- 1Science Unit, Lingnan University, Hong Kong, China
- 2The Hong Kong Bird Watching Society, Hong Kong, China
- 3School of Biological Sciences, The University of Hong Kong, Hong Kong, China
Along the East Asian-Australasian flyway (EAAF), waterbirds are threatened by a wide range of human activities. Studies have shown that wintering populations of many species have declined in Australia and Japan; however, long term data along China’s coast are limited. In this study, we analyzed data collected from monthly bird surveys to quantify population trends of wintering waterbirds from 1998 to 2017 in the Deep Bay area, South China. Of the 42 species studied, 12 declined, while nine increased significantly. Phylogenetic comparative analysis revealed that population trends were negatively correlated to reliance on the Yellow Sea and body size. Further, waterbird species breeding in Southern Siberia declined more than those breeding in East Asia. These findings, coupled with a relatively high number of increasing species, support the continual preservation of wetlands in the Deep Bay area. This study provides another case study showing that data collected from wintering sites provide insights on the threats along migratory pathway and inform conservation actions. As such, we encourage population surveys in the EAAF to continue, particularly along the coast of China.
Introduction
Studying population trends of threatened species to identify drivers of population change is crucial for conservation. This is challenging for mobile species, such as migratory waterbirds (Webster et al., 2002; Piersma et al., 2016). Many waterbird species travel across hemispheres annually, and their populations can be impacted by a wide range of environmental and anthropogenic factors in breeding grounds, stopover sites, and wintering grounds (Amano et al., 2010; Catry et al., 2013; Clemens et al., 2016; Howard et al., 2020). The driving factors can vary greatly in scale, ranging from global climate change to local habitat transformation (Amano et al., 2010; Pavón-Jordán et al., 2015). As such, quantification of population trends at different locations (breeding, stopover, and wintering) can provide useful information to assess migratory waterbird conservation status and evaluate the threats along their migratory pathways (Murray et al., 2017; Wang et al., 2018).
Waterbirds of the East Asian-Australasian flyway (EAAF) have been attracted conservation attention. Habitat transformation along the coast of China, especially in the Yellow Sea area, has caused prominent declines of several species; this is supported by the studies revealing declines of species that rely on Yellow Sea as essential stopover sites at wintering sites in Japan, Australia, and New Zealand (Amano et al., 2010; Murray et al., 2015, 2017; Studds et al., 2017). Besides habitat transformation, waterbirds of the EAAF also experience a wide range of other threats (Wang et al., 2018), including hunting (Gallo-Cajiao et al., 2020), alien invasive species (Gan et al., 2009), pollution (Zhao et al., 2016), and climate change (Iwamura et al., 2013; Wikramanayake et al., 2020).
Previous studies have quantified population trends of migratory waterbirds in China, but the population trends of nearly half (48%) of species were unknown because of the lack of long-term, systematic survey data (Wang et al., 2018; Wetlands International, 2021). Systematic surveys along the coast of China only began in 2005 (Bai et al., 2015). However, systematic waterbird surveys were done in the Deep Bay area, South China since 1998. This represents a valuable dataset to quantify population trends of waterbirds in the EAAF because of two major reasons. First, the area represents one of the most important wintering sites for waterbirds, since the area holds over 20% of EAAF populations for 13 waterbird species (Bai et al., 2015). Second, to our knowledge, the dataset is from the longest (≥20 years) systematic survey of wintering waterbird populations along the coast of China.
The quantification of waterbird population trends in the Deep Bay makes it possible for us to evaluate the impacts of different threats along the EAAF. This has been done through comparative trait-based analysis to assess the influence of species traits on population trends of EAAF waterbirds in Japan (Amano et al., 2010), Australia, and New Zealand (Murray et al., 2017). In the present study, we applied a similar approach on the wintering population in the Deep Bay area, providing the first case study along the coast of China. We take a three-step approach. First, we quantified the population trends of 42 wintering waterbird species in the Deep Bay area. Second, we used remote sensing to quantify the extent of habitat transformation, providing baseline information to evaluate the influence of local habitat changes on population trends. Lastly, we performed a comparative trait-based analysis to identify species traits associated with local and regional threats that correlate with population trends among species. From this work, we provide insight on the threats at different geographical scales and provide recommendations on the conservation of threatened waterbirds along the EAAF.
Materials and Methods
Bird Survey
The Hong Kong Bird Watching Society started to conduct surveys on wintering waterbirds in the Deep Bay area in 1979, providing data to the international counts organized by the International Waterfowl Research Bureau and Asian Wetland Bureau (Melville, 1980; Carey, 1994). Since 1998, the Hong Kong Government began to fund the monitoring of waterbirds—systematic, monthly surveys have been carried out in the Deep Bay area, which encompasses two regions: (1) the northwest New Territories, Hong Kong Special Administrative Region China, and (2) Futian Mangrove National Nature Reserve, Shenzhen, Guangdong Province, China. The study area contains a mixture of different wetland types, including commercial aquaculture ponds, mangroves, and intertidal mudflats (more details in Pang et al., 2020). Synchronized surveys were conducted along 16 fixed, non-overlapping transects distributed across the study areas by trained surveyors. Among these 16 transects, 15 are located in Hong Kong while one are in Shenzhen, China. The surveyor recorded the abundance of all waterbird species, using binoculars and/or telescopes. To maintain survey consistency, all surveyors had over 3 years of birdwatching experience and received training provided by the Hong Kong Bird Watching Society. The training consisted of two parts, including lectures and practical sessions. The lectures provided candidate surveyors information on the project background, survey methods, and safety issues. For the practical sessions, all candidate surveyors were required to join at least three surveys led by a trained surveyors. For the time of survey, all surveys were done during high tide, when the intertidal mudflat was largely submerged, and waterbirds visited high-tide roosting areas.
Habitat Changes
To quantify the extent of habitat transformation in the Deep Bay area from late 1990s to late 2010s, we used Système Pour l’Observation de la Terre (SPOT) images and open data. SPOT 4 and SPOT 5 images taken, respectively in 2000 and 2008 during dry season (October to February) of Hong Kong were selected to eliminate cloud contamination. SPOT images were employed due to their high resolution (6–10 m), allowing for visualization of greater detail in the land cover and more precise land use classification. All remote sensing images were processed through geometric correction, false color composition, image mosaic, and supervised classification using the ArcGIS 10.5. Over 1,000 training samples were obtained from different sources to improve the image classification accuracy. For 2018, the habitat/land use data were obtained from WWF open data1 and supplemented by Google Earth high spatial resolution images. Land use was classified into 15 habitats according to the methodology stated in the Terrestrial Habitat Mapping proposed by the Sustainable Development Unit, Hong Kong Government2. The 15 habitat types was further categorized into five habitats, including aquaculture ponds, human settlement, intertidal mudflat, mangrove, and other vegetation.
Population Trends
We included data collected from 3 months (December, January, and February), when migratory waterbirds complete their southward migration and have not started northward migration (Clemens et al., 2016). In total, 60 surveys were included in the analyses (3 months × 20 years). To avoid spurious results caused by rare and infrequent species, we selected species with at least 10 individuals recorded in 10 surveys. To quantify wintering bird population trends, we used generalized additive mixed models (GAMM) to determine non-linear population trends. We quantified the trends for three focal periods, including the entire study period (1998–2017) and two 10-year periods (1998–2007 and 2008–2017) because we aimed to understand the population trends over the entire study period and if the trends changed during the study period. We included the number of birds as a dependent factor and year (e.g., December 1998, January 1999, and February 1999 regarded as the winter of 1998) as an independent factor. To account for variation between sites, we included transect as a random factor. We used the package poptrend in software R to fit a log-linear model with quasipoisson or negative binomial distribution using restricted maximum likelihood (Knape, 2016; R Core Team, 2019). We chose the data distribution based on examination of model residuals and explained deviance (Bell et al., 2020). We used the function change of R package poptrend to estimate percentage of population change; we considered that there was a significant trend when the standard errors did not overlap with zero, positive values indicated increasing trends whereas negative values indicated decreasing trends.
