- 1SymbuLab, Carleton College, Computer Science, Northfield, MN, United States
- 2Digital Evolution Lab, Michigan State University, Department of Computer Science, Program in Ecology, Evolution, and Behavior, BEACON Center for the Study of Evolution in Action, East Lansing, MI, United States
- 3ZE3 Lab, University of Michigan, Department of Ecology and Evolutionary Biology & Center for the Study of Complex Systems, Ann Arbor, MI, United States
Symbiosis, the living together of unlike organisms as symbionts, is ubiquitous in the natural world. Symbioses occur within and across all scales of life, from microbial to macro-faunal systems. Further, the interactions between symbionts are multimodal in both strength and type, can span from parasitic to mutualistic within one partnership, and persist over generations. Studying the ecological and evolutionary dynamics of symbiosis in natural or laboratory systems poses a wide range of challenges, including the long time scales at which symbioses evolve de novo, the limited capacity to experimentally control symbiotic interactions, the weak resolution at which we can quantify interactions, and the idiosyncrasies of current model systems. These issues are especially challenging when seeking to understand the ecological effects and evolutionary pressures on and of a symbiosis, such as how a symbiosis may shift between parasitic and mutualistic modes and how that shift impacts the dynamics of the partner population. In digital evolution, populations of computational organisms compete, mutate, and evolve in a virtual environment. Digital evolution features perfect data tracking and allows for experimental manipulations that are impractical or impossible in natural systems. Furthermore, modern computational power allows experimenters to observe thousands of generations of evolution in minutes (as opposed to several months or years), which greatly expands the range of possible studies. As such, digital evolution is poised to become a keystone technique in our methodological repertoire for studying the ecological and evolutionary dynamics of symbioses. Here, we review how digital evolution has been used to study symbiosis, and we propose a series of open questions that digital evolution is well-positioned to answer.
1. Introduction
In an 1878 lecture, Heinrich Anton de Bary introduced the term “symbiosis” in a biological context to describe intimate associations between different types of organisms (Oulhen et al., 2016). Symbiosis is ubiquitous in the natural world, especially when considering microbial symbionts such as the human microbiome(s) (Paracer and Ahmadjian, 2000) (see Table 1 for relevant definitions). Due to this pervasiveness, most species have co-evolved with symbionts in the past and continue to do so in the present. Symbioses have been hypothesized to influence the evolution of sexual recombination (Tooby, 1982; Ebert and Hamilton, 1996; Morran et al., 2011), complexity (Dawkins and Krebs, 1979; Vermeij, 1993; Moran, 2007), diversity (Zaman et al., 2011), evolvability (Williams, 2013), and cooperation (Diggle et al., 2007), among many other behaviors (Vermeij, 1994). Indeed, symbiosis in all of its varieties is essential to our understanding of how life on our planet evolved, how it may continue to evolve, and how its evolutionary mechanisms can be harnessed to solve other problems.
However, there are still many open questions about the general patterns and processes of symbiotic coevolution, for example:
1. Does a co-evolving symbiont decrease the partner's evolutionary rate?
2. Do symbioses promote the evolution of novel traits?
3. Is the effect of coevolution on the partner species different if the symbiont is parasitic or mutualistic? If so, why?
4. What factors promote the evolution of symbiosis toward a mutualistic or parasitic dynamic?
5. Does an obligate symbiosis always evolve from a facultative symbiosis? If not, what determines the direction of evolution?
These questions are difficult to answer because of the interplay between the evolutionary pressures selecting for a particular kind of symbiosis (parasitic, commensalistic, or mutualistic; obligate or facultative; etc.) and the evolutionary pressures resulting from that symbiosis. For example, the presence of co-evolving parasites typically selects for greater diversity in the host population (Zaman et al., 2011), but there may then be pressure on the symbiont species to evolve away from parasitism (Drew et al., 2021).
There have been many efforts to study the long-term evolutionary dynamics of symbiosis, including observational and experimental studies of well-known symbiotic systems (Anstett et al., 1997; Bshary and Grutter, 2006; Dean et al., 2009; Laanto et al., 2017), analytically tractable mathematical models (Akçay, 2015), and artificial biological systems (Momeni et al., 2011). Each approach has strengths and weaknesses. Biological systems are required to truly understand symbiosis in nature, but testing the general principles underpinning the evolutionary origins and consequences of symbiotic relationships can be challenging due to the time scales over which the dynamics occur (even bacteria require months to evolve, Sachs et al., 2011) and the idiosyncrasies of any single system. It is also difficult and expensive to track every symbiotic genetic trait in an evolving biological population. On the other end of the spectrum, population-level analytically tractable mathematical models are faster and less expensive than using biological systems, but pay for those benefits by making many consequential simplifying assumptions. These assumptions can include that populations have infinite size (Adami et al., 2016), species have a limited number of possible genotypes, and forgoing the ability to track individual-level interactions between organisms (Hellweger et al., 2016).
Digital evolution is an individual- or agent-based modeling approach (DeAngelis and Mooij, 2005; Railsback and Grimm, 2019) to studying evolution that strikes a balance between the open-ended nature of biological systems and the speed, cost, and transparency of analytically tractable mathematical models. In digital evolution, populations of computational organisms compete for resources, reproduce, mutate, and evolve in a digital environment (described in further detail in section 3; Figure 1).
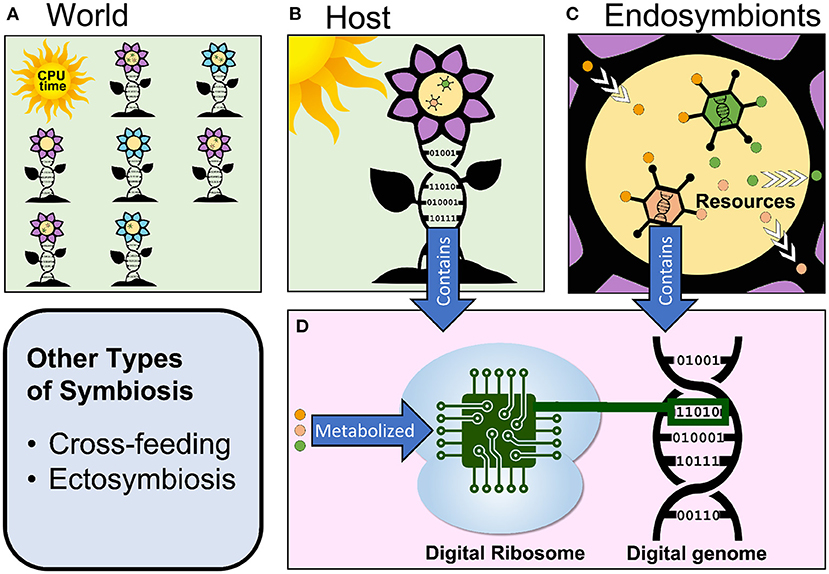
Figure 1. Overview of the components of a hypothetical digital evolution system that supports endosymbiosis. (A) A virtual world contains computational organisms of different phenotypes and provides them with space (in the computer's memory) and resources (CPU cycles in this example). (B) Each host organism has a digital genome and can contain one or more endosymbionts. The host takes in resources from the environment. (C) Endosymbionts each have a digital genome as well, exist inside of their host, and might metabolize some of the resources that their host takes in. (D) Every host and endosymbiont's digital genome is processed by a digital ribosome to produce the organism's phenotype. This process requires resources to occur.
Digital evolution experiments have been used to address important questions on a wide range of topics, including the evolution of complexity (Adami et al., 2000; Lenski et al., 2003), sexual recombination (Misevic et al., 2010), and division of labor (Goldsby et al., 2012, 2014). Here, we argue that digital evolution is a valuable framework for investigating both the evolutionary origins and evolutionary consequences of symbiosis. We first discuss the finer details of symbiosis and digital evolution in sections 2 through 4. We then review the history of symbiosis in digital evolution in section 5, and we show that symbiosis has been a common theme throughout the history of digital evolution research. In section 6, we review more recent experiments conducted with digital evolution systems that have yielded valuable insights about symbiosis, including the effect of parasites on the evolution of host complexity (Zaman et al., 2014) and diversity (Zaman et al., 2011), the role of vertical transmission and spatial structure on the evolution of mutualism or parasitism (Vostinar and Ofria, 2019), and the role of mutualistic symbiosis on population diversity (Pachepsky et al., 2002). Finally, in section 7, we suggest open questions in symbiosis and future directions in digital evolution that will be best suited to answering those questions.
2. Symbiosis Scope and Working Definition
While there has been debate about what particular dynamics symbiosis includes, for the purposes of this review, we consider it to be any interaction that (1) is close and long-term at the individual level to the extent that the interaction has a significant impact on the evolution of the species involved; (2) involves at least two different species (to the extent that species are able to be defined); and (3) is beneficial to one or more involved species. Specifically, symbiosis includes interactions that are beneficial to all involved species (mutualistic), beneficial to one involved species and neutral to the other (commensal), or detrimental for one species but beneficial to another (parasitic). Note that an interaction can be a mutualism without being a mutualistic symbiosis if members of different species cooperate with each other but individuals are not engaged in a close and long-term interaction with consistent individuals of the other species (Daida et al., 1996). The same is true for parasitism and commensalism.
In particular, we are not considering predator-prey relationships to fall under symbiosis for this review for two reasons. First, predator-prey relationships typically do not involve specific individuals living in close proximity (e.g., individuals of one species living within or on individuals of another species) over a prolonged period of time as is the case in symbiotic relationships (Combes, 2001; Lafferty and Kuris, 2002; Raffel et al., 2008), which changes the evolutionary pressures and mechanisms involved. Second, predator-prey dynamics in digital evolution are reviewed by Dolson and Ofria in this issue (Dolson and Ofria, 2021).
Finally, we do specifically include syntrophy or cross-feeding, where one species lives off of the metabolic products of another species in an “obligately mutualistic metabolism” (Morris et al., 2013), if the relationship is specific and long-term at the individual level, such that it would have a significant impact on the evolution of the involved species. For the relationship to be specific at the individual level, spatial structure is usually necessary to enable two individuals to regularly share resources, which excludes well-mixed systems from the scope.
3. What Is Digital Evolution?
Digital evolution experiments have emerged as a powerful research framework with which evolution can be studied. The precise meaning of “digital evolution” has varied over time and by research group, ranging from narrow definitions (e.g., populations of self-replicating computer programs that compete for resources, mutate, and evolve, Wilke and Adami, 2002) to broader definitions (e.g., any digital instantiation of evolution, Lehman et al., 2020). Broadly, however, digital evolution features populations of computational organisms that compete, mutate, and evolve in a virtual world, as shown in Figure 1. We limit the scope of our review to digital evolution systems with the following characteristics (described in more detail below):
1. Instantiates the evolution of populations of individuals in silico,
2. Has a practically infinite number of possible genomes,
3. Has a complex genotype-to-phenotype mapping due (at least in part) to the effects of symbiosis, and
4. Has implicit fitness.
3.1. Digital Instantiation of Evolution
As described by Thomas Ray, an early pioneer of digital evolution research, “[t]he object of an [artificial life] instantiation is to introduce the natural form and process of life into an artificial medium” (Ray, 1993). Digital evolution (as we define it in this review) does not attempt to simulate biological organisms and their evolution; instead, digital evolution systems instantiate evolutionary processes in a digital medium, allowing experimenters to directly observe evolution in populations of digital organisms (Pennock, 2007).
To accomplish this instantiation, digital evolution systems are specified at the individual level (as opposed to the population level) and include the requisites for evolution by natural selection: reproduction with inheritance, competition, variation, and time. Digital evolution systems define the genomes of organisms in many different ways, but organisms are always able to reproduce (often only asexually) and pass their genomes on to their offspring. Digital organisms most often compete for limited space in the environment (e.g., with offspring replacing random organisms) or for other limited resources (e.g., CPU cycles or energy). Variation is often introduced through mutations, but it can also arise during sexual reproduction (e.g., cross-over).
3.2. (Practically) Unlimited Number of Genomes
Genomes in digital evolution systems vary greatly in complexity and form, ranging from relatively short computer programs (using a specialized computer language), to sequences of 0's and 1's, to single floating point numbers (Hindré et al., 2012). However, there must be a practically unlimited number of possible genomes. That is, populations must be capable of containing many different mutants at one time, and the space of possible genomes should be too large for an evolving population to enumerate during an experiment. One of the strengths of digital evolution is its ability to support and compare a variety of selection-mutation regimes, including a “weak selection, strong mutation” regime (Adami et al., 2016) where mutants arise and co-exist in the population, leading to complex ecological dynamics.
3.3. Complex Genotype-to-Phenotype Map
Digital evolution systems include complex genotype-to-phenotype mappings, such that a single genotype can express different phenotypes depending on chance and the endogenous and exogenous factors affecting an individual organism at a given point in time. Because there are many possible genotypes, typically one phenotype can also be achieved by many different genotypes. In systems that support symbioses, symbionts can additionally affect the phenotype of a digital organism in complex ways.
