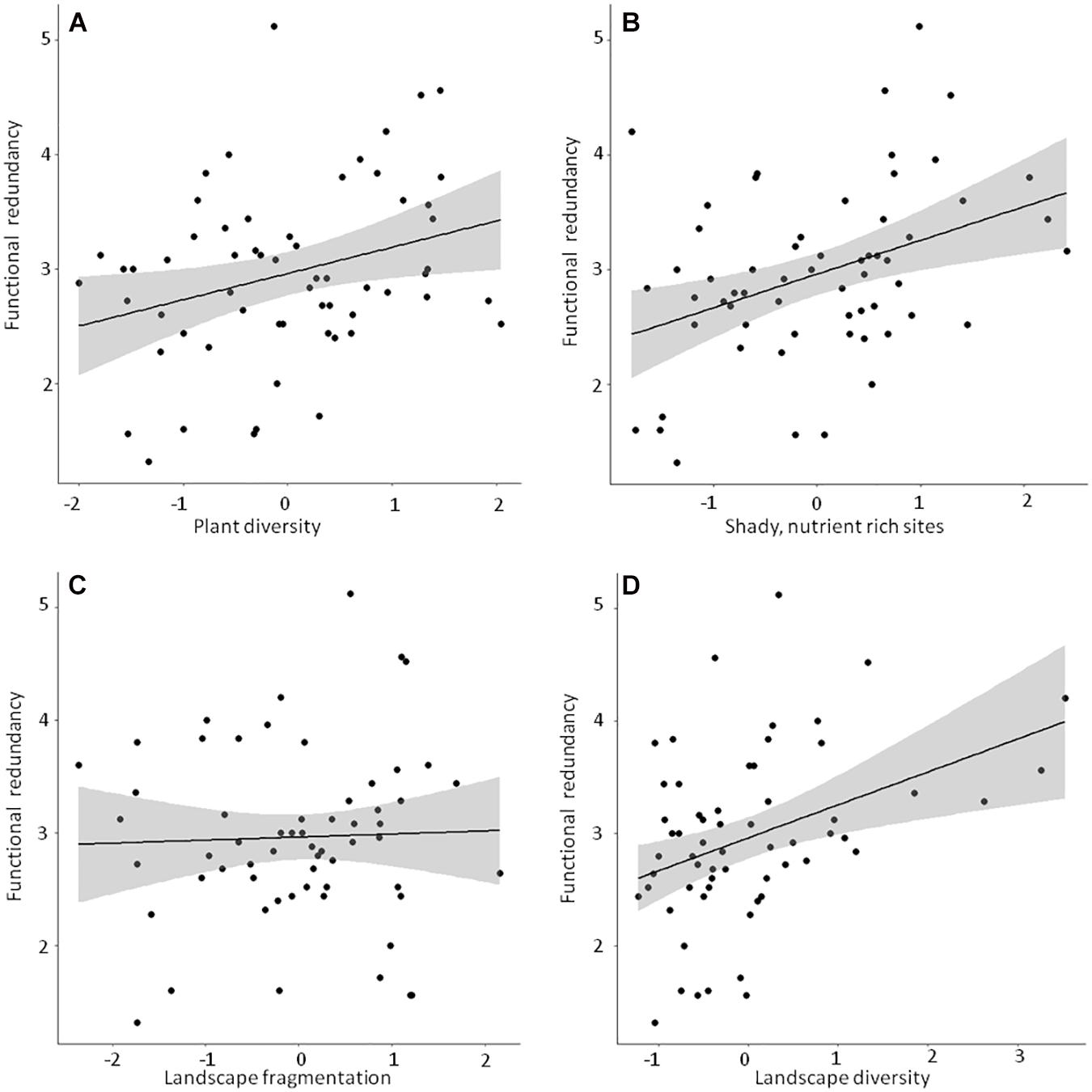
Figure 5. Linear bivariate regressions showing the relationships between functional redundancy and (A) Plant diversity, (B) Shady, humid, nutrient rich sites, (C) Landscape fragmentation and (D) Landscape diversity. Detailed results of the linear mixed effects models (shown as black line and shaded area) can be looked up in Supplementary Table 4.
For FEve, we observed differences in model behavior between the abundance weighted and un-weighted index versions. The best model for abundance weighted FEve revealed ‘Landscape diversity’, the ‘Humidity-nutrient gradient’ (both negatively correlated) and ‘Landscape fragmentation’ (positive correlation) as significant predictors (Table 3). Twenty-one percent of the total variation could be explained by this model. Without abundance weighting, the best model for FEve (viz. the regularity of species distribution) combined the ‘Humidity-nutrient gradient’, ‘Plant diversity’ (both positively correlated) and ‘Landscape fragmentation’ (negative relationship) as predictors (Table 3). The model captured 26% of the total variation. FDiv and FDis were not correlated to any of the tested factors in single predictor models (Supplementary Table 4). Multivariate models for FDiv and FDis only had small R2 values and therefore explained but minor fractions of variation (Table 3).
Discussion
We analyzed multiple environmental gradients and their influence on various aspects of functional diversity of a species-rich group of insects within two nature reserves. Especially FRic and niche occupation may give insight into the possible multifunctionality of the local ecosystem, as we infer that with higher niche occupation, the function of the local ecosystem is guaranteed. Functional redundancy, on the other hand, can be seen as an indicator for ecosystem resilience (Feit et al., 2019), as with more species occupying the same functional group, it is less likely that the loss of single species immediately leads to a loss of functioning (‘insurance hypothesis’: Yachi and Loreau, 1999). Conversely, any further loss of species at sites with already low functional redundancy may directly translate into the reduction of ecosystem functionality, as some functional groups are then no longer occupied.