Factors Influencing Population Trends
To explore the relationships between population trends and selected species traits, we obtained linear changes in population by estimating linear population trends using generalized linear mixed models (GLMM) with logit link function and zero-inflated Poisson or negative binomial error. Estimates (percentage of population changes) of GAMM were not used because the estimates are sensitive to initial counts which resulted in exceptionally large increases in some species (black-winged stilt, great knot, Pacific golden plover; Table 1). Zero-inflated models were used because over 67% of count were zeros. We included the total number of birds recorded as a dependent factor, year as an independent factor, and transect as a random factor. Then we examined the correlation between linear population changes of species (slope coefficient of year) and species traits using phylogenetic generalized least squares (PGLS), which accounts for phylogenetic relationships between species (Felsenstein, 1985). We obtained the phylogeny of the study species from the BirdTree database3. We developed a consensus phylogeny from 1,000 plausible phylogenetic trees using Hackett phylogenetic backbones (9,993 OTUs) (Jetz et al., 2012). We constructed the consensus phylogeny and did the PGLS using the function consensus.edges and pgls in R package phytools respectively (Revell, 2012). The eight species traits included in our models were breeding range, wintering range, breeding habitat, wintering habitat, body size, reliance on Yellow Sea as key habitats, reliance on aquaculture ponds, and intertidal mudflats. We collected information on distribution range and habitat use from Billerman et al. (2020). We followed the categorization in Amano et al. (2010) for breeding range (East Asia, South Siberia, and High Arctic), wintering range (East Asia, Southeast Asia, and the Oceania), breeding habitat and wintering habitat (generalist, freshwater, and coastal specialists). Body size was recorded as the maximum body size for each species using data in Billerman et al. (2020). Species relying on the use of Yellow Sea as their staging sites were reported to show population decline recently (Melville et al., 2016); to explore the effect of the Yellow Sea-reliance on the population trend in the Deep Bay area, species were categorized into those depending on the Yellow Sea and those that do not. Reliance on the Yellow Sea is defined as having more than 30% of the EAAF population staging during migration or breeding in the Yellow Sea, with data collected from Barter (2002); Amano et al. (2010), Wetlands International (2021), and Choi et al. (2020). In the Deep Bay area, waterbirds relying on either aquaculture ponds or intertidal mudflats may be influenced by a change in coverage of aquaculture ponds and intertidal mudflats. We quantified the reliance on aquaculture ponds and intertidal mudflats into four levels by calculating the proportion of individuals recorded from these two habitats (heavy ≥ 50%; moderate = 10–50%; light = 1–9% and; no = 0%), following the approach of Fujioka et al. (2010) who evaluated the importance of rice fields to shorebirds. We assessed multicollinearity in independent factors by calculating generalized variance inflation factors (GVIF); we excluded wintering ground that has GVIF > 2 (Fox and Monette, 1992). We built models for the three focal periods separately. To identify the most influential factors from the full models, we used the function dredge in the package MuMIn in software R to build models by fitting all possible combinations of independent factors (Barton, 2011). We determined the best models according to Akaike’s Information Criterion values corrected for small sample size (AICc); candidate models with < 2 units of difference in AICc value with the top model were considered as the set of best fitting models (Burnham and Anderson, 2002). We calculated final estimates and standard errors for species traits by averaging the parameters from the best fitting models. We applied Wald’s Z test to test the significance of the correlations between annual population changes and species traits (Bolker et al., 2009). We did the analysis separately for three focal periods: 1998–2017, 1998–2007, and 2008–2017. GLMM was built using the R package glmmTMB (Brooks et al., 2017).
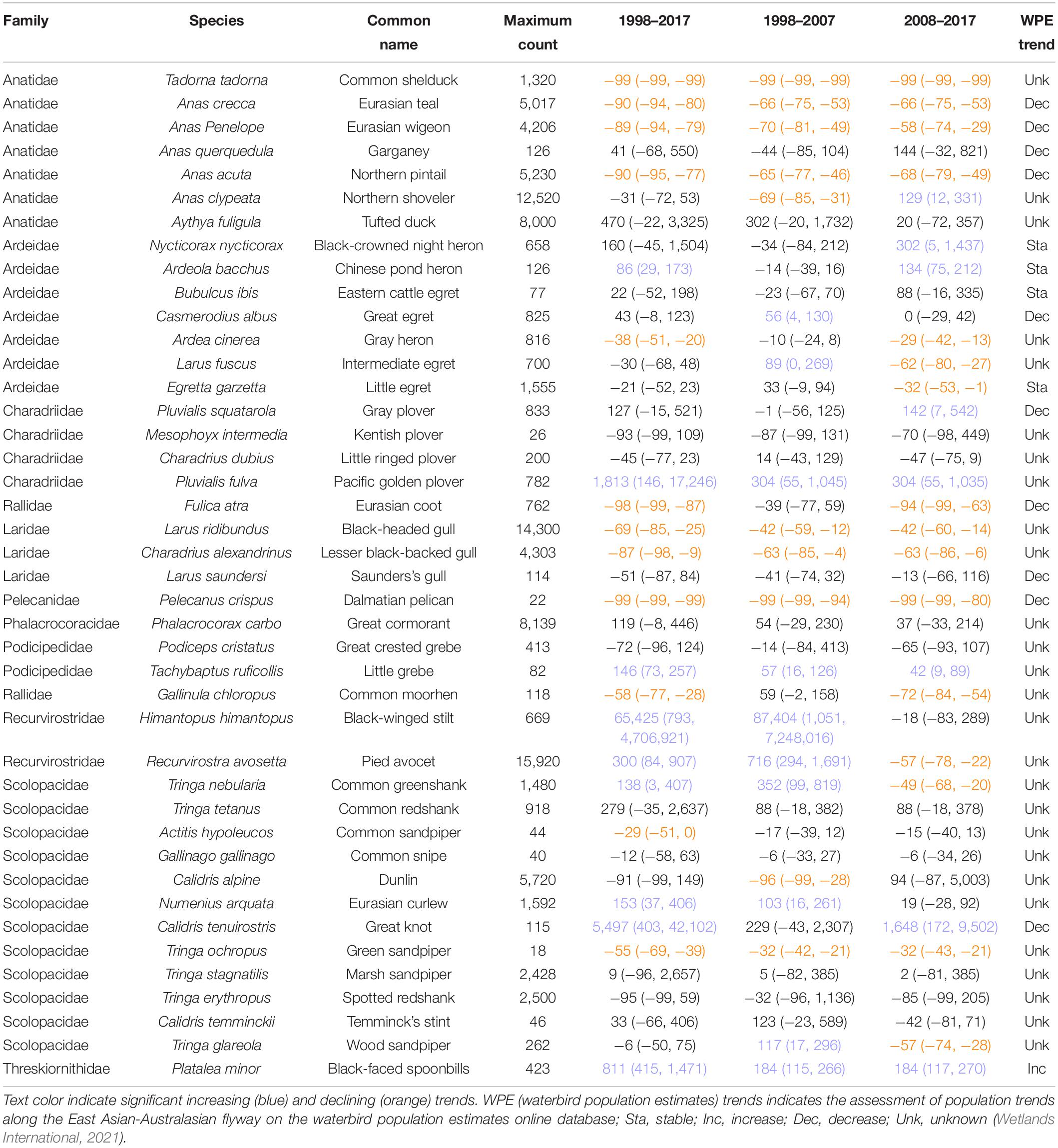
Table 1. Estimated percentage of population changes for 42 wintering waterbirds species in the Deep Bay area from 1998 to 2017 calculated by generalized additive mixed models using the package poptrend in software R.