3.4. Implicit Fitness
Finally, here we focus on systems in which organism fitness is implicit. That is, an organism's fitness is determined by its ability to survive and reproduce in its computational environment (as influenced by chance events, its phenotype, life history, environmental conditions, interactions with other organisms, etc.). Because an organism's fitness is dependent on its phenotype and there is a non-trivial mapping between genotype and phenotype, there is also a non-trivial relationship between an organism's genotype and its fitness.
There is a spectrum between explicit and implicit fitness, with difficult-to-categorize systems in the middle. However, this distinction excludes many evolutionary algorithms where an individual's fitness is explicitly assessed using external criteria based on its genome (e.g., a fitness or objective function) and an artificial selection algorithm determines which individuals serve as parents to contribute their genetic material to the next generation.
4. Benefits of Digital Evolution
Digital evolution serves as a valuable methodological bridge between traditional population-level mathematical models and biological model systems. In particular, digital evolution, like individual/agent-based modeling in general, is well-suited to discovering and testing novel theory, which can then enable more targeted and tractable experiments using biological organisms.
Traditional population-level mathematical models of evolution necessarily make simplifying assumptions to remain tractable and solvable. Individual-based modeling, in general, and digital evolution systems, in particular, relax many of these simplifying assumptions, including many that are relevant to the study of symbiosis. For example, digital evolution systems contain finite populations, whereas many traditional models must assume infinite populations. Additionally, many population-level models assume a strong-selection, weak-mutation regime, which can prevent multiple mutations from simultaneously coexisting in a population. In contrast, digital evolution systems are not constrained to particular mutation-selection regimes and many mutations can simultaneously coexist and interact in complex ways. Complex spatial structures are also difficult to represent in many population-level models; however, digital evolution systems support arbitrary spatial structures (e.g., Dolson et al., 2017). Finally, population-level models generally focus on only population-level dynamics and fail to capture organism-level details, such as an individual host of a particular phenotype specifically associating with an individual symbiont of a particular phenotype.
Biological model systems have their own set of limitations, including the expense in materials and human labor required. Digital evolution allows experimenters to observe hundreds of thousands of generations of evolution in hours or days, depending on the complexity of the organisms, virtual world, and the size of the population(s). Moreover, digital evolution experiments can be easily scaled up with increased computational power without the need for increased human labor or physical reagents.
Digital evolution systems also facilitate experimental manipulations and analyses that go beyond what is possible in laboratory experiments. For example, digital evolution experiments allow for precise control over basic parameters such as population size, mutation rates and sizes, and the exact trade-offs (or at least the mechanisms responsible for trade-offs) for partners in a symbiotic relationship. They also support analyses that would be prohibitively difficult with biological model organisms, including testing the entire lineage of a symbiont with one of its partner genotypes, eliminating mutations, and conducting comprehensive “knock-out” experiments investigating specific functionality.
The perfect control of digital evolution systems also means that they can have perfect replicability. While experiments in digital evolution systems typically have large amounts of randomness and noise, that randomness is controlled by a pseudo-random number generator that ensures that the exact sequence of random events can be perfectly recreated. Most currently-used digital evolution platforms are open source, meaning all of their computer code is publicly available, and therefore anyone is theoretically able to exactly recreate published findings. This combination of factors leads to an ideal medium for maximum replicability, scientific integrity, and transparency, which has been of growing concern to evolutionary biologists and ecologists (Lagisz and O'Dea, 2021).
Finally, digital evolution systems offer researchers the opportunity to study evolution in organisms that share no ancestry with carbon-based life (Ray, 1991; Wilke and Adami, 2002). All life on Earth is DNA or RNA based and likely descended from a single common ancestor. This shared history is a possible confounding factor, making it difficult to disentangle general principles of symbiosis from the possible artifacts of shared history and substrate (DNA and RNA). By reproducing results across biological and digital systems, we can disentangle general principles from effects specific to a particular model organism or planetary body (Wilke and Adami, 2002).
5. Symbiosis in Early Digital Evolution Systems
Computer viruses have long inspired the use of computers and computational agents as model systems for studying life (Spafford, 1994). From that inspiration, many digital evolution systems were created to better study evolutionary dynamics, and indeed, symbiosis—especially parasitism—is a recurring theme in early digital evolution systems. Here, we examine digital evolution's history, focusing our attention on systems that supported symbiosis and on systems that directly inspired symbiosis-enabled systems.
5.1. EVOLVE
The EVOLVE software system is one of the earliest examples of digital evolution (Conrad and Pattee, 1970; Conrad and Strizich, 1985; Rizki and Conrad, 1985; Brewster and Conrad, 1998). EVOLVE included environmental factors such as light intensity and temperature, as well as biologically-inspired organism genomes comprising up to 40 genes, each containing up to 200 nucleic acid bases. The organisms collected resources, which they then spent to avoid starvation and reproduce. O'Callaghan and Conrad (1992) introduced symbiosis in EVOLVE III by allowing organisms to gather resources from other organisms. The main symbiotic interaction they observed was parasitic, resulting in increased population size when symbiotic interactions were enabled because of the novel niche created.
5.2. RAM
Taylor and colleagues developed the RAM digital evolution system, demonstrating its use as a model system for studying lek formation among sage grouse, mosquito control under different environmental conditions, and predator-prey dynamics (Taylor et al., 1987). In subsequent work, Taylor and colleagues used the RAM system to study the ecological dynamics of the Hydra-Chlorella system (Taylor et al., 1989). In this system, 100 Hydra host organisms were represented with individual LISP computer programs and memory. Several algal organisms (represented with their own computer programs) were associated with each host. While the system would have been capable of evolutionary dynamics (there was reproduction with the possibility of mutation and competition for space), the experiments were not run long enough to demonstrate evolutionary timescales and the populations were very small due to computational limitations.
5.3. Core World
The inspiration for many modern digital evolution systems that are still actively supported and in use can be traced directly back to the 1984 computer game “Core War” (Dewdney, 1984). In Core War, human competitors used a simplified assembly language called Redcode to write “gladiatorial” computer programs that competed for space in the simulated core memory of a computer. To win a bout of Core War, a program had to shut down all of the processes associated with its competitor programs. Many of the most successful programs engaged in self-replication. Such replicator programs repeatedly created copies of themselves, each of which also repeatedly copied themselves ad infinitum. Thus, if one copy were to be destroyed by an adversary, other copies would still persist and continue replicating. Replicators could grow exponentially in memory, rapidly outcompeting other programs. To our knowledge, none of these replicator strategies engaged in any form of symbiosis. Despite having populations of self-replicating programs and competition, evolution did not occur in Core War because replicators always created perfect copies of themselves.
Inspired by Core War, Rasmussen and colleagues created Core World, in which they introduced the possibility for random mutations when a program copied itself (Rasmussen et al., 1989, 1990). The command used by replicator programs to copy themselves was imperfect, sometimes writing a random instruction instead of copying the intended instruction. With these mutations, Core World succeeded in facilitating the evolution of populations of computer programs. The Core World system, however, proved to be ill-suited for studying evolution. Programs written in Redcode were unlikely to survive mutations, and as such, quickly accumulated deleterious mutations that often drove the populations to extinction.
5.4. Tierra
Ecologist Thomas Ray's Tierra system (Ray, 1991) innovated on the design of Core World and facilitated some of the first successful evolution experiments with self-replicating computer programs. Genomes in Tierra were more evolvable than those in Core World, because mutated daughter programs were less likely to be broken. Unlike Core World, Tierra protected “living” programs from being overwritten by their competitors, requiring programs to explicitly request a protected block of memory into which they could copy themselves. When the population grew to the environment's carrying capacity, Tierra removed the oldest programs from the population to make room for new programs to be born.
In initial experiments using Tierra, Ray founded populations with an ancestral program capable only of self-replication (Ray, 1991). Competition for space dominated these early studies, resulting in a strong selection pressure for organisms to increase their replication rate. Ray observed organisms with shorter genomes, which could be copied more quickly, evolve and outcompete organisms with longer genomes, which took longer to reproduce themselves.
In Tierra, Ray unexpectedly observed the evolution of obligate parasites—programs that co-opted the copy machinery of their competitors to copy themselves. While Ray labeled these programs parasites, they did not directly harm their hosts. Instead, they competed for the same limited resource (space) as the “host” organisms, indirectly harming their host's fitness by preventing the host from being able to reproduce if the environment lacked sufficient space for the host's offspring. In this way, they could be thought of as brood parasites. In most experiments, Ray observed an evolutionary arms race between hosts and parasites. Would-be host programs evolved mechanisms for resisting parasites, and in turn, the parasites evolved to penetrate those defensive mechanisms.
Ray subsequently observed what he termed “hyper-parasites,” which parasitized the original indirect parasites. A hyper-parasite directly stole resources (CPU cycles) from its host by tricking the host into replicating the parasite's genome when the host attempted to replicate its own genome. These hyper-parasites eventually evolved to become obligate cooperators with other hyper-parasites, relying on their neighbors' copy machinery to successfully reproduce. Finally, cheaters emerged that did not contain the copy machinery, instead taking advantage of neighbors' copy machinery, and replicating more quickly as a result.
Mutualism was never observed to evolve de novo in Tierra. To test if it was possible in his system, Ray created an artificial mutualism by hand-coding two species that each contained only part of the necessary code to copy themselves and relied on one another. Such mutualists were able to persist, demonstrating that mutualism could exist in Tierra (Ray, 1992).
The richness of observed evolutionary dynamics in Tierra was initially surprising, given the simplicity of Tierra's environment. Research in digital evolution at this point in time, however, was mostly observational, mirroring the historical stage of biological research when it relied most heavily on careful observation due to the difficulty of experimental manipulation. The next stage in digital evolution research would come with Tierra's intellectual successor.
5.5. Avida
The Avida Digital Evolution Platform expanded on the design of Tierra, adding cross-feeding behavior and true parasitism, as well as a suite of sophisticated data tracking tools and the ability for researchers to configure complex environments (Adami and Brown, 1994; Cooper and Ofria, 2002; Ofria and Wilke, 2004; Ofria et al., 2009; Zaman et al., 2011). With this support, digital evolution progressed beyond observational studies of the natural history of virtual organisms to controlled experimental studies.
In Avida, self-replicating “digital organisms” compete for space on a lattice of grid spaces (Ofria et al., 2009). When an organism reproduces, its offspring is placed in a nearby space (or in a random space if the population is well-mixed), replacing any occupants of that space. As in Tierra, improvements to the speed of self-replication are advantageous during competition for space in the environment, and organisms in Avida can increase their replication rates by evolving greater replication efficiency (e.g., using a more compact encoding). Additionally, Avida introduced the concept of resources that can be “metabolized” by a digital organism to accelerate the rate at which it expresses its genome (i.e., its “metabolic rate”). Resources in Avida are associated with completing designated tasks, such as computing Boolean logic functions on inputs from the environment. Avida gives experimenters fine-grained control over how resources are configured, including their abundance (Cooper and Ofria, 2002), spatial distribution (Dolson et al., 2017), and metabolic effects (Canino-Koning et al., 2016, 2019).
Symbiosis was made possible in Avida when Zaman et al. (2011) added support for parasites. These parasites are obligate endosymbionts that steal a user-configured amount of resources (in the form of CPU cycles) from their hosts. Parasites can horizontally transmit their offspring to other hosts by copying their genome and then attempting to inject their offspring into another host. The injection is only successful, however, if the parasite performed one of the same tasks as the would-be host, similar to how bacteriophage target bacteria's outer-membrane proteins to enter a host (Kutter and Sulakvelidze, 2004).
Recently, Luo and colleagues introduced the possibility of cross-feeding into Avida (Luo et al., 2019). Organisms can create waste byproducts that can be further metabolized by other organisms. These byproducts are not spatially limited, however, and instead are accessible to all organisms in the world. This simplification therefore precludes the evolution of symbiosis via cross-feeding, because individual organisms cannot engage in close and long-term interactions with others in their vicinity.
6. Review of Previous Findings
As digital evolution techniques have matured, researchers have leveraged those techniques to make valuable contributions to the study of symbiosis' evolutionary origins and consequences. Their findings can generally be grouped into three categories that we review here: (1) symbiosis' effect on sustained population diversity, (2) symbiosis' effect on the evolution of complexity, and (3) the factors that select for the evolution of a parasitic or mutualistic symbiosis. A summary and comparison of the modern digital evolution systems that support symbiosis is presented in Tables 2, 3.