General Characteristics of Moth Functional Diversity
The functional group divisions of moth species clearly reflected the different habitat structures available to these insects in the study area, mainly consisting of forest sites, but also reed and open habitat patches. Overall, the 387 moth species recorded covered a wide range in functional trait space and could be grouped into 25 clusters according to a multitude of trait data. The by far biggest of these groups consisted of forest species, whose caterpillars live concealed on deciduous trees. In contrast, conifer feeders, species living inside wood and moths with caterpillars living gregariously had lowest species numbers. The presence of various moss and lichen feeders as well as over 25 detritivorous species also mirrors the wide variety of feeding niches available to Lepidopterans in the two studied reserves. Such a broad representation of functional niche space is typical for Lepidoptera in a near-natural forest area (Summerville and Crist, 2003; Thorn et al., 2015).
Niche occupation at all 60 sites was never less than 60%, and every niche was on average occupied by three species per site. Yet, in PdC niche occupation and functional redundancy was in general lower than in PsV, what mirrors the reduced habitat structural richness, compared to PsV (Uhl, 2020). Some of the PdC sites are completely dominated by monotonous pine forest stands. Open grassland or reed vegetation was largely missing, particularly in the center of the reserve. This lack of alternative habitats seems decisive for the absence of species like Laelia coenosa and Schoenobius gigantella which are bound to reed areas (Uhl, 2020). We therefore conclude that habitat mosaics within conservation areas significantly enhance functional redundancy on site and therefore ameliorate local ecosystem resilience. These findings are in line with previous studies pointing out the importance of local environmental heterogeneity for ecosystem resilience (Oliver et al., 2015). Functional redundancy within moth assemblages was especially low at six locations (one of them situated in PsV, five in PdC). With a value below two, these moth communities showed almost no redundancy as every functional group was on average only occupied by one species, if at all. This was not only true for functional groups comprising species bound to special habitats, but also for typical forest moth groups (Figure 2 and Supplementary Figure 1). At these impoverished sites, any further erosion in species richness might directly affect ecosystem resilience as some niches would become vacant. Most of these functionally impoverished locations were surrounded by monotonous landscapes and were locally characterized as nutrient-poor, dry forest stands. Importantly, low landscape diversity values at these sites were not driven by land use outside the reserves – which was represented by the ‘Anthropogenic influence’-axis – but by the monotonous habitat structure inside the reserves. Especially the five functionally most impoverished moth assemblages in PdC were located near the reserve center, where only few other near-natural habitats were breaking up the pine stands.
With our small-scaled analyses of functional diversity patterns, we were able to explain up to 50% of the variation within our data. The residual variation might have been driven by local microclimatic and weather conditions, which are known to strongly affect moth flight-to-light-behavior. Cooler night time temperatures result in fewer moths being on the wing, while in warmer nights insects are more active and consequently more likely to get caught by a light trap (Jonason et al., 2014). Also insect behavior at light traps can be influenced by temperatures, with lower temperatures favoring immediate settling and therefore reducing the probability of moths to fall into the sampling container (Wölfling et al., 2016). We always sampled moths in warm and dry nights. However, even small changes in temperature might have affected the number of moths being caught. By pooling the data from early and high summer, we additionally tried to minimize such sampling night effects, but still some variation in insect samples might result from weather conditions. Some bias furthermore might have occurred because of inter-annual fluctuations in moth species abundances, as data sampling was split up over three years. Due to the large number of light trap locations and a limited number of light traps that could be run simultaneously, we were not able to avoid this potential bias.
Within the multivariate models, only four of the ten candidate predictors (five local and five landscape-scale PC-axes), representing 28 condensed raw variables, had a major effect on functional diversity of insect assemblages, as these four factors were included in the best models selected via AIC. ‘Landscape diversity’ and the local ‘Humidity-nutrient gradient’ were the strongest predictors for functional richness, redundancy and niche occupation among moths, as indicated by their beta coefficients. Furthermore, these two predictors seem to play equally important roles for insect functional diversity, supporting our first hypothesis. At shady sites with humid and nutrient-rich soils, surrounded by diverse near-natural landscapes within 1,000 m radius, we found in general more niches to be occupied by moths, more species per functional group and – in consequence – higher functional richness. Furthermore, plant diversity (positively) and landscape fragmentation (negatively) always played a role in the best models. Previous findings already showed that moth taxonomic species richness and diversity is promoted by near-natural vegetation and large-scale landscape structure (Merckx et al., 2012a; Botham et al., 2015; Root et al., 2017). By including also functional species traits, our results demonstrate that these proxies of habitat quality and quantity also affect community attributes that potentially translate into ecosystem multifunctionality and resilience.
Habitat Quantity Aspects and Functional Diversity
With regard to habitat quantity at the landscape scale, landscape diversity was the most important predictor for our measures of insect functional diversity. Surrounding landscape diversity affected community attributes related to ecosystem function (occupancy) and resilience (redundancy) inside protected nature reserves. Positive effects of landscape-scale diversity on functional redundancy have already been found in agricultural systems (Feit et al., 2019). However, to our knowledge there was to date no evidence on the landscape context also influencing the functional richness of insects inside nature reserves. Nevertheless, effects of landscape context on local insect biomass and diversity (Seibold et al., 2019) might have indicated such a correlation to exist.