Results
We recorded 130 wintering waterbird species from 1998 to 2017, and we included 42 species in the analysis. Of the 42 species, the population of nine species (black-faced spoonbill, black-winged stilt, Chinese pond heron, common greenshank, Eurasian curlew, great knot, little grebe, Pacific golden plover, pied avocet) increased, and 12 species (black-headed gull, common moorhen, common sandpiper, common shelduck, Dalmatian pelican, Eurasian coot, Eurasian teal, Eurasian wigeon, green sandpiper, gray heron, lesser black-backed gull, northern pintail) declined from 1998 to 2017 (Table 1 and Figures 1, 2). Among the nine increasing species, three species (black-faced spoonbill, little grebe, and Pacific golden plover) increased consistently in both 10-year periods (1998–2007 and 2008–2017). Among the 12 declining species, eight species (black-headed gull, common shelduck, Dalmatian pelican, Eurasian teal, Eurasian wigeon, green sandpiper, lesser black-backed gull, and northern pintail) decreased consistently in both 10-year periods. Five species exhibited reverse trends in the two 10-year periods; common greenshank, intermediate egret, pied avocet, and wood sandpiper increased from 1998 to 2007 and then decreased from 2008 to 2017, whereas northern shoveler decreased from 1998 to 2007 and increased from 2008 to 2017.
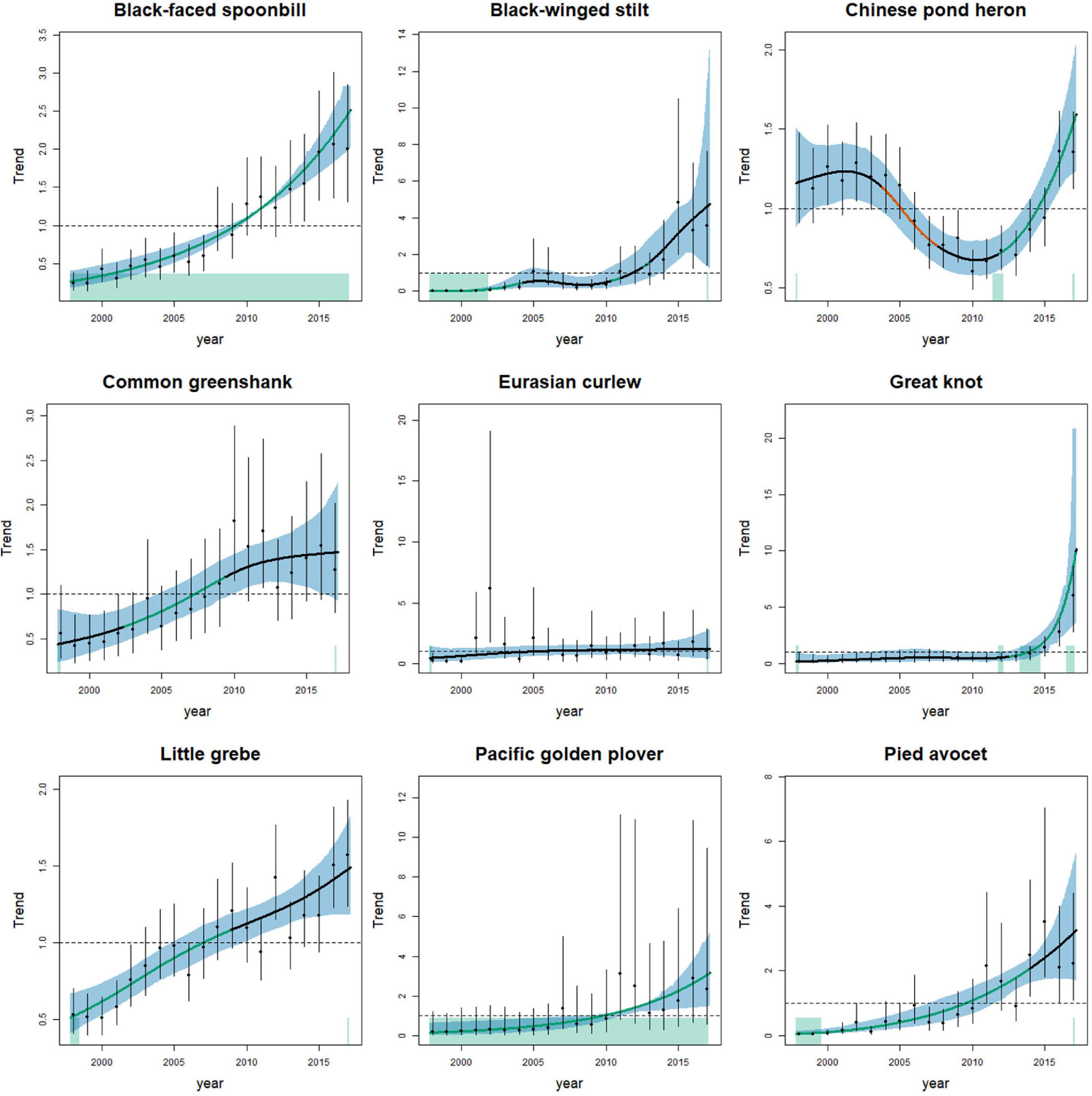
Figure 1. Relative changes in the abundance of nine wintering waterbird species that showed a significantly increasing trend from 1998 to 2017 in the Deep Bay area. Solid lines indicate estimated long-term trends. The trend lines are colored for the period with significantly increasing (green) or decreasing (orange) trends. Vertical lines and blue shaded areas indicate the 95% confidence intervals. The green rectangle at the bottom of panels indicate periods with significantly positive curvature. The y-axis shows the partial residuals of year in the generalized additive mixed models.