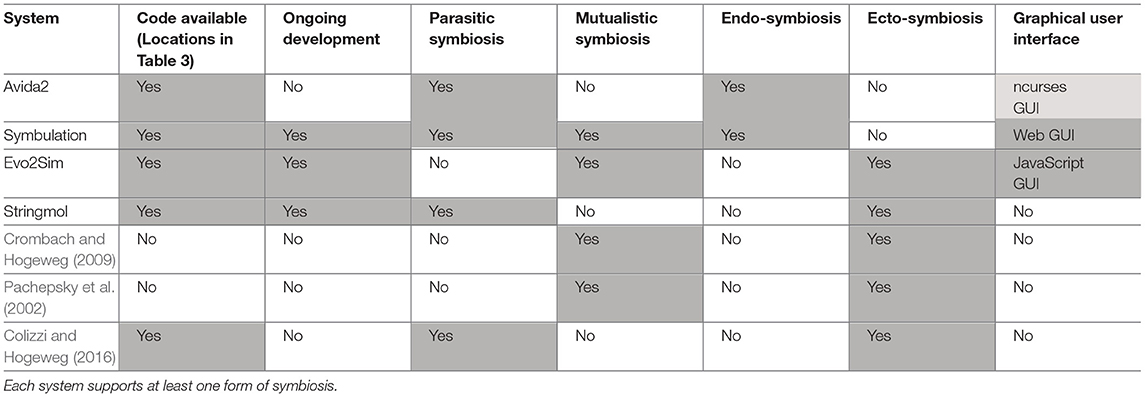
Table 2. A comparison of the digital evolution software systems used in the work reviewed in section 6.
6.1. Diversity
Digital evolution research has long featured studies of diversity, including symbiosis' role in its origins and maintenance (Ray, 1992). For example, Ray (1992) evolved two populations of digital organisms, introducing a parasite to one population but not the other to determine how host-parasite coevolution influenced diversity. After the evolutionary run, Ray observed that the population without parasites contained eight “species,” whereas the population with parasites contained sixteen species. However, Ray did not further investigate why the addition of parasites doubled the final diversity sustained in the population.
6.1.1. Parasitism
Parasites are hypothesized to be able to increase host diversity through several mechanisms (Brockhurst et al., 2004). Theoretically, parasites will experience selection to infect the most prevalent host species (all else being equal), which then could decrease that host species' fitness and enable other species to increase in prevalence, leading again to pressure for the parasite to target the now most prevalent host (Benton, 2009). Beyond such eco-evolutionary dynamics, parasites may pressure hosts to rapidly evolve, potentially resulting in faster host diversification than would otherwise be expected (Bérénos et al., 2011). However, empirically disentangling the particular mechanisms underlying host diversification requires the ability to manipulate both host-parasite interactions and the potential for novel variation, which can be prohibitively difficult in biological experimental systems.
Zaman et al. (2011) used the Avida Digital Evolution Platform to investigate the effects of rapid evolution on host-parasite diversity. Zaman and colleagues found that parasites increased host diversity through a combination of ecological and rapid evolutionary dynamics. Specifically, they found that:
1. Host populations that co-evolved with parasites had significantly more diversity over long timescales than hosts not co-evolving with parasites.
2. When all mutations were prevented (after an initial period of evolution), host populations with parasites were still more diverse than populations without parasites.
3. The presence of parasites significantly amplified the diversity generated from novel variation (mutations).
These results indicate that the presence of co-evolving parasites interacts with novel variation (via mutations) to increase host diversity through rapid evolutionary dynamics beyond what would be expected from novel variation or parasites alone.
From those findings, the question remained: what causes that interaction between co-evolving parasites and novel variation? Novel variation via mutations can be split into adaptive and non-adaptive mutations. To answer this question, it was necessary to determine what role the non-adaptive mutations play in the increase of host diversity in the presence of parasites. Digital evolution systems such as Avida are ideal for gaining insight into this dynamic because they can programatically prevent non-adaptive mutations, allowing researchers to determine what effect non-adaptive mutations have on host diversity. Fortuna and colleagues pursued this question by experimentally preventing non-adaptive mutations in hosts co-evolving with parasites (Fortuna et al., 2017). They found that, when only adaptive mutations were allowed, host phenotypic diversity was significantly lower than when both non-adaptive and adaptive mutations were allowed. This result indicates that non-adaptive mutations play a significant role in the host phenotypic diversity resulting from co-evolution with parasites.
In both Zaman et al. (2011) and Fortuna et al. (2017), hosts were limited to nine different behaviors to obtain resources, which, in turn, limited the ways in which parasites could infect hosts. This limit is not realistic and raises the question: what level of diversity could evolve in a population with more possible resource-rewarding behaviors? To answer this question, Zaman updated the Avida digital evolution system to support many more resource-uptake behaviors. Zaman also increased the carrying capacity of the Avida world to determine if there was an upper bound to the diversity the system could support (Zaman, 2018). He found that, when the population carrying capacity was increased, the evolved host and parasite population diversity increased with more possible behaviors in an unbounded trajectory. This result indicates that the diversity capable of being supported in the Avida digital evolution system with parasites is likely much greater than previously seen.
6.1.2. Mutualism
Much of the focus of previous research has been on parasites' effect on host diversity. However, mutualistic symbioses can also impact species diversity. For example, cross-feeding is an ecological interaction where one organism produces a waste byproduct that another organism is able to metabolize (Seth and Taga, 2014); if two organisms from separate species are able to metabolize one another's waste products, they can form a mutualistic symbiosis. This mechanism has been previously used to construct engineered biological symbioses (Momeni et al., 2011) and has been studied with several digital evolution systems.
Rocabert et al. (2017) demonstrated the importance of temporal variability (through batch culture conditions) to the evolution of a one-way cross-feeding relationship in the digital evolution system Evo2Sim. Organisms in Evo2Sim consist of genomes that contain “genomic units” that can be non-coding, promoters, or enzyme coding units. These genomic units determine the metabolic network of the organism, leading to a complex interplay of various concentrations of metabolites both inside and outside of the organism and different proteins determining the flow of those metabolites. Specific essential metabolites determine if an organism is able to reproduce when a space is cleared near them (through the death of neighboring organism), however there is a threshold of any metabolite that is lethal to the organism. The metabolites produced by an organism diffuse at a user-controlled rate, enabling the possibility of one organism relying on the nutrients output by a neighboring organism (and therefore decreasing the toxicity in the space), leading to mutualistic symbiosis.
Rocabert et al. (2017) used this system to investigate specific dynamics that had been observed in the Long-Term Evolution Experiment (LTEE, Rozen and Lenski, 2000; Elena and Lenski, 2003). Two ecotypes had been observed, one (L) that grows on the primary nutrient provided, and another (S) that grows on the by-product produced by Rocabert and colleagues investigated how this cross-feeding relationship could avoid breakdown through competitive exclusion and remain stable over evolutionary time periods. They demonstrated that temporal variation in the amount of primary resource availability was critical to the stable co-existence of these two ecotypes, such as in batch culture conditions. In batch culture conditions, the ecotype that fed on the primary resource, which they called A, grew rapidly when fresh resources were introduced periodically. However, organisms of type A soon ran out of resource and a toxic amount of by-product started to build up. This build-up enabled organisms of type B, which could digest A's by-product, to proliferate. Environments that lacked this resource seasonality, such as a chemostat, had brief appearances of type B, but the symbiosis was never stable because organisms of type A were able to eventually outcompete organisms of type B. In the chemostat environment, type A organisms did not need type B to decrease the toxicity because byproducts were cleared from the system, preventing a mutualistic symbiosis from forming.
These results demonstrate that mutualistic symbiosis through cross-feeding can increase the diversity of species or ecotypes in a system, but only when the environment is such that both ecotypes suffer in the absence of the other. In the absence of a detrimental fitness affect without the partner, competitive exclusion will cause the cross-feeding relationship to be unstable over evolutionary timescales.
While the previous study focused on only two ecotypes, Crombach and Hogeweg (2009) investigated the dynamics of resource cycling by individual organisms and groups by enabling coevolution of many ecotypes in a digital evolution system. In their system, organisms were able to sense types of resources (in the form of bitstrings) near them and were able to metabolize the resource by outputing a matching bitstring. This process changed the resource to another type, allowing resources to cycle between many types over time. There was the potential for mutualistic symbiosis through local cross-feeding if a species evolved to rely on another species to change a resource to a type the first species could better metabolize. However, there was also the possibility that organisms would instead evolve to be generalist consumers and not rely on others.
Many of Crombach and Hogeweg (2009) investigations were not focused on the effect or evolution of mutualistic symbiosis. Instead they were focused on the difference between the null model, where organisms were randomly moved around, and the local model, where organisms maintained a consistent position. When organisms were not randomly moved, they had both consistent partners and consistent resources. Therefore, they couldn't separate the effect of symbiosis from the effect of the organisms interacting with consistent resources.
However, Crombach and Hogeweg (2009) did confirm mutualistic symbiosis through local cross-feeding evolved by verifying in some experiments that some organisms were dependent on others for resources. This relationship evolved only when there was strong direct selection on organisms to be able to process local resources. Local cross-feeding did not, however, cause a meaningful or significant difference in population diversity from non-symbiotic population-level cross-feeding in the null model. This result indicates that mutualistic symbiosis will not necessarily increase population diversity, especially when other dynamics dominate a system.
Finally, Pachepsky et al. (2002) focused specifically on the effect on population diversity of mutualistic symbiosis through cross-feeding. In this digital evolution system, when an organism digested resources, they produced waste of the resource types that they took in but could not digest in their local area. Therefore, organisms that were located near each other and happen to be able to digest different resource types could evolve a mutualistic symbiosis through cross-feeding in this system. Because this was a digital evolution system, the effect of cross-feeding mutualisms on diversity could be directly measured by running control treatments without the production of predictable waste byproducts (instead a random byproduct was produced), thus guaranteeing that cross-feeding mutualisms could not evolve while maintaining the amount of resources in the environment. They found that, when organisms produced consistent waste byproducts, mutualistic symbioses were able to evolve and a larger number of phenotypes were able to persist in the environment. This result was found to be independent of whether novel variation through mutation was enabled or disabled.
Pachepsky et al. (2002) also found that the total number of organisms in the population was actually lower with cross-feeding mechanisms enabled. This was likely because the organisms specialized and became dependent on their symbiotic partners, limiting their population growth. Communities with cross-feeding were also found to be more stable after an environmental perturbation (in the form of a change in the type of resource coming into the system) than communities without cross-feeding. This result was due to the communities with cross-feeding being able to digest a wider range of resources as a whole than communities without cross-feeding.
6.1.3. Diversity Summary
Taken together, the research on population diversity using digital evolution systems with symbionts indicates that both parasitic and mutualistic symbioses can increase diversity through the creation of new niches with or without novel variation (through mutation). When the symbionts are parasitic, the fitness benefits of escaping from them create novel niches for hosts. However, when symbionts are mutualistic, it is the reliance on the partner that creates the novel niches.
The relationship between diversity and population size is more complicated. An increased carrying capacity, along with a sufficient number of possible behaviors in the presence of parasites, can lead to an unbounded increase in diversity. However, a mutualistic symbiosis can limit population growth because of the partners' dependence on one another. This limit on population growth may mean that mutualistic symbiosis eventually limits or slows the diversity achievable in a population.
Finally, none of the digital evolution systems used in the reviewed studies allowed for the evolution of symbiosis along the parasitism-mutualism spectrum. Determining how such evolution interacts with diversity remains an open question (discussed further in section 7).
6.2. Complexity
Complex traits are found everywhere in nature, from the complex organs such as eyes that first concerned Darwin, to the many biochemical pathways that allow each of us to function (Goldsmith, 1990; Szostak et al., 2001). However, many questions remain surrounding the processes by which this complexity could have arisen (Simpson and Simpson, 1949; Dawkins, 1997; Szostak et al., 2001; Shanahan, 2004; Lynch, 2007; Gould, 2011). In particular, there is no inherent link between complexity and fitness: if an organism is needlessly complex, its fitness will likely suffer. The question is then: why do some niches favor organisms with more complex traits?
Methodological challenges in biological systems have severely limited experimental testing of hypotheses for how complexity could have arisen. Observational studies of historical data are unable to determine causation, and experimental evolution studies require extraordinary time and resources due to the timescales necessary for complexity to evolve (Dawkins and Krebs, 1979; Hazen et al., 2007). There also is not firm consensus on an objective way to measure the complexity of biological organismal traits, leading to rhetorical arguments that can further obscure and delay empirical research (Adami et al., 2000; Adami, 2002).
Digital evolution systems are able to overcome this difficulty by limiting possible behaviors to those that are easily quantified as more or less complex, such as the exact size of the genome needing to be replicated (Liard et al., 2020) or the number of basic operations necessary to complete a logic task (Lenski et al., 2003). Digital evolution systems are also able to evolve hundreds of thousands of generations of organisms in days or weeks while keeping a perfect genealogical record.
The most basic functionality necessary for the evolution of complexity is the ability to replicate. In the RNA World Hypothesis, however, this replication was not self-replication, but instead replication of other RNA strings (Gilbert, 1986). This form of replication raises the issue of parasitic or cheater strings, as discussed by Colizzi and Hogeweg (2016), that are copied frequently, but do not in turn replicate other strings and thus lead to the whole system breaking down. However, Colizzi and Hogeweg (2016) used a digital evolution system with RNA-inspired replicators to demonstrate that, in the presence of spatial structure, these parasitic strings may actually increase the overall replication efficiency of a population of replicators.