Besides the diversity of near-natural habitats around the reserves, also the shape and structure of landscape elements influenced moth functional richness. Sites surrounded by a landscape with higher edge density had in general lower functional redundancy, fewer occupied niches and in consequence lower functional richness. More landscape edges can affect how species disperse throughout a region (Collinge and Palmer, 2002) or influence a habitat’s microclimate through edge effects (Schmidt et al., 2017). However, the influence of landscape fragmentation on moth functional diversity was much less pronounced than the effect of landscape diversity. Landscape fragmentation only occurred as significant factor within combined models, but never was correlated with functional richness aspects, when tested as predictor in bivariate models. In fact, edge density might have some limitations in representing a measure for landscape fragmentation. First, edges are not equal: A habitat border between forest and open water builds a much stronger contrast than a habitat edge between reed and open water. Resulting differences in edge structure and microclimate can of course also have effects on biotic communities (Laurance et al., 2007). Second, edge density/landscape fragmentation might easily be confused with habitat fragmentation, although both terms describe completely different things. Habitat fragmentation is typically analyzed on larger scales, comparing multiple habitat patches that differ in isolation and patch size. Both of these components – habitat isolation and habitat area – are important drivers of larger-scale biodiversity patterns and can affect the biodiversity-ecosystem functioning relationship (Liu et al., 2018). Our edge density/landscape fragmentation factor, in contrast, neither reflected with patch sizes nor isolation effects. It is rather a term for increasing landscape configurational diversity and decreasing landscape permeability for dispersing insects.
Altogether, landscape compositional (viz. landscape diversity) and configurational (viz. landscape fragmentation) heterogeneity were driving the functional diversity of moth communities inside the studied nature reserves, with composition being more important than configuration. A diverse landscape composition might offer more niches and therefore favor the establishment of a functionally diverse moth community (supporting the landscape divergence hypothesis of Laurance et al., 2007). Perović et al. (2015) investigated these two aspects of landscape heterogeneity in grassland areas and found landscape composition to affect butterfly taxonomic diversity, while configuration only seemed to play a role for functional composition. Contrastingly, in studies on agri-environmental schemes, only configurational heterogeneity and not crop diversity (as a measure for landscape composition) influenced pollinators (Hass et al., 2018). The importance of composition and configuration therefore is strongly dependent on the focal ecosystem and seems also to vary among groups of organisms that are studied. In agricultural areas for example, unmanaged edges and hedgerows can serve as important microhabitats for insects (Merckx et al., 2012b), whereas edges in forest habitats can represent insurmountable barriers for some forest species (Slade et al., 2013).
Other landscape-scale predictors did not substantially affect moth functional diversity, although we had expected some correlations to occur with the small-scaled ‘Habitat diversity’-axis or the ‘Anthropogenic influence’ gradient. It seems that directly neighboring surroundings within 200m radius were by far not as important for the functional integrity of local moth assemblages as larger-scale availability of different natural habitats (Merckx et al., 2012b, 2018). Perhaps the rather high mobility of many moths here plays a role and leads to small-scale neighborhoods being not as important as the wider surroundings. For the ‘Anthropogenic influence’-axis, we had expected some negative correlations, as other studies already have shown that intensified land use in the vicinity of conservation areas also affects nearby natural habitats (Seibold et al., 2019). However, for the two coastal nature reserves, surrounding agriculture and industrial areas seem to play minor roles in their immediate vicinity. Perhaps, separate consideration of the two reserves might yield different results, as for PsV some relationships between the proximity of industrial areas and local micro-moth FD inside this reserve have already been established (Uhl et al., 2016; Uhl, 2020). Anthropogenic influences might therefore affect biodiversity punctually and have to be investigated individually for each reserve (Uhl, 2020). More generally, however, our results indicate that the two rather big natural areas are able to preserve insect biodiversity to some extent. By further ameliorating the diversity of natural habitats inside the reserves or the connectivity between natural areas of different habitat structures, conservation efforts could be even more successful.
Local Habitat Quality Aspects and Functional Diversity
As expected, local habitat quality was always important for moth communities. Mainly two specific predictors emerged as relevant: The ‘Humidity-nutrient gradient’ and ‘Plant diversity’. In the two nature reserves under study, the first of these factors describes successional stages of forest development, which once started as rather open pine plantations and since then have developed toward a near-natural forest vegetation (Wölfling et al., 2019). At some places in the reserves, the dry and open structure of the ancient plantations is still visible, whereas other locations are nowadays characterized by forest offering shady and more humid habitat conditions for insects. The availability of water and nutrients is in general a limiting factor for vegetation in coastal Mediterranean ecosystems (Pérez-Ramos et al., 2012). Here, natural succession can also play an important role for the natural water cycle, as shadier sites retain more humid soils by reducing direct sun light (Von Arx et al., 2012). The retention of water is especially important for Mediterranean ecosystems being prone to increasing drought events in terms of climate change (Barredo et al., 2016). Besides these positive effects for water management, shady sides can also stabilize microclimatic conditions (Kovács et al., 2017). Structurally rich forests and stable microclimate, arising from secondary succession, might finally result in more insect species being able to inhabit such places, enhancing functional richness and therefore also ameliorating ecosystem functionality. Additionally, plant diversity played a role for maintaining a functionally rich insect community. Obviously, the more different plant species grow at a location, the more herbivorous insect species may find suitable food resources at this site. This positive effect of plant richness on moth species diversity has already been observed for different forest management regimes (Root et al., 2017) and for small-scaled site-to-site variation in the same two forest reserves as investigated here (Uhl et al., 2020b).
