High-Quality Draft Genome Sequence of Pantanalinema sp. GBBB05, a Cyanobacterium From Cerrado Biome
- 1Department of Biological Oceanography, Oceanographic Institute, University of São Paulo, São Paulo, Brazil
- 2Graduate Program in Health and Environment, Federal University of Maranhão, São Luís, Brazil
- 3Graduate Program in Oceanography, Federal University of Maranhão, São Luís, Brazil
- 4Graduate Program in Health Sciences, Federal University of Maranhão, São Luís, Brazil
- 5Department of Pathology, Center for Biological and Health Sciences, Federal University of Maranhão, São Luís, Brazil
- 6Laboratório de Ciclo Celular, Butantan Institute, São Paulo, Brazil
- 7Graduate Program in Biotechnology, Federal University of Pará, Belém, Brazil
- 8Department of Microbiology, Faculty of Agriculture and Forestry, University of Helsinki, Helsinki, Finland
- 9Department of Biochemistry, Institute of Chemistry, University of São Paulo, São Paulo, Brazil
- 10Department of Biology, Center for Biological and Health Sciences, Federal University of Maranhão, São Luís, Brazil
Introduction
Cyanobacteria comprise one of the oldest and most diverse phyla in the Bacteria domain and are recognized for their importance in the biosphere evolution. Members of this phylum can be found in a wide variety of environments reflecting their photosynthetic ability, adaptability to various environmental conditions, and diversified metabolism. Such characteristics make cyanobacteria one of the preferred targets for research on bioactive compounds and new enzymes (Schirrmeister et al., 2011; Dittmann et al., 2015).
Pantanalinema was described as a new genus of the Leptolyngbyaceae cyanobacterial family by a polyphasic approach, which included morphological characteristics, 16S rRNA gene phylogeny, 16S-23S ITS rRNA secondary structures, and physiological characteristics such as adaptability to pH variations (Vaz et al., 2015).
This genus has been described only in Brazilian biomes such as the Pantanal and the Amazon, the first isolates being found in a lake. These Pantanalinema isolates were characterized by their ability to grow over a wide pH range (pH 4 to 11) as well as to modify the culture medium pH around neutrality (pH 6 to 7.4). Due to these characteristics, it is thought that this genus can occupy a variety of ecological niches, such as alkaline or slightly acidic water bodies (Vaz et al., 2015; Genuário et al., 2017). Taxonomic classification of Pantanalinema isolates requires the use of molecular markers as this genus is morphologically very similar to the recently described genus Amazoninema, which, in turn, has comparable morphology to other genera of the Leptolyngbyaceae family (Genuário et al., 2018).
In this work, we report the genome sequence of a new Pantanalinema strain, named GBBB05, which was isolated from the Brazilian Cerrado biome. This is the first genome assembly for the Pantanalinema genus, which, along with the analyses provided here, is expected to enhance our understanding of this genus's metabolic potential.
Value of Data
Pantanalinema is a recently described new genus of cyanobacteria. Here we describe the first genome of a strain in this genus. The high-quality draft genome assembled from an environmental culture shows the feasibility of this method, especially for cyanobacteria samples that are underrepresented in metagenomic samples and difficult to obtain from axenic cultures. The reported genome will allow further understanding of this species' biology.
Results
Total DNA isolated from a non-axenic unialgal culture of strain GBBB05 was sequenced using the MiSeq-Illumina platform. The sequencing generated 8,728,802 reads, and after quality control, de novo assembly and binning of 5,745,014 quality-filtered PE reads allowed the recovery of eight bacterial genomes with completeness varying from 50 to 99.5% (Supplementary Table 1). With the initial taxonomic placement using GTDB-Tk (Chaumeil et al., 2020) and the module “classify_bins” from Metawrap (Uritskiy et al., 2018) (Supplementary Table 2), one of the recovered genomes (GBBB05) is from cyanobacteria (Leptolyngbyaceae family) while the other seven are from heterotrophic bacteria.