The digital evolution system designed by Colizzi and Hogeweg (2016) consists of a population of replicators, which cannot replicate themselves, but instead must form “complexes” with a neighboring replicator to facilitate the replication of one of the strings. Which string is actually copied depends on characteristics of the replicators involved, meaning that parasitic or cheater string types can evolve that are copied more often than other types. Note that, like Ray's original “parasites” discussed in section 5.4, these parasites are more similar to brood parasites, since they do not directly harm the host and instead their offspring compete with the host's offspring for resources and space.
With this system, Colizzi and Hogeweg (2016) experimentally determined the effect of parasites and spatial structure on the evolution of replication and complexity. They found that, in the presence of spatial structure, the presence of co-evolving parasites actually increased the replication efficiency and complexity of the “host” strings. This result was due to the parasites dying out in small patches, leading to pressure on the surviving strings to replicate quickly to take advantage of these patches.
Hickinbotham et al. (2021) further investigated the evolution and effects of parasites in a digital evolution RNA world using an automata chemistry system, Stringmol (StringMol, 2021). They found that the presence of co-evolving parasites led hosts to evolve increasingly complex strategies to differentiate themselves from parasites as well as defend against parasites.
In Stringmol, replicators are a string of computer programming instructions that are not capable of replicating themselves (StringMol, 2021). Instead, they must bind to another string and copy that string, which may be a copy of themselves. Like Tierra (Ray, 1991), Stringmol strongly favors the evolution smaller organisms (those with fewer instructions to copy) as well as replicators that are more frequently the string copied instead of the string doing the copying, which Hickinbotham and colleagues termed parasites. However, again, these “parasites” do not directly harm the host and instead their offspring compete with the host's offspring, a process reminiscent of brood parasites in the natural world. In addition, replicators randomly engage with other replicators nearby instead of engaging in a lifelong symbiotic interaction with one other individual (Hickinbotham et al., 2021). Because organisms do not move, there is high likelihood of repeated interactions between two specific organisms. Thus, the interactions in this system are on the edge of parasitic symbiosis.
Hickinbotham and colleagues found that the evolution of parasites could have several different effects on the evolution of complexity of the host replicators (Hickinbotham et al., 2021). On one extreme, the evolution of parasites frequently caused the population to go extinct (12 out of 20 populations). For the remaining eight, the population typically increased in size due to an evolved increase in replicating efficiency. In addition, host replicators evolved several strategies for combating parasites:
1. Reduced replication rates of hosts decreased the competitive advantage of parasites because parasites relied on hosts to replicate them.
2. Introduction of fixed reproductive costs further decreased the competitive advantage of parasites because the relative cost was less for other hosts than for parasites.
3. Increasingly complex mechanisms for discriminating self from parasites enabled hosts to avoid copying parasites.
In addition to the effect of parasites on host evolution, Hickinbotham and colleagues also observed interesting dynamics in parasite evolution. Instead of separate parasite lineages as is often assumed, new parasites in Stringmol continually arose from mutated replicators (Hickinbotham et al., 2021). They suggest that novel parasites may flourish because the features they inherit from their immediate replicator ancestor may allow them to circumvent that replicator's defense system. When the replicator subsequently evolves effective defenses against these parasites, the parasites die out and are replaced by newly parasitic offspring of the replicator. They point out that if these observed dynamics of parasite evolution hold in the prebiotic RNA world, that could lead to significantly different outcomes than expected from co-evolving independent host and parasite species (Hickinbotham et al., 2021).
While the previous articles focused on ectosymbiotic parasites' effect in a prebiotic system, Zaman et al. (2014) focused on the effects of endosymbiotic parasites on the behavioral complexity of self-replicating hosts. They hypothesized that co-evolving symbionts could influence the evolution of host complexity in two ways. First, based on the Red Queen Hypothesis, they hypothesized that the presence of co-evolving parasites could directly select for increased complexity. The Red Queen Hypothesis posits that co-evolving species can be in an arms race that forces them both to continually evolve to compete with each other (Dawkins and Krebs, 1979).
However, there is no inherent reason that escalation must occur (Thompson, 2005a,b), leading to Zaman and colleagues' second hypothesis: “genetic memory” can lead to the evolution of more complex strategies (Zaman et al., 2014). Zaman and colleagues point out that an arms race could be cyclical, such that the species involved cycle between two or more strategies of equal complexity. This cycle is prevented if one or both species retain a “genetic memory” of strategies that counter all previously encountered partners, forcing their opponents to continually evolve novel strategies to compete. If there are a limited number of unique simple strategies in a symbiotic system, they will eventually be exhausted. Once this occurs, the populations must evolve more complex strategies if they are to continue adapting.
Using the same parasites in the Avida digital evolution system as discussed in the previous section, Zaman and colleagues leveraged the clear definition of complexity in the Avida system to determine how parasites affect hosts' evolution to perform complex tasks (Zaman et al., 2014). In this system, hosts were under pressure to escape their parasites by changing their resource-gathering behavior. Hosts could do this by simply cycling between several simple behaviors if their parasites lost the ability to infect extinct hosts (i.e., lacked “genetic memory”).
Zaman and colleagues evolved populations both with and without parasites and recorded the complexity of the logic tasks the organisms evolved. Note that in these experiments, as opposed to the default configuration, there was no direct benefit to becoming more complex. Instead, a benefit for increased complexity could arise as a byproduct of co-evolving with parasites. They found that host populations with co-evolving parasites evolved significantly more complex behavior than hosts evolving without parasites. This increase in complexity could have been due to the step-wise escalation (i.e., arms race) between hosts and parasites, or it could have emerged because parasites were exerting some additional pressure on the hosts not to cycle back to previously evolved phenotypes.
To determine the cause of the increased host complexity, Zaman and colleagues experimentally manipulated the Avida system to eliminate any advantage to parasites in retaining a broad host range (i.e., their “genetic memory”) and reran their experiment. If the hosts had evolved increased complexity due only to the arms race with parasites, then they should have reached the same level of complexity. However, hosts evolved under this experimental design evolved significantly less complexity than hosts evolved with normal co-evolving parasites. In fact, these hosts were indistinguishable from host populations evolved without parasites. These results indicate that it was the diversity of co-evolving hosts and parasites that caused the parasites to retain a broad host range (a “genetic memory”), creating selection for increasingly complex hosts. Zaman and colleagues termed this the Population-Genetic Memory Hypothesis (Zaman et al., 2014).
Co-evolution with parasites could also affect the evolution of complexity via evolution of increased evolvability to escape those parasites. Zaman et al. (2014) were able to conduct an evolvability analysis by mapping every phenotypic change to every possible point mutation for the most prevalent host genotype from all populations of hosts with and without parasites at the end of the evolution experiments. They found that function-switching mutations were >10-fold more common in hosts that evolved with parasites than in hosts that evolved without parasites. This result indicates that co-evolving with parasites indirectly selected for more evolvable genomes, because hosts were then more likely to avoid infection.
Overall, these findings indicate that the presence of parasites can cause an increase in the complexity and evolvability of hosts. They also demonstrate the value of using digital evolution in examining these questions, because it enables increased control of possible complex behaviors and analysis of mutational effects.
However, previous work in digital evolution has not investigated the effect of mutualistic symbionts on the evolution of complexity, nor how endosymbiosis affects the evolution of the symbionts. These and other open questions are discussed in section 7.
6.3. Parasitism-Mutualism Spectrum
The research discussed in the previous two sections has focused on the effect of a strictly parasitic or mutualistic symbiosis on various aspects of population dynamics. However, symbionts can evolve along a spectrum between parasitism and mutualism. Another important question is then: what influences that evolution? Two hypothesized influences are the vertical transmission rate and spatial structure. Vertical transmission (when a symbiont's offspring are able to infect a host's offspring) is hypothesized to align the reproductive interests of symbiont and host, because the symbiont's fitness can benefit from increases in the host's fitness (Shapiro and Turner, 2014). However, experimentally manipulating vertical transmission rate over evolutionary timescales can be difficult or impossible in many biological systems. Spatial structure (when offspring remain spatially close to their parents instead of dispersing away) has generally been found to increase the selection for cooperation, because it enables cooperators to stay near related organisms who are likely also cooperators, making cooperation both more beneficial and more resistant to cheating organisms (Yamamura et al., 2004). Part of the effect of spatial structure on cooperation, however, relies on kin selection (where a benefit to related organisms increases a focal organism's indirect fitness) and may not apply directly to cooperation between organisms of different species (Griffin and West, 2002). Therefore, determining experimentally the effect of these two factors on the evolution between parasitism and mutualism has historically been challenging.
To overcome this difficulty, Vostinar and Ofria created a simple digital evolution system to investigate these dynamics (Vostinar and Ofria, 2019). The Symbulation system currently supports a population of host organisms and a population of obligate endosymbionts. Organisms reproduce when they accrue enough resources, making fitness implicitly defined. An organism's phenotype depends on both its own genotype and its partners, and there are many possible genomes. In addition, organisms can evolve behavior along a spectrum from antagonistic (where the symbiont is parasitic and the host is defensive) to mutualistic (where both partners actively contribute resources to the other).
In this system, Vostinar and Ofria experimentally manipulated vertical transmission rate and whether offspring were placed immediately next to their parent or randomly in the world. They found that both with and without spatial structure, vertical transmission rate did increase selection for mutualistic behavior between the host and symbiont when there was sufficient starting variation in the population. However, regardless of vertical transmission rate, mutualism did not evolve when the hosts and symbionts started from an antagonistic relationship and parasitism did not evolve when the hosts and symbionts started from a mutualistic relationship (Vostinar and Ofria, 2019).
While the effect of vertical transmission rate was independent of spatial structure, Vostinar and Ofria found that the effect of spatial structure was not independent of the vertical transmission rate (Vostinar and Ofria, 2019). At high and low amounts of vertical transmission, the presence of spatial structure increased the amount of mutualistic behavior or decreased the amount of antagonistic behavior, respectively. However, at intermediate values of vertical transmission, the presence of spatial structure significantly decreased the amount of mutualistic behavior, in some treatments to the point that an antagonistic relationship evolved instead. Vostinar and Ofria hypothesized this to be due to a decreased amount of local symbiont diversity, which caused a reduction in the average mutualistic quality of symbionts that could infect naive hosts, selecting against mutualistic behavior (Vostinar and Ofria, 2019). These results indicate that vertical transmission does select for mutualistic behavior, but it is insufficient on its own, and it has complex interactions with spatial structure and population diversity which warrant further study as discussed in the following section.
7. Discussion and Future Directions
As detailed in the previous section, there have been several exciting breakthroughs regarding the evolutionary dynamics of symbiosis that were made possible by digital evolution systems. However many questions remain, some of which current systems could investigate, and some of which will require expansion of current systems or new digital evolution systems altogether. A number of previous reviews raise many interesting questions regarding the evolution of symbiosis (Daida et al., 1996; Bergstrom et al., 2003; Auld and Tinsley, 2015; Strona, 2015; Drew et al., 2021). Here, we focus on those that digital evolution is particularly well-suited to address.
In this section, we first discuss how digital evolution results can be compared to results from biological systems. We then discuss specific questions that digital evolution systems could be used to address. These questions are grouped into two categories: (1) the effects that a co-evolving symbiont has on its partner's evolutionary trajectory and vice versa and (2) the factors that select for the initial evolution of the varying forms of symbiosis. Finally, we detail the gaps in current systems that are most in need of implementation to answer these pressing questions.
7.1. Digital Evolution Results in Relation to Biological Systems
As discussed in section 3, digital evolution systems typically do not attempt to simulate specific biological systems. Instead, they aim to find general processes and patterns likely to also be found in biological systems. Because of this, digital evolution can be viewed as a faster and less expensive way to initially test hypotheses about evolutionary processes. If the results found in digital evolution systems are promising, researchers may then choose to invest the time and resources to test if the results are also observed in biological systems.
The insights gained regarding symbiosis in digital evolution systems have impacted the biological literature several times. For example, Rocabert et al. (2017)'s findings were cited by Zhao et al. (2019) to potentially explain dynamics observed in human gut microbiomes, and Crombach and Hogeweg (2009)'s findings were included in Hindré et al. (2012), which reviewed bacterial adaptive dynamics. Finally, Zaman et al. (2014) has been quite influential on research regarding bacteriophage-host evolutionary dynamics (Masri et al., 2015; Burmeister et al., 2016; Stroud and Losos, 2016; Bento et al., 2017; Queller and Strassmann, 2018; Furubayashi et al., 2020; Ryan, 2020).
However, it is not necessarily possible or practical to replicate experiments from digital evolution in biological systems with current technology. For example, preventing any mutations from occurring, or only allowing mutations of a certain type, while allowing the populations to continue otherwise as normal is currently impossible in biological systems. Other forms of analysis are also currently infeasible, such as collecting a complete genetic sequence on every individual organism, identifying all mutations that have occurred in all populations, and determining what effects (if any) those mutations had (such as the evolvability analysis done by Zaman et al., 2014).