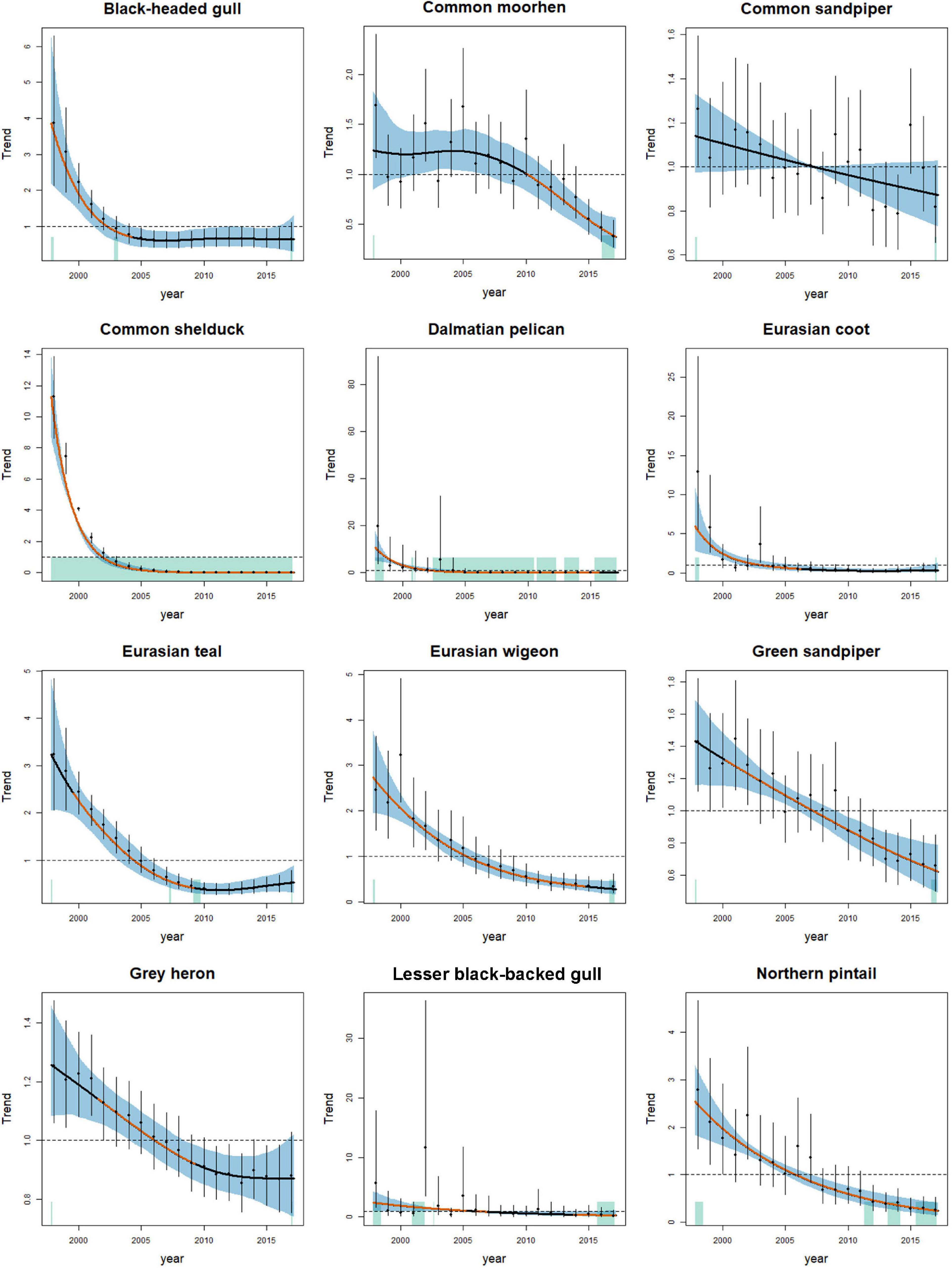
Figure 2. Relative changes in the abundance of 12 wintering waterbird species that showed a significantly decreasing trend from 1998 to 2017 in the inner Deep Bay area. Solid lines indicate estimated long-term trends. The trend lines are colored for the period with significantly increasing (green) or decreasing (orange) trends. Vertical lines and blue shaded areas indicate the 95% confidence intervals. The green rectangle at the bottom of panels indicate periods with significantly positive curvature. The y-axis shows the partial residuals of year in the generalized additive mixed models.
In the Deep Bay area, satellite maps revealed a consistent decline in coverage of intertidal mudflat (56–43%), and a consistent increase in coverage of mangrove (8–15%) and other vegetation (3–14%) from 2000 to 2018 (Figure 3). The coverage of aquaculture pond decreased from 2000 to 2008 (30–20%) and then increased from 2008 to 2018 (20–28%), whereas the coverage of human settlements increased from 2000 to 2008 (3–5%) and decreased from 2008 to 2018 (5–1%).
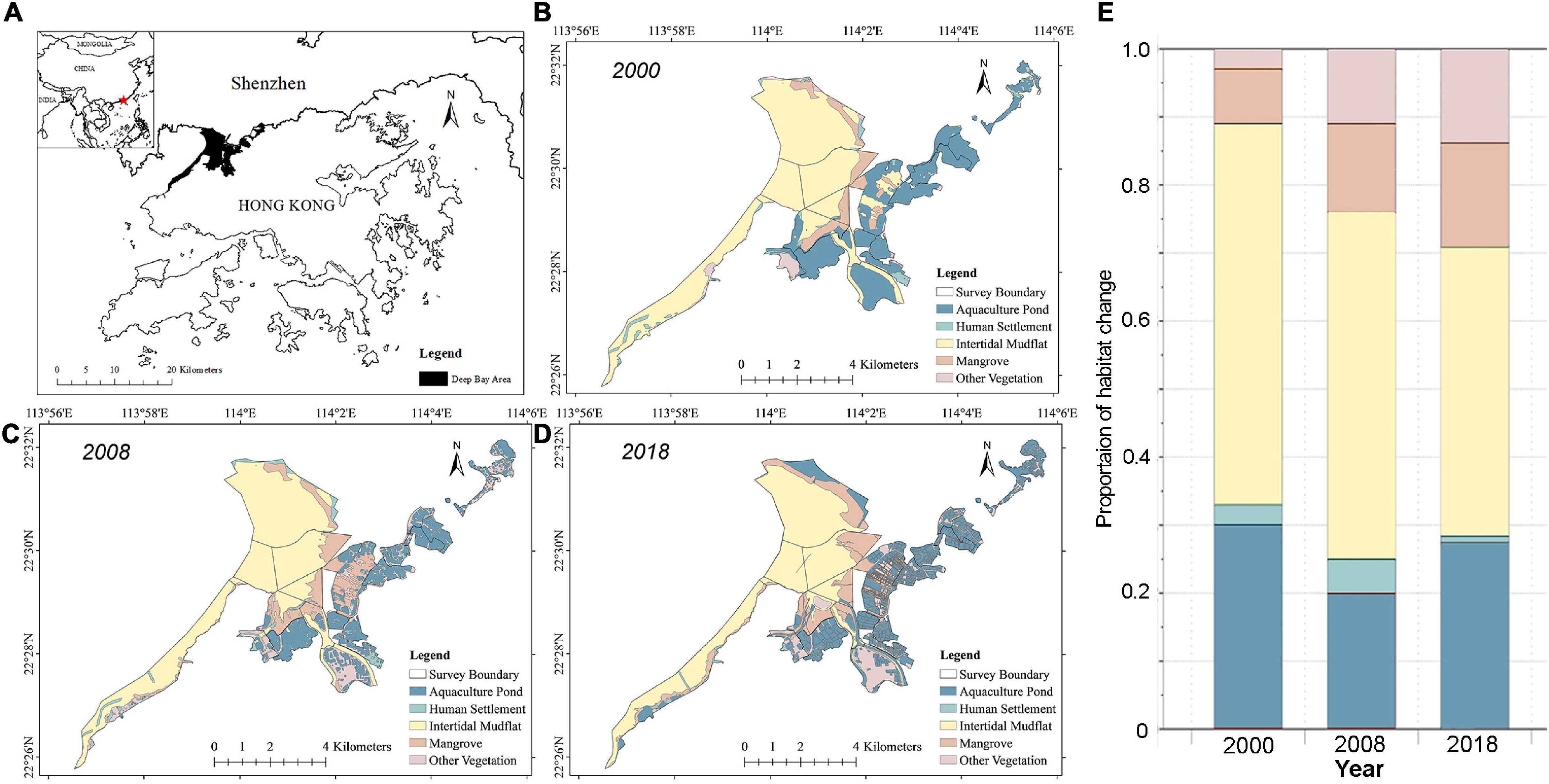
Figure 3. Habitat maps of the study area in the Deep Bay area (A), South China in (B) 2000, (C) 2008, and (D) 2018; and (E) proportion of habitat coverage in the study area in 2000, 2008, and 2018.