The cyanobacterial genome of the GBBB05 strain was assembled into 94 contigs and has an estimated size of 7,181,771 bp and a GC content of 48.43% (Table 1). The genome showed high completeness (99.05%) and low contamination (0.4%). Selected features of the GBBB05 genome are presented in Table 1 and Supplementary Table 1.
Based on the morphology shown in the culture, the GBBB05 strain was assigned to either Pantanalinema or Amazoninema genera for which there are no genome sequences currently available. In silico DDH values with reference genomes from the Leptolyngbyaceae family ranged from 18.4 to 22.8% (Supplementary Table 5). Nevertheless, phylogenomic analysis grouped GBBB05 with one representative genome of the genus Leptolyngbya with 100% bootstrap support (Figure 1A). Phylogenetic reconstruction using Bayesian inference of partial 16S rRNA sequences from Leptolyngbyaceae shows that GBBB05 belongs to the Pantanalinema genus (Figure 1C).
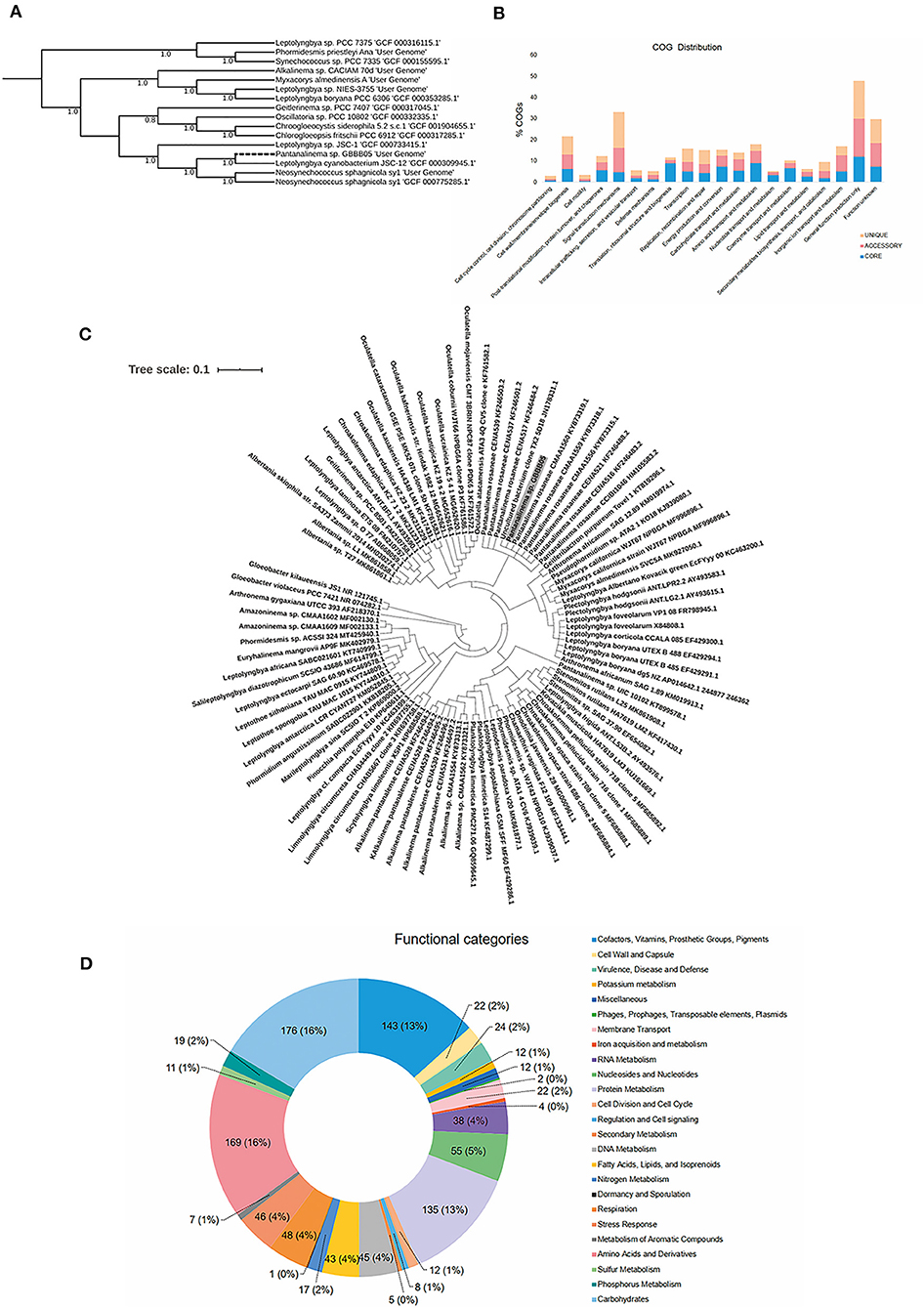
Figure 1. (A) An approximately maximum-likelihood phylogenetic tree using a set of 49 core universal genes defined by COG (Clusters of Orthologous Groups) gene families on Phylogenetic Species Tree App based on closely related genomes selected from the public KBase genomes imported from NCBI-RefSeq. The Jukes-Cantor model of nucleotide evolution was used with 1,000 resamples. Pantanalinema GBBB05 is highlighted with a black edge. The numbers beside each node indicate the percentage bootstrap values. Default parameters are used by Species Tree App. (B) COG distribution of core, accessory, and unique genes in all four analyzed genomes generated by Bacterial Pan Genome Analysis Tool (BPGA). (C) Pantanalinema GBBB05 Bayesian tree reconstructed using MrBayes based on partial 16S rRNA sequence (alignment of 1,179 bp) with Gloeobacter as the outgroup. Sequences of other Leptolyngbyaceae strains obtained from GenBank are represented with their respective accession numbers. Pantanalinema GBBB05 strain is highlighted in gray background. (D) Functional categories identified in Pantanalinema GBBB05 genome by RAST server. Total number of genes related to each category and its respective percentage in brackets.