Further, the goal of many digital evolution systems and studies is to create an instantiation of evolution, not a simulation. Therefore, results do not need to be verified in biological systems, because they are accurate observations of the system in which they were found, in the same way that results from any particular model biological system are valid observations of the model system. As with any biological system, it is possible for the results in a digital evolution system to be due to artifacts or idiosyncrasies of the system. Ultimately, the aim is usually to find generalizable processes and patterns, and the only way to know if a process is generalizable is to look for it in many systems, both digital and biological.
7.2. How Does the Presence of a Co-evolving Symbiont Alter Host Evolutionary Directions and Vice Versa?
The first set of open questions we will discuss focuses on the interaction between co-evolving symbionts. The presence of a co-evolving symbiotic partner is likely to influence the evolutionary dynamics of a species in many ways. Here we discuss eight dynamics—complexity, diversity, competition, sexual reproduction, eusociality, range expansions/shifts, and open-ended evolution—that have been hypothesized to be influenced by a co-evolving symbiont and are well-suited to being studied further using digital evolution.
7.2.1. Complexity
As discussed previously, evolution in biological systems has produced a plethora of incredibly complex traits, leading to the question: what mechanisms selected for such complex traits? Previous research (detailed in section 6) determined that the presence of co-evolving parasites can cause hosts to evolve greater complexity due to population-genetic memory (Zaman et al., 2014). However, that work focused solely on obligate parasites; other forms of symbiosis could influence the evolution of complexity in many ways as well.
Outside of our definition of digital evolution, previous research has leveraged the idea of “symbiotic scaffolding” to evolve organisms able to solve mathematical problems (Watson et al., 2000). The hypothesis of symbiotic scaffolding is that a mutualistic symbiont can provide functional scaffolds, enabling a host to evolve more complex traits before then being under selection to evolve away from dependence on the symbiont. However, it is an open question if the host would actually evolve away from depending on its symbiotic partner (and what conditions would select for that outcome) (Moran, 2007). Another possible outcome could be that the presence of a mutualistic symbiont would select against increased beneficial complexity in the host, because the symbiont is already providing that benefit. The presence of a mutualistic symbiont could also select against the evolution of further complexity in the host if otherwise beneficial mutations interfered with the symbiotic mechanisms.
Finally, many observations of biological systems have noted a loss of complexity due to evolutionary degradation in obligate endosymbionts (McCutcheon and Moran, 2012; Bennett and Moran, 2015). This could be due to several possible factors, including decreased selective pressure on vertically transmitted endosymbionts, decreased opportunities for endosymbionts to purge deleterious mutations, reduced need for certain costly genes (The Black Queen Hypothesis, Morris et al., 2012; Mas et al., 2016) and increased protection for endosymbionts from competition and predation in the sheltered environments their hosts provide. Digital evolution systems capable of similar processes with symbiosis may be helpful in determining general patterns, such as when degradation can occur and what factors could prevent it.
7.2.2. Diversity
As discussed in section 6, previous results have shown that greater phenotypic diversity emerges when parasitic or mutualistic symbionts coevolve than when the two species evolve in isolation. Similar dynamics are likely to occur at different time and spatial scales, leading to greater species-level diversity as well. However, because digital evolution systems typically focus on asexual organisms, the separation between species-level and phenotype-level diversity within these systems is often ambiguous and unclear. Therefore, the term “diversity” in this section refers to both species-level and phenotype-level diversity.
Beyond the previously discussed scenarios, there are also situations in which the presence of symbionts is expected to decrease the amount of diversity a population evolves. For example, mathematical models have shown that, if there is a cost to deleterious mutations, a host species co-evolving with parasites may evolve to have a mutation rate of zero, decreasing diversity of the host population, while the parasite mutation rate increases (Haraguchi and Sasaki, 1996). However, a higher mutation rate in hosts could enable them to escape co-evolving parasites and would likely lead to higher host and parasite population diversity (Haraguchi and Sasaki, 1996). Therefore, further investigation is needed to determine how each of these competing pressures on the mutation rate of hosts influences the amount of host population diversity.
On the other side of the symbiosis spectrum, previous work has demonstrated that mutualistic symbionts can increase diversity if they enable new niches (Pachepsky et al., 2002). However, dependence on mutualistic symbionts may restrict further evolution and population growth, thereby decreasing diversity in the long term compared to populations without mutualistic symbiosis. Other mutualistic symbioses may actually be driven by mutual exploitation, and so there may be pressure for the partners to escape each other (Vidal and Segraves, 2021). Which factors influence these two outcomes is an open question.
Further, previous work has shown that having an obligate relationship with a symbiotic partner could make a species more vulnerable if that partner begins to struggle due to environmental change, as is hypothesized to be the case in numerous biological systems including coral reefs (Hughes et al., 2018; Husnik and Keeling, 2019). Early digital evolution work observed cascading extinctions in a population of symbiotic organisms, but not in a parallel population of non-symbiotic organisms (O'Callaghan and Conrad, 1992). Other work on non-symbiotic cross-feeding in digital evolution has examined the effect of mass extinctions (Luo et al., 2019), and future work that enables symbiotic mutualism would be valuable to determine the robustness of such populations. Given there are potentially conflicting mechanisms acting on diversity in the presence of mutualistic symbiosis (novel niches and vulnerability to extinction), determining how these mechanisms interact in various systems would be beneficial, especially considering the growing concerns of mass extinction in Earth's biosphere.
7.2.3. Competition
The Escape-from-Enemy/Parasite-Escape hypothesis is the idea that some invasive species may be successful because they have escaped their natural parasites and pathogens (Wolfe, 2002). This dynamic is one example of how the presence (and then sudden absence) of symbionts can affect competition between hosts. However, over evolutionary timescales, there could be selective pressure for parasites in the novel environment to evolve to infect the invasive host if it is similar enough to an existing host (Chalkowski et al., 2018; Kołodziej-Sobocińska et al., 2018). In the presence of competing hosts, a generalist parasite can even be beneficial to a host species if it did more harm to a competitor (termed “apparent competition”), as hypothesized to occur in some bacteriophage/bacteria systems (Jones et al., 2020). Determining when each of these dynamics is likely to occur is a fruitful area of future research using digital evolution.
Mutualistic symbioses have somewhat more complicated effects on competition. Invasions of species that compete with the mutualists's hosts present a new opportunity for the symbiont population, but this opportunity also risks further displacing their original host species. Because evolution is short-sighted, exploiting opportunities to infect an invasive host species could come at a long-term detriment to the symbiont's population. How competition is realized when mutualistic interactions are faced with these opportunities and/or challenges is an open question.
Finally, there is a class of ectosymbiotic relationships where a symbiont is able to interact with multiple hosts simultaneously, most notably root mycorrhizae (Simard and Durall, 2004). There are many hypotheses about how these symbionts modulate competition between hosts, because the symbiont could gain the most benefit when all of its hosts are successful (Lareen et al., 2016). Exploring the evolutionary effects of ectosymbionts on hosts in competition, and especially the differential effects when those ectosymbionts are parasitic or mutualistic, is an exciting possible direction for digital evolution research, though it will require significant development in the existing digital evolution systems.
7.2.4. Asexual to Sexual
Sexual reproduction is a ubiquitous strategy in biological organisms. However, it has several costs associated with it (Lehtonen et al., 2012), including (1) time and resources spent finding a mate; (2) the presence of males that cannot produce offspring slowing population growth in species without hermaphroditism; and (3) only half of an individual's genes make it into each offspring. One hypothesis for why sexual reproduction has been such a successful strategy despite these costs is that it enables rapid evolution to escape from parasites (Hartfield and Keightley, 2012). Lynch et al. (2018) experimentally demonstrated that the presence of parasites favors sexual recombination in Caenorhabditis elegans.
Historically, the default life-history in digital evolution systems has been asexual reproduction, partly because it is much easier to implement and analyze. However, some attempts have been made to introduce sexual reproduction into digital evolution systems and determine what factors select for its evolution and maintenance. These investigations have found that rapidly changing environments do select for sexual reproduction (Misevic et al., 2010). However, to the best of our knowledge, coevolution with parasites has never been sufficient to favor sexual over asexual hosts in Avida.
It has also been observed that some parasites have retained the ability to facultatively engage in sexual reproduction (Heitman, 2006). This strategy could enable them to spread rapidly via asexual reproduction when that is most beneficial, and explore genetic space rapidly with sexual reproduction when conditions are less favorable. This is another hypothesis that would be ideal to study in a digital evolution system that supports sexual reproduction.
7.2.5. Eusociality
Many organisms live in groups of various sizes (Krause et al., 2002). This lifestyle facilitates the spreading of both parasitic and mutualistic symbionts (Sarkar et al., 2020). It has been hypothesized that the presence of mutualistic symbionts could actually select for group living because of the benefit of gaining the best symbionts (Lombardo, 2008). However, in most situations, parasitic symbionts would likely also be present and potentially selecting against group living. Therefore, experimentally determining the viability of these hypotheses would be beneficial. Digital evolution systems have been used to explore cooperation within species extensively (Goldsby et al., 2012; Frenoy et al., 2013; Moreno and Ofria, 2019; Vostinar et al., 2019), so they would be well-suited to introducing mutualistic and parasitic symbionts to determine their effect.
7.2.6. Range Expansions and Shifts
Symbionts can evolve to either expand or shift which hosts they infect, and many factors likely influence those evolutionary dynamics, including co-evolution with the original host(s) (Gupta et al., 2021). A host range expansion is an example of a generalist strategy in which the symbiont is able to infect the new host while maintaining its ability to infect the original host. A host shift is a specialist strategy in which a subset of the symbiont group speciates, evolving the ability to infect the new host while losing the ability to infect the original host. Predicting the likelihood of these events is especially important when considering parasitic symbionts, because these evolutionary dynamics play a role in a pathogenic spillover (Longdon et al., 2014). Pathogenic spillovers are important across fields including human and veterinary medicine (Longdon et al., 2014), agriculture (Sylla et al., 2019), and conservation (Yadav et al., 2019). Many of the fundamental evolutionary dynamics underpinning host range expansions and shifts, however, are only partially understood. For example, Fortuna et al. (2019) found that whether bacteriophage (viruses that infect bacteria) evolved to be generalists or specialists was strongly influenced by the amount of resources available over time.
There are limitations inherent in biological coevolution studies that can be overcome in digital evolution and would enable a better understanding of otherwise indistinguishable dynamics. For example, digital evolution allows us to observe pathogenic spillovers not only as they have happened (as in biological studies), but also how they could happen, and understand the factors that contribute to them. Further, as discussed in section 4, digital evolution grants the researcher fine-grained control over the digital organisms' mutation rates, inter- and intraspecies contact rates, and genomes, enabling a more controlled and thorough analysis.
The presence of co-evolving symbionts could also affect the host's realized niche (Jones et al., 2020; Hoang et al., 2021). For example, the presence of co-evolving parasites could select for a change to the host's geographical distribution to escape those parasites. Conversely, mutualistic symbionts that provide additional defensive or metabolic traits could enable hosts to expand their realized niche (Hoang et al., 2021). Digital evolution systems that support perfect control of each of these varying possible effects will be instrumental in determining how such dynamics could play out.
7.2.7. Open-Ended Evolution
Finally, a recurring question in digital evolution is what conditions are necessary for open-ended evolution to occur. As detailed by Taylor et al. (2016), the definition of open-ended evolution is not settled. In general, it is the idea of a system in which continuously novel and “interesting” organisms are able to evolve, such as the Earth's biosphere. It is often framed as a theoretical question, focused on what is missing from current digital evolution systems that keeps them from attaining the diversity and complexity seen in biological organisms.
The question relevant to this review is if symbiosis is necessary or sufficient (or neither) for a system to exhibit open-ended evolution. The ubiquity of symbiosis in the natural world is a strong argument for it being necessary. However, as discussed previously, the goal of digital evolution is not to simulate the natural world. A valuable component of an open-ended system is likely to be a continually changing environment (though perhaps neither necessary nor sufficient), and co-evolving symbionts can provide continuous change. Conversely, the emergence of symbiosis from free-living ancestors could be considered an indication of open-ended evolution.
We have detailed here only some of the many ways in which symbiosis can influence the evolutionary dynamics of the species involved. While there will always be exceptions, these dynamics make a strong argument that the possibility and emergence of symbiosis in a digital evolution system should be considered a hallmark of open-ended evolution. It is an open question if symbiosis could be sufficient for open-ended evolution to occur.
7.3. What Factors Select for the de novo Evolution of Symbiosis, Parasitism, and Mutualism?
Another set of questions that digital evolution is well-suited to address focuses on the evolution of symbiosis itself. As previously discussed, a symbiosis has several axes upon which it can vary:
1. The overall benefit or cost to the host, which determines if the symbiosis is parasitic, commensal, or mutualistic
2. The level of dependence of the symbiont and host on the symbiosis, which determines if the symbiosis is obligate or facultative for each partner
3. The physical position of the symbiont in relation to the host, which determines if the interaction is an endosymbiosis, ectosymbiosis, etc.