Contrary to expectation, all other descriptors of local habitat conditions, such as ‘Conifer cover’, ‘Tree health’ and ‘Old, open forest’, did not substantially affect FRic and redundancy. However, the latter two predictors were included in the best model for niche occupancy, supporting our second hypothesis. Niche occupation was declining where fewer dead standing trees were found. This might underline the importance of deadwood for forest ecosystems, as decaying wood material itself is a food resource for some insect species (Gossner et al., 2013). Decaying trees that remain standing upright inside a forest contribute to the formation of forest gaps which in turn facilitate more herb and shrub species to grow. Indeed, old open forest sites had more niches occupied by moths than younger and dense forest stands, indicating that with growing forest age, more niches become available and subsequently more insect species are able to establish and occupy these habitat structures (Schowalter, 2017). However, these relationships that are rather well documented for relationships between insect species richness and vegetation succession in many other systems, only described minor fractions of variation in moth functional diversity in our study and were far less important than plant richness and the humidity-nutrient gradient.
Conclusion
Even though based on an in-depth study in just two conservation areas, our results underline the equally strong importance of habitat quality and quantity aspects for the functional integrity of species-rich insect communities within nature reserves. For preserving local biodiversity and counteracting insect loss, there are mainly two conclusions that can be derived from our results. First, local management should aim at increasing the diversity of near-natural habitats within and beyond the boundaries of reserves. This is important for ameliorating functional redundancy and therefore ecosystem resilience on site. Especially for woodland habitats, maintaining structurally-rich, old-grown forests with diverse understory, forest gaps and high plant species richness can contribute to preserving insect biodiversity, which in turn is essential for terrestrial ecosystem functionality (Weisser and Siemann, 2013). Second, our results show that local management needs to be supported by landscape-scale actions, even if conservation areas are relatively large. Ameliorating the diversity of near-natural landscape elements and simultaneously reducing landscape fragmentation can enhance the functional richness of local insect communities. The importance of landscape-scale actions to promote diverse habitats and connectivity is therefore crucial for conservation success, as local management alone can likely not preserve biodiversity in isolated nature reserves in the long run.
Data Availability Statement
The original contributions presented in the study are included in the article/Supplementary Material, further inquiries can be directed to the corresponding author/s.
Author Contributions
BU developed the study design and formulated the research questions. BU and MW sampled the field data. MW organized permits for field work. BU wrote the manuscript and performed the statistical analyses. MW and KF edited and revised the manuscript. KF supervised the work. All authors contributed to the article and approved the submitted version.
Funding
BU gratefully acknowledges financial support from the Faculty of Life Sciences, University of Vienna, and the Heinrich Böll Stiftung, Berlin.
Conflict of Interest
The authors declare that the research was conducted in the absence of any commercial or financial relationships that could be construed as a potential conflict of interest.
Acknowledgments
We are thankful to Angela Vistoli and Corbaro Lamberto (Commune di Ravenna) for issuing collecting permits. Enrica Burioli (Commune di Ravenna) kindly provided information on the history of the two forest reserves. We also like to thank Oliver Schweiger, Ben Woodcock, Thomas Merckx and further reviewers for multiple helpful comments on our manuscript. We especially thank Michelle Dorenkamp, Ute Fricke and Franziska Schäfer, who assisted during field work.
Supplementary Material
The Supplementary Material for this article can be found online at: https://www.frontiersin.org/articles/10.3389/fevo.2021.637371/full#supplementary-material
References
Alison, J., Duffield, S. J., Morecroft, M. D., Marrs, R. H., and Hodgson, J. A. (2017). Successful restoration of moth abundance and species-richness in grassland created under agri-envirnomental schemes. Biol. Conserv. 213, 51–58. doi: 10.1016/j.biocon.2017.07.003
Allan, E., Manning, P., Alt, F., Binkenstein, J., Blaser, S., Blüthgen, N., et al. (2015). Land use intensification alters ecosystem multifunctionality via loss of biodiversity and changes to functional composition. Ecol. Lett. 18, 834–843. doi: 10.1111/ele.12469
Anderson, M. J., Ellingsen, K. E., and McArdle, B. H. (2006). Multivariate dispersion as a measure of beta diversity. Ecol. Lett. 9, 683–693. doi: 10.1111/j.1461-0248.2006.00926.x
Andreatta, G. (2010). Proposta di un ‘silvomuseo’ nelle pinete storiche di Ravenna. Forest@ J. Silvicult. For. Ecol. 7, 237–246.
Axmacher, J. C., and Fiedler, K. (2004). Manual versus automatic moth sampling at equal light sources – a comparison of catches from Mt. Kilimanjaro. J. Lepidopterists. Soc. 58, 196–202.