Body size, breeding habitat, breeding range, wintering habitat, reliance on the Yellow Sea, and dependence on aquaculture ponds were included in the most parsimonious models of PGLS (Supplementary Table 2). Species breeding in Southern Siberia declined more than those breeding in East Asia from 1998 to 2007 (Estimate = −0.204 ± 0.072; P = 0.008; Figure 4) and from 2008 to 2017 (Estimate = −0.166 ± 0.049; P = 0.001), whereas the species breeding High Arctic had similar trends with those breeding in the other two ranges in three focal periods (Table 2 and Figure 4). From 1998 to 2007, species that relied on the Yellow Sea declined more (Estimate = −0.193 ± 0.073; P = 0.013). From 2008 to 2017, body size had a negative impact on population changes (Estimate = −0.003 ± 0.001; P < 0.001).
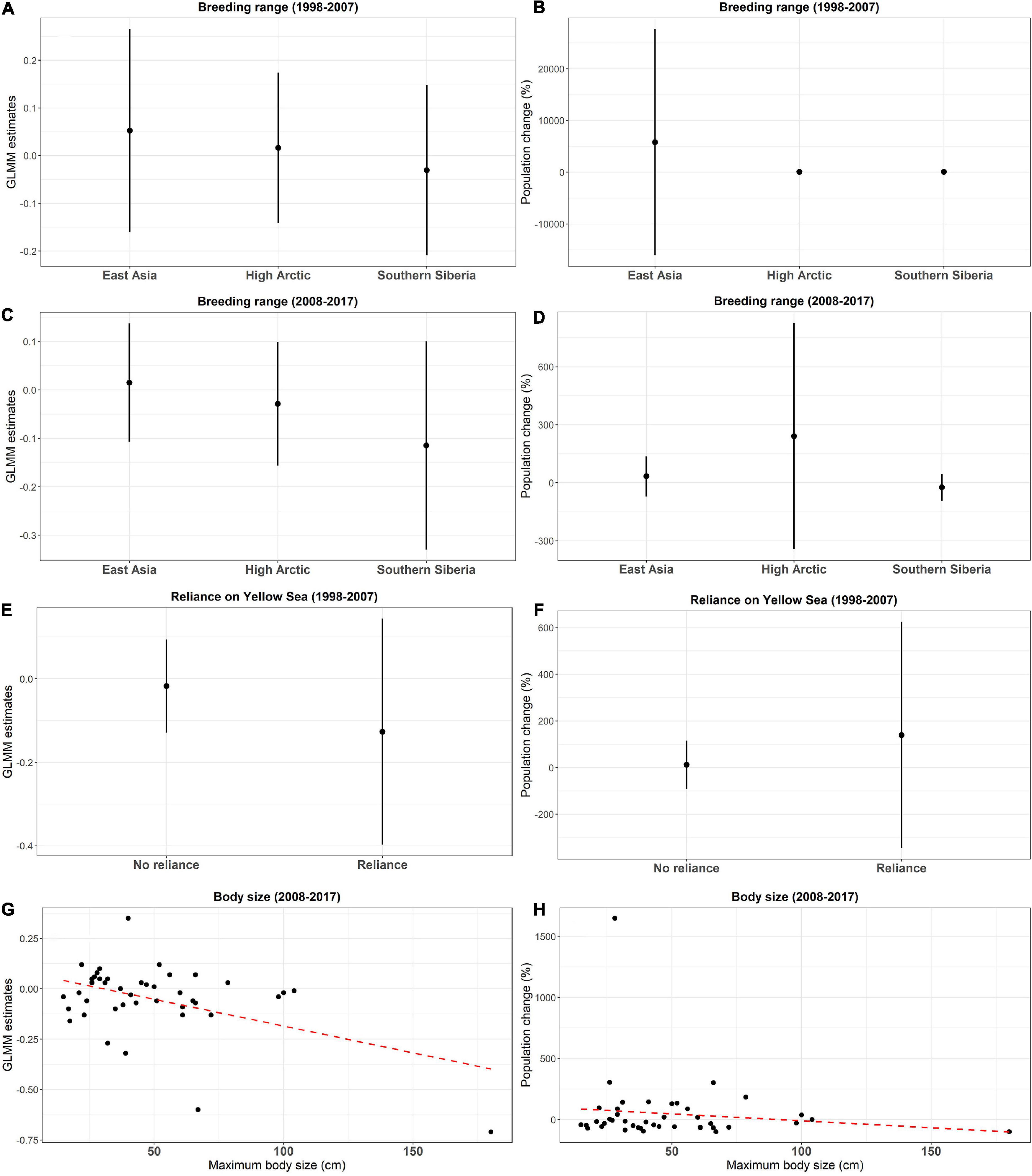
Figure 4. Estimates of generalized linear mixed models (GLMM) (left column) and percentage of populations changes (estimated by generalized additive mixed models) (right column) for the significant species traits in the phylogenetic generalized least squares (PGLS) models for population trends of waterbirds in the inner Deep Bay area: panels (A,B) breeding range from 1998 to 2007, panels (C,D) breeding range from 2008 to 2017, panels (E,F) reliance on Yellow Sea from 1998 to 2007, and panels (G,H) body size from 2008 to 2017. For panels (A–F), dots represent means and lines indicate standard deviations. For panels (G,H), the red lines indicate linear regression lines for the relationship between GLMM estimates and percentages of population changes and body sizes.
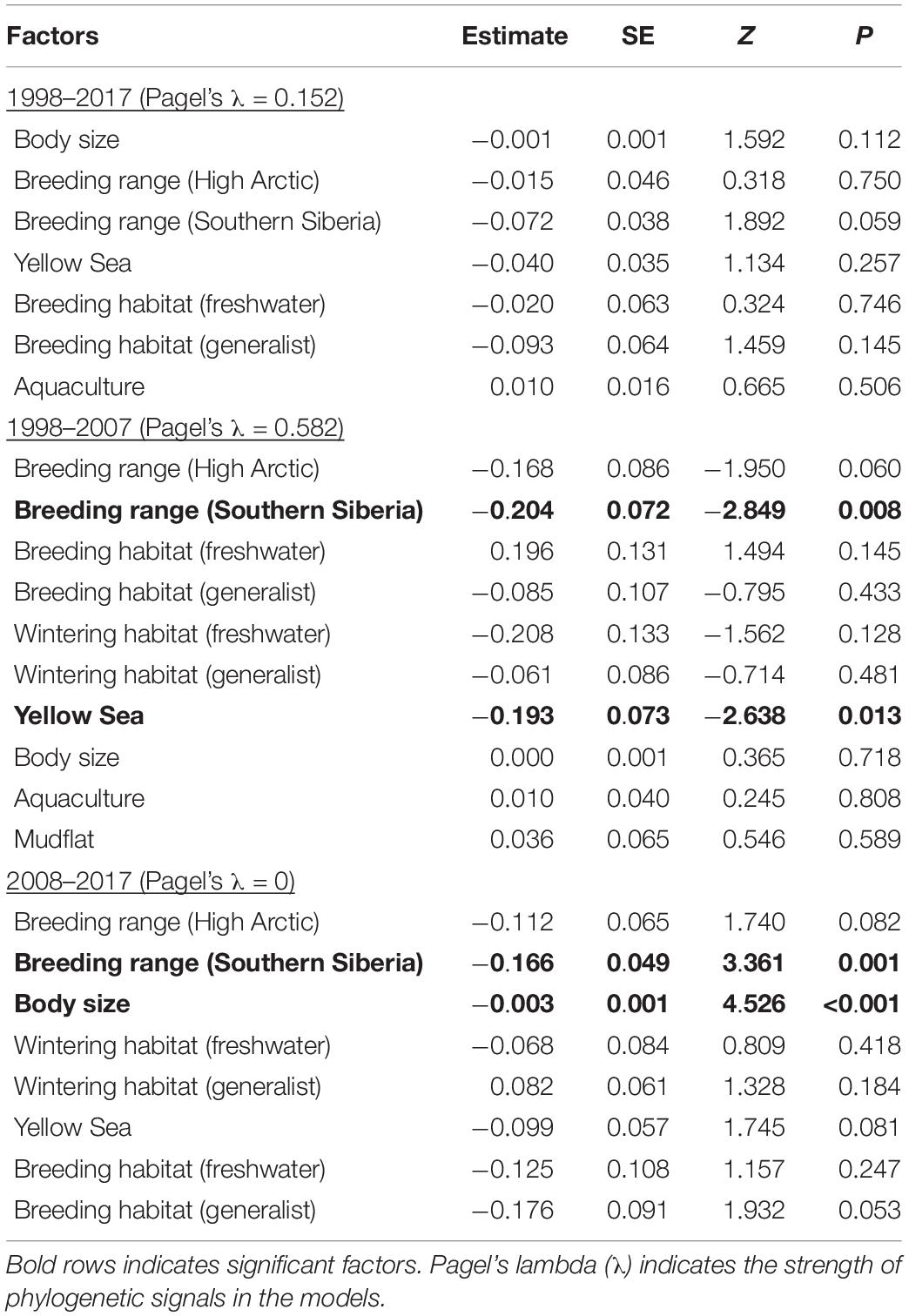
Table 2. Estimates of factors influencing population changes of 42 wintering water species in the Deep Bay area from 1998 to 2017 in the best phylogenetic generalized least squares models.