These categories are all independent spectrums, and systems can shift along them over both individual lifetimes and evolutionary time. A symbiosis can also be a precursor to an egalitarian major evolutionary transition where the symbiosis becomes so tightly linked that the partners become a single organism (Estrela et al., 2016). Here, we propose specific hypotheses and more general questions surrounding the evolution of symbiosis that could be addressed with present or future digital evolution systems.
Endosymbiosis, a relationship where an organism lives inside its symbiotic partner, is an interesting phenomenon with both benefits and costs (Chomicki et al., 2020; Puri et al., 2021). It offers a potentially sheltered environment inside a host (or a more hostile environment, if the host is defensive) and the possibility of vertical transmission to the host offspring. However, it brings the risks of overspecialization and the complications of coevolution, as discussed previously. Therefore, a general open question is when would endosymbiosis be favored to evolve, and how does the form of the symbiosis influence that evolution?
Once inside a host, an endosymbiont could evolve away from its initial relationship to be mutualistic, parasitic, or commensal, or it could retain the initial effect it had on its host. As discussed in section 6, previous results indicate vertical transmission is insufficient for de novo evolution of a mutualistic endosymbiosis, because the endosymbiont went extinct before mutualism evolved. Clearly, the endosymbiont species must have the capability to survive long enough for any evolutionary pressures to affect them and their hosts.
There are many possible paths that an endosymbiotic species could follow, such as from a facultative mutualist toward an obligate parasite, or an obligate parasite to a mutualist (the Co-opted Antagonist Hypothesis, Johnson et al., 2021). Their relative likelihoods and contributing factors could be investigated in a digital evolution system that supports de novo evolution of endosymbiosis. Further, transitions from endosymbiotic parasites to mutualists appear to be fairly common in biological systems and determining the underlying mechanisms for this transition would be valuable for the field (Drew et al., 2021). Similar questions can be asked regarding ectosymbiotic dynamics, especially those that enable a symbiont to simultaneously interact with multiple hosts.
Finally, previous results have shown that spatial structure can affect the evolution of symbiosis in complex ways (Vostinar and Ofria, 2019). However, these results are only for obligate endosymbionts. Determining how spatial structure influences the evolution of symbiosis in other cases will also be important.
7.4. Gaps in Current Systems
Early digital evolution systems (reviewed in section 5) were designed without a focus on enabling symbiosis. While some preliminary symbiotic interactions were observed, they were necessarily limited, because all organisms were required to occupy cells in a two dimensional grid. Additional functionality needed to be added to enable endosymbiosis, as was done by Zaman and colleagues in Avida (Zaman et al., 2011). Symbulation, created by Vostinar (Vostinar, 2021), was designed with symbiosis specifically in mind, but is a fairly recent addition to the software landscape and therefore missing many desirable features.
Ideally, improvements to existing systems, or the development of a new digital evolution system that supports symbiosis, will contain support for many forms of symbiotic interaction. To answer the questions discussed in the previous subsection, it will be necessary for such systems to support the evolution between different symbiotic dynamics. Specifically, we recommend that digital evolution systems for the study of symbiosis contain:
1. Support for evolution along the parasitism-mutualism spectrum
2. Support for evolution between free-living and endosymbiont strategies and between facultative and obligate strategies
3. Multi-level symbiosis, such that an organism can be a host as well as a symbiont to a super-host
4. Multi-infection, such that symbionts are able to interact ecologically within a host
5. Support for ectosymbiont strategies, especially allowing for symbionts that associate with multiple hosts simultaneously
6. Support for asexual and sexual reproduction to enable studying the role of co-evolving symbionts on the evolution and maintenance of sexual reproduction
7. Support for host predation to enable the emergence of parasites with complex lifecycles (Auld and Tinsley, 2015).
In addition, while improvements in underlying technology have enabled digital evolution systems to support fairly large populations and the evolution of hundreds of thousands of generations in long experiments, there is always room for further improvement. In particular, most digital evolution systems do not take advantage of the parallel processing capabilities of modern machines, though efforts are being made in that area (Moreno et al., 2021).
Finally, future digital evolution systems for the study of symbiosis should aim to improve access and ease of use. Many previous and current systems can only be used for evolutionary experiments when run on high-performance computing systems, which are not universally accessible. Improvements are being made in this regard, particularly with the emergence of digital evolution systems that can run in an internet browser, such as those supported by the Empirical platform1. Further, digital evolution systems usually rely on advanced programming languages such as C++, making it challenging for less-experienced programmers to make modifications. However, researchers could design the systems with this knowledge in mind, making efforts to improve the modularity and readability of the systems' codebases.
8. Conclusion
Our goal in this review is to present how the evolution of symbiosis has been, and can be, studied with digital evolution systems. We have demonstrated how digital evolution methods will continue to be valuable tools in determining the generalizable processes and patterns involved in symbiosis, and we have shown how digital evolution techniques complement both biological and mathematical approaches. A new generation of digital evolution systems built with symbiosis as a focus will be ideal to address the many open questions left in the field, and we hope to see more collaboration between the associated subfields in the future for the benefit of all.
Author Contributions
All authors wrote the manuscript and approved its final version.
Funding
This research was supported by the National Science Foundation (NSF) through the BEACON Center (DBI-0939454), a subaward to AV (MCB-1750125), a Graduate Research Fellowship to AL (DGE-1424871), and support to LZ (DEB-1813069). Support was provided by the Department of Defense (DoD) through a fellowship to KS from the National Defense Science & Engineering Graduate (NDSEG) Fellowship Program.
Conflict of Interest
The authors declare that the research was conducted in the absence of any commercial or financial relationships that could be construed as a potential conflict of interest.
Publisher's Note
All claims expressed in this article are solely those of the authors and do not necessarily represent those of their affiliated organizations, or those of the publisher, the editors and the reviewers. Any product that may be evaluated in this article, or claim that may be made by its manufacturer, is not guaranteed or endorsed by the publisher.
Acknowledgments
We would like to thank the pioneers of digital evolution for founding such an exciting field and methodology, and Dr. Charles Ofria for introducing each of us to it.
Footnotes
1. ^Software available online at: https://empirical.readthedocs.io/en/latest/.
References
Adami, C., and Brown, C. T. (1994). “Evolutionary learning in the 2D artificial life system,” in Artificial Life IV: Proceedings of the Fourth International Workshop on the Synthesis and Simulation of Living Systems, Vol. 4 (MIT Press), 377.
Adami, C., Ofria, C., and Collier, T. C. (2000). Evolution of biological complexity. Proc. Natl. Acad. Sci. U.S.A. 97, 4463–4468. doi: 10.1073/pnas.97.9.4463
Adami, C., Schossau, J., and Hintze, A. (2016). Evolutionary game theory using agent-based methods. Phys. Life Rev. 19, 1–26. doi: 10.1016/j.plrev.2016.08.015
Akçay, E. (2015). “Evolutionary models of mutualism,” in Mutualism, ed J. L. Bronstein (Oxford: Oxford University Press), 57–76. doi: 10.1093/acprof:oso/9780199675654.003.0004
Anstett, M. C., Hossaert-McKey, M., and Kjellberg, F. (1997). Figs and fig pollinators: evolutionary conflicts in a coevoled mutualism. Trends Ecol. Evol. 12, 94–99. doi: 10.1016/S0169-5347(96)10064-1
Auld, S. K., and Tinsley, M. (2015). The evolutionary ecology of complex lifecycle parasites: linking phenomena with mechanisms. Heredity 114, 125–132. doi: 10.1038/hdy.2014.84
Bennett, G. M., and Moran, N. A. (2015). Heritable symbiosis: the advantages and perils of an evolutionary rabbit hole. Proc. Natl. Acad. Sci. U.S.A. 112, 10169–10176. doi: 10.1073/pnas.1421388112
Bento, G., Routtu, J., Fields, P. D., Bourgeois, Y., Du Pasquier, L., and Ebert, D. (2017). The genetic basis of resistance and matching-allele interactions of a host-parasite system: The daphnia magna-pasteuria ramosa model. PLoS Genet. 13:e1006596. doi: 10.1371/journal.pgen.1006596
Benton, M. J. (2009). The red queen and the court jester: species diversity and the role of biotic and abiotic factors through time. Science 323, 728–732. doi: 10.1126/science.1157719
Bérénos, C., Wegner, K. M., and Schmid-Hempel, P. (2011). Antagonistic coevolution with parasites maintains host genetic diversity: an experimental test. Proc. R. Soc. B Biol. Sci. 278, 218–224. doi: 10.1098/rspb.2010.1211
Bergstrom, C. T., Bronstein, J. L., Bshary, R., Connor, R. C., Daly, M., Frank, S. A., et al. (2003). “Interspecific mutualism: puzzles and predictions,” in Genetic and Cultural Evolution of Cooperation, ed P. Hammerstein (Cambridge, MA: MIT Press), 241–256.
Brewster, J., and Conrad, M. (1998). “Evolve IV: a metabolically-based artificial ecosystem model,” in International Conference on Evolutionary Programming (Berlin; Heidelberg: Springer), 473–482. doi: 10.1007/BFb0040799
Brockhurst, M. A., Rainey, P. B., and Buckling, A. (2004). The effect of spatial heterogeneity and parasites on the evolution of host diversity. Proc. R. Soc. Lond. Ser. B Biol. Sci. 271, 107–111. doi: 10.1098/rspb.2003.2556
Bryson, D., Baer, B., Vostinar, A., Ofria, C., Barrick, J., Zaman, L., et al. (2021). devosoft/avida: 2021-07-03-v2.14.0 (2.14.0). Zenodo. doi: 10.5281/zenodo.5068026
Bshary, R., and Grutter, A. S. (2006). Image scoring and cooperation in a cleaner fish mutualism. Nature 441, 975–978. doi: 10.1038/nature04755
Burmeister, A. R., Lenski, R. E., and Meyer, J. R. (2016). Host coevolution alters the adaptive landscape of a virus. Proc. R. Soc. B Biol. Sci. 283:20161528. doi: 10.1098/rspb.2016.1528
Canino-Koning, R., Wiser, M. J., and Ofria, C. (2016). “The evolution of evolvability: changing environments promote rapid adaptation in digital organisms,” in Proceedings of the Artificial Life Conference 2016 (Cancun: MIT Press), 268–275. doi: 10.7551/978-0-262-33936-0-ch047
Canino-Koning, R., Wiser, M. J., and Ofria, C. (2019). Fluctuating environments select for short-term phenotypic variation leading to long-term exploration. PLoS Comput. Biol. 15:e1006445. doi: 10.1371/journal.pcbi.1006445
Chalkowski, K., Lepczyk, C. A., and Zohdy, S. (2018). Parasite ecology of invasive species: conceptual framework and new hypotheses. Trends Parasitol. 34, 655–663. doi: 10.1016/j.pt.2018.05.008
Chomicki, G., Kiers, E. T., and Renner, S. S. (2020). The evolution of mutualistic dependence. Annu. Rev. Ecol. Evol. Syst. 51, 409–432. doi: 10.1146/annurev-ecolsys-110218-024629
Colizzi, E. S., and Hogeweg, P. (2016). Parasites sustain and enhance RNA-like replicators through spatial self-organisation. PLoS Comput. Biol. 12:e1004902. doi: 10.1371/journal.pcbi.1004902
Combes, C. (2001). Parasitism: The Ecology and Evolution of Intimate Interactions. Chicago, IL: University of Chicago Press.
Conrad, M., and Pattee, H. (1970). Evolution experiments with an artificial ecosystem. J. Theoret. Biol. 28, 393–409. doi: 10.1016/0022-5193(70)90077-9
Conrad, M., and Strizich, M. (1985). Evolve II: a computer model of an evolving ecosystem. Biosystems 17, 245–258. doi: 10.1016/0303-2647(85)90079-6
Cooper, T. F., and Ofria, C. (2002). “Evolution of stable ecosystems in populations of digital organisms,” in Proceedings of the Eighth International Conference on Artificial Life, ICAL 2003 (Cambridge, MA: MIT Press), 227–232.
Crombach, A., and Hogeweg, P. (2009). Evolution of resource cycling in ecosystems and individuals. BMC Evol. Biol. 9:122. doi: 10.1186/1471-2148-9-122
Daida, J. M., Grasso, C. S., Stanhope, S. A., and Ross, S. J. (1996). “Symbionticism and complex adaptive systems I: Implications of having symbiosis occur in nature,” in Evolutionary Programming V: Proceedings of the Fifth Annual Conference on Evolutionary Programming (Cambridge, MA: The MIT Press), 177–186.