Barredo, J. I., Caudullo, G., and Dosio, A. (2016). Mediterranean habitat loss under future climate conditions: assessing impacts on the Natura 2000 protected area network. Appl. Geogr. 75, 83–92. doi: 10.1016/j.apgeog.2016.08.003
Bates, A. J., Sadler, J. P., Grundy, D., Lowe, N., Davis, G., Baker, D., et al. (2014). Garden and landscape-scale correlates of moths of differing conservation status: significant effects of urbanization and habitat diversity. PLoS One 9:e86925. doi: 10.1371/journal.pone.0086925
Beck, J., and McCain, C. M. (2020). Just bird food? – On the value of invertebrate macroecology. Front. Biogeogr. 12:e47684. doi: 10.21425/F5FBG47684
Bellwood, D. R., Wainwright, P. C., Fulton, C. J., and Hoey, A. S. (2005). Functional versatility supports coral reef biodiversity. Proc. R. Soc. B 273, 101–107. doi: 10.1098/rspb.2005.3276
Botham, M. S., Fernandez-Ploquin, E. C., Brereton, T., Harrower, C. A., Roy, D. B., and Heard, M. S. (2015). Lepidoptera communities across an agricultural gradient: how important are habitat area and habitat diversity in supporting high diversity? J. Insect Conserv. 19, 403–420. doi: 10.1007/s10841-015-9760-y
Bruno, D., Gutiérrez-Cánovas, C., Sánchez-Fernández, D., Velasco, J., and Nilsson, C. (2016). Impacts of environmental filters on functional redundancy in riparian vegetation. J. Appl. Ecol. 53, 846–855. doi: 10.1111/1365-2664.12619
Chisté, M. N., Modi, K., Gossner, M. M., Simons, N. K., Köhler, G., Weisser, W. W., et al. (2016). Losers, winners, and opportunists: how grassland land-use intensity affects orthopteran communities. Ecosphere 7:e01545. doi: 10.1002/ecs2.1545
Clavel, J., Julliard, R., and Devictor, V. (2011). Worldwide decline of specialist species: toward a global functional homogenization? Front. Ecol. Environ. 9, 222–228. doi: 10.1890/080216
Collinge, S. K., and Palmer, T. M. (2002). The influences of patch shape and boundary contrast on insect response to fragmentation in California grasslands. Landsc. Ecol. 17, 647–656. doi: 10.1023/A:1021536302195
Dennis, R. L., Dapporto, L., and Dover, J. W. (2014). Ten years of the resource-based habitat paradigm: the biotope-habitat issue and implications for conserving butterfly diversity. J. Insect Biodivers. 2, 1–32. doi: 10.12976/jib/2014.2.8
Diaz, S., Lavorel, S., de Bello, F., Quétier, F., Grigulis, K., and Robson, T. M. (2007). Incorporating plant functional diversity effects in ecosystem service assessments. PNAS 104, 20684–20689. doi: 10.1073/pnas.0704716104
Duffy, J. E., Godwin, C. M., and Cardinale, B. J. (2017). Biodiversity effects in the wild are common and as strong as key drivers of productivity. Nature 549:261. doi: 10.1038/nature23886
Fahrig, L. (2013). Rethinking patch size and isolation effects: the habitat amount hypothesis. J. Biogeogr. 40, 1649–1663. doi: 10.1111/jbi.12130
Feit, B., Blüthgen, N., Traugott, M., and Jonsson, M. (2019). Resilience of ecosystem processes: a new approach shows that functional redundancy of biological control services is reduced by landscape simplification. Ecol. Lett. 22, 1568–1577. doi: 10.1111/ele.13347
Fiedler, K., Wrbka, T., and Dullinger, S. (2017). Pluralism in grassland management promotes butterfly diversity in a large Central European conservation area. J. Insect Conserv. 21, 277–285. doi: 10.1007/s10841-017-9974-2
Gagic, V., Bartomeus, I., Jonsson, T., Taylor, A., Winqvist, C., Fischer, C., et al. (2015). Functional identity and diversity of animals predict ecosystem functioning better than species-based indices. Proc. R. Soc. B 282:20142620. doi: 10.1098/rspb.2014.2620
Gámez-Virués, S., Perović, D. J., Gossner, M. M., Börschig, C., Blüthgen, N., de Jong, H., et al. (2015). Landscape simplification filters species traits and drives biotic homogenization. Nat. Commun. 6:8568. doi: 10.1038/ncomms9568
Gossner, M. M., Lachat, T., Brunet, J., Isacsson, G., Bouget, C., Brustel, H., et al. (2013). Current near-to-nature forest management effects on functional trait composition of saproxylic beetles in beech forests. Conserv. Biol. 27, 605–614. doi: 10.1111/cobi.12023
Greenop, A., Woodcock, B. A., Wilby, A., Cook, S. M., and Pywell, R. F. (2018). Functional diversity positively affects prey suppression by invertebrate predators: a meta-analysis. Ecology 99, 1771–1782. doi: 10.1002/ecy.2378
Guariento, E., Strutzenberger, P., Truxa, C., and Fiedler, K. (2020). The trinity of ecological contrasts: a case study on rich insect assemblages by means of species, functional and phylogenetic diversity measures. BMC Ecol. 20:29. doi: 10.1186/s12898-020-00298-3
Habel, J. C., Samways, M. J., and Schmitt, T. (2019a). Mitigating the precipitous decline of terrestrial european insects: requirements for a new strategy. Biodivers. Conserv. 28, 1343–1360. doi: 10.1007/s10531-019-01741-8
Habel, J. C., Ulrich, W., Biburger, N., Seibold, S., and Schmitt, T. (2019b). Agricultural intensification drives butterfly decline. Insect Conserv. Divers. 12, 289–295. doi: 10.1111/icad.12343
Hass, A. L., Kormann, U. G., Tscharntke, T., Clough, Y., Bosem Baillod, A., Sirami, C., et al. (2018). Landscape configurational heterogeneity by small-scale agriculture, not crop diversity, maintains pollinators and plant reproduction in western Europe. Proc. R. Soc. B 285:20172242. doi: 10.1098/rspb.2017.2242
Isbell, F., Cowles, J., Dee, L. E., Loreau, M., Reich, P. B., Gonzalez, A., et al. (2018). Quantifying effects of biodiversity on ecosystem functioning across times and places. Ecol. Lett. 21, 763–778. doi: 10.1111/ele.12928
Jonason, D., Franzén, M., and Ranius, T. (2014). Surveying moths using light traps: effects of weather and time of year. PLoS One 9:e92453. doi: 10.1371/journal.pone.0092453
Knuff, A. K., Staab, M., Frey, J., Dormann, C. F., Asbeck, T., and Klein, A. M. (2020). Insect abundance in managed forests benefits from multi-layered vegetation. Basic Appl. Ecol. 48, 124–135. doi: 10.1016/j.baae.2020.09.002
Kovács, B., Tinya, F., and Ódor, P. (2017). Stand structural drivers of microclimate in mature temperate mixed forests. Agric. For. Meteorol. 234–235, 11–21. doi: 10.1016/j.agrformet.2016.11.268
Laliberté, E., and Legendre, P. (2010). A distance-based framework for measuring functional diversity from multiple traits. Ecology 91, 299–305. doi: 10.1890/08-2244.1
Laliberté, E., Wells, J. A., DeClerck, F., Metcalfe, D. J., Catterall, C. P., Queiroz, C., et al. (2010). Land-use intensification reduces functional redundancy and response diversity in plant communities. Ecol. Lett. 13, 76–86. doi: 10.1111/j.1461-0248.2009.01403.x
Laurance, W. F., Nascimento, H. E., Laurance, S. G., Andrade, A., Ewers, R. M., Harms, K. E., et al. (2007). Habitat fragmentation, variable edge effects, and the landscape-divergence hypothesis. PLoS One 2:e1017. doi: 10.1371/journal.pone.0001017
Leather, S. R. (2018). ‘Ecological Armageddon’ – More evidence for the drastic decline in insect numbers. Ann. Appl. Biol. 172, 1–3. doi: 10.1111/aab.12410
Legras, G., and Gaertner, J. (2018). Assessing functional evenness with the FEve index: a word of warning. Ecol. Indic. 90, 257–260. doi: 10.1016/j.ecolind.2018.03.020
Lewis, R. J., Pakeman, R. J., Angus, S., and Marrs, R. H. (2014). Using compositional and functional indicators for biodiversity conservation monitoring of semi-natural grasslands in Scotland. Biol. Conserv. 175, 82–93. doi: 10.1016/j.biocon.2014.04.018
Liu, J., Wilson, M., Hu, G., Liu, J., Wu, J., and Yu, M. (2018). How does habitat fragmentation affect the biodiversity and ecosystem functioning relationship. Landsc. Ecol. 33, 314–352. doi: 10.1007/s10980-018-0620-5
Macior, L. W. (1971). Co-evolution of plants and animals – systematic insights from plant-insect interactions. Taxon 20, 17–28. doi: 10.2307/1218530
Malfitano, A. (2002). Alle origini della politica di tutela ambientale in Italia. Luigi Rava e la nuova pineta ‘storica’ di Ravenna. Storia Futuro 1, 1–18.
Mangels, J., Fiedler, K., Schneider, F. D., and Blüthgen, N. (2017). Diversity and trait composition of moths respond to land-use intensification in grasslands: generalists replace specialists. Biodivers. Conserv. 26, 3385–3405. doi: 10.1007/s10531-017-1411-z
Mason, N. W. H., and De Bello, F. (2013). Functional diversity: a tool for answering challenging ecological questions. J. Veg. Sci. 24, 777–780.
Mason, N. W. H., Mouillot, D., Lee, W. G., and Wilson, J. B. (2005). Functional richness, functional evenness and functional divergence: the primary components of functional diversity. Oikos 111, 112–118. doi: 10.1111/j.0030-1299.2005.13886.x
Merckx, T., Dantas de Miranda, M., and Pereira, H. M. (2019). Habitat amount, not patch size and isolation, drives species richness of macro-moth communities in countryside landscapes. J. Biogeogr. 46, 956–967. doi: 10.1111/jbi.13544
Merckx, T., Kaiser, A., and Van Dyck, H. (2018). Increased body size along urbanization gradients at both community and intraspecific level in macro-moths. Glob. Change Biol. 24, 3837–3848. doi: 10.1111/gcb.14151
Merckx, T., Feber, R. E., Hoare, D. J., Parsons, M. S., Kelly, C. J., Bourn, N. A., et al. (2012a). Conserving threatened Lepidoptera: towards an effective woodland management policy in landscapes under intense human land-use. Biol. Conserv. 149, 32–39. doi: 10.1016/j.biocon.2012.02.005
Merckx, T., Marini, L., Feber, R. E., and Macdonald, D. W. (2012b). Hedgerow trees and extended-width field margins enhance macro-moth diversity: implications for management. J. Appl. Ecol. 49, 1396–1404. doi: 10.1111/j.1365-2664.2012.02211.x
Merckx, T., and Van Dyck, H. (2019). Urbanization-driven homogenization is more pronounced and happens at wider spatial scales in nocturnal and mobile flying insects. Glob. Ecol. Biogeogr. 28, 1440–1455. doi: 10.1111/geb.12969
Mitchell, K. (2010). Quantitative Analysis by the Point-Centered Quarter Method. Available online at: https://arxiv.org/pdf/1010.3303.pdf (accessed September 17, 2020).
Mori, A. S., Furukawa, T., and Sasaki, T. (2013). Response diversity determines the resilience of ecosystems to environmental change. Biol. Rev. 88, 349–364. doi: 10.1111/brv.12004
Mouchet, M. A., Villéger, S., Mason, N. W. H., and Mouillot, D. (2010). Functional diversity measures: an overview of their redundancy and their ability to discriminate community assembly rules. Funct. Ecol. 24, 867–876.
Newbold, T., Hudson, L. N., Hill, S. L. L., Contu, S., Lysenko, I., Senior, R. A., et al. (2015). Global effects of land use on local terrestrial biodiversity. Nature 520, 45–50. doi: 10.1038/nature14324
Niu, K., He, J. S., Zhang, S., and Lechowicz, M. J. (2016). Grazing increases functional richness but not functional divergence in tibetan alpine meadow plant communities. Biodivers. Conserv. 25, 2441–2452. doi: 10.1007/s10531-015-0960-2
Oksanen, J., Blanchet, G., Friendly, M., Kindt, R., Legendre, P., McGlinn, D., et al. (2018). Vegan: Community Ecology Package. R Package Version 2.5 - 2.
Oliver, T. H., Heard, M. S., Isaac, N. J. B., Roy, D. B., Procter, D., Eigenbrod, F., et al. (2015). Biodiversity and resilience of ecosystem functions. Trends Ecol. Evol. 30, 673–684. doi: 10.1016/j.tree.2015.08.009
Pérez-Ramos, I. M., Roumet, C., Cruz, P., Blanchard, A., Autran, P., and Garnier, E. (2012). Evidence for a ‘plant community economics spectrum’ driven by nutrient and water limitations in a mediterranean rangeland of southern France. J. Ecol. 100, 1315–1327. doi: 10.1111/1365-2745.12000
Perović, D., Gámez-Virués, S., Börschig, C., Klein, A., Krauss, J., Steckel, J., et al. (2015). Configurational landscape heterogeneity shapes functional community composition of grassland butterflies. J. Appl. Ecol. 52, 505–513. doi: 10.1111/1365-2664.12394
Piano, E., Souffreau, C., Merckx, T., Baardsen, L. F., Backeljau, T., Bonte, D., et al. (2020). Urbanization drives cross-taxon declines in abundance and diversity at multiple spatial scales. Glob. Change Biol. 26, 1196–1211. doi: 10.1111/gcb.14934
Pinheiro, J., Bates, D., Debroy, S., Sarkar, D., and R Core Team (2018). nlme: Linear and Nonlinear Mixed Effects Models. R Package Version 3.1-137.
QGISDevelopmentTeam (2018). QGIS Geographic Information System. Open Source Geospatial Foundation Project. Available online at: http://qgis.osgeo.org (accessed July 10, 2020).
R Core Team (2018). R: A Language and Environment for Statistical Computing. Vienna: R foundation for statistical computing.
Root, H. T., Verschuyl, J., Stokely, T., Hammond, P., Scherr, M. A., and Betts, M. G. (2017). Plant diversity enhances moth diversity in an intensive forest management experiment. Ecol. Appl. 27, 134–142. doi: 10.1002/eap.1426
Saunders, M. E. (2019). No simple answers for insect conservation: media hype has missed the biggest concern that ecologists and entomologists have about six-legged life: how little we know about it. Am. Sci. 107, 148–152.
Schmidt, M., Jochheim, H., Kersebaum, K., Lischeid, G., and Nendel, K. (2017). Gradients of microclimate, carbon and nitrogen in transition zones of fragmented landscapes – a review. Agric. For. Meteorol. 232, 659–671. doi: 10.1016/j.agrformet.2016.10.022
Schowalter, T. (2017). Arthropod diversity and functional importance in old-growth forests of North America. Forests 8:97. doi: 10.3390/f8040097
Seibold, S., Gossner, M. M., Simons, N. K., Blüthgen, N., Müller, J., Ambarli, D., et al. (2019). Arthropod decline in grasslands and forests is associated with landscape-level drivers. Nature 574, 671–674. doi: 10.1038/s41586-019-1684-3
Slade, E. M., Merckx, T., Riutta, T., Bebber, D. P., Redhead, D., Riordan, P., et al. (2013). Life-history traits and landscape characteristics predict macro-moth responses to forest fragmentation. Ecology 94, 1519–1530. doi: 10.1890/12-1366.1
Suding, K. N., Lavorel, S., Chapin, F. S., Cornelissen, J. H. C., Díaz, S., Garnier, E., et al. (2008). Scaling environmental change through the community-level: a trait-based response-and-effect framework for plants. Glob. Change Biol. 14, 1125–1140. doi: 10.1111/j.1365-2486.2008.01557.x
Summerville, K., and Crist, T. (2003). Determinants of lepidopteran community composition and species diversity in eastern deciduous forests: roles of season, eco-region and patch size. Oikos 100, 134–148. doi: 10.1034/j.1600-0706.2003.11992.x
Thorn, S., Hacker, H. H., Seibold, S., Jehl, H., Bässler, C., and Müller, J. (2015). Guild-specific responses of forest Lepidoptera highlight conservation-oriented forest management – implications from conifer-dominated forests. For. Ecol. Manag. 337, 41–47. doi: 10.1016/j.foreco.2014.10.031
Tscharntke, T., Tylianakis, J. M., Rand, T. A., Didham, R. K., Fahrig, L., Batáry, P., et al. (2012). Landscape moderation of biodiversity patterns and processes – eight hypotheses. Biol. Rev. Camb. Philos. Soc. 87, 661–685. doi: 10.1111/j.1469-185X.2011.00216.x
Uhl, B. (2020). How Much are Isolated Relict Forest Reserves Influenced by Local and External Factors? A Case Study on Vegetation and Moths in Northern Italy. Vienna: University of Vienna. Ph. D. dissertation.
Uhl, B., Wölfling, M., Fiala, B., and Fiedler, K. (2016). Micro-moth communities mirror environmental stress gradients within a mediterranean nature reserve. Basic Appl. Ecol. 17, 273–281. doi: 10.1016/j.baae.2015.10.002
Uhl, B., Wölfling, M., and Fiedler, K. (2020a). Local, forest stand and landscape-scale correlates of plant communities in isolated coastal forest reserves. Plant Biosyst. 155, 1–22. doi: 10.1080/11263504.2020.1762776
Uhl, B., Wölfling, M., and Fiedler, K. (2020b). Understanding small-scale insect diversity patterns inside two nature reserves – the role of local and landscape factors. Biodivers. Conserv. 29, 2399–2418. doi: 10.1007/s10531-020-01981-z
Venables, W. N., and Ripley, B. D. (2002). Modern Applied Statistics with S. Fourth Edition. New York, NY: Springer.
Villéger, S., Mason, N. W. H., and Mouillot, D. (2008). New multidimensional functional diversity indices for a multifaceted framework in functional ecology. Ecology 89, 2290–2301. doi: 10.1890/07-1206.1
Von Arx, G., Dobbertin, M., and Rebetez, M. (2012). Spatio-temporal effects of forest canopy on understory microclimate in a long-term experiment in Switzerland. Agric. For. Meteorol. 16, 144–155. doi: 10.1016/j.agrformet.2012.07.018
Warton, D. I., and Hui, F. K. C. (2011). The arcsine is asinine: the analysis of proportions in ecology. Ecology 92, 3–10. doi: 10.1890/10-0340.1
Wölfling, M., Becker, M. C., Uhl, B., Traub, A., and Fiedler, K. (2016). How differences in the settling behaviour of moths (Lepidoptera) may contribute to sampling bias when using automated light traps. Eur. J. Entomol. 113:502. doi: 10.14411/eje.2016.066
Wölfling, M., Uhl, B., and Fiedler, K. (2019). Multi-decadal surveys in a Mediterranean forest reserve – do succession and isolation drive moth species richness? Nat. Conserv. 35, 25–40. doi: 10.3897/natureconservation.35.32934
Woodcock, B. A., Garratt, M. P. D., Powney, G. D., Shaw, R. F., Osborne, J. L., Soroka, J., et al. (2019). Meta-analysis reveals that pollinator functional diversity and abundance enhance crop pollination and yield. Nat. Commun. 10:1481. doi: 10.1038/s41467-019-09393-6
Woodcock, B. A., Harrower, C., Redhead, J., Edwards, M., Vanbergen, A. J., Heard, M. S., et al. (2014). National patterns of functional diversity and redundancy in predatory ground beetles and bees associated with key UK arable crops. J. Appl. Ecol. 51, 142–115. doi: 10.1111/1365-2664.12171
Keywords: landscape diversity, local factors, functional redundancy, Lepidoptera, functional richness, isolated nature reserves, mediterranean
Citation: Uhl B, Wölfling M and Fiedler K (2021) Qualitative and Quantitative Loss of Habitat at Different Spatial Scales Affects Functional Moth Diversity. Front. Ecol. Evol. 9:637371. doi: 10.3389/fevo.2021.637371
Received: 03 December 2020; Accepted: 07 April 2021;
Published: 30 April 2021.
Edited by:
Orsolya Valkó, Centre for Ecological Research, Hungarian Academy of Science, HungaryReviewed by:
Miguel Alfonso Ortega-Huerta, National Autonomous University of Mexico, MexicoMohammad Imam Hasan Reza, Independent researcher, Chittagong, Bangladesh
Copyright © 2021 Uhl, Wölfling and Fiedler. This is an open-access article distributed under the terms of the Creative Commons Attribution License (CC BY). The use, distribution or reproduction in other forums is permitted, provided the original author(s) and the copyright owner(s) are credited and that the original publication in this journal is cited, in accordance with accepted academic practice. No use, distribution or reproduction is permitted which does not comply with these terms.
*Correspondence: Konrad Fiedler, a29ucmFkLmZpZWRsZXJAdW5pdmllLmFjLmF0