Discussion
In this study, we quantified the 20-year population trends of wintering waterbirds in the Deep Bay area. The overall population trends of most study species (26 out of 42) are unknown (Wetlands International, 2021; Table 1)—this study supplements information to quantifying their population trends along the rapidly changing EAAF. Further, we identified species traits of waterbirds that correlated with their population trends, which shed light on the threats they experience across their breeding, migratory, and wintering grounds.
Declining Species and Threats
We identified 12 species that displayed apparent declines. Three of these species (Eurasian coot, northern pintail, and Dalmatian pelican) were assessed to be declining in a regional assessment (Wetlands International, 2021), suggesting that they have dwindling populations along the EAAF and deserve immediate conservation attention. For the other nine declining species, it is uncertain if the decline we detected was confined to the Deep Bay area because of different trends observed in other wintering sites or limited population data elsewhere. It is of conservation concern that four (out of six) duck species declined significantly, including the common shelduck, common teal, Eurasian wigeon, and northern pintail. The common teal displayed opposite population trends in two wintering sites, decreasing in Japan and increasing in Poyang Lake, China, whereas the Eurasian wigeon decreased in Poyang Lake but remained stable in Japan (Kasahara and Koyama, 2010; Wang et al., 2017). The common shelduck declined dramatically, with no record after 2013. In contrast, recent survey data detected an increasing trend of the common shelduck along the coast of China between 2012 and 2019 (Choi et al., 2020). The Deep Bay area is one of the southernmost wintering grounds for the common shelduck. Other studies have shown that if population decline occurs, fewer birds move as far south for wintering, such that the decline can be first detected in the southernmost wintering ground (Cresswell and Bauer, 2014; Clemens et al., 2016).
Limited information is available for the other declining species (black-headed gull, lesser black-backed gull, common moorhen, common sandpiper, green sandpiper, and gray heron). In addition to the lack of long-term data from other wintering sites, the vast breeding range of these species also renders flyway-wide population estimation challenging, if not impossible. Continued monitoring at wintering sites, especially along the coast of China, will elucidate whether these species declining in the Deep Bay area are experiencing a species-wide population decline or changing wintering distribution.
Increasing Species and Habitat Transformation
In this study, we identified nine species (out of 42) that increased significantly. Such proportion of increasing species (21%) was higher than that in Australia (0%) (Clemens et al., 2016), but similar to that in Japan (29%) (Kasahara and Koyama, 2010). Among these increasing species, the black-faced spoonbill is the only species with sufficient data elsewhere supporting a population increase at a regional scale due to international conservation efforts for almost three decades (Sung et al., 2017; Wetlands International, 2021). For other species, there has been no information suggesting a growing population along the EAAF. In the Deep Bay area, there were considerably decline in coverage of intertidal mudflat (56–43%) whereas the changes were small for aquaculture pond (30–28%) and mangrove (8–15%) (Figure 3). However, the change of wetland area in the Deep Bay was small compared to that some stopover and wintering sites of waterbirds along the coast of China [e.g., the Yellow Sea area (>50% from 1950 to 2010) (Murray et al., 2015) and Yancheng Nature Reserve in Jiangsu Province (>72% from 1976 to 2007) (Zuo et al., 2012)] (Figure 3). Therefore, it is possible that the habitat degradation in other wintering sites led to redistribution of wintering populations, resulting in increasing trends for some species in Hong Kong.
Ecological Correlates of Population Trends
The low variation in wetland coverage may have contributed to our results that population trends of wintering populations were correlated with species traits associated with breeding grounds and stopover sites, rather than wintering sites. For the effect of breeding grounds, we found that species breeding in Southern Siberia declined more than those breeding in East Asia, while both had similar trends with the species breeding in High Arctic (Figure 4). Many species have vast breeding ranges; the difference may be related to large-scale environmental changes, such as climate change. A global study found that waterbird populations decreased more with decreasing latitude under climate change (Amano et al., 2020), which is opposite of our findings. Alternatively, migratory distance correlates with breeding ground and may explain the influence of breeding ground. In a study of land birds in Finland, long-distance migrants declined more than short-distance migrants under a warming climate (Virkkala et al., 2018). It is also possible that Southern Siberia experienced region-wide habitat degradation, although such data are currently unavailable to evaluate this possibility.
We found that species that rely on the Yellow Sea declined more than other species, which agrees to previous studies along the EAAF (Amano et al., 2010; Clemens et al., 2016). Despite the tremendous ecological value of the tidal flats in the Yellow Sea area, tidal mudflats have declined by over 50% in the last 50 years, mainly due to coastal reclamation (Murray et al., 2015). Urgent conservation actions are essential to reverse the negative impacts of such rapid habitat loss and degradation. Extended from our study, there are more passage migrant species (both spring and autumn) relying on the Yellow Sea (e.g., curlew sandpiper, red knot, red-necked stint) than wintering species in the Deep Bay area, therefore it is worthwhile to investigate if reliance on the Yellow Sea also influences population trends of spring and autumn migrants.
In this study, larger species declined more than small species from 2008 to 2017, which might be related to hunting (Purvis et al., 2000). Both large and small waterbird species are hunted (Barter et al., 1997), however, larger species are favored by contemporary hunters (Gallo-Cajiao et al., 2020). Supporting this, the Dalmatian Pelican and ducks, the larger species in this study, has experienced a drastic decline across the EAAF, with hunting as the major threat (Batbayar et al., 2007; Ming et al., 2012). It should be noted that body size did not influence population trends from 1998 to 2007. As hunting intensity of waterbirds was probably similar or lower in recent years, we believe that there may be other contributing factors. Larger species are more vulnerable to population decline because of their lower fecundity (Purvis et al., 2000). Other threats (e.g., habitat degradation or climate change) in breeding, stopover or other wintering sites may lead to a more drastic population decline in larger species. Nonetheless, our results signal further studies to determine the effect of various threats, especially hunting, on waterbirds of different body sizes (Fox and Monette, 1992).