Dawkins, R., and Krebs, J. R. (1979). Arms races between and within species. Proc. R. Soc. Lond. Ser. B. Biol. Sci. 205, 489–511. doi: 10.1098/rspb.1979.0081
Dean, J. M., Mescher, M. C., and De Moraes, C. M. (2009). Plant-rhizobia mutualism influences aphid abundance on soybean. Plant Soil 323, 187–196. doi: 10.1007/s11104-009-9924-1
DeAngelis, D. L., and Mooij, W. M. (2005). Individual-based modeling of ecological and evolutionary processes. Annu. Rev. Ecol. Evol. Syst. 36, 147–168. doi: 10.1146/annurev.ecolsys.36.102003.152644
Dewdney, A. K. (1984). Computer recreations. Sci. Am. 250, 14–23. doi: 10.1038/scientificamerican0584-14
Diggle, S. P., Griffin, A. S., Campbell, G. S., and West, S. A. (2007). Cooperation and conflict in quorum-sensing bacterial populations. Nature 450, 411–414. doi: 10.1038/nature06279
Dolson, E. L., Perez, S. G., Olson, R. S., and Ofria, C. (2017). “Spatial resource heterogeneity creates local hotspots of evolutionary potential,” in Proceedings of the ECAL 2017, the Fourteenth European Conference on Artificial Life. ECAL 2017, the Fourteenth European Conference on Artificial Life (Lyon: ASME), 122–129.
Drew, G. C., Stevens, E. J., and King, K. C. (2021). Microbial evolution and transitions along the parasite-mutualist continuum. Nat. Rev. Microbiol. 19, 623–638. doi: 10.1038/s41579-021-00550-7
Ebert, D., and Hamilton, W. D. (1996). Sex against virulence: the coevolution of parasitic diseases. Trends Ecol. Evol. 11, 79–82. doi: 10.1016/0169-5347(96)81047-0
Elena, S. F., and Lenski, R. E. (2003). Evolution experiments with microorganisms: the dynamics and genetic bases of adaptation. Nat. Rev. Genet. 4, 457–469. doi: 10.1038/nrg1088
Estrela, S., Kerr, B., and Morris, J. J. (2016). Transitions in individuality through symbiosis. Curr. Opin. Microbiol. 31, 191–198. doi: 10.1016/j.mib.2016.04.007
Fortuna, M. A., Barbour, M. A., Zaman, L., Hall, A. R., Buckling, A., and Bascompte, J. (2019). Coevolutionary dynamics shape the structure of bacteria-phage infection networks. Evolution 73, 1001–1011. doi: 10.1111/evo.13731
Fortuna, M. A., Zaman, L., Wagner, A., and Bascompte, J. (2017). Non-adaptive origins of evolutionary innovations increase network complexity in interacting digital organisms. Philos. Trans. R. Soc. B Biol. Sci. 372:20160431. doi: 10.1098/rstb.2016.0431
Frenoy, A., Taddei, F., and Misevic, D. (2013). Genetic architecture promotes the evolution and maintenance of cooperation. PLoS Comput. Biol. 9:e1003339. doi: 10.1371/journal.pcbi.1003339
Furubayashi, T., Ueda, K., Bansho, Y., Motooka, D., Nakamura, S., Mizuuchi, R., et al. (2020). Emergence and diversification of a host-parasite RNA ecosystem through darwinian evolution. eLife 9:e56038. doi: 10.7554/eLife.56038
Goldsby, H. J., Dornhaus, A., Kerr, B., and Ofria, C. (2012). Task-switching costs promote the evolution of division of labor and shifts in individuality. Proc. Natl. Acad. Sci. U.S.A. 109, 13686–13691. doi: 10.1073/pnas.1202233109
Goldsby, H. J., Knoester, D. B., Ofria, C., and Kerr, B. (2014). The evolutionary origin of somatic cells under the dirty work hypothesis. PLoS Biol. 12:e1001858. doi: 10.1371/journal.pbio.1001858
Goldsmith, T. H. (1990). Optimization, constraint, and history in the evolution of eyes. Q. Rev. Biol. 65, 281–322. doi: 10.1086/416840
Griffin, A. S., and West, S. A. (2002). Kin selection: fact and fiction. Trends Ecol. Evol. 17, 15–21. doi: 10.1016/S0169-5347(01)02355-2
Gupta, A., Zaman, L., Strobel, H. M., Gallie, J., Burmeister, A. R., Kerr, B., et al. (2021). Host-parasite coevolution promotes innovation through deformations in fitness landscapes. bioRxiv. doi: 10.1101/2021.06.25.449783
Haraguchi, Y., and Sasaki, A. (1996). Host-parasite arms race in mutation modifications: indefinite escalation despite a heavy load? J. Theoret. Biol. 183, 121–137. doi: 10.1006/jtbi.1996.9999
Hartfield, M., and Keightley, P. D. (2012). Current hypotheses for the evolution of sex and recombination. Integr. Zool. 7, 192–209. doi: 10.1111/j.1749-4877.2012.00284.x
Hazen, R. M., Griffin, P. L., Carothers, J. M., and Szostak, J. W. (2007). Functional information and the emergence of biocomplexity. Proc. Natl. Acad. Sci. U.S.A. 104(Suppl 1), 8574–8581. doi: 10.1073/pnas.0701744104
Heitman, J. (2006). Sexual reproduction and the evolution of microbial pathogens. Curr. Biol. 16, R711–R725. doi: 10.1016/j.cub.2006.07.064
Hellweger, F. L., Clegg, R. J., Clark, J. R., Plugge, C. M., and Kreft, J.-U. (2016). Advancing microbial sciences by individual-based modelling. Nat. Rev. Microbiol. 14, 461–471. doi: 10.1038/nrmicro.2016.62
Hickinbotham, S. J., Stepney, S., and Hogeweg, P. (2021). Nothing in evolution makes sense except in the light of parasitism: evolution of complex replication strategies. R. Soc. Open Sci. 8:210441. doi: 10.1098/rsos.210441
Hindré, T., Knibbe, C., Beslon, G., and Schneider, D. (2012). New insights into bacterial adaptation through in vivo and in silico experimental evolution. Nat. Rev. Microbiol. 10, 352–365. doi: 10.1038/nrmicro2750
Hoang, K. L., Gerardo, N. M., and Morran, L. T. (2021). Association with a novel protective microbe facilitates host adaptation to a stressful environment. Evol. Lett. 5, 118–129. doi: 10.1002/evl3.223
Hughes, T. P., Kerry, J. T., Baird, A. H., Connolly, S. R., Dietzel, A., Eakin, C. M., et al. (2018). Global warming transforms coral reef assemblages. Nature 556, 492–496. doi: 10.1038/s41586-018-0041-2
Husnik, F., and Keeling, P. J. (2019). The fate of obligate endosymbionts: reduction, integration, or extinction. Curr. Opin. Genet. Dev. 58, 1–8. doi: 10.1016/j.gde.2019.07.014
Johnson, C. A., Smith, G. P., Yule, K., Davidowitz, G., Bronstein, J. L., and Ferriére, R. (2021). Coevolutionary transitions from antagonism to mutualism explained by the co-opted antagonist hypothesis. Nat. Commun. 12, 1–11. doi: 10.1038/s41467-021-23177-x
Jones, J. E., Le Sage, V., and Lakdawala, S. S. (2020). Viral and host heterogeneity and their effects on the viral life cycle. Nat. Rev. Microbiol. 19, 272–282. doi: 10.1038/s41579-020-00449-9
Kołodziej-Sobocińska, M., Brzeziński, M., Niemczynowicz, A., and Zalewski, A. (2018). High parasite infection level in non-native invasive species: it is just a matter of time. Ecography 41, 1283–1294. doi: 10.1111/ecog.03362
Krause, J., Ruxton, G. D., Ruxton, G., and Ruxton, I. G. (2002). Living in Groups. Oxford: Oxford University Press.
Kutter, E., and Sulakvelidze, A. (2004). Bacteriophages: Biology and Applications. Boca Raton, FL: CRC Press. doi: 10.1201/9780203491751
Laanto, E., Hoikkala, V., Ravantti, J., and Sundberg, L.-R. (2017). Long-term genomic coevolution of host-parasite interaction in the natural environment. Nat. Commun. 8, 1–8. doi: 10.1038/s41467-017-00158-7
Lafferty, K. D., and Kuris, A. M. (2002). Trophic strategies, animal diversity and body size. Trends Ecol. Evol. 17, 507–513. doi: 10.1016/S0169-5347(02)02615-0
Lagisz, M., and O'Dea, R. E. (2021). SORTEE. Available online at: https://www.sortee.org/ (accessed October 12, 2021).
Lareen, A., Burton, F., and Schäfer, P. (2016). Plant root-microbe communication in shaping root microbiomes. Plant Mol. Biol. 90, 575–587. doi: 10.1007/s11103-015-0417-8
Lehman, J., Clune, J., Misevic, D., Adami, C., Altenberg, L., Beaulieu, J., et al. (2020). The surprising creativity of digital evolution: a collection of anecdotes from the evolutionary computation and artificial life research communities. Artif. Life 26, 274–306. doi: 10.1162/artl_a_00319
Lehtonen, J., Jennions, M. D., and Kokko, H. (2012). The many costs of sex. Trends Ecol. Evol. 27, 172–178. doi: 10.1016/j.tree.2011.09.016
Lenski, R. E., Ofria, C., Pennock, R. T., and Adami, C. (2003). The evolutionary origin of complex features. Nature 423, 139–144. doi: 10.1038/nature01568
Liard, V., Parsons, D. P., Rouzaud-Cornabas, J., and Beslon, G. (2020). The complexity ratchet: stronger than selection, stronger than evolvability, weaker than robustness. Artif. Life 26, 38–57. doi: 10.1162/artl_a_00312
Lombardo, M. P. (2008). Access to mutualistic endosymbiotic microbes: an underappreciated benefit of group living. Behav. Ecol. Sociobiol. 62, 479–497. doi: 10.1007/s00265-007-0428-9
Longdon, B., Brockhurst, M. A., Russell, C. A., Welch, J. J., and Jiggins, F. M. (2014). The evolution and genetics of virus host shifts. PLoS Pathog. 10:e1004395. doi: 10.1371/journal.ppat.1004395
Luo, T.-t., Heier, L., Khan, Z. A., Hasan, F., Reitan, T., Yasseen, A. S., et al. (2019). Examining community stability in the face of mass extinction in communities of digital organisms. Artif. Life 24, 250–276. doi: 10.1162/artl_a_00272
Lynch, M. (2007). The frailty of adaptive hypotheses for the origins of organismal complexity. Proc. Natl. Acad. Sci. U.S.A. 104(Suppl 1), 8597–8604. doi: 10.1073/pnas.0702207104
Lynch, Z. R., Penley, M. J., and Morran, L. T. (2018). Turnover in local parasite populations temporarily favors host outcrossing over self-fertilization during experimental evolution. Ecol. Evol. 8, 6652–6662. doi: 10.1002/ece3.4150
Mas, A., Jamshidi, S., Lagadeuc, Y., Eveillard, D., and Vandenkoornhuyse, P. (2016). Beyond the black queen hypothesis. ISME J. 10, 2085–2091. doi: 10.1038/ismej.2016.22
Masri, L., Branca, A., Sheppard, A. E., Papkou, A., Laehnemann, D., Guenther, P. S., et al. (2015). Host-pathogen coevolution: the selective advantage of bacillus thuringiensis virulence and its cry toxin genes. PLoS Biol. 13:e1002169. doi: 10.1371/journal.pbio.1002169
McCutcheon, J. P., and Moran, N. A. (2012). Extreme genome reduction in symbiotic bacteria. Nat. Rev. Microbiol. 10, 13–26. doi: 10.1038/nrmicro2670
Misevic, D., Ofria, C., and Lenski, R. E. (2010). Experiments with digital organisms on the origin and maintenance of sex in changing environments. J. Heredity 101(Suppl_1), S46–S54. doi: 10.1093/jhered/esq017
Momeni, B., Chen, C.-C., Hillesland, K. L., Waite, A., and Shou, W. (2011). Using artificial systems to explore the ecology and evolution of symbioses. Cell. Mol. Life Sci. 68, 1353–1368. doi: 10.1007/s00018-011-0649-y
Moran, N. A. (2007). Symbiosis as an adaptive process and source of phenotypic complexity. Proc. Natl. Acad. Sci. U.S.A. 104(Suppl 1), 8627–8633. doi: 10.1073/pnas.0611659104
Moreno, M. A., and Ofria, C. (2019). Toward open-ended fraternal transitions in individuality. Artif. Life 25, 117–133. doi: 10.1162/artl_a_00284
Moreno, M. A., Papa, S. R., and Ofria, C. (2021). Conduit: A c++ library for best-effort high performance computing. arXiv [Preprint]. arXiv:2105.10486. doi: 10.1145/3449726.3463205
Morran, L. T., Schmidt, O. G., Gelarden, I. A., Parrish, R. C., and Lively, C. M. (2011). Running with the Red Queen: host-parasite coevolution selects for biparental sex. Science 333, 216–218. doi: 10.1126/science.1206360
Morris, B. E., Henneberger, R., Huber, H., and Moissl-Eichinger, C. (2013). Microbial syntrophy: interaction for the common good. FEMS Microbiol. Rev. 37, 384–406. doi: 10.1111/1574-6976.12019
Morris, J. J., Lenski, R. E., and Zinser, E. R. (2012). The black queen hypothesis: evolution of dependencies through adaptive gene loss. mBio 3:e00036-12. doi: 10.1128/mBio.00036-12
O'Callaghan, J., and Conrad, M. (1992). Symbiotic interactions in the evolve III ecosystem model. Biosystems 26, 199–209. doi: 10.1016/0303-2647(92)90025-T
Ofria, C., Bryson, D. M., and Wilke, C. O. (2009). “Avida: a software platform for research in computational evolutionary biology,” in Artificial Life Models in Software, eds M. Komosinski and A. Adamatzky (London: Springer), 3–35. doi: 10.1007/978-1-84882-285-6_1
Ofria, C., and Wilke, C. O. (2004). Avida: a software platform for research in computational evolutionary biology. Artif. Life 10, 191–229. doi: 10.1162/106454604773563612
Oulhen, N., Schulz, B. J., and Carrier, T. J. (2016). English translation of Heinrich Anton de Bary's 1878 speech, ‘Die Erscheinung der Symbiose' (‘De la symbiose'). Symbiosis 69, 131–139. doi: 10.1007/s13199-016-0409-8
Pachepsky, E., Taylor, T., and Jones, S. (2002). Mutualism promotes diversity and stability in a simple artificial ecosystem. Artif. Life 8, 5–24. doi: 10.1162/106454602753694747
Paracer, S., and Ahmadjian, V. (2000). Symbiosis: An Introduction to Biological Associations. Oxford: Oxford University Press.