Pan-genome analysis revealed that the Pantanalinema sp. GBBB05 shares a set of 1,624 core genes (~28% CDSs) with the reference strains Leptolyngbya PCC 6306, Leptolyngbya JSC 12, and Geitlerinema PCC 7407 (Figure 1B and Supplementary Table 6).
The functional prediction performed by the RAST annotation found about 25 functional categories (Figure 1D). Among all the categories, “Carbohydrates” with 176, “Amino Acids and Derivatives” with 169, “Cofactors, Vitamins, Prosthetic Groups, Pigments” with 143, and “Protein metabolism” with 135 genes were the biggest group. In the category “Secondary metabolites,” five genes related to plant alkaloids and plant hormones (auxins) were identified. Six genes related to metal (cadmium, cobalt, mercury, and zinc) resistance and two to fluoroquinolones were found.
Analyses using antiSMASH resulted in the prediction of 17 biosynthetic gene clusters (BGCs), which included the following: 5 clusters for terpene production; 4 bacteriocin clusters; 1 cluster for betalactone biosynthesis; 1 cluster for resorcinol production; 1 mixed module of polyketide synthase (PKS) type 1 and non-ribosomal peptide synthetase (NRPS) related to nostophycin; and 6 clusters containing NRPS biosynthetic pathways (Supplementary Table 7).
Analysis in the NaPDoS server, using the default setting, predicted 11 CDSs related to metabolite production in domain C pathways and 4 CDSs related to metabolite production in KS domain pathways (Supplementary Tables 8, 9).
The PRISM4 analysis identified seven gene clusters: five non-ribosomal peptide, one mixed module of polyketide synthase (PKS) type 1 and non-ribosomal peptide synthetase (NRPS), and one prochlorosin cluster (Supplementary Table 10).
Materials and Methods
Growth Conditions and Genomic DNA Isolation
The strain GBBB05 was isolated from a waterfall in the outside border of the Chapada das Mesas National Park, Carolina County, Maranhão State, Brazil (S07°02.6575 / W047°30.4508). Aerobic cultivation was performed in the BG-11 medium (Stanier and Cohen-Bazire, 1977) under the illumination of 3–15 μmol photons m−2 s−1 for 4 weeks at 28°C. Non-axenic unialgal culture was obtained by the spread plate method and serial dilutions, and each step was monitored with an optical microscope. Total DNA was extracted from 25 ml of stationary phase non-axenic unialgal culture using the PowerPlant kit (MoBio, California, USA) according to the manufacturer's instructions and stored at −20°C. DNA purity and concentration were evaluated by the absorbance at 260 and 280 nm on a NanoDrop ND-2000 Spectrophotometer (Thermo Fisher Scientific, Waltham, MA, USA). DNA concentration was further quantified with a Quant-iT Picogreen dsDNA assay kit (Thermo Fisher Scientific, Waltham, MA, USA).
Genome Sequencing, Assembly, and Taxonomic Classification
The sequencing library was prepared with 30 ng of total DNA using the Illumina Nextera DNA library preparation kit (Illumina, Inc.) and sequenced with MiSeq-Illumina platform (Illumina, Inc., San Diego, USA) using the MiSeq Reagent kit v2 (500-cycle format). Paired-end (PE) reads were quality-filtered and trimmed (PHRED quality score ≥ 30) with Read_qc module from Metawrap (Uritskiy et al., 2018). De novo assembly was performed with the Metawrap Assembly module using metaSPAdes v. 3. 13 (Nurk et al., 2017). Assembled contigs were subjected to three different binning rounds using CONCOCT (Alneberg et al., 2014), MaxBin2 (Wu et al., 2016), and MetaBAT2 (Kang et al., 2019). The results were compared using the Bin_refinement module, and the highest-quality bins were selected. The genome statistics were obtained through CheckM (Parks et al., 2015). Digital DNA–DNA hybridization (DDH) values were calculated using the Genome-To-Genome Distance Calculator (GGDC) server (Meier-Kolthoff et al., 2013, 2014). GTDB-TK (Chaumeil et al., 2020) and Classify_bins from metaWRAP (Uritskiy et al., 2018) were used to do the genomic classification (Supplementary Table 1). All parameters were kept at default values.
Phylogenetic Analysis
A phylogenomic tree of strain GBBB05 closely related genomes was constructed using the Species Tree App present in KBase (Arkin et al., 2018). Further phylogenetic analysis was performed using the GBBB05 16S rRNA partial sequence retrieved from its genome assembly plus 89 partial 16S rRNA sequences from various strains of the Leptolyngbyaceae family and Gloeobacter genus, retrieved from the NCBI. The nucleotide substitution model used was SYM+G, determined by PAUP 4.0b101 and MrModeltest 2 (Nylander, 2004). The tree was constructed using MrBayes 3.2.6 (Ronquist et al., 2012), running with 107 generations, sampling every 100th iteration. TRACER 1.6 (Rambaut et al., 2018) was used to check the performance of tree construction with all parameters at default settings.
Genome Annotation and Functional Analyses
The genome assembly was submitted to the Prokaryotic Genome Annotation Pipeline (PGAP) (Tatusova et al., 2016) at the National Center for Biotechnology Information (NCBI). The CRISPRCasFinder server (Couvin et al., 2018) was used to predict CRISPR sequences and CAS genes, and the PHAST web server tool (Zhou et al., 2011) was used to identify the phage-related sequences (Table 1). Pan-genome analysis was performed using the Bacterial Pan Genome Analysis Tool (BPGA) (Chaudhari et al., 2016), with default parameters. To predict gene clusters related to secondary metabolite production, the online servers AntiSMASH 5.0 (Blin et al., 2019) and PRISM4 (Skinnider et al., 2017) were used with all their options enabled. For the detection of C domains and KS domains, the NaPDoS online server pipeline was used with its default configuration (Ziemert et al., 2012). Prediction of functional categories was performed using RAST server annotation at default settings (Aziz et al., 2008; Overbeek et al., 2014).
Data Availability Statement
The datasets presented in this study can be found in online repositories. The names of the repository/repositories and accession number(s) can be found at: NCBI [accession: PRJNA560270].
Author Contributions
HD, AS, JS, KS, DF, and LD conceived and supervised this study. RS, MO, and PM collected and established the enriched cultures of Pantanalinema. LF, AB, IR, PS, RR, CM, and AL performed sequencing and bioinformatic analysis. LF, AB, HD, AS, JS, EG, DF, and LD wrote and revised the manuscript. All authors read and approved the final manuscript.
Funding
This work was supported by Conselho Nacional de Desenvolvimento Científico e Tecnológico (CNPq) [Grant number 421085/2016-9], Fundação de Amparo à Pesquisa e ao Desenvolvimento Científico e Tecnológico do Maranhão (FAPEMA) [Grant numbers UNIVERSAL-00845/16, COOPI-07813/17, STGI-08150/17, ESTAGIO-NACIONAL-05333/18], and Coordenação de Aperfeiçoamento de Pessoal de Nível Superior (CAPES) [Grant number CAPES FINANCE CODE 001]. RS and RR were supported with fellowship from CAPES. AB and MO received fellowships from FAPEMA.
Conflict of Interest
The authors declare that the research was conducted in the absence of any commercial or financial relationships that could be construed as a potential conflict of interest.
Acknowledgments
LF, IR, AS, and JS acknowledge the fellowship support received from CNPq. LD acknowledges all the support received from the Marie Curie Alumni Association and the authors thank Dr. Layla F. Martins from the Center for Advanced Technologies in Genomics, Instituto de Química/USP, for technical assistance on Illumina sequencing.
Supplementary Material
The Supplementary Material for this article can be found online at: https://www.frontiersin.org/articles/10.3389/fevo.2021.639852/full#supplementary-material
Footnotes
1. ^Available at: http://phylosolutions.com/paup-test/.
References
Alneberg, J., Bjarnason, B. S., de Bruijn, I., Schirmer, M., Quick, J., Ijaz, U. Z., et al. (2014). Binning metagenomic contigs by coverage and composition. Nat. Methods 11, 1144–1146. doi: 10.1038/nmeth.3103
Arkin, A. P., Cottingham, R. W., Henry, C. S., Harris, N. L., Stevens, R. L., Maslov, S., et al. (2018). KBase: the United States department of energy systems biology knowledgebase. Nat. Biotechnol. 36, 566–569. doi: 10.1038/nbt.4163
Aziz, R. K., Bartels, D., Best, A., DeJongh, M., Disz, T., Edwards, R. A., et al. (2008). The RAST Server: rapid annotations using subsystems technology. BMC Genomics 9:75. doi: 10.1186/1471-2164-9-75
Blin, K., Shaw, S., Steinke, K., Villebro, R., Ziemert, N., Lee, S. Y., et al. (2019). AntiSMASH 5.0: updates to the secondary metabolite genome mining pipeline. Nucleic Acids Res. 47, W81–W87. doi: 10.1093/nar/gkz310
Chaudhari, N. M., Gupta, V. K., and Dutta, C. (2016). BPGA-an ultra-fast pan-genome analysis pipeline. Sci. Rep. 6, 1–10. doi: 10.1038/srep24373
Chaumeil, P. A., Mussig, A. J., Hugenholtz, P., and Parks, D. H. (2020). GTDB-Tk: a toolkit to classify genomes with the genome taxonomy database. Bioinformatics 36, 1925–1927. doi: 10.1093/bioinformatics/btz848
Couvin, D., Bernheim, A., Toffano-Nioche, C., Touchon, M., Michalik, J., Néron, B., et al. (2018). CRISPRCasFinder, an update of CRISRFinder, includes a portable version, enhanced performance and integrates search for Cas proteins. Nucleic Acids Res. 46, W246–W251. doi: 10.1093/nar/gky425
Dittmann, E., Gugger, M., Sivonen, K., and Fewer, D. P. (2015). Natural product biosynthetic diversity and comparative genomics of the cyanobacteria. Trends Microbiol. 23, 642–652. doi: 10.1016/j.tim.2015.07.008
Genuário, D. B., De Souza, W. R., Monteiro, R. T. R., Sant'Anna, C. L., and Melo, I. S. (2018). Amazoninema gen. Nov., (Synechococcales, Pseudanabaenaceae) a novel cyanobacteria genus from Brazilian Amazonian rivers. Int. J. Syst. Evol. Microbiol. 68, 2249–2257. doi: 10.1099/ijsem.0.002821
Genuário, D. B., Vaz, M. G. M. V., and Melo, I. S. de (2017). Phylogenetic insights into the diversity of homocytous cyanobacteria from Amazonian rivers. Mol. Phylogenet. Evol. 116, 120–135. doi: 10.1016/j.ympev.2017.08.010
Kang, D. D., Li, F., Kirton, E., Thomas, A., Egan, R., An, H., et al. (2019). MetaBAT 2: an adaptive binning algorithm for robust and efficient genome reconstruction from metagenome assemblies. PeerJ 2019, 1–13. doi: 10.7717/peerj.7359
Meier-Kolthoff, J. P., Auch, A. F., Klenk, H. P., and Göker, M. (2013). Genome sequence-based species delimitation with confidence intervals and improved distance functions. BMC Bioinformatics 14:60. doi: 10.1186/1471-2105-14-60
Meier-Kolthoff, J. P., Klenk, H. P., and Göker, M. (2014). Taxonomic use of DNA G+C content and DNA-DNA hybridization in the genomic age. Int. J. Syst. Evol. Microbiol. 64, 352–356. doi: 10.1099/ijs.0.056994-0
Nurk, S., Meleshko, D., Korobeynikov, A., and Pevzner, P. A. (2017). MetaSPAdes: a new versatile metagenomic assembler. Genome Res. 27, 824–834. doi: 10.1101/gr.213959.116
Nylander, J. (2004). MrModeltest V2. Program Distributed by the Author. Uppsala: Uppsala University.
Overbeek, R., Olson, R., Pusch, G. D., Olsen, G. J., Davis, J. J., Disz, T., et al. (2014). The SEED and the Rapid Annotation of microbial genomes using Subsystems Technology (RAST). Nucleic Acids Res. 42, 206–214. doi: 10.1093/nar/gkt1226
Parks, D. H., Imelfort, M., Skennerton, C. T., Hugenholtz, P., and Tyson, G. W. (2015). CheckM: assessing the quality of microbial genomes recovered from isolates, single cells, and metagenomes. Genome Res. 25, 1043–1055. doi: 10.1101/gr.186072.114
Rambaut, A., Drummond, A. J., Xie, D., Baele, G., and Suchard, M. A. (2018). Posterior summarisation in Bayesian phylogenetics using Tracer 1.7. Syst. Biol.syy032. doi: 10.1093/sysbio/syy032
Ronquist, F., Teslenko, M., Van Der Mark, P., Ayres, D. L., Darling, A., Höhna, S., et al. (2012). Mrbayes 3.2: efficient bayesian phylogenetic inference and model choice across a large model space. Syst. Biol. 61, 539–542. doi: 10.1093/sysbio/sys029
Schirrmeister, B. E., Antonelli, A., and Bagheri, H. C. (2011). The origin of multicellularity in cyanobacteria. BMC Evol. Biol. 11:45. doi: 10.1186/1471-2148-11-45