Conclusion
We quantified the wintering populations of 42 species, providing important baseline information to evaluate their flyway-wide conservation status. Conservation attention should be paid to the declining species, including ducks and larger species that are susceptible to hunting. Compared to other studies, a relatively high proportion of species exhibited increasing trends, providing evidence to support the continued protection against development of wetlands in the Deep Bay area. Population trends of wintering waterbirds were affected by factors associated with breeding and stopover grounds, particularly the negative impacts of habitat transformation in the Yellow Sea area. Our results enhance our understanding of the population status and threats to waterbird species, which allow us to formulate and prioritize conservation actions for waterbird species along the rapidly changing EAAF.
Data Availability Statement
The raw data supporting the conclusions of this article will be made available by the authors, without undue reservation.
Ethics Statement
Ethical review and approval was not required for the animal study because this study does not involve any procedures that need ethical review and approval.
Author Contributions
Y-HS, CP, TL, and YY: experimental design. CP, TL, PW, and YY: data collection. Y-HS, CP, TL, PW, and YY: data analysis. Y-HS, CP, PW, and YY: manuscript preparation. All authors contributed to manuscript revision, read and approved the submitted version.
Funding
This project was funded by the Agriculture, Fisheries and Conservation Department of the Hong Kong Special Administration Region as a part of the implementation of a Conservation Strategy and Management Plan for the Ramsar Site since 1998.
Conflict of Interest
The authors declare that the research was conducted in the absence of any commercial or financial relationships that could be construed as a potential conflict of interest.
Acknowledgments
We would like to thank G. J. Carey, former HKBWS coordinator of the waterbird monitoring program, for structuring this waterbird count from a simple-and-basic volunteer-based survey into a standardized, systematic monitoring program in mid-1990s. We are grateful to Agriculture, Fisheries and Conservation Department, Hong Kong Government for their assistance, including issuing permits to enter the Mai Po Nature Reserve and approving the use of data of the Mai Po Inner Deep Bay Ramsar Site Waterbird Monitoring Program. We would also like to thank Shenzhen Bird Watching Society and Guangdong Neilingding Futian National Nature Reserve for carrying out surveys at Shenzhen. We are also grateful to Ivan Tse and Helen Fong for assistance in the administrative work of this waterbird monitoring program. We would further like to thank Jonathan Fong for comments on an earlier draft of the manuscript. Last but not least, we would like to thank many members of Hong Kong Bird Watching Society who helped with bird surveys of this study, without whom this monitoring program could not have been done.
Supplementary Material
The Supplementary Material for this article can be found online at: https://www.frontiersin.org/articles/10.3389/fevo.2021.658084/full#supplementary-material
Footnotes
- ^ https://globil-panda.opendata.arcgis.com/datasets/mai-po-wetland-habitats
- ^ https://www.epd.gov.hk/epd/english/environmentinhk/eia_planning/sea/terresthab.html
- ^ http://birdtree.org/
References
Amano, T., Székely, T., Koyama, K., Amano, H., and Sutherland, W. J. (2010). A framework for monitoring the status of populations: an example from wader populations in the East Asian–Australasian flyway. Biol. Conserv. 143, 2238–2247. doi: 10.1016/j.biocon.2010.06.010
Amano, T., Székely, T., Wauchope, H. S., Sandel, B., Nagy, S., Mundkur, T., et al. (2020). Responses of global waterbird populations to climate change vary with latitude. Nat. Clim. Change 10, 959–964. doi: 10.1038/s41558-020-0872-3
Bai, Q., Chen, J., Chen, Z., Dong, G., Dong, J., Dong, W., et al. (2015). Identification of coastal wetlands of international importance for waterbirds: a review of China Coastal Waterbird Surveys 2005–2013. Avian Res. 6:12. doi: 10.1186/s40657-015-0021-2
Barter, M. (2002). Shorebirds of the Yellow Sea–Importance, Threats and Conservation Status. Wetlands International Global Series 9 and International Wader Studies 12. Wageningen: Wetlands International.
Barter, M., Qian, F., Tang, S., Yuan, X., and Tonkinson, D. (1997). Hunting of migratory waders on Chongmin Dao: a declining occupation. Stilt 31, 19–22.
Barton, K. (2011). MuMIn: Multi-Model Inference. R Package Version 1.0.0. Available online at: http://CRAN.R-project.org/package/MuMIn/ (accessed 20 Aug 2016)
Batbayar, N., Baraunlich, A., Natsagdorj, T., Setev, S., and Sovd, G. (2007). Conservation of the critically endangered east Asian population of Dalmatian Pelican Pelecanus crispus in western Mongolia. BirdingASIA 7, 68–74.
Bell, J. R., Blumgart, D., and Shortall, C. R. (2020). Are insects declining and at what rate? An analysis of standardised, systematic catches of aphid and moth abundances across Great Britain. Insect Conserv. Diver. 13, 115–126. doi: 10.1111/icad.12412
Billerman, S. M., Keeney, B. K., Rodewald, P. G., and Schulenberg, T. S. (2020). Birds of the World. Available online at: https://birdsoftheworld.org/bow/home (accessed 25 Jun 2020)
Bolker, B. M., Brooks, M. E., Clark, C. J., Geange, S. W., Poulsen, J. R., Stevens, M. H. H., et al. (2009). Generalized linear mixed models: a practical guide for ecology and evolution. Trends Ecol. Evol. 24, 127–135. doi: 10.1016/j.tree.2008.10.008
Brooks, M. E., Kristensen, K., van Benthem, K. J., Magnusson, A., Berg, C. W., Nielsen, A., et al. (2017). glmmTMB balances speed and flexibility among packages for zero-inflated generalized linear mixed modeling. R J. 9, 378–400.
Burnham, K. P., and Anderson, D. R. (2002). Model Selection and Multimodel Inference: A Practical Information-Theoretic Approach, 2nd Edn. New York, NY: Springer-Verlag.
Carey G. J. (ed.). (1994). “Winter waterfowl counts 1992-93,” in Hong Kong Bird Report 1993, (Hong Kong: Hong Kong Bird Watching Society).
Catry, P., Dias, M. P., Phillips, R. A., and Granadeiro, J. P. (2013). Carry-over effects from breeding modulate the annual cycle of a long-distance migrant: an experimental demonstration. Ecology 94, 1230–1235. doi: 10.1890/12-2177.1
Choi, C. Y., Li, J., and Xue, W. J. (2020). China Coastal Waterbird Census Report (Jan. 2012 – Dec. 2019). Hong Kong: Hong Kong Bird Watching Society.
Clemens, R., Rogers, D. I., Hansen, B. D., Gosbell, K., Minton, C. D. T., Straw, P., et al. (2016). Continental-scale decreases in shorebird populations in Australia. Emu Austral Ornithol. 116, 119–135. doi: 10.1071/mu15056
Cresswell, W., and Bauer, S. (2014). Migratory connectivity of Palaearctic-African migratory birds and their responses to environmental change: the serial residency hypothesis. Ibis 156, 493–510. doi: 10.1111/ibi.12168
Felsenstein, J. (1985). Phylogenies and the comparative method. Am. Nat. 125, 1–15. doi: 10.1086/284325
Fox, J., and Monette, G. (1992). Generalized collinearity diagnostics. J. Am. Stat. Assoc. 87, 178–183.