Pennock, R. T. (2007). Models, simulations, instantiations, and evidence: the case of digital evolution. J. Exp. Theoret. Artif. Intell. 19, 29–42. doi: 10.1080/09528130601116113
Puri, K. M., Butardo, V., and Sumer, H. (2021). Evaluation of natural endosymbiosis for progress towards artificial endosymbiosis. Symbiosis 84, 1–17. doi: 10.1007/s13199-020-00741-5
Queller, D. C., and Strassmann, J. E. (2018). Evolutionary conflict. Annu. Rev. Ecol. Evol. Syst. 49, 73–93. doi: 10.1146/annurev-ecolsys-110617-062527
Raffel, T. R., Martin, L. B., and Rohr, J. R. (2008). Parasites as predators: unifying natural enemy ecology. Trends Ecol. Evol. 23, 610–618. doi: 10.1016/j.tree.2008.06.015
Railsback, S. F., and Grimm, V. (2019). Agent-Based and Individual-Based Modeling: A Practical Introduction. Princeton, NJ: Princeton University Press.
Rasmussen, S., Feldberg, R., Hindsholm, M., and Knudsen, C. (1989). “Core evolution: emergence of cooperative structures in a computational chemistry,” in Conference on Emergent Computation (Los Alamos, NM).
Rasmussen, S., Knudsen, C., Feldberg, R., and Hindsholm, M. (1990). The coreworld: Emergence and evolution of cooperative structures in a computational chemistry. Phys. D Nonlinear Phenomena 42, 111–134. doi: 10.1016/0167-2789(90)90070-6
Ray, T. S. (1991). “An approach to the synthesis of life,” in Artificial Life II, eds C. G. Langton, C. Taylor, J. D. Farmer, and S. Rasmussen (Redwood City, CA:Addison-Wesley), 371–408.
Ray, T. S. (1992). Evolution, Ecology and Optimization of Digital Organisms. Technical report. Santa Fe Institute, Santa Fe, NM.
Ray, T. S. (1993). An evolutionary approach to synthetic biology: Zen and the art of creating life. Artif. Life 1, 179–209. doi: 10.1162/artl.1993.1.1_2.179
Rizki, M. M., and Conrad, M. (1985). Evolve III: a discrete events model of an evolutionary ecosystem. Biosystems 18, 121–133. doi: 10.1016/0303-2647(85)90065-6
Rocabert, C., Knibbe, C., and Beslon, G. (2021). Evo2sim. Codebase. Available online at: https://github.com/charlesrocabert/Evo2Sim
Rocabert, C., Knibbe, C., Consuegra, J., Schneider, D., and Beslon, G. (2017). Beware batch culture: Seasonality and niche construction predicted to favor bacterial adaptive diversification. PLoS Comput. Biol. 13:e1005459. doi: 10.1371/journal.pcbi.1005459
Rozen, D. E., and Lenski, R. E. (2000). Long-term experimental evolution in Escherichia coli. VIII. dynamics of a balanced polymorphism. Am. Nat. 155, 24–35. doi: 10.1086/303299
Ryan, F. (2020). “Viral symbiosis in the origins and evolution of life with a particular focus on the placental mammals,” in Symbiosis: Cellular, Molecular, Medical and Evolutionary Aspects. Results and Problems in Cell Differentiation, Vol. 69, ed M. Kloc (Cham: Springer), 3–24. doi: 10.1007/978-3-030-51849-3_1
Sachs, J. L., Skophammer, R. G., and Regus, J. U. (2011). Evolutionary transitions in bacterial symbiosis. Proc. Natl. Acad. Sci. U.S.A. 108(Suppl 2), 10800–10807. doi: 10.1073/pnas.1100304108
Sarkar, A., Harty, S., Johnson, K. V.-A., Moeller, A. H., Archie, E. A., Schell, L. D., et al. (2020). Microbial transmission in animal social networks and the social microbiome. Nat. Ecol. Evol. 4, 1020–1035. doi: 10.1038/s41559-020-1220-8
Seth, E. C., and Taga, M. E. (2014). Nutrient cross-feeding in the microbial world. Front. Microbiol. 5:350. doi: 10.3389/fmicb.2014.00350
Shanahan, T. (2004). The Evolution of Darwinism: Selection, Adaptation and Progress in Evolutionary Biology. Cambridge: Cambridge University Press.
Shapiro, J. W., and Turner, P. E. (2014). The impact of transmission mode on the evolution of benefits provided by microbial symbionts. Ecol. Evol. 4, 3350–3361. doi: 10.1002/ece3.1166
Simard, S. W., and Durall, D. M. (2004). Mycorrhizal networks: a review of their extent, function, and importance. Can. J. Bot. 82, 1140–1165. doi: 10.1139/b04-116
Simpson, G. G., and Simpson, L. (1949). The Meaning of Evolution: A Study of the History of Life and of Its Significance for Man, Vol. 25. New Haven, CT: Yale University Press. doi: 10.5962/bhl.title.40704
Spafford, E. H. (1994). Computer viruses as artificial life. Artif. Life 1, 249–265. doi: 10.1162/artl.1994.1.3.249
StringMol (2021). Stringmol. Codebase. Available online at: https://github.com/franticspider/stringmol
Strona, G. (2015). Past, present and future of host-parasite co-extinctions. Int. J. Parasitol. Parasites Wildlife 4, 431–441. doi: 10.1016/j.ijppaw.2015.08.007
Stroud, J. T., and Losos, J. B. (2016). Ecological opportunity and adaptive radiation. Annu. Rev. Ecol. Evol. Syst. 47, 507–532. doi: 10.1146/annurev-ecolsys-121415-032254
Sylla, S., Brévault, T., Monticelli, L. S., Diarra, K., and Desneux, N. (2019). Geographic variation of host preference by the invasive tomato leaf miner tuta absoluta: implications for host range expansion. J. Pest Sci. 92, 1387–1396. doi: 10.1007/s10340-019-01094-9
Szostak, J. W., Bartel, D. P., and Luisi, P. L. (2001). Synthesizing life. Nature 409, 387–390. doi: 10.1038/35053176
Taylor, C. E., Jefferson, D. R., Turner, S. R., and Goldman, S. R. (1987). “RAM: artificial life for the exploration of complex biological systems,” in Proceedings of the Interdisciplinary Workshop on the Synthesis and Simulation of Living Systems (ALIFE '87), ed C. Langton (Los Alamos, NM: Addison-Wesley), 275–296.
Taylor, C. E., Muscatine, L., and Jefferson, D. R. (1989). Maintenance and breakdown of the hydra-chlorella symbiosis: a computer model. Proc. R. Soc. Lond. B Biol. Sci. 238, 277–289. doi: 10.1098/rspb.1989.0081
Taylor, T., Bedau, M., Channon, A., Ackley, D., Banzhaf, W., Beslon, G., et al. (2016). Open-ended evolution: perspectives from the OEE workshop in york. Artif. Life 22, 408–423. doi: 10.1162/ARTL_a_00210
Thompson, J. N. (2005a). Coevolution: the geographic mosaic of coevolutionary arms races. Curr. Biol. 15, R992–R994. doi: 10.1016/j.cub.2005.11.046
Thompson, J. N. (2005b). The Geographic Mosaic of Coevolution. Chicago, IL: University of Chicago Press. doi: 10.7208/chicago/9780226118697.001.0001
Tooby, J. (1982). Pathogens, polymorphism, and the evolution of sex. J. Theoret. Biol. 97, 557–576. doi: 10.1016/0022-5193(82)90358-7
Vermeij, G. J. (1993). Evolution and Escalation: An Ecological History of Life. Princeton, NJ: Princeton University Press.
Vermeij, G. J. (1994). The evolutionary interaction among species: selection, escalation, and coevolution. Annu. Rev. Ecol. Syst. 25, 219–236. doi: 10.1146/annurev.es.25.110194.001251
Vidal, M. C., and Segraves, K. A. (2021). Coevolved mutualists experience fluctuating costs and benefits over time. Evolution 75, 219–230. doi: 10.1111/evo.14155
Vostinar, A. E., Goldsby, H. J., and Ofria, C. (2019). Suicidal selection: programmed cell death can evolve in unicellular organisms due solely to kin selection. Ecol. Evol. 9, 9129–9136. doi: 10.1002/ece3.5460
Vostinar, A. E., and Ofria, C. (2019). Spatial structure can decrease symbiotic cooperation. Artif. Life 24, 229–249. doi: 10.1162/artl_a_00273
Watson, R. A., Reil, T., Pollack, J. B., Bedau, M., McCaskill, J., Packard, N., (eds.). (2000). “Mutualism, parasitism, and evolutionary adaptation,” in Proceedings of Artificial Life VII, 170–178.
Wilke, C. O., and Adami, C. (2002). The biology of digital organisms. Trends Ecol. Evol. 17, 528–532. doi: 10.1016/S0169-5347(02)02612-5
Williams, H. T. (2013). Phage-induced diversification improves host evolvability. BMC Evol. Biol. 13:17. doi: 10.1186/1471-2148-13-17
Wolfe, L. M. (2002). Why alien invaders succeed: support for the escape-from-enemy hypothesis. Am. Nat. 160, 705–711. doi: 10.1086/343872
Yadav, A. K., Rajak, K. K., Bhatt, M., Kumar, A., Chakravarti, S., Sankar, M., et al. (2019). Comparative sequence analysis of morbillivirus receptors and its implication in host range expansion. Can. J. Microbiol. 65, 783–794. doi: 10.1139/cjm-2019-0008
Yamamura, N., Higashi, M., Behera, N., and Yuichiro Wakano, J. (2004). Evolution of mutualism through spatial effects. J. Theoret. Biol. 226, 421–428. doi: 10.1016/j.jtbi.2003.09.016
Zaman, L. (2018). “Investigating open-ended coevolution in digital organisms,” in Artificial Life Conference Proceedings (Cambridge, MA: MIT Press), 258–259. doi: 10.1162/isal_a_00052
Zaman, L., Devangam, S., and Ofria, C. (2011). “Rapid host-parasite coevolution drives the production and maintenance of diversity in digital organisms,” in Proceedings of the 13th Annual Conference on Genetic and Evolutionary Computation - GECCO '11 (Dublin: ACM Press), 219. doi: 10.1145/2001576.2001607
Zaman, L., Meyer, J. R., Devangam, S., Bryson, D. M., Lenski, R. E., and Ofria, C. (2014). Coevolution drives the emergence of complex traits and promotes evolvability. PLoS Biol. 12:e1002023. doi: 10.1371/journal.pbio.1002023
Keywords: symbiosis, digital evolution, parasitism, mutualism, artificial life, experimental evolution, coevolution
Citation: Vostinar AE, Skocelas KG, Lalejini A and Zaman L (2021) Symbiosis in Digital Evolution: Past, Present, and Future. Front. Ecol. Evol. 9:739047. doi: 10.3389/fevo.2021.739047
Received: 09 June 2021; Accepted: 04 October 2021;
Published: 05 November 2021.
Edited by:
Miguel A. Fortuna, Estación Biológica de Doñana (EBD), SpainReviewed by:
Guillaume Beslon, Inria Grenoble - Rhône-Alpes Research Centre, FranceAdam Clark, University of Graz, Austria
Copyright © 2021 Vostinar, Skocelas, Lalejini and Zaman. This is an open-access article distributed under the terms of the Creative Commons Attribution License (CC BY). The use, distribution or reproduction in other forums is permitted, provided the original author(s) and the copyright owner(s) are credited and that the original publication in this journal is cited, in accordance with accepted academic practice. No use, distribution or reproduction is permitted which does not comply with these terms.
*Correspondence: Anya E. Vostinar, YW55YS52b3N0aW5hckBnbWFpbC5jb20=