Skinnider, M. A., Merwin, N. J., Johnston, C. W., and Magarvey, N. A. (2017). PRISM 3: Expanded prediction of natural product chemical structures from microbial genomes. Nucleic Acids Res. 45, W49–W54. doi: 10.1093/nar/gkx320
Stanier, R. Y., and Cohen-Bazire, G. (1977). Phototrophic prokaryotes: the cyanobacteria. Ann. Rev. Microbiol. 31, 225–274. doi: 10.1146/annurev.mi.31.100177.001301
Tatusova, T., Dicuccio, M., Badretdin, A., Chetvernin, V., Nawrocki, E. P., Zaslavsky, L., et al. (2016). NCBI prokaryotic genome annotation pipeline. Nucleic Acids Res. 44, 6614–6624. doi: 10.1093/nar/gkw569
Uritskiy, G. V., Diruggiero, J., and Taylor, J. (2018). MetaWRAP - a flexible pipeline for genome-resolved metagenomic data analysis 08 Information and Computing Sciences 0803 Computer Software 08 Information and Computing Sciences 0806 Information Systems. Microbiome 6, 1–13. doi: 10.1186/s40168-018-0541-1
Vaz, M. G. M. V., Genuário, D. B., Andreote, A. P. D., Malone, C. F. S., Sant'Anna, C. L., Barbiero, L., et al. (2015). Pantanalinema gen. nov. and Alkalinema gen. nov.: novel pseudanabaenacean genera (Cyanobacteria) isolated from saline–alkaline lakes. Int. J. Syst. Evol. Microbiol. 65, 298–308. doi: 10.1099/ijs.0.070110-0
Wu, Y. W., Simmons, B. A., and Singer, S. W. (2016). MaxBin 2.0: an automated binning algorithm to recover genomes from multiple metagenomic datasets. Bioinformatics 32, 605–607. doi: 10.1093/bioinformatics/btv638
Zhou, Y., Liang, Y., Lynch, K. H., Dennis, J. J., and Wishart, D. S. (2011). PHAST: a fast phage search tool. Nucleic Acids Res. 39, 347–352. doi: 10.1093/nar/gkr485
Keywords: enriched metagenomics, genome mining, Leptolyngbyaceae, non-axenic, phylogeny
Citation: Ferreira LSdS, Butarelli ACdA, Sousa RdC, Oliveira MAd, Moraes PHG, Ribeiro IS, Sousa PFR, Dall'Agnol HMB, Lima ARJ, Gonçalves EC, Sivonen K, Fewer D, Riyuzo R, Piroupo CM, da Silva AM, Setubal JC and Dall'Agnol LT (2021) High-Quality Draft Genome Sequence of Pantanalinema sp. GBBB05, a Cyanobacterium From Cerrado Biome. Front. Ecol. Evol. 9:639852. doi: 10.3389/fevo.2021.639852
Received: 10 December 2020; Accepted: 05 May 2021;
Published: 17 June 2021.
Edited by:
Sucheta Tripathy, Indian Institute of Chemical Biology (CSIR), IndiaReviewed by:
Shu Cheng, University of Arizona, United StatesLaura Baxter, University of Warwick, United Kingdom
Copyright © 2021 Ferreira, Butarelli, Sousa, Oliveira, Moraes, Ribeiro, Sousa, Dall'Agnol, Lima, Gonçalves, Sivonen, Fewer, Riyuzo, Piroupo, da Silva, Setubal and Dall'Agnol. This is an open-access article distributed under the terms of the Creative Commons Attribution License (CC BY). The use, distribution or reproduction in other forums is permitted, provided the original author(s) and the copyright owner(s) are credited and that the original publication in this journal is cited, in accordance with accepted academic practice. No use, distribution or reproduction is permitted which does not comply with these terms.
*Correspondence: Leonardo Teixeira Dall'Agnol, leonardo.td@ufma.br