Fujioka, M., Don Lee, S., Kurechi, M., and Yoshida, H. (2010). Bird use of rice fields in Korea and Japan. Waterbirds 33, 8–29. doi: 10.1675/063.033.s102
Gallo-Cajiao, E., Morrison, T. H., Woodworth, B. K., Lees, A. C., Naves, L. C., Yong, D. L., et al. (2020). Extent and potential impact of hunting on migratory shorebirds in the Asia-Pacific. Biol. Conserv. 246:108582. doi: 10.1016/j.biocon.2020.108582
Gan, X., Cai, Y., Choi, C., Ma, Z., Chen, J., and Li, B. (2009). Potential impacts of invasive Spartina alterniflora on spring bird communities at Chongming Dongtan, a Chinese wetland of international importance. Estuar. Coast. Shelf Sci. 83, 211–218. doi: 10.1016/j.ecss.2009.03.026
Howard, C., Stephens, P. A., Pearce-Higgins, J. W., Gregory, R. D., Butchart, S. H. M., and Willis, S. G. (2020). Disentangling the relative roles of climate and land cover change in driving the long-term population trends of European migratory birds. Divers. Distrib. 26, 1442–1455. doi: 10.1111/ddi.13144
Iwamura, T., Possingham, H. P., Chades, I., Minton, C., Murray, N. J., Rogers, D. I., et al. (2013). Migratory connectivity magnifies the consequences of habitat loss from sea-level rise for shorebird populations. Proc. R. Soc. B Biol. Sci. 280:20130325. doi: 10.1098/rspb.2013.0325
Jetz, W., Thomas, G. H., Joy, J. B., Hartmann, K., and Mooers, A. O. (2012). The global diversity of birds in space and time. Nature 491, 444–448. doi: 10.1038/nature11631
Kasahara, S., and Koyama, K. (2010). Population trends of common wintering waterfowl in Japan: participatory monitoring data from 1996 to 2009. Ornithol. Sci. 9, 23–36.
Knape, J. (2016). Decomposing trends in Swedish bird populations using generalized additive mixed models. J. Appl. Ecol. 53, 1852–1861. doi: 10.1111/1365-2664.12720
Melville D. S. (ed.). (1980). “Waterfowl count 14th January 1979,” in Hong Kong Bird Report 1978, (Hong Kong: Hong Kong Bird Watching Society).
Melville, D. S., Chen, Y., and Ma, Z. J. (2016). Shorebirds along the Yellow Sea coast of China face an uncertain future – a review of threats. Emu 116, 100–110. doi: 10.1071/Mu15045
Ming, R. M., Zhang, T., Blank, D., Ding, P., and Zhao, X. M. (2012). Geese and ducks killed by poison and analysis of poaching cases in China. Goose Bull. 15, 2–11.
Murray, N. J., Ma, Z., and Fuller, R. A. (2015). Tidal flats of the Yellow Sea: a review of ecosystem status and anthropogenic threats. Austral Ecol. 40, 472–481. doi: 10.1111/aec.12211
Murray, N. J., Marra, P. P., Fuller, R. A., Clemens, R. S., Dhanjal-Adams, K., Gosbell, K. B., et al. (2017). The large-scale drivers of population declines in a long-distance migratory shorebird. Ecography 41, 867–876. doi: 10.1111/ecog.02957
Pang, C. C., Sung, Y. H., Chung, Y. T., Ying, H. K., Fong, H. H. N., and Yu, Y. T. (2020). Spatial ecology of little egret (Egretta garzetta) in Hong Kong uncovers preference for commercial fishponds. PeerJ. 8:e9893.
Pavón-Jordán, D., Fox, A. D., Clausen, P., Dagys, M., Deceuninck, B., Devos, K., et al. (2015). Climate-driven changes in winter abundance of a migratory waterbird in relation to EU protected areas. Divers. Distrib. 21, 571–582. doi: 10.1111/ddi.12300
Piersma, T., Lok, T., Chen, Y., Hassell, C. J., Yang, H.-Y., Boyle, A., et al. (2016). Simultaneous declines in summer survival of three shorebird species signals a flyway at risk. J. Appl. Ecol. 53, 479–490. doi: 10.1111/1365-2664.12582
Purvis, A., Gittleman, J. L., Cowlishaw, G., and Mace, G. M. (2000). Predicting extinction risk in declining species. Proc. R. Soc. B Biol. Sci. 267, 1947–1952. doi: 10.1098/rspb.2000.1234
R Core Team (2019). R: A Language And Environment For Statistical Computing. Vienna: R foundation for statistical computing.
Revell, L. J. (2012). phytools: an R package for phylogenetic comparative biology (and other things). Methods Ecol. Evol. 3, 217–223. doi: 10.1111/j.2041-210X.2011.00169.x
Studds, C. E., Kendall, B. E., Murray, N. J., Wilson, H. B., Rogers, D. I., Clemens, R. S., et al. (2017). Rapid population decline in migratory shorebirds relying on Yellow Sea tidal mudflats as stopover sites. Nat. Commun. 8:14895. doi: 10.1038/ncomms14895
Sung, Y.-H., Tse, I. W.-L., and Yu, Y.-T. (2017). Population trends of the Black-faced Spoonbill Platalea minor: analysis of data from international synchronised censuses. Bird Conserv. Int. 28, 157–167. doi: 10.1017/s0959270917000016
Virkkala, R., Rajasärkkä, A., Heikkinen, R. K., Kuusela, S., Leikola, N., and Pöyry, J. (2018). Birds in boreal protected areas shift northwards in the warming climate but show different rates of population decline. Biol. Conserv. 226, 271–279. doi: 10.1016/j.biocon.2018.08.015
Wang, W., Fraser, J. D., and Chen, J. (2017). Distribution and long-term population trends of wintering waterbirds in Poyang Lake, China Wetlands 39, 125–135. doi: 10.1007/s13157-017-0981-6
Wang, X., Kuang, F., Tan, K., and Ma, Z. (2018). Population trends, threats, and conservation recommendations for waterbirds in China. Avian Res. 9:14. doi: 10.1186/s40657-018-0106-9
Webster, M. S., Marra, P. P., Haig, S. M., Bensch, S., and Holmes, R. T. (2002). Links between worlds: unraveling migratory connectivity. Trends Ecol. Evol. 17, 76–83. doi: 10.1016/s0169-5347(01)02380-1
Wetlands International (2021). Waterbird Population Estimates. Available online at: http://wetlands.org (accessed 7 Jan 2021)
Wikramanayake, E., Or, C., Costa, C., Wen, X., Cheung, F., and Shapiro, A. (2020). A climate adaptation strategy for Mai Po Inner Deep Bay Ramsar site: steppingstone to climate proofing the East Asian-Australasian Flyway. PLoS One 15:e0239945. doi: 10.1371/journal.pone.0239945
Zhao, Z., Lu, Z., and Wu, J. (2016). Polycyclic aromatic hydrocarbons (PAHs) and organochlorine pesticides (OCPs) in sediments from lakes along the middle-lower reaches of the Yangtze River and the Huaihe River of China. Limnol. Oceanogr. 61, 47–60. doi: 10.1002/lno.10197
Keywords: bird, coastal wetlands, conservation, East Asian-Australasian flyway, Ramsar site, shorebirds
Citation: Sung Y-H, Pang C, Li TC, Wong PPY and Yu Y (2021) Ecological Correlates of 20-Year Population Trends of Wintering Waterbirds in Deep Bay, South China. Front. Ecol. Evol. 9:658084. doi: 10.3389/fevo.2021.658084
Received: 25 January 2021; Accepted: 01 April 2021;
Published: 20 April 2021.
Edited by:
Yang Liu, Sun Yat-sen University, ChinaReviewed by:
Dan Liang, Princeton University, United StatesQing Zhao, University of Missouri, United States
Copyright © 2021 Sung, Pang, Li, Wong and Yu. This is an open-access article distributed under the terms of the Creative Commons Attribution License (CC BY). The use, distribution or reproduction in other forums is permitted, provided the original author(s) and the copyright owner(s) are credited and that the original publication in this journal is cited, in accordance with accepted academic practice. No use, distribution or reproduction is permitted which does not comply with these terms.
*Correspondence: Yat-tung Yu, bfspoonbill@hkbws.org